### What changes were proposed in this pull request?
Currently, inferring nested structs is always using `MapType`.
This behavior causes an issue because it infers the schema with a value type of the first field of the struct as below:
```python
data = [{"inside_struct": {"payment": 100.5, "name": "Lee"}}]
df = spark.createDataFrame(data)
df.show(truncate=False)
+--------------------------------+
|inside_struct |
+--------------------------------+
|{name -> null, payment -> 100.5}|
+--------------------------------+
```
The "name" became `null`, but it should've been `"Lee"`.
In this case, we need to be able to infer the schema with a `StructType` instead of a `MapType`.
Therefore, this PR proposes adding an new configuration `spark.sql.pyspark.inferNestedDictAsStruct.enabled` to handle which type is used for inferring nested structs.
- When `spark.sql.pyspark.inferNestedDictAsStruct.enabled` is `false` (by default), inferring nested structs by `MapType`
- When `spark.sql.pyspark.inferNestedDictAsStruct.enabled` is `true`, inferring nested structs by `StructType`
### Why are the changes needed?
Because always inferring the nested structs by `MapType` doesn't work properly for some cases.
### Does this PR introduce _any_ user-facing change?
New configuration `spark.sql.pyspark.inferNestedDictAsStruct.enabled` is added.
### How was this patch tested?
Added an unit test
Closes#33214 from itholic/SPARK-35929.
Lead-authored-by: itholic <haejoon.lee@databricks.com>
Co-authored-by: Hyukjin Kwon <gurwls223@gmail.com>
Signed-off-by: Hyukjin Kwon <gurwls223@apache.org>
### What changes were proposed in this pull request?
By default, AQE will set `COALESCE_PARTITIONS_MIN_PARTITION_NUM` to the spark default parallelism, which is usually quite big. This is to keep the parallelism on par with non-AQE, to avoid perf regressions.
However, this usually leads to many small/empty partitions, and hurts performance (although not worse than non-AQE). Users usually blindly set `COALESCE_PARTITIONS_MIN_PARTITION_NUM` to 1, which makes this config quite useless.
This PR adds a new config to set the min partition size, to avoid too small partitions after coalescing. By default, Spark will not respect the target size, and only respect this min partition size, to maximize the parallelism and avoid perf regression in AQE. This PR also adds a bool config to respect the target size when coalescing partitions, and it's recommended to set it to get better overall performance. This PR also deprecates the `COALESCE_PARTITIONS_MIN_PARTITION_NUM` config.
### Why are the changes needed?
AQE is default on now, we should make the perf better in the default case.
### Does this PR introduce _any_ user-facing change?
yes, a new config.
### How was this patch tested?
new tests
Closes#33172 from cloud-fan/aqe2.
Authored-by: Wenchen Fan <wenchen@databricks.com>
Signed-off-by: Wenchen Fan <wenchen@databricks.com>
### What changes were proposed in this pull request?
This PR proposes move `to_pandas_on_spark` function from `pyspark.pandas.frame` to `pyspark.sql.dataframe`, and added the related tests to the PySpark DataFrame tests.
### Why are the changes needed?
Because now the Koalas is ported into PySpark, so we don't need to Spark auto-patch anymore.
And also `to_pandas_on_spark` is belongs to the pandas-on-Spark DataFrame doesn't look make sense.
### Does this PR introduce _any_ user-facing change?
No, it's kinda internal refactoring stuff.
### How was this patch tested?
Added the related tests and manually check they're passed.
Closes#33054 from itholic/SPARK-35605.
Authored-by: itholic <haejoon.lee@databricks.com>
Signed-off-by: Hyukjin Kwon <gurwls223@apache.org>
### What changes were proposed in this pull request?
This PR proposes to use a proper built-in exceptions instead of the plain `Exception` in Python.
While I am here, I fixed another minor issue at `DataFrams.schema` together:
```diff
- except AttributeError as e:
- raise Exception(
- "Unable to parse datatype from schema. %s" % e)
+ except Exception as e:
+ raise ValueError(
+ "Unable to parse datatype from schema. %s" % e) from e
```
Now it catches all exceptions during schema parsing, chains the exception with `ValueError`. Previously it only caught `AttributeError` that does not catch all cases.
### Why are the changes needed?
For users to expect the proper exceptions.
### Does this PR introduce _any_ user-facing change?
Yeah, the exception classes became different but should be compatible because previous exception was plain `Exception` which other exceptions inherit.
### How was this patch tested?
Existing unittests should cover,
Closes#31238Closes#32650 from HyukjinKwon/SPARK-32194.
Authored-by: Hyukjin Kwon <gurwls223@apache.org>
Signed-off-by: Hyukjin Kwon <gurwls223@apache.org>
### What changes were proposed in this pull request?
Provide clearer error message tied to the user's Python code if incorrect parameters are passed to `DataFrame.show` rather than the message about a missing JVM method the user is not calling directly.
```
py4j.Py4JException: Method showString([class java.lang.Boolean, class java.lang.Integer, class java.lang.Boolean]) does not exist
at py4j.reflection.ReflectionEngine.getMethod(ReflectionEngine.java:318)
at py4j.reflection.ReflectionEngine.getMethod(ReflectionEngine.java:326)
at py4j.Gateway.invoke(Gateway.java:274)
at py4j.commands.AbstractCommand.invokeMethod(AbstractCommand.java:132)
at py4j.commands.CallCommand.execute(CallCommand.java:79)
at py4j.GatewayConnection.run(GatewayConnection.java:238)
at java.lang.Thread.run(Thread.java:748
```
### Why are the changes needed?
For faster debugging through actionable error message.
### Does this PR introduce _any_ user-facing change?
No change for the correct parameters but different error messages for the parameters triggering an exception.
### How was this patch tested?
- unit test
- manually in PySpark REPL
Closes#32555 from gerashegalov/df_show_validation.
Authored-by: Gera Shegalov <gera@apache.org>
Signed-off-by: Hyukjin Kwon <gurwls223@apache.org>
### What changes were proposed in this pull request?
This PR fixes the same issue as #32424.
```py
from pyspark.sql.functions import flatten, struct, transform
df = spark.sql("SELECT array(1, 2, 3) as numbers, array('a', 'b', 'c') as letters")
df.select(flatten(
transform(
"numbers",
lambda number: transform(
"letters",
lambda letter: struct(number.alias("n"), letter.alias("l"))
)
)
).alias("zipped")).show(truncate=False)
```
**Before:**
```
+------------------------------------------------------------------------+
|zipped |
+------------------------------------------------------------------------+
|[{a, a}, {b, b}, {c, c}, {a, a}, {b, b}, {c, c}, {a, a}, {b, b}, {c, c}]|
+------------------------------------------------------------------------+
```
**After:**
```
+------------------------------------------------------------------------+
|zipped |
+------------------------------------------------------------------------+
|[{1, a}, {1, b}, {1, c}, {2, a}, {2, b}, {2, c}, {3, a}, {3, b}, {3, c}]|
+------------------------------------------------------------------------+
```
### Why are the changes needed?
To produce the correct results.
### Does this PR introduce _any_ user-facing change?
Yes, it fixes the results to be correct as mentioned above.
### How was this patch tested?
Added a unit test as well as manually.
Closes#32523 from ueshin/issues/SPARK-35382/nested_higher_order_functions.
Authored-by: Takuya UESHIN <ueshin@databricks.com>
Signed-off-by: Hyukjin Kwon <gurwls223@apache.org>
### What changes were proposed in this pull request?
This PR corrects some exception type when the function input params are failed to validate due to TypeError.
In order to convenient to review, there are 3 commits in this PR:
- Standardize input validation error type on sql
- Standardize input validation error type on ml
- Standardize input validation error type on pandas
### Why are the changes needed?
As suggestion from Python exception doc [1]: "Raised when an operation or function is applied to an object of inappropriate type.", but there are many Value error are raised in some pyspark code, this patch fix them.
[1] https://docs.python.org/3/library/exceptions.html#TypeError
Note that: this patch only addresses the exsiting some wrong raise type for input validation, the input validation decorator/framework which mentioned in [SPARK-35176](https://issues.apache.org/jira/browse/SPARK-35176), would be submited in a speparated patch.
### Does this PR introduce _any_ user-facing change?
Yes, code can raise the right TypeError instead of ValueError.
### How was this patch tested?
Existing test case and UT
Closes#32368 from Yikun/SPARK-35176.
Authored-by: Yikun Jiang <yikunkero@gmail.com>
Signed-off-by: HyukjinKwon <gurwls223@apache.org>
### What changes were proposed in this pull request?
This PR fixes an issue that `quoteIfNeeded` quotes a name only if it contains `.` or ``` ` ```.
This method should quote it if it contains non-word characters.
### Why are the changes needed?
It's a potential bug.
### Does this PR introduce _any_ user-facing change?
No.
### How was this patch tested?
New test.
Closes#31964 from sarutak/fix-quoteIfNeeded.
Authored-by: Kousuke Saruta <sarutak@oss.nttdata.com>
Signed-off-by: Wenchen Fan <wenchen@databricks.com>
### What changes were proposed in this pull request?
This PR fixes an issue that `sql` method in the following classes which take qualified names don't quote the qualified names properly.
* UnresolvedAttribute
* AttributeReference
* Alias
One instance caused by this issue is reported in SPARK-34626.
```
UnresolvedAttribute("a" :: "b" :: Nil).sql
`a.b` // expected: `a`.`b`
```
And other instances are like as follows.
```
UnresolvedAttribute("a`b"::"c.d"::Nil).sql
a`b.`c.d` // expected: `a``b`.`c.d`
AttributeReference("a.b", IntegerType)(qualifier = "c.d"::Nil).sql
c.d.`a.b` // expected: `c.d`.`a.b`
Alias(AttributeReference("a", IntegerType)(), "b.c")(qualifier = "d.e"::Nil).sql
`a` AS d.e.`b.c` // expected: `a` AS `d.e`.`b.c`
```
### Why are the changes needed?
This is a bug.
### Does this PR introduce _any_ user-facing change?
No.
### How was this patch tested?
New test.
Closes#31754 from sarutak/fix-qualified-names.
Authored-by: Kousuke Saruta <sarutak@oss.nttdata.com>
Signed-off-by: Wenchen Fan <wenchen@databricks.com>
### What changes were proposed in this pull request?
pyrolite 4.21 introduced and enabled value comparison by default (`valueCompare=true`) during object memoization and serialization: https://github.com/irmen/Pyrolite/blob/pyrolite-4.21/java/src/main/java/net/razorvine/pickle/Pickler.java#L112-L122
This change has undesired effect when we serialize a row (actually `GenericRowWithSchema`) to be passed to python: https://github.com/apache/spark/blob/branch-3.0/sql/core/src/main/scala/org/apache/spark/sql/execution/python/EvaluatePython.scala#L60. A simple example is that
```
new GenericRowWithSchema(Array(1.0, 1.0), StructType(Seq(StructField("_1", DoubleType), StructField("_2", DoubleType))))
```
and
```
new GenericRowWithSchema(Array(1, 1), StructType(Seq(StructField("_1", IntegerType), StructField("_2", IntegerType))))
```
are currently equal and the second instance is replaced to the short code of the first one during serialization.
### Why are the changes needed?
The above can cause nasty issues like the one in https://issues.apache.org/jira/browse/SPARK-34545 description:
```
>>> from pyspark.sql.functions import udf
>>> from pyspark.sql.types import *
>>>
>>> def udf1(data_type):
def u1(e):
return e[0]
return udf(u1, data_type)
>>>
>>> df = spark.createDataFrame([((1.0, 1.0), (1, 1))], ['c1', 'c2'])
>>>
>>> df = df.withColumn("c3", udf1(DoubleType())("c1"))
>>> df = df.withColumn("c4", udf1(IntegerType())("c2"))
>>>
>>> df.select("c3").show()
+---+
| c3|
+---+
|1.0|
+---+
>>> df.select("c4").show()
+---+
| c4|
+---+
| 1|
+---+
>>> df.select("c3", "c4").show()
+---+----+
| c3| c4|
+---+----+
|1.0|null|
+---+----+
```
This is because during serialization from JVM to Python `GenericRowWithSchema(1.0, 1.0)` (`c1`) is memoized first and when `GenericRowWithSchema(1, 1)` (`c2`) comes next, it is replaced to some short code of the `c1` (instead of serializing `c2` out) as they are `equal()`. The python functions then runs but the return type of `c4` is expected to be `IntegerType` and if a different type (`DoubleType`) comes back from python then it is discarded: https://github.com/apache/spark/blob/branch-3.0/sql/core/src/main/scala/org/apache/spark/sql/execution/python/EvaluatePython.scala#L108-L113
After this PR:
```
>>> df.select("c3", "c4").show()
+---+---+
| c3| c4|
+---+---+
|1.0| 1|
+---+---+
```
### Does this PR introduce _any_ user-facing change?
Yes, fixes a correctness issue.
### How was this patch tested?
Added new UT + manual tests.
Closes#31682 from peter-toth/SPARK-34545-fix-row-comparison.
Authored-by: Peter Toth <peter.toth@gmail.com>
Signed-off-by: Sean Owen <srowen@gmail.com>
### What changes were proposed in this pull request?
Fixes a Python UDF `plus_one` used in `GroupedAggPandasUDFTests` to always return float (double) values.
### Why are the changes needed?
The Python UDF `plus_one` used in `GroupedAggPandasUDFTests` is always returning `v + 1` regardless of its type. The return type of the UDF is 'double', so if the input is int, the result will be `null`.
```py
>>> df = spark.range(10).toDF('id') \
... .withColumn("vs", array([lit(i * 1.0) + col('id') for i in range(20, 30)])) \
... .withColumn("v", explode(col('vs'))) \
... .drop('vs') \
... .withColumn('w', lit(1.0))
>>> udf('double')
... def plus_one(v):
... assert isinstance(v, (int, float))
... return v + 1
...
>>> pandas_udf('double', PandasUDFType.GROUPED_AGG)
... def sum_udf(v):
... return v.sum()
...
>>> df.groupby(plus_one(df.id)).agg(sum_udf(df.v)).show()
+------------+----------+
|plus_one(id)|sum_udf(v)|
+------------+----------+
| null| 2900.0|
+------------+----------+
```
This is meaningless and should be:
```py
>>> udf('double')
... def plus_one(v):
... assert isinstance(v, (int, float))
... return float(v + 1)
...
>>> df.groupby(plus_one(df.id)).agg(sum_udf(df.v)).sort('plus_one(id)').show()
+------------+----------+
|plus_one(id)|sum_udf(v)|
+------------+----------+
| 1.0| 245.0|
| 2.0| 255.0|
| 3.0| 265.0|
| 4.0| 275.0|
| 5.0| 285.0|
| 6.0| 295.0|
| 7.0| 305.0|
| 8.0| 315.0|
| 9.0| 325.0|
| 10.0| 335.0|
+------------+----------+
```
### Does this PR introduce _any_ user-facing change?
No, test-only.
### How was this patch tested?
Fixed the test.
Closes#31730 from ueshin/issues/SPARK-34610/test_pandas_udf_grouped_agg.
Authored-by: Takuya UESHIN <ueshin@databricks.com>
Signed-off-by: HyukjinKwon <gurwls223@apache.org>
### What changes were proposed in this pull request?
Creating a Pandas dataframe via Apache Arrow currently can use twice as much memory as the final result, because during the conversion, both Pandas and Arrow retain a copy of the data. Arrow has a "self-destruct" mode now (Arrow >= 0.16) to avoid this, by freeing each column after conversion. This PR integrates support for this in toPandas, handling a couple of edge cases:
self_destruct has no effect unless the memory is allocated appropriately, which is handled in the Arrow serializer here. Essentially, the issue is that self_destruct frees memory column-wise, but Arrow record batches are oriented row-wise:
```
Record batch 0: allocation 0: column 0 chunk 0, column 1 chunk 0, ...
Record batch 1: allocation 1: column 0 chunk 1, column 1 chunk 1, ...
```
In this scenario, Arrow will drop references to all of column 0's chunks, but no memory will actually be freed, as the chunks were just slices of an underlying allocation. The PR copies each column into its own allocation so that memory is instead arranged as so:
```
Record batch 0: allocation 0 column 0 chunk 0, allocation 1 column 1 chunk 0, ...
Record batch 1: allocation 2 column 0 chunk 1, allocation 3 column 1 chunk 1, ...
```
The optimization is disabled by default, and can be enabled with the Spark SQL conf "spark.sql.execution.arrow.pyspark.selfDestruct.enabled" set to "true". We can't always apply this optimization because it's more likely to generate a dataframe with immutable buffers, which Pandas doesn't always handle well, and because it is slower overall (since it only converts one column at a time instead of in parallel).
### Why are the changes needed?
This lets us load larger datasets - in particular, with N bytes of memory, before we could never load a dataset bigger than N/2 bytes; now the overhead is more like N/1.25 or so.
### Does this PR introduce _any_ user-facing change?
Yes - it adds a new SQL conf "spark.sql.execution.arrow.pyspark.selfDestruct.enabled"
### How was this patch tested?
See the [mailing list](http://apache-spark-developers-list.1001551.n3.nabble.com/DISCUSS-Reducing-memory-usage-of-toPandas-with-Arrow-quot-self-destruct-quot-option-td30149.html) - it was tested with Python memory_profiler. Unit tests added to check memory within certain bounds and correctness with the option enabled.
Closes#29818 from lidavidm/spark-32953.
Authored-by: David Li <li.davidm96@gmail.com>
Signed-off-by: Bryan Cutler <cutlerb@gmail.com>
### What changes were proposed in this pull request?
This PR completes snake_case rule at functions APIs across the languages, see also SPARK-10621.
In more details, this PR:
- Adds `count_distinct` in Scala Python, and R, and document that `count_distinct` is encouraged. This was not deprecated because `countDistinct` is pretty commonly used. We could deprecate in the future releases.
- (Scala-specific) adds `typedlit` but doesn't deprecate `typedLit` which is arguably commonly used. Likewise, we could deprecate in the future releases.
- Deprecates and renames:
- `sumDistinct` -> `sum_distinct`
- `bitwiseNOT` -> `bitwise_not`
- `shiftLeft` -> `shiftleft` (matched with SQL name in `FunctionRegistry`)
- `shiftRight` -> `shiftright` (matched with SQL name in `FunctionRegistry`)
- `shiftRightUnsigned` -> `shiftrightunsigned` (matched with SQL name in `FunctionRegistry`)
- (Scala-specific) `callUDF` -> `call_udf`
### Why are the changes needed?
To keep the consistent naming in APIs.
### Does this PR introduce _any_ user-facing change?
Yes, it deprecates some APIs and add new renamed APIs as described above.
### How was this patch tested?
Unittests were added.
Closes#31408 from HyukjinKwon/SPARK-34306.
Authored-by: HyukjinKwon <gurwls223@apache.org>
Signed-off-by: HyukjinKwon <gurwls223@apache.org>
### What changes were proposed in this pull request?
Adds `NullType` support for Arrow executions.
### Why are the changes needed?
As Arrow supports null type, we can convert `NullType` between PySpark and pandas with Arrow enabled.
### Does this PR introduce _any_ user-facing change?
Yes, if a user has a DataFrame including `NullType`, it will be able to convert with Arrow enabled.
### How was this patch tested?
Added tests.
Closes#31285 from ueshin/issues/SPARK-33489/arrow_nulltype.
Authored-by: Takuya UESHIN <ueshin@databricks.com>
Signed-off-by: HyukjinKwon <gurwls223@apache.org>
### What changes were proposed in this pull request?
This PR is a follow-up of #29138 and #29195 to add more tests for `slice` function.
### Why are the changes needed?
The original PRs are missing tests with column-based arguments instead of literals.
### Does this PR introduce _any_ user-facing change?
No.
### How was this patch tested?
Added tests and existing tests.
Closes#31159 from ueshin/issues/SPARK-32338/slice_tests.
Authored-by: Takuya UESHIN <ueshin@databricks.com>
Signed-off-by: HyukjinKwon <gurwls223@apache.org>
### What changes were proposed in this pull request?
This PR proposes to:
- Make doctests simpler to show the usage (since we're not running them now).
- Use the test utils to drop the tables if exists.
### Why are the changes needed?
Better docs and code readability.
### Does this PR introduce _any_ user-facing change?
No, dev-only. It includes some doc changes in unreleased branches.
### How was this patch tested?
Manually tested.
```bash
cd python
./run-tests --python-executable=python3.9,python3.8 --testnames "pyspark.sql.tests.test_streaming StreamingTests"
```
Closes#30873 from HyukjinKwon/SPARK-33836.
Authored-by: HyukjinKwon <gurwls223@apache.org>
Signed-off-by: Jungtaek Lim <kabhwan.opensource@gmail.com>
### What changes were proposed in this pull request?
This PR proposes to expose `DataStreamReader.table` (SPARK-32885) and `DataStreamWriter.toTable` (SPARK-32896) to PySpark, which are the only way to read and write with table in Structured Streaming.
### Why are the changes needed?
Please refer SPARK-32885 and SPARK-32896 for rationalizations of these public APIs. This PR only exposes them to PySpark.
### Does this PR introduce _any_ user-facing change?
Yes, PySpark users will be able to read and write with table in Structured Streaming query.
### How was this patch tested?
Manually tested.
> v1 table
>> create table A and ingest to the table A
```
spark.sql("""
create table table_pyspark_parquet (
value long,
`timestamp` timestamp
) USING parquet
""")
df = spark.readStream.format('rate').option('rowsPerSecond', 100).load()
query = df.writeStream.toTable('table_pyspark_parquet', checkpointLocation='/tmp/checkpoint5')
query.lastProgress
query.stop()
```
>> read table A and ingest to the table B which doesn't exist
```
df2 = spark.readStream.table('table_pyspark_parquet')
query2 = df2.writeStream.toTable('table_pyspark_parquet_nonexist', format='parquet', checkpointLocation='/tmp/checkpoint2')
query2.lastProgress
query2.stop()
```
>> select tables
```
spark.sql("DESCRIBE TABLE table_pyspark_parquet").show()
spark.sql("SELECT * FROM table_pyspark_parquet").show()
spark.sql("DESCRIBE TABLE table_pyspark_parquet_nonexist").show()
spark.sql("SELECT * FROM table_pyspark_parquet_nonexist").show()
```
> v2 table (leveraging Apache Iceberg as it provides V2 table and custom catalog as well)
>> create table A and ingest to the table A
```
spark.sql("""
create table iceberg_catalog.default.table_pyspark_v2table (
value long,
`timestamp` timestamp
) USING iceberg
""")
df = spark.readStream.format('rate').option('rowsPerSecond', 100).load()
query = df.select('value', 'timestamp').writeStream.toTable('iceberg_catalog.default.table_pyspark_v2table', checkpointLocation='/tmp/checkpoint_v2table_1')
query.lastProgress
query.stop()
```
>> ingest to the non-exist table B
```
df2 = spark.readStream.format('rate').option('rowsPerSecond', 100).load()
query2 = df2.select('value', 'timestamp').writeStream.toTable('iceberg_catalog.default.table_pyspark_v2table_nonexist', checkpointLocation='/tmp/checkpoint_v2table_2')
query2.lastProgress
query2.stop()
```
>> ingest to the non-exist table C partitioned by `value % 10`
```
df3 = spark.readStream.format('rate').option('rowsPerSecond', 100).load()
df3a = df3.selectExpr('value', 'timestamp', 'value % 10 AS partition').repartition('partition')
query3 = df3a.writeStream.partitionBy('partition').toTable('iceberg_catalog.default.table_pyspark_v2table_nonexist_partitioned', checkpointLocation='/tmp/checkpoint_v2table_3')
query3.lastProgress
query3.stop()
```
>> select tables
```
spark.sql("DESCRIBE TABLE iceberg_catalog.default.table_pyspark_v2table").show()
spark.sql("SELECT * FROM iceberg_catalog.default.table_pyspark_v2table").show()
spark.sql("DESCRIBE TABLE iceberg_catalog.default.table_pyspark_v2table_nonexist").show()
spark.sql("SELECT * FROM iceberg_catalog.default.table_pyspark_v2table_nonexist").show()
spark.sql("DESCRIBE TABLE iceberg_catalog.default.table_pyspark_v2table_nonexist_partitioned").show()
spark.sql("SELECT * FROM iceberg_catalog.default.table_pyspark_v2table_nonexist_partitioned").show()
```
Closes#30835 from HeartSaVioR/SPARK-33836.
Lead-authored-by: Jungtaek Lim <kabhwan.opensource@gmail.com>
Co-authored-by: Jungtaek Lim (HeartSaVioR) <kabhwan.opensource@gmail.com>
Signed-off-by: HyukjinKwon <gurwls223@apache.org>
### What changes were proposed in this pull request?
This replaces deprecated API usage in PySpark tests with the preferred APIs. These have been deprecated for some time and usage is not consistent within tests.
- https://docs.python.org/3/library/unittest.html#deprecated-aliases
### Why are the changes needed?
For consistency and eventual removal of deprecated APIs.
### Does this PR introduce _any_ user-facing change?
No
### How was this patch tested?
Existing tests
Closes#30557 from BryanCutler/replace-deprecated-apis-in-tests.
Authored-by: Bryan Cutler <cutlerb@gmail.com>
Signed-off-by: HyukjinKwon <gurwls223@apache.org>
### What changes were proposed in this pull request?
This PR intends to fix typos in the sub-modules:
* `R`
* `common`
* `dev`
* `mlib`
* `external`
* `project`
* `streaming`
* `resource-managers`
* `python`
Split per srowen https://github.com/apache/spark/pull/30323#issuecomment-728981618
NOTE: The misspellings have been reported at 706a726f87 (commitcomment-44064356)
### Why are the changes needed?
Misspelled words make it harder to read / understand content.
### Does this PR introduce _any_ user-facing change?
There are various fixes to documentation, etc...
### How was this patch tested?
No testing was performed
Closes#30402 from jsoref/spelling-R_common_dev_mlib_external_project_streaming_resource-managers_python.
Authored-by: Josh Soref <jsoref@users.noreply.github.com>
Signed-off-by: Sean Owen <srowen@gmail.com>
### What changes were proposed in this pull request?
This PR adds the following functions (introduced in Scala API with SPARK-33061):
- `acosh`
- `asinh`
- `atanh`
to Python and R.
### Why are the changes needed?
Feature parity.
### Does this PR introduce _any_ user-facing change?
New functions.
### How was this patch tested?
New unit tests.
Closes#30501 from zero323/SPARK-33563.
Authored-by: zero323 <mszymkiewicz@gmail.com>
Signed-off-by: HyukjinKwon <gurwls223@apache.org>
### What changes were proposed in this pull request?
This change adds MapType support for PySpark with Arrow, if using pyarrow >= 2.0.0.
### Why are the changes needed?
MapType was previous unsupported with Arrow.
### Does this PR introduce _any_ user-facing change?
User can now enable MapType for `createDataFrame()`, `toPandas()` with Arrow optimization, and with Pandas UDFs.
### How was this patch tested?
Added new PySpark tests for createDataFrame(), toPandas() and Scalar Pandas UDFs.
Closes#30393 from BryanCutler/arrow-add-MapType-SPARK-24554.
Authored-by: Bryan Cutler <cutlerb@gmail.com>
Signed-off-by: HyukjinKwon <gurwls223@apache.org>
### What changes were proposed in this pull request?
Removes encoding of the JVM response in `pyspark.sql.column.Column.__repr__`.
### Why are the changes needed?
API consistency and improved readability of the expressions.
### Does this PR introduce _any_ user-facing change?
Before this change
col("abc")
col("wąż")
result in
Column<b'abc'>
Column<b'w\xc4\x85\xc5\xbc'>
After this change we'll get
Column<'abc'>
Column<'wąż'>
### How was this patch tested?
Existing tests and manual inspection.
Closes#30322 from zero323/SPARK-33415.
Authored-by: zero323 <mszymkiewicz@gmail.com>
Signed-off-by: HyukjinKwon <gurwls223@apache.org>
### What changes were proposed in this pull request?
This PR adds support for passing `Column`s as input to PySpark sorting functions.
### Why are the changes needed?
According to SPARK-26979, PySpark functions should support both Column and str arguments, when possible.
### Does this PR introduce _any_ user-facing change?
PySpark users can now provide both `Column` and `str` as an argument for `asc*` and `desc*` functions.
### How was this patch tested?
New unit tests.
Closes#30227 from zero323/SPARK-33257.
Authored-by: zero323 <mszymkiewicz@gmail.com>
Signed-off-by: HyukjinKwon <gurwls223@apache.org>
### What changes were proposed in this pull request?
As the Python evaluation consumes the parent iterator in a separate thread, it could consume more data from the parent even after the task ends and the parent is closed. Thus, we should use `ContextAwareIterator` to stop consuming after the task ends.
### Why are the changes needed?
Python/Pandas UDF right after off-heap vectorized reader could cause executor crash.
E.g.,:
```py
spark.range(0, 100000, 1, 1).write.parquet(path)
spark.conf.set("spark.sql.columnVector.offheap.enabled", True)
def f(x):
return 0
fUdf = udf(f, LongType())
spark.read.parquet(path).select(fUdf('id')).head()
```
This is because, the Python evaluation consumes the parent iterator in a separate thread and it consumes more data from the parent even after the task ends and the parent is closed. If an off-heap column vector exists in the parent iterator, it could cause segmentation fault which crashes the executor.
### Does this PR introduce _any_ user-facing change?
No.
### How was this patch tested?
Added tests, and manually.
Closes#30177 from ueshin/issues/SPARK-33277/python_pandas_udf.
Authored-by: Takuya UESHIN <ueshin@databricks.com>
Signed-off-by: HyukjinKwon <gurwls223@apache.org>
### What changes were proposed in this pull request?
This PR intends to fix bus for casting data from/to PythonUserDefinedType. A sequence of queries to reproduce this issue is as follows;
```
>>> from pyspark.sql import Row
>>> from pyspark.sql.functions import col
>>> from pyspark.sql.types import *
>>> from pyspark.testing.sqlutils import *
>>>
>>> row = Row(point=ExamplePoint(1.0, 2.0))
>>> df = spark.createDataFrame([row])
>>> df.select(col("point").cast(PythonOnlyUDT()))
Traceback (most recent call last):
File "<stdin>", line 1, in <module>
File "/Users/maropu/Repositories/spark/spark-master/python/pyspark/sql/dataframe.py", line 1402, in select
jdf = self._jdf.select(self._jcols(*cols))
File "/Users/maropu/Repositories/spark/spark-master/python/lib/py4j-0.10.9-src.zip/py4j/java_gateway.py", line 1305, in __call__
File "/Users/maropu/Repositories/spark/spark-master/python/pyspark/sql/utils.py", line 111, in deco
return f(*a, **kw)
File "/Users/maropu/Repositories/spark/spark-master/python/lib/py4j-0.10.9-src.zip/py4j/protocol.py", line 328, in get_return_value
py4j.protocol.Py4JJavaError: An error occurred while calling o44.select.
: java.lang.NullPointerException
at org.apache.spark.sql.types.UserDefinedType.acceptsType(UserDefinedType.scala:84)
at org.apache.spark.sql.catalyst.expressions.Cast$.canCast(Cast.scala:96)
at org.apache.spark.sql.catalyst.expressions.CastBase.checkInputDataTypes(Cast.scala:267)
at org.apache.spark.sql.catalyst.expressions.CastBase.resolved$lzycompute(Cast.scala:290)
at org.apache.spark.sql.catalyst.expressions.CastBase.resolved(Cast.scala:290)
```
A root cause of this issue is that, since `PythonUserDefinedType#userClassis` always null, `isAssignableFrom` in `UserDefinedType#acceptsType` throws a null exception. To fix it, this PR defines `acceptsType` in `PythonUserDefinedType` and filters out the null case in `UserDefinedType#acceptsType`.
### Why are the changes needed?
Bug fixes.
### Does this PR introduce _any_ user-facing change?
No.
### How was this patch tested?
Added tests.
Closes#30169 from maropu/FixPythonUDTCast.
Authored-by: Takeshi Yamamuro <yamamuro@apache.org>
Signed-off-by: Dongjoon Hyun <dhyun@apple.com>
### What changes were proposed in this pull request?
- [x] Expand dictionary definitions into standalone functions.
- [x] Fix annotations for ordering functions.
### Why are the changes needed?
To simplify further maintenance of docstrings.
### Does this PR introduce _any_ user-facing change?
No.
### How was this patch tested?
Existing tests.
Closes#30143 from zero323/SPARK-32084.
Authored-by: zero323 <mszymkiewicz@gmail.com>
Signed-off-by: HyukjinKwon <gurwls223@apache.org>
## What changes were proposed in this pull request?
Adds a SQL function `raise_error` which underlies the refactored `assert_true` function. `assert_true` now also (optionally) accepts a custom error message field.
`raise_error` is exposed in SQL, Python, Scala, and R.
`assert_true` was previously only exposed in SQL; it is now also exposed in Python, Scala, and R.
### Why are the changes needed?
Improves usability of `assert_true` by clarifying error messaging, and adds the useful helper function `raise_error`.
### Does this PR introduce _any_ user-facing change?
Yes:
- Adds `raise_error` function to the SQL, Python, Scala, and R APIs.
- Adds `assert_true` function to the SQL, Python and R APIs.
### How was this patch tested?
Adds unit tests in SQL, Python, Scala, and R for `assert_true` and `raise_error`.
Closes#29947 from karenfeng/spark-32793.
Lead-authored-by: Karen Feng <karen.feng@databricks.com>
Co-authored-by: Hyukjin Kwon <gurwls223@gmail.com>
Signed-off-by: HyukjinKwon <gurwls223@apache.org>
### What changes were proposed in this pull request?
This PR adds `dropFields` method to:
- PySpark `Column`
- SparkR `Column`
### Why are the changes needed?
Feature parity.
### Does this PR introduce _any_ user-facing change?
No, new API.
### How was this patch tested?
- New unit tests.
- Manual verification of examples / doctests.
- Manual run of MyPy tests
Closes#29967 from zero323/SPARK-32511-FOLLOW-UP-PYSPARK-SPARKR.
Authored-by: zero323 <mszymkiewicz@gmail.com>
Signed-off-by: HyukjinKwon <gurwls223@apache.org>
### What changes were proposed in this pull request?
This improves error handling when a failure in conversion from Pandas to Arrow occurs. And fixes tests to be compatible with upcoming Arrow 2.0.0 release.
### Why are the changes needed?
Current tests will fail with Arrow 2.0.0 because of a change in error message when the schema is invalid. For these cases, the current error message also includes information on disabling safe conversion config, which is mainly meant for floating point truncation and overflow. The tests have been updated to use a message that is show for past Arrow versions, and upcoming.
If the user enters an invalid schema, the error produced by pyarrow is not consistent and either `TypeError` or `ArrowInvalid`, with the latter being caught, and raised as a `RuntimeError` with the extra info.
The error handling is improved by:
- narrowing the exception type to `TypeError`s, which `ArrowInvalid` is a subclass and what is raised on safe conversion failures.
- The exception is only raised with additional information on disabling "spark.sql.execution.pandas.convertToArrowArraySafely" if it is enabled in the first place.
- The original exception is chained to better show it to the user.
### Does this PR introduce _any_ user-facing change?
Yes, the error re-raised changes from a RuntimeError to a ValueError, which better categorizes this type of error and in-line with the original Arrow error.
### How was this patch tested?
Existing tests, using pyarrow 1.0.1 and 2.0.0-snapshot
Closes#29951 from BryanCutler/arrow-better-handle-pandas-errors-SPARK-33073.
Authored-by: Bryan Cutler <cutlerb@gmail.com>
Signed-off-by: HyukjinKwon <gurwls223@apache.org>
### What changes were proposed in this pull request?
`nth_value` was added at SPARK-27951. This PR adds the corresponding PySpark API.
### Why are the changes needed?
To support the consistent APIs
### Does this PR introduce _any_ user-facing change?
Yes, it introduces a new PySpark function API.
### How was this patch tested?
Unittest was added.
Closes#29899 from HyukjinKwon/SPARK-33020.
Authored-by: HyukjinKwon <gurwls223@apache.org>
Signed-off-by: Dongjoon Hyun <dhyun@apple.com>
### What changes were proposed in this pull request?
Move functions related test cases from `test_context.py` to `test_functions.py`.
### Why are the changes needed?
To group the similar test cases.
### Does this PR introduce _any_ user-facing change?
Nope, test-only.
### How was this patch tested?
Jenkins and GitHub Actions should test.
Closes#29898 from HyukjinKwon/SPARK-33021.
Authored-by: HyukjinKwon <gurwls223@apache.org>
Signed-off-by: Dongjoon Hyun <dhyun@apple.com>
### What changes were proposed in this pull request?
This PR proposes migration of [`pyspark-stubs`](https://github.com/zero323/pyspark-stubs) into Spark codebase.
### Why are the changes needed?
### Does this PR introduce _any_ user-facing change?
Yes. This PR adds type annotations directly to Spark source.
This can impact interaction with development tools for users, which haven't used `pyspark-stubs`.
### How was this patch tested?
- [x] MyPy tests of the PySpark source
```
mypy --no-incremental --config python/mypy.ini python/pyspark
```
- [x] MyPy tests of Spark examples
```
MYPYPATH=python/ mypy --no-incremental --config python/mypy.ini examples/src/main/python/ml examples/src/main/python/sql examples/src/main/python/sql/streaming
```
- [x] Existing Flake8 linter
- [x] Existing unit tests
Tested against:
- `mypy==0.790+dev.e959952d9001e9713d329a2f9b196705b028f894`
- `mypy==0.782`
Closes#29591 from zero323/SPARK-32681.
Authored-by: zero323 <mszymkiewicz@gmail.com>
Signed-off-by: HyukjinKwon <gurwls223@apache.org>
### What changes were proposed in this pull request?
This PR adds a `withField` method on the pyspark Column class to call the Scala API method added in https://github.com/apache/spark/pull/27066.
### Why are the changes needed?
To update the Python API to match a new feature in the Scala API.
### Does this PR introduce _any_ user-facing change?
No.
### How was this patch tested?
New unit test
Closes#29699 from Kimahriman/feature/pyspark-with-field.
Authored-by: Adam Binford <adam.binford@radiantsolutions.com>
Signed-off-by: HyukjinKwon <gurwls223@apache.org>
### What changes were proposed in this pull request?
Change `CreateFunctionCommand` code that add class check before create function.
### Why are the changes needed?
We have different behavior between create permanent function and temporary function when function class is invaild. e.g.,
```
create function f as 'test.non.exists.udf';
-- Time taken: 0.104 seconds
create temporary function f as 'test.non.exists.udf'
-- Error in query: Can not load class 'test.non.exists.udf' when registering the function 'f', please make sure it is on the classpath;
```
And Hive also fails both of them.
### Does this PR introduce _any_ user-facing change?
Yes, user will get exception when create a invalid udf.
### How was this patch tested?
New test.
Closes#29502 from ulysses-you/function.
Authored-by: ulysses <youxiduo@weidian.com>
Signed-off-by: Wenchen Fan <wenchen@databricks.com>
https://issues.apache.org/jira/browse/SPARK-32719
### What changes were proposed in this pull request?
Add a check to detect missing imports. This makes sure that if we use a specific class, it should be explicitly imported (not using a wildcard).
### Why are the changes needed?
To make sure that the quality of the Python code is up to standard.
### Does this PR introduce _any_ user-facing change?
No
### How was this patch tested?
Existing unit-tests and Flake8 static analysis
Closes#29563 from Fokko/fd-add-check-missing-imports.
Authored-by: Fokko Driesprong <fokko@apache.org>
Signed-off-by: HyukjinKwon <gurwls223@apache.org>
### What changes were proposed in this pull request?
This is a follow up PR to #29328 to apply the same constraint where `path` option cannot coexist with path parameter to `DataFrameWriter.save()`, `DataStreamReader.load()` and `DataStreamWriter.start()`.
### Why are the changes needed?
The current behavior silently overwrites the `path` option if path parameter is passed to `DataFrameWriter.save()`, `DataStreamReader.load()` and `DataStreamWriter.start()`.
For example,
```
Seq(1).toDF.write.option("path", "/tmp/path1").parquet("/tmp/path2")
```
will write the result to `/tmp/path2`.
### Does this PR introduce _any_ user-facing change?
Yes, if `path` option coexists with path parameter to any of the above methods, it will throw `AnalysisException`:
```
scala> Seq(1).toDF.write.option("path", "/tmp/path1").parquet("/tmp/path2")
org.apache.spark.sql.AnalysisException: There is a 'path' option set and save() is called with a path parameter. Either remove the path option, or call save() without the parameter. To ignore this check, set 'spark.sql.legacy.pathOptionBehavior.enabled' to 'true'.;
```
The user can restore the previous behavior by setting `spark.sql.legacy.pathOptionBehavior.enabled` to `true`.
### How was this patch tested?
Added new tests.
Closes#29543 from imback82/path_option.
Authored-by: Terry Kim <yuminkim@gmail.com>
Signed-off-by: Wenchen Fan <wenchen@databricks.com>
### What changes were proposed in this pull request?
This PR enhances `Catalog.createTable()` to allow users to set the table's description. This corresponds to the following SQL syntax:
```sql
CREATE TABLE ...
COMMENT 'this is a fancy table';
```
### Why are the changes needed?
This brings the Scala/Python catalog APIs a bit closer to what's already possible via SQL.
### Does this PR introduce any user-facing change?
Yes, it adds a new parameter to `Catalog.createTable()`.
### How was this patch tested?
Existing unit tests:
```sh
./python/run-tests \
--python-executables python3.7 \
--testnames 'pyspark.sql.tests.test_catalog,pyspark.sql.tests.test_context'
```
```
$ ./build/sbt
testOnly org.apache.spark.sql.internal.CatalogSuite org.apache.spark.sql.CachedTableSuite org.apache.spark.sql.hive.MetastoreDataSourcesSuite org.apache.spark.sql.hive.execution.HiveDDLSuite
```
Closes#27908 from nchammas/SPARK-31000-table-description.
Authored-by: Nicholas Chammas <nicholas.chammas@liveramp.com>
Signed-off-by: HyukjinKwon <gurwls223@apache.org>
### What changes were proposed in this pull request?
add test_createDataFrame_empty_partition in pyspark arrow tests
### Why are the changes needed?
test edge cases.
### Does this PR introduce _any_ user-facing change?
no.
### How was this patch tested?
N/A
Closes#29398 from WeichenXu123/add_one_pyspark_arrow_test.
Authored-by: Weichen Xu <weichen.xu@databricks.com>
Signed-off-by: HyukjinKwon <gurwls223@apache.org>
Disallow the use of unused imports:
- Unnecessary increases the memory footprint of the application
- Removes the imports that are required for the examples in the docstring from the file-scope to the example itself. This keeps the files itself clean, and gives a more complete example as it also includes the imports :)
```
fokkodriesprongFan spark % flake8 python | grep -i "imported but unused"
python/pyspark/cloudpickle.py:46:1: F401 'functools.partial' imported but unused
python/pyspark/cloudpickle.py:55:1: F401 'traceback' imported but unused
python/pyspark/heapq3.py:868:5: F401 '_heapq.*' imported but unused
python/pyspark/__init__.py:61:1: F401 'pyspark.version.__version__' imported but unused
python/pyspark/__init__.py:62:1: F401 'pyspark._globals._NoValue' imported but unused
python/pyspark/__init__.py:115:1: F401 'pyspark.sql.SQLContext' imported but unused
python/pyspark/__init__.py:115:1: F401 'pyspark.sql.HiveContext' imported but unused
python/pyspark/__init__.py:115:1: F401 'pyspark.sql.Row' imported but unused
python/pyspark/rdd.py:21:1: F401 're' imported but unused
python/pyspark/rdd.py:29:1: F401 'tempfile.NamedTemporaryFile' imported but unused
python/pyspark/mllib/regression.py:26:1: F401 'pyspark.mllib.linalg.SparseVector' imported but unused
python/pyspark/mllib/clustering.py:28:1: F401 'pyspark.mllib.linalg.SparseVector' imported but unused
python/pyspark/mllib/clustering.py:28:1: F401 'pyspark.mllib.linalg.DenseVector' imported but unused
python/pyspark/mllib/classification.py:26:1: F401 'pyspark.mllib.linalg.SparseVector' imported but unused
python/pyspark/mllib/feature.py:28:1: F401 'pyspark.mllib.linalg.DenseVector' imported but unused
python/pyspark/mllib/feature.py:28:1: F401 'pyspark.mllib.linalg.SparseVector' imported but unused
python/pyspark/mllib/feature.py:30:1: F401 'pyspark.mllib.regression.LabeledPoint' imported but unused
python/pyspark/mllib/tests/test_linalg.py:18:1: F401 'sys' imported but unused
python/pyspark/mllib/tests/test_linalg.py:642:5: F401 'pyspark.mllib.tests.test_linalg.*' imported but unused
python/pyspark/mllib/tests/test_feature.py:21:1: F401 'numpy.random' imported but unused
python/pyspark/mllib/tests/test_feature.py:21:1: F401 'numpy.exp' imported but unused
python/pyspark/mllib/tests/test_feature.py:23:1: F401 'pyspark.mllib.linalg.Vector' imported but unused
python/pyspark/mllib/tests/test_feature.py:23:1: F401 'pyspark.mllib.linalg.VectorUDT' imported but unused
python/pyspark/mllib/tests/test_feature.py:185:5: F401 'pyspark.mllib.tests.test_feature.*' imported but unused
python/pyspark/mllib/tests/test_util.py:97:5: F401 'pyspark.mllib.tests.test_util.*' imported but unused
python/pyspark/mllib/tests/test_stat.py:23:1: F401 'pyspark.mllib.linalg.Vector' imported but unused
python/pyspark/mllib/tests/test_stat.py:23:1: F401 'pyspark.mllib.linalg.SparseVector' imported but unused
python/pyspark/mllib/tests/test_stat.py:23:1: F401 'pyspark.mllib.linalg.DenseVector' imported but unused
python/pyspark/mllib/tests/test_stat.py:23:1: F401 'pyspark.mllib.linalg.VectorUDT' imported but unused
python/pyspark/mllib/tests/test_stat.py:23:1: F401 'pyspark.mllib.linalg._convert_to_vector' imported but unused
python/pyspark/mllib/tests/test_stat.py:23:1: F401 'pyspark.mllib.linalg.DenseMatrix' imported but unused
python/pyspark/mllib/tests/test_stat.py:23:1: F401 'pyspark.mllib.linalg.SparseMatrix' imported but unused
python/pyspark/mllib/tests/test_stat.py:23:1: F401 'pyspark.mllib.linalg.MatrixUDT' imported but unused
python/pyspark/mllib/tests/test_stat.py:181:5: F401 'pyspark.mllib.tests.test_stat.*' imported but unused
python/pyspark/mllib/tests/test_streaming_algorithms.py:18:1: F401 'time.time' imported but unused
python/pyspark/mllib/tests/test_streaming_algorithms.py:18:1: F401 'time.sleep' imported but unused
python/pyspark/mllib/tests/test_streaming_algorithms.py:470:5: F401 'pyspark.mllib.tests.test_streaming_algorithms.*' imported but unused
python/pyspark/mllib/tests/test_algorithms.py:295:5: F401 'pyspark.mllib.tests.test_algorithms.*' imported but unused
python/pyspark/tests/test_serializers.py:90:13: F401 'xmlrunner' imported but unused
python/pyspark/tests/test_rdd.py:21:1: F401 'sys' imported but unused
python/pyspark/tests/test_rdd.py:29:1: F401 'pyspark.resource.ResourceProfile' imported but unused
python/pyspark/tests/test_rdd.py:885:5: F401 'pyspark.tests.test_rdd.*' imported but unused
python/pyspark/tests/test_readwrite.py:19:1: F401 'sys' imported but unused
python/pyspark/tests/test_readwrite.py:22:1: F401 'array.array' imported but unused
python/pyspark/tests/test_readwrite.py:309:5: F401 'pyspark.tests.test_readwrite.*' imported but unused
python/pyspark/tests/test_join.py:62:5: F401 'pyspark.tests.test_join.*' imported but unused
python/pyspark/tests/test_taskcontext.py:19:1: F401 'shutil' imported but unused
python/pyspark/tests/test_taskcontext.py:325:5: F401 'pyspark.tests.test_taskcontext.*' imported but unused
python/pyspark/tests/test_conf.py:36:5: F401 'pyspark.tests.test_conf.*' imported but unused
python/pyspark/tests/test_broadcast.py:148:5: F401 'pyspark.tests.test_broadcast.*' imported but unused
python/pyspark/tests/test_daemon.py:76:5: F401 'pyspark.tests.test_daemon.*' imported but unused
python/pyspark/tests/test_util.py:77:5: F401 'pyspark.tests.test_util.*' imported but unused
python/pyspark/tests/test_pin_thread.py:19:1: F401 'random' imported but unused
python/pyspark/tests/test_pin_thread.py:149:5: F401 'pyspark.tests.test_pin_thread.*' imported but unused
python/pyspark/tests/test_worker.py:19:1: F401 'sys' imported but unused
python/pyspark/tests/test_worker.py:26:5: F401 'resource' imported but unused
python/pyspark/tests/test_worker.py:203:5: F401 'pyspark.tests.test_worker.*' imported but unused
python/pyspark/tests/test_profiler.py:101:5: F401 'pyspark.tests.test_profiler.*' imported but unused
python/pyspark/tests/test_shuffle.py:18:1: F401 'sys' imported but unused
python/pyspark/tests/test_shuffle.py:171:5: F401 'pyspark.tests.test_shuffle.*' imported but unused
python/pyspark/tests/test_rddbarrier.py:43:5: F401 'pyspark.tests.test_rddbarrier.*' imported but unused
python/pyspark/tests/test_context.py:129:13: F401 'userlibrary.UserClass' imported but unused
python/pyspark/tests/test_context.py:140:13: F401 'userlib.UserClass' imported but unused
python/pyspark/tests/test_context.py:310:5: F401 'pyspark.tests.test_context.*' imported but unused
python/pyspark/tests/test_appsubmit.py:241:5: F401 'pyspark.tests.test_appsubmit.*' imported but unused
python/pyspark/streaming/dstream.py:18:1: F401 'sys' imported but unused
python/pyspark/streaming/tests/test_dstream.py:27:1: F401 'pyspark.RDD' imported but unused
python/pyspark/streaming/tests/test_dstream.py:647:5: F401 'pyspark.streaming.tests.test_dstream.*' imported but unused
python/pyspark/streaming/tests/test_kinesis.py:83:5: F401 'pyspark.streaming.tests.test_kinesis.*' imported but unused
python/pyspark/streaming/tests/test_listener.py:152:5: F401 'pyspark.streaming.tests.test_listener.*' imported but unused
python/pyspark/streaming/tests/test_context.py:178:5: F401 'pyspark.streaming.tests.test_context.*' imported but unused
python/pyspark/testing/utils.py:30:5: F401 'scipy.sparse' imported but unused
python/pyspark/testing/utils.py:36:5: F401 'numpy as np' imported but unused
python/pyspark/ml/regression.py:25:1: F401 'pyspark.ml.tree._TreeEnsembleParams' imported but unused
python/pyspark/ml/regression.py:25:1: F401 'pyspark.ml.tree._HasVarianceImpurity' imported but unused
python/pyspark/ml/regression.py:29:1: F401 'pyspark.ml.wrapper.JavaParams' imported but unused
python/pyspark/ml/util.py:19:1: F401 'sys' imported but unused
python/pyspark/ml/__init__.py:25:1: F401 'pyspark.ml.pipeline' imported but unused
python/pyspark/ml/pipeline.py:18:1: F401 'sys' imported but unused
python/pyspark/ml/stat.py:22:1: F401 'pyspark.ml.linalg.DenseMatrix' imported but unused
python/pyspark/ml/stat.py:22:1: F401 'pyspark.ml.linalg.Vectors' imported but unused
python/pyspark/ml/tests/test_training_summary.py:18:1: F401 'sys' imported but unused
python/pyspark/ml/tests/test_training_summary.py:364:5: F401 'pyspark.ml.tests.test_training_summary.*' imported but unused
python/pyspark/ml/tests/test_linalg.py:381:5: F401 'pyspark.ml.tests.test_linalg.*' imported but unused
python/pyspark/ml/tests/test_tuning.py:427:9: F401 'pyspark.sql.functions as F' imported but unused
python/pyspark/ml/tests/test_tuning.py:757:5: F401 'pyspark.ml.tests.test_tuning.*' imported but unused
python/pyspark/ml/tests/test_wrapper.py:120:5: F401 'pyspark.ml.tests.test_wrapper.*' imported but unused
python/pyspark/ml/tests/test_feature.py:19:1: F401 'sys' imported but unused
python/pyspark/ml/tests/test_feature.py:304:5: F401 'pyspark.ml.tests.test_feature.*' imported but unused
python/pyspark/ml/tests/test_image.py:19:1: F401 'py4j' imported but unused
python/pyspark/ml/tests/test_image.py:22:1: F401 'pyspark.testing.mlutils.PySparkTestCase' imported but unused
python/pyspark/ml/tests/test_image.py:71:5: F401 'pyspark.ml.tests.test_image.*' imported but unused
python/pyspark/ml/tests/test_persistence.py:456:5: F401 'pyspark.ml.tests.test_persistence.*' imported but unused
python/pyspark/ml/tests/test_evaluation.py:56:5: F401 'pyspark.ml.tests.test_evaluation.*' imported but unused
python/pyspark/ml/tests/test_stat.py:43:5: F401 'pyspark.ml.tests.test_stat.*' imported but unused
python/pyspark/ml/tests/test_base.py:70:5: F401 'pyspark.ml.tests.test_base.*' imported but unused
python/pyspark/ml/tests/test_param.py:20:1: F401 'sys' imported but unused
python/pyspark/ml/tests/test_param.py:375:5: F401 'pyspark.ml.tests.test_param.*' imported but unused
python/pyspark/ml/tests/test_pipeline.py:62:5: F401 'pyspark.ml.tests.test_pipeline.*' imported but unused
python/pyspark/ml/tests/test_algorithms.py:333:5: F401 'pyspark.ml.tests.test_algorithms.*' imported but unused
python/pyspark/ml/param/__init__.py:18:1: F401 'sys' imported but unused
python/pyspark/resource/tests/test_resources.py:17:1: F401 'random' imported but unused
python/pyspark/resource/tests/test_resources.py:20:1: F401 'pyspark.resource.ResourceProfile' imported but unused
python/pyspark/resource/tests/test_resources.py:75:5: F401 'pyspark.resource.tests.test_resources.*' imported but unused
python/pyspark/sql/functions.py:32:1: F401 'pyspark.sql.udf.UserDefinedFunction' imported but unused
python/pyspark/sql/functions.py:34:1: F401 'pyspark.sql.pandas.functions.pandas_udf' imported but unused
python/pyspark/sql/session.py:30:1: F401 'pyspark.sql.types.Row' imported but unused
python/pyspark/sql/session.py:30:1: F401 'pyspark.sql.types.StringType' imported but unused
python/pyspark/sql/readwriter.py:1084:5: F401 'pyspark.sql.Row' imported but unused
python/pyspark/sql/context.py:26:1: F401 'pyspark.sql.types.IntegerType' imported but unused
python/pyspark/sql/context.py:26:1: F401 'pyspark.sql.types.Row' imported but unused
python/pyspark/sql/context.py:26:1: F401 'pyspark.sql.types.StringType' imported but unused
python/pyspark/sql/context.py:27:1: F401 'pyspark.sql.udf.UDFRegistration' imported but unused
python/pyspark/sql/streaming.py:1212:5: F401 'pyspark.sql.Row' imported but unused
python/pyspark/sql/tests/test_utils.py:55:5: F401 'pyspark.sql.tests.test_utils.*' imported but unused
python/pyspark/sql/tests/test_pandas_map.py:18:1: F401 'sys' imported but unused
python/pyspark/sql/tests/test_pandas_map.py:22:1: F401 'pyspark.sql.functions.pandas_udf' imported but unused
python/pyspark/sql/tests/test_pandas_map.py:22:1: F401 'pyspark.sql.functions.PandasUDFType' imported but unused
python/pyspark/sql/tests/test_pandas_map.py:119:5: F401 'pyspark.sql.tests.test_pandas_map.*' imported but unused
python/pyspark/sql/tests/test_catalog.py:193:5: F401 'pyspark.sql.tests.test_catalog.*' imported but unused
python/pyspark/sql/tests/test_group.py:39:5: F401 'pyspark.sql.tests.test_group.*' imported but unused
python/pyspark/sql/tests/test_session.py:361:5: F401 'pyspark.sql.tests.test_session.*' imported but unused
python/pyspark/sql/tests/test_conf.py:49:5: F401 'pyspark.sql.tests.test_conf.*' imported but unused
python/pyspark/sql/tests/test_pandas_cogrouped_map.py:19:1: F401 'sys' imported but unused
python/pyspark/sql/tests/test_pandas_cogrouped_map.py:21:1: F401 'pyspark.sql.functions.sum' imported but unused
python/pyspark/sql/tests/test_pandas_cogrouped_map.py:21:1: F401 'pyspark.sql.functions.PandasUDFType' imported but unused
python/pyspark/sql/tests/test_pandas_cogrouped_map.py:29:5: F401 'pandas.util.testing.assert_series_equal' imported but unused
python/pyspark/sql/tests/test_pandas_cogrouped_map.py:32:5: F401 'pyarrow as pa' imported but unused
python/pyspark/sql/tests/test_pandas_cogrouped_map.py:248:5: F401 'pyspark.sql.tests.test_pandas_cogrouped_map.*' imported but unused
python/pyspark/sql/tests/test_udf.py:24:1: F401 'py4j' imported but unused
python/pyspark/sql/tests/test_pandas_udf_typehints.py:246:5: F401 'pyspark.sql.tests.test_pandas_udf_typehints.*' imported but unused
python/pyspark/sql/tests/test_functions.py:19:1: F401 'sys' imported but unused
python/pyspark/sql/tests/test_functions.py:362:9: F401 'pyspark.sql.functions.exists' imported but unused
python/pyspark/sql/tests/test_functions.py:387:5: F401 'pyspark.sql.tests.test_functions.*' imported but unused
python/pyspark/sql/tests/test_pandas_udf_scalar.py:21:1: F401 'sys' imported but unused
python/pyspark/sql/tests/test_pandas_udf_scalar.py:45:5: F401 'pyarrow as pa' imported but unused
python/pyspark/sql/tests/test_pandas_udf_window.py:355:5: F401 'pyspark.sql.tests.test_pandas_udf_window.*' imported but unused
python/pyspark/sql/tests/test_arrow.py:38:5: F401 'pyarrow as pa' imported but unused
python/pyspark/sql/tests/test_pandas_grouped_map.py:20:1: F401 'sys' imported but unused
python/pyspark/sql/tests/test_pandas_grouped_map.py:38:5: F401 'pyarrow as pa' imported but unused
python/pyspark/sql/tests/test_dataframe.py:382:9: F401 'pyspark.sql.DataFrame' imported but unused
python/pyspark/sql/avro/functions.py:125:5: F401 'pyspark.sql.Row' imported but unused
python/pyspark/sql/pandas/functions.py:19:1: F401 'sys' imported but unused
```
After:
```
fokkodriesprongFan spark % flake8 python | grep -i "imported but unused"
fokkodriesprongFan spark %
```
### What changes were proposed in this pull request?
Removing unused imports from the Python files to keep everything nice and tidy.
### Why are the changes needed?
Cleaning up of the imports that aren't used, and suppressing the imports that are used as references to other modules, preserving backward compatibility.
### Does this PR introduce _any_ user-facing change?
No.
### How was this patch tested?
Adding the rule to the existing Flake8 checks.
Closes#29121 from Fokko/SPARK-32319.
Authored-by: Fokko Driesprong <fokko@apache.org>
Signed-off-by: Dongjoon Hyun <dongjoon@apache.org>
### What changes were proposed in this pull request?
The current error message from `_infer_type` in `_infer_schema` only includes the unsupported column type but not the column name. This PR adds the column name in the error message to make it easier for users to identify which column should they drop or convert.
### Why are the changes needed?
Improve user experience.
### Does this PR introduce _any_ user-facing change?
Yes. The error message from `_infer_schema` is changed.
Before:
"not supported type: foo"
After:
"Column bar contains not supported type: foo"
### How was this patch tested?
Updated the existing unit test.
Closes#29365 from liangz1/types-error-colname.
Authored-by: Liang Zhang <liang.zhang@databricks.com>
Signed-off-by: HyukjinKwon <gurwls223@apache.org>
### What changes were proposed in this pull request?
This is a follow-up of #29138 which added overload `slice` function to accept `Column` for `start` and `length` in Scala.
This PR is updating the equivalent Python function to accept `Column` as well.
### Why are the changes needed?
Now that Scala version accepts `Column`, Python version should also accept it.
### Does this PR introduce _any_ user-facing change?
Yes, PySpark users will also be able to pass Column object to `start` and `length` parameter in `slice` function.
### How was this patch tested?
Added tests.
Closes#29195 from ueshin/issues/SPARK-32338/slice.
Authored-by: Takuya UESHIN <ueshin@databricks.com>
Signed-off-by: HyukjinKwon <gurwls223@apache.org>
### What changes were proposed in this pull request?
- Adds `DataFramWriterV2` class.
- Adds `writeTo` method to `pyspark.sql.DataFrame`.
- Adds related SQL partitioning functions (`years`, `months`, ..., `bucket`).
### Why are the changes needed?
Feature parity.
### Does this PR introduce any user-facing change?
No.
### How was this patch tested?
Added new unit tests.
TODO: Should we test against `org.apache.spark.sql.connector.InMemoryTableCatalog`? If so, how to expose it in Python tests?
Closes#27331 from zero323/SPARK-29157.
Authored-by: zero323 <mszymkiewicz@gmail.com>
Signed-off-by: HyukjinKwon <gurwls223@apache.org>
### What changes were proposed in this pull request?
This PR proposes to port the test case from https://github.com/apache/spark/pull/29098 to branch-3.0 and master. In the master and branch-3.0, this was fixed together at ecaa495b1f but no partition case is not being tested.
### Why are the changes needed?
To improve test coverage.
### Does this PR introduce _any_ user-facing change?
No, test-only.
### How was this patch tested?
Unit test was forward-ported.
Closes#29099 from HyukjinKwon/SPARK-32300-1.
Authored-by: HyukjinKwon <gurwls223@apache.org>
Signed-off-by: HyukjinKwon <gurwls223@apache.org>
### What changes were proposed in this pull request?
`datetime` is already imported a few lines below :)
ce27cc54c1/python/pyspark/sql/tests/test_pandas_udf_scalar.py (L24)
### Why are the changes needed?
This is the last instance of the duplicate import.
### Does this PR introduce _any_ user-facing change?
No.
### How was this patch tested?
Manual.
Closes#29109 from Fokko/SPARK-32311.
Authored-by: Fokko Driesprong <fokko@apache.org>
Signed-off-by: Dongjoon Hyun <dongjoon@apache.org>
### What changes were proposed in this pull request?
This PR aims to drop Python 2.7, 3.4 and 3.5.
Roughly speaking, it removes all the widely known Python 2 compatibility workarounds such as `sys.version` comparison, `__future__`. Also, it removes the Python 2 dedicated codes such as `ArrayConstructor` in Spark.
### Why are the changes needed?
1. Unsupport EOL Python versions
2. Reduce maintenance overhead and remove a bit of legacy codes and hacks for Python 2.
3. PyPy2 has a critical bug that causes a flaky test, SPARK-28358 given my testing and investigation.
4. Users can use Python type hints with Pandas UDFs without thinking about Python version
5. Users can leverage one latest cloudpickle, https://github.com/apache/spark/pull/28950. With Python 3.8+ it can also leverage C pickle.
### Does this PR introduce _any_ user-facing change?
Yes, users cannot use Python 2.7, 3.4 and 3.5 in the upcoming Spark version.
### How was this patch tested?
Manually tested and also tested in Jenkins.
Closes#28957 from HyukjinKwon/SPARK-32138.
Authored-by: HyukjinKwon <gurwls223@apache.org>
Signed-off-by: HyukjinKwon <gurwls223@apache.org>
### What changes were proposed in this pull request?
This PR aims to run the Spark tests in Github Actions.
To briefly explain the main idea:
- Reuse `dev/run-tests.py` with SBT build
- Reuse the modules in `dev/sparktestsupport/modules.py` to test each module
- Pass the modules to test into `dev/run-tests.py` directly via `TEST_ONLY_MODULES` environment variable. For example, `pyspark-sql,core,sql,hive`.
- `dev/run-tests.py` _does not_ take the dependent modules into account but solely the specified modules to test.
Another thing to note might be `SlowHiveTest` annotation. Running the tests in Hive modules takes too much so the slow tests are extracted and it runs as a separate job. It was extracted from the actual elapsed time in Jenkins:
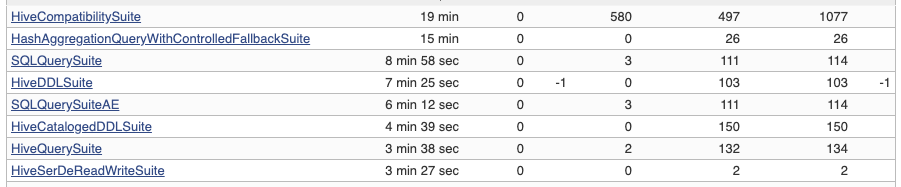
So, Hive tests are separated into to jobs. One is slow test cases, and the other one is the other test cases.
_Note that_ the current GitHub Actions build virtually copies what the default PR builder on Jenkins does (without other profiles such as JDK 11, Hadoop 2, etc.). The only exception is Kinesis https://github.com/apache/spark/pull/29057/files#diff-04eb107ee163a50b61281ca08f4e4c7bR23
### Why are the changes needed?
Last week and onwards, the Jenkins machines became very unstable for many reasons:
- Apparently, the machines became extremely slow. Almost all tests can't pass.
- One machine (worker 4) started to have the corrupt `.m2` which fails the build.
- Documentation build fails time to time for an unknown reason in Jenkins machine specifically. This is disabled for now at https://github.com/apache/spark/pull/29017.
- Almost all PRs are basically blocked by this instability currently.
The advantages of using Github Actions:
- To avoid depending on few persons who can access to the cluster.
- To reduce the elapsed time in the build - we could split the tests (e.g., SQL, ML, CORE), and run them in parallel so the total build time will significantly reduce.
- To control the environment more flexibly.
- Other contributors can test and propose to fix Github Actions configurations so we can distribute this build management cost.
Note that:
- The current build in Jenkins takes _more than 7 hours_. With Github actions it takes _less than 2 hours_
- We can now control the environments especially for Python easily.
- The test and build look more stable than the Jenkins'.
### Does this PR introduce _any_ user-facing change?
No, dev-only change.
### How was this patch tested?
Tested at https://github.com/HyukjinKwon/spark/pull/4Closes#29057 from HyukjinKwon/migrate-to-github-actions.
Authored-by: HyukjinKwon <gurwls223@apache.org>
Signed-off-by: Dongjoon Hyun <dongjoon@apache.org>
### What changes were proposed in this pull request?
Improve the error message in test GroupedMapInPandasTests.test_grouped_over_window_with_key to show the incorrect values.
### Why are the changes needed?
This test failure has come up often in Arrow testing because it tests a struct with timestamp values through a Pandas UDF. The current error message is not helpful as it doesn't show the incorrect values, only that it failed. This change will instead raise an assertion error with the incorrect values on a failure.
Before:
```
======================================================================
FAIL: test_grouped_over_window_with_key (pyspark.sql.tests.test_pandas_grouped_map.GroupedMapInPandasTests)
----------------------------------------------------------------------
Traceback (most recent call last):
File "/spark/python/pyspark/sql/tests/test_pandas_grouped_map.py", line 588, in test_grouped_over_window_with_key
self.assertTrue(all([r[0] for r in result]))
AssertionError: False is not true
```
After:
```
======================================================================
ERROR: test_grouped_over_window_with_key (pyspark.sql.tests.test_pandas_grouped_map.GroupedMapInPandasTests)
----------------------------------------------------------------------
...
AssertionError: {'start': datetime.datetime(2018, 3, 20, 0, 0), 'end': datetime.datetime(2018, 3, 25, 0, 0)}, != {'start': datetime.datetime(2020, 3, 20, 0, 0), 'end': datetime.datetime(2020, 3, 25, 0, 0)}
```
### Does this PR introduce _any_ user-facing change?
No
### How was this patch tested?
Improved existing test
Closes#28987 from BryanCutler/pandas-grouped-map-test-output-SPARK-32162.
Authored-by: Bryan Cutler <cutlerb@gmail.com>
Signed-off-by: HyukjinKwon <gurwls223@apache.org>