### What changes were proposed in this pull request?
This PR aims to upgrade PySpark's embedded cloudpickle to the latest cloudpickle v1.5.0 (See https://github.com/cloudpipe/cloudpickle/blob/v1.5.0/cloudpickle/cloudpickle.py)
### Why are the changes needed?
There are many bug fixes. For example, the bug described in the JIRA:
dill unpickling fails because they define `types.ClassType`, which is undefined in dill. This results in the following error:
```
Traceback (most recent call last):
File "/usr/local/lib/python3.6/site-packages/apache_beam/internal/pickler.py", line 279, in loads
return dill.loads(s)
File "/usr/local/lib/python3.6/site-packages/dill/_dill.py", line 317, in loads
return load(file, ignore)
File "/usr/local/lib/python3.6/site-packages/dill/_dill.py", line 305, in load
obj = pik.load()
File "/usr/local/lib/python3.6/site-packages/dill/_dill.py", line 577, in _load_type
return _reverse_typemap[name]
KeyError: 'ClassType'
```
See also https://github.com/cloudpipe/cloudpickle/issues/82. This was fixed for cloudpickle 1.3.0+ (https://github.com/cloudpipe/cloudpickle/pull/337), but PySpark's cloudpickle.py doesn't have this change yet.
More notably, now it supports C pickle implementation with Python 3.8 which hugely improve performance. This is already adopted in another project such as Ray.
### Does this PR introduce _any_ user-facing change?
Yes, as described above, the bug fixes. Internally, users also could leverage the fast cloudpickle backed by C pickle.
### How was this patch tested?
Jenkins will test it out.
Closes#29114 from HyukjinKwon/SPARK-32094.
Authored-by: HyukjinKwon <gurwls223@apache.org>
Signed-off-by: HyukjinKwon <gurwls223@apache.org>
### What changes were proposed in this pull request?
set params default values in trait ...Params in both Scala and Python.
I will do this in two PRs. I will change classification, regression, clustering and fpm in this PR. Will change the rest in another PR.
### Why are the changes needed?
Make ML has the same default param values between estimator and its corresponding transformer, and also between Scala and Python.
### Does this PR introduce _any_ user-facing change?
No
### How was this patch tested?
Existing tests
Closes#29112 from huaxingao/set_default.
Authored-by: Huaxin Gao <huaxing@us.ibm.com>
Signed-off-by: Huaxin Gao <huaxing@us.ibm.com>
### What changes were proposed in this pull request?
Add training summary for FMClassificationModel...
### Why are the changes needed?
so that user can get the training process status, such as loss value of each iteration and total iteration number.
### Does this PR introduce _any_ user-facing change?
Yes
FMClassificationModel.summary
FMClassificationModel.evaluate
### How was this patch tested?
new tests
Closes#28960 from huaxingao/fm_summary.
Authored-by: Huaxin Gao <huaxing@us.ibm.com>
Signed-off-by: Huaxin Gao <huaxing@us.ibm.com>
### What changes were proposed in this pull request?
This PR will remove references to these "blacklist" and "whitelist" terms besides the blacklisting feature as a whole, which can be handled in a separate JIRA/PR.
This touches quite a few files, but the changes are straightforward (variable/method/etc. name changes) and most quite self-contained.
### Why are the changes needed?
As per discussion on the Spark dev list, it will be beneficial to remove references to problematic language that can alienate potential community members. One such reference is "blacklist" and "whitelist". While it seems to me that there is some valid debate as to whether these terms have racist origins, the cultural connotations are inescapable in today's world.
### Does this PR introduce _any_ user-facing change?
In the test file `HiveQueryFileTest`, a developer has the ability to specify the system property `spark.hive.whitelist` to specify a list of Hive query files that should be tested. This system property has been renamed to `spark.hive.includelist`. The old property has been kept for compatibility, but will log a warning if used. I am open to feedback from others on whether keeping a deprecated property here is unnecessary given that this is just for developers running tests.
### How was this patch tested?
Existing tests should be suitable since no behavior changes are expected as a result of this PR.
Closes#28874 from xkrogen/xkrogen-SPARK-32036-rename-blacklists.
Authored-by: Erik Krogen <ekrogen@linkedin.com>
Signed-off-by: Thomas Graves <tgraves@apache.org>
### What changes were proposed in this pull request?
This PR aims to test PySpark with Python 3.8 in Github Actions. In the script side, it is already ready:
4ad9bfd53b/python/run-tests.py (L161)
This PR includes small related fixes together:
1. Install Python 3.8
2. Only install one Python implementation instead of installing many for SQL and Yarn test cases because they need one Python executable in their test cases that is higher than Python 2.
3. Do not install Python 2 which is not needed anymore after we dropped Python 2 at SPARK-32138
4. Remove a comment about installing PyPy3 on Jenkins - SPARK-32278. It is already installed.
### Why are the changes needed?
Currently, only PyPy3 and Python 3.6 are being tested with PySpark in Github Actions. We should test the latest version of Python as well because some optimizations can be only enabled with Python 3.8+. See also https://github.com/apache/spark/pull/29114
### Does this PR introduce _any_ user-facing change?
No, dev-only.
### How was this patch tested?
Was not tested. Github Actions build in this PR will test it out.
Closes#29116 from HyukjinKwon/test-python3.8-togehter.
Authored-by: HyukjinKwon <gurwls223@apache.org>
Signed-off-by: Dongjoon Hyun <dongjoon@apache.org>
### What changes were proposed in this pull request?
This PR proposes to port the test case from https://github.com/apache/spark/pull/29098 to branch-3.0 and master. In the master and branch-3.0, this was fixed together at ecaa495b1f but no partition case is not being tested.
### Why are the changes needed?
To improve test coverage.
### Does this PR introduce _any_ user-facing change?
No, test-only.
### How was this patch tested?
Unit test was forward-ported.
Closes#29099 from HyukjinKwon/SPARK-32300-1.
Authored-by: HyukjinKwon <gurwls223@apache.org>
Signed-off-by: HyukjinKwon <gurwls223@apache.org>
### What changes were proposed in this pull request?
`datetime` is already imported a few lines below :)
ce27cc54c1/python/pyspark/sql/tests/test_pandas_udf_scalar.py (L24)
### Why are the changes needed?
This is the last instance of the duplicate import.
### Does this PR introduce _any_ user-facing change?
No.
### How was this patch tested?
Manual.
Closes#29109 from Fokko/SPARK-32311.
Authored-by: Fokko Driesprong <fokko@apache.org>
Signed-off-by: Dongjoon Hyun <dongjoon@apache.org>
# What changes were proposed in this pull request?
While seeing if we can use mypy for checking the Python types, I've stumbled across this missing import:
34fa913311/python/pyspark/ml/feature.py (L5773-L5774)
### Why are the changes needed?
The `import` is required because it's used.
### Does this PR introduce _any_ user-facing change?
No.
### How was this patch tested?
Manual.
Closes#29108 from Fokko/SPARK-32309.
Authored-by: Fokko Driesprong <fokko@apache.org>
Signed-off-by: Dongjoon Hyun <dongjoon@apache.org>
### What changes were proposed in this pull request?
This PR aims to drop Python 2.7, 3.4 and 3.5.
Roughly speaking, it removes all the widely known Python 2 compatibility workarounds such as `sys.version` comparison, `__future__`. Also, it removes the Python 2 dedicated codes such as `ArrayConstructor` in Spark.
### Why are the changes needed?
1. Unsupport EOL Python versions
2. Reduce maintenance overhead and remove a bit of legacy codes and hacks for Python 2.
3. PyPy2 has a critical bug that causes a flaky test, SPARK-28358 given my testing and investigation.
4. Users can use Python type hints with Pandas UDFs without thinking about Python version
5. Users can leverage one latest cloudpickle, https://github.com/apache/spark/pull/28950. With Python 3.8+ it can also leverage C pickle.
### Does this PR introduce _any_ user-facing change?
Yes, users cannot use Python 2.7, 3.4 and 3.5 in the upcoming Spark version.
### How was this patch tested?
Manually tested and also tested in Jenkins.
Closes#28957 from HyukjinKwon/SPARK-32138.
Authored-by: HyukjinKwon <gurwls223@apache.org>
Signed-off-by: HyukjinKwon <gurwls223@apache.org>
### What changes were proposed in this pull request?
This PR aims to run the Spark tests in Github Actions.
To briefly explain the main idea:
- Reuse `dev/run-tests.py` with SBT build
- Reuse the modules in `dev/sparktestsupport/modules.py` to test each module
- Pass the modules to test into `dev/run-tests.py` directly via `TEST_ONLY_MODULES` environment variable. For example, `pyspark-sql,core,sql,hive`.
- `dev/run-tests.py` _does not_ take the dependent modules into account but solely the specified modules to test.
Another thing to note might be `SlowHiveTest` annotation. Running the tests in Hive modules takes too much so the slow tests are extracted and it runs as a separate job. It was extracted from the actual elapsed time in Jenkins:
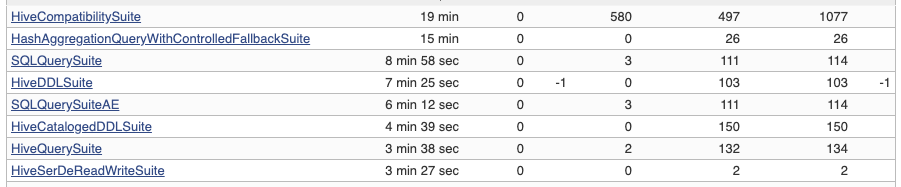
So, Hive tests are separated into to jobs. One is slow test cases, and the other one is the other test cases.
_Note that_ the current GitHub Actions build virtually copies what the default PR builder on Jenkins does (without other profiles such as JDK 11, Hadoop 2, etc.). The only exception is Kinesis https://github.com/apache/spark/pull/29057/files#diff-04eb107ee163a50b61281ca08f4e4c7bR23
### Why are the changes needed?
Last week and onwards, the Jenkins machines became very unstable for many reasons:
- Apparently, the machines became extremely slow. Almost all tests can't pass.
- One machine (worker 4) started to have the corrupt `.m2` which fails the build.
- Documentation build fails time to time for an unknown reason in Jenkins machine specifically. This is disabled for now at https://github.com/apache/spark/pull/29017.
- Almost all PRs are basically blocked by this instability currently.
The advantages of using Github Actions:
- To avoid depending on few persons who can access to the cluster.
- To reduce the elapsed time in the build - we could split the tests (e.g., SQL, ML, CORE), and run them in parallel so the total build time will significantly reduce.
- To control the environment more flexibly.
- Other contributors can test and propose to fix Github Actions configurations so we can distribute this build management cost.
Note that:
- The current build in Jenkins takes _more than 7 hours_. With Github actions it takes _less than 2 hours_
- We can now control the environments especially for Python easily.
- The test and build look more stable than the Jenkins'.
### Does this PR introduce _any_ user-facing change?
No, dev-only change.
### How was this patch tested?
Tested at https://github.com/HyukjinKwon/spark/pull/4Closes#29057 from HyukjinKwon/migrate-to-github-actions.
Authored-by: HyukjinKwon <gurwls223@apache.org>
Signed-off-by: Dongjoon Hyun <dongjoon@apache.org>
# What changes were proposed in this pull request?
current problems:
```
mlp = MultilayerPerceptronClassifier(layers=[2, 2, 2], seed=123)
model = mlp.fit(df)
path = tempfile.mkdtemp()
model_path = path + "/mlp"
model.save(model_path)
model2 = MultilayerPerceptronClassificationModel.load(model_path)
self.assertEqual(model2.getSolver(), "l-bfgs") # this fails because model2.getSolver() returns 'auto'
model2.transform(df)
# this fails with Exception pyspark.sql.utils.IllegalArgumentException: MultilayerPerceptronClassifier_dec859ed24ec parameter solver given invalid value auto.
```
FMClassifier/Regression and GeneralizedLinearRegression have the same problems.
Here are the root cause of the problems:
1. In HasSolver, both Scala and Python default solver to 'auto'
2. On Scala side, mlp overrides the default of solver to 'l-bfgs', FMClassifier/Regression overrides the default of solver to 'adamW', and glr overrides the default of solver to 'irls'
3. On Scala side, mlp overrides the default of solver in MultilayerPerceptronClassificationParams, so both MultilayerPerceptronClassification and MultilayerPerceptronClassificationModel have 'l-bfgs' as default
4. On Python side, mlp overrides the default of solver in MultilayerPerceptronClassification, so it has default as 'l-bfgs', but MultilayerPerceptronClassificationModel doesn't override the default so it gets the default from HasSolver which is 'auto'. In theory, we don't care about the solver value or any other params values for MultilayerPerceptronClassificationModel, because we have the fitted model already. That's why on Python side, we never set default values for any of the XXXModel.
5. when calling getSolver on the loaded mlp model, it calls this line of code underneath:
```
def _transfer_params_from_java(self):
"""
Transforms the embedded params from the companion Java object.
"""
......
# SPARK-14931: Only check set params back to avoid default params mismatch.
if self._java_obj.isSet(java_param):
value = _java2py(sc, self._java_obj.getOrDefault(java_param))
self._set(**{param.name: value})
......
```
that's why model2.getSolver() returns 'auto'. The code doesn't get the default Scala value (in this case 'l-bfgs') to set to Python param, so it takes the default value (in this case 'auto') on Python side.
6. when calling model2.transform(df), it calls this underneath:
```
def _transfer_params_to_java(self):
"""
Transforms the embedded params to the companion Java object.
"""
......
if self.hasDefault(param):
pair = self._make_java_param_pair(param, self._defaultParamMap[param])
pair_defaults.append(pair)
......
```
Again, it gets the Python default solver which is 'auto', and this caused the Exception
7. Currently, on Scala side, for some of the algorithms, we set default values in the XXXParam, so both estimator and transformer get the default value. However, for some of the algorithms, we only set default in estimators, and the XXXModel doesn't get the default value. On Python side, we never set defaults for the XXXModel. This causes the default value inconsistency.
8. My proposed solution: set default params in XXXParam for both Scala and Python, so both the estimator and transformer have the same default value for both Scala and Python. I currently only changed solver in this PR. If everyone is OK with the fix, I will change all the other params as well.
I hope my explanation makes sense to your folks :)
### Why are the changes needed?
Fix bug
### Does this PR introduce _any_ user-facing change?
No
### How was this patch tested?
existing and new tests
Closes#29060 from huaxingao/solver_parity.
Authored-by: Huaxin Gao <huaxing@us.ibm.com>
Signed-off-by: Sean Owen <srowen@gmail.com>
### What changes were proposed in this pull request?
This PR proposes to partially reverts the simple string in `NullType` at https://github.com/apache/spark/pull/28833: `NullType.simpleString` back from `unknown` to `null`.
### Why are the changes needed?
- Technically speaking, it's orthogonal with the issue itself, SPARK-20680.
- It needs some more discussion, see https://github.com/apache/spark/pull/28833#issuecomment-655277714
### Does this PR introduce _any_ user-facing change?
It reverts back the user-facing changes at https://github.com/apache/spark/pull/28833.
The simple string of `NullType` is back to `null`.
### How was this patch tested?
I just logically reverted. Jenkins should test it out.
Closes#29041 from HyukjinKwon/SPARK-20680.
Authored-by: HyukjinKwon <gurwls223@apache.org>
Signed-off-by: Dongjoon Hyun <dongjoon@apache.org>
### What changes were proposed in this pull request?
This PR proposes to disallow to create `SparkContext` in executors, e.g., in UDFs.
### Why are the changes needed?
Currently executors can create SparkContext, but shouldn't be able to create it.
```scala
sc.range(0, 1).foreach { _ =>
new SparkContext(new SparkConf().setAppName("test").setMaster("local"))
}
```
### Does this PR introduce _any_ user-facing change?
Yes, users won't be able to create `SparkContext` in executors.
### How was this patch tested?
Addes tests.
Closes#28986 from ueshin/issues/SPARK-32160/disallow_spark_context_in_executors.
Authored-by: Takuya UESHIN <ueshin@databricks.com>
Signed-off-by: HyukjinKwon <gurwls223@apache.org>
### What changes were proposed in this pull request?
This is the new PR which to address the close one #17953
1. support "void" primitive data type in the `AstBuilder`, point it to `NullType`
2. forbid creating tables with VOID/NULL column type
### Why are the changes needed?
1. Spark is incompatible with hive void type. When Hive table schema contains void type, DESC table will throw an exception in Spark.
>hive> create table bad as select 1 x, null z from dual;
>hive> describe bad;
OK
x int
z void
In Spark2.0.x, the behaviour to read this view is normal:
>spark-sql> describe bad;
x int NULL
z void NULL
Time taken: 4.431 seconds, Fetched 2 row(s)
But in lastest Spark version, it failed with SparkException: Cannot recognize hive type string: void
>spark-sql> describe bad;
17/05/09 03:12:08 ERROR thriftserver.SparkSQLDriver: Failed in [describe bad]
org.apache.spark.SparkException: Cannot recognize hive type string: void
Caused by: org.apache.spark.sql.catalyst.parser.ParseException:
DataType void() is not supported.(line 1, pos 0)
== SQL ==
void
^^^
... 61 more
org.apache.spark.SparkException: Cannot recognize hive type string: void
2. Hive CTAS statements throws error when select clause has NULL/VOID type column since HIVE-11217
In Spark, creating table with a VOID/NULL column should throw readable exception message, include
- create data source table (using parquet, json, ...)
- create hive table (with or without stored as)
- CTAS
### Does this PR introduce any user-facing change?
No
### How was this patch tested?
Add unit tests
Closes#28833 from LantaoJin/SPARK-20680_COPY.
Authored-by: LantaoJin <jinlantao@gmail.com>
Signed-off-by: Dongjoon Hyun <dongjoon@apache.org>
### What changes were proposed in this pull request?
Improve the error message in test GroupedMapInPandasTests.test_grouped_over_window_with_key to show the incorrect values.
### Why are the changes needed?
This test failure has come up often in Arrow testing because it tests a struct with timestamp values through a Pandas UDF. The current error message is not helpful as it doesn't show the incorrect values, only that it failed. This change will instead raise an assertion error with the incorrect values on a failure.
