### What changes were proposed in this pull request?
This patch fixes the missed spot - the test initializes FileStreamSinkLog with its "output" directory instead of "metadata" directory, hence the verification against sink log was no-op.
### Why are the changes needed?
Without the fix, the verification against sink log was no-op.
### Does this PR introduce _any_ user-facing change?
No.
### How was this patch tested?
Checked with debugger in test, and verified `allFiles()` returns non-zero entries. (It returned zero entry, as there's no metadata.)
Closes#28930 from HeartSaVioR/SPARK-29999-FOLLOWUP-fix-test.
Authored-by: Jungtaek Lim (HeartSaVioR) <kabhwan.opensource@gmail.com>
Signed-off-by: Wenchen Fan <wenchen@databricks.com>
### What changes were proposed in this pull request?
This PR tries to unify the method `getReader` and `getReaderForRange` in `ShuffleManager`.
### Why are the changes needed?
Reduce the duplicate codes, simplify the implementation, and for better maintenance.
### Does this PR introduce _any_ user-facing change?
No.
### How was this patch tested?
Covered by existing tests.
Closes#28895 from Ngone51/unify-getreader.
Authored-by: yi.wu <yi.wu@databricks.com>
Signed-off-by: Wenchen Fan <wenchen@databricks.com>
### What changes were proposed in this pull request?
This patch proposes to coalesce partitions for repartition by expressions without specifying number of partitions, when AQE is enabled.
### Why are the changes needed?
When repartition by some partition expressions, users can specify number of partitions or not. If the number of partitions is specified, we should not coalesce partitions because it breaks user expectation. But if without specifying number of partitions, AQE should be able to coalesce partitions as other shuffling.
### Does this PR introduce _any_ user-facing change?
Yes. After this change, if users don't specify the number of partitions when repartitioning data by expressions, AQE will coalesce partitions.
### How was this patch tested?
Added unit test.
Closes#28900 from viirya/SPARK-32056.
Authored-by: Liang-Chi Hsieh <viirya@gmail.com>
Signed-off-by: Wenchen Fan <wenchen@databricks.com>
### What changes were proposed in this pull request?
This is a followup of https://github.com/apache/spark/pull/28876/files
This PR proposes to use the name of the original expression, as the alias name of the normalization expression.
### Why are the changes needed?
make the query plan looks pretty when EXPLAIN.
### Does this PR introduce _any_ user-facing change?
No
### How was this patch tested?
manually explain the query
Closes#28919 from cloud-fan/follow.
Authored-by: Wenchen Fan <wenchen@databricks.com>
Signed-off-by: Dongjoon Hyun <dongjoon@apache.org>
### What changes were proposed in this pull request?
This PR fix `UserDefinedType.equal()` by comparing the UDT class instead of checking `acceptsType()`.
### Why are the changes needed?
It's weird that equality comparison between two UDT types can have different result by switching the order:
```scala
// ExampleSubTypeUDT.userClass is a subclass of ExampleBaseTypeUDT.userClass
val udt1 = new ExampleBaseTypeUDT
val udt2 = new ExampleSubTypeUDT
println(udt1 == udt2) // true
println(udt2 == udt1) // false
```
### Does this PR introduce _any_ user-facing change?
Yes.
Before:
```scala
// ExampleSubTypeUDT.userClass is a subclass of ExampleBaseTypeUDT.userClass
val udt1 = new ExampleBaseTypeUDT
val udt2 = new ExampleSubTypeUDT
println(udt1 == udt2) // true
println(udt2 == udt1) // false
```
After:
```scala
// ExampleSubTypeUDT.userClass is a subclass of ExampleBaseTypeUDT.userClass
val udt1 = new ExampleBaseTypeUDT
val udt2 = new ExampleSubTypeUDT
println(udt1 == udt2) // false
println(udt2 == udt1) // false
```
### How was this patch tested?
Added a unit test.
Closes#28923 from Ngone51/fix-udt-equal.
Authored-by: yi.wu <yi.wu@databricks.com>
Signed-off-by: Dongjoon Hyun <dongjoon@apache.org>
### What changes were proposed in this pull request?
The `optimizedPlan` in IncrementalExecution should also be scoped in `withActive`.
### Why are the changes needed?
Follow-up of SPARK-30798 for the Streaming side.
### Does this PR introduce _any_ user-facing change?
No.
### How was this patch tested?
Existing UT.
Closes#28936 from xuanyuanking/SPARK-30798-follow.
Authored-by: Yuanjian Li <xyliyuanjian@gmail.com>
Signed-off-by: Dongjoon Hyun <dongjoon@apache.org>
### What changes were proposed in this pull request?
Add benchmarks for interval constructor `make_interval` and measure perf of 4 cases:
1. Constant (year, month)
2. Constant (week, day)
3. Constant (hour, minute, second, second fraction)
4. All fields are NOT constant.
The benchmark results are generated in the environment:
| Item | Description |
| ---- | ----|
| Region | us-west-2 (Oregon) |
| Instance | r3.xlarge |
| AMI | ubuntu/images/hvm-ssd/ubuntu-bionic-18.04-amd64-server-20190722.1 (ami-06f2f779464715dc5) |
| Java | OpenJDK 64-Bit Server VM 1.8.0_252 and OpenJDK 64-Bit Server VM 11.0.7+10 |
### Why are the changes needed?
To have a base line for future perf improvements of `make_interval`, and to prevent perf regressions in the future.
### Does this PR introduce _any_ user-facing change?
No
### How was this patch tested?
By running `IntervalBenchmark` via:
```
$ SPARK_GENERATE_BENCHMARK_FILES=1 build/sbt "sql/test:runMain org.apache.spark.sql.execution.benchmark.IntervalBenchmark"
```
Closes#28905 from MaxGekk/benchmark-make_interval.
Authored-by: Max Gekk <max.gekk@gmail.com>
Signed-off-by: Dongjoon Hyun <dongjoon@apache.org>
### What changes were proposed in this pull request?
Add American timezone during timestamp_seconds doctest
### Why are the changes needed?
`timestamp_seconds` doctest in `functions.py` used default timezone to get expected result
For example:
```python
>>> time_df = spark.createDataFrame([(1230219000,)], ['unix_time'])
>>> time_df.select(timestamp_seconds(time_df.unix_time).alias('ts')).collect()
[Row(ts=datetime.datetime(2008, 12, 25, 7, 30))]
```
But when we have a non-american timezone, the test case will get different test result.
For example, when we set current timezone as `Asia/Shanghai`, the test result will be
```
[Row(ts=datetime.datetime(2008, 12, 25, 23, 30))]
```
So no matter where we run the test case ,we will always get the expected permanent result if we set the timezone on one specific area.
### Does this PR introduce _any_ user-facing change?
No
### How was this patch tested?
Unit test
Closes#28932 from GuoPhilipse/SPARK-32088-fix-timezone-issue.
Lead-authored-by: GuoPhilipse <46367746+GuoPhilipse@users.noreply.github.com>
Co-authored-by: GuoPhilipse <guofei_ok@126.com>
Signed-off-by: Dongjoon Hyun <dongjoon@apache.org>
### What changes were proposed in this pull request?
This pull request fixes a bug present in the csv type inference.
We have problems when we have different types in the same column.
**Previously:**
```
$ cat /example/f1.csv
col1
43200000
true
spark.read.csv(path="file:///example/*.csv", header=True, inferSchema=True).show()
+----+
|col1|
+----+
|null|
|true|
+----+
root
|-- col1: boolean (nullable = true)
```
**Now**
```
spark.read.csv(path="file:///example/*.csv", header=True, inferSchema=True).show()
+-------------+
|col1 |
+-------------+
|43200000 |
|true |
+-------------+
root
|-- col1: string (nullable = true)
```
Previously the hierarchy of type inference is the following:
> IntegerType
> > LongType
> > > DecimalType
> > > > DoubleType
> > > > > TimestampType
> > > > > > BooleanType
> > > > > > > StringType
So, when, for example, we have integers in one column, and the last element is a boolean, all the column is inferred as a boolean column incorrectly and all the number are shown as null when you see the data
We need the following hierarchy. When we have different numeric types in the column it will be resolved correctly. And when we have other different types it will be resolved as a String type column
> IntegerType
> > LongType
> > > DecimalType
> > > > DoubleType
> > > > > StringType
> TimestampType
> > StringType
> BooleanType
> > StringType
> StringType
### Why are the changes needed?
Fix the bug explained
### Does this PR introduce _any_ user-facing change?
No
### How was this patch tested?
Unit test and manual tests
Closes#28896 from planga82/feature/SPARK-32025_csv_inference.
