[SPARK-35813][SQL][DOCS] Add new adaptive config into sql-performance-tuning docs
### What changes were proposed in this pull request? Add new configs in sql-performance-tuning docs. * spark.sql.adaptive.coalescePartitions.parallelismFirst * spark.sql.adaptive.coalescePartitions.minPartitionSize * spark.sql.adaptive.autoBroadcastJoinThreshold * spark.sql.adaptive.maxShuffledHashJoinLocalMapThreshold ### Why are the changes needed? Help user to find them. ### Does this PR introduce _any_ user-facing change? yes, docs changed. ### How was this patch tested? 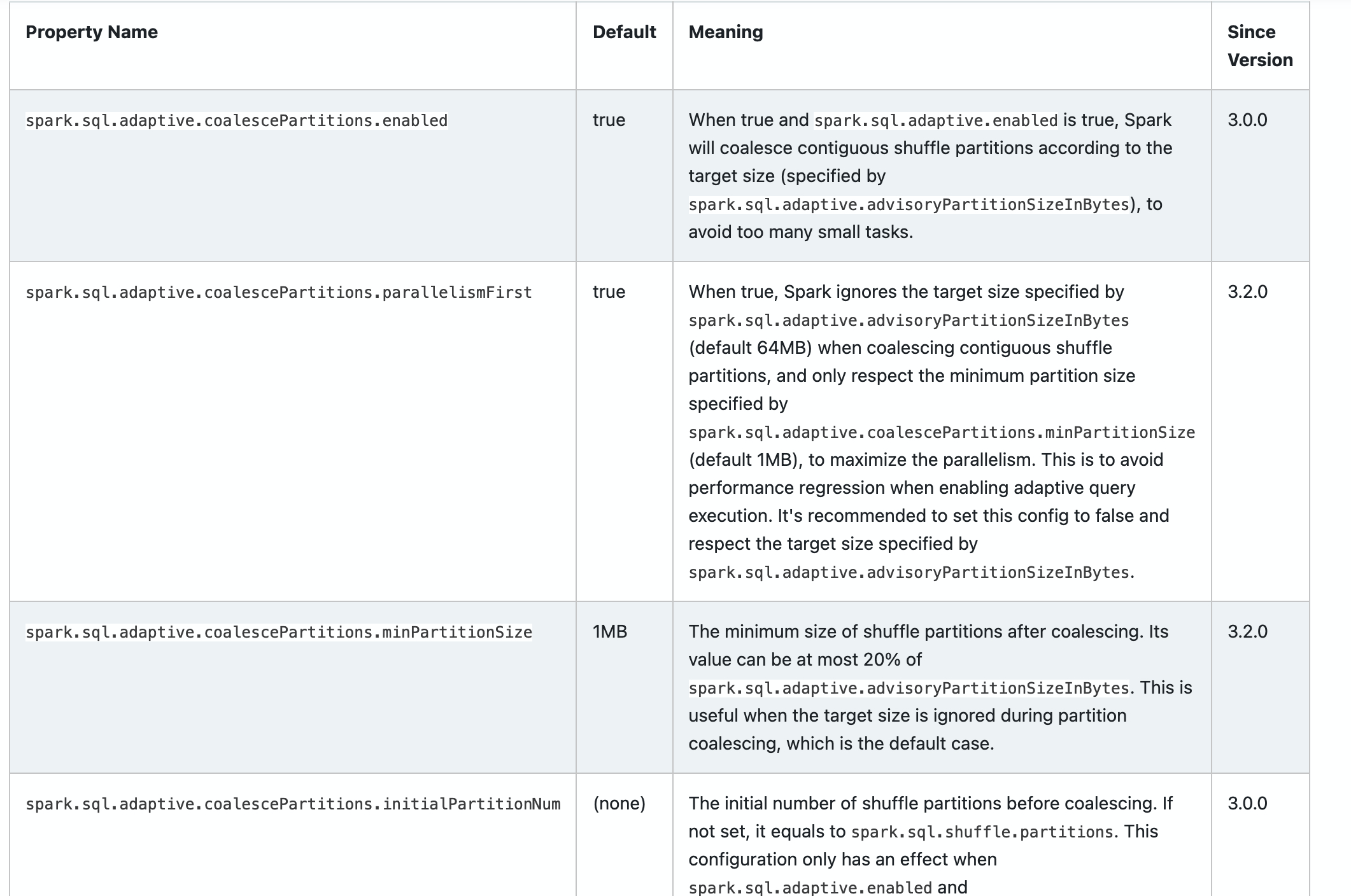 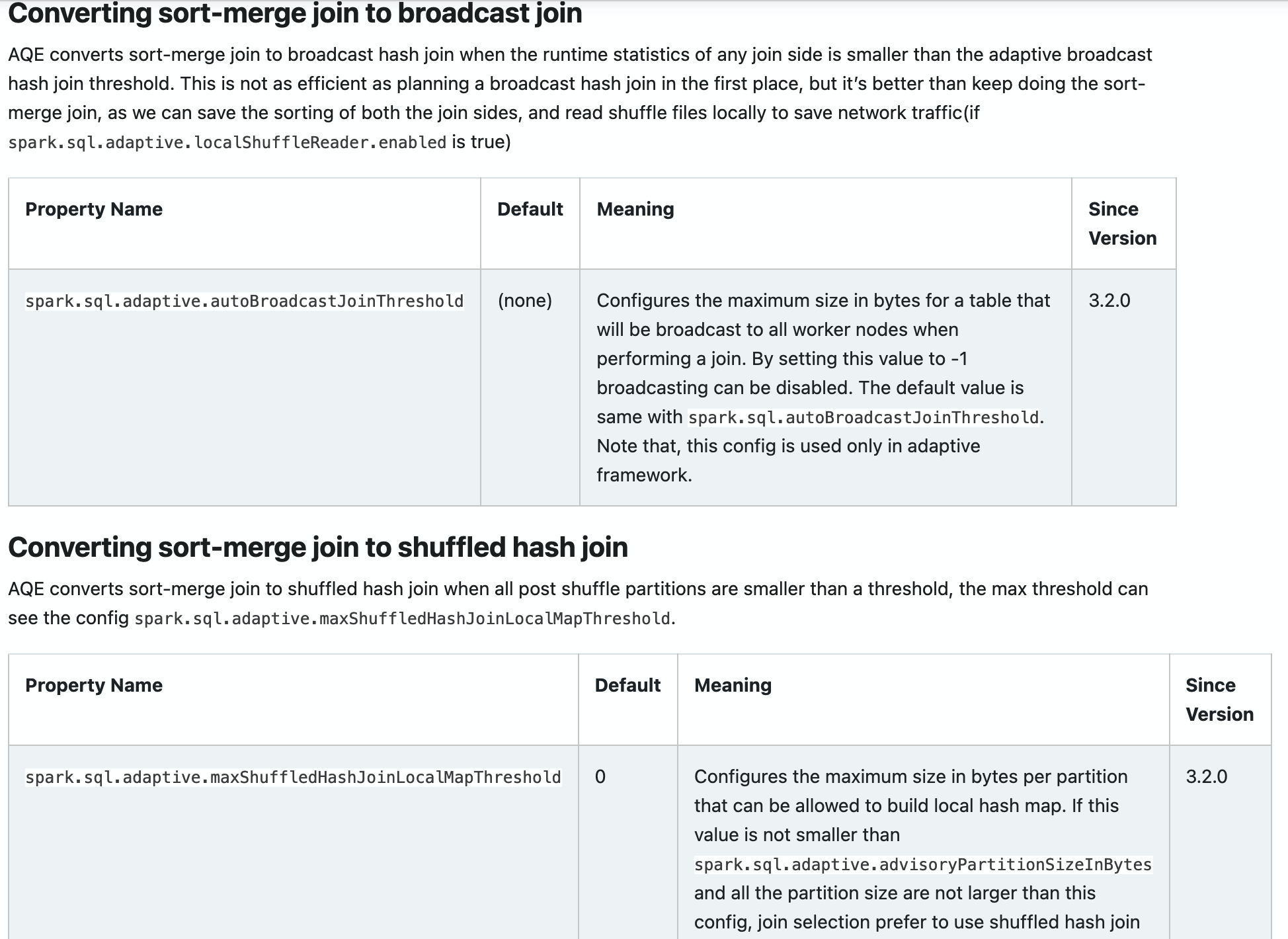 Closes #32960 from ulysses-you/SPARK-35813. Authored-by: ulysses-you <ulyssesyou18@gmail.com> Signed-off-by: Wenchen Fan <wenchen@databricks.com>
This commit is contained in:
parent
95e6c6e3e9
commit
0e9786c712
|
@ -249,12 +249,20 @@ This feature coalesces the post shuffle partitions based on the map output stati
|
|||
<td>3.0.0</td>
|
||||
</tr>
|
||||
<tr>
|
||||
<td><code>spark.sql.adaptive.coalescePartitions.minPartitionNum</code></td>
|
||||
<td>Default Parallelism</td>
|
||||
<td><code>spark.sql.adaptive.coalescePartitions.parallelismFirst</code></td>
|
||||
<td>true</td>
|
||||
<td>
|
||||
The minimum number of shuffle partitions after coalescing. If not set, the default value is the default parallelism of the Spark cluster. This configuration only has an effect when <code>spark.sql.adaptive.enabled</code> and <code>spark.sql.adaptive.coalescePartitions.enabled</code> are both enabled.