Before:
```
======================================================================
FAIL: test_grouped_over_window_with_key (pyspark.sql.tests.test_pandas_grouped_map.GroupedMapInPandasTests)
----------------------------------------------------------------------
Traceback (most recent call last):
File "/spark/python/pyspark/sql/tests/test_pandas_grouped_map.py", line 588, in test_grouped_over_window_with_key
self.assertTrue(all([r[0] for r in result]))
AssertionError: False is not true
```
After:
```
======================================================================
ERROR: test_grouped_over_window_with_key (pyspark.sql.tests.test_pandas_grouped_map.GroupedMapInPandasTests)
----------------------------------------------------------------------
...
AssertionError: {'start': datetime.datetime(2018, 3, 20, 0, 0), 'end': datetime.datetime(2018, 3, 25, 0, 0)}, != {'start': datetime.datetime(2020, 3, 20, 0, 0), 'end': datetime.datetime(2020, 3, 25, 0, 0)}
```
### Does this PR introduce _any_ user-facing change?
No
### How was this patch tested?
Improved existing test
Closes#28987 from BryanCutler/pandas-grouped-map-test-output-SPARK-32162.
Authored-by: Bryan Cutler <cutlerb@gmail.com>
Signed-off-by: HyukjinKwon <gurwls223@apache.org>
### What changes were proposed in this pull request?
Minor fix so that the documentation of `getActiveSession` is fixed.
The sample code snippet doesn't come up formatted rightly, added spacing for this to be fixed.
Also added return to docs.
### Why are the changes needed?
The sample code is getting mixed up as description in the docs.
[Current Doc Link](http://spark.apache.org/docs/latest/api/python/pyspark.sql.html?highlight=getactivesession#pyspark.sql.SparkSession.getActiveSession)

### Does this PR introduce _any_ user-facing change?
Yes, documentation of getActiveSession is fixed.
And added description about return.
### How was this patch tested?
Adding a spacing between description and code seems to fix the issue.
Closes#28978 from animenon/docs_minor.
Authored-by: animenon <animenon@mail.com>
Signed-off-by: HyukjinKwon <gurwls223@apache.org>
### What changes were proposed in this pull request?
Add summary to RandomForestClassificationModel...
### Why are the changes needed?
so user can get a summary of this classification model, and retrieve common metrics such as accuracy, weightedTruePositiveRate, roc (for binary), pr curves (for binary), etc.
### Does this PR introduce _any_ user-facing change?
Yes
```
RandomForestClassificationModel.summary
RandomForestClassificationModel.evaluate
```
### How was this patch tested?
Add new tests
Closes#28913 from huaxingao/rf_summary.
Authored-by: Huaxin Gao <huaxing@us.ibm.com>
Signed-off-by: Sean Owen <srowen@gmail.com>
### What changes were proposed in this pull request?
Modify the example for `timestamp_seconds` and replace `collect()` by `show()`.
### Why are the changes needed?
The SQL config `spark.sql.session.timeZone` doesn't influence on the `collect` in the example. The code below demonstrates that:
```
$ export TZ="UTC"
```
```python
>>> from pyspark.sql.functions import timestamp_seconds
>>> spark.conf.set("spark.sql.session.timeZone", "America/Los_Angeles")
>>> time_df = spark.createDataFrame([(1230219000,)], ['unix_time'])
>>> time_df.select(timestamp_seconds(time_df.unix_time).alias('ts')).collect()
[Row(ts=datetime.datetime(2008, 12, 25, 15, 30))]
```
The expected time is **07:30 but we get 15:30**.
### Does this PR introduce _any_ user-facing change?
No
### How was this patch tested?
By running the modified example via:
```
$ ./python/run-tests --modules=pyspark-sql
```
Closes#28959 from MaxGekk/SPARK-32088-fix-timezone-issue-followup.
Authored-by: Max Gekk <max.gekk@gmail.com>
Signed-off-by: HyukjinKwon <gurwls223@apache.org>
### What changes were proposed in this pull request?
Add American timezone during timestamp_seconds doctest
### Why are the changes needed?
`timestamp_seconds` doctest in `functions.py` used default timezone to get expected result
For example:
```python
>>> time_df = spark.createDataFrame([(1230219000,)], ['unix_time'])
>>> time_df.select(timestamp_seconds(time_df.unix_time).alias('ts')).collect()
[Row(ts=datetime.datetime(2008, 12, 25, 7, 30))]
```
But when we have a non-american timezone, the test case will get different test result.
For example, when we set current timezone as `Asia/Shanghai`, the test result will be
```
[Row(ts=datetime.datetime(2008, 12, 25, 23, 30))]
```
So no matter where we run the test case ,we will always get the expected permanent result if we set the timezone on one specific area.
### Does this PR introduce _any_ user-facing change?
No
### How was this patch tested?
Unit test
Closes#28932 from GuoPhilipse/SPARK-32088-fix-timezone-issue.
Lead-authored-by: GuoPhilipse <46367746+GuoPhilipse@users.noreply.github.com>
Co-authored-by: GuoPhilipse <guofei_ok@126.com>
Signed-off-by: Dongjoon Hyun <dongjoon@apache.org>
### What changes were proposed in this pull request?
Add training summary for LinearSVCModel......
### Why are the changes needed?
so that user can get the training process status, such as loss value of each iteration and total iteration number.
### Does this PR introduce _any_ user-facing change?
Yes
```LinearSVCModel.summary```
```LinearSVCModel.evaluate```
### How was this patch tested?
new tests
Closes#28884 from huaxingao/svc_summary.
Authored-by: Huaxin Gao <huaxing@us.ibm.com>
Signed-off-by: Sean Owen <srowen@gmail.com>
### What changes were proposed in this pull request?
Adding support to Association Rules in Spark ml.fpm.
### Why are the changes needed?
Support is an indication of how frequently the itemset of an association rule appears in the database and suggests if the rules are generally applicable to the dateset. Refer to [wiki](https://en.wikipedia.org/wiki/Association_rule_learning#Support) for more details.
### Does this PR introduce _any_ user-facing change?
Yes. Associate Rules now have support measure
### How was this patch tested?
existing and new unit test
Closes#28903 from huaxingao/fpm.
Authored-by: Huaxin Gao <huaxing@us.ibm.com>
Signed-off-by: Sean Owen <srowen@gmail.com>
### What changes were proposed in this pull request?
When you use floats are index of pandas, it creates a Spark DataFrame with a wrong results as below when Arrow is enabled:
```bash
./bin/pyspark --conf spark.sql.execution.arrow.pyspark.enabled=true
```
```python
>>> import pandas as pd
>>> spark.createDataFrame(pd.DataFrame({'a': [1,2,3]}, index=[2., 3., 4.])).show()
+---+
| a|
+---+
| 1|
| 1|
| 2|
+---+
```
This is because direct slicing uses the value as index when the index contains floats:
```python
>>> pd.DataFrame({'a': [1,2,3]}, index=[2., 3., 4.])[2:]
a
2.0 1
3.0 2
4.0 3
>>> pd.DataFrame({'a': [1,2,3]}, index=[2., 3., 4.]).iloc[2:]
a
4.0 3
>>> pd.DataFrame({'a': [1,2,3]}, index=[2, 3, 4])[2:]
a
4 3
```
This PR proposes to explicitly use `iloc` to positionally slide when we create a DataFrame from a pandas DataFrame with Arrow enabled.
FWIW, I was trying to investigate why direct slicing refers the index value or the positional index sometimes but I stopped investigating further after reading this https://pandas.pydata.org/pandas-docs/stable/getting_started/10min.html#selection
> While standard Python / Numpy expressions for selecting and setting are intuitive and come in handy for interactive work, for production code, we recommend the optimized pandas data access methods, `.at`, `.iat`, `.loc` and `.iloc`.
### Why are the changes needed?
To create the correct Spark DataFrame from a pandas DataFrame without a data loss.
### Does this PR introduce _any_ user-facing change?
Yes, it is a bug fix.
```bash
./bin/pyspark --conf spark.sql.execution.arrow.pyspark.enabled=true
```
```python
import pandas as pd
spark.createDataFrame(pd.DataFrame({'a': [1,2,3]}, index=[2., 3., 4.])).show()
```
Before:
```
+---+
| a|
+---+
| 1|
| 1|
| 2|
+---+
```
After:
```
+---+
| a|
+---+
| 1|
| 2|
| 3|
+---+
```
### How was this patch tested?
Manually tested and unittest were added.
Closes#28928 from HyukjinKwon/SPARK-32098.
Authored-by: HyukjinKwon <gurwls223@apache.org>
Signed-off-by: Bryan Cutler <cutlerb@gmail.com>
### What changes were proposed in this pull request?
Add a generic ClassificationSummary trait
### Why are the changes needed?
Add a generic ClassificationSummary trait so all the classification models can use it to implement summary.
Currently in classification, we only have summary implemented in ```LogisticRegression```. There are requests to implement summary for ```LinearSVCModel``` in https://issues.apache.org/jira/browse/SPARK-20249 and to implement summary for ```RandomForestClassificationModel``` in https://issues.apache.org/jira/browse/SPARK-23631. If we add a generic ClassificationSummary trait and put all the common code there, we can easily add summary to ```LinearSVCModel``` and ```RandomForestClassificationModel```, and also add summary to all the other classification models.
We can use the same approach to add a generic RegressionSummary trait to regression package and implement summary for all the regression models.
### Does this PR introduce _any_ user-facing change?
### How was this patch tested?
existing tests
Closes#28710 from huaxingao/summary_trait.
Authored-by: Huaxin Gao <huaxing@us.ibm.com>
Signed-off-by: Sean Owen <srowen@gmail.com>
### What changes were proposed in this pull request?
This PR proposes to remove the warning about multi-thread in local properties, and change the guide to use `collectWithJobGroup` for multi-threads for now because:
- It is too noisy to users who don't use multiple threads - the number of this single thread case is arguably more prevailing.
- There was a critical issue found about pin-thread mode SPARK-32010, which will be fixed in Spark 3.1.
- To smoothly migrate, `RDD.collectWithJobGroup` was added, which will be deprecated in Spark 3.1 with SPARK-32010 fixed.
I will target to deprecate `RDD.collectWithJobGroup`, and make this pin-thread mode stable in Spark 3.1. In the future releases, I plan to make this mode as a default mode, and remove `RDD.collectWithJobGroup` away.
### Why are the changes needed?
To avoid guiding users a feature with a critical issue, and provide a proper workaround for now.
### Does this PR introduce _any_ user-facing change?
Yes, warning message and documentation.
### How was this patch tested?
Manually tested:
Before:
```
>>> spark.sparkContext.setLocalProperty("a", "b")
/.../spark/python/pyspark/util.py:141: UserWarning: Currently, 'setLocalProperty' (set to local
properties) with multiple threads does not properly work.
Internally threads on PVM and JVM are not synced, and JVM thread can be reused for multiple
threads on PVM, which fails to isolate local properties for each thread on PVM.
To work around this, you can set PYSPARK_PIN_THREAD to true (see SPARK-22340). However,
note that it cannot inherit the local properties from the parent thread although it isolates each
thread on PVM and JVM with its own local properties.
To work around this, you should manually copy and set the local properties from the parent thread
to the child thread when you create another thread.
```
After:
```
>>> spark.sparkContext.setLocalProperty("a", "b")
```
Closes#28845 from HyukjinKwon/SPARK-32011.
Authored-by: HyukjinKwon <gurwls223@apache.org>
Signed-off-by: HyukjinKwon <gurwls223@apache.org>
### What changes were proposed in this pull request?
This patch adds user-specified fold column support to `CrossValidator`. User can assign fold numbers to dataset instead of letting Spark do random splits.
### Why are the changes needed?
This gives `CrossValidator` users more flexibility in splitting folds.
### Does this PR introduce _any_ user-facing change?
Yes, a new `foldCol` param is added to `CrossValidator`. User can use it to specify custom fold splitting.
### How was this patch tested?
Added unit tests.
Closes#28704 from viirya/SPARK-31777.
Authored-by: Liang-Chi Hsieh <viirya@gmail.com>
Signed-off-by: Liang-Chi Hsieh <liangchi@uber.com>
## What changes were proposed in this pull request?
we fail casting from numeric to timestamp by default.
## Why are the changes needed?
casting from numeric to timestamp is not a non-standard,meanwhile it may generate different result between spark and other systems,for example hive
## Does this PR introduce any user-facing change?
Yes,user cannot cast numeric to timestamp directly,user have to use the following function to achieve the same effect:TIMESTAMP_SECONDS/TIMESTAMP_MILLIS/TIMESTAMP_MICROS
## How was this patch tested?
unit test added
Closes#28593 from GuoPhilipse/31710-fix-compatibility.
Lead-authored-by: GuoPhilipse <guofei_ok@126.com>
Co-authored-by: GuoPhilipse <46367746+GuoPhilipse@users.noreply.github.com>
Signed-off-by: Wenchen Fan <wenchen@databricks.com>
### What changes were proposed in this pull request?
In LogisticRegression and LinearRegression, if set maxIter=n, the model.summary.totalIterations returns n+1 if the training procedure does not drop out. This is because we use ```objectiveHistory.length``` as totalIterations, but ```objectiveHistory``` contains init sate, thus ```objectiveHistory.length``` is 1 larger than number of training iterations.
### Why are the changes needed?
correctness
### Does this PR introduce _any_ user-facing change?
No
### How was this patch tested?
add new tests and also modify existing tests
Closes#28786 from huaxingao/summary_iter.
Authored-by: Huaxin Gao <huaxing@us.ibm.com>
Signed-off-by: Sean Owen <srowen@gmail.com>
### What changes were proposed in this pull request?
This is similar with 64cb6f7066
The test `StreamingLogisticRegressionWithSGDTests.test_training_and_prediction` seems also flaky. This PR just increases the timeout to 3 mins too. The cause is very likely the time elapsed.
See https://amplab.cs.berkeley.edu/jenkins/job/SparkPullRequestBuilder/123787/testReport/pyspark.mllib.tests.test_streaming_algorithms/StreamingLogisticRegressionWithSGDTests/test_training_and_prediction/
```
Traceback (most recent call last):
File "/home/jenkins/workspace/SparkPullRequestBuilder2/python/pyspark/mllib/tests/test_streaming_algorithms.py", line 330, in test_training_and_prediction
eventually(condition, timeout=60.0)
File "/home/jenkins/workspace/SparkPullRequestBuilder2/python/pyspark/testing/utils.py", line 90, in eventually
% (timeout, lastValue))
AssertionError: Test failed due to timeout after 60 sec, with last condition returning: Latest errors: 0.67, 0.71, 0.78, 0.7, 0.75, 0.74, 0.73, 0.69, 0.62, 0.71, 0.69, 0.75, 0.72, 0.77, 0.71, 0.74, 0.76, 0.78, 0.7, 0.78, 0.8, 0.74, 0.77, 0.75, 0.76, 0.76, 0.75
```
### Why are the changes needed?
To make PR builds more stable.
### Does this PR introduce _any_ user-facing change?
No.
### How was this patch tested?
Jenkins will test them out.
Closes#28798 from HyukjinKwon/SPARK-31966.
Authored-by: HyukjinKwon <gurwls223@apache.org>
Signed-off-by: Dongjoon Hyun <dongjoon@apache.org>
### What changes were proposed in this pull request?
This PR proposes to move the doctests in `registerJavaUDAF` and `registerJavaFunction` to the proper unittests that run conditionally when the test classes are present.
Both tests are dependent on the test classes in JVM side, `test.org.apache.spark.sql.JavaStringLength` and `test.org.apache.spark.sql.MyDoubleAvg`. So if you run the tests against the plain `sbt package`, it fails as below:
```
**********************************************************************
File "/.../spark/python/pyspark/sql/udf.py", line 366, in pyspark.sql.udf.UDFRegistration.registerJavaFunction
Failed example:
spark.udf.registerJavaFunction(
"javaStringLength", "test.org.apache.spark.sql.JavaStringLength", IntegerType())
Exception raised:
Traceback (most recent call last):
...
test.org.apache.spark.sql.JavaStringLength, please make sure it is on the classpath;
...
6 of 7 in pyspark.sql.udf.UDFRegistration.registerJavaFunction
2 of 4 in pyspark.sql.udf.UDFRegistration.registerJavaUDAF
***Test Failed*** 8 failures.
```
### Why are the changes needed?
In order to support to run the tests against the plain SBT build. See also https://spark.apache.org/developer-tools.html
### Does this PR introduce _any_ user-facing change?
No, it's test-only.
### How was this patch tested?
Manually tested as below:
```bash
./build/sbt -DskipTests -Phive-thriftserver clean package
cd python
./run-tests --python-executable=python3 --testname="pyspark.sql.udf UserDefinedFunction"
./run-tests --python-executable=python3 --testname="pyspark.sql.tests.test_udf UDFTests"
```
```bash
./build/sbt -DskipTests -Phive-thriftserver clean test:package
cd python
./run-tests --python-executable=python3 --testname="pyspark.sql.udf UserDefinedFunction"
./run-tests --python-executable=python3 --testname="pyspark.sql.tests.test_udf UDFTests"
```
Closes#28795 from HyukjinKwon/SPARK-31965.
Authored-by: HyukjinKwon <gurwls223@apache.org>
Signed-off-by: Dongjoon Hyun <dongjoon@apache.org>
### What changes were proposed in this pull request?
When using pyarrow to convert a Pandas categorical column, use `is_categorical` instead of trying to import `CategoricalDtype`
### Why are the changes needed?
The import for `CategoricalDtype` had changed from Pandas 0.23 to 1.0 and pyspark currently tries both locations. Using `is_categorical` is a more stable API.
### Does this PR introduce _any_ user-facing change?
No
### How was this patch tested?
Existing tests
Closes#28793 from BryanCutler/arrow-use-is_categorical-SPARK-31964.
Authored-by: Bryan Cutler <cutlerb@gmail.com>
Signed-off-by: HyukjinKwon <gurwls223@apache.org>
### What changes were proposed in this pull request?
This is another approach to fix the issue. See the previous try https://github.com/apache/spark/pull/28745. It was too invasive so I took more conservative approach.
This PR proposes to resolve grouping attributes separately first so it can be properly referred when `FlatMapGroupsInPandas` and `FlatMapCoGroupsInPandas` are resolved without ambiguity.
Previously,
```python
from pyspark.sql.functions import *
df = spark.createDataFrame([[1, 1]], ["column", "Score"])
pandas_udf("column integer, Score float", PandasUDFType.GROUPED_MAP)
def my_pandas_udf(pdf):
return pdf.assign(Score=0.5)
df.groupby('COLUMN').apply(my_pandas_udf).show()
```
was failed as below:
```
pyspark.sql.utils.AnalysisException: "Reference 'COLUMN' is ambiguous, could be: COLUMN, COLUMN.;"
```
because the unresolved `COLUMN` in `FlatMapGroupsInPandas` doesn't know which reference to take from the child projection.
