## What changes were proposed in this pull request?
`ReadAheadInputStream` was introduced in https://github.com/apache/spark/pull/18317/ to optimize reading spill files from disk.
However, from the profiles it seems that the hot path of reading small amounts of data (like readInt) is inefficient - it involves taking locks, and multiple checks.
Optimize locking: Lock is not needed when simply accessing the active buffer. Only lock when needing to swap buffers or trigger async reading, or get information about the async state.
Optimize short-path single byte reads, that are used e.g. by Java library DataInputStream.readInt.
The asyncReader used to call "read" only once on the underlying stream, that never filled the underlying buffer when it was wrapping an LZ4BlockInputStream. If the buffer was returned unfilled, that would trigger the async reader to be triggered to fill the read ahead buffer on each call, because the reader would see that the active buffer is below the refill threshold all the time.
However, filling the full buffer all the time could introduce increased latency, so also add an `AtomicBoolean` flag for the async reader to return earlier if there is a reader waiting for data.
Remove `readAheadThresholdInBytes` and instead immediately trigger async read when switching the buffers. It allows to simplify code paths, especially the hot one that then only has to check if there is available data in the active buffer, without worrying if it needs to retrigger async read. It seems to have positive effect on perf.
## How was this patch tested?
It was noticed as a regression in some workloads after upgrading to Spark 2.3.
It was particularly visible on TPCDS Q95 running on instances with fast disk (i3 AWS instances).
Running with profiling:
* Spark 2.2 - 5.2-5.3 minutes 9.5% in LZ4BlockInputStream.read
* Spark 2.3 - 6.4-6.6 minutes 31.1% in ReadAheadInputStream.read
* Spark 2.3 + fix - 5.3-5.4 minutes 13.3% in ReadAheadInputStream.read - very slightly slower, practically within noise.
We didn't see other regressions, and many workloads in general seem to be faster with Spark 2.3 (not investigated if thanks to async readed, or unrelated).
Author: Juliusz Sompolski <julek@databricks.com>
Closes#20555 from juliuszsompolski/SPARK-23366.
## What changes were proposed in this pull request?
When we run concurrent jobs using the same rdd which is marked to do checkpoint. If one job has finished running the job, and start the process of RDD.doCheckpoint, while another job is submitted, then submitStage and submitMissingTasks will be called. In [submitMissingTasks](https://github.com/apache/spark/blob/master/core/src/main/scala/org/apache/spark/scheduler/DAGScheduler.scala#L961), will serialize taskBinaryBytes and calculate task partitions which are both affected by the status of checkpoint, if the former is calculated before doCheckpoint finished, while the latter is calculated after doCheckpoint finished, when run task, rdd.compute will be called, for some rdds with particular partition type such as [UnionRDD](https://github.com/apache/spark/blob/master/core/src/main/scala/org/apache/spark/rdd/UnionRDD.scala) who will do partition type cast, will get a ClassCastException because the part params is actually a CheckpointRDDPartition.
This error occurs because rdd.doCheckpoint occurs in the same thread that called sc.runJob, while the task serialization occurs in the DAGSchedulers event loop.
## How was this patch tested?
the exist uts and also add a test case in DAGScheduerSuite to show the exception case.
Author: huangtengfei <huangtengfei@huangtengfeideMacBook-Pro.local>
Closes#20244 from ivoson/branch-taskpart-mistype.
## What changes were proposed in this pull request?
The purpose of this PR to reflect the stage level blacklisting on the executor tab for the currently active stages.
After this change in the executor tab at the Status column one of the following label will be:
- "Blacklisted" when the executor is blacklisted application level (old flag)
- "Dead" when the executor is not Blacklisted and not Active
- "Blacklisted in Stages: [...]" when the executor is Active but the there are active blacklisted stages for the executor. Within the [] coma separated active stageIDs are listed.
- "Active" when the executor is Active and there is no active blacklisted stages for the executor
## How was this patch tested?
Both with unit tests and manually.
#### Manual test
Spark was started as:
```bash
bin/spark-shell --master "local-cluster[2,1,1024]" --conf "spark.blacklist.enabled=true" --conf "spark.blacklist.stage.maxFailedTasksPerExecutor=1" --conf "spark.blacklist.application.maxFailedTasksPerExecutor=10"
```
And the job was:
```scala
import org.apache.spark.SparkEnv
val pairs = sc.parallelize(1 to 10000, 10).map { x =>
if (SparkEnv.get.executorId.toInt == 0) throw new RuntimeException("Bad executor")
else {
Thread.sleep(10)
(x % 10, x)
}
}
val all = pairs.cogroup(pairs)
all.collect()
```
UI screenshots about the running:
- One executor is blacklisted in the two stages:

- One stage completes the other one is still running:

- Both stages are completed:

### Unit tests
In AppStatusListenerSuite.scala both the node blacklisting for a stage and the executor blacklisting for stage are tested.
Author: “attilapiros” <piros.attila.zsolt@gmail.com>
Closes#20408 from attilapiros/SPARK-23189.
## What changes were proposed in this pull request?
In this PR StorageStatus is made to private and simplified a bit moreover SparkContext.getExecutorStorageStatus method is removed. The reason of keeping StorageStatus is that it is usage from SparkContext.getRDDStorageInfo.
Instead of the method SparkContext.getExecutorStorageStatus executor infos are extended with additional memory metrics such as usedOnHeapStorageMemory, usedOffHeapStorageMemory, totalOnHeapStorageMemory, totalOffHeapStorageMemory.
## How was this patch tested?
By running existing unit tests.
Author: “attilapiros” <piros.attila.zsolt@gmail.com>
Author: Attila Zsolt Piros <2017933+attilapiros@users.noreply.github.com>
Closes#20546 from attilapiros/SPARK-20659.
## What changes were proposed in this pull request?
In the `getBlockData`,`blockId.reduceId` is the `Int` type, when it is greater than 2^28, `blockId.reduceId*8` will overflow
In the `decompress0`, `len` and `unitSize` are Int type, so `len * unitSize` may lead to overflow
## How was this patch tested?
N/A
Author: liuxian <liu.xian3@zte.com.cn>
Closes#20581 from 10110346/overflow2.
## What changes were proposed in this pull request?
Currently, we use SBT and MAVN to spark unit test, are affected by the parameters of `spark.testing`. However, when using the IDE test tool, `spark.testing` support is not very good, sometimes need to be manually added to the beforeEach. example: HiveSparkSubmitSuite RPackageUtilsSuite SparkSubmitSuite. The PR unified `spark.testing` parameter extraction to SparkFunSuite, support IDE test tool, and the test code is more compact.
## How was this patch tested?
the existed test cases.
Author: caoxuewen <cao.xuewen@zte.com.cn>
Closes#20582 from heary-cao/sparktesting.
## What changes were proposed in this pull request?
In the `checkIndexAndDataFile`,the `blocks` is the ` Int` type, when it is greater than 2^28, `blocks*8` will overflow, and this will result in an error result.
In fact, `blocks` is actually the number of partitions.
## How was this patch tested?
Manual test
Author: liuxian <liu.xian3@zte.com.cn>
Closes#20544 from 10110346/overflow.
## What changes were proposed in this pull request?
When `DebugFilesystem` closes opened stream, if any exception occurs, we still need to remove the open stream record from `DebugFilesystem`. Otherwise, it goes to report leaked filesystem connection.
## How was this patch tested?
Existing tests.
Author: Liang-Chi Hsieh <viirya@gmail.com>
Closes#20524 from viirya/SPARK-23345.
## What changes were proposed in this pull request?
This is a follow-up of #20492 which broke lint-java checks.
This pr fixes the lint-java issues.
```
[ERROR] src/main/java/org/apache/spark/util/collection/unsafe/sort/UnsafeSorterSpillReader.java:[79] (sizes) LineLength: Line is longer than 100 characters (found 114).
```
## How was this patch tested?
Checked manually in my local environment.
Author: Takuya UESHIN <ueshin@databricks.com>
Closes#20514 from ueshin/issues/SPARK-23310/fup1.
## What changes were proposed in this pull request?
When a task is still running, metrics like executorRunTime are not available. Then `schedulerDelay` will be almost the same as `duration` and that's confusing.
This PR makes `schedulerDelay` return 0 when the task is running which is the same behavior as 2.2.
## How was this patch tested?
`AppStatusUtilsSuite.schedulerDelay`
Author: Shixiong Zhu <zsxwing@gmail.com>
Closes#20493 from zsxwing/SPARK-23326.
## What changes were proposed in this pull request?
Sort jobs/stages/tasks/queries with the completed timestamp before cleaning up them to make the behavior consistent with 2.2.
## How was this patch tested?
- Jenkins.
- Manually ran the following codes and checked the UI for jobs/stages/tasks/queries.
```
spark.ui.retainedJobs 10
spark.ui.retainedStages 10
spark.sql.ui.retainedExecutions 10
spark.ui.retainedTasks 10
```
```
new Thread() {
override def run() {
spark.range(1, 2).foreach { i =>
Thread.sleep(10000)
}
}
}.start()
Thread.sleep(5000)
for (_ <- 1 to 20) {
new Thread() {
override def run() {
spark.range(1, 2).foreach { i =>
}
}
}.start()
}
Thread.sleep(15000)
spark.range(1, 2).foreach { i =>
}
sc.makeRDD(1 to 100, 100).foreach { i =>
}
```
Author: Shixiong Zhu <zsxwing@gmail.com>
Closes#20481 from zsxwing/SPARK-23307.
## What changes were proposed in this pull request?
Shuffle Index temporay file is used for atomic creating shuffle index file, it is not needed when the index file already exists after another attempts of same task had it done.
## How was this patch tested?
exitsting ut
cc squito
Author: Kent Yao <yaooqinn@hotmail.com>
Closes#20422 from yaooqinn/SPARK-23253.
## What changes were proposed in this pull request?
here is race condition in TaskMemoryManger, which may cause OOM.
The memory released may be taken by another task because there is a gap between releaseMemory and acquireMemory, e.g., UnifiedMemoryManager, causing the OOM. if the current is the only one that can perform spill. It can happen to BytesToBytesMap, as it only spill required bytes.
Loop on current consumer if it still has memory to release.
## How was this patch tested?
The race contention is hard to reproduce, but the current logic seems causing the issue.
Please review http://spark.apache.org/contributing.html before opening a pull request.
Author: Zhan Zhang <zhanzhang@fb.com>
Closes#20480 from zhzhan/oom.
First the bad news: there's an unfixable race in the launcher code.
(By unfixable I mean it would take a lot more effort than this change
to fix it.) The good news is that it should only affect super short
lived applications, such as the one run by the flaky test, so it's
possible to work around it in our test.
The fix also uncovered an issue with the recently added "closeAndWait()"
method; closing the connection would still possibly cause data loss,
so this change waits a while for the connection to finish itself, and
closes the socket if that times out. The existing connection timeout
is reused so that if desired it's possible to control how long to wait.
As part of that I also restored the old behavior that disconnect() would
force a disconnection from the child app; the "wait for data to arrive"
approach is only taken when disposing of the handle.
I tested this by inserting a bunch of sleeps in the test and the socket
handling code in the launcher library; with those I was able to reproduce
the error from the jenkins jobs. With the changes, even with all the
sleeps still in place, all tests pass.
Author: Marcelo Vanzin <vanzin@cloudera.com>
Closes#20462 from vanzin/SPARK-23020.
## What changes were proposed in this pull request?
`channel.write(buf)` may not write the whole buffer since the underlying channel is a FileChannel, we should retry until the whole buffer is written.
## How was this patch tested?
Jenkins
Author: Shixiong Zhu <zsxwing@gmail.com>
Closes#20461 from zsxwing/SPARK-23289.
## What changes were proposed in this pull request?
Rename the public APIs and names of pandas udfs.
- `PANDAS SCALAR UDF` -> `SCALAR PANDAS UDF`
- `PANDAS GROUP MAP UDF` -> `GROUPED MAP PANDAS UDF`
- `PANDAS GROUP AGG UDF` -> `GROUPED AGG PANDAS UDF`
## How was this patch tested?
The existing tests
Author: gatorsmile <gatorsmile@gmail.com>
Closes#20428 from gatorsmile/renamePandasUDFs.
## What changes were proposed in this pull request?
History server not showing incomplete/running applications when spark.history.ui.maxApplications property is set to a value that is smaller than the total number of applications.
## How was this patch tested?
Verified manually against master and 2.2.2 branch.
Author: Paul Mackles <pmackles@adobe.com>
Closes#20335 from pmackles/SPARK-23088.
The JVM seems to be doing early binding of classes that the Hive provider
depends on, causing an error to be thrown before it was caught by the code
in the class.
The fix wraps the creation of the provider in a try..catch so that
the provider can be ignored when dependencies are missing.
Added a unit test (which fails without the fix), and also tested
that getting tokens still works in a real cluster.
Author: Marcelo Vanzin <vanzin@cloudera.com>
Closes#20399 from vanzin/SPARK-23209.
## What changes were proposed in this pull request?
Currently shuffle repartition uses RoundRobinPartitioning, the generated result is nondeterministic since the sequence of input rows are not determined.
The bug can be triggered when there is a repartition call following a shuffle (which would lead to non-deterministic row ordering), as the pattern shows below:
upstream stage -> repartition stage -> result stage
(-> indicate a shuffle)
When one of the executors process goes down, some tasks on the repartition stage will be retried and generate inconsistent ordering, and some tasks of the result stage will be retried generating different data.
The following code returns 931532, instead of 1000000:
```
import scala.sys.process._
import org.apache.spark.TaskContext
val res = spark.range(0, 1000 * 1000, 1).repartition(200).map { x =>
x
}.repartition(200).map { x =>
if (TaskContext.get.attemptNumber == 0 && TaskContext.get.partitionId < 2) {
throw new Exception("pkill -f java".!!)
}
x
}
res.distinct().count()
```
In this PR, we propose a most straight-forward way to fix this problem by performing a local sort before partitioning, after we make the input row ordering deterministic, the function from rows to partitions is fully deterministic too.
The downside of the approach is that with extra local sort inserted, the performance of repartition() will go down, so we add a new config named `spark.sql.execution.sortBeforeRepartition` to control whether this patch is applied. The patch is default enabled to be safe-by-default, but user may choose to manually turn it off to avoid performance regression.
This patch also changes the output rows ordering of repartition(), that leads to a bunch of test cases failure because they are comparing the results directly.
## How was this patch tested?
Add unit test in ExchangeSuite.
With this patch(and `spark.sql.execution.sortBeforeRepartition` set to true), the following query returns 1000000:
```
import scala.sys.process._
import org.apache.spark.TaskContext
spark.conf.set("spark.sql.execution.sortBeforeRepartition", "true")
val res = spark.range(0, 1000 * 1000, 1).repartition(200).map { x =>
x
}.repartition(200).map { x =>
if (TaskContext.get.attemptNumber == 0 && TaskContext.get.partitionId < 2) {
throw new Exception("pkill -f java".!!)
}
x
}
res.distinct().count()
res7: Long = 1000000
```
Author: Xingbo Jiang <xingbo.jiang@databricks.com>
Closes#20393 from jiangxb1987/shuffle-repartition.
## What changes were proposed in this pull request?
The code logic between `MemoryStore.putIteratorAsValues` and `Memory.putIteratorAsBytes` are almost same, so we should reduce the duplicate code between them.
## How was this patch tested?
Existing UT.
Author: Xianyang Liu <xianyang.liu@intel.com>
Closes#19285 from ConeyLiu/rmemorystore.
## What changes were proposed in this pull request?
Currently,the deserializeStream in ExternalAppendOnlyMap#DiskMapIterator init when DiskMapIterator instance created.This will cause memory use overhead when ExternalAppendOnlyMap spill too much times.
We can avoid this by making deserializeStream init when it is used the first time.
This patch make deserializeStream init lazily.
## How was this patch tested?
Exist tests
Author: zhoukang <zhoukang199191@gmail.com>
Closes#20292 from caneGuy/zhoukang/lay-diskmapiterator.