Authored-by: Pablo Langa <soypab@gmail.com>
Signed-off-by: HyukjinKwon <gurwls223@apache.org>
### What changes were proposed in this pull request?
This PR tries to address the comment: https://github.com/apache/spark/pull/28645#discussion_r442183888
It changes `canUpCast/canCast` to allow cast from sub UDT to base UDT, in order to achieve the goal to allow UserDefinedType to use `ExpressionEncoder` to deserialize rows in ScalaUDF as well.
One thing that needs to mention is, even we allow cast from sub UDT to base UDT, it doesn't really do the cast in `Cast`. Because, yet, sub UDT and base UDT are considered as the same type(because of #16660), see:
5264164a67/sql/catalyst/src/main/scala/org/apache/spark/sql/types/UserDefinedType.scala (L81-L86)5264164a67/sql/catalyst/src/main/scala/org/apache/spark/sql/types/UserDefinedType.scala (L92-L95)
Therefore, the optimize rule `SimplifyCast` will eliminate the cast at the end.
### Why are the changes needed?
Reduce the special case caused by `UserDefinedType` in `ResolveEncodersInUDF` and `ScalaUDF`.
### Does this PR introduce _any_ user-facing change?
No.
### How was this patch tested?
It should be covered by the test of `SPARK-19311`, which is also updated a little in this PR.
Closes#28920 from Ngone51/fix-udf-udt.
Authored-by: yi.wu <yi.wu@databricks.com>
Signed-off-by: Wenchen Fan <wenchen@databricks.com>
### What changes were proposed in this pull request?
Reset listenerRegistered when application end.
### Why are the changes needed?
Within a jvm, stop and create `SparkContext` multi times will cause the bug.
### Does this PR introduce _any_ user-facing change?
No.
### How was this patch tested?
Add UT.
Closes#28899 from ulysses-you/SPARK-32062.
Authored-by: ulysses <youxiduo@weidian.com>
Signed-off-by: Wenchen Fan <wenchen@databricks.com>
### What changes were proposed in this pull request?
Replace Decimal by Int op in the `MakeInterval` & `MakeTimestamp` expression. For instance, `(secs * Decimal(MICROS_PER_SECOND)).toLong` can be replaced by the unscaled long because the former one already contains microseconds.
### Why are the changes needed?
To improve performance.
Before:
```
make_timestamp(): Best Time(ms) Avg Time(ms) Stdev(ms) Rate(M/s) Per Row(ns) Relative
------------------------------------------------------------------------------------------------------------------------
...
make_timestamp(2019, 1, 2, 3, 4, 50.123456) 94 99 4 10.7 93.8 38.8X
```
After:
```
make_timestamp(2019, 1, 2, 3, 4, 50.123456) 76 92 15 13.1 76.5 48.1X
```
### Does this PR introduce _any_ user-facing change?
No
### How was this patch tested?
- By existing test suites `IntervalExpressionsSuite`, `DateExpressionsSuite` and etc.
- Re-generate results of `MakeDateTimeBenchmark` in the environment:
| Item | Description |
| ---- | ----|
| Region | us-west-2 (Oregon) |
| Instance | r3.xlarge |
| AMI | ubuntu/images/hvm-ssd/ubuntu-bionic-18.04-amd64-server-20190722.1 (ami-06f2f779464715dc5) |
| Java | OpenJDK 64-Bit Server VM 1.8.0_252 and OpenJDK 64-Bit Server VM 11.0.7+10 |
Closes#28886 from MaxGekk/make_interval-opt-decimal.
Authored-by: Max Gekk <max.gekk@gmail.com>
Signed-off-by: Wenchen Fan <wenchen@databricks.com>
### What changes were proposed in this pull request?
This PR changes the references of the `PartialMerge`/`Final` `AggregateExpression` from `aggBufferAttributes` to `inputAggBufferAttributes`.
After this change, the tests of `SPARK-31620` can fail on the assertion of `QueryTest.assertEmptyMissingInput`. So, this PR also fixes it by overriding the `inputAggBufferAttributes` of the Aggregate operators.
### Why are the changes needed?
With my understanding of Aggregate framework, especially, according to the logic of `AggUtils.planAggXXX`, I think for the `PartialMerge`/`Final` `AggregateExpression` the right references should be `inputAggBufferAttributes`.
### Does this PR introduce _any_ user-facing change?
No.
### How was this patch tested?
Before this patch, for an Aggregate operator, its input attributes will always be equal to or more than(because it refers to its own attributes while it should refer to the attributes from the child) its reference attributes. Therefore, its missing inputs must always be empty and break nothing. Thus, it's impossible to add a UT for this patch.
However, after correcting the right references in this PR, the problem is then exposed by `QueryTest.assertEmptyMissingInput` in the UT of SPARK-31620, since missing inputs are no longer always empty. This PR can fix the problem.
Closes#28869 from Ngone51/fix-agg-reference.
Authored-by: yi.wu <yi.wu@databricks.com>
Signed-off-by: Wenchen Fan <wenchen@databricks.com>
### What changes were proposed in this pull request?
This patch applies `NormalizeFloatingNumbers` to distinct aggregate to fix a regression of distinct aggregate on NaNs.
### Why are the changes needed?
We added `NormalizeFloatingNumbers` optimization rule in 3.0.0 to normalize special floating numbers (NaN and -0.0). But it is missing in distinct aggregate so causes a regression. We need to apply this rule on distinct aggregate to fix it.
### Does this PR introduce _any_ user-facing change?
Yes, fixing a regression of distinct aggregate on NaNs.
### How was this patch tested?
Added unit test.
Closes#28876 from viirya/SPARK-32038.
Authored-by: Liang-Chi Hsieh <viirya@gmail.com>
Signed-off-by: Dongjoon Hyun <dongjoon@apache.org>
### What changes were proposed in this pull request?
Add compatibility tests for streaming state store format.
### Why are the changes needed?
After SPARK-31894, we have a validation checking for the streaming state store. It's better to add integrated tests in the PR builder as soon as the breaking changes introduced.
### Does this PR introduce _any_ user-facing change?
No.
### How was this patch tested?
Test only.
Closes#28725 from xuanyuanking/compatibility_check.
Authored-by: Yuanjian Li <xyliyuanjian@gmail.com>
Signed-off-by: Wenchen Fan <wenchen@databricks.com>
### What changes were proposed in this pull request?
Add a new config `spark.sql.files.minPartitionNum` to control file split partition in local session.
### Why are the changes needed?
Aims to control file split partitions in session level.
More details see discuss in [PR-28778](https://github.com/apache/spark/pull/28778).
### Does this PR introduce _any_ user-facing change?
Yes, new config.
### How was this patch tested?
Add UT.
Closes#28853 from ulysses-you/SPARK-32019.
Authored-by: ulysses <youxiduo@weidian.com>
Signed-off-by: Dongjoon Hyun <dongjoon@apache.org>
### What changes were proposed in this pull request?
Change precision of seconds and its fraction from 8 to 18 to be able to construct intervals of max allowed microseconds value (long).
### Why are the changes needed?
To improve UX of Spark SQL.
### Does this PR introduce _any_ user-facing change?
Yes
### How was this patch tested?
- Add tests to IntervalExpressionsSuite
- Add an example to the `MakeInterval` expression
- Add tests to `interval.sql`
Closes#28873 from MaxGekk/make_interval-sec-precision.