After this fix, it resolves the child projection first with grouping keys and pass, to `FlatMapGroupsInPandas`, the attribute as a grouping key from the child projection that is positionally selected.
### Why are the changes needed?
To resolve grouping keys correctly.
### Does this PR introduce _any_ user-facing change?
Yes,
```python
from pyspark.sql.functions import *
df = spark.createDataFrame([[1, 1]], ["column", "Score"])
pandas_udf("column integer, Score float", PandasUDFType.GROUPED_MAP)
def my_pandas_udf(pdf):
return pdf.assign(Score=0.5)
df.groupby('COLUMN').apply(my_pandas_udf).show()
```
```python
df1 = spark.createDataFrame([(1, 1)], ("column", "value"))
df2 = spark.createDataFrame([(1, 1)], ("column", "value"))
df1.groupby("COLUMN").cogroup(
df2.groupby("COLUMN")
).applyInPandas(lambda r, l: r + l, df1.schema).show()
```
Before:
```
pyspark.sql.utils.AnalysisException: Reference 'COLUMN' is ambiguous, could be: COLUMN, COLUMN.;
```
```
pyspark.sql.utils.AnalysisException: cannot resolve '`COLUMN`' given input columns: [COLUMN, COLUMN, value, value];;
'FlatMapCoGroupsInPandas ['COLUMN], ['COLUMN], <lambda>(column#9L, value#10L, column#13L, value#14L), [column#22L, value#23L]
:- Project [COLUMN#9L, column#9L, value#10L]
: +- LogicalRDD [column#9L, value#10L], false
+- Project [COLUMN#13L, column#13L, value#14L]
+- LogicalRDD [column#13L, value#14L], false
```
After:
```
+------+-----+
|column|Score|
+------+-----+
| 1| 0.5|
+------+-----+
```
```
+------+-----+
|column|value|
+------+-----+
| 2| 2|
+------+-----+
```
### How was this patch tested?
Unittests were added and manually tested.
Closes#28777 from HyukjinKwon/SPARK-31915-another.
Authored-by: HyukjinKwon <gurwls223@apache.org>
Signed-off-by: Bryan Cutler <cutlerb@gmail.com>
### What changes were proposed in this pull request?
This PR aims to support both pandas 0.23 and 1.0.
### Why are the changes needed?
```
$ pip install pandas==0.23.2
$ python -c "import pandas.CategoricalDtype"
Traceback (most recent call last):
File "<string>", line 1, in <module>
ModuleNotFoundError: No module named 'pandas.CategoricalDtype'
$ python -c "from pandas.api.types import CategoricalDtype"
```
### Does this PR introduce _any_ user-facing change?
No.
### How was this patch tested?
Pass the Jenkins.
```
$ pip freeze | grep pandas
pandas==0.23.2
$ python/run-tests.py --python-executables python --modules pyspark-sql
...
Tests passed in 359 seconds
```
Closes#28789 from williamhyun/williamhyun-patch-2.
Authored-by: William Hyun <williamhyun3@gmail.com>
Signed-off-by: Dongjoon Hyun <dongjoon@apache.org>
### What changes were proposed in this pull request?
This PR proposes to make `PythonFunction` holds `Seq[Byte]` instead of `Array[Byte]` to be able to compare if the byte array has the same values for the cache manager.
### Why are the changes needed?
Currently the cache manager doesn't use the cache for `udf` if the `udf` is created again even if the functions is the same.
```py
>>> func = lambda x: x
>>> df = spark.range(1)
>>> df.select(udf(func)("id")).cache()
```
```py
>>> df.select(udf(func)("id")).explain()
== Physical Plan ==
*(2) Project [pythonUDF0#14 AS <lambda>(id)#12]
+- BatchEvalPython [<lambda>(id#0L)], [pythonUDF0#14]
+- *(1) Range (0, 1, step=1, splits=12)
```
This is because `PythonFunction` holds `Array[Byte]`, and `equals` method of array equals only when the both array is the same instance.
### Does this PR introduce _any_ user-facing change?
Yes, if the user reuse the Python function for the UDF, the cache manager will detect the same function and use the cache for it.
### How was this patch tested?
I added a test case and manually.
```py
>>> df.select(udf(func)("id")).explain()
== Physical Plan ==
InMemoryTableScan [<lambda>(id)#12]
+- InMemoryRelation [<lambda>(id)#12], StorageLevel(disk, memory, deserialized, 1 replicas)
+- *(2) Project [pythonUDF0#5 AS <lambda>(id)#3]
+- BatchEvalPython [<lambda>(id#0L)], [pythonUDF0#5]
+- *(1) Range (0, 1, step=1, splits=12)
```
Closes#28774 from ueshin/issues/SPARK-31945/udf_cache.
Authored-by: Takuya UESHIN <ueshin@databricks.com>
Signed-off-by: HyukjinKwon <gurwls223@apache.org>
### What changes were proposed in this pull request?
This PR proposes to fix wordings in the Python UDF exception error message from:
From:
> An exception was thrown from Python worker in the executor. The below is the Python worker stacktrace.
To:
> An exception was thrown from the Python worker. Please see the stack trace below.
It removes "executor" because Python worker is technically a separate process, and remove the duplicated wording "Python worker" .
### Why are the changes needed?
To give users better exception messages.
### Does this PR introduce _any_ user-facing change?
No, it's in unreleased branches only. If RC3 passes, yes, it will change the exception message.
### How was this patch tested?
Manually tested.
Closes#28762 from HyukjinKwon/SPARK-31849-followup-2.
Authored-by: HyukjinKwon <gurwls223@apache.org>
Signed-off-by: HyukjinKwon <gurwls223@apache.org>
### What changes were proposed in this pull request?
This PR proposes to use existing util `org.apache.spark.util.Utils.exceptionString` for the same codes at:
```python
jwriter = jvm.java.io.StringWriter()
e.printStackTrace(jvm.java.io.PrintWriter(jwriter))
stacktrace = jwriter.toString()
```
### Why are the changes needed?
To deduplicate codes. Plus, less communication between JVM and Py4j.
### Does this PR introduce _any_ user-facing change?
No.
### How was this patch tested?
Manually tested.
Closes#28749 from HyukjinKwon/SPARK-31849-followup.
Authored-by: HyukjinKwon <gurwls223@apache.org>
Signed-off-by: HyukjinKwon <gurwls223@apache.org>
### What changes were proposed in this pull request?
This PR proposes to add one more newline to clearly separate JVM and Python tracebacks:
Before:
```
Traceback (most recent call last):
...
pyspark.sql.utils.AnalysisException: Reference 'column' is ambiguous, could be: column, column.;
JVM stacktrace:
org.apache.spark.sql.AnalysisException: Reference 'column' is ambiguous, could be: column, column.;
...
```
After:
```
Traceback (most recent call last):
...
pyspark.sql.utils.AnalysisException: Reference 'column' is ambiguous, could be: column, column.;
JVM stacktrace:
org.apache.spark.sql.AnalysisException: Reference 'column' is ambiguous, could be: column, column.;
...
```
This is kind of a followup of e69466056f (SPARK-31849).
### Why are the changes needed?
To make it easier to read.
### Does this PR introduce _any_ user-facing change?
It's in the unreleased branches.
### How was this patch tested?
Manually tested.
Closes#28732 from HyukjinKwon/python-minor.
Authored-by: HyukjinKwon <gurwls223@apache.org>
Signed-off-by: HyukjinKwon <gurwls223@apache.org>
### What changes were proposed in this pull request?
Scala:
```scala
scala> spark.range(10).explain("cost")
```
```
== Optimized Logical Plan ==
Range (0, 10, step=1, splits=Some(12)), Statistics(sizeInBytes=80.0 B)
== Physical Plan ==
*(1) Range (0, 10, step=1, splits=12)
```
PySpark:
```python
>>> spark.range(10).explain("cost")
```
```
Traceback (most recent call last):
File "<stdin>", line 1, in <module>
File "/.../spark/python/pyspark/sql/dataframe.py", line 333, in explain
raise TypeError(err_msg)
TypeError: extended (optional) should be provided as bool, got <class 'str'>
```
In addition, it is consistent with other codes too, for example, `DataFrame.sample` also can support `DataFrame.sample(1.0)` and `DataFrame.sample(False)`.
### Why are the changes needed?
To provide the consistent API support across APIs.
### Does this PR introduce _any_ user-facing change?
Nope, it's only changes in unreleased branches.
If this lands to master only, yes, users will be able to set `mode` as `df.explain("...")` in Spark 3.1.
After this PR:
```python
>>> spark.range(10).explain("cost")
```
```
== Optimized Logical Plan ==
Range (0, 10, step=1, splits=Some(12)), Statistics(sizeInBytes=80.0 B)
== Physical Plan ==
*(1) Range (0, 10, step=1, splits=12)
```
### How was this patch tested?
Unittest was added and manually tested as well to make sure:
```python
spark.range(10).explain(True)
spark.range(10).explain(False)
spark.range(10).explain("cost")
spark.range(10).explain(extended="cost")
spark.range(10).explain(mode="cost")
spark.range(10).explain()
spark.range(10).explain(True, "cost")
spark.range(10).explain(1.0)
```
Closes#28711 from HyukjinKwon/SPARK-31895.
Authored-by: HyukjinKwon <gurwls223@apache.org>
Signed-off-by: HyukjinKwon <gurwls223@apache.org>
### What changes were proposed in this pull request?
It increases the timeout for `StreamingLinearRegressionWithTests.test_train_prediction`
```
Traceback (most recent call last):
File "/home/jenkins/workspace/SparkPullRequestBuilder3/python/pyspark/mllib/tests/test_streaming_algorithms.py", line 503, in test_train_prediction
self._eventually(condition)
File "/home/jenkins/workspace/SparkPullRequestBuilder3/python/pyspark/mllib/tests/test_streaming_algorithms.py", line 69, in _eventually
lastValue = condition()
File "/home/jenkins/workspace/SparkPullRequestBuilder3/python/pyspark/mllib/tests/test_streaming_algorithms.py", line 498, in condition
self.assertGreater(errors[1] - errors[-1], 2)
AssertionError: 1.672640157855923 not greater than 2
```
This could likely happen when the PySpark tests run in parallel and it become slow.
### Why are the changes needed?
To make the tests less flaky. Seems it's being reported multiple times:
https://amplab.cs.berkeley.edu/jenkins/job/SparkPullRequestBuilder/123144/consoleFullhttps://amplab.cs.berkeley.edu/jenkins/job/SparkPullRequestBuilder/123146/testReport/https://amplab.cs.berkeley.edu/jenkins/job/SparkPullRequestBuilder/123141/testReport/https://amplab.cs.berkeley.edu/jenkins/job/SparkPullRequestBuilder/123142/testReport/
### Does this PR introduce _any_ user-facing change?
No, test-only.
### How was this patch tested?
Jenkins will test it out.
Closes#28701 from HyukjinKwon/SPARK-29137.
Authored-by: HyukjinKwon <gurwls223@apache.org>
Signed-off-by: Dongjoon Hyun <dongjoon@apache.org>
### What changes were proposed in this pull request?
This PR proposes to make PySpark exception more Pythonic by hiding JVM stacktrace by default. It can be enabled by turning on `spark.sql.pyspark.jvmStacktrace.enabled` configuration.
```
Traceback (most recent call last):
...
pyspark.sql.utils.PythonException:
An exception was thrown from Python worker in the executor. The below is the Python worker stacktrace.
Traceback (most recent call last):
...
```
If this `spark.sql.pyspark.jvmStacktrace.enabled` is enabled, it appends:
```
JVM stacktrace:
org.apache.spark.Exception: ...
...
```
For example, the codes below:
```python
from pyspark.sql.functions import udf
udf
def divide_by_zero(v):
raise v / 0
spark.range(1).select(divide_by_zero("id")).show()
```
will show an error messages that looks like Python exception thrown from the local.
<details>
<summary>Python exception message when <code>spark.sql.pyspark.jvmStacktrace.enabled</code> is off (default)</summary>
```
Traceback (most recent call last):
File "<stdin>", line 1, in <module>
File "/.../spark/python/pyspark/sql/dataframe.py", line 427, in show
print(self._jdf.showString(n, 20, vertical))
File "/.../spark/python/lib/py4j-0.10.9-src.zip/py4j/java_gateway.py", line 1305, in __call__
File "/.../spark/python/pyspark/sql/utils.py", line 131, in deco
raise_from(converted)
File "<string>", line 3, in raise_from
pyspark.sql.utils.PythonException:
An exception was thrown from Python worker in the executor. The below is the Python worker stacktrace.
Traceback (most recent call last):
File "/.../spark/python/lib/pyspark.zip/pyspark/worker.py", line 605, in main
process()
File "/.../spark/python/lib/pyspark.zip/pyspark/worker.py", line 597, in process
serializer.dump_stream(out_iter, outfile)
File "/.../spark/python/lib/pyspark.zip/pyspark/serializers.py", line 223, in dump_stream
self.serializer.dump_stream(self._batched(iterator), stream)
File "/.../spark/python/lib/pyspark.zip/pyspark/serializers.py", line 141, in dump_stream
for obj in iterator:
File "/.../spark/python/lib/pyspark.zip/pyspark/serializers.py", line 212, in _batched
for item in iterator:
File "/.../spark/python/lib/pyspark.zip/pyspark/worker.py", line 450, in mapper
result = tuple(f(*[a[o] for o in arg_offsets]) for (arg_offsets, f) in udfs)
File "/.../spark/python/lib/pyspark.zip/pyspark/worker.py", line 450, in <genexpr>
result = tuple(f(*[a[o] for o in arg_offsets]) for (arg_offsets, f) in udfs)
File "/.../spark/python/lib/pyspark.zip/pyspark/worker.py", line 90, in <lambda>
return lambda *a: f(*a)
File "/.../spark/python/lib/pyspark.zip/pyspark/util.py", line 107, in wrapper
return f(*args, **kwargs)
File "<stdin>", line 3, in divide_by_zero
ZeroDivisionError: division by zero
```
</details>
<details>
<summary>Python exception message when <code>spark.sql.pyspark.jvmStacktrace.enabled</code> is on</summary>
```
Traceback (most recent call last):
File "<stdin>", line 1, in <module>
File "/.../spark/python/pyspark/sql/dataframe.py", line 427, in show
print(self._jdf.showString(n, 20, vertical))
File "/.../spark/python/lib/py4j-0.10.9-src.zip/py4j/java_gateway.py", line 1305, in __call__
File "/.../spark/python/pyspark/sql/utils.py", line 137, in deco
raise_from(converted)
File "<string>", line 3, in raise_from
pyspark.sql.utils.PythonException:
An exception was thrown from Python worker in the executor. The below is the Python worker stacktrace.