## What changes were proposed in this pull request?
[Ticket](https://issues.apache.org/jira/browse/SPARK-22297)
- one of the tests seems to produce unreliable results due to execution speed variability
Since the original test was trying to connect to the test server with `40 ms` timeout, and the test server replied after `50 ms`, the error might be produced under the following conditions:
- it might occur that the test server replies correctly after `50 ms`
- but the client does only receive the timeout after `51 ms`s
- this might happen if the executor has to schedule a big number of threads, and decides to delay the thread/actor that is responsible to watch the timeout, because of high CPU load
- running an entire test suite usually produces high loads on the CPU executing the tests
## How was this patch tested?
The test's check cases remain the same and the set-up emulates the previous version's.
Author: Mark Petruska <petruska.mark@gmail.com>
Closes#19671 from mpetruska/SPARK-22297.
## What changes were proposed in this pull request?
This is a follow-up of #20297 which broke lint-java checks.
This pr fixes the lint-java issues.
```
[ERROR] src/test/java/org/apache/spark/launcher/BaseSuite.java:[21,8] (imports) UnusedImports: Unused import - java.util.concurrent.TimeUnit.
[ERROR] src/test/java/org/apache/spark/launcher/SparkLauncherSuite.java:[27,8] (imports) UnusedImports: Unused import - java.util.concurrent.TimeUnit.
```
## How was this patch tested?
Checked manually in my local environment.
Author: Takuya UESHIN <ueshin@databricks.com>
Closes#20376 from ueshin/issues/SPARK-23020/fup1.
## What changes were proposed in this pull request?
In this PR stage blacklisting is propagated to UI by introducing a new Spark listener event (SparkListenerExecutorBlacklistedForStage) which indicates the executor is blacklisted for a stage. Either because of the number of failures are exceeded a limit given for an executor (spark.blacklist.stage.maxFailedTasksPerExecutor) or because of the whole node is blacklisted for a stage (spark.blacklist.stage.maxFailedExecutorsPerNode). In case of the node is blacklisting all executors will listed as blacklisted for the stage.
Blacklisting state for a selected stage can be seen "Aggregated Metrics by Executor" table's blacklisting column, where after this change three possible labels could be found:
- "for application": when the executor is blacklisted for the application (see the configuration spark.blacklist.application.maxFailedTasksPerExecutor for details)
- "for stage": when the executor is **only** blacklisted for the stage
- "false" : when the executor is not blacklisted at all
## How was this patch tested?
It is tested both manually and with unit tests.
#### Unit tests
- HistoryServerSuite
- TaskSetBlacklistSuite
- AppStatusListenerSuite
#### Manual test for executor blacklisting
Running Spark as a local cluster:
```
$ bin/spark-shell --master "local-cluster[2,1,1024]" --conf "spark.blacklist.enabled=true" --conf "spark.blacklist.stage.maxFailedTasksPerExecutor=1" --conf "spark.blacklist.application.maxFailedTasksPerExecutor=10" --conf "spark.eventLog.enabled=true"
```
Executing:
``` scala
import org.apache.spark.SparkEnv
sc.parallelize(1 to 10, 10).map { x =>
if (SparkEnv.get.executorId == "0") throw new RuntimeException("Bad executor")
else (x % 3, x)
}.reduceByKey((a, b) => a + b).collect()
```
To see result check the "Aggregated Metrics by Executor" section at the bottom of picture:

#### Manual test for node blacklisting
Running Spark as on a cluster:
``` bash
./bin/spark-shell --master yarn --deploy-mode client --executor-memory=2G --num-executors=8 --conf "spark.blacklist.enabled=true" --conf "spark.blacklist.stage.maxFailedTasksPerExecutor=1" --conf "spark.blacklist.stage.maxFailedExecutorsPerNode=1" --conf "spark.blacklist.application.maxFailedTasksPerExecutor=10" --conf "spark.eventLog.enabled=true"
```
And the job was:
``` scala
import org.apache.spark.SparkEnv
sc.parallelize(1 to 10000, 10).map { x =>
if (SparkEnv.get.executorId.toInt >= 4) throw new RuntimeException("Bad executor")
else (x % 3, x)
}.reduceByKey((a, b) => a + b).collect()
```
The result is:

Here you can see apiros3.gce.test.com was node blacklisted for the stage because of failures on executor 4 and 5. As expected executor 3 is also blacklisted even it has no failures itself but sharing the node with 4 and 5.
Author: “attilapiros” <piros.attila.zsolt@gmail.com>
Author: Attila Zsolt Piros <2017933+attilapiros@users.noreply.github.com>
Closes#20203 from attilapiros/SPARK-22577.
## What changes were proposed in this pull request?
#20002 purposed a way to safe check the default partitioner, however, if `spark.default.parallelism` is set, the defaultParallelism still could be smaller than the proper number of partitions for upstreams RDDs. This PR tries to extend the approach to address the condition when `spark.default.parallelism` is set.
The requirements where the PR helps with are :
- Max partitioner is not eligible since it is atleast an order smaller, and
- User has explicitly set 'spark.default.parallelism', and
- Value of 'spark.default.parallelism' is lower than max partitioner
- Since max partitioner was discarded due to being at least an order smaller, default parallelism is worse - even though user specified.
Under the rest cases, the changes should be no-op.
## How was this patch tested?
Add corresponding test cases in `PairRDDFunctionsSuite` and `PartitioningSuite`.
Author: Xingbo Jiang <xingbo.jiang@databricks.com>
Closes#20091 from jiangxb1987/partitioner.
## What changes were proposed in this pull request?
Add support for using pandas UDFs with groupby().agg().
This PR introduces a new type of pandas UDF - group aggregate pandas UDF. This type of UDF defines a transformation of multiple pandas Series -> a scalar value. Group aggregate pandas UDFs can be used with groupby().agg(). Note group aggregate pandas UDF doesn't support partial aggregation, i.e., a full shuffle is required.
This PR doesn't support group aggregate pandas UDFs that return ArrayType, StructType or MapType. Support for these types is left for future PR.
## How was this patch tested?
GroupbyAggPandasUDFTests
Author: Li Jin <ice.xelloss@gmail.com>
Closes#19872 from icexelloss/SPARK-22274-groupby-agg.
## What changes were proposed in this pull request?
Typo fixes
## How was this patch tested?
Local build / Doc-only changes
Author: Jacek Laskowski <jacek@japila.pl>
Closes#20344 from jaceklaskowski/typo-fixes.
## What changes were proposed in this pull request?
The allJobs and the job pages attempt to use stage attempt and DAG visualization from the store, but for long running jobs they are not guaranteed to be retained, leading to exceptions when these pages are rendered.
To fix it `store.lastStageAttempt(stageId)` and `store.operationGraphForJob(jobId)` are wrapped in `store.asOption` and default values are used if the info is missing.
## How was this patch tested?
Manual testing of the UI, also using the test command reported in SPARK-23121:
./bin/spark-submit --class org.apache.spark.examples.streaming.HdfsWordCount ./examples/jars/spark-examples_2.11-2.4.0-SNAPSHOT.jar /spark
Closes#20287
Author: Sandor Murakozi <smurakozi@gmail.com>
Closes#20330 from smurakozi/SPARK-23121.
## What changes were proposed in this pull request?
ClosureCleaner moved from warning to debug
## How was this patch tested?
Existing tests
Author: Rekha Joshi <rekhajoshm@gmail.com>
Author: rjoshi2 <rekhajoshm@gmail.com>
Closes#20337 from rekhajoshm/SPARK-11630-1.
The race in the code is because the handle might update
its state to the wrong state if the connection handling
thread is still processing incoming data; so the handle
needs to wait for the connection to finish up before
checking the final state.
The race in the test is because when waiting for a handle
to reach a final state, the waitFor() method needs to wait
until all handle state is updated (which also includes
waiting for the connection thread above to finish).
Otherwise, waitFor() may return too early, which would cause
a bunch of different races (like the listener not being yet
notified of the state change, or being in the middle of
being notified, or the handle not being properly disposed
and causing postChecks() to assert).
On top of that I found, by code inspection, a couple of
potential races that could make a handle end up in the
wrong state when being killed.
The original version of this fix introduced the flipped
version of the first race described above; the connection
closing might override the handle state before the
handle might have a chance to do cleanup. The fix there
is to only dispose of the handle from the connection
when there is an error, and let the handle dispose
itself in the normal case.
The fix also caused a bug in YarnClusterSuite to be surfaced;
the code was checking for a file in the classpath that was
not expected to be there in client mode. Because of the above
issues, the error was not propagating correctly and the (buggy)
test was incorrectly passing.
Tested by running the existing unit tests a lot (and not
seeing the errors I was seeing before).
Author: Marcelo Vanzin <vanzin@cloudera.com>
Closes#20297 from vanzin/SPARK-23020.
## What changes were proposed in this pull request?
The clean up logic on the worker perviously determined the liveness of a
particular applicaiton based on whether or not it had running executors.
This would fail in the case that a directory was made for a driver
running in cluster mode if that driver had no running executors on the
same machine. To preserve driver directories we consider both executors
and running drivers when checking directory liveness.
## How was this patch tested?
Manually started up two node cluster with a single core on each node. Turned on worker directory cleanup and set the interval to 1 second and liveness to one second. Without the patch the driver directory is removed immediately after the app is launched. With the patch it is not
### Without Patch
```
INFO 2018-01-05 23:48:24,693 Logging.scala:54 - Asked to launch driver driver-20180105234824-0000
INFO 2018-01-05 23:48:25,293 Logging.scala:54 - Changing view acls to: cassandra
INFO 2018-01-05 23:48:25,293 Logging.scala:54 - Changing modify acls to: cassandra
INFO 2018-01-05 23:48:25,294 Logging.scala:54 - Changing view acls groups to:
INFO 2018-01-05 23:48:25,294 Logging.scala:54 - Changing modify acls groups to:
INFO 2018-01-05 23:48:25,294 Logging.scala:54 - SecurityManager: authentication disabled; ui acls disabled; users with view permissions: Set(cassandra); groups with view permissions: Set(); users with modify permissions: Set(cassandra); groups with modify permissions: Set()
INFO 2018-01-05 23:48:25,330 Logging.scala:54 - Copying user jar file:/home/automaton/writeRead-0.1.jar to /var/lib/spark/worker/driver-20180105234824-0000/writeRead-0.1.jar
INFO 2018-01-05 23:48:25,332 Logging.scala:54 - Copying /home/automaton/writeRead-0.1.jar to /var/lib/spark/worker/driver-20180105234824-0000/writeRead-0.1.jar
INFO 2018-01-05 23:48:25,361 Logging.scala:54 - Launch Command: "/usr/lib/jvm/jdk1.8.0_40//bin/java" ....
****
INFO 2018-01-05 23:48:56,577 Logging.scala:54 - Removing directory: /var/lib/spark/worker/driver-20180105234824-0000 ### << Cleaned up
****
--
One minute passes while app runs (app has 1 minute sleep built in)
--
WARN 2018-01-05 23:49:58,080 ShuffleSecretManager.java:73 - Attempted to unregister application app-20180105234831-0000 when it is not registered
INFO 2018-01-05 23:49:58,081 ExternalShuffleBlockResolver.java:163 - Application app-20180105234831-0000 removed, cleanupLocalDirs = false
INFO 2018-01-05 23:49:58,081 ExternalShuffleBlockResolver.java:163 - Application app-20180105234831-0000 removed, cleanupLocalDirs = false
INFO 2018-01-05 23:49:58,082 ExternalShuffleBlockResolver.java:163 - Application app-20180105234831-0000 removed, cleanupLocalDirs = true
INFO 2018-01-05 23:50:00,999 Logging.scala:54 - Driver driver-20180105234824-0000 exited successfully
```
With Patch
```
INFO 2018-01-08 23:19:54,603 Logging.scala:54 - Asked to launch driver driver-20180108231954-0002
INFO 2018-01-08 23:19:54,975 Logging.scala:54 - Changing view acls to: automaton
INFO 2018-01-08 23:19:54,976 Logging.scala:54 - Changing modify acls to: automaton
INFO 2018-01-08 23:19:54,976 Logging.scala:54 - Changing view acls groups to:
INFO 2018-01-08 23:19:54,976 Logging.scala:54 - Changing modify acls groups to:
INFO 2018-01-08 23:19:54,976 Logging.scala:54 - SecurityManager: authentication disabled; ui acls disabled; users with view permissions: Set(automaton); groups with view permissions: Set(); users with modify permissions: Set(automaton); groups with modify permissions: Set()
INFO 2018-01-08 23:19:55,029 Logging.scala:54 - Copying user jar file:/home/automaton/writeRead-0.1.jar to /var/lib/spark/worker/driver-20180108231954-0002/writeRead-0.1.jar
INFO 2018-01-08 23:19:55,031 Logging.scala:54 - Copying /home/automaton/writeRead-0.1.jar to /var/lib/spark/worker/driver-20180108231954-0002/writeRead-0.1.jar
INFO 2018-01-08 23:19:55,038 Logging.scala:54 - Launch Command: ......
INFO 2018-01-08 23:21:28,674 ShuffleSecretManager.java:69 - Unregistered shuffle secret for application app-20180108232000-0000
INFO 2018-01-08 23:21:28,675 ExternalShuffleBlockResolver.java:163 - Application app-20180108232000-0000 removed, cleanupLocalDirs = false
INFO 2018-01-08 23:21:28,675 ExternalShuffleBlockResolver.java:163 - Application app-20180108232000-0000 removed, cleanupLocalDirs = false
INFO 2018-01-08 23:21:28,681 ExternalShuffleBlockResolver.java:163 - Application app-20180108232000-0000 removed, cleanupLocalDirs = true
INFO 2018-01-08 23:21:31,703 Logging.scala:54 - Driver driver-20180108231954-0002 exited successfully
*****
INFO 2018-01-08 23:21:32,346 Logging.scala:54 - Removing directory: /var/lib/spark/worker/driver-20180108231954-0002 ### < Happening AFTER the Run completes rather than during it
*****
```
Author: Russell Spitzer <Russell.Spitzer@gmail.com>
Closes#20298 from RussellSpitzer/SPARK-22976-master.
The code was sorting "0" as "less than" negative values, which is a little
wrong. Fix is simple, most of the changes are the added test and related
cleanup.
Author: Marcelo Vanzin <vanzin@cloudera.com>
Closes#20284 from vanzin/SPARK-23103.
Detect the deletion of event log files from storage, and remove
data about the related application attempt in the SHS.
Also contains code to fix SPARK-21571 based on code by ericvandenbergfb.
Author: Marcelo Vanzin <vanzin@cloudera.com>
Closes#20138 from vanzin/SPARK-20664.
## What changes were proposed in this pull request?
Spark ui about the contents of the form need to have hidden and show features, when the table records very much. Because sometimes you do not care about the record of the table, you just want to see the contents of the next table, but you have to scroll the scroll bar for a long time to see the contents of the next table.
Currently we have about 500 workers, but I just wanted to see the logs for the running applications table. I had to scroll through the scroll bars for a long time to see the logs for the running applications table.