Authored-by: Max Gekk <max.gekk@gmail.com>
Signed-off-by: Dongjoon Hyun <dongjoon@apache.org>
### What changes were proposed in this pull request?
When two bucketed tables with different number of buckets are joined, it can introduce a full shuffle:
```
spark.conf.set("spark.sql.autoBroadcastJoinThreshold", "0")
val df1 = (0 until 20).map(i => (i % 5, i % 13, i.toString)).toDF("i", "j", "k")
val df2 = (0 until 20).map(i => (i % 7, i % 11, i.toString)).toDF("i", "j", "k")
df1.write.format("parquet").bucketBy(8, "i").saveAsTable("t1")
df2.write.format("parquet").bucketBy(4, "i").saveAsTable("t2")
val t1 = spark.table("t1")
val t2 = spark.table("t2")
val joined = t1.join(t2, t1("i") === t2("i"))
joined.explain
== Physical Plan ==
*(5) SortMergeJoin [i#44], [i#50], Inner
:- *(2) Sort [i#44 ASC NULLS FIRST], false, 0
: +- Exchange hashpartitioning(i#44, 200), true, [id=#105]
: +- *(1) Project [i#44, j#45, k#46]
: +- *(1) Filter isnotnull(i#44)
: +- *(1) ColumnarToRow
: +- FileScan parquet default.t1[i#44,j#45,k#46] Batched: true, DataFilters: [isnotnull(i#44)], Format: Parquet, Location: InMemoryFileIndex[...], PartitionFilters: [], PushedFilters: [IsNotNull(i)], ReadSchema: struct<i:int,j:int,k:string>, SelectedBucketsCount: 8 out of 8
+- *(4) Sort [i#50 ASC NULLS FIRST], false, 0
+- Exchange hashpartitioning(i#50, 200), true, [id=#115]
+- *(3) Project [i#50, j#51, k#52]
+- *(3) Filter isnotnull(i#50)
+- *(3) ColumnarToRow
+- FileScan parquet default.t2[i#50,j#51,k#52] Batched: true, DataFilters: [isnotnull(i#50)], Format: Parquet, Location: InMemoryFileIndex[...], PartitionFilters: [], PushedFilters: [IsNotNull(i)], ReadSchema: struct<i:int,j:int,k:string>, SelectedBucketsCount: 4 out of 4
```
This PR proposes to introduce coalescing buckets when the following conditions are met to eliminate the full shuffle:
- Join is the sort merge one (which is created only for equi-join).
- Join keys match with output partition expressions on their respective sides.
- The larger bucket number is divisible by the smaller bucket number.
- `spark.sql.bucketing.coalesceBucketsInSortMergeJoin.enabled` is set to `true`.
- The ratio of the number of buckets should be less than the value set in `spark.sql.bucketing.coalesceBucketsInSortMergeJoin.maxBucketRatio`.
### Why are the changes needed?
Eliminating the full shuffle can benefit for scenarios where two large tables are joined. Especially when the tables are already bucketed but differ in the number of buckets, we could take advantage of it.
### Does this PR introduce any user-facing change?
If the bucket coalescing conditions explained above are met, a full shuffle can be eliminated (also note that you will see `SelectedBucketsCount: 8 out of 8 (Coalesced to 4)` in the physical plan):
```
spark.conf.set("spark.sql.autoBroadcastJoinThreshold", "0")
spark.conf.set("spark.sql.bucketing.coalesceBucketsInSortMergeJoin.enabled", "true")
val df1 = (0 until 20).map(i => (i % 5, i % 13, i.toString)).toDF("i", "j", "k")
val df2 = (0 until 20).map(i => (i % 7, i % 11, i.toString)).toDF("i", "j", "k")
df1.write.format("parquet").bucketBy(8, "i").saveAsTable("t1")
df2.write.format("parquet").bucketBy(4, "i").saveAsTable("t2")
val t1 = spark.table("t1")
val t2 = spark.table("t2")
val joined = t1.join(t2, t1("i") === t2("i"))
joined.explain
== Physical Plan ==
*(3) SortMergeJoin [i#44], [i#50], Inner
:- *(1) Sort [i#44 ASC NULLS FIRST], false, 0
: +- *(1) Project [i#44, j#45, k#46]
: +- *(1) Filter isnotnull(i#44)
: +- *(1) ColumnarToRow
: +- FileScan parquet default.t1[i#44,j#45,k#46] Batched: true, DataFilters: [isnotnull(i#44)], Format: Parquet, Location: InMemoryFileIndex[...], PartitionFilters: [], PushedFilters: [IsNotNull(i)], ReadSchema: struct<i:int,j:int,k:string>, SelectedBucketsCount: 8 out of 8 (Coalesced to 4)
+- *(2) Sort [i#50 ASC NULLS FIRST], false, 0
+- *(2) Project [i#50, j#51, k#52]
+- *(2) Filter isnotnull(i#50)
+- *(2) ColumnarToRow
+- FileScan parquet default.t2[i#50,j#51,k#52] Batched: true, DataFilters: [isnotnull(i#50)], Format: Parquet, Location: InMemoryFileIndex[...], PartitionFilters: [], PushedFilters: [IsNotNull(i)], ReadSchema: struct<i:int,j:int,k:string>, SelectedBucketsCount: 4 out of 4
```
### How was this patch tested?
Added unit tests
Closes#28123 from imback82/coalescing_bucket.
Authored-by: Terry Kim <yuminkim@gmail.com>
Signed-off-by: Takeshi Yamamuro <yamamuro@apache.org>
### What changes were proposed in this pull request?
This PR adds support for typed Scala UDF to accept composed type of case class, e.g. Seq[T], Array[T], Map[Int, T] (assuming T is case class type), as input parameter type.
### Why are the changes needed?
After #27937, typed Scala UDF now has supported case class as its input parameter type. However, it can not accept the composed type of case class, such as Seq[T], Array[T], Map[Int, T] (assuming T is case class type), which causing confuse(e.g. https://github.com/apache/spark/pull/27937#discussion_r422699979) to the user.
### Does this PR introduce _any_ user-facing change?
Yes.
Run the query:
```
scala> case class Person(name: String, age: Int)
scala> Seq((1, Seq(Person("Jack", 5)))).toDF("id", "persons").withColumn("ages", udf{ s: Seq[Person] => s.head.age }.apply(col("persons"))).show
```
Before:
```
org.apache.spark.SparkException: Failed to execute user defined function($read$$Lambda$2861/628175152: (array<struct<name:string,age:int>>) => int)
at org.apache.spark.sql.catalyst.expressions.ScalaUDF.eval(ScalaUDF.scala:1129)
at org.apache.spark.sql.catalyst.expressions.Alias.eval(namedExpressions.scala:156)
at org.apache.spark.sql.catalyst.expressions.InterpretedMutableProjection.apply(InterpretedMutableProjection.scala:83)
at org.apache.spark.sql.catalyst.optimizer.ConvertToLocalRelation$$anonfun$apply$17.$anonfun$applyOrElse$69(Optimizer.scala:1492)
at scala.collection.TraversableLike.$anonfun$map$1(TraversableLike.scala:238)
....
Caused by: java.lang.ClassCastException: org.apache.spark.sql.catalyst.expressions.GenericRowWithSchema cannot be cast to Person
at $anonfun$res3$1(<console>:30)
at $anonfun$res3$1$adapted(<console>:30)
at org.apache.spark.sql.catalyst.expressions.ScalaUDF.$anonfun$f$2(ScalaUDF.scala:156)
at org.apache.spark.sql.catalyst.expressions.ScalaUDF.eval(ScalaUDF.scala:1126)
... 142 more
```
After:
```
+---+-----------+----+
| id| persons|ages|
+---+-----------+----+
| 1|[[Jack, 5]]| [5]|
+---+-----------+----+
```
### How was this patch tested?
Added tests.
Closes#28645 from Ngone51/impr-udf.
Authored-by: yi.wu <yi.wu@databricks.com>
Signed-off-by: Wenchen Fan <wenchen@databricks.com>
### What changes were proposed in this pull request?
Introduce UnsafeRow format validation for streaming state store.
### Why are the changes needed?
Currently, Structured Streaming directly puts the UnsafeRow into StateStore without any schema validation. It's a dangerous behavior when users reusing the checkpoint file during migration. Any changes or bug fix related to the aggregate function may cause random exceptions, even the wrong answer, e.g SPARK-28067.
### Does this PR introduce _any_ user-facing change?
Yes. If the underlying changes are detected when the checkpoint is reused during migration, the InvalidUnsafeRowException will be thrown.
### How was this patch tested?
UT added. Will also add integrated tests for more scenario in another PR separately.
Closes#28707 from xuanyuanking/SPARK-31894.
Lead-authored-by: Yuanjian Li <xyliyuanjian@gmail.com>
Co-authored-by: Yuanjian Li <yuanjian.li@databricks.com>
Signed-off-by: Wenchen Fan <wenchen@databricks.com>
### What changes were proposed in this pull request?
1. Move TimeZoneUTC and TimeZoneGMT to DateTimeTestUtils
2. Remove TimeZoneGMT
3. Use ZoneId.systemDefault() instead of defaultTimeZone().toZoneId
4. Alias SQLDate & SQLTimestamp to internal types of DateType and TimestampType
5. Avoid one `*` `DateTimeUtils`.`in fromJulianDay()`
6. Use toTotalMonths in `DateTimeUtils`.`subtractDates()`
7. Remove `julianCommonEraStart`, `timestampToString()`, `microsToEpochDays()`, `epochDaysToMicros()`, `instantToDays()` from `DateTimeUtils`.