Traceback (most recent call last):
File "/.../spark/python/lib/pyspark.zip/pyspark/worker.py", line 605, in main
process()
File "/.../spark/python/lib/pyspark.zip/pyspark/worker.py", line 597, in process
serializer.dump_stream(out_iter, outfile)
File "/.../spark/python/lib/pyspark.zip/pyspark/serializers.py", line 223, in dump_stream
self.serializer.dump_stream(self._batched(iterator), stream)
File "/.../spark/python/lib/pyspark.zip/pyspark/serializers.py", line 141, in dump_stream
for obj in iterator:
File "/.../spark/python/lib/pyspark.zip/pyspark/serializers.py", line 212, in _batched
for item in iterator:
File "/.../spark/python/lib/pyspark.zip/pyspark/worker.py", line 450, in mapper
result = tuple(f(*[a[o] for o in arg_offsets]) for (arg_offsets, f) in udfs)
File "/.../spark/python/lib/pyspark.zip/pyspark/worker.py", line 450, in <genexpr>
result = tuple(f(*[a[o] for o in arg_offsets]) for (arg_offsets, f) in udfs)
File "/.../spark/python/lib/pyspark.zip/pyspark/worker.py", line 90, in <lambda>
return lambda *a: f(*a)
File "/.../spark/python/lib/pyspark.zip/pyspark/util.py", line 107, in wrapper
return f(*args, **kwargs)
File "<stdin>", line 3, in divide_by_zero
ZeroDivisionError: division by zero
JVM stacktrace:
org.apache.spark.SparkException: Job aborted due to stage failure: Task 0 in stage 1.0 failed 4 times, most recent failure: Lost task 0.3 in stage 1.0 (TID 4, 192.168.35.193, executor 0): org.apache.spark.api.python.PythonException: Traceback (most recent call last):
File "/.../spark/python/lib/pyspark.zip/pyspark/worker.py", line 605, in main
process()
File "/.../spark/python/lib/pyspark.zip/pyspark/worker.py", line 597, in process
serializer.dump_stream(out_iter, outfile)
File "/.../spark/python/lib/pyspark.zip/pyspark/serializers.py", line 223, in dump_stream
self.serializer.dump_stream(self._batched(iterator), stream)
File "/.../spark/python/lib/pyspark.zip/pyspark/serializers.py", line 141, in dump_stream
for obj in iterator:
File "/.../spark/python/lib/pyspark.zip/pyspark/serializers.py", line 212, in _batched
for item in iterator:
File "/.../spark/python/lib/pyspark.zip/pyspark/worker.py", line 450, in mapper
result = tuple(f(*[a[o] for o in arg_offsets]) for (arg_offsets, f) in udfs)
File "/.../spark/python/lib/pyspark.zip/pyspark/worker.py", line 450, in <genexpr>
result = tuple(f(*[a[o] for o in arg_offsets]) for (arg_offsets, f) in udfs)
File "/.../spark/python/lib/pyspark.zip/pyspark/worker.py", line 90, in <lambda>
return lambda *a: f(*a)
File "/.../spark/python/lib/pyspark.zip/pyspark/util.py", line 107, in wrapper
return f(*args, **kwargs)
File "<stdin>", line 3, in divide_by_zero
ZeroDivisionError: division by zero
at org.apache.spark.api.python.BasePythonRunner$ReaderIterator.handlePythonException(PythonRunner.scala:516)
at org.apache.spark.sql.execution.python.PythonUDFRunner$$anon$2.read(PythonUDFRunner.scala:81)
at org.apache.spark.sql.execution.python.PythonUDFRunner$$anon$2.read(PythonUDFRunner.scala:64)
at org.apache.spark.api.python.BasePythonRunner$ReaderIterator.hasNext(PythonRunner.scala:469)
at org.apache.spark.InterruptibleIterator.hasNext(InterruptibleIterator.scala:37)
at scala.collection.Iterator$$anon$11.hasNext(Iterator.scala:489)
at scala.collection.Iterator$$anon$10.hasNext(Iterator.scala:458)
at scala.collection.Iterator$$anon$10.hasNext(Iterator.scala:458)
at org.apache.spark.sql.catalyst.expressions.GeneratedClass$GeneratedIteratorForCodegenStage2.processNext(Unknown Source)
at org.apache.spark.sql.execution.BufferedRowIterator.hasNext(BufferedRowIterator.java:43)
at org.apache.spark.sql.execution.WholeStageCodegenExec$$anon$1.hasNext(WholeStageCodegenExec.scala:753)
at org.apache.spark.sql.execution.SparkPlan.$anonfun$getByteArrayRdd$1(SparkPlan.scala:340)
at org.apache.spark.rdd.RDD.$anonfun$mapPartitionsInternal$2(RDD.scala:898)
at org.apache.spark.rdd.RDD.$anonfun$mapPartitionsInternal$2$adapted(RDD.scala:898)
at org.apache.spark.rdd.MapPartitionsRDD.compute(MapPartitionsRDD.scala:52)
at org.apache.spark.rdd.RDD.computeOrReadCheckpoint(RDD.scala:373)
at org.apache.spark.rdd.RDD.iterator(RDD.scala:337)
at org.apache.spark.scheduler.ResultTask.runTask(ResultTask.scala:90)
at org.apache.spark.scheduler.Task.run(Task.scala:127)
at org.apache.spark.executor.Executor$TaskRunner.$anonfun$run$3(Executor.scala:469)
at org.apache.spark.util.Utils$.tryWithSafeFinally(Utils.scala:1377)
at org.apache.spark.executor.Executor$TaskRunner.run(Executor.scala:472)
at java.util.concurrent.ThreadPoolExecutor.runWorker(ThreadPoolExecutor.java:1149)
at java.util.concurrent.ThreadPoolExecutor$Worker.run(ThreadPoolExecutor.java:624)
at java.lang.Thread.run(Thread.java:748)
Driver stacktrace:
at org.apache.spark.scheduler.DAGScheduler.failJobAndIndependentStages(DAGScheduler.scala:2117)
at org.apache.spark.scheduler.DAGScheduler.$anonfun$abortStage$2(DAGScheduler.scala:2066)
at org.apache.spark.scheduler.DAGScheduler.$anonfun$abortStage$2$adapted(DAGScheduler.scala:2065)
at scala.collection.mutable.ResizableArray.foreach(ResizableArray.scala:62)
at scala.collection.mutable.ResizableArray.foreach$(ResizableArray.scala:55)
at scala.collection.mutable.ArrayBuffer.foreach(ArrayBuffer.scala:49)
at org.apache.spark.scheduler.DAGScheduler.abortStage(DAGScheduler.scala:2065)
at org.apache.spark.scheduler.DAGScheduler.$anonfun$handleTaskSetFailed$1(DAGScheduler.scala:1021)
at org.apache.spark.scheduler.DAGScheduler.$anonfun$handleTaskSetFailed$1$adapted(DAGScheduler.scala:1021)
at scala.Option.foreach(Option.scala:407)
at org.apache.spark.scheduler.DAGScheduler.handleTaskSetFailed(DAGScheduler.scala:1021)
at org.apache.spark.scheduler.DAGSchedulerEventProcessLoop.doOnReceive(DAGScheduler.scala:2297)
at org.apache.spark.scheduler.DAGSchedulerEventProcessLoop.onReceive(DAGScheduler.scala:2246)
at org.apache.spark.scheduler.DAGSchedulerEventProcessLoop.onReceive(DAGScheduler.scala:2235)
at org.apache.spark.util.EventLoop$$anon$1.run(EventLoop.scala:49)
at org.apache.spark.scheduler.DAGScheduler.runJob(DAGScheduler.scala:823)
at org.apache.spark.SparkContext.runJob(SparkContext.scala:2108)
at org.apache.spark.SparkContext.runJob(SparkContext.scala:2129)
at org.apache.spark.SparkContext.runJob(SparkContext.scala:2148)
at org.apache.spark.sql.execution.SparkPlan.executeTake(SparkPlan.scala:467)
at org.apache.spark.sql.execution.SparkPlan.executeTake(SparkPlan.scala:420)
at org.apache.spark.sql.execution.CollectLimitExec.executeCollect(limit.scala:47)
at org.apache.spark.sql.Dataset.collectFromPlan(Dataset.scala:3653)
at org.apache.spark.sql.Dataset.$anonfun$head$1(Dataset.scala:2695)
at org.apache.spark.sql.Dataset.$anonfun$withAction$1(Dataset.scala:3644)
at org.apache.spark.sql.execution.SQLExecution$.$anonfun$withNewExecutionId$5(SQLExecution.scala:103)
at org.apache.spark.sql.execution.SQLExecution$.withSQLConfPropagated(SQLExecution.scala:163)
at org.apache.spark.sql.execution.SQLExecution$.$anonfun$withNewExecutionId$1(SQLExecution.scala:90)
at org.apache.spark.sql.SparkSession.withActive(SparkSession.scala:763)
at org.apache.spark.sql.execution.SQLExecution$.withNewExecutionId(SQLExecution.scala:64)
at org.apache.spark.sql.Dataset.withAction(Dataset.scala:3642)
at org.apache.spark.sql.Dataset.head(Dataset.scala:2695)
at org.apache.spark.sql.Dataset.take(Dataset.scala:2902)
at org.apache.spark.sql.Dataset.getRows(Dataset.scala:300)
at org.apache.spark.sql.Dataset.showString(Dataset.scala:337)
at sun.reflect.NativeMethodAccessorImpl.invoke0(Native Method)
at sun.reflect.NativeMethodAccessorImpl.invoke(NativeMethodAccessorImpl.java:62)
at sun.reflect.DelegatingMethodAccessorImpl.invoke(DelegatingMethodAccessorImpl.java:43)
at java.lang.reflect.Method.invoke(Method.java:498)
at py4j.reflection.MethodInvoker.invoke(MethodInvoker.java:244)
at py4j.reflection.ReflectionEngine.invoke(ReflectionEngine.java:357)
at py4j.Gateway.invoke(Gateway.java:282)
at py4j.commands.AbstractCommand.invokeMethod(AbstractCommand.java:132)
at py4j.commands.CallCommand.execute(CallCommand.java:79)
at py4j.GatewayConnection.run(GatewayConnection.java:238)
at java.lang.Thread.run(Thread.java:748)
Caused by: org.apache.spark.api.python.PythonException: Traceback (most recent call last):
File "/.../spark/python/lib/pyspark.zip/pyspark/worker.py", line 605, in main
process()
File "/.../spark/python/lib/pyspark.zip/pyspark/worker.py", line 597, in process
serializer.dump_stream(out_iter, outfile)
File "/.../spark/python/lib/pyspark.zip/pyspark/serializers.py", line 223, in dump_stream
self.serializer.dump_stream(self._batched(iterator), stream)
File "/.../spark/python/lib/pyspark.zip/pyspark/serializers.py", line 141, in dump_stream
for obj in iterator:
File "/.../spark/python/lib/pyspark.zip/pyspark/serializers.py", line 212, in _batched
for item in iterator:
File "/.../spark/python/lib/pyspark.zip/pyspark/worker.py", line 450, in mapper
result = tuple(f(*[a[o] for o in arg_offsets]) for (arg_offsets, f) in udfs)
File "/.../spark/python/lib/pyspark.zip/pyspark/worker.py", line 450, in <genexpr>
result = tuple(f(*[a[o] for o in arg_offsets]) for (arg_offsets, f) in udfs)
File "/.../spark/python/lib/pyspark.zip/pyspark/worker.py", line 90, in <lambda>
return lambda *a: f(*a)
File "/.../spark/python/lib/pyspark.zip/pyspark/util.py", line 107, in wrapper
return f(*args, **kwargs)
File "<stdin>", line 3, in divide_by_zero
ZeroDivisionError: division by zero
at org.apache.spark.api.python.BasePythonRunner$ReaderIterator.handlePythonException(PythonRunner.scala:516)
at org.apache.spark.sql.execution.python.PythonUDFRunner$$anon$2.read(PythonUDFRunner.scala:81)
at org.apache.spark.sql.execution.python.PythonUDFRunner$$anon$2.read(PythonUDFRunner.scala:64)
at org.apache.spark.api.python.BasePythonRunner$ReaderIterator.hasNext(PythonRunner.scala:469)
at org.apache.spark.InterruptibleIterator.hasNext(InterruptibleIterator.scala:37)
at scala.collection.Iterator$$anon$11.hasNext(Iterator.scala:489)
at scala.collection.Iterator$$anon$10.hasNext(Iterator.scala:458)
at scala.collection.Iterator$$anon$10.hasNext(Iterator.scala:458)
at org.apache.spark.sql.catalyst.expressions.GeneratedClass$GeneratedIteratorForCodegenStage2.processNext(Unknown Source)
at org.apache.spark.sql.execution.BufferedRowIterator.hasNext(BufferedRowIterator.java:43)
at org.apache.spark.sql.execution.WholeStageCodegenExec$$anon$1.hasNext(WholeStageCodegenExec.scala:753)
at org.apache.spark.sql.execution.SparkPlan.$anonfun$getByteArrayRdd$1(SparkPlan.scala:340)
at org.apache.spark.rdd.RDD.$anonfun$mapPartitionsInternal$2(RDD.scala:898)
at org.apache.spark.rdd.RDD.$anonfun$mapPartitionsInternal$2$adapted(RDD.scala:898)
at org.apache.spark.rdd.MapPartitionsRDD.compute(MapPartitionsRDD.scala:52)
at org.apache.spark.rdd.RDD.computeOrReadCheckpoint(RDD.scala:373)
at org.apache.spark.rdd.RDD.iterator(RDD.scala:337)
at org.apache.spark.scheduler.ResultTask.runTask(ResultTask.scala:90)
at org.apache.spark.scheduler.Task.run(Task.scala:127)
at org.apache.spark.executor.Executor$TaskRunner.$anonfun$run$3(Executor.scala:469)
at org.apache.spark.util.Utils$.tryWithSafeFinally(Utils.scala:1377)
at org.apache.spark.executor.Executor$TaskRunner.run(Executor.scala:472)
at java.util.concurrent.ThreadPoolExecutor.runWorker(ThreadPoolExecutor.java:1149)
at java.util.concurrent.ThreadPoolExecutor$Worker.run(ThreadPoolExecutor.java:624)
... 1 more
```
</details>
<details>
<summary>Python exception message without this change</summary>
```
Traceback (most recent call last):
File "<stdin>", line 1, in <module>
File "/.../spark/python/pyspark/sql/dataframe.py", line 427, in show
print(self._jdf.showString(n, 20, vertical))
File "/.../spark/python/lib/py4j-0.10.9-src.zip/py4j/java_gateway.py", line 1305, in __call__
File "/.../spark/python/pyspark/sql/utils.py", line 98, in deco
return f(*a, **kw)
File "/.../spark/python/lib/py4j-0.10.9-src.zip/py4j/protocol.py", line 328, in get_return_value
py4j.protocol.Py4JJavaError: An error occurred while calling o160.showString.
: org.apache.spark.SparkException: Job aborted due to stage failure: Task 10 in stage 5.0 failed 4 times, most recent failure: Lost task 10.3 in stage 5.0 (TID 37, 192.168.35.193, executor 3): org.apache.spark.api.python.PythonException: Traceback (most recent call last):
File "/.../spark/python/lib/pyspark.zip/pyspark/worker.py", line 605, in main
process()
File "/.../spark/python/lib/pyspark.zip/pyspark/worker.py", line 597, in process
serializer.dump_stream(out_iter, outfile)
File "/.../spark/python/lib/pyspark.zip/pyspark/serializers.py", line 223, in dump_stream
self.serializer.dump_stream(self._batched(iterator), stream)
File "/.../spark/python/lib/pyspark.zip/pyspark/serializers.py", line 141, in dump_stream
for obj in iterator:
File "/.../spark/python/lib/pyspark.zip/pyspark/serializers.py", line 212, in _batched
for item in iterator:
File "/.../spark/python/lib/pyspark.zip/pyspark/worker.py", line 450, in mapper
result = tuple(f(*[a[o] for o in arg_offsets]) for (arg_offsets, f) in udfs)
File "/.../spark/python/lib/pyspark.zip/pyspark/worker.py", line 450, in <genexpr>
result = tuple(f(*[a[o] for o in arg_offsets]) for (arg_offsets, f) in udfs)
File "/.../spark/python/lib/pyspark.zip/pyspark/worker.py", line 90, in <lambda>
return lambda *a: f(*a)
File "/.../spark/python/lib/pyspark.zip/pyspark/util.py", line 107, in wrapper
return f(*args, **kwargs)
File "<stdin>", line 3, in divide_by_zero
ZeroDivisionError: division by zero
at org.apache.spark.api.python.BasePythonRunner$ReaderIterator.handlePythonException(PythonRunner.scala:516)
at org.apache.spark.sql.execution.python.PythonUDFRunner$$anon$2.read(PythonUDFRunner.scala:81)
at org.apache.spark.sql.execution.python.PythonUDFRunner$$anon$2.read(PythonUDFRunner.scala:64)
at org.apache.spark.api.python.BasePythonRunner$ReaderIterator.hasNext(PythonRunner.scala:469)
at org.apache.spark.InterruptibleIterator.hasNext(InterruptibleIterator.scala:37)
at scala.collection.Iterator$$anon$11.hasNext(Iterator.scala:489)
at scala.collection.Iterator$$anon$10.hasNext(Iterator.scala:458)
at scala.collection.Iterator$$anon$10.hasNext(Iterator.scala:458)
at org.apache.spark.sql.catalyst.expressions.GeneratedClass$GeneratedIteratorForCodegenStage2.processNext(Unknown Source)
at org.apache.spark.sql.execution.BufferedRowIterator.hasNext(BufferedRowIterator.java:43)
at org.apache.spark.sql.execution.WholeStageCodegenExec$$anon$1.hasNext(WholeStageCodegenExec.scala:753)
at org.apache.spark.sql.execution.SparkPlan.$anonfun$getByteArrayRdd$1(SparkPlan.scala:340)
at org.apache.spark.rdd.RDD.$anonfun$mapPartitionsInternal$2(RDD.scala:898)
at org.apache.spark.rdd.RDD.$anonfun$mapPartitionsInternal$2$adapted(RDD.scala:898)
at org.apache.spark.rdd.MapPartitionsRDD.compute(MapPartitionsRDD.scala:52)
at org.apache.spark.rdd.RDD.computeOrReadCheckpoint(RDD.scala:373)
at org.apache.spark.rdd.RDD.iterator(RDD.scala:337)
at org.apache.spark.scheduler.ResultTask.runTask(ResultTask.scala:90)
at org.apache.spark.scheduler.Task.run(Task.scala:127)
at org.apache.spark.executor.Executor$TaskRunner.$anonfun$run$3(Executor.scala:469)
at org.apache.spark.util.Utils$.tryWithSafeFinally(Utils.scala:1377)
at org.apache.spark.executor.Executor$TaskRunner.run(Executor.scala:472)
at java.util.concurrent.ThreadPoolExecutor.runWorker(ThreadPoolExecutor.java:1149)
at java.util.concurrent.ThreadPoolExecutor$Worker.run(ThreadPoolExecutor.java:624)
at java.lang.Thread.run(Thread.java:748)
Driver stacktrace:
at org.apache.spark.scheduler.DAGScheduler.failJobAndIndependentStages(DAGScheduler.scala:2117)
at org.apache.spark.scheduler.DAGScheduler.$anonfun$abortStage$2(DAGScheduler.scala:2066)
at org.apache.spark.scheduler.DAGScheduler.$anonfun$abortStage$2$adapted(DAGScheduler.scala:2065)
at scala.collection.mutable.ResizableArray.foreach(ResizableArray.scala:62)
at scala.collection.mutable.ResizableArray.foreach$(ResizableArray.scala:55)