|
||||
When true, Spark ignores the target size specified by <code>spark.sql.adaptive.advisoryPartitionSizeInBytes</code> (default 64MB) when coalescing contiguous shuffle partitions, and only respect the minimum partition size specified by <code>spark.sql.adaptive.coalescePartitions.minPartitionSize</code> (default 1MB), to maximize the parallelism. This is to avoid performance regression when enabling adaptive query execution. It's recommended to set this config to false and respect the target size specified by <code>spark.sql.adaptive.advisoryPartitionSizeInBytes</code>.
|
||||
</td>
|
||||
<td>3.0.0</td>
|
||||
<td>3.2.0</td>
|
||||
</tr>
|
||||
<tr>
|
||||
<td><code>spark.sql.adaptive.coalescePartitions.minPartitionSize</code></td>
|
||||
<td>1MB</td>
|
||||
<td>
|
||||
The minimum size of shuffle partitions after coalescing. Its value can be at most 20% of <code>spark.sql.adaptive.advisoryPartitionSizeInBytes</code>. This is useful when the target size is ignored during partition coalescing, which is the default case.
|
||||
</td>
|
||||
<td>3.2.0</td>
|
||||
</tr>
|
||||
<tr>
|
||||
<td><code>spark.sql.adaptive.coalescePartitions.initialPartitionNum</code></td>
|
||||
|
@ -275,7 +283,32 @@ This feature coalesces the post shuffle partitions based on the map output stati
|
|||
</table>
|
||||
|
||||
### Converting sort-merge join to broadcast join
|
||||
AQE converts sort-merge join to broadcast hash join when the runtime statistics of any join side is smaller than the broadcast hash join threshold. This is not as efficient as planning a broadcast hash join in the first place, but it's better than keep doing the sort-merge join, as we can save the sorting of both the join sides, and read shuffle files locally to save network traffic(if `spark.sql.adaptive.localShuffleReader.enabled` is true)
|
||||
AQE converts sort-merge join to broadcast hash join when the runtime statistics of any join side is smaller than the adaptive broadcast hash join threshold. This is not as efficient as planning a broadcast hash join in the first place, but it's better than keep doing the sort-merge join, as we can save the sorting of both the join sides, and read shuffle files locally to save network traffic(if `spark.sql.adaptive.localShuffleReader.enabled` is true)
|
||||
<table class="table">
|
||||
<tr><th>Property Name</th><th>Default</th><th>Meaning</th><th>Since Version</th></tr>
|
||||
<tr>
|
||||
<td><code>spark.sql.adaptive.autoBroadcastJoinThreshold</code></td>
|
||||
<td>(none)</td>
|
||||
<td>
|
||||
Configures the maximum size in bytes for a table that will be broadcast to all worker nodes when performing a join. By setting this value to -1 broadcasting can be disabled. The default value is same with <code>spark.sql.autoBroadcastJoinThreshold</code>. Note that, this config is used only in adaptive framework.
|
||||
</td>
|
||||
<td>3.2.0</td>
|
||||
</tr>
|
||||
</table>
|
||||
|
||||
### Converting sort-merge join to shuffled hash join
|
||||
AQE converts sort-merge join to shuffled hash join when all post shuffle partitions are smaller than a threshold, the max threshold can see the config `spark.sql.adaptive.maxShuffledHashJoinLocalMapThreshold`.
|
||||
<table class="table">
|
||||
<tr><th>Property Name</th><th>Default</th><th>Meaning</th><th>Since Version</th></tr>
|
||||
<tr>
|
||||
<td><code>spark.sql.adaptive.maxShuffledHashJoinLocalMapThreshold</code></td>
|
||||
<td>0</td>
|
||||
<td>
|
||||
Configures the maximum size in bytes per partition that can be allowed to build local hash map. If this value is not smaller than <code>spark.sql.adaptive.advisoryPartitionSizeInBytes</code> and all the partition size are not larger than this config, join selection prefer to use shuffled hash join instead of sort merge join regardless of the value of <code>spark.sql.join.preferSortMergeJoin</code>.
|
||||
</td>
|
||||
<td>3.2.0</td>
|
||||
</tr>
|
||||
</table>
|
||||
|
||||
### Optimizing Skew Join
|
||||
Data skew can severely downgrade the performance of join queries. This feature dynamically handles skew in sort-merge join by splitting (and replicating if needed) skewed tasks into roughly evenly sized tasks. It takes effect when both `spark.sql.adaptive.enabled` and `spark.sql.adaptive.skewJoin.enabled` configurations are enabled.
|
||||
|
|
Loading…
Reference in a new issue