In order to ensure functional consistency, I modified the Master Page, Worker Page, Job Page, Stage Page, Task Page, Configuration Page, Storage Page, Pool Page.
fix before:
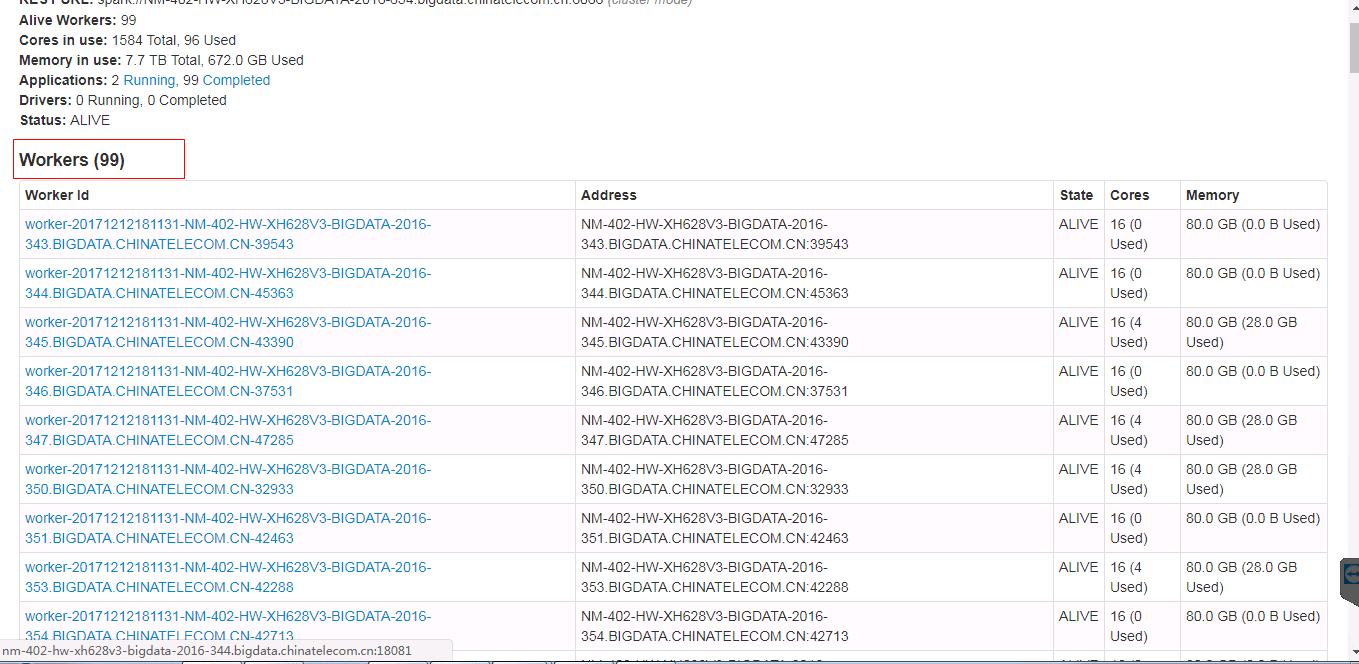
fix after:
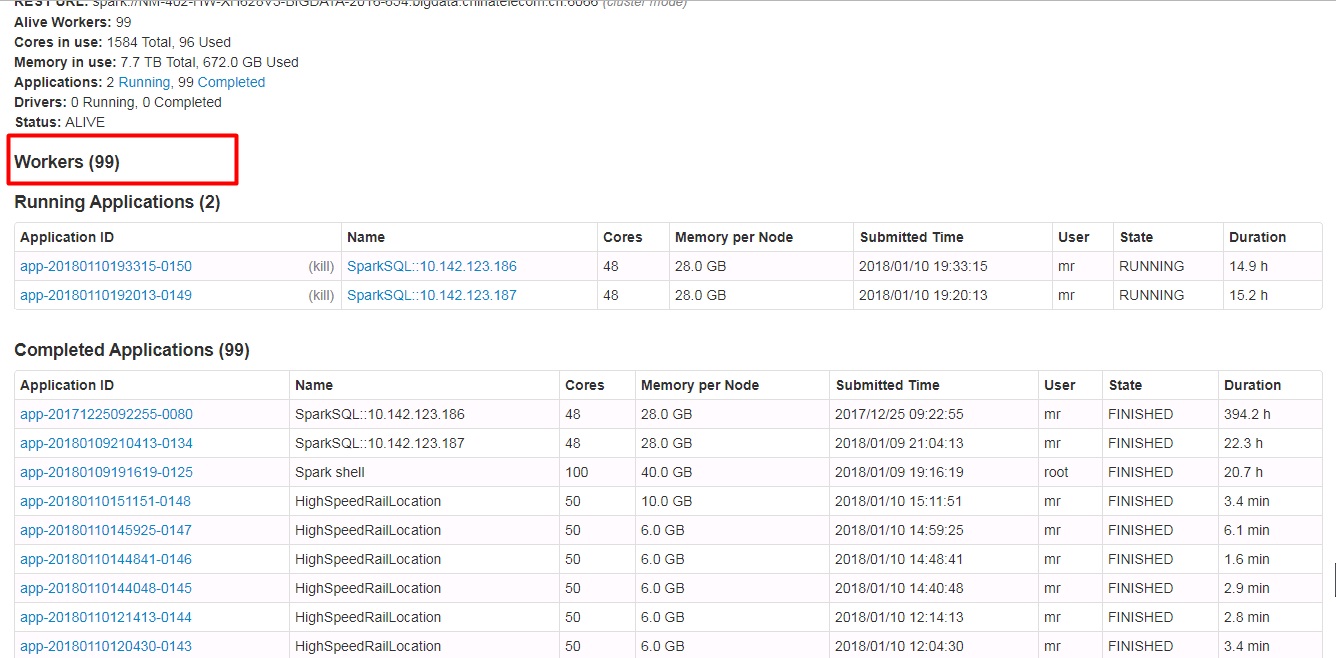
## How was this patch tested?
manual tests
Please review http://spark.apache.org/contributing.html before opening a pull request.
Author: guoxiaolong <guo.xiaolong1@zte.com.cn>
Closes#20216 from guoxiaolongzte/SPARK-23024.
## What changes were proposed in this pull request?
This PR completes the docs, specifying the default units assumed in configuration entries of type size.
This is crucial since unit-less values are accepted and the user might assume the base unit is bytes, which in most cases it is not, leading to hard-to-debug problems.
## How was this patch tested?
This patch updates only documentation only.
Author: Fernando Pereira <fernando.pereira@epfl.ch>
Closes#20269 from ferdonline/docs_units.
## What changes were proposed in this pull request?
Stage's task page table will throw an exception when there's no complete tasks. Furthermore, because the `dataSize` doesn't take running tasks into account, so sometimes UI cannot show the running tasks. Besides table will only be displayed when first task is finished according to the default sortColumn("index").
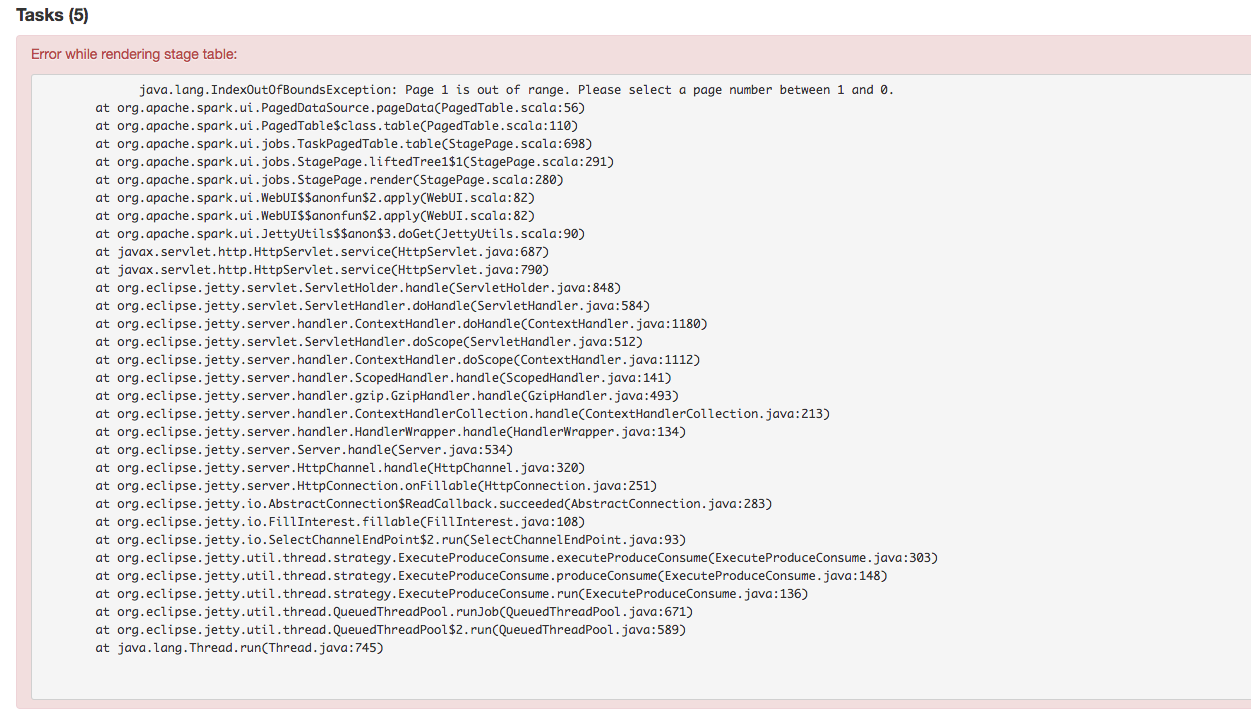
To reproduce this issue, user could try `sc.parallelize(1 to 20, 20).map { i => Thread.sleep(10000); i }.collect()` or `sc.parallelize(1 to 20, 20).map { i => Thread.sleep((20 - i) * 1000); i }.collect` to reproduce the above issue.
Here propose a solution to fix it. Not sure if it is a right fix, please help to review.
## How was this patch tested?
Manual test.
Author: jerryshao <sshao@hortonworks.com>
Closes#20315 from jerryshao/SPARK-23147.
## What changes were proposed in this pull request?
Temporarily ignoring flaky test `SparkLauncherSuite.testInProcessLauncher` to de-flake the builds. This should be re-enabled when SPARK-23020 is merged.
## How was this patch tested?
N/A (Test Only Change)
Author: Sameer Agarwal <sameerag@apache.org>
Closes#20291 from sameeragarwal/disable-test-2.
## What changes were proposed in this pull request?
Lots of our tests don't properly shutdown everything they create, and end up leaking lots of threads. For example, `TaskSetManagerSuite` doesn't stop the extra `TaskScheduler` and `DAGScheduler` it creates. There are a couple more instances, eg. in `DAGSchedulerSuite`.
This PR adds the possibility to print out the not properly stopped thread list after a test suite executed. The format is the following:
```
===== FINISHED o.a.s.scheduler.DAGSchedulerSuite: 'task end event should have updated accumulators (SPARK-20342)' =====
...
===== Global thread whitelist loaded with name /thread_whitelist from classpath: rpc-client.*, rpc-server.*, shuffle-client.*, shuffle-server.*' =====
ScalaTest-run:
===== THREADS NOT STOPPED PROPERLY =====
ScalaTest-run: dag-scheduler-event-loop
ScalaTest-run: globalEventExecutor-2-5
ScalaTest-run:
===== END OF THREAD DUMP =====
ScalaTest-run:
===== EITHER PUT THREAD NAME INTO THE WHITELIST FILE OR SHUT IT DOWN PROPERLY =====
```
With the help of this leaking threads has been identified in TaskSetManagerSuite. My intention is to hunt down and fix such bugs in later PRs.
## How was this patch tested?
Manual: TaskSetManagerSuite test executed and found out where are the leaking threads.
Automated: Pass the Jenkins.
Author: Gabor Somogyi <gabor.g.somogyi@gmail.com>
Closes#19893 from gaborgsomogyi/SPARK-16139.
The race in the code is because the handle might update
its state to the wrong state if the connection handling
thread is still processing incoming data; so the handle
needs to wait for the connection to finish up before
checking the final state.
The race in the test is because when waiting for a handle
to reach a final state, the waitFor() method needs to wait
until all handle state is updated (which also includes
waiting for the connection thread above to finish).
Otherwise, waitFor() may return too early, which would cause
a bunch of different races (like the listener not being yet
notified of the state change, or being in the middle of
being notified, or the handle not being properly disposed
and causing postChecks() to assert).
On top of that I found, by code inspection, a couple of
potential races that could make a handle end up in the
wrong state when being killed.
Tested by running the existing unit tests a lot (and not
seeing the errors I was seeing before).
Author: Marcelo Vanzin <vanzin@cloudera.com>
Closes#20223 from vanzin/SPARK-23020.
## What changes were proposed in this pull request?
In 2.2, Spark UI displayed the stage description if the job description was not set. This functionality was broken, the GUI has shown no description in this case. In addition, the code uses jobName and
jobDescription instead of stageName and stageDescription when JobTableRowData is created.
In this PR the logic producing values for the job rows was modified to find the latest stage attempt for the job and use that as a fallback if job description was missing.
StageName and stageDescription are also set using values from stage and jobName/description is used only as a fallback.
## How was this patch tested?
Manual testing of the UI, using the code in the bug report.
Author: Sandor Murakozi <smurakozi@gmail.com>
Closes#20251 from smurakozi/SPARK-23051.
## What changes were proposed in this pull request?
We are now forced to use `pyspark/daemon.py` and `pyspark/worker.py` in PySpark.
This doesn't allow a custom modification for it (well, maybe we can still do this in a super hacky way though, for example, setting Python executable that has the custom modification). Because of this, for example, it's sometimes hard to debug what happens inside Python worker processes.
This is actually related with [SPARK-7721](https://issues.apache.org/jira/browse/SPARK-7721) too as somehow Coverage is unable to detect the coverage from `os.fork`. If we have some custom fixes to force the coverage, it works fine.
This is also related with [SPARK-20368](https://issues.apache.org/jira/browse/SPARK-20368). This JIRA describes Sentry support which (roughly) needs some changes within worker side.
With this configuration advanced users will be able to do a lot of pluggable workarounds and we can meet such potential needs in the future.
As an example, let's say if I configure the module `coverage_daemon` and had `coverage_daemon.py` in the python path:
```python
import os
from pyspark import daemon
if "COVERAGE_PROCESS_START" in os.environ:
from pyspark.worker import main
def _cov_wrapped(*args, **kwargs):
import coverage
cov = coverage.coverage(
config_file=os.environ["COVERAGE_PROCESS_START"])
cov.start()
try:
main(*args, **kwargs)
finally:
cov.stop()
cov.save()
daemon.worker_main = _cov_wrapped
if __name__ == '__main__':
daemon.manager()
```
I can track the coverages in worker side too.
More importantly, we can leave the main code intact but allow some workarounds.
## How was this patch tested?
Manually tested.
Author: hyukjinkwon <gurwls223@gmail.com>
Closes#20151 from HyukjinKwon/configuration-daemon-worker.
## What changes were proposed in this pull request?
This pr to make `0` as a valid value for `spark.dynamicAllocation.executorIdleTimeout`.
For details, see the jira description: https://issues.apache.org/jira/browse/SPARK-22870.
## How was this patch tested?
N/A
Author: Yuming Wang <yumwang@ebay.com>
Author: Yuming Wang <wgyumg@gmail.com>
Closes#20080 from wangyum/SPARK-22870.
## What changes were proposed in this pull request?
Spark still use a few years old version 3.2.11. This change is to upgrade json4s to 3.5.3.
Note that this change does not include the Jackson update because the Jackson version referenced in json4s 3.5.3 is 2.8.4, which has a security vulnerability ([see](https://issues.apache.org/jira/browse/SPARK-20433)).
## How was this patch tested?
Existing unit tests and build.
Author: shimamoto <chibochibo@gmail.com>
Closes#20233 from shimamoto/upgrade-json4s.
## What changes were proposed in this pull request?
This patch bumps the master branch version to `2.4.0-SNAPSHOT`.
## How was this patch tested?
N/A
Author: gatorsmile <gatorsmile@gmail.com>
Closes#20222 from gatorsmile/bump24.
When resources happen to be constrained on an executor the first time a broadcast variable is instantiated it is persisted to disk by the BlockManager. Consequently, every subsequent call to TorrentBroadcast::readBroadcastBlock from other instances of that broadcast variable spawns another instance of the underlying value. That is, broadcast variables are spawned once per executor **unless** memory is constrained, in which case every instance of a broadcast variable is provided with a unique copy of the underlying value.
This patch fixes the above by explicitly caching the underlying values using weak references in a ReferenceMap.
Author: ho3rexqj <ho3rexqj@gmail.com>
Closes#20183 from ho3rexqj/fix/cache-broadcast-values.
There are two main changes to speed up rendering of the tasks list
when rendering the stage page.
The first one makes the code only load the tasks being shown in the
current page of the tasks table, and information related to only
those tasks. One side-effect of this change is that the graph that
shows task-related events now only shows events for the tasks in
the current page, instead of the previously hardcoded limit of "events
for the first 1000 tasks". That ends up helping with readability,
though.
To make sorting efficient when using a disk store, the task wrapper
was extended to include many new indices, one for each of the sortable
columns in the UI, and metrics for which quantiles are calculated.
The second changes the way metric quantiles are calculated for stages.
Instead of using the "Distribution" class to process data for all task
metrics, which requires scanning all tasks of a stage, the code now
uses the KVStore "skip()" functionality to only read tasks that contain
interesting information for the quantiles that are desired.
This is still not cheap; because there are many metrics that the UI
and API track, the code needs to scan the index for each metric to
gather the information. Savings come mainly from skipping deserialization
when using the disk store, but the in-memory code also seems to be
faster than before (most probably because of other changes in this
patch).
To make subsequent calls faster, some quantiles are cached in the
status store. This makes UIs much faster after the first time a stage
has been loaded.
With the above changes, a lot of code in the UI layer could be simplified.
Author: Marcelo Vanzin <vanzin@cloudera.com>
Closes#20013 from vanzin/SPARK-20657.
## What changes were proposed in this pull request?
This patch modifies Spark's `MemoryAllocator` implementations so that `free(MemoryBlock)` mutates the passed block to clear pointers (in the off-heap case) or null out references to backing `long[]` arrays (in the on-heap case). The goal of this change is to add an extra layer of defense against use-after-free bugs because currently it's hard to detect corruption caused by blind writes to freed memory blocks.
## How was this patch tested?
New unit tests in `PlatformSuite`, including new tests for existing functionality because we did not have sufficient mutation coverage of the on-heap memory allocator's pooling logic.
Author: Josh Rosen <joshrosen@databricks.com>
Closes#20191 from JoshRosen/SPARK-22997-add-defenses-against-use-after-free-bugs-in-memory-allocator.
## What changes were proposed in this pull request?
This patch fixes a severe asynchronous IO bug in Spark's Netty-based file transfer code. At a high-level, the problem is that an unsafe asynchronous `close()` of a pipe's source channel creates a race condition where file transfer code closes a file descriptor then attempts to read from it. If the closed file descriptor's number has been reused by an `open()` call then this invalid read may cause unrelated file operations to return incorrect results. **One manifestation of this problem is incorrect query results.**
For a high-level overview of how file download works, take a look at the control flow in `NettyRpcEnv.openChannel()`: this code creates a pipe to buffer results, then submits an asynchronous stream request to a lower-level TransportClient. The callback passes received data to the sink end of the pipe. The source end of the pipe is passed back to the caller of `openChannel()`. Thus `openChannel()` returns immediately and callers interact with the returned pipe source channel.
Because the underlying stream request is asynchronous, errors may occur after `openChannel()` has returned and after that method's caller has started to `read()` from the returned channel. For example, if a client requests an invalid stream from a remote server then the "stream does not exist" error may not be received from the remote server until after `openChannel()` has returned. In order to be able to propagate the "stream does not exist" error to the file-fetching application thread, this code wraps the pipe's source channel in a special `FileDownloadChannel` which adds an `setError(t: Throwable)` method, then calls this `setError()` method in the FileDownloadCallback's `onFailure` method.
It is possible for `FileDownloadChannel`'s `read()` and `setError()` methods to be called concurrently from different threads: the `setError()` method is called from within the Netty RPC system's stream callback handlers, while the `read()` methods are called from higher-level application code performing remote stream reads.
The problem lies in `setError()`: the existing code closed the wrapped pipe source channel. Because `read()` and `setError()` occur in different threads, this means it is possible for one thread to be calling `source.read()` while another asynchronously calls `source.close()`. Java's IO libraries do not guarantee that this will be safe and, in fact, it's possible for these operations to interleave in such a way that a lower-level `read()` system call occurs right after a `close()` call. In the best-case, this fails as a read of a closed file descriptor; in the worst-case, the file descriptor number has been re-used by an intervening `open()` operation and the read corrupts the result of an unrelated file IO operation being performed by a different thread.
The solution here is to remove the `stream.close()` call in `onError()`: the thread that is performing the `read()` calls is responsible for closing the stream in a `finally` block, so there's no need to close it here. If that thread is blocked in a `read()` then it will become unblocked when the sink end of the pipe is closed in `FileDownloadCallback.onFailure()`.
After making this change, we also need to refine the `read()` method to always check for a `setError()` result, even if the underlying channel `read()` call has succeeded.
This patch also makes a slight cleanup to a dodgy-looking `catch e: Exception` block to use a safer `try-finally` error handling idiom.
This bug was introduced in SPARK-11956 / #9941 and is present in Spark 1.6.0+.
## How was this patch tested?
This fix was tested manually against a workload which non-deterministically hit this bug.
Author: Josh Rosen <joshrosen@databricks.com>
Closes#20179 from JoshRosen/SPARK-22982-fix-unsafe-async-io-in-file-download-channel.
## What changes were proposed in this pull request?
In current implementation of RDD.take, we overestimate the number of partitions we need to try by 50%:
`(1.5 * num * partsScanned / buf.size).toInt`
However, when the number is small, the result of `.toInt` is not what we want.
E.g, 2.9 will become 2, which should be 3.
Use Math.ceil to fix the problem.
Also clean up the code in RDD.scala.
## How was this patch tested?
Unit test
Author: Wang Gengliang <ltnwgl@gmail.com>
Closes#20200 from gengliangwang/Take.
## What changes were proposed in this pull request?
In current implementation, the function `isFairScheduler` is always false, since it is comparing String with `SchedulingMode`
Author: Wang Gengliang <ltnwgl@gmail.com>
Closes#20186 from gengliangwang/isFairScheduler.
## What changes were proposed in this pull request?
1. Deprecate attemptId in StageInfo and add `def attemptNumber() = attemptId`
2. Replace usage of stageAttemptId with stageAttemptNumber
## How was this patch tested?
I manually checked the compiler warning info
Author: Xianjin YE <advancedxy@gmail.com>
Closes#20178 from advancedxy/SPARK-22952.
## What changes were proposed in this pull request?
Register spark.history.ui.port as a known spark conf to be used in substitution expressions even if it's not set explicitly.
## How was this patch tested?
Added unit test to demonstrate the issue
Author: Gera Shegalov <gera@apache.org>
Author: Gera Shegalov <gshegalov@salesforce.com>
Closes#20098 from gerashegalov/gera/register-SHS-port-conf.
## What changes were proposed in this pull request?
We missed enabling `spark.files` and `spark.jars` in https://github.com/apache/spark/pull/19954. The result is that remote dependencies specified through `spark.files` or `spark.jars` are not included in the list of remote dependencies to be downloaded by the init-container. This PR fixes it.
## How was this patch tested?
Manual tests.
vanzin This replaces https://github.com/apache/spark/pull/20157.
foxish
Author: Yinan Li <liyinan926@gmail.com>
Closes#20160 from liyinan926/SPARK-22757.
The code in LiveListenerBus was queueing events before start in the
queues themselves; so in situations like the following:
bus.post(someEvent)
bus.addToEventLogQueue(listener)
bus.start()
"someEvent" would not be delivered to "listener" if that was the first
listener in the queue, because the queue wouldn't exist when the
event was posted.
This change buffers the events before starting the bus in the bus itself,
so that they can be delivered to all registered queues when the bus is
started.
Also tweaked the unit tests to cover the behavior above.
Author: Marcelo Vanzin <vanzin@cloudera.com>
Closes#20039 from vanzin/SPARK-22850.
## What changes were proposed in this pull request?
When overwriting a partitioned table with dynamic partition columns, the behavior is different between data source and hive tables.
data source table: delete all partition directories that match the static partition values provided in the insert statement.
hive table: only delete partition directories which have data written into it
This PR adds a new config to make users be able to choose hive's behavior.
## How was this patch tested?
new tests
Author: Wenchen Fan <wenchen@databricks.com>
Closes#18714 from cloud-fan/overwrite-partition.
## What changes were proposed in this pull request?
stageAttemptId added in TaskContext and corresponding construction modification
## How was this patch tested?
Added a new test in TaskContextSuite, two cases are tested:
1. Normal case without failure
2. Exception case with resubmitted stages
Link to [SPARK-22897](https://issues.apache.org/jira/browse/SPARK-22897)
Author: Xianjin YE <advancedxy@gmail.com>
Closes#20082 from advancedxy/SPARK-22897.
## What changes were proposed in this pull request?
This reverts commit 5fd0294ff8 because of a huge performance regression.
I manually fixed a minor conflict in `OneForOneBlockFetcher.java`.
`Files.newInputStream` returns `sun.nio.ch.ChannelInputStream`. `ChannelInputStream` doesn't override `InputStream.skip`, so it's using the default `InputStream.skip` which just consumes and discards data. This causes a huge performance regression when reading shuffle files.
## How was this patch tested?
Jenkins
Author: Shixiong Zhu <zsxwing@gmail.com>
Closes#20119 from zsxwing/revert-SPARK-21475.
The scheduled task was racing with the test code and could influence
the values returned to the test, triggering assertions. The change adds
a new config that is only used during testing, and overrides it
on the affected test suite.
The issue in the bug can be reliably reproduced by reducing the interval
in the test (e.g. to 10ms).
While there, fixed an exception that shows up in the logs while these
tests run, and simplified some code (which was also causing misleading
log messages in the log output of the test).
Author: Marcelo Vanzin <vanzin@cloudera.com>
Closes#20050 from vanzin/SPARK-22864.
This change adds a new configuration option and support code that limits
how much disk space the SHS will use. The default value is pretty generous
so that applications will, hopefully, only rarely need to be replayed
because of their disk stored being evicted.
This works by keeping track of how much data each application is using.
Also, because it's not possible to know, before replaying, how much space
will be needed, it's possible that usage will exceed the configured limit
temporarily. The code uses the concept of a "lease" to try to limit how
much the SHS will exceed the limit in those cases.
Active UIs are also tracked, so they're never deleted. This works in
tandem with the existing option of how many active UIs are loaded; because
unused UIs will be unloaded, their disk stores will also become candidates
for deletion. If the data is not deleted, though, re-loading the UI is
pretty quick.
Author: Marcelo Vanzin <vanzin@cloudera.com>
Closes#20011 from vanzin/SPARK-20654.
This change adds a new launcher that allows applications to be run
in a separate thread in the same process as the calling code. To
achieve that, some code from the child process implementation was
moved to abstract classes that implement the common functionality,
and the new launcher inherits from those.
The new launcher was added as a new class, instead of implemented
as a new option to the existing SparkLauncher, to avoid ambigous
APIs. For example, SparkLauncher has ways to set the child app's
environment, modify SPARK_HOME, or control the logging of the
child process, none of which apply to in-process apps.
The in-process launcher has limitations: it needs Spark in the
context class loader of the calling thread, and it's bound by
Spark's current limitation of a single client-mode application
per JVM. It also relies on the recently added SparkApplication
trait to make sure different apps don't mess up each other's
configuration, so config isolation is currently limited to cluster mode.
I also chose to keep the same socket-based communication for in-process
apps, even though it might be possible to avoid it for in-process
mode. That helps both implementations share more code.
Tested with new and existing unit tests, and with a simple app that
uses the launcher; also made sure the app ran fine with older launcher
jar to check binary compatibility.
Author: Marcelo Vanzin <vanzin@cloudera.com>
Closes#19591 from vanzin/SPARK-11035.
Port code from the old executors listener to the new one, so that
the driver logs present in the application start event are kept.
Author: Marcelo Vanzin <vanzin@cloudera.com>
Closes#20038 from vanzin/SPARK-22836.
## What changes were proposed in this pull request?
This PR cleans up a few Java linter errors for Apache Spark 2.3 release.
## How was this patch tested?
```bash
$ dev/lint-java
Using `mvn` from path: /usr/local/bin/mvn
Checkstyle checks passed.
```
We can see the result from [Travis CI](https://travis-ci.org/dongjoon-hyun/spark/builds/322470787), too.
Author: Dongjoon Hyun <dongjoon@apache.org>
Closes#20101 from dongjoon-hyun/fix-java-lint.
## What changes were proposed in this pull request?
In SPARK-20586 the flag `deterministic` was added to Scala UDF, but it is not available for python UDF. This flag is useful for cases when the UDF's code can return different result with the same input. Due to optimization, duplicate invocations may be eliminated or the function may even be invoked more times than it is present in the query. This can lead to unexpected behavior.
This PR adds the deterministic flag, via the `asNondeterministic` method, to let the user mark the function as non-deterministic and therefore avoid the optimizations which might lead to strange behaviors.
## How was this patch tested?
Manual tests:
```
>>> from pyspark.sql.functions import *
>>> from pyspark.sql.types import *
>>> df_br = spark.createDataFrame([{'name': 'hello'}])
>>> import random
>>> udf_random_col = udf(lambda: int(100*random.random()), IntegerType()).asNondeterministic()
>>> df_br = df_br.withColumn('RAND', udf_random_col())
>>> random.seed(1234)
>>> udf_add_ten = udf(lambda rand: rand + 10, IntegerType())
>>> df_br.withColumn('RAND_PLUS_TEN', udf_add_ten('RAND')).show()
+-----+----+-------------+
| name|RAND|RAND_PLUS_TEN|
+-----+----+-------------+
|hello| 3| 13|
+-----+----+-------------+
```
Author: Marco Gaido <marcogaido91@gmail.com>
Author: Marco Gaido <mgaido@hortonworks.com>
Closes#19929 from mgaido91/SPARK-22629.
## What changes were proposed in this pull request?
In choosing a Partitioner to use for a cogroup-like operation between a number of RDDs, the default behaviour was if some of the RDDs already have a partitioner, we choose the one amongst them with the maximum number of partitions.
This behaviour, in some cases, could hit the 2G limit (SPARK-6235). To illustrate one such scenario, consider two RDDs:
rDD1: with smaller data and smaller number of partitions, alongwith a Partitioner.
rDD2: with much larger data and a larger number of partitions, without a Partitioner.
The cogroup of these two RDDs could hit the 2G limit, as a larger amount of data is shuffled into a smaller number of partitions.
This PR introduces a safety-check wherein the Partitioner is chosen only if either of the following conditions are met:
1. if the number of partitions of the RDD associated with the Partitioner is greater than or equal to the max number of upstream partitions; or
2. if the number of partitions of the RDD associated with the Partitioner is less than and within a single order of magnitude of the max number of upstream partitions.
## How was this patch tested?
Unit tests in PartitioningSuite and PairRDDFunctionsSuite
Author: sujithjay <sujith@logistimo.com>
Closes#20002 from sujithjay/SPARK-22465.
## What changes were proposed in this pull request?
register following classes in Kryo:
`org.apache.spark.mllib.regression.LabeledPoint`
`org.apache.spark.mllib.clustering.VectorWithNorm`
`org.apache.spark.ml.feature.LabeledPoint`
`org.apache.spark.ml.tree.impl.TreePoint`
`org.apache.spark.ml.tree.impl.BaggedPoint` seems also need to be registered, but I don't know how to do it in this safe way.
WeichenXu123 cloud-fan
## How was this patch tested?
added tests
Author: Zheng RuiFeng <ruifengz@foxmail.com>
Closes#19950 from zhengruifeng/labeled_kryo.
The code was ignoring SparkListenerLogStart, which was added
somewhat recently to record the Spark version used to generate
an event log.
Author: Marcelo Vanzin <vanzin@cloudera.com>
Closes#20049 from vanzin/SPARK-22854.
## What changes were proposed in this pull request?
In https://github.com/apache/spark/pull/19681 we introduced a new interface called `AppStatusPlugin`, to register listeners and set up the UI for both live and history UI.
However I think it's an overkill for live UI. For example, we should not register `SQLListener` if users are not using SQL functions. Previously we register the `SQLListener` and set up SQL tab when `SparkSession` is firstly created, which indicates users are going to use SQL functions. But in #19681 , we register the SQL functions during `SparkContext` creation. The same thing should apply to streaming too.
I think we should keep the previous behavior, and only use this new interface for history server.
To reflect this change, I also rename the new interface to `SparkHistoryUIPlugin`
This PR also refines the tests for sql listener.
## How was this patch tested?
existing tests
Author: Wenchen Fan <wenchen@databricks.com>
Closes#19981 from cloud-fan/listener.
## What changes were proposed in this pull request?
Upgrade Spark to Arrow 0.8.0 for Java and Python. Also includes an upgrade of Netty to 4.1.17 to resolve dependency requirements.
The highlights that pertain to Spark for the update from Arrow versoin 0.4.1 to 0.8.0 include:
* Java refactoring for more simple API
* Java reduced heap usage and streamlined hot code paths
* Type support for DecimalType, ArrayType
* Improved type casting support in Python
* Simplified type checking in Python
## How was this patch tested?
Existing tests
Author: Bryan Cutler <cutlerb@gmail.com>
Author: Shixiong Zhu <zsxwing@gmail.com>
Closes#19884 from BryanCutler/arrow-upgrade-080-SPARK-22324.
## What changes were proposed in this pull request?
In AppStatusListener's onStageSubmitted(event: SparkListenerStageSubmitted) method, there are duplicate code:
```
// schedulingPool was assigned twice with the same code
stage.schedulingPool = Option(event.properties).flatMap { p =>
Option(p.getProperty("spark.scheduler.pool"))
}.getOrElse(SparkUI.DEFAULT_POOL_NAME)
...
...
...
stage.schedulingPool = Option(event.properties).flatMap { p =>
Option(p.getProperty("spark.scheduler.pool"))
}.getOrElse(SparkUI.DEFAULT_POOL_NAME)
```
But, it does not make any sense to do this and there are no comment to explain for this.
## How was this patch tested?
N/A
Author: wuyi <ngone_5451@163.com>
Closes#20033 from Ngone51/dev-spark-22847.
## What changes were proposed in this pull request?
In the previous PR https://github.com/apache/spark/pull/5755#discussion_r157848354, we dropped `(-[classifier])` from the retrieval pattern. We should add it back; otherwise,
> If this pattern for instance doesn't has the [type] or [classifier] token, Ivy will download the source/javadoc artifacts to the same file as the regular jar.
## How was this patch tested?
The existing tests
Author: gatorsmile <gatorsmile@gmail.com>
Closes#20037 from gatorsmile/addClassifier.
## What changes were proposed in this pull request?
Currently, the task memory manager throws an OutofMemory error when there is an IO exception happens in spill() - https://github.com/apache/spark/blob/master/core/src/main/java/org/apache/spark/memory/TaskMemoryManager.java#L194. Similarly there any many other places in code when if a task is not able to acquire memory due to an exception we throw an OutofMemory error which kills the entire executor and hence failing all the tasks that are running on that executor instead of just failing one single task.
## How was this patch tested?
Unit tests
Author: Sital Kedia <skedia@fb.com>
Closes#20014 from sitalkedia/skedia/upstream_SPARK-22827.
## What changes were proposed in this pull request?
When calling explain on a query, the output can contain sensitive information. We should provide an admin/user to redact such information.
Before this PR, the plan of SS is like this
```
== Physical Plan ==
*HashAggregate(keys=[value#6], functions=[count(1)], output=[value#6, count(1)#12L])
+- StateStoreSave [value#6], state info [ checkpoint = file:/private/var/folders/vx/j0ydl5rn0gd9mgrh1pljnw900000gn/T/temporary-91c6fac0-609f-4bc8-ad57-52c189f06797/state, runId = 05a4b3af-f02c-40f8-9ff9-a3e18bae496f, opId = 0, ver = 0, numPartitions = 5], Complete, 0
+- *HashAggregate(keys=[value#6], functions=[merge_count(1)], output=[value#6, count#18L])
+- StateStoreRestore [value#6], state info [ checkpoint = file:/private/var/folders/vx/j0ydl5rn0gd9mgrh1pljnw900000gn/T/temporary-91c6fac0-609f-4bc8-ad57-52c189f06797/state, runId = 05a4b3af-f02c-40f8-9ff9-a3e18bae496f, opId = 0, ver = 0, numPartitions = 5]
+- *HashAggregate(keys=[value#6], functions=[merge_count(1)], output=[value#6, count#18L])
+- Exchange hashpartitioning(value#6, 5)
+- *HashAggregate(keys=[value#6], functions=[partial_count(1)], output=[value#6, count#18L])
+- *SerializeFromObject [staticinvoke(class org.apache.spark.unsafe.types.UTF8String, StringType, fromString, input[0, java.lang.String, true], true, false) AS value#6]
+- *MapElements <function1>, obj#5: java.lang.String
+- *DeserializeToObject value#30.toString, obj#4: java.lang.String
+- LocalTableScan [value#30]
```
After this PR, we can get the following output if users set `spark.redaction.string.regex` to `file:/[\\w_]+`
```
== Physical Plan ==
*HashAggregate(keys=[value#6], functions=[count(1)], output=[value#6, count(1)#12L])
+- StateStoreSave [value#6], state info [ checkpoint = *********(redacted)/var/folders/vx/j0ydl5rn0gd9mgrh1pljnw900000gn/T/temporary-e7da9b7d-3ec0-474d-8b8c-927f7d12ed72/state, runId = 8a9c3761-93d5-4896-ab82-14c06240dcea, opId = 0, ver = 0, numPartitions = 5], Complete, 0
+- *HashAggregate(keys=[value#6], functions=[merge_count(1)], output=[value#6, count#32L])
+- StateStoreRestore [value#6], state info [ checkpoint = *********(redacted)/var/folders/vx/j0ydl5rn0gd9mgrh1pljnw900000gn/T/temporary-e7da9b7d-3ec0-474d-8b8c-927f7d12ed72/state, runId = 8a9c3761-93d5-4896-ab82-14c06240dcea, opId = 0, ver = 0, numPartitions = 5]
+- *HashAggregate(keys=[value#6], functions=[merge_count(1)], output=[value#6, count#32L])
+- Exchange hashpartitioning(value#6, 5)
+- *HashAggregate(keys=[value#6], functions=[partial_count(1)], output=[value#6, count#32L])
+- *SerializeFromObject [staticinvoke(class org.apache.spark.unsafe.types.UTF8String, StringType, fromString, input[0, java.lang.String, true], true, false) AS value#6]
+- *MapElements <function1>, obj#5: java.lang.String
+- *DeserializeToObject value#27.toString, obj#4: java.lang.String
+- LocalTableScan [value#27]
```
## How was this patch tested?
Added a test case
Author: gatorsmile <gatorsmile@gmail.com>
Closes#19985 from gatorsmile/redactPlan.
This change restores the functionality that keeps a limited number of
different types (jobs, stages, etc) depending on configuration, to avoid
the store growing indefinitely over time.
The feature is implemented by creating a new type (ElementTrackingStore)
that wraps a KVStore and allows triggers to be set up for when elements
of a certain type meet a certain threshold. Triggers don't need to
necessarily only delete elements, but the current API is set up in a way
that makes that use case easier.
The new store also has a trigger for the "close" call, which makes it
easier for listeners to register code for cleaning things up and flushing
partial state to the store.
The old configurations for cleaning up the stored elements from the core
and SQL UIs are now active again, and the old unit tests are re-enabled.
Author: Marcelo Vanzin <vanzin@cloudera.com>
Closes#19751 from vanzin/SPARK-20653.
## What changes were proposed in this pull request?
Changes discussed in https://github.com/apache/spark/pull/19946#discussion_r157063535
docker -> container, since with CRI, we are not limited to running only docker images.
## How was this patch tested?
Manual testing
Author: foxish <ramanathana@google.com>
Closes#19995 from foxish/make-docker-container.
## What changes were proposed in this pull request?
This PR added the missing service metadata for `KubernetesClusterManager`. Without the metadata, the service loader couldn't load `KubernetesClusterManager`, and caused the driver to fail to create a `ExternalClusterManager`, as being reported in SPARK-22778. The PR also changed the `k8s:` prefix used to `k8s://`, which is what existing Spark on k8s users are familiar and used to.
## How was this patch tested?
Manual testing verified that the fix resolved the issue in SPARK-22778.
/cc vanzin felixcheung jiangxb1987
Author: Yinan Li <liyinan926@gmail.com>
Closes#19972 from liyinan926/fix-22778.
## What changes were proposed in this pull request?
Missing some changes about limit in TaskSetManager.scala
## How was this patch tested?
running tests
Please review http://spark.apache.org/contributing.html before opening a pull request.
Author: kellyzly <kellyzly@126.com>
Closes#19976 from kellyzly/SPARK-22660.2.
SQLConf allows some callers to define a custom default value for
configs, and that complicates a little bit the handling of fallback
config entries, since most of the default value resolution is
hidden by the config code.
This change peaks into the internals of these fallback configs
to figure out the correct default value, and also returns the
current human-readable default when showing the default value
(e.g. through "set -v").
Author: Marcelo Vanzin <vanzin@cloudera.com>
Closes#19974 from vanzin/SPARK-22779.
Use a semaphore to synchronize the tasks with the listener code
that is trying to cancel the job or stage, so that the listener
won't try to cancel a job or stage that has already finished.
Author: Marcelo Vanzin <vanzin@cloudera.com>
Closes#19956 from vanzin/SPARK-22764.
## What changes were proposed in this pull request?
PR closed with all the comments -> https://github.com/apache/spark/pull/19793
It solves the problem when submitting a wrong CreateSubmissionRequest to Spark Dispatcher was causing a bad state of Dispatcher and making it inactive as a Mesos framework.
https://issues.apache.org/jira/browse/SPARK-22574
## How was this patch tested?
All spark test passed successfully.
It was tested sending a wrong request (without appArgs) before and after the change. The point is easy, check if the value is null before being accessed.
This was before the change, leaving the dispatcher inactive:
```
Exception in thread "Thread-22" java.lang.NullPointerException
at org.apache.spark.scheduler.cluster.mesos.MesosClusterScheduler.getDriverCommandValue(MesosClusterScheduler.scala:444)
at org.apache.spark.scheduler.cluster.mesos.MesosClusterScheduler.buildDriverCommand(MesosClusterScheduler.scala:451)
at org.apache.spark.scheduler.cluster.mesos.MesosClusterScheduler.org$apache$spark$scheduler$cluster$mesos$MesosClusterScheduler$$createTaskInfo(MesosClusterScheduler.scala:538)
at org.apache.spark.scheduler.cluster.mesos.MesosClusterScheduler$$anonfun$scheduleTasks$1.apply(MesosClusterScheduler.scala:570)
at org.apache.spark.scheduler.cluster.mesos.MesosClusterScheduler$$anonfun$scheduleTasks$1.apply(MesosClusterScheduler.scala:555)
at scala.collection.mutable.ResizableArray$class.foreach(ResizableArray.scala:59)
at scala.collection.mutable.ArrayBuffer.foreach(ArrayBuffer.scala:48)
at org.apache.spark.scheduler.cluster.mesos.MesosClusterScheduler.scheduleTasks(MesosClusterScheduler.scala:555)
at org.apache.spark.scheduler.cluster.mesos.MesosClusterScheduler.resourceOffers(MesosClusterScheduler.scala:621)
```
And after:
```
"message" : "Malformed request: org.apache.spark.deploy.rest.SubmitRestProtocolException: Validation of message CreateSubmissionRequest failed!\n\torg.apache.spark.deploy.rest.SubmitRestProtocolMessage.validate(SubmitRestProtocolMessage.scala:70)\n\torg.apache.spark.deploy.rest.SubmitRequestServlet.doPost(RestSubmissionServer.scala:272)\n\tjavax.servlet.http.HttpServlet.service(HttpServlet.java:707)\n\tjavax.servlet.http.HttpServlet.service(HttpServlet.java:790)\n\torg.spark_project.jetty.servlet.ServletHolder.handle(ServletHolder.java:845)\n\torg.spark_project.jetty.servlet.ServletHandler.doHandle(ServletHandler.java:583)\n\torg.spark_project.jetty.server.handler.ContextHandler.doHandle(ContextHandler.java:1180)\n\torg.spark_project.jetty.servlet.ServletHandler.doScope(ServletHandler.java:511)\n\torg.spark_project.jetty.server.handler.ContextHandler.doScope(ContextHandler.java:1112)\n\torg.spark_project.jetty.server.handler.ScopedHandler.handle(ScopedHandler.java:141)\n\torg.spark_project.jetty.server.handler.HandlerWrapper.handle(HandlerWrapper.java:134)\n\torg.spark_project.jetty.server.Server.handle(Server.java:524)\n\torg.spark_project.jetty.server.HttpChannel.handle(HttpChannel.java:319)\n\torg.spark_project.jetty.server.HttpConnection.onFillable(HttpConnection.java:253)\n\torg.spark_project.jetty.io.AbstractConnection$ReadCallback.succeeded(AbstractConnection.java:273)\n\torg.spark_project.jetty.io.FillInterest.fillable(FillInterest.java:95)\n\torg.spark_project.jetty.io.SelectChannelEndPoint$2.run(SelectChannelEndPoint.java:93)\n\torg.spark_project.jetty.util.thread.strategy.ExecuteProduceConsume.executeProduceConsume(ExecuteProduceConsume.java:303)\n\torg.spark_project.jetty.util.thread.strategy.ExecuteProduceConsume.produceConsume(ExecuteProduceConsume.java:148)\n\torg.spark_project.jetty.util.thread.strategy.ExecuteProduceConsume.run(ExecuteProduceConsume.java:136)\n\torg.spark_project.jetty.util.thread.QueuedThreadPool.runJob(QueuedThreadPool.java:671)\n\torg.spark_project.jetty.util.thread.QueuedThreadPool$2.run(QueuedThreadPool.java:589)\n\tjava.lang.Thread.run(Thread.java:745)"
```
Author: German Schiavon <germanschiavon@gmail.com>
Closes#19966 from Gschiavon/fix-submission-request.
## What changes were proposed in this pull request?
While spark code changes, there are new events in event log: #19649
And we used to maintain a whitelist to avoid exceptions: #15663
Currently Spark history server will stop parsing on unknown events or unrecognized properties. We may still see part of the UI data.
For better compatibility, we can ignore unknown events and parse through the log file.
## How was this patch tested?
Unit test
Author: Wang Gengliang <ltnwgl@gmail.com>
Closes#19953 from gengliangwang/ReplayListenerBus.
… than spark.network.timeout or not
## What changes were proposed in this pull request?
If spark.executor.heartbeatInterval bigger than spark.network.timeout,it will almost always cause exception below.
`Job aborted due to stage failure: Task 4763 in stage 3.0 failed 4 times, most recent failure: Lost task 4763.3 in stage 3.0 (TID 22383, executor id: 4761, host: xxx): ExecutorLostFailure (executor 4761 exited caused by one of the running tasks) Reason: Executor heartbeat timed out after 154022 ms`
Since many users do not get that point.He will set spark.executor.heartbeatInterval incorrectly.
This patch check this case when submit applications.
## How was this patch tested?
Test in cluster
Author: zhoukang <zhoukang199191@gmail.com>
Closes#19942 from caneGuy/zhoukang/check-heartbeat.
## What changes were proposed in this pull request?
It solves the problem when submitting a wrong CreateSubmissionRequest to Spark Dispatcher was causing a bad state of Dispatcher and making it inactive as a Mesos framework.
https://issues.apache.org/jira/browse/SPARK-22574
## How was this patch tested?
All spark test passed successfully.
It was tested sending a wrong request (without appArgs) before and after the change. The point is easy, check if the value is null before being accessed.
This was before the change, leaving the dispatcher inactive:
```
Exception in thread "Thread-22" java.lang.NullPointerException
at org.apache.spark.scheduler.cluster.mesos.MesosClusterScheduler.getDriverCommandValue(MesosClusterScheduler.scala:444)
at org.apache.spark.scheduler.cluster.mesos.MesosClusterScheduler.buildDriverCommand(MesosClusterScheduler.scala:451)
at org.apache.spark.scheduler.cluster.mesos.MesosClusterScheduler.org$apache$spark$scheduler$cluster$mesos$MesosClusterScheduler$$createTaskInfo(MesosClusterScheduler.scala:538)
at org.apache.spark.scheduler.cluster.mesos.MesosClusterScheduler$$anonfun$scheduleTasks$1.apply(MesosClusterScheduler.scala:570)
at org.apache.spark.scheduler.cluster.mesos.MesosClusterScheduler$$anonfun$scheduleTasks$1.apply(MesosClusterScheduler.scala:555)
at scala.collection.mutable.ResizableArray$class.foreach(ResizableArray.scala:59)
at scala.collection.mutable.ArrayBuffer.foreach(ArrayBuffer.scala:48)
at org.apache.spark.scheduler.cluster.mesos.MesosClusterScheduler.scheduleTasks(MesosClusterScheduler.scala:555)
at org.apache.spark.scheduler.cluster.mesos.MesosClusterScheduler.resourceOffers(MesosClusterScheduler.scala:621)
```
And after:
```
"message" : "Malformed request: org.apache.spark.deploy.rest.SubmitRestProtocolException: Validation of message CreateSubmissionRequest failed!\n\torg.apache.spark.deploy.rest.SubmitRestProtocolMessage.validate(SubmitRestProtocolMessage.scala:70)\n\torg.apache.spark.deploy.rest.SubmitRequestServlet.doPost(RestSubmissionServer.scala:272)\n\tjavax.servlet.http.HttpServlet.service(HttpServlet.java:707)\n\tjavax.servlet.http.HttpServlet.service(HttpServlet.java:790)\n\torg.spark_project.jetty.servlet.ServletHolder.handle(ServletHolder.java:845)\n\torg.spark_project.jetty.servlet.ServletHandler.doHandle(ServletHandler.java:583)\n\torg.spark_project.jetty.server.handler.ContextHandler.doHandle(ContextHandler.java:1180)\n\torg.spark_project.jetty.servlet.ServletHandler.doScope(ServletHandler.java:511)\n\torg.spark_project.jetty.server.handler.ContextHandler.doScope(ContextHandler.java:1112)\n\torg.spark_project.jetty.server.handler.ScopedHandler.handle(ScopedHandler.java:141)\n\torg.spark_project.jetty.server.handler.HandlerWrapper.handle(HandlerWrapper.java:134)\n\torg.spark_project.jetty.server.Server.handle(Server.java:524)\n\torg.spark_project.jetty.server.HttpChannel.handle(HttpChannel.java:319)\n\torg.spark_project.jetty.server.HttpConnection.onFillable(HttpConnection.java:253)\n\torg.spark_project.jetty.io.AbstractConnection$ReadCallback.succeeded(AbstractConnection.java:273)\n\torg.spark_project.jetty.io.FillInterest.fillable(FillInterest.java:95)\n\torg.spark_project.jetty.io.SelectChannelEndPoint$2.run(SelectChannelEndPoint.java:93)\n\torg.spark_project.jetty.util.thread.strategy.ExecuteProduceConsume.executeProduceConsume(ExecuteProduceConsume.java:303)\n\torg.spark_project.jetty.util.thread.strategy.ExecuteProduceConsume.produceConsume(ExecuteProduceConsume.java:148)\n\torg.spark_project.jetty.util.thread.strategy.ExecuteProduceConsume.run(ExecuteProduceConsume.java:136)\n\torg.spark_project.jetty.util.thread.QueuedThreadPool.runJob(QueuedThreadPool.java:671)\n\torg.spark_project.jetty.util.thread.QueuedThreadPool$2.run(QueuedThreadPool.java:589)\n\tjava.lang.Thread.run(Thread.java:745)"
```
Author: German Schiavon <germanschiavon@gmail.com>
Closes#19793 from Gschiavon/fix-submission-request.
## What changes were proposed in this pull request?
This PR proposes to print warnings before creating local by `java.io.File`.
I think we can't just simply disallow and throw an exception for such cases of `hdfs:/tmp/foo` case because it might break compatibility. Note that `hdfs:/tmp/foo` creates a directory called `hdfs:/`.
There were many discussion here about whether we should support this in other file systems or not; however, since the JIRA targets "Spark's local dir should accept only local paths", here I tried to resolve it by simply printing warnings.
I think we could open another JIRA and design doc if this is something we should support, separately. Another note, for your information, [SPARK-1529](https://issues.apache.org/jira/browse/SPARK-1529) is resolved as `Won't Fix`.
**Before**
```
./bin/spark-shell --conf spark.local.dir=file:/a/b/c
```
This creates a local directory as below:
```
file:/
└── a
└── b
└── c
...
```
**After**
```bash
./bin/spark-shell --conf spark.local.dir=file:/a/b/c
```
Now, it prints a warning as below:
```
...
17/12/09 21:58:49 WARN Utils: The configured local directories are not expected to be URIs; however, got suspicious values [file:/a/b/c]. Please check your configured local directories.
...
```
```bash
./bin/spark-shell --conf spark.local.dir=file:/a/b/c,/tmp/a/b/c,hdfs:/a/b/c
```
It also works with comma-separated ones:
```
...
17/12/09 22:05:01 WARN Utils: The configured local directories are not expected to be URIs; however, got suspicious values [file:/a/b/c, hdfs:/a/b/c]. Please check your configured local directories.
...
```
## How was this patch tested?
Manually tested:
```
./bin/spark-shell --conf spark.local.dir=C:\\a\\b\\c
./bin/spark-shell --conf spark.local.dir=/tmp/a/b/c
./bin/spark-shell --conf spark.local.dir=a/b/c
./bin/spark-shell --conf spark.local.dir=a/b/c,/tmp/a/b/c,C:\\a\\b\\c
```
Author: hyukjinkwon <gurwls223@gmail.com>
Closes#19934 from HyukjinKwon/SPARK-3685.
This PR contains implementation of the basic submission client for the cluster mode of Spark on Kubernetes. It's step 2 from the step-wise plan documented [here](https://github.com/apache-spark-on-k8s/spark/issues/441#issuecomment-330802935).
This addition is covered by the [SPIP](http://apache-spark-developers-list.1001551.n3.nabble.com/SPIP-Spark-on-Kubernetes-td22147.html) vote which passed on Aug 31.
This PR and #19468 together form a MVP of Spark on Kubernetes that allows users to run Spark applications that use resources locally within the driver and executor containers on Kubernetes 1.6 and up. Some changes on pom and build/test setup are copied over from #19468 to make this PR self contained and testable.
The submission client is mainly responsible for creating the Kubernetes pod that runs the Spark driver. It follows a step-based approach to construct the driver pod, as the code under the `submit.steps` package shows. The steps are orchestrated by `DriverConfigurationStepsOrchestrator`. `Client` creates the driver pod and waits for the application to complete if it's configured to do so, which is the case by default.
This PR also contains Dockerfiles of the driver and executor images. They are included because some of the environment variables set in the code would not make sense without referring to the Dockerfiles.
* The patch contains unit tests which are passing.
* Manual testing: ./build/mvn -Pkubernetes clean package succeeded.
* It is a subset of the entire changelist hosted at http://github.com/apache-spark-on-k8s/spark which is in active use in several organizations.
* There is integration testing enabled in the fork currently hosted by PepperData which is being moved over to RiseLAB CI.
* Detailed documentation on trying out the patch in its entirety is in: https://apache-spark-on-k8s.github.io/userdocs/running-on-kubernetes.html
cc rxin felixcheung mateiz (shepherd)
k8s-big-data SIG members & contributors: mccheah foxish ash211 ssuchter varunkatta kimoonkim erikerlandson tnachen ifilonenko liyinan926
Author: Yinan Li <liyinan926@gmail.com>
Closes#19717 from liyinan926/spark-kubernetes-4.
…structures
## What changes were proposed in this pull request?
In general, the SHS pages now use the public API types to represent applications. Some internal code paths still used its own view of what applications and attempts look like (`ApplicationHistoryInfo` and `ApplicationAttemptInfo`), declared in ApplicationHistoryProvider.scala.
This pull request removes these classes and updates the rest of the code to use `status.api.v1.ApplicationInfo` and `status.api.v1.ApplicationAttemptInfo` instead.
Furthermore `status.api.v1.ApplicationInfo` and `status.api.v1.ApplicationAttemptInfo` were changed to case class to
- facilitate copying instances
- equality checking in test code
- nicer toString()
To simplify the code a bit `v1.` prefixes were also removed from occurrences of v1.ApplicationInfo and v1.ApplicationAttemptInfo as there is no more ambiguity between classes in history and status.api.v1.
## How was this patch tested?
By running existing automated tests.
Author: Sandor Murakozi <smurakozi@gmail.com>
Closes#19920 from smurakozi/SPARK-21672.
## What changes were proposed in this pull request?
During Spark shutdown, if there are some active tasks, sometimes they will complete with incorrect results. The issue is in PythonRunner where it is returning partial result instead of throwing exception during Spark shutdown.
This patch makes it so that these tasks fail instead of complete with partial results.
## How was this patch tested?
Existing tests.
Author: Li Jin <ice.xelloss@gmail.com>
Closes#19852 from icexelloss/python-runner-shutdown.
## What changes were proposed in this pull request?
Follow-up to earlier commit.
The peak memory of BytesToBytesMap is not updated in more places - spill() and destructiveIterator().
## How was this patch tested?
Manually.
Author: Juliusz Sompolski <julek@databricks.com>
Closes#19923 from juliuszsompolski/SPARK-22721cd.
…a-2.12 and JDK9
## What changes were proposed in this pull request?
Some compile error after upgrading to scala-2.12
```javascript
spark_source/core/src/main/scala/org/apache/spark/executor/Executor.scala:455: ambiguous reference to overloaded definition, method limit in class ByteBuffer of type (x$1: Int)java.nio.ByteBuffer
method limit in class Buffer of type ()Int
match expected type ?
val resultSize = serializedDirectResult.limit
error
```
The limit method was moved from ByteBuffer to the superclass Buffer and it can no longer be called without (). The same reason for position method.
```javascript
/home/zly/prj/oss/jdk9_HOS_SOURCE/spark_source/sql/hive/src/main/scala/org/apache/spark/sql/hive/execution/ScriptTransformationExec.scala:427: ambiguous reference to overloaded definition, [error] both method putAll in class Properties of type (x$1: java.util.Map[_, _])Unit [error] and method putAll in class Hashtable of type (x$1: java.util.Map[_ <: Object, _ <: Object])Unit [error] match argument types (java.util.Map[String,String])
[error] props.putAll(outputSerdeProps.toMap.asJava)
[error] ^
```
This is because the key type is Object instead of String which is unsafe.
## How was this patch tested?
running tests
Please review http://spark.apache.org/contributing.html before opening a pull request.
Author: kellyzly <kellyzly@126.com>
Closes#19854 from kellyzly/SPARK-22660.
## What changes were proposed in this pull request?
I propose that BlockManagerMasterEndpoint.removeRdd() should catch and log any IOExceptions it receives. As it is now, the exception can bubble up to the main thread and kill user applications when called from RDD.unpersist(). I think this change is a better experience for the end user.
I chose to catch the exception in BlockManagerMasterEndpoint.removeRdd() instead of RDD.unpersist() because this way the RDD.unpersist() blocking option will still work correctly. Otherwise, blocking will get short circuited by the first error.
## How was this patch tested?
This patch was tested with a job that shows the job killing behavior mentioned above.
rxin, it looks like you originally wrote this method, I would appreciate it if you took a look. Thanks.
This contribution is my original work and is licensed under the project's open source license.
Author: Brad Kaiser <kaiserb@us.ibm.com>
Closes#19836 from brad-kaiser/catch-unpersist-exception.
## What changes were proposed in this pull request?
BytesToBytesMap doesn't update peak memory usage before shrinking back to initial capacity in reset(), so after a disk spill one never knows what was the size of hash table was before spilling.
## How was this patch tested?
Checked manually.
Author: Juliusz Sompolski <julek@databricks.com>
Closes#19915 from juliuszsompolski/SPARK-22721.
## What changes were proposed in this pull request?
I see the two instances where the exception is occurring.
**Instance 1:**
```
17/11/10 15:49:32 ERROR util.Utils: Uncaught exception in thread driver-revive-thread
org.apache.spark.SparkException: Could not find CoarseGrainedScheduler.
at org.apache.spark.rpc.netty.Dispatcher.postMessage(Dispatcher.scala:160)
at org.apache.spark.rpc.netty.Dispatcher.postOneWayMessage(Dispatcher.scala:140)
at org.apache.spark.rpc.netty.NettyRpcEnv.send(NettyRpcEnv.scala:187)
at org.apache.spark.rpc.netty.NettyRpcEndpointRef.send(NettyRpcEnv.scala:521)
at org.apache.spark.scheduler.cluster.CoarseGrainedSchedulerBackend$DriverEndpoint$$anon$1$$anonfun$run$1$$anonfun$apply$mcV$sp$1.apply(CoarseGrainedSchedulerBackend.scala:125)
at org.apache.spark.scheduler.cluster.CoarseGrainedSchedulerBackend$DriverEndpoint$$anon$1$$anonfun$run$1$$anonfun$apply$mcV$sp$1.apply(CoarseGrainedSchedulerBackend.scala:125)
at scala.Option.foreach(Option.scala:257)
at org.apache.spark.scheduler.cluster.CoarseGrainedSchedulerBackend$DriverEndpoint$$anon$1$$anonfun$run$1.apply$mcV$sp(CoarseGrainedSchedulerBackend.scala:125)
at org.apache.spark.util.Utils$.tryLogNonFatalError(Utils.scala:1344)
at org.apache.spark.scheduler.cluster.CoarseGrainedSchedulerBackend$DriverEndpoint$$anon$1.run(CoarseGrainedSchedulerBackend.scala:124)
at java.util.concurrent.Executors$RunnableAdapter.call(Executors.java:511)
at java.util.concurrent.FutureTask.runAndReset(FutureTask.java:308)
at java.util.concurrent.ScheduledThreadPoolExecutor$ScheduledFutureTask.access$301(ScheduledThreadPoolExecutor.java:180)
at java.util.concurrent.ScheduledThreadPoolExecutor$ScheduledFutureTask.run(ScheduledThreadPoolExecutor.java:294)
at java.util.concurrent.ThreadPoolExecutor.runWorker(ThreadPoolExecutor.java:1142)
at java.util.concurrent.ThreadPoolExecutor$Worker.run(ThreadPoolExecutor.java:617)
at java.lang.Thread.run(Thread.java:745)
```
In CoarseGrainedSchedulerBackend.scala, driver-revive-thread starts with DriverEndpoint.onStart() and keeps sending the ReviveOffers messages periodically till it gets shutdown as part DriverEndpoint.onStop(). There is no proper coordination between the driver-revive-thread(shutdown) and the RpcEndpoint unregister, RpcEndpoint unregister happens first and then driver-revive-thread shuts down as part of DriverEndpoint.onStop(), In-between driver-revive-thread may try to send the ReviveOffers message which is leading to the above exception.
To fix this issue, this PR moves the shutting down of driver-revive-thread to CoarseGrainedSchedulerBackend.stop() which executes before the DriverEndpoint unregister.
**Instance 2:**
```
17/11/10 16:31:38 ERROR cluster.YarnSchedulerBackend$YarnSchedulerEndpoint: Error requesting driver to remove executor 1 for reason Executor for container container_1508535467865_0226_01_000002 exited because of a YARN event (e.g., pre-emption) and not because of an error in the running job.
org.apache.spark.SparkException: Could not find CoarseGrainedScheduler.
at org.apache.spark.rpc.netty.Dispatcher.postMessage(Dispatcher.scala:160)
at org.apache.spark.rpc.netty.Dispatcher.postLocalMessage(Dispatcher.scala:135)
at org.apache.spark.rpc.netty.NettyRpcEnv.ask(NettyRpcEnv.scala:229)
at org.apache.spark.rpc.netty.NettyRpcEndpointRef.ask(NettyRpcEnv.scala:516)
at org.apache.spark.rpc.RpcEndpointRef.ask(RpcEndpointRef.scala:63)
at org.apache.spark.scheduler.cluster.YarnSchedulerBackend$YarnSchedulerEndpoint$$anonfun$receive$1.applyOrElse(YarnSchedulerBackend.scala:269)
at org.apache.spark.rpc.netty.Inbox$$anonfun$process$1.apply$mcV$sp(Inbox.scala:117)
at org.apache.spark.rpc.netty.Inbox.safelyCall(Inbox.scala:205)
at org.apache.spark.rpc.netty.Inbox.process(Inbox.scala:101)
at org.apache.spark.rpc.netty.Dispatcher$MessageLoop.run(Dispatcher.scala:221)
at java.util.concurrent.ThreadPoolExecutor.runWorker(ThreadPoolExecutor.java:1142)
at java.util.concurrent.ThreadPoolExecutor$Worker.run(ThreadPoolExecutor.java:617)
at java.lang.Thread.run(Thread.java:745)
```
Here YarnDriverEndpoint tries to send remove executor messages after the Yarn scheduler backend service stop, which is leading to the above exception. To avoid the above exception,
1) We may add a condition(which checks whether service has stopped or not) before sending executor remove message
2) Add a warn log message in onFailure case when the service is already stopped
In this PR, chosen the 2) option which adds a log message in the case of onFailure without the exception stack trace since the option 1) would need to to go through for every remove executor message.
## How was this patch tested?
I verified it manually, I don't see these exceptions with the PR changes.
Author: Devaraj K <devaraj@apache.org>
Closes#19741 from devaraj-kavali/SPARK-14228.
## What changes were proposed in this pull request?
I was looking at the config code today and found that configs defined using ConfigBuilder.fallbackConf didn't trigger onCreate function. This patch fixes it.
This doesn't require backporting since we currently have no configs that use it.
## How was this patch tested?
Added a test case for all the config final creator functions in ConfigEntrySuite.
Author: Reynold Xin <rxin@databricks.com>
Closes#19905 from rxin/SPARK-22710.
## What changes were proposed in this pull request?
As the doc says "For accumulator updates performed inside actions only, Spark guarantees that each task’s update to the accumulator will only be applied once, i.e. restarted tasks will not update the value."
But currently the code doesn't guarantee this.
## How was this patch tested?
New added tests.
Author: Carson Wang <carson.wang@intel.com>
Closes#19877 from carsonwang/fixAccum.
The main goal of this change is to allow multiple cluster-mode
submissions from the same JVM, without having them end up with
mixed configuration. That is done by extending the SparkApplication
trait, and doing so was reasonably trivial for standalone and
mesos modes.
For YARN mode, there was a complication. YARN used a "SPARK_YARN_MODE"
system property to control behavior indirectly in a whole bunch of
places, mainly in the SparkHadoopUtil / YarnSparkHadoopUtil classes.
Most of the changes here are removing that.
Since we removed support for Hadoop 1.x, some methods that lived in
YarnSparkHadoopUtil can now live in SparkHadoopUtil. The remaining
methods don't need to be part of the class, and can be called directly
from the YarnSparkHadoopUtil object, so now there's a single
implementation of SparkHadoopUtil.
There were two places in the code that relied on SPARK_YARN_MODE to
make decisions about YARN-specific functionality, and now explicitly check
the master from the configuration for that instead:
* fetching the external shuffle service port, which can come from the YARN
configuration.
* propagation of the authentication secret using Hadoop credentials. This also
was cleaned up a little to not need so many methods in `SparkHadoopUtil`.
With those out of the way, actually changing the YARN client
to extend SparkApplication was easy.
Tested with existing unit tests, and also by running YARN apps
with auth and kerberos both on and off in a real cluster.
Author: Marcelo Vanzin <vanzin@cloudera.com>
Closes#19631 from vanzin/SPARK-22372.
I have modified SparkHadoopWriter so that executors and the driver always use consistent JobIds during the hadoop commit. Before SPARK-18191, spark always used the rddId, it just incorrectly named the variable stageId. After SPARK-18191, it used the rddId as the jobId on the driver's side, and the stageId as the jobId on the executors' side. With this change executors and the driver will consistently uses rddId as the jobId. Also with this change, during the hadoop commit protocol spark uses actual stageId to check whether a stage can be committed unlike before that it was using executors' jobId to do this check.
In addition to the existing unit tests, a test has been added to check whether executors and the driver are using the same JobId. The test failed before this change and passed after applying this fix.
Author: Reza Safi <rezasafi@cloudera.com>
Closes#19848 from rezasafi/stagerddsimple.
## What changes were proposed in this pull request?
Use a separate Spark event queue for StreamingQueryListenerBus so that if there are many non-streaming events, streaming query listeners don't need to wait for other Spark listeners and can catch up.
## How was this patch tested?
Jenkins
Author: Shixiong Zhu <zsxwing@gmail.com>
Closes#19838 from zsxwing/SPARK-22638.
https://issues.apache.org/jira/browse/SPARK-22653
executorRef.address can be null, pass the executorAddress which accounts for it being null a few lines above the fix.
Manually tested this patch. You can reproduce the issue by running a simple spark-shell in yarn client mode with dynamic allocation and request some executors up front. Let those executors idle timeout. Get a heap dump. Without this fix, you will see that addressToExecutorId still contains the ids, with the fix addressToExecutorId is properly cleaned up.
Author: Thomas Graves <tgraves@oath.com>
Closes#19850 from tgravescs/SPARK-22653.
## What changes were proposed in this pull request?
This updates a behavior of `addJar` method of `sparkContext` class. If path without any scheme is passed as input it is used literally without url encoding/decoding it.
## How was this patch tested?
A unit test is added for this.
Author: Jakub Dubovsky <jakub.dubovsky@seznam.cz>
Closes#19834 from james64/SPARK-22585-encode-add-jar.
The only remaining use of this class was the SparkStatusTracker, which
was modified to use the new status store. The test code to wait for
executors was moved to TestUtils and now uses the SparkStatusTracker API.
Indirectly, ConsoleProgressBar also uses this data. Because it has
some lower latency requirements, a shortcut to efficiently get the
active stages from the active listener was added to the AppStateStore.
Now that all UI code goes through the status store to get its data,
the FsHistoryProvider can be cleaned up to only replay event logs
when needed - that is, when there is no pre-existing disk store for
the application.
As part of this change I also modified the streaming UI to read the needed
data from the store, which was missed in the previous patch that made
JobProgressListener redundant.
Author: Marcelo Vanzin <vanzin@cloudera.com>
Closes#19750 from vanzin/SPARK-20650.
## What changes were proposed in this pull request?
This is a stripped down version of the `KubernetesClusterSchedulerBackend` for Spark with the following components:
- Static Allocation of Executors
- Executor Pod Factory
- Executor Recovery Semantics
It's step 1 from the step-wise plan documented [here](https://github.com/apache-spark-on-k8s/spark/issues/441#issuecomment-330802935).
This addition is covered by the [SPIP vote](http://apache-spark-developers-list.1001551.n3.nabble.com/SPIP-Spark-on-Kubernetes-td22147.html) which passed on Aug 31 .
## How was this patch tested?
- The patch contains unit tests which are passing.
- Manual testing: `./build/mvn -Pkubernetes clean package` succeeded.
- It is a **subset** of the entire changelist hosted in http://github.com/apache-spark-on-k8s/spark which is in active use in several organizations.
- There is integration testing enabled in the fork currently [hosted by PepperData](spark-k8s-jenkins.pepperdata.org:8080) which is being moved over to RiseLAB CI.
- Detailed documentation on trying out the patch in its entirety is in: https://apache-spark-on-k8s.github.io/userdocs/running-on-kubernetes.html
cc rxin felixcheung mateiz (shepherd)
k8s-big-data SIG members & contributors: mccheah ash211 ssuchter varunkatta kimoonkim erikerlandson liyinan926 tnachen ifilonenko
Author: Yinan Li <liyinan926@gmail.com>
Author: foxish <ramanathana@google.com>
Author: mcheah <mcheah@palantir.com>
Closes#19468 from foxish/spark-kubernetes-3.
## What changes were proposed in this pull request?
In adaptive execution, the map output statistics of all mappers will be aggregated after previous stage is successfully executed. Driver takes the aggregation job while it will get slow when the number of `mapper * shuffle partitions` is large, since it only uses single thread to compute. This PR uses multi-thread to deal with this single point bottleneck.
## How was this patch tested?
Test cases are in `MapOutputTrackerSuite.scala`
Author: GuoChenzhao <chenzhao.guo@intel.com>
Author: gczsjdy <gczsjdy1994@gmail.com>
Closes#19763 from gczsjdy/single_point_mapstatistics.
## What changes were proposed in this pull request?
Currently history server v2 failed to start if `listing.ldb` is corrupted.
This patch get rid of the corrupted `listing.ldb` and re-create it.
The exception handling follows [opening disk store for app](0ffa7c488f/core/src/main/scala/org/apache/spark/deploy/history/FsHistoryProvider.scala (L307))
## How was this patch tested?
manual test
Please review http://spark.apache.org/contributing.html before opening a pull request.
Author: Wang Gengliang <ltnwgl@gmail.com>
Closes#19786 from gengliangwang/listingException.
This change hooks up the config reader to `SparkConf.getDeprecatedConfig`,
so that config constants with deprecated names generate the proper warnings.
It also changes two deprecated configs from the new "alternatives" system to
the old deprecation system, since they're not yet hooked up to each other.
Added a few unit tests to verify the desired behavior.
Author: Marcelo Vanzin <vanzin@cloudera.com>
Closes#19760 from vanzin/SPARK-22533.
This PR enables to use ``OffHeapColumnVector`` when ``spark.sql.columnVector.offheap.enable`` is set to ``true``. While ``ColumnVector`` has two implementations ``OnHeapColumnVector`` and ``OffHeapColumnVector``, only ``OnHeapColumnVector`` is always used.
This PR implements the followings
- Pass ``OffHeapColumnVector`` to ``ColumnarBatch.allocate()`` when ``spark.sql.columnVector.offheap.enable`` is set to ``true``
- Free all of off-heap memory regions by ``OffHeapColumnVector.close()``
- Ensure to call ``OffHeapColumnVector.close()``
Use existing tests
Author: Kazuaki Ishizaki <ishizaki@jp.ibm.com>
Closes#17436 from kiszk/SPARK-20101.
## What changes were proposed in this pull request?
This PR proposes to add a flag to control if PySpark should use daemon or not.
Actually, SparkR already has a flag for useDaemon:
478fbc866f/core/src/main/scala/org/apache/spark/api/r/RRunner.scala (L362)
It'd be great if we have this flag too. It makes easier to debug Windows specific issue.
## How was this patch tested?
Manually tested.
Author: hyukjinkwon <gurwls223@gmail.com>
Closes#19782 from HyukjinKwon/use-daemon-flag.
## What changes were proposed in this pull request?
ScalaTest 3.0 uses an implicit `Signaler`. This PR makes it sure all Spark tests uses `ThreadSignaler` explicitly which has the same default behavior of interrupting a thread on the JVM like ScalaTest 2.2.x. This will reduce potential flakiness.
## How was this patch tested?
This is testsuite-only update. This should passes the Jenkins tests.
Author: Dongjoon Hyun <dongjoon@apache.org>
Closes#19784 from dongjoon-hyun/use_thread_signaler.
## What changes were proposed in this pull request?
* Add a "function type" argument to pandas_udf.
* Add a new public enum class `PandasUdfType` in pyspark.sql.functions
* Refactor udf related code from pyspark.sql.functions to pyspark.sql.udf
* Merge "PythonUdfType" and "PythonEvalType" into a single enum class "PythonEvalType"
Example:
```
from pyspark.sql.functions import pandas_udf, PandasUDFType
pandas_udf('double', PandasUDFType.SCALAR):
def plus_one(v):
return v + 1
```
## Design doc
https://docs.google.com/document/d/1KlLaa-xJ3oz28xlEJqXyCAHU3dwFYkFs_ixcUXrJNTc/edit
## How was this patch tested?
Added PandasUDFTests
## TODO:
* [x] Implement proper enum type for `PandasUDFType`
* [x] Update documentation
* [x] Add more tests in PandasUDFTests
Author: Li Jin <ice.xelloss@gmail.com>
Closes#19630 from icexelloss/spark-22409-pandas-udf-type.
## What changes were proposed in this pull request?
Ensure HighlyCompressedMapStatus calculates correct avgSize
## How was this patch tested?
New unit test added.
Author: yucai <yucai.yu@intel.com>
Closes#19765 from yucai/avgsize.
## What changes were proposed in this pull request?
`PythonRunner.MonitorThread` should give the task a little time to finish before forcibly killing the python worker. This will reduce the chance of the race condition a lot. I also improved the log a bit to find out the task to blame when it's stuck.
## How was this patch tested?
Jenkins
Author: Shixiong Zhu <zsxwing@gmail.com>
Closes#19762 from zsxwing/SPARK-22535.
## What changes were proposed in this pull request?
tl;dr: Add a class, `MesosHadoopDelegationTokenManager` that updates delegation tokens on a schedule on the behalf of Spark Drivers. Broadcast renewed credentials to the executors.
## The problem
We recently added Kerberos support to Mesos-based Spark jobs as well as Secrets support to the Mesos Dispatcher (SPARK-16742, SPARK-20812, respectively). However the delegation tokens have a defined expiration. This poses a problem for long running Spark jobs (e.g. Spark Streaming applications). YARN has a solution for this where a thread is scheduled to renew the tokens they reach 75% of their way to expiration. It then writes the tokens to HDFS for the executors to find (uses a monotonically increasing suffix).
## This solution
We replace the current method in `CoarseGrainedSchedulerBackend` which used to discard the token renewal time with a protected method `fetchHadoopDelegationTokens`. Now the individual cluster backends are responsible for overriding this method to fetch and manage token renewal. The delegation tokens themselves, are still part of the `CoarseGrainedSchedulerBackend` as before.
In the case of Mesos renewed Credentials are broadcasted to the executors. This maintains all transfer of Credentials within Spark (as opposed to Spark-to-HDFS). It also does not require any writing of Credentials to disk. It also does not require any GC of old files.
## How was this patch tested?
Manually against a Kerberized HDFS cluster.
Thank you for the reviews.
Author: ArtRand <arand@soe.ucsc.edu>
Closes#19272 from ArtRand/spark-21842-450-kerberos-ticket-renewal.
## What changes were proposed in this pull request?
Do not include jdbc properties which may contain credentials in logging a logical plan with `SaveIntoDataSourceCommand` in it.
## How was this patch tested?
building locally and trying to reproduce (per the steps in https://issues.apache.org/jira/browse/SPARK-22479):
```
== Parsed Logical Plan ==
SaveIntoDataSourceCommand org.apache.spark.sql.execution.datasources.jdbc.JdbcRelationProvider570127fa, Map(dbtable -> test20, driver -> org.postgresql.Driver, url -> *********(redacted), password -> *********(redacted)), ErrorIfExists
+- Range (0, 100, step=1, splits=Some(8))
== Analyzed Logical Plan ==
SaveIntoDataSourceCommand org.apache.spark.sql.execution.datasources.jdbc.JdbcRelationProvider570127fa, Map(dbtable -> test20, driver -> org.postgresql.Driver, url -> *********(redacted), password -> *********(redacted)), ErrorIfExists
+- Range (0, 100, step=1, splits=Some(8))
== Optimized Logical Plan ==
SaveIntoDataSourceCommand org.apache.spark.sql.execution.datasources.jdbc.JdbcRelationProvider570127fa, Map(dbtable -> test20, driver -> org.postgresql.Driver, url -> *********(redacted), password -> *********(redacted)), ErrorIfExists
+- Range (0, 100, step=1, splits=Some(8))
== Physical Plan ==
Execute SaveIntoDataSourceCommand
+- SaveIntoDataSourceCommand org.apache.spark.sql.execution.datasources.jdbc.JdbcRelationProvider570127fa, Map(dbtable -> test20, driver -> org.postgresql.Driver, url -> *********(redacted), password -> *********(redacted)), ErrorIfExists
+- Range (0, 100, step=1, splits=Some(8))
```
Author: osatici <osatici@palantir.com>
Closes#19708 from onursatici/os/redact-jdbc-creds.
With the new UI store, the API resource classes have a lot less code,
since there's no need for complicated translations between the UI
types and the API types. So the code ended up with a bunch of files
with a single method declared in them.
This change re-structures the API code so that it uses less classes;
mainly, most sub-resources were removed, and the code to deal with
single-attempt and multi-attempt apps was simplified.
The only change was the addition of a method to return a single
attempt's information; that was missing in the old API, so trying
to retrieve "/v1/applications/appId/attemptId" would result in a
404 even if the attempt existed (and URIs under that one would
return valid data).
The streaming API resources also overtook the same treatment, even
though the data is not stored in the new UI store.
Author: Marcelo Vanzin <vanzin@cloudera.com>
Closes#19748 from vanzin/SPARK-20649.
This change replaces the SQLListener with a new implementation that
saves the data to the same store used by the SparkContext's status
store. For that, the types used by the old SQLListener had to be
updated a bit so that they're more serialization-friendly.
The interface for getting data from the store was abstracted into
a new class, SQLAppStatusStore (following the convention used in
core).
Another change is the way that the SQL UI hooks up into the core
UI or the SHS. The old "SparkHistoryListenerFactory" was replaced
with a new "AppStatePlugin" that more explicitly differentiates
between the two use cases: processing events, and showing the UI.
Both live apps and the SHS use this new API (previously, it was
restricted to the SHS).
Note on the above: this causes a slight change of behavior for
live apps; the SQL tab will only show up after the first execution
is started.
The metrics gathering code was re-worked a bit so that the types
used are less memory hungry and more serialization-friendly. This
reduces memory usage when using in-memory stores, and reduces load
times when using disk stores.
Tested with existing and added unit tests. Note one unit test was
disabled because it depends on SPARK-20653, which isn't in yet.
Author: Marcelo Vanzin <vanzin@cloudera.com>
Closes#19681 from vanzin/SPARK-20652.
This change is a little larger because there's a whole lot of logic
behind these pages, all really tied to internal types and listeners,
and some of that logic had to be implemented in the new listener and
the needed data exposed through the API types.
- Added missing StageData and ExecutorStageSummary fields which are
used by the UI. Some json golden files needed to be updated to account
for new fields.
- Save RDD graph data in the store. This tries to re-use existing types as
much as possible, so that the code doesn't need to be re-written. So it's
probably not very optimal.
- Some old classes (e.g. JobProgressListener) still remain, since they're used
in other parts of the code; they're not used by the UI anymore, though, and
will be cleaned up in a separate change.
- Save information about active pools in the store. This data is not really used
in the SHS, but it's not a lot of data so it's still recorded when replaying
applications.
- Because the new store sorts things slightly differently from the previous
code, some json golden files had some elements within them shuffled around.
- The retention unit test in UISeleniumSuite was disabled because the code
to throw away old stages / tasks hasn't been added yet.
- The job description field in the API tries to follow the old behavior, which
makes it be empty most of the time, even though there's information to fill it
in. For stages, a new field was added to hold the description (which is basically
the job description), so that the UI can be rendered in the old way.
- A new stage status ("SKIPPED") was added to account for the fact that the API
couldn't represent that state before. Without this, the stage would show up as
"PENDING" in the UI, which is now based on API types.
- The API used to expose "executorRunTime" as the value of the task's duration,
which wasn't really correct (also because that value was easily available
from the metrics object); this change fixes that by storing the correct duration,
which also means a few expectation files needed to be updated to account for
the new durations and sorting differences due to the changed values.
- Added changes to implement SPARK-20713 and SPARK-21922 in the new code.
Tested with existing unit tests (and by using the UI a lot).
Author: Marcelo Vanzin <vanzin@cloudera.com>
Closes#19698 from vanzin/SPARK-20648.
## What changes were proposed in this pull request?
Small fix. Using bufferedInputStream for dataDeserializeStream.
## How was this patch tested?
Existing UT.
Author: Xianyang Liu <xianyang.liu@intel.com>
Closes#19735 from ConeyLiu/smallfix.
## What changes were proposed in this pull request?
There are still some algorithms based on mllib, such as KMeans. For now, many mllib common class (such as: Vector, DenseVector, SparseVector, Matrix, DenseMatrix, SparseMatrix) are not registered in Kryo. So there are some performance issues for those object serialization or deserialization.
Previously dicussed: https://github.com/apache/spark/pull/19586
## How was this patch tested?
New test case.
Author: Xianyang Liu <xianyang.liu@intel.com>
Closes#19661 from ConeyLiu/register_vector.
Continuation of PR#19528 (https://github.com/apache/spark/pull/19529#issuecomment-340252119)
The problem with the maven build in the previous PR was the new tests.... the creation of a spark session outside the tests meant there was more than one spark session around at a time.
I was using the spark session outside the tests so that the tests could share data; I've changed it so that each test creates the data anew.
Author: Nathan Kronenfeld <nicole.oresme@gmail.com>
Author: Nathan Kronenfeld <nkronenfeld@uncharted.software>
Closes#19705 from nkronenfeld/alternative-style-tests-2.
## What changes were proposed in this pull request?
Adds java.nio bufferedPool memory metrics to metrics system which includes both direct and mapped memory.
## How was this patch tested?
Manually tested and checked direct and mapped memory metrics too available in metrics system using Console sink.
Here is the sample console output
application_1509655862825_0016.2.jvm.direct.capacity
value = 19497
application_1509655862825_0016.2.jvm.direct.count
value = 6
application_1509655862825_0016.2.jvm.direct.used
value = 19498
application_1509655862825_0016.2.jvm.mapped.capacity
value = 0
application_1509655862825_0016.2.jvm.mapped.count
value = 0
application_1509655862825_0016.2.jvm.mapped.used
value = 0
Author: Srinivasa Reddy Vundela <vsr@cloudera.com>
Closes#19709 from vundela/SPARK-22483.
This required adding information about StreamBlockId to the store,
which is not available yet via the API. So an internal type was added
until there's a need to expose that information in the API.
The UI only lists RDDs that have cached partitions, and that information
wasn't being correctly captured in the listener, so that's also fixed,
along with some minor (internal) API adjustments so that the UI can
get the correct data.
Because of the way partitions are cached, some optimizations w.r.t. how
often the data is flushed to the store could not be applied to this code;
because of that, some different ways to make the code more performant
were added to the data structures tracking RDD blocks, with the goal of
avoiding expensive copies when lots of blocks are being updated.
Tested with existing and updated unit tests.
Author: Marcelo Vanzin <vanzin@cloudera.com>
Closes#19679 from vanzin/SPARK-20647.
## What changes were proposed in this pull request?
Fixing nits in MetricsSystemSuite file
1) Using Sink instead of Source while casting
2) Using meaningful naming for variables, which reflect their usage
## How was this patch tested?
Ran the tests locally and all of them passing
Author: Srinivasa Reddy Vundela <vsr@cloudera.com>
Closes#19699 from vundela/master.
## What changes were proposed in this pull request?
Preliminary changes to get ClosureCleaner to work with Scala 2.12. Makes many usages just work, but not all. This does _not_ resolve the JIRA.
## How was this patch tested?
Existing tests
Author: Sean Owen <sowen@cloudera.com>
Closes#19675 from srowen/SPARK-14540.0.
The executors page is built on top of the REST API, so the page itself
was easy to hook up to the new code.
Some other pages depend on the `ExecutorListener` class that is being
removed, though, so they needed to be modified to use data from the
new store. Fortunately, all they seemed to need is the map of executor
logs, so that was somewhat easy too.
The executor timeline graph required adding some properties to the
ExecutorSummary API type. Instead of following the previous code,
which stored all the listener events in memory, the timeline is
now created based on the data available from the API.
I had to change some of the test golden files because the old code would
return executors in "random" order (since it used a mutable Map instead
of something that returns a sorted list), and the new code returns executors
in id order.
Tested with existing unit tests.
Author: Marcelo Vanzin <vanzin@cloudera.com>
Closes#19678 from vanzin/SPARK-20646.
This change modifies the status listener to collect the information
needed to render the envionment page, and populates that page and the
API with information collected by the listener.
Tested with existing and added unit tests.
Author: Marcelo Vanzin <vanzin@cloudera.com>
Closes#19677 from vanzin/SPARK-20645.
…alization.
## What changes were proposed in this pull request?
Use non-linear containsKey operation for serialized maps, lookup into underlying map.
## How was this patch tested?
unit tests
Author: Alexander Istomin <istomin@rutarget.ru>
Closes#19553 from Whoosh/SPARK-22330.
There are two somewhat unrelated things going on in this patch, but
both are meant to make integration of individual UI pages later on
much easier.
The first part is some tweaking of the code in the listener so that
it does less updates of the kvstore for data that changes fast; for
example, it avoids writing changes down to the store for every
task-related event, since those can arrive very quickly at times.
Instead, for these kinds of events, it chooses to only flush things
if a certain interval has passed. The interval is based on how often
the current spark-shell code updates the progress bar for jobs, so
that users can get reasonably accurate data.
The code also delays as much as possible hitting the underlying kvstore
when replaying apps in the history server. This is to avoid unnecessary
writes to disk.
The second set of changes prepare the history server and SparkUI for
integrating with the kvstore. A new class, AppStatusStore, is used
for translating between the stored data and the types used in the
UI / API. The SHS now populates a kvstore with data loaded from
event logs when an application UI is requested.
Because this store can hold references to disk-based resources, the
code was modified to retrieve data from the store under a read lock.
This allows the SHS to detect when the store is still being used, and
only update it (e.g. because an updated event log was detected) when
there is no other thread using the store.
This change ended up creating a lot of churn in the ApplicationCache
code, which was cleaned up a lot in the process. I also removed some
metrics which don't make too much sense with the new code.
Tested with existing and added unit tests, and by making sure the SHS
still works on a real cluster.
Author: Marcelo Vanzin <vanzin@cloudera.com>
Closes#19582 from vanzin/SPARK-20644.
## What changes were proposed in this pull request?
This PR replaces the old the maximum array size (`Int.MaxValue`) with the new one (`ByteArrayMethods.MAX_ROUNDED_ARRAY_LENGTH`).
This PR also refactor the code to calculate the new array size to easily understand why we have to use `newSize - 2` for allocating a new array.
## How was this patch tested?
Used the existing test
Author: Kazuaki Ishizaki <ishizaki@jp.ibm.com>
Closes#19650 from kiszk/SPARK-22254.
## What changes were proposed in this pull request?
This proposed patch is about making Spark executor task metrics available as Dropwizard metrics. This is intended to be of aid in monitoring Spark jobs and when drilling down on performance troubleshooting issues.
## How was this patch tested?
Manually tested on a Spark cluster (see JIRA for an example screenshot).
Author: LucaCanali <luca.canali@cern.ch>
Closes#19426 from LucaCanali/SparkTaskMetricsDropWizard.
## What changes were proposed in this pull request?
Using zstd compression for Spark jobs spilling 100s of TBs of data, we could reduce the amount of data written to disk by as much as 50%. This translates to significant latency gain because of reduced disk io operations. There is a degradation CPU time by 2 - 5% because of zstd compression overhead, but for jobs which are bottlenecked by disk IO, this hit can be taken.
## Benchmark
Please note that this benchmark is using real world compute heavy production workload spilling TBs of data to disk
| | zstd performance as compred to LZ4 |
| ------------- | -----:|
| spill/shuffle bytes | -48% |
| cpu time | + 3% |
| cpu reservation time | -40%|
| latency | -40% |
## How was this patch tested?
Tested by running few jobs spilling large amount of data on the cluster and amount of intermediate data written to disk reduced by as much as 50%.
Author: Sital Kedia <skedia@fb.com>
Closes#18805 from sitalkedia/skedia/upstream_zstd.
## What changes were proposed in this pull request?
Handling the NonFatal exceptions while starting the external shuffle service, if there are any NonFatal exceptions it logs and continues without the external shuffle service.
## How was this patch tested?
I verified it manually, it logs the exception and continues to serve without external shuffle service when BindException occurs.
Author: Devaraj K <devaraj@apache.org>
Closes#19396 from devaraj-kavali/SPARK-22172.
## What changes were proposed in this pull request?
PeriodicRDDCheckpointer was already moved out of mllib in Spark-5484
## How was this patch tested?
existing tests
Author: Zheng RuiFeng <ruifengz@foxmail.com>
Closes#19618 from zhengruifeng/checkpointer_doc.
## What changes were proposed in this pull request?
We often see the issue of Spark jobs stuck because the Executor Allocation Manager does not ask for any executor even if there are pending tasks in case dynamic allocation is turned on. Looking at the logic in Executor Allocation Manager, which calculates the running tasks, it can happen that the calculation will be wrong and the number of running tasks can become negative.
## How was this patch tested?
Added unit test
Author: Sital Kedia <skedia@fb.com>
Closes#19580 from sitalkedia/skedia/fix_stuck_job.
## What changes were proposed in this pull request?
In `UnsafeInMemorySorter`, one record may take 32 bytes: 1 `long` for pointer, 1 `long` for key-prefix, and another 2 `long`s as the temporary buffer for radix sort.
In `UnsafeExternalSorter`, we set the `DEFAULT_NUM_ELEMENTS_FOR_SPILL_THRESHOLD` to be `1024 * 1024 * 1024 / 2`, and hoping the max size of point array to be 8 GB. However this is wrong, `1024 * 1024 * 1024 / 2 * 32` is actually 16 GB, and if we grow the point array before reach this limitation, we may hit the max-page-size error.
Users may see exception like this on large dataset:
```
Caused by: java.lang.IllegalArgumentException: Cannot allocate a page with more than 17179869176 bytes
at org.apache.spark.memory.TaskMemoryManager.allocatePage(TaskMemoryManager.java:241)
at org.apache.spark.memory.MemoryConsumer.allocatePage(MemoryConsumer.java:121)
at org.apache.spark.util.collection.unsafe.sort.UnsafeExternalSorter.acquireNewPageIfNecessary(UnsafeExternalSorter.java:374)
at org.apache.spark.util.collection.unsafe.sort.UnsafeExternalSorter.insertRecord(UnsafeExternalSorter.java:396)
at org.apache.spark.sql.execution.UnsafeExternalRowSorter.insertRow(UnsafeExternalRowSorter.java:94)
...
```
Setting `DEFAULT_NUM_ELEMENTS_FOR_SPILL_THRESHOLD` to a smaller number is not enough, users can still set the config to a big number and trigger the too large page size issue. This PR fixes it by explicitly handling the too large page size exception in the sorter and spill.
This PR also change the type of `spark.shuffle.spill.numElementsForceSpillThreshold` to int, because it's only compared with `numRecords`, which is an int. This is an internal conf so we don't have a serious compatibility issue.
## How was this patch tested?
TODO
Author: Wenchen Fan <wenchen@databricks.com>
Closes#18251 from cloud-fan/sort.
## What changes were proposed in this pull request?
When the given closure uses some fields defined in super class, `ClosureCleaner` can't figure them and don't set it properly. Those fields will be in null values.
## How was this patch tested?
Added test.
Author: Liang-Chi Hsieh <viirya@gmail.com>
Closes#19556 from viirya/SPARK-22328.
The initial listener code is based on the existing JobProgressListener (and others),
and tries to mimic their behavior as much as possible. The change also includes
some minor code movement so that some types and methods from the initial history
server code code can be reused.
The code introduces a few mutable versions of public API types, used internally,
to make it easier to update information without ugly copy methods, and also to
make certain updates cheaper.
Note the code here is not 100% correct. This is meant as a building ground for
the UI integration in the next milestones. As different parts of the UI are
ported, fixes will be made to the different parts of this code to account
for the needed behavior.
I also added annotations to API types so that Jackson is able to correctly
deserialize options, sequences and maps that store primitive types.
Author: Marcelo Vanzin <vanzin@cloudera.com>
Closes#19383 from vanzin/SPARK-20643.