at scala.collection.mutable.ArrayBuffer.foreach(ArrayBuffer.scala:49)
at org.apache.spark.scheduler.DAGScheduler.abortStage(DAGScheduler.scala:2065)
at org.apache.spark.scheduler.DAGScheduler.$anonfun$handleTaskSetFailed$1(DAGScheduler.scala:1021)
at org.apache.spark.scheduler.DAGScheduler.$anonfun$handleTaskSetFailed$1$adapted(DAGScheduler.scala:1021)
at scala.Option.foreach(Option.scala:407)
at org.apache.spark.scheduler.DAGScheduler.handleTaskSetFailed(DAGScheduler.scala:1021)
at org.apache.spark.scheduler.DAGSchedulerEventProcessLoop.doOnReceive(DAGScheduler.scala:2297)
at org.apache.spark.scheduler.DAGSchedulerEventProcessLoop.onReceive(DAGScheduler.scala:2246)
at org.apache.spark.scheduler.DAGSchedulerEventProcessLoop.onReceive(DAGScheduler.scala:2235)
at org.apache.spark.util.EventLoop$$anon$1.run(EventLoop.scala:49)
at org.apache.spark.scheduler.DAGScheduler.runJob(DAGScheduler.scala:823)
at org.apache.spark.SparkContext.runJob(SparkContext.scala:2108)
at org.apache.spark.SparkContext.runJob(SparkContext.scala:2129)
at org.apache.spark.SparkContext.runJob(SparkContext.scala:2148)
at org.apache.spark.sql.execution.SparkPlan.executeTake(SparkPlan.scala:467)
at org.apache.spark.sql.execution.SparkPlan.executeTake(SparkPlan.scala:420)
at org.apache.spark.sql.execution.CollectLimitExec.executeCollect(limit.scala:47)
at org.apache.spark.sql.Dataset.collectFromPlan(Dataset.scala:3653)
at org.apache.spark.sql.Dataset.$anonfun$head$1(Dataset.scala:2695)
at org.apache.spark.sql.Dataset.$anonfun$withAction$1(Dataset.scala:3644)
at org.apache.spark.sql.execution.SQLExecution$.$anonfun$withNewExecutionId$5(SQLExecution.scala:103)
at org.apache.spark.sql.execution.SQLExecution$.withSQLConfPropagated(SQLExecution.scala:163)
at org.apache.spark.sql.execution.SQLExecution$.$anonfun$withNewExecutionId$1(SQLExecution.scala:90)
at org.apache.spark.sql.SparkSession.withActive(SparkSession.scala:763)
at org.apache.spark.sql.execution.SQLExecution$.withNewExecutionId(SQLExecution.scala:64)
at org.apache.spark.sql.Dataset.withAction(Dataset.scala:3642)
at org.apache.spark.sql.Dataset.head(Dataset.scala:2695)
at org.apache.spark.sql.Dataset.take(Dataset.scala:2902)
at org.apache.spark.sql.Dataset.getRows(Dataset.scala:300)
at org.apache.spark.sql.Dataset.showString(Dataset.scala:337)
at sun.reflect.NativeMethodAccessorImpl.invoke0(Native Method)
at sun.reflect.NativeMethodAccessorImpl.invoke(NativeMethodAccessorImpl.java:62)
at sun.reflect.DelegatingMethodAccessorImpl.invoke(DelegatingMethodAccessorImpl.java:43)
at java.lang.reflect.Method.invoke(Method.java:498)
at py4j.reflection.MethodInvoker.invoke(MethodInvoker.java:244)
at py4j.reflection.ReflectionEngine.invoke(ReflectionEngine.java:357)
at py4j.Gateway.invoke(Gateway.java:282)
at py4j.commands.AbstractCommand.invokeMethod(AbstractCommand.java:132)
at py4j.commands.CallCommand.execute(CallCommand.java:79)
at py4j.GatewayConnection.run(GatewayConnection.java:238)
at java.lang.Thread.run(Thread.java:748)
Caused by: org.apache.spark.api.python.PythonException: Traceback (most recent call last):
File "/.../spark/python/lib/pyspark.zip/pyspark/worker.py", line 605, in main
process()
File "/.../spark/python/lib/pyspark.zip/pyspark/worker.py", line 597, in process
serializer.dump_stream(out_iter, outfile)
File "/.../spark/python/lib/pyspark.zip/pyspark/serializers.py", line 223, in dump_stream
self.serializer.dump_stream(self._batched(iterator), stream)
File "/.../spark/python/lib/pyspark.zip/pyspark/serializers.py", line 141, in dump_stream
for obj in iterator:
File "/.../spark/python/lib/pyspark.zip/pyspark/serializers.py", line 212, in _batched
for item in iterator:
File "/.../spark/python/lib/pyspark.zip/pyspark/worker.py", line 450, in mapper
result = tuple(f(*[a[o] for o in arg_offsets]) for (arg_offsets, f) in udfs)
File "/.../spark/python/lib/pyspark.zip/pyspark/worker.py", line 450, in <genexpr>
result = tuple(f(*[a[o] for o in arg_offsets]) for (arg_offsets, f) in udfs)
File "/.../spark/python/lib/pyspark.zip/pyspark/worker.py", line 90, in <lambda>
return lambda *a: f(*a)
File "/.../spark/python/lib/pyspark.zip/pyspark/util.py", line 107, in wrapper
return f(*args, **kwargs)
File "<stdin>", line 3, in divide_by_zero
ZeroDivisionError: division by zero
at org.apache.spark.api.python.BasePythonRunner$ReaderIterator.handlePythonException(PythonRunner.scala:516)
at org.apache.spark.sql.execution.python.PythonUDFRunner$$anon$2.read(PythonUDFRunner.scala:81)
at org.apache.spark.sql.execution.python.PythonUDFRunner$$anon$2.read(PythonUDFRunner.scala:64)
at org.apache.spark.api.python.BasePythonRunner$ReaderIterator.hasNext(PythonRunner.scala:469)
at org.apache.spark.InterruptibleIterator.hasNext(InterruptibleIterator.scala:37)
at scala.collection.Iterator$$anon$11.hasNext(Iterator.scala:489)
at scala.collection.Iterator$$anon$10.hasNext(Iterator.scala:458)
at scala.collection.Iterator$$anon$10.hasNext(Iterator.scala:458)
at org.apache.spark.sql.catalyst.expressions.GeneratedClass$GeneratedIteratorForCodegenStage2.processNext(Unknown Source)
at org.apache.spark.sql.execution.BufferedRowIterator.hasNext(BufferedRowIterator.java:43)
at org.apache.spark.sql.execution.WholeStageCodegenExec$$anon$1.hasNext(WholeStageCodegenExec.scala:753)
at org.apache.spark.sql.execution.SparkPlan.$anonfun$getByteArrayRdd$1(SparkPlan.scala:340)
at org.apache.spark.rdd.RDD.$anonfun$mapPartitionsInternal$2(RDD.scala:898)
at org.apache.spark.rdd.RDD.$anonfun$mapPartitionsInternal$2$adapted(RDD.scala:898)
at org.apache.spark.rdd.MapPartitionsRDD.compute(MapPartitionsRDD.scala:52)
at org.apache.spark.rdd.RDD.computeOrReadCheckpoint(RDD.scala:373)
at org.apache.spark.rdd.RDD.iterator(RDD.scala:337)
at org.apache.spark.scheduler.ResultTask.runTask(ResultTask.scala:90)
at org.apache.spark.scheduler.Task.run(Task.scala:127)
at org.apache.spark.executor.Executor$TaskRunner.$anonfun$run$3(Executor.scala:469)
at org.apache.spark.util.Utils$.tryWithSafeFinally(Utils.scala:1377)
at org.apache.spark.executor.Executor$TaskRunner.run(Executor.scala:472)
at java.util.concurrent.ThreadPoolExecutor.runWorker(ThreadPoolExecutor.java:1149)
at java.util.concurrent.ThreadPoolExecutor$Worker.run(ThreadPoolExecutor.java:624)
... 1 more
```
</details>
<br/>
Another example with Python 3.7:
```python
sql("a")
```
<details>
<summary>Python exception message when <code>spark.sql.pyspark.jvmStacktrace.enabled</code> is off (default)</summary>
```
Traceback (most recent call last):
File "<stdin>", line 1, in <module>
File "/.../spark/python/pyspark/sql/session.py", line 646, in sql
return DataFrame(self._jsparkSession.sql(sqlQuery), self._wrapped)
File "/.../spark/python/lib/py4j-0.10.9-src.zip/py4j/java_gateway.py", line 1305, in __call__
File "/.../spark/python/pyspark/sql/utils.py", line 131, in deco
raise_from(converted)
File "<string>", line 3, in raise_from
pyspark.sql.utils.ParseException:
mismatched input 'a' expecting {'(', 'ADD', 'ALTER', 'ANALYZE', 'CACHE', 'CLEAR', 'COMMENT', 'COMMIT', 'CREATE', 'DELETE', 'DESC', 'DESCRIBE', 'DFS', 'DROP', 'EXPLAIN', 'EXPORT', 'FROM', 'GRANT', 'IMPORT', 'INSERT', 'LIST', 'LOAD', 'LOCK', 'MAP', 'MERGE', 'MSCK', 'REDUCE', 'REFRESH', 'REPLACE', 'RESET', 'REVOKE', 'ROLLBACK', 'SELECT', 'SET', 'SHOW', 'START', 'TABLE', 'TRUNCATE', 'UNCACHE', 'UNLOCK', 'UPDATE', 'USE', 'VALUES', 'WITH'}(line 1, pos 0)
== SQL ==
a
^^^
```
</details>
<details>
<summary>Python exception message when <code>spark.sql.pyspark.jvmStacktrace.enabled</code> is on</summary>
```
Traceback (most recent call last):
File "<stdin>", line 1, in <module>
File "/.../spark/python/pyspark/sql/session.py", line 646, in sql
return DataFrame(self._jsparkSession.sql(sqlQuery), self._wrapped)
File "/.../spark/python/lib/py4j-0.10.9-src.zip/py4j/java_gateway.py", line 1305, in __call__
File "/.../spark/python/pyspark/sql/utils.py", line 131, in deco
raise_from(converted)
File "<string>", line 3, in raise_from
pyspark.sql.utils.ParseException:
mismatched input 'a' expecting {'(', 'ADD', 'ALTER', 'ANALYZE', 'CACHE', 'CLEAR', 'COMMENT', 'COMMIT', 'CREATE', 'DELETE', 'DESC', 'DESCRIBE', 'DFS', 'DROP', 'EXPLAIN', 'EXPORT', 'FROM', 'GRANT', 'IMPORT', 'INSERT', 'LIST', 'LOAD', 'LOCK', 'MAP', 'MERGE', 'MSCK', 'REDUCE', 'REFRESH', 'REPLACE', 'RESET', 'REVOKE', 'ROLLBACK', 'SELECT', 'SET', 'SHOW', 'START', 'TABLE', 'TRUNCATE', 'UNCACHE', 'UNLOCK', 'UPDATE', 'USE', 'VALUES', 'WITH'}(line 1, pos 0)
== SQL ==
a
^^^
JVM stacktrace:
org.apache.spark.sql.catalyst.parser.ParseException:
mismatched input 'a' expecting {'(', 'ADD', 'ALTER', 'ANALYZE', 'CACHE', 'CLEAR', 'COMMENT', 'COMMIT', 'CREATE', 'DELETE', 'DESC', 'DESCRIBE', 'DFS', 'DROP', 'EXPLAIN', 'EXPORT', 'FROM', 'GRANT', 'IMPORT', 'INSERT', 'LIST', 'LOAD', 'LOCK', 'MAP', 'MERGE', 'MSCK', 'REDUCE', 'REFRESH', 'REPLACE', 'RESET', 'REVOKE', 'ROLLBACK', 'SELECT', 'SET', 'SHOW', 'START', 'TABLE', 'TRUNCATE', 'UNCACHE', 'UNLOCK', 'UPDATE', 'USE', 'VALUES', 'WITH'}(line 1, pos 0)
== SQL ==
a
^^^
at org.apache.spark.sql.catalyst.parser.ParseException.withCommand(ParseDriver.scala:266)
at org.apache.spark.sql.catalyst.parser.AbstractSqlParser.parse(ParseDriver.scala:133)
at org.apache.spark.sql.execution.SparkSqlParser.parse(SparkSqlParser.scala:49)
at org.apache.spark.sql.catalyst.parser.AbstractSqlParser.parsePlan(ParseDriver.scala:81)
at org.apache.spark.sql.SparkSession.$anonfun$sql$2(SparkSession.scala:604)
at org.apache.spark.sql.catalyst.QueryPlanningTracker.measurePhase(QueryPlanningTracker.scala:111)
at org.apache.spark.sql.SparkSession.$anonfun$sql$1(SparkSession.scala:604)
at org.apache.spark.sql.SparkSession.withActive(SparkSession.scala:763)
at org.apache.spark.sql.SparkSession.sql(SparkSession.scala:601)
at sun.reflect.NativeMethodAccessorImpl.invoke0(Native Method)
at sun.reflect.NativeMethodAccessorImpl.invoke(NativeMethodAccessorImpl.java:62)
at sun.reflect.DelegatingMethodAccessorImpl.invoke(DelegatingMethodAccessorImpl.java:43)
at java.lang.reflect.Method.invoke(Method.java:498)
at py4j.reflection.MethodInvoker.invoke(MethodInvoker.java:244)
at py4j.reflection.ReflectionEngine.invoke(ReflectionEngine.java:357)
at py4j.Gateway.invoke(Gateway.java:282)
at py4j.commands.AbstractCommand.invokeMethod(AbstractCommand.java:132)
at py4j.commands.CallCommand.execute(CallCommand.java:79)
at py4j.GatewayConnection.run(GatewayConnection.java:238)
at java.lang.Thread.run(Thread.java:748)
```
</details>
<details>
<summary>Python exception message without this change</summary>
```
Traceback (most recent call last):
File "/.../spark/python/pyspark/sql/utils.py", line 98, in deco
return f(*a, **kw)
File "/.../spark/python/lib/py4j-0.10.9-src.zip/py4j/protocol.py", line 328, in get_return_value
py4j.protocol.Py4JJavaError: An error occurred while calling o26.sql.
: org.apache.spark.sql.catalyst.parser.ParseException:
mismatched input 'a' expecting {'(', 'ADD', 'ALTER', 'ANALYZE', 'CACHE', 'CLEAR', 'COMMENT', 'COMMIT', 'CREATE', 'DELETE', 'DESC', 'DESCRIBE', 'DFS', 'DROP', 'EXPLAIN', 'EXPORT', 'FROM', 'GRANT', 'IMPORT', 'INSERT', 'LIST', 'LOAD', 'LOCK', 'MAP', 'MERGE', 'MSCK', 'REDUCE', 'REFRESH', 'REPLACE', 'RESET', 'REVOKE', 'ROLLBACK', 'SELECT', 'SET', 'SHOW', 'START', 'TABLE', 'TRUNCATE', 'UNCACHE', 'UNLOCK', 'UPDATE', 'USE', 'VALUES', 'WITH'}(line 1, pos 0)
== SQL ==
a
^^^
at org.apache.spark.sql.catalyst.parser.ParseException.withCommand(ParseDriver.scala:266)
at org.apache.spark.sql.catalyst.parser.AbstractSqlParser.parse(ParseDriver.scala:133)
at org.apache.spark.sql.execution.SparkSqlParser.parse(SparkSqlParser.scala:49)
at org.apache.spark.sql.catalyst.parser.AbstractSqlParser.parsePlan(ParseDriver.scala:81)
at org.apache.spark.sql.SparkSession.$anonfun$sql$2(SparkSession.scala:604)
at org.apache.spark.sql.catalyst.QueryPlanningTracker.measurePhase(QueryPlanningTracker.scala:111)
at org.apache.spark.sql.SparkSession.$anonfun$sql$1(SparkSession.scala:604)
at org.apache.spark.sql.SparkSession.withActive(SparkSession.scala:763)
at org.apache.spark.sql.SparkSession.sql(SparkSession.scala:601)
at sun.reflect.NativeMethodAccessorImpl.invoke0(Native Method)
at sun.reflect.NativeMethodAccessorImpl.invoke(NativeMethodAccessorImpl.java:62)
at sun.reflect.DelegatingMethodAccessorImpl.invoke(DelegatingMethodAccessorImpl.java:43)
at java.lang.reflect.Method.invoke(Method.java:498)
at py4j.reflection.MethodInvoker.invoke(MethodInvoker.java:244)
at py4j.reflection.ReflectionEngine.invoke(ReflectionEngine.java:357)
at py4j.Gateway.invoke(Gateway.java:282)
at py4j.commands.AbstractCommand.invokeMethod(AbstractCommand.java:132)
at py4j.commands.CallCommand.execute(CallCommand.java:79)
at py4j.GatewayConnection.run(GatewayConnection.java:238)
at java.lang.Thread.run(Thread.java:748)
During handling of the above exception, another exception occurred:
Traceback (most recent call last):
File "<stdin>", line 1, in <module>
File "/.../spark/python/pyspark/sql/session.py", line 646, in sql
return DataFrame(self._jsparkSession.sql(sqlQuery), self._wrapped)
File "/.../spark/python/lib/py4j-0.10.9-src.zip/py4j/java_gateway.py", line 1305, in __call__
File "/.../spark/python/pyspark/sql/utils.py", line 102, in deco
raise converted
pyspark.sql.utils.ParseException:
mismatched input 'a' expecting {'(', 'ADD', 'ALTER', 'ANALYZE', 'CACHE', 'CLEAR', 'COMMENT', 'COMMIT', 'CREATE', 'DELETE', 'DESC', 'DESCRIBE', 'DFS', 'DROP', 'EXPLAIN', 'EXPORT', 'FROM', 'GRANT', 'IMPORT', 'INSERT', 'LIST', 'LOAD', 'LOCK', 'MAP', 'MERGE', 'MSCK', 'REDUCE', 'REFRESH', 'REPLACE', 'RESET', 'REVOKE', 'ROLLBACK', 'SELECT', 'SET', 'SHOW', 'START', 'TABLE', 'TRUNCATE', 'UNCACHE', 'UNLOCK', 'UPDATE', 'USE', 'VALUES', 'WITH'}(line 1, pos 0)
== SQL ==
a
^^^
```
</details>
### Why are the changes needed?
Currently, PySpark exceptions are very unfriendly to Python users with causing a bunch of JVM stacktrace. See "Python exception message without this change" above.
### Does this PR introduce _any_ user-facing change?
Yes, it will change the exception message. See the examples above.
### How was this patch tested?
Manually tested by
```bash
./bin/pyspark --conf spark.sql.pyspark.jvmStacktrace.enabled=true
```
and running the examples above.
Closes#28661 from HyukjinKwon/python-debug.
Authored-by: HyukjinKwon <gurwls223@apache.org>
Signed-off-by: HyukjinKwon <gurwls223@apache.org>
### What changes were proposed in this pull request?
This PR manually specifies the class for the input array being used in `(SparkContext|StreamingContext).union`. It fixes a regression introduced from SPARK-25737.
```python
rdd1 = sc.parallelize([1,2,3,4,5])
rdd2 = sc.parallelize([6,7,8,9,10])
pairRDD1 = rdd1.zip(rdd2)
sc.union([pairRDD1, pairRDD1]).collect()
```
in the current master and `branch-3.0`:
```
Traceback (most recent call last):
File "<stdin>", line 1, in <module>
File "/.../spark/python/pyspark/context.py", line 870, in union
jrdds[i] = rdds[i]._jrdd
File "/.../spark/python/lib/py4j-0.10.9-src.zip/py4j/java_collections.py", line 238, in __setitem__
File "/.../spark/python/lib/py4j-0.10.9-src.zip/py4j/java_collections.py", line 221, in __set_item
File "/.../spark/python/lib/py4j-0.10.9-src.zip/py4j/protocol.py", line 332, in get_return_value
py4j.protocol.Py4JError: An error occurred while calling None.None. Trace:
py4j.Py4JException: Cannot convert org.apache.spark.api.java.JavaPairRDD to org.apache.spark.api.java.JavaRDD
at py4j.commands.ArrayCommand.convertArgument(ArrayCommand.java:166)
at py4j.commands.ArrayCommand.setArray(ArrayCommand.java:144)
at py4j.commands.ArrayCommand.execute(ArrayCommand.java:97)
at py4j.GatewayConnection.run(GatewayConnection.java:238)
at java.lang.Thread.run(Thread.java:748)
```
which works in Spark 2.4.5:
```
[(1, 6), (2, 7), (3, 8), (4, 9), (5, 10), (1, 6), (2, 7), (3, 8), (4, 9), (5, 10)]
```
It assumed the class of the input array is the same `JavaRDD` or `JavaDStream`; however, that can be different such as `JavaPairRDD`.