8. Make splitDate() private.
9. Remove `def daysToMicros(days: Int): Long` and `def microsToDays(micros: Long): Int`.
### Why are the changes needed?
This simplifies the common code related to date-time operations, and should improve maintainability. In particular:
1. TimeZoneUTC and TimeZoneGMT are moved to DateTimeTestUtils because they are used only in tests
2. TimeZoneGMT can be removed because it is equal to TimeZoneUTC
3. After the PR #27494, Spark expressions and DateTimeUtils functions switched to ZoneId instead of TimeZone completely. `defaultTimeZone()` with `TimeZone` as return type is not needed anymore.
4. SQLDate and SQLTimestamp types can be explicitly aliased to internal types of DateType and and TimestampType instead of declaring this in a comment.
5. Avoid one `*` `DateTimeUtils`.`in fromJulianDay()`.
6. Use toTotalMonths in `DateTimeUtils`.`subtractDates()`.
### Does this PR introduce any user-facing change?
No
### How was this patch tested?
By existing test suites
Closes#27617 from MaxGekk/move-time-zone-consts.
Lead-authored-by: Max Gekk <max.gekk@gmail.com>
Co-authored-by: Maxim Gekk <max.gekk@gmail.com>
Signed-off-by: Wenchen Fan <wenchen@databricks.com>
### What changes were proposed in this pull request?
Better error message when SPARK_HOME or spark,test.home is not set.
### Why are the changes needed?
Currently the error message is not easily consumable as it prints (see below) the real error after printing the current environment which is rather long.
**Old output**
`
time.name" -> "Java(TM) SE Runtime Environment", "sun.boot.library.path" -> "/Library/Java/JavaVirtualMachines/jdk1.8.0_221.jdk/Contents/Home/jre/lib",
"java.vm.version" -> "25.221-b11",
. . .
. . .
. . .
) did not contain key "SPARK_HOME" spark.test.home or SPARK_HOME is not set.
at org.scalatest.Assertions.newAssertionFailedExceptio
`
**New output**
An exception or error caused a run to abort: spark.test.home or SPARK_HOME is not set.
org.scalatest.exceptions.TestFailedException: spark.test.home or SPARK_HOME is not set
### Does this PR introduce any user-facing change?
`
No.
### How was this patch tested?
Ran the tests in intellej manually to see the new error.
Closes#28825 from dilipbiswal/minor-spark-31950-followup.
Authored-by: Dilip Biswal <dkbiswal@gmail.com>
Signed-off-by: HyukjinKwon <gurwls223@apache.org>
### What changes were proposed in this pull request?
1. Add method `getTimeFormatters` to `HiveResult` which creates timestamp and date formatters.
2. Move creation of `dateFormatter` and `timestampFormatter` from the constructor of the `HiveResult` object to `HiveResult. hiveResultString()` via `getTimeFormatters`. This allows to resolve time zone ID from Spark's session time zone `spark.sql.session.timeZone` and create date/timestamp formatters only once before collecting `java.sql.Timestamp`/`java.sql.Date` values.
3. Create date/timestamp formatters once in SparkExecuteStatementOperation.
### Why are the changes needed?
To fix perf regression comparing to Spark 2.4
### Does this PR introduce _any_ user-facing change?
No
### How was this patch tested?
- By existing test suite `HiveResultSuite` and etc.
- Re-generate benchmarks results of `DateTimeBenchmark` in the environment:
| Item | Description |
| ---- | ----|
| Region | us-west-2 (Oregon) |
| Instance | r3.xlarge |
| AMI | ubuntu/images/hvm-ssd/ubuntu-bionic-18.04-amd64-server-20190722.1 (ami-06f2f779464715dc5) |
| Java | OpenJDK 64-Bit Server VM 1.8.0_252 and OpenJDK 64-Bit Server VM 11.0.7+10 |
Closes#28842 from MaxGekk/opt-toHiveString-oss-master.
Authored-by: Max Gekk <max.gekk@gmail.com>
Signed-off-by: Wenchen Fan <wenchen@databricks.com>
### What changes were proposed in this pull request?
When loading DataFrames from JDBC datasource with Kerberos authentication, remote executors (yarn-client/cluster etc. modes) fail to establish a connection due to lack of Kerberos ticket or ability to generate it.
This is a real issue when trying to ingest data from kerberized data sources (SQL Server, Oracle) in enterprise environment where exposing simple authentication access is not an option due to IT policy issues.
In this PR I've added MS SQL support.
What this PR contains:
* Added `MSSQLConnectionProvider`
* Added `MSSQLConnectionProviderSuite`
* Changed MS SQL JDBC driver to use the latest (test scope only)
* Changed `MsSqlServerIntegrationSuite` docker image to use the latest
* Added a version comment to `MariaDBConnectionProvider` to increase trackability
### Why are the changes needed?
Missing JDBC kerberos support.
### Does this PR introduce _any_ user-facing change?
Yes, now user is able to connect to MS SQL using kerberos.
### How was this patch tested?
* Additional + existing unit tests
* Existing integration tests
* Test on cluster manually
Closes#28635 from gaborgsomogyi/SPARK-31337.
Authored-by: Gabor Somogyi <gabor.g.somogyi@gmail.com>
Signed-off-by: Marcelo Vanzin <vanzin@apache.org>
### What changes were proposed in this pull request?
Replace `CAST(... AS TIMESTAMP` by `TIMESTAMP_SECONDS` in the following benchmarks:
- ExtractBenchmark
- DateTimeBenchmark
- FilterPushdownBenchmark
- InExpressionBenchmark
### Why are the changes needed?
The benchmarks fail w/o the changes:
```
[info] Running benchmark: datetime +/- interval
[info] Running case: date + interval(m)
[error] Exception in thread "main" org.apache.spark.sql.AnalysisException: cannot resolve 'CAST(`id` AS TIMESTAMP)' due to data type mismatch: cannot cast bigint to timestamp,you can enable the casting by setting spark.sql.legacy.allowCastNumericToTimestamp to true,but we strongly recommend using function TIMESTAMP_SECONDS/TIMESTAMP_MILLIS/TIMESTAMP_MICROS instead.; line 1 pos 5;
[error] 'Project [(cast(cast(id#0L as timestamp) as date) + 1 months) AS (CAST(CAST(id AS TIMESTAMP) AS DATE) + INTERVAL '1 months')#2]
[error] +- Range (0, 10000000, step=1, splits=Some(1))
```
### Does this PR introduce _any_ user-facing change?
No
### How was this patch tested?
By running the affected benchmarks.
Closes#28843 from MaxGekk/GuoPhilipse-31710-fix-compatibility-followup.
Authored-by: Max Gekk <max.gekk@gmail.com>
Signed-off-by: Wenchen Fan <wenchen@databricks.com>
## What changes were proposed in this pull request?
we fail casting from numeric to timestamp by default.
## Why are the changes needed?
casting from numeric to timestamp is not a non-standard,meanwhile it may generate different result between spark and other systems,for example hive
## Does this PR introduce any user-facing change?
Yes,user cannot cast numeric to timestamp directly,user have to use the following function to achieve the same effect:TIMESTAMP_SECONDS/TIMESTAMP_MILLIS/TIMESTAMP_MICROS
## How was this patch tested?
unit test added
Closes#28593 from GuoPhilipse/31710-fix-compatibility.
Lead-authored-by: GuoPhilipse <guofei_ok@126.com>
Co-authored-by: GuoPhilipse <46367746+GuoPhilipse@users.noreply.github.com>
Signed-off-by: Wenchen Fan <wenchen@databricks.com>
### What changes were proposed in this pull request?
This PR renames the variable from "numLateInputs" to "numRowsDroppedByWatermark" so that it becomes self-explanation.
### Why are the changes needed?
This is originated from post-review, see https://github.com/apache/spark/pull/28607#discussion_r439853232
### Does this PR introduce _any_ user-facing change?
No, as SPARK-24634 is not introduced in any release yet.
### How was this patch tested?
Existing UTs.
Closes#28828 from HeartSaVioR/SPARK-24634-v3-followup.
Authored-by: Jungtaek Lim (HeartSaVioR) <kabhwan.opensource@gmail.com>
Signed-off-by: HyukjinKwon <gurwls223@apache.org>
### What changes were proposed in this pull request?
This PR partially revert SPARK-31292 in order to provide a hot-fix for a bug in `Dataset.dropDuplicates`; we must preserve the input order of `colNames` for `groupCols` because the Streaming's state store depends on the `groupCols` order.
### Why are the changes needed?
Bug fix.
### Does this PR introduce _any_ user-facing change?
No.
### How was this patch tested?
Added tests in `DataFrameSuite`.
Closes#28830 from maropu/SPARK-31990.
Authored-by: Takeshi Yamamuro <yamamuro@apache.org>
Signed-off-by: Dongjoon Hyun <dongjoon@apache.org>
### What changes were proposed in this pull request?
- Modify `DateTimeRebaseBenchmark` to benchmark the default date-time rebasing mode - `EXCEPTION` for saving/loading dates/timestamps from/to parquet files. The mode is benchmarked for modern timestamps after 1900-01-01 00:00:00Z and dates after 1582-10-15.
- Regenerate benchmark results in the environment:
| Item | Description |
| ---- | ----|
| Region | us-west-2 (Oregon) |
| Instance | r3.xlarge |
| AMI | ubuntu/images/hvm-ssd/ubuntu-bionic-18.04-amd64-server-20190722.1 (ami-06f2f779464715dc5) |
| Java | OpenJDK 64-Bit Server VM 1.8.0_252 and OpenJDK 64-Bit Server VM 11.0.7+10 |
### Why are the changes needed?
The `EXCEPTION` rebasing mode is the default mode of the SQL configs `spark.sql.legacy.parquet.datetimeRebaseModeInRead` and `spark.sql.legacy.parquet.datetimeRebaseModeInWrite`. The changes are needed to improve benchmark coverage for default settings.
### Does this PR introduce _any_ user-facing change?
No
### How was this patch tested?
By running the benchmark and check results manually.
Closes#28829 from MaxGekk/benchmark-exception-mode.
Authored-by: Max Gekk <max.gekk@gmail.com>
Signed-off-by: Wenchen Fan <wenchen@databricks.com>
### What changes were proposed in this pull request?
In #28485 pagination support for tables of Structured Streaming Tab was added.
It missed 2 things:
* For sorting duration column, `String` was used which sometimes gives wrong results(consider `"3 ms"` and `"12 ms"`). Now we first sort the duration column and then convert it to readable String
* Status column was not made sortable.
### Why are the changes needed?
To fix the wrong result for sorting and making Status column sortable.
### Does this PR introduce _any_ user-facing change?
No
### How was this patch tested?
After changes:
<img width="1677" alt="Screenshot 2020-06-08 at 2 18 48 PM" src="https://user-images.githubusercontent.com/15366835/84010992-153fa280-a993-11ea-9846-bf176f2ec5d7.png">
Closes#28752 from iRakson/ssTests.
Authored-by: iRakson <raksonrakesh@gmail.com>
Signed-off-by: Sean Owen <srowen@gmail.com>
### What changes were proposed in this pull request?
Please refer https://issues.apache.org/jira/browse/SPARK-24634 to see rationalization of the issue.
This patch adds a new metric to count the number of inputs arrived later than watermark plus allowed delay. To make changes simpler, this patch doesn't count the exact number of input rows which are later than watermark plus allowed delay. Instead, this patch counts the inputs which are dropped in the logic of operator. The difference of twos are shown in streaming aggregation: to optimize the calculation, streaming aggregation "pre-aggregates" the input rows, and later checks the lateness against "pre-aggregated" inputs, hence the number might be reduced.
The new metric will be provided via two places:
1. On Spark UI: check the metrics in stateful operator nodes in query execution details page in SQL tab
2. On Streaming Query Listener: check "numLateInputs" in "stateOperators" in QueryProcessEvent.
### Why are the changes needed?
Dropping late inputs means that end users might not get expected outputs. Even end users may indicate the fact and tolerate the result (as that's what allowed lateness is for), but they should be able to observe whether the current value of allowed lateness drops inputs or not so that they can adjust the value.
Also, whatever the chance they have multiple of stateful operators in a single query, if Spark drops late inputs "between" these operators, it becomes "correctness" issue. Spark should disallow such possibility, but given we already provided the flexibility, at least we should provide the way to observe the correctness issue and decide whether they should make correction of their query or not.
### Does this PR introduce _any_ user-facing change?
Yes. End users will be able to retrieve the information of late inputs via two ways:
1. SQL tab in Spark UI
2. Streaming Query Listener
### How was this patch tested?
New UTs added & existing UTs are modified to reflect the change.
And ran manual test reproducing SPARK-28094.
I've picked the specific case on "B outer C outer D" which is enough to represent the "intermediate late row" issue due to global watermark.
https://gist.github.com/jammann/b58bfbe0f4374b89ecea63c1e32c8f17
Spark logs warning message on the query which means SPARK-28074 is working correctly,
```
20/05/30 17:52:47 WARN UnsupportedOperationChecker: Detected pattern of possible 'correctness' issue due to global watermark. The query contains stateful operation which can emit rows older than the current watermark plus allowed late record delay, which are "late rows" in downstream stateful operations and these rows can be discarded. Please refer the programming guide doc for more details.;
Join LeftOuter, ((D_FK#28 = D_ID#87) AND (B_LAST_MOD#26-T30000ms = D_LAST_MOD#88-T30000ms))
:- Join LeftOuter, ((C_FK#27 = C_ID#58) AND (B_LAST_MOD#26-T30000ms = C_LAST_MOD#59-T30000ms))
: :- EventTimeWatermark B_LAST_MOD#26: timestamp, 30 seconds
: : +- Project [v#23.B_ID AS B_ID#25, v#23.B_LAST_MOD AS B_LAST_MOD#26, v#23.C_FK AS C_FK#27, v#23.D_FK AS D_FK#28]
: : +- Project [from_json(StructField(B_ID,StringType,false), StructField(B_LAST_MOD,TimestampType,false), StructField(C_FK,StringType,true), StructField(D_FK,StringType,true), value#21, Some(UTC)) AS v#23]
: : +- Project [cast(value#8 as string) AS value#21]
: : +- StreamingRelationV2 org.apache.spark.sql.kafka010.KafkaSourceProvider3a7fd18c, kafka, org.apache.spark.sql.kafka010.KafkaSourceProvider$KafkaTable396d2958, org.apache.spark.sql.util.CaseInsensitiveStringMapa51ee61a, [key#7, value#8, topic#9, partition#10, offset#11L, timestamp#12, timestampType#13], StreamingRelation DataSource(org.apache.spark.sql.SparkSessiond221af8,kafka,List(),None,List(),None,Map(inferSchema -> true, startingOffsets -> earliest, subscribe -> B, kafka.bootstrap.servers -> localhost:9092),None), kafka, [key#0, value#1, topic#2, partition#3, offset#4L, timestamp#5, timestampType#6]
: +- EventTimeWatermark C_LAST_MOD#59: timestamp, 30 seconds
: +- Project [v#56.C_ID AS C_ID#58, v#56.C_LAST_MOD AS C_LAST_MOD#59]
: +- Project [from_json(StructField(C_ID,StringType,false), StructField(C_LAST_MOD,TimestampType,false), value#54, Some(UTC)) AS v#56]
: +- Project [cast(value#41 as string) AS value#54]
: +- StreamingRelationV2 org.apache.spark.sql.kafka010.KafkaSourceProvider3f507373, kafka, org.apache.spark.sql.kafka010.KafkaSourceProvider$KafkaTable7b6736a4, org.apache.spark.sql.util.CaseInsensitiveStringMapa51ee61b, [key#40, value#41, topic#42, partition#43, offset#44L, timestamp#45, timestampType#46], StreamingRelation DataSource(org.apache.spark.sql.SparkSessiond221af8,kafka,List(),None,List(),None,Map(inferSchema -> true, startingOffsets -> earliest, subscribe -> C, kafka.bootstrap.servers -> localhost:9092),None), kafka, [key#33, value#34, topic#35, partition#36, offset#37L, timestamp#38, timestampType#39]
+- EventTimeWatermark D_LAST_MOD#88: timestamp, 30 seconds
+- Project [v#85.D_ID AS D_ID#87, v#85.D_LAST_MOD AS D_LAST_MOD#88]
+- Project [from_json(StructField(D_ID,StringType,false), StructField(D_LAST_MOD,TimestampType,false), value#83, Some(UTC)) AS v#85]
+- Project [cast(value#70 as string) AS value#83]
+- StreamingRelationV2 org.apache.spark.sql.kafka010.KafkaSourceProvider2b90e779, kafka, org.apache.spark.sql.kafka010.KafkaSourceProvider$KafkaTable36f8cd29, org.apache.spark.sql.util.CaseInsensitiveStringMapa51ee620, [key#69, value#70, topic#71, partition#72, offset#73L, timestamp#74, timestampType#75], StreamingRelation DataSource(org.apache.spark.sql.SparkSessiond221af8,kafka,List(),None,List(),None,Map(inferSchema -> true, startingOffsets -> earliest, subscribe -> D, kafka.bootstrap.servers -> localhost:9092),None), kafka, [key#62, value#63, topic#64, partition#65, offset#66L, timestamp#67, timestampType#68]
```
and we can find the late inputs from the batch 4 as follows:
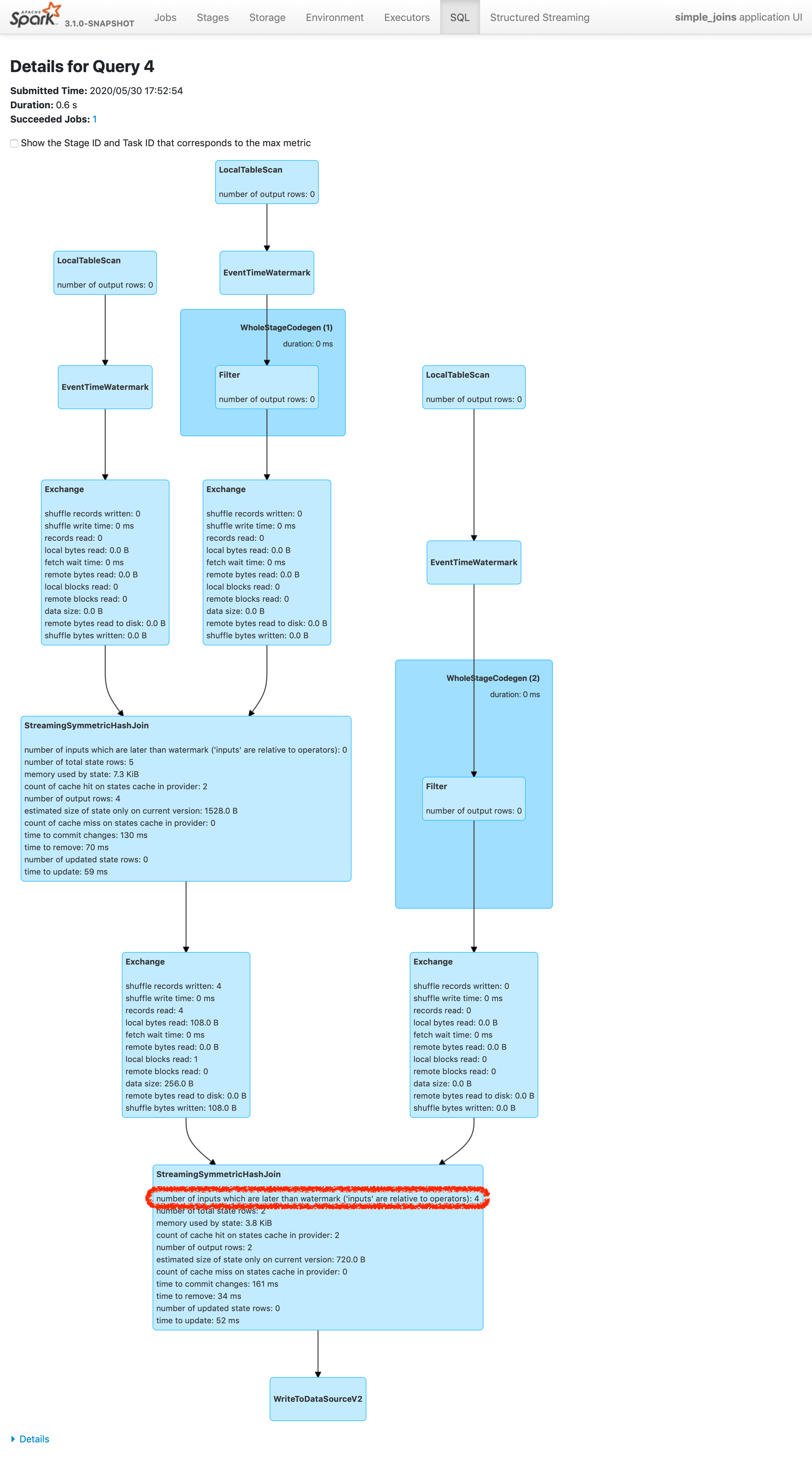
which represents intermediate inputs are being lost, ended up with correctness issue.
Closes#28607 from HeartSaVioR/SPARK-24634-v3.
Authored-by: Jungtaek Lim (HeartSaVioR) <kabhwan.opensource@gmail.com>
Signed-off-by: HyukjinKwon <gurwls223@apache.org>
### What changes were proposed in this pull request?
A unit test is added
Partition duplicate check added in `org.apache.spark.sql.execution.datasources.PartitioningUtils#validatePartitionColumn`
### Why are the changes needed?
When people write data with duplicate partition column, it will cause a `org.apache.spark.sql.AnalysisException: Found duplicate column ...` in loading data from the writted.
### Does this PR introduce _any_ user-facing change?
Yes.
It will prevent people from using duplicate partition columns to write data.
1. Before the PR:
It will look ok at `df.write.partitionBy("b", "b").csv("file:///tmp/output")`,
but get an exception when read:
`spark.read.csv("file:///tmp/output").show()`
org.apache.spark.sql.AnalysisException: Found duplicate column(s) in the partition schema: `b`;
2. After the PR:
`df.write.partitionBy("b", "b").csv("file:///tmp/output")` will trigger the exception:
org.apache.spark.sql.AnalysisException: Found duplicate column(s) b, b: `b`;
### How was this patch tested?
Unit test.
Closes#28814 from TJX2014/master-SPARK-31968.
Authored-by: TJX2014 <xiaoxingstack@gmail.com>
Signed-off-by: Dongjoon Hyun <dongjoon@apache.org>
### What changes were proposed in this pull request?
Currently we only push nested column pruning through a few operators such as LIMIT, SAMPLE, etc. This patch extends the feature to other operators including RepartitionByExpression, Join.
### Why are the changes needed?
Currently nested column pruning only applied on a few operators. It limits the benefit of nested column pruning. Extending nested column pruning coverage to make this feature more generally applied through different queries.
### Does this PR introduce _any_ user-facing change?
Yes. More SQL operators are covered by nested column pruning.
### How was this patch tested?
Added unit test, end-to-end tests.
Closes#28556 from viirya/others-column-pruning.
Authored-by: Liang-Chi Hsieh <viirya@gmail.com>
Signed-off-by: HyukjinKwon <gurwls223@apache.org>
### What changes were proposed in this pull request?
This PR upgrades HtmlUnit.
Selenium and Jetty also upgraded because of dependency.
### Why are the changes needed?
Recently, a security issue which affects HtmlUnit is reported.
https://nvd.nist.gov/vuln/detail/CVE-2020-5529
According to the report, arbitrary code can be run by malicious users.
HtmlUnit is used for test so the impact might not be large but it's better to upgrade it just in case.
### Does this PR introduce _any_ user-facing change?
No.
### How was this patch tested?
Existing testcases.
Closes#28585 from sarutak/upgrade-htmlunit.
Authored-by: Kousuke Saruta <sarutak@oss.nttdata.com>
Signed-off-by: Sean Owen <srowen@gmail.com>
### What changes were proposed in this pull request?
This is a followup of https://github.com/apache/spark/pull/23388 .
https://github.com/apache/spark/pull/23388 has an issue: it doesn't handle subquery expressions and assumes they will be turned into joins. However, this is not true for non-correlated subquery expressions.
This PR fixes this issue. It now doesn't skip `Subquery`, and subquery expressions will be handled by `OptimizeSubqueries`, which runs the optimizer with the subquery.
Note that, correlated subquery expressions will be handled twice: once in `OptimizeSubqueries`, once later when it becomes join. This is OK as `NormalizeFloatingNumbers` is idempotent now.
### Why are the changes needed?
fix a bug
### Does this PR introduce _any_ user-facing change?
yes, see the newly added test.
### How was this patch tested?
new test
Closes#28785 from cloud-fan/normalize.
Authored-by: Wenchen Fan <wenchen@databricks.com>
Signed-off-by: Wenchen Fan <wenchen@databricks.com>
### What changes were proposed in this pull request?
This PR proposes to remove package private in classes/objects in sql.execution package, as per SPARK-16964.
### Why are the changes needed?
This is per post-hoc review comment, see https://github.com/apache/spark/pull/24996#discussion_r437126445
### Does this PR introduce _any_ user-facing change?
No.
### How was this patch tested?
N/A
Closes#28790 from HeartSaVioR/SPARK-28199-FOLLOWUP-apply-SPARK-16964.
Authored-by: Jungtaek Lim (HeartSaVioR) <kabhwan.opensource@gmail.com>
Signed-off-by: Dongjoon Hyun <dongjoon@apache.org>
### What changes were proposed in this pull request?
This PR updates the test case to accept Hadoop 2/3 error message correctly.
### Why are the changes needed?
SPARK-31935(#28760) breaks Hadoop 3.2 UT because Hadoop 2 and Hadoop 3 have different exception messages.
In https://github.com/apache/spark/pull/28791, there are two test suites missed the fix
### Does this PR introduce _any_ user-facing change?
No
### How was this patch tested?
Unit test
Closes#28796 from gengliangwang/SPARK-31926-followup.
Authored-by: Gengliang Wang <gengliang.wang@databricks.com>
Signed-off-by: Dongjoon Hyun <dongjoon@apache.org>
### What changes were proposed in this pull request?
This reverts commit b9737c3c22 while keeping following changes
* set default value of `spark.sql.adaptive.skewJoin.skewedPartitionFactor` to 5
* improve tests
* remove unused imports
### Why are the changes needed?
As discussed in https://github.com/apache/spark/pull/28669#issuecomment-641044531, revert SPARK-31864 for optimizing skew join to work for extremely clustered keys.
### Does this PR introduce _any_ user-facing change?
No.
### How was this patch tested?
Existing tests.
Closes#28770 from manuzhang/spark-31942.
Authored-by: manuzhang <owenzhang1990@gmail.com>
Signed-off-by: Wenchen Fan <wenchen@databricks.com>
### What changes were proposed in this pull request?
If a datetime pattern contains no year field, the day of year field should not be ignored if exists
e.g.
```
spark-sql> select to_timestamp('31', 'DD');
1970-01-01 00:00:00
spark-sql> select to_timestamp('31 30', 'DD dd');
1970-01-30 00:00:00
spark.sql.legacy.timeParserPolicy legacy
spark-sql> select to_timestamp('31', 'DD');
1970-01-31 00:00:00
spark-sql> select to_timestamp('31 30', 'DD dd');
NULL
```
This PR only fixes some corner cases that use 'D' pattern to parse datetimes and there is w/o 'y'.
### Why are the changes needed?
fix some corner cases
### Does this PR introduce _any_ user-facing change?
yes, the day of year field will not be ignored
### How was this patch tested?
add unit tests.
Closes#28766 from yaooqinn/SPARK-31939.
Authored-by: Kent Yao <yaooqinn@hotmail.com>
Signed-off-by: Wenchen Fan <wenchen@databricks.com>
### What changes were proposed in this pull request?
This PR updates the test case to accept Hadoop 2/3 error message correctly.
### Why are the changes needed?
SPARK-31935(https://github.com/apache/spark/pull/28760) breaks Hadoop 3.2 UT because Hadoop 2 and Hadoop 3 have different exception messages.
### Does this PR introduce _any_ user-facing change?
No.
### How was this patch tested?
Pass the Jenkins with both Hadoop 2/3 or do the following manually.
**Hadoop 2.7**
```
$ build/sbt "sql/testOnly *.FileBasedDataSourceSuite -- -z SPARK-31935"
...
[info] All tests passed.
```
**Hadoop 3.2**
```
$ build/sbt "sql/testOnly *.FileBasedDataSourceSuite -- -z SPARK-31935" -Phadoop-3.2
...
[info] All tests passed.
```
Closes#28791 from dongjoon-hyun/SPARK-31935.
Authored-by: Dongjoon Hyun <dongjoon@apache.org>
Signed-off-by: Dongjoon Hyun <dongjoon@apache.org>
### What changes were proposed in this pull request?
This is another approach to fix the issue. See the previous try https://github.com/apache/spark/pull/28745. It was too invasive so I took more conservative approach.
This PR proposes to resolve grouping attributes separately first so it can be properly referred when `FlatMapGroupsInPandas` and `FlatMapCoGroupsInPandas` are resolved without ambiguity.
Previously,
```python
from pyspark.sql.functions import *
df = spark.createDataFrame([[1, 1]], ["column", "Score"])
pandas_udf("column integer, Score float", PandasUDFType.GROUPED_MAP)
def my_pandas_udf(pdf):
return pdf.assign(Score=0.5)
df.groupby('COLUMN').apply(my_pandas_udf).show()
```
was failed as below:
```
pyspark.sql.utils.AnalysisException: "Reference 'COLUMN' is ambiguous, could be: COLUMN, COLUMN.;"
```
because the unresolved `COLUMN` in `FlatMapGroupsInPandas` doesn't know which reference to take from the child projection.
After this fix, it resolves the child projection first with grouping keys and pass, to `FlatMapGroupsInPandas`, the attribute as a grouping key from the child projection that is positionally selected.
### Why are the changes needed?
To resolve grouping keys correctly.
### Does this PR introduce _any_ user-facing change?
Yes,
```python
from pyspark.sql.functions import *
df = spark.createDataFrame([[1, 1]], ["column", "Score"])
pandas_udf("column integer, Score float", PandasUDFType.GROUPED_MAP)
def my_pandas_udf(pdf):
return pdf.assign(Score=0.5)
df.groupby('COLUMN').apply(my_pandas_udf).show()
```
```python
df1 = spark.createDataFrame([(1, 1)], ("column", "value"))
df2 = spark.createDataFrame([(1, 1)], ("column", "value"))
df1.groupby("COLUMN").cogroup(
df2.groupby("COLUMN")
).applyInPandas(lambda r, l: r + l, df1.schema).show()
```
Before:
```
pyspark.sql.utils.AnalysisException: Reference 'COLUMN' is ambiguous, could be: COLUMN, COLUMN.;
```
```
pyspark.sql.utils.AnalysisException: cannot resolve '`COLUMN`' given input columns: [COLUMN, COLUMN, value, value];;
'FlatMapCoGroupsInPandas ['COLUMN], ['COLUMN], <lambda>(column#9L, value#10L, column#13L, value#14L), [column#22L, value#23L]
:- Project [COLUMN#9L, column#9L, value#10L]
: +- LogicalRDD [column#9L, value#10L], false
+- Project [COLUMN#13L, column#13L, value#14L]
+- LogicalRDD [column#13L, value#14L], false
```
After:
```
+------+-----+
|column|Score|
+------+-----+
| 1| 0.5|
+------+-----+
```
```
+------+-----+
|column|value|
+------+-----+
| 2| 2|
+------+-----+
```
### How was this patch tested?
Unittests were added and manually tested.
Closes#28777 from HyukjinKwon/SPARK-31915-another.
Authored-by: HyukjinKwon <gurwls223@apache.org>
Signed-off-by: Bryan Cutler <cutlerb@gmail.com>
### What changes were proposed in this pull request?
This is a followup of https://github.com/apache/spark/pull/28695 , to fix the problem completely.
The root cause is that, `df("col").as("name")` is not a column reference anymore, and should not have the special column metadata. However, this was broken in ba7adc4949 (diff-ac415c903887e49486ba542a65eec980L1050-L1053)
This PR fixes the regression, by strip the special column metadata in `Column.name`, which is the behavior before https://github.com/apache/spark/pull/28326 .
### Why are the changes needed?
Fix a regression. We shouldn't fail if there is no ambiguous self-join.
### Does this PR introduce _any_ user-facing change?
Yes, the query in the test can run now.
### How was this patch tested?
updated test
Closes#28783 from cloud-fan/self-join.
Authored-by: Wenchen Fan <wenchen@databricks.com>
Signed-off-by: Dongjoon Hyun <dongjoon@apache.org>
### What changes were proposed in this pull request?
Currently we only push nested column pruning from a Project through a few operators such as LIMIT, SAMPLE, etc. There are a few operators like Aggregate, Expand which can prune nested columns by themselves, without a Project on top.
This patch extends the feature to those operators.
### Why are the changes needed?
Currently nested column pruning only applied on a few cases. It limits the benefit of nested column pruning. Extending nested column pruning coverage to make this feature more generally applied through different queries.
### Does this PR introduce _any_ user-facing change?
Yes. More SQL operators are covered by nested column pruning.
### How was this patch tested?
Added unit test, end-to-end tests.
Closes#28560 from viirya/SPARK-27217-2.
Authored-by: Liang-Chi Hsieh <viirya@gmail.com>
Signed-off-by: HyukjinKwon <gurwls223@apache.org>
### What changes were proposed in this pull request?
This PR proposes to make `PythonFunction` holds `Seq[Byte]` instead of `Array[Byte]` to be able to compare if the byte array has the same values for the cache manager.
### Why are the changes needed?
Currently the cache manager doesn't use the cache for `udf` if the `udf` is created again even if the functions is the same.
```py
>>> func = lambda x: x
>>> df = spark.range(1)
>>> df.select(udf(func)("id")).cache()
```
```py
>>> df.select(udf(func)("id")).explain()
== Physical Plan ==
*(2) Project [pythonUDF0#14 AS <lambda>(id)#12]
+- BatchEvalPython [<lambda>(id#0L)], [pythonUDF0#14]
+- *(1) Range (0, 1, step=1, splits=12)
```
This is because `PythonFunction` holds `Array[Byte]`, and `equals` method of array equals only when the both array is the same instance.
### Does this PR introduce _any_ user-facing change?
Yes, if the user reuse the Python function for the UDF, the cache manager will detect the same function and use the cache for it.
### How was this patch tested?
I added a test case and manually.
```py
>>> df.select(udf(func)("id")).explain()
== Physical Plan ==
InMemoryTableScan [<lambda>(id)#12]
+- InMemoryRelation [<lambda>(id)#12], StorageLevel(disk, memory, deserialized, 1 replicas)
+- *(2) Project [pythonUDF0#5 AS <lambda>(id)#3]
+- BatchEvalPython [<lambda>(id#0L)], [pythonUDF0#5]
+- *(1) Range (0, 1, step=1, splits=12)
```
Closes#28774 from ueshin/issues/SPARK-31945/udf_cache.
Authored-by: Takuya UESHIN <ueshin@databricks.com>
Signed-off-by: HyukjinKwon <gurwls223@apache.org>
### What changes were proposed in this pull request?
Mkae Hadoop file system config effective in data source options.
From `org.apache.hadoop.fs.FileSystem.java`:
```
public static FileSystem get(URI uri, Configuration conf) throws IOException {
String scheme = uri.getScheme();
String authority = uri.getAuthority();
if (scheme == null && authority == null) { // use default FS
return get(conf);
}
if (scheme != null && authority == null) { // no authority
URI defaultUri = getDefaultUri(conf);
if (scheme.equals(defaultUri.getScheme()) // if scheme matches default
&& defaultUri.getAuthority() != null) { // & default has authority
return get(defaultUri, conf); // return default
}
}
String disableCacheName = String.format("fs.%s.impl.disable.cache", scheme);
if (conf.getBoolean(disableCacheName, false)) {
return createFileSystem(uri, conf);
}
return CACHE.get(uri, conf);
}
```
Before changes, the file system configurations in data source options are not propagated in `DataSource.scala`.
After changes, we can specify authority and URI schema related configurations for scanning file systems.
This problem only exists in data source V1. In V2, we already use `sparkSession.sessionState.newHadoopConfWithOptions(options)` in `FileTable`.
### Why are the changes needed?
Allow users to specify authority and URI schema related Hadoop configurations for file source reading.
### Does this PR introduce _any_ user-facing change?
Yes, the file system related Hadoop configuration in data source option will be effective on reading.
### How was this patch tested?
Unit test
Closes#28760 from gengliangwang/ds_conf.
Authored-by: Gengliang Wang <gengliang.wang@databricks.com>
Signed-off-by: Gengliang Wang <gengliang.wang@databricks.com>
### What changes were proposed in this pull request?
Currently, `date_format` and `from_unixtime`, `unix_timestamp`,`to_unix_timestamp`, `to_timestamp`, `to_date` have different exception handling behavior for formatting datetime values.
In this PR, we apply the exception handling behavior of `date_format` to `from_unixtime`, `unix_timestamp`,`to_unix_timestamp`, `to_timestamp` and `to_date`.
In the phase of creating the datetime formatted or formating, exceptions will be raised.
e.g.
```java
spark-sql> select date_format(make_timestamp(1, 1 ,1,1,1,1), 'yyyyyyyyyyy-MM-aaa');
20/05/28 15:25:38 ERROR SparkSQLDriver: Failed in [select date_format(make_timestamp(1, 1 ,1,1,1,1), 'yyyyyyyyyyy-MM-aaa')]
org.apache.spark.SparkUpgradeException: You may get a different result due to the upgrading of Spark 3.0: Fail to recognize 'yyyyyyyyyyy-MM-aaa' pattern in the DateTimeFormatter. 1) You can set spark.sql.legacy.timeParserPolicy to LEGACY to restore the behavior before Spark 3.0. 2) You can form a valid datetime pattern with the guide from https://spark.apache.org/docs/latest/sql-ref-datetime-pattern.html
```
```java
spark-sql> select date_format(make_timestamp(1, 1 ,1,1,1,1), 'yyyyyyyyyyy-MM-AAA');
20/05/28 15:26:10 ERROR SparkSQLDriver: Failed in [select date_format(make_timestamp(1, 1 ,1,1,1,1), 'yyyyyyyyyyy-MM-AAA')]
java.lang.IllegalArgumentException: Illegal pattern character: A
```
```java
spark-sql> select date_format(make_timestamp(1,1,1,1,1,1), 'yyyyyyyyyyy-MM-dd');
20/05/28 15:23:23 ERROR SparkSQLDriver: Failed in [select date_format(make_timestamp(1,1,1,1,1,1), 'yyyyyyyyyyy-MM-dd')]
java.lang.ArrayIndexOutOfBoundsException: 11
at java.time.format.DateTimeFormatterBuilder$NumberPrinterParser.format(DateTimeFormatterBuilder.java:2568)
```
In the phase of parsing, `DateTimeParseException | DateTimeException | ParseException` will be suppressed, but `SparkUpgradeException` will still be raised
e.g.
```java
spark-sql> set spark.sql.legacy.timeParserPolicy=exception;
spark.sql.legacy.timeParserPolicy exception
spark-sql> select to_timestamp("2020-01-27T20:06:11.847-0800", "yyyy-MM-dd'T'HH:mm:ss.SSSz");
20/05/28 15:31:15 ERROR SparkSQLDriver: Failed in [select to_timestamp("2020-01-27T20:06:11.847-0800", "yyyy-MM-dd'T'HH:mm:ss.SSSz")]
org.apache.spark.SparkUpgradeException: You may get a different result due to the upgrading of Spark 3.0: Fail to parse '2020-01-27T20:06:11.847-0800' in the new parser. You can set spark.sql.legacy.timeParserPolicy to LEGACY to restore the behavior before Spark 3.0, or set to CORRECTED and treat it as an invalid datetime string.
```
```java
spark-sql> set spark.sql.legacy.timeParserPolicy=corrected;
spark.sql.legacy.timeParserPolicy corrected
spark-sql> select to_timestamp("2020-01-27T20:06:11.847-0800", "yyyy-MM-dd'T'HH:mm:ss.SSSz");
NULL
spark-sql> set spark.sql.legacy.timeParserPolicy=legacy;
spark.sql.legacy.timeParserPolicy legacy
spark-sql> select to_timestamp("2020-01-27T20:06:11.847-0800", "yyyy-MM-dd'T'HH:mm:ss.SSSz");
2020-01-28 12:06:11.847
```
### Why are the changes needed?
Consistency
### Does this PR introduce _any_ user-facing change?
Yes, invalid datetime patterns will fail `from_unixtime`, `unix_timestamp`,`to_unix_timestamp`, `to_timestamp` and `to_date` instead of resulting `NULL`
### How was this patch tested?
add more tests
Closes#28650 from yaooqinn/SPARK-31830.
Authored-by: Kent Yao <yaooqinn@hotmail.com>
Signed-off-by: Wenchen Fan <wenchen@databricks.com>