This fix is based on redsanket's initial approach, and will be co-authored.
### Why are the changes needed?
To fix a regression from Spark 2.4.5.
### Does this PR introduce _any_ user-facing change?
No, it's only in unreleased branches. This is to fix a regression.
### How was this patch tested?
Manually tested, and a unittest was added.
Closes#28648 from HyukjinKwon/SPARK-31788.
Authored-by: HyukjinKwon <gurwls223@apache.org>
Signed-off-by: HyukjinKwon <gurwls223@apache.org>
### What changes were proposed in this pull request?
Add instance weight support in LogisticRegressionSummary
### Why are the changes needed?
LogisticRegression, MulticlassClassificationEvaluator and BinaryClassificationEvaluator support instance weight. We should support instance weight in LogisticRegressionSummary too.
### Does this PR introduce _any_ user-facing change?
No
### How was this patch tested?
Add new tests
Closes#28657 from huaxingao/weighted_summary.
Authored-by: Huaxin Gao <huaxing@us.ibm.com>
Signed-off-by: Sean Owen <srowen@gmail.com>
### What changes were proposed in this pull request?
Followup to make assertions from recent test consistent with the rest of the module
### Why are the changes needed?
Better to use assertions from `unittest` and be consistent
### Does this PR introduce _any_ user-facing change?
No
### How was this patch tested?
Existing tests
Closes#28659 from BryanCutler/arrow-category-test-fix-SPARK-25351.
Authored-by: Bryan Cutler <cutlerb@gmail.com>
Signed-off-by: HyukjinKwon <gurwls223@apache.org>
### What changes were proposed in this pull request?
Adds `inputFiles()` method to PySpark `DataFrame`. Using this, PySpark users can list all files constituting a `DataFrame`.
**Before changes:**
```
>>> spark.read.load("examples/src/main/resources/people.json", format="json").inputFiles()
Traceback (most recent call last):
File "<stdin>", line 1, in <module>
File "/***/***/spark/python/pyspark/sql/dataframe.py", line 1388, in __getattr__
"'%s' object has no attribute '%s'" % (self.__class__.__name__, name))
AttributeError: 'DataFrame' object has no attribute 'inputFiles'
```
**After changes:**
```
>>> spark.read.load("examples/src/main/resources/people.json", format="json").inputFiles()
[u'file:///***/***/spark/examples/src/main/resources/people.json']
```
### Why are the changes needed?
This method is already supported for spark with scala and java.
### Does this PR introduce _any_ user-facing change?
Yes, Now users can list all files of a DataFrame using `inputFiles()`
### How was this patch tested?
UT added.
Closes#28652 from iRakson/SPARK-31763.
Authored-by: iRakson <raksonrakesh@gmail.com>
Signed-off-by: HyukjinKwon <gurwls223@apache.org>
Handle Pandas category type while converting from python with Arrow enabled. The category column will be converted to whatever type the category elements are as is the case with Arrow disabled.
### Does this PR introduce any user-facing change?
No
### How was this patch tested?
New unit tests were added for `createDataFrame` and scalar `pandas_udf`
Closes#26585 from jalpan-randeri/feature-pyarrow-dictionary-type.
Authored-by: Jalpan Randeri <randerij@amazon.com>
Signed-off-by: Bryan Cutler <cutlerb@gmail.com>
### What changes were proposed in this pull request?
Add weight support in ClusteringEvaluator
### Why are the changes needed?
Currently, BinaryClassificationEvaluator, RegressionEvaluator, and MulticlassClassificationEvaluator support instance weight, but ClusteringEvaluator doesn't, so we will add instance weight support in ClusteringEvaluator.
### Does this PR introduce _any_ user-facing change?
Yes.
ClusteringEvaluator.setWeightCol
### How was this patch tested?
add new unit test
Closes#28553 from huaxingao/weight_evaluator.
Authored-by: Huaxin Gao <huaxing@us.ibm.com>
Signed-off-by: Sean Owen <srowen@gmail.com>
### What changes were proposed in this pull request?
UnionRDD of PairRDDs causing a bug. The fix is to check for instance type before proceeding
### Why are the changes needed?
Changes are needed to avoid users running into issues with union rdd operation with any other type other than JavaRDD.
### Does this PR introduce _any_ user-facing change?
Yes
Before:
SparkSession available as 'spark'.
>>> rdd1 = sc.parallelize([1,2,3,4,5])
>>> rdd2 = sc.parallelize([6,7,8,9,10])
>>> pairRDD1 = rdd1.zip(rdd2)
>>> unionRDD1 = sc.union([pairRDD1, pairRDD1])
Traceback (most recent call last): File "<stdin>", line 1, in <module> File "/home/gs/spark/latest/python/pyspark/context.py", line 870,
in union jrdds[i] = rdds[i]._jrdd
File "/home/gs/spark/latest/python/lib/py4j-0.10.9-src.zip/py4j/java_collections.py", line 238, in setitem File "/home/gs/spark/latest/python/lib/py4j-0.10.9-src.zip/py4j/java_collections.py", line 221,
in __set_item File "/home/gs/spark/latest/python/lib/py4j-0.10.9-src.zip/py4j/protocol.py", line 332, in get_return_value py4j.protocol.Py4JError: An error occurred while calling None.None. Trace: py4j.Py4JException: Cannot convert org.apache.spark.api.java.JavaPairRDD to org.apache.spark.api.java.JavaRDD at py4j.commands.ArrayCommand.convertArgument(ArrayCommand.java:166) at py4j.commands.ArrayCommand.setArray(ArrayCommand.java:144) at py4j.commands.ArrayCommand.execute(ArrayCommand.java:97) at py4j.GatewayConnection.run(GatewayConnection.java:238) at java.lang.Thread.run(Thread.java:748)
After:
>>> rdd2 = sc.parallelize([6,7,8,9,10])
>>> pairRDD1 = rdd1.zip(rdd2)
>>> unionRDD1 = sc.union([pairRDD1, pairRDD1])
>>> unionRDD1.collect()
[(1, 6), (2, 7), (3, 8), (4, 9), (5, 10), (1, 6), (2, 7), (3, 8), (4, 9), (5, 10)]
### How was this patch tested?
Tested with the reproduced piece of code above manually
Closes#28603 from redsanket/SPARK-31788.
Authored-by: schintap <schintap@verizonmedia.com>
Signed-off-by: HyukjinKwon <gurwls223@apache.org>
### What changes were proposed in this pull request?
This PR proposes to only allow the import of `ResourceInformation` as below:
```
pyspark.resource.ResourceInformation
```
instead of
```
pyspark.ResourceInformation
pyspark.resource.ResourceInformation
```
because `pyspark.resource` is a separate module, and it is documented so.
The constructor of `ResourceInformation` isn't supposed to directly call anyway.
### Why are the changes needed?
To keep the code structure coherent.
### Does this PR introduce _any_ user-facing change?
No, it will be in the unreleased branches.
### How was this patch tested?
Manually tested via importing:
Before:
```python
>>> import pyspark
>>> pyspark.ResourceInformation
<class 'pyspark.resource.information.ResourceInformation'>
>>> pyspark.resource.ResourceInformation
<class 'pyspark.resource.information.ResourceInformation'>
```
After:
```python
>>> import pyspark
>>> pyspark.ResourceInformation
Traceback (most recent call last):
File "<stdin>", line 1, in <module>
AttributeError: module 'pyspark' has no attribute 'ResourceInformation'
>>> pyspark.resource.ResourceInformation
<class 'pyspark.resource.information.ResourceInformation'>
```
Also tested via
```bash
cd python
./run-tests --python-executables=python3 --modules=pyspark-core,pyspark-resource
```
Jenkins will test and existing tests should cover.
Closes#28589 from HyukjinKwon/SPARK-31767.
Authored-by: HyukjinKwon <gurwls223@apache.org>
Signed-off-by: Dongjoon Hyun <dongjoon@apache.org>
### What changes were proposed in this pull request?
This PR is kind of a followup for SPARK-29641 and SPARK-28234. This PR proposes:
1.. Document the new `pyspark.resource` module introduced at 95aec091e4, in PySpark API docs.
2.. Move classes into fewer and simpler modules
Before:
```
pyspark
├── resource
│ ├── executorrequests.py
│ │ ├── class ExecutorResourceRequest
│ │ └── class ExecutorResourceRequests
│ ├── taskrequests.py
│ │ ├── class TaskResourceRequest
│ │ └── class TaskResourceRequests
│ ├── resourceprofilebuilder.py
│ │ └── class ResourceProfileBuilder
│ ├── resourceprofile.py
│ │ └── class ResourceProfile
└── resourceinformation
└── class ResourceInformation
```
After:
```
pyspark
└── resource
├── requests.py
│ ├── class ExecutorResourceRequest
│ ├── class ExecutorResourceRequests
│ ├── class TaskResourceRequest
│ └── class TaskResourceRequests
├── profile.py
│ ├── class ResourceProfileBuilder
│ └── class ResourceProfile
└── information.py
└── class ResourceInformation
```
3.. Minor docstring fix e.g.:
```diff
- param name the name of the resource
- param addresses an array of strings describing the addresses of the resource
+ :param name: the name of the resource
+ :param addresses: an array of strings describing the addresses of the resource
+
+ .. versionadded:: 3.0.0
```
### Why are the changes needed?
To document APIs, and move Python modules to fewer and simpler modules.
### Does this PR introduce _any_ user-facing change?
No, the changes are in unreleased branches.
### How was this patch tested?
Manually tested via:
```bash
cd python
./run-tests --python-executables=python3 --modules=pyspark-core
./run-tests --python-executables=python3 --modules=pyspark-resource
```
Closes#28569 from HyukjinKwon/SPARK-28234-SPARK-29641-followup.
Authored-by: HyukjinKwon <gurwls223@apache.org>
Signed-off-by: Dongjoon Hyun <dongjoon@apache.org>
This commit is published into the public domain.
### What changes were proposed in this pull request?
Some syntax issues in docstrings have been fixed.
### Why are the changes needed?
In some places, the documentation did not render as intended, e.g. parameter documentations were not formatted as such.
### Does this PR introduce any user-facing change?
Slight improvements in documentation.
### How was this patch tested?
Manual testing and `dev/lint-python` run. No new Sphinx warnings arise due to this change.
Closes#28559 from DavidToneian/SPARK-31739.
Authored-by: David Toneian <david@toneian.com>
Signed-off-by: HyukjinKwon <gurwls223@apache.org>
### What changes were proposed in this pull request?
Return LogisticRegressionSummary for multiclass logistic regression evaluate in PySpark
### Why are the changes needed?
Currently we have
```
since("2.0.0")
def evaluate(self, dataset):
if not isinstance(dataset, DataFrame):
raise ValueError("dataset must be a DataFrame but got %s." % type(dataset))
java_blr_summary = self._call_java("evaluate", dataset)
return BinaryLogisticRegressionSummary(java_blr_summary)
```
we should return LogisticRegressionSummary for multiclass logistic regression
### Does this PR introduce _any_ user-facing change?
Yes
return LogisticRegressionSummary instead of BinaryLogisticRegressionSummary for multiclass logistic regression in Python
### How was this patch tested?
unit test
Closes#28503 from huaxingao/lr_summary.
Authored-by: Huaxin Gao <huaxing@us.ibm.com>
Signed-off-by: Sean Owen <srowen@gmail.com>
### What changes were proposed in this pull request?
1, add new param blockSize;
2, if blockSize==1, keep original behavior, code path trainOnRows;
3, if blockSize>1, standardize and stack input vectors to blocks (like ALS/MLP), code path trainOnBlocks
### Why are the changes needed?
performance gain on dense dataset HIGGS:
1, save about 45% RAM;
2, 3X faster with openBLAS
### Does this PR introduce any user-facing change?
add a new expert param `blockSize`
### How was this patch tested?
added testsuites
Closes#27473 from zhengruifeng/blockify_gmm.
Authored-by: zhengruifeng <ruifengz@foxmail.com>
Signed-off-by: zhengruifeng <ruifengz@foxmail.com>
### What changes were proposed in this pull request?
Add Python version of
```
Since("3.1.0")
def test(
dataset: DataFrame,
featuresCol: String,
labelCol: String,
flatten: Boolean): DataFrame
```
### Why are the changes needed?
parity between scala and python
### Does this PR introduce _any_ user-facing change?
yes
new method
```
Since("3.1.0")
def test(
dataset: DataFrame,
featuresCol: String,
labelCol: String,
flatten: Boolean): DataFrame
```
in PySpark ANOVATest/ChisqTest/FValueTest
### How was this patch tested?
New doctest
Closes#28483 from huaxingao/flatten_py.
Authored-by: Huaxin Gao <huaxing@us.ibm.com>
Signed-off-by: Sean Owen <srowen@gmail.com>
### What changes were proposed in this pull request?
1, add new param blockSize;
2, add a new class InstanceBlock;
3, if blockSize==1, keep original behavior; if blockSize>1, stack input vectors to blocks (like ALS/MLP);
4, if blockSize>1, standardize the input outside of optimization procedure;
### Why are the changes needed?
it will obtain performance gain on dense datasets, such as epsilon
1, reduce RAM to persist traing dataset; (save about 40% RAM)
2, use Level-2 BLAS routines; (~10X speedup)
### Does this PR introduce _any_ user-facing change?
Yes, a new param is added
### How was this patch tested?
existing and added testsuites
Closes#28473 from zhengruifeng/blockify_aft.
Authored-by: zhengruifeng <ruifengz@foxmail.com>
Signed-off-by: zhengruifeng <ruifengz@foxmail.com>
### What changes were proposed in this pull request?
Add ANOVASelector and FValueSelector to PySpark
### Why are the changes needed?
ANOVASelector and FValueSelector have been implemented in Scala. We need to implement these in Python as well.
### Does this PR introduce _any_ user-facing change?
Yes. Add Python version of ANOVASelector and FValueSelector
### How was this patch tested?
new doctest
Closes#28464 from huaxingao/selector_py.
Authored-by: Huaxin Gao <huaxing@us.ibm.com>
Signed-off-by: zhengruifeng <ruifengz@foxmail.com>
### What changes were proposed in this pull request?
1, add new param blockSize;
2, add a new class InstanceBlock;
3, if blockSize==1, keep original behavior; if blockSize>1, stack input vectors to blocks (like ALS/MLP);
4, if blockSize>1, standardize the input outside of optimization procedure;
### Why are the changes needed?
it will obtain performance gain on dense datasets, such as `epsilon`
1, reduce RAM to persist traing dataset; (save about 40% RAM)
2, use Level-2 BLAS routines; (up to 6X(squaredError)~12X(huber) speedup)
### Does this PR introduce _any_ user-facing change?
Yes, a new param is added
### How was this patch tested?
existing and added testsuites
Closes#28471 from zhengruifeng/blockify_lir_II.
Authored-by: zhengruifeng <ruifengz@foxmail.com>
Signed-off-by: zhengruifeng <ruifengz@foxmail.com>
### What changes were proposed in this pull request?
The RuntimeReplaceable ones are runtime replaceable, thus, their original parameters are not going to be resolved to PrettyAttribute and remain debug style string if we directly implement their `sql` methods with their parameters' `sql` methods.
This PR is raised with suggestions by maropu and cloud-fan https://github.com/apache/spark/pull/28402/files#r417656589. In this PR, we re-implement the `sql` methods of the RuntimeReplaceable ones with toPettySQL
### Why are the changes needed?
Consistency of schema output between RuntimeReplaceable expressions and normal ones.
For example, `date_format` vs `to_timestamp`, before this PR, they output differently
#### Before
```sql
select date_format(timestamp '2019-10-06', 'yyyy-MM-dd uuuu')
struct<date_format(TIMESTAMP '2019-10-06 00:00:00', yyyy-MM-dd uuuu):string>
select to_timestamp("2019-10-06S10:11:12.12345", "yyyy-MM-dd'S'HH:mm:ss.SSSSSS")
struct<to_timestamp('2019-10-06S10:11:12.12345', 'yyyy-MM-dd\'S\'HH:mm:ss.SSSSSS'):timestamp>
```
#### After
```sql
select date_format(timestamp '2019-10-06', 'yyyy-MM-dd uuuu')
struct<date_format(TIMESTAMP '2019-10-06 00:00:00', yyyy-MM-dd uuuu):string>
select to_timestamp("2019-10-06T10:11:12'12", "yyyy-MM-dd'T'HH:mm:ss''SSSS")
struct<to_timestamp(2019-10-06T10:11:12'12, yyyy-MM-dd'T'HH:mm:ss''SSSS):timestamp>
````
### Does this PR introduce _any_ user-facing change?
Yes, the schema output style changed for the runtime replaceable expressions as shown in the above example
### How was this patch tested?
regenerate all related tests
Closes#28420 from yaooqinn/SPARK-31615.
Authored-by: Kent Yao <yaooqinn@hotmail.com>
Signed-off-by: Takeshi Yamamuro <yamamuro@apache.org>
### What changes were proposed in this pull request?
1, reorg the `fit` method in LR to several blocks (`createModel`, `createBounds`, `createOptimizer`, `createInitCoefWithInterceptMatrix`);
2, add new param blockSize;
3, if blockSize==1, keep original behavior, code path `trainOnRows`;
4, if blockSize>1, standardize and stack input vectors to blocks (like ALS/MLP), code path `trainOnBlocks`
### Why are the changes needed?
On dense dataset `epsilon_normalized.t`:
1, reduce RAM to persist traing dataset; (save about 40% RAM)
2, use Level-2 BLAS routines; (4x ~ 5x faster)
### Does this PR introduce _any_ user-facing change?
Yes, a new param is added
### How was this patch tested?
existing and added testsuites
Closes#28458 from zhengruifeng/blockify_lor_II.
Authored-by: zhengruifeng <ruifengz@foxmail.com>
Signed-off-by: zhengruifeng <ruifengz@foxmail.com>
### What changes were proposed in this pull request?
Add VarianceThresholdSelector to PySpark
### Why are the changes needed?
parity between Scala and Python
### Does this PR introduce any user-facing change?
Yes.
VarianceThresholdSelector is added to PySpark
### How was this patch tested?
new doctest
Closes#28409 from huaxingao/variance_py.
Authored-by: Huaxin Gao <huaxing@us.ibm.com>
Signed-off-by: Sean Owen <srowen@gmail.com>
### What changes were proposed in this pull request?
1, add new param `blockSize`;
2, add a new class InstanceBlock;
3, **if `blockSize==1`, keep original behavior; if `blockSize>1`, stack input vectors to blocks (like ALS/MLP);**
4, if `blockSize>1`, standardize the input outside of optimization procedure;
### Why are the changes needed?
1, reduce RAM to persist traing dataset; (save about 40% RAM)
2, use Level-2 BLAS routines; (4x ~ 5x faster on dataset `epsilon`)
### Does this PR introduce any user-facing change?
Yes, a new param is added
### How was this patch tested?
existing and added testsuites
Closes#28349 from zhengruifeng/blockify_svc_II.
Authored-by: zhengruifeng <ruifengz@foxmail.com>
Signed-off-by: zhengruifeng <ruifengz@foxmail.com>
### What changes were proposed in this pull request?
I add a new API in pyspark RDD class:
def collectWithJobGroup(self, groupId, description, interruptOnCancel=False)
This API do the same thing with `rdd.collect`, but it can specify the job group when do collect.
The purpose of adding this API is, if we use:
```
sc.setJobGroup("group-id...")
rdd.collect()
```
The `setJobGroup` API in pyspark won't work correctly. This related to a bug discussed in
https://issues.apache.org/jira/browse/SPARK-31549
Note:
This PR is a rather temporary workaround for `PYSPARK_PIN_THREAD`, and as a step to migrate to `PYSPARK_PIN_THREAD` smoothly. It targets Spark 3.0.
- `PYSPARK_PIN_THREAD` is unstable at this moment that affects whole PySpark applications.
- It is impossible to make it runtime configuration as it has to be set before JVM is launched.
- There is a thread leak issue between Python and JVM. We should address but it's not a release blocker for Spark 3.0 since the feature is experimental. I plan to handle this after Spark 3.0 due to stability.
Once `PYSPARK_PIN_THREAD` is enabled by default, we should remove this API out ideally. I will target to deprecate this API in Spark 3.1.
### Why are the changes needed?
Fix bug.
### Does this PR introduce any user-facing change?
A develop API in pyspark: `pyspark.RDD. collectWithJobGroup`
### How was this patch tested?
Unit test.
Closes#28395 from WeichenXu123/collect_with_job_group.
Authored-by: Weichen Xu <weichen.xu@databricks.com>
Signed-off-by: HyukjinKwon <gurwls223@apache.org>
### What changes were proposed in this pull request?
This PR proposes to use a different approach instead of breaking it per Micheal's rubric added at https://spark.apache.org/versioning-policy.html. It deprecates the behaviour for now. It will be gradually removed in the future releases.
After this change,
```python
import warnings
warnings.simplefilter("always")
from pyspark.sql.functions import *
df = spark.range(2)
map_col = create_map(lit(0), lit(100), lit(1), lit(200))
df.withColumn("mapped", map_col.getItem(col('id'))).show()
```
```
/.../python/pyspark/sql/column.py:311: DeprecationWarning: A column as 'key' in getItem is
deprecated as of Spark 3.0, and will not be supported in the future release. Use `column[key]`
or `column.key` syntax instead.
DeprecationWarning)
...
```
```python
import warnings
warnings.simplefilter("always")
from pyspark.sql.functions import *
df = spark.range(2)
struct_col = struct(lit(0), lit(100), lit(1), lit(200))
df.withColumn("struct", struct_col.getField(lit("col1"))).show()
```
```
/.../spark/python/pyspark/sql/column.py:336: DeprecationWarning: A column as 'name'
in getField is deprecated as of Spark 3.0, and will not be supported in the future release. Use
`column[name]` or `column.name` syntax instead.
DeprecationWarning)
```
### Why are the changes needed?
To prevent the radical behaviour change after the amended versioning policy.
### Does this PR introduce any user-facing change?
Yes, it will show the deprecated warning message.
### How was this patch tested?
Manually tested.
Closes#28327 from HyukjinKwon/SPARK-29664.
Authored-by: HyukjinKwon <gurwls223@apache.org>
Signed-off-by: HyukjinKwon <gurwls223@apache.org>
### What changes were proposed in this pull request?
Fix Pyspark CrossValidator/TrainValidationSplit with pipeline estimator cannot save and load model.
Most pyspark estimators/transformers inherit `JavaParams`, but some estimators are special (in order to support pure python implemented nested estimators/transformers):
* Pipeline
* OneVsRest
* CrossValidator
* TrainValidationSplit
But note that, currently, in pyspark, estimators listed above, their model reader/writer do NOT support pure python implemented nested estimators/transformers. Because they use java reader/writer wrapper as python side reader/writer.
Pyspark CrossValidator/TrainValidationSplit model reader/writer require all estimators define the `_transfer_param_map_to_java` and `_transfer_param_map_from_java` (used in model read/write).
OneVsRest class already defines the two methods, but Pipeline do not, so it lead to this bug.
In this PR I add `_transfer_param_map_to_java` and `_transfer_param_map_from_java` into Pipeline class.
### Why are the changes needed?
Bug fix.
### Does this PR introduce any user-facing change?
No
### How was this patch tested?
Unit test.
Manually test in pyspark shell:
1) CrossValidator with Simple Pipeline estimator
```
from pyspark.ml import Pipeline
from pyspark.ml.classification import LogisticRegression
from pyspark.ml.evaluation import BinaryClassificationEvaluator
from pyspark.ml.feature import HashingTF, Tokenizer
from pyspark.ml.tuning import CrossValidator, CrossValidatorModel, ParamGridBuilder
training = spark.createDataFrame([
(0, "a b c d e spark", 1.0),
(1, "b d", 0.0),
(2, "spark f g h", 1.0),
(3, "hadoop mapreduce", 0.0),
(4, "b spark who", 1.0),
(5, "g d a y", 0.0),
(6, "spark fly", 1.0),
(7, "was mapreduce", 0.0),
], ["id", "text", "label"])
# Configure an ML pipeline, which consists of tree stages: tokenizer, hashingTF, and lr.
tokenizer = Tokenizer(inputCol="text", outputCol="words")
hashingTF = HashingTF(inputCol=tokenizer.getOutputCol(), outputCol="features")
lr = LogisticRegression(maxIter=10)
pipeline = Pipeline(stages=[tokenizer, hashingTF, lr])
paramGrid = ParamGridBuilder() \
.addGrid(hashingTF.numFeatures, [10, 100, 1000]) \
.addGrid(lr.regParam, [0.1, 0.01]) \
.build()
crossval = CrossValidator(estimator=pipeline,
estimatorParamMaps=paramGrid,
evaluator=BinaryClassificationEvaluator(),
numFolds=2) # use 3+ folds in practice
# Run cross-validation, and choose the best set of parameters.
cvModel = crossval.fit(training)
cvModel.save('/tmp/cv_model001')
CrossValidatorModel.load('/tmp/cv_model001')
```
2) CrossValidator with Pipeline estimator which include a OneVsRest estimator stage, and OneVsRest estimator nest a LogisticRegression estimator.
```
from pyspark.ml.linalg import Vectors
from pyspark.ml import Estimator, Model
from pyspark.ml.classification import LogisticRegression, LogisticRegressionModel, OneVsRest
from pyspark.ml.evaluation import BinaryClassificationEvaluator, \
MulticlassClassificationEvaluator, RegressionEvaluator
from pyspark.ml.linalg import Vectors
from pyspark.ml.param import Param, Params
from pyspark.ml.tuning import CrossValidator, CrossValidatorModel, ParamGridBuilder, \
TrainValidationSplit, TrainValidationSplitModel
from pyspark.sql.functions import rand
from pyspark.testing.mlutils import SparkSessionTestCase
dataset = spark.createDataFrame(
[(Vectors.dense([0.0]), 0.0),
(Vectors.dense([0.4]), 1.0),
(Vectors.dense([0.5]), 0.0),
(Vectors.dense([0.6]), 1.0),
(Vectors.dense([1.0]), 1.0)] * 10,
["features", "label"])
ova = OneVsRest(classifier=LogisticRegression())
lr1 = LogisticRegression().setMaxIter(100)
lr2 = LogisticRegression().setMaxIter(150)
grid = ParamGridBuilder().addGrid(ova.classifier, [lr1, lr2]).build()
evaluator = MulticlassClassificationEvaluator()
pipeline = Pipeline(stages=[ova])
cv = CrossValidator(estimator=pipeline, estimatorParamMaps=grid, evaluator=evaluator)
cvModel = cv.fit(dataset)
cvModel.save('/tmp/model002')
cvModel2 = CrossValidatorModel.load('/tmp/model002')
```
TrainValidationSplit testing code are similar so I do not paste them.
Closes#28279 from WeichenXu123/fix_pipeline_tuning.
Authored-by: Weichen Xu <weichen.xu@databricks.com>
Signed-off-by: Xiangrui Meng <meng@databricks.com>
### What changes were proposed in this pull request?
As part of the Stage level scheduling features, add the Python api's to set resource profiles.
This also adds the functionality to properly apply the pyspark memory configuration when specified in the ResourceProfile. The pyspark memory configuration is being passed in the task local properties. This was an easy way to get it to the PythonRunner that needs it. I modeled this off how the barrier task scheduling is passing the addresses. As part of this I added in the JavaRDD api's because those are needed by python.
### Why are the changes needed?
python api for this feature
### Does this PR introduce any user-facing change?
Yes adds the java and python apis for user to specify a ResourceProfile to use stage level scheduling.
### How was this patch tested?
unit tests and manually tested on yarn. Tests also run to verify it errors properly on standalone and local mode where its not yet supported.
Closes#28085 from tgravescs/SPARK-29641-pr-base.
Lead-authored-by: Thomas Graves <tgraves@nvidia.com>
Co-authored-by: Thomas Graves <tgraves@apache.org>
Signed-off-by: HyukjinKwon <gurwls223@apache.org>
### What changes were proposed in this pull request?
The RC fails to install against Python 2.7 via `pip`. We deprecated but didn't remove Python 2, 3.4 and 3.5 support yet. This PR partially reverts the changes from SPARK-29672 to recover Python 2, 3.4 and 3.5 pip installation.
```bash
python2.7 -m pip install https://dist.apache.org/repos/dist/dev/spark/v3.0.0-rc1-bin/pyspark-3.0.0.tar.gz
```
```
...
Collecting https://dist.apache.org/repos/dist/dev/spark/v3.0.0-rc1-bin/pyspark-3.0.0.tar.gz
Using cached https://dist.apache.org/repos/dist/dev/spark/v3.0.0-rc1-bin/pyspark-3.0.0.tar.gz (203.0 MB)
ERROR: Command errored out with exit status 1:
command: /System/Library/Frameworks/Python.framework/Versions/2.7/Resources/Python.app/Contents/MacOS/Python -c 'import sys, setuptools, tokenize; sys.argv[0] = '"'"'/private/var/folders/_1/bzcp960d0hlb988k90654z2w0000gp/T/pip-req-build-sfCnmZ/setup.py'"'"'; __file__='"'"'/private/var/folders/_1/bzcp960d0hlb988k90654z2w0000gp/T/pip-req-build-sfCnmZ/setup.py'"'"';f=getattr(tokenize, '"'"'open'"'"', open)(__file__);code=f.read().replace('"'"'\r\n'"'"', '"'"'\n'"'"');f.close();exec(compile(code, __file__, '"'"'exec'"'"'))' egg_info --egg-base /private/var/folders/_1/bzcp960d0hlb988k90654z2w0000gp/T/pip-req-build-sfCnmZ/pip-egg-info
cwd: /private/var/folders/_1/bzcp960d0hlb988k90654z2w0000gp/T/pip-req-build-sfCnmZ/
Complete output (6 lines):
Traceback (most recent call last):
File "<string>", line 1, in <module>
File "/private/var/folders/_1/bzcp960d0hlb988k90654z2w0000gp/T/pip-req-build-sfCnmZ/setup.py", line 27
file=sys.stderr)
^
SyntaxError: invalid syntax
----------------------------------------
ERROR: Command errored out with exit status 1: python setup.py egg_info Check the logs for full command output.
```
### Why are the changes needed?
To keep the deprecated support instead of removing.
### Does this PR introduce any user-facing change?
No, it's the change in unreleased branches only yet.
### How was this patch tested?
```bash
./build/mvn -DskipTests -Phive -Phive-thriftserver clean package
cd python
python2.7 setup.py sdist
python2.7 -m pip install dist/pyspark-3.1.0.dev0.tar.gz
```
Closes#28243 from HyukjinKwon/SPARK-29672-followup.
Authored-by: HyukjinKwon <gurwls223@apache.org>
Signed-off-by: HyukjinKwon <gurwls223@apache.org>
### What changes were proposed in this pull request?
1. Combine `BarrierRequestToSync` and `AllGatherRequestToSync` into `RequestToSync`, which is distinguished by `RequestMethod` type.
2. Remove unnecessary Json serialization/deserialization
3. Clean up some codes to make runBarrier() and `BarrierCoordinator` more general
4. Remove unused imports.
### Why are the changes needed?
To make codes simpler for better maintain in the future.
### Does this PR introduce any user-facing change?
No.
### How was this patch tested?
This is pure code refactor, so should be covered by existed tests.
Closes#28117 from Ngone51/refactor_barrier.
Authored-by: yi.wu <yi.wu@databricks.com>
Signed-off-by: Xingbo Jiang <xingbo.jiang@databricks.com>
### What changes were proposed in this pull request?
This PR is adding support duplicated column names for `toPandas` with Arrow execution.
### Why are the changes needed?
When we execute `toPandas()` with Arrow execution, it fails if the column names have duplicates.
```py
>>> spark.sql("select 1 v, 1 v").toPandas()
Traceback (most recent call last):
File "<stdin>", line 1, in <module>
File "/path/to/lib/python3.7/site-packages/pyspark/sql/dataframe.py", line 2132, in toPandas
pdf = table.to_pandas()
File "pyarrow/array.pxi", line 441, in pyarrow.lib._PandasConvertible.to_pandas
File "pyarrow/table.pxi", line 1367, in pyarrow.lib.Table._to_pandas
File "/path/to/lib/python3.7/site-packages/pyarrow/pandas_compat.py", line 653, in table_to_blockmanager
columns = _deserialize_column_index(table, all_columns, column_indexes)
File "/path/to/lib/python3.7/site-packages/pyarrow/pandas_compat.py", line 704, in _deserialize_column_index
columns = _flatten_single_level_multiindex(columns)
File "/path/to/lib/python3.7/site-packages/pyarrow/pandas_compat.py", line 937, in _flatten_single_level_multiindex
raise ValueError('Found non-unique column index')
ValueError: Found non-unique column index
```
### Does this PR introduce any user-facing change?
Yes, previously we will face an error above, but after this PR, we will see the result:
```py
>>> spark.sql("select 1 v, 1 v").toPandas()
v v
0 1 1
```
### How was this patch tested?
Added and modified related tests.
Closes#28210 from ueshin/issues/SPARK-31441/to_pandas.
Authored-by: Takuya UESHIN <ueshin@databricks.com>
Signed-off-by: HyukjinKwon <gurwls223@apache.org>
### What changes were proposed in this pull request?
Update default datetime pattern from `yyyy-MM-dd'T'HH:mm:ss.SSSXXX ` to `yyyy-MM-dd'T'HH:mm:ss[.SSS][XXX] ` for JSON/CSV APIs documentations
### Why are the changes needed?
doc fix
### Does this PR introduce any user-facing change?
Yes, the documentation will change
### How was this patch tested?
Passing Jenkins
Closes#28204 from yaooqinn/SPARK-31414-F.
Authored-by: Kent Yao <yaooqinn@hotmail.com>
Signed-off-by: HyukjinKwon <gurwls223@apache.org>
### What changes were proposed in this pull request?
This PR explicitly mention that the requirement of Iterator of Series to Iterator of Series and Iterator of Multiple Series to Iterator of Series (previously Scalar Iterator pandas UDF).
The actual limitation of this UDF is the same length of the _entire input and output_, instead of each series's length. Namely you can do something as below:
```python
from typing import Iterator, Tuple
import pandas as pd
from pyspark.sql.functions import pandas_udf
pandas_udf("long")
def func(
iterator: Iterator[pd.Series]) -> Iterator[pd.Series]:
return iter([pd.concat(iterator)])
spark.range(100).select(func("id")).show()
```
This characteristic allows you to prefetch the data from the iterator to speed up, compared to the regular Scalar to Scalar (previously Scalar pandas UDF).
### Why are the changes needed?
To document the correct restriction and characteristics of a feature.
### Does this PR introduce any user-facing change?
Yes in the documentation but only in unreleased branches.
### How was this patch tested?
Github Actions should test the documentation build
Closes#28160 from HyukjinKwon/SPARK-30722-followup.
Authored-by: HyukjinKwon <gurwls223@apache.org>
Signed-off-by: HyukjinKwon <gurwls223@apache.org>
### What changes were proposed in this pull request?
This PR proposes to improve the error message from Scalar iterator pandas UDF.
### Why are the changes needed?
To show the correct error messages.
### Does this PR introduce any user-facing change?
Yes, but only in unreleased branches.
```python
import pandas as pd
from pyspark.sql.functions import pandas_udf, PandasUDFType
pandas_udf('long', PandasUDFType.SCALAR_ITER)
def pandas_plus_one(iterator):
for _ in iterator:
yield pd.Series(1)
spark.range(10).repartition(1).select(pandas_plus_one("id")).show()
```
```python
import pandas as pd
from pyspark.sql.functions import pandas_udf, PandasUDFType
pandas_udf('long', PandasUDFType.SCALAR_ITER)
def pandas_plus_one(iterator):
for _ in iterator:
yield pd.Series(list(range(20)))
spark.range(10).repartition(1).select(pandas_plus_one("id")).show()
```
**Before:**
```
RuntimeError: The number of output rows of pandas iterator UDF should
be the same with input rows. The input rows number is 10 but the output
rows number is 1.
```
```
AssertionError: Pandas MAP_ITER UDF outputted more rows than input rows.
```
**After:**
```
RuntimeError: The length of output in Scalar iterator pandas UDF should be
the same with the input's; however, the length of output was 1 and the length
of input was 10.
```
```
AssertionError: Pandas SCALAR_ITER UDF outputted more rows than input rows.
```
### How was this patch tested?
Unittests were fixed accordingly.
Closes#28135 from HyukjinKwon/SPARK-26412-followup.
Authored-by: HyukjinKwon <gurwls223@apache.org>
Signed-off-by: HyukjinKwon <gurwls223@apache.org>
### What changes were proposed in this pull request?
This PR proposes to show a better error message when a user mistakenly installs `pyspark` from PIP but the default `python` does not point out the corresponding `pip`. See https://stackoverflow.com/questions/46286436/running-pyspark-after-pip-install-pyspark/49587560 as an example.
It can be reproduced as below:
I have two Python executables. `python` is Python 3.7, `pip` binds with Python 3.7 and `python2.7` is Python 2.7.
```bash
pip install pyspark
```
```bash
pyspark
```
```
...
Welcome to
____ __
/ __/__ ___ _____/ /__
_\ \/ _ \/ _ `/ __/ '_/
/__ / .__/\_,_/_/ /_/\_\ version 2.4.5
/_/
Using Python version 3.7.3 (default, Mar 27 2019 09:23:15)
SparkSession available as 'spark'.
...
```
```bash
PYSPARK_PYTHON=python2.7 pyspark
```
```
Could not find valid SPARK_HOME while searching ['/Users', '/usr/local/Cellar/python/3.7.5/Frameworks/Python.framework/Versions/3.7/bin']
/usr/local/Cellar/python/3.7.5/Frameworks/Python.framework/Versions/3.7/bin/pyspark: line 24: /bin/load-spark-env.sh: No such file or directory
/usr/local/Cellar/python/3.7.5/Frameworks/Python.framework/Versions/3.7/bin/pyspark: line 77: /bin/spark-submit: No such file or directory
/usr/local/Cellar/python/3.7.5/Frameworks/Python.framework/Versions/3.7/bin/pyspark: line 77: exec: /bin/spark-submit: cannot execute: No such file or directory
```
### Why are the changes needed?
There are multiple questions outside about this error and they have no idea what's going on. See:
- https://stackoverflow.com/questions/46286436/running-pyspark-after-pip-install-pyspark/49587560
- https://stackoverflow.com/questions/45991888/path-issue-could-not-find-valid-spark-home-while-searching
- https://stackoverflow.com/questions/49707239/pyspark-could-not-find-valid-spark-home
- https://stackoverflow.com/questions/55569985/pyspark-could-not-find-valid-spark-home
- https://stackoverflow.com/questions/48296474/error-could-not-find-valid-spark-home-while-searching-pycharm-in-windows
- https://github.com/ContinuumIO/anaconda-issues/issues/8076
The answer is usually setting `SPARK_HOME`; however this isn't completely correct.
It works if you set `SPARK_HOME` because `pyspark` executable script directly imports the library by using `SPARK_HOME` (see https://github.com/apache/spark/blob/master/bin/pyspark#L52-L53) instead of the default package location specified via `python` executable. So, this way you use a package installed in a different Python, which isn't ideal.
### Does this PR introduce any user-facing change?
Yes, it fixes the error message better.
**Before:**
```
Could not find valid SPARK_HOME while searching ['/Users', '/usr/local/Cellar/python/3.7.5/Frameworks/Python.framework/Versions/3.7/bin']
...
```
**After:**
```
Could not find valid SPARK_HOME while searching ['/Users', '/usr/local/Cellar/python/3.7.5/Frameworks/Python.framework/Versions/3.7/bin']
Did you install PySpark via a package manager such as pip or Conda? If so,
PySpark was not found in your Python environment. It is possible your
Python environment does not properly bind with your package manager.
Please check your default 'python' and if you set PYSPARK_PYTHON and/or
PYSPARK_DRIVER_PYTHON environment variables, and see if you can import
PySpark, for example, 'python -c 'import pyspark'.
If you cannot import, you can install by using the Python executable directly,
for example, 'python -m pip install pyspark [--user]'. Otherwise, you can also
explicitly set the Python executable, that has PySpark installed, to
PYSPARK_PYTHON or PYSPARK_DRIVER_PYTHON environment variables, for example,
'PYSPARK_PYTHON=python3 pyspark'.
...
```
### How was this patch tested?
Manually tested as described above.
Closes#28152 from HyukjinKwon/SPARK-31382.
Authored-by: HyukjinKwon <gurwls223@apache.org>
Signed-off-by: HyukjinKwon <gurwls223@apache.org>
### What changes were proposed in this pull request?
This patch proposed to skip predicates on PythonUDFs to be pushdown through Aggregate.
### Why are the changes needed?
The predicates on PythonUDFs cannot be pushdown through Aggregate. Pushed down predicates cannot be evaluate because PythonUDFs cannot be evaluated on Filter and cause error like:
```
Caused by: java.lang.UnsupportedOperationException: Cannot generate code for expression: mean(input[1, struct<bar:bigint>, true].bar)
at org.apache.spark.sql.catalyst.expressions.Unevaluable.doGenCode(Expression.scala:304)
at org.apache.spark.sql.catalyst.expressions.Unevaluable.doGenCode$(Expression.scala:303)
at org.apache.spark.sql.catalyst.expressions.PythonUDF.doGenCode(PythonUDF.scala:52)
at org.apache.spark.sql.catalyst.expressions.Expression.$anonfun$genCode$3(Expression.scala:146)
at scala.Option.getOrElse(Option.scala:189)
at org.apache.spark.sql.catalyst.expressions.Expression.genCode(Expression.scala:141)
at org.apache.spark.sql.catalyst.expressions.CastBase.doGenCode(Cast.scala:821)
at org.apache.spark.sql.catalyst.expressions.Expression.$anonfun$genCode$3(Expression.scala:146)
at scala.Option.getOrElse(Option.scala:189)
```
### Does this PR introduce any user-facing change?
Yes. Previously the predicates on PythonUDFs will be pushdown through Aggregate can cause error. After this change, the query can work.
### How was this patch tested?
Unit test.
Closes#28089 from viirya/SPARK-30921.
Authored-by: Liang-Chi Hsieh <viirya@gmail.com>
Signed-off-by: HyukjinKwon <gurwls223@apache.org>
### What changes were proposed in this pull request?
A small documentation change to clarify that the `rand()` function produces values in `[0.0, 1.0)`.
### Why are the changes needed?
`rand()` uses `Rand()` - which generates values in [0, 1) ([documented here](a1dbcd13a3/sql/catalyst/src/main/scala/org/apache/spark/sql/catalyst/expressions/randomExpressions.scala (L71))). The existing documentation suggests that 1.0 is a possible value returned by rand (i.e for a distribution written as `X ~ U(a, b)`, x can be a or b, so `U[0.0, 1.0]` suggests the value returned could include 1.0).
### Does this PR introduce any user-facing change?
Only documentation changes.
### How was this patch tested?
Documentation changes only.
Closes#28071 from Smeb/master.
Authored-by: Ben Ryves <benjamin.ryves@getyourguide.com>
Signed-off-by: HyukjinKwon <gurwls223@apache.org>
### What changes were proposed in this pull request?
In the PR, I propose to update the doc for the `timeZone` option in JSON/CSV datasources and for the `tz` parameter of the `from_utc_timestamp()`/`to_utc_timestamp()` functions, and to restrict format of config's values to 2 forms:
1. Geographical regions, such as `America/Los_Angeles`.
2. Fixed offsets - a fully resolved offset from UTC. For example, `-08:00`.
### Why are the changes needed?
Other formats such as three-letter time zone IDs are ambitious, and depend on the locale. For example, `CST` could be U.S. `Central Standard Time` and `China Standard Time`. Such formats have been already deprecated in JDK, see [Three-letter time zone IDs](https://docs.oracle.com/javase/8/docs/api/java/util/TimeZone.html).
### Does this PR introduce any user-facing change?
No
### How was this patch tested?
By running `./dev/scalastyle`, and manual testing.
Closes#28051 from MaxGekk/doc-time-zone-option.
Authored-by: Maxim Gekk <max.gekk@gmail.com>
Signed-off-by: Wenchen Fan <wenchen@databricks.com>
### What changes were proposed in this pull request?
Based on the discussion in the mailing list [[Proposal] Modification to Spark's Semantic Versioning Policy](http://apache-spark-developers-list.1001551.n3.nabble.com/Proposal-Modification-to-Spark-s-Semantic-Versioning-Policy-td28938.html) , this PR is to add back the following APIs whose maintenance cost are relatively small.
- functions.toDegrees/toRadians
- functions.approxCountDistinct
- functions.monotonicallyIncreasingId
- Column.!==
- Dataset.explode
- Dataset.registerTempTable
- SQLContext.getOrCreate, setActive, clearActive, constructors
Below is the other removed APIs in the original PR, but not added back in this PR [https://issues.apache.org/jira/browse/SPARK-25908]:
- Remove some AccumulableInfo .apply() methods
- Remove non-label-specific multiclass precision/recall/fScore in favor of accuracy
- Remove unused Python StorageLevel constants
- Remove unused multiclass option in libsvm parsing
- Remove references to deprecated spark configs like spark.yarn.am.port
- Remove TaskContext.isRunningLocally
- Remove ShuffleMetrics.shuffle* methods
- Remove BaseReadWrite.context in favor of session
### Why are the changes needed?
Avoid breaking the APIs that are commonly used.
### Does this PR introduce any user-facing change?
Adding back the APIs that were removed in 3.0 branch does not introduce the user-facing changes, because Spark 3.0 has not been released.
### How was this patch tested?
Added a new test suite for these APIs.
Author: gatorsmile <gatorsmile@gmail.com>
Author: yi.wu <yi.wu@databricks.com>
Closes#27821 from gatorsmile/addAPIBackV2.
### What changes were proposed in this pull request?
This PR proposes to make pandas function APIs (`groupby.(cogroup.)applyInPandas` and `mapInPandas`) to ignore Python type hints.
### Why are the changes needed?
Python type hints are optional. It shouldn't affect where pandas UDFs are not used.
This is also a future work for them to support other type hints. We shouldn't at least throw an exception at this moment.
### Does this PR introduce any user-facing change?
No, it's master-only change.
```python
import pandas as pd
def pandas_plus_one(pdf: pd.DataFrame) -> pd.DataFrame:
return pdf + 1
spark.range(10).groupby('id').applyInPandas(pandas_plus_one, schema="id long").show()
```
```python
import pandas as pd
def pandas_plus_one(left: pd.DataFrame, right: pd.DataFrame) -> pd.DataFrame:
return left + 1
spark.range(10).groupby('id').cogroup(spark.range(10).groupby("id")).applyInPandas(pandas_plus_one, schema="id long").show()
```
```python
from typing import Iterator
import pandas as pd
def pandas_plus_one(iter: Iterator[pd.DataFrame]) -> Iterator[pd.DataFrame]:
return map(lambda v: v + 1, iter)
spark.range(10).mapInPandas(pandas_plus_one, schema="id long").show()
```
**Before:**
Exception
**After:**
```
+---+
| id|
+---+
| 1|
| 2|
| 3|
| 4|
| 5|
| 6|
| 7|
| 8|
| 9|
| 10|
+---+
```
### How was this patch tested?
Closes#28052 from HyukjinKwon/SPARK-31287.
Authored-by: HyukjinKwon <gurwls223@apache.org>
Signed-off-by: HyukjinKwon <gurwls223@apache.org>
### What changes were proposed in this pull request?
Based on the discussion in the mailing list [[Proposal] Modification to Spark's Semantic Versioning Policy](http://apache-spark-developers-list.1001551.n3.nabble.com/Proposal-Modification-to-Spark-s-Semantic-Versioning-Policy-td28938.html) , this PR is to add back the following APIs whose maintenance cost are relatively small.
- HiveContext
- createExternalTable APIs
### Why are the changes needed?
Avoid breaking the APIs that are commonly used.
### Does this PR introduce any user-facing change?
Adding back the APIs that were removed in 3.0 branch does not introduce the user-facing changes, because Spark 3.0 has not been released.
### How was this patch tested?
add a new test suite for createExternalTable APIs.
Closes#27815 from gatorsmile/addAPIsBack.
Lead-authored-by: gatorsmile <gatorsmile@gmail.com>
Co-authored-by: yi.wu <yi.wu@databricks.com>
Signed-off-by: gatorsmile <gatorsmile@gmail.com>
### What changes were proposed in this pull request?
Add ANOVATest and FValueTest to PySpark
### Why are the changes needed?
Parity between Scala and Python.
### Does this PR introduce any user-facing change?
Yes. Python ANOVATest and FValueTest
### How was this patch tested?
doctest
Closes#28012 from huaxingao/stats-python.
Authored-by: Huaxin Gao <huaxing@us.ibm.com>
Signed-off-by: zhengruifeng <ruifengz@foxmail.com>
### What changes were proposed in this pull request?
When `toPandas` API works on duplicate column names produced from operators like join, we see the error like:
```
ValueError: The truth value of a Series is ambiguous. Use a.empty, a.bool(), a.item(), a.any() or a.all().
```
This patch fixes the error in `toPandas` API.
### Why are the changes needed?
To make `toPandas` work on dataframe with duplicate column names.
### Does this PR introduce any user-facing change?
Yes. Previously calling `toPandas` API on a dataframe with duplicate column names will fail. After this patch, it will produce correct result.
### How was this patch tested?
Unit test.
Closes#28025 from viirya/SPARK-31186.
Authored-by: Liang-Chi Hsieh <viirya@gmail.com>
Signed-off-by: HyukjinKwon <gurwls223@apache.org>
### What changes were proposed in this pull request?
the link for `partition discovery` is malformed, because for releases, there will contains` /docs/<version>/` in the full URL.
### Why are the changes needed?
fix doc
### Does this PR introduce any user-facing change?
no
### How was this patch tested?
`SKIP_SCALADOC=1 SKIP_RDOC=1 SKIP_SQLDOC=1 jekyll serve` locally verified
Closes#28017 from yaooqinn/doc.
Authored-by: Kent Yao <yaooqinn@hotmail.com>
Signed-off-by: HyukjinKwon <gurwls223@apache.org>
### What changes were proposed in this pull request?
Fix errors and missing parts for datetime pattern document
1. The pattern we use is similar to DateTimeFormatter and SimpleDateFormat but not identical. So we shouldn't use any of them in the API docs but use a link to the doc of our own.
2. Some pattern letters are missing
3. Some pattern letters are explicitly banned - Set('A', 'c', 'e', 'n', 'N')
4. the second fraction pattern different logic for parsing and formatting
### Why are the changes needed?
fix and improve doc
### Does this PR introduce any user-facing change?
yes, new and updated doc
### How was this patch tested?
pass Jenkins
viewed locally with `jekyll serve`
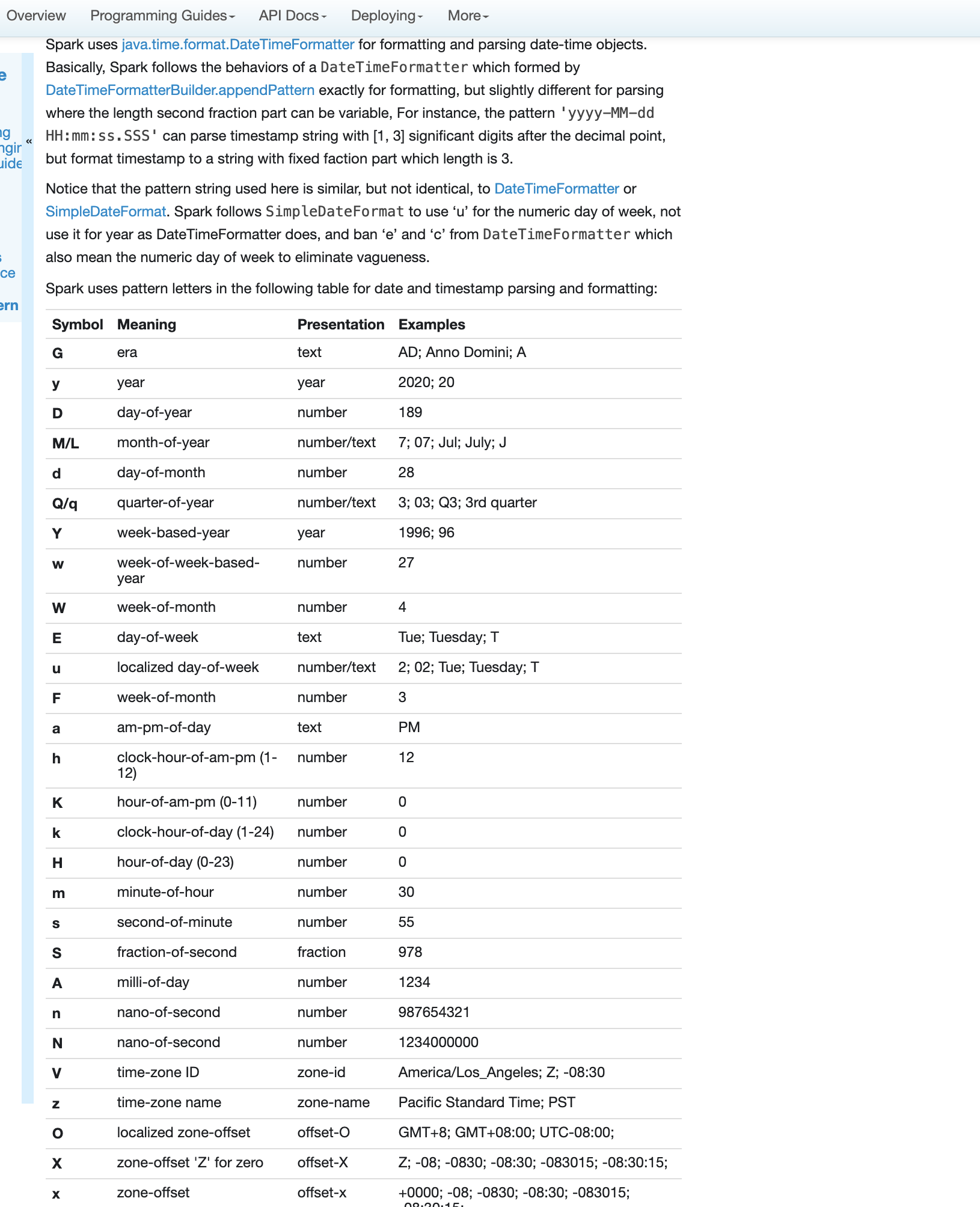
Closes#27956 from yaooqinn/SPARK-31189.
Authored-by: Kent Yao <yaooqinn@hotmail.com>
Signed-off-by: Wenchen Fan <wenchen@databricks.com>
### What changes were proposed in this pull request?
- Adds following overloaded variants to Scala `o.a.s.sql.functions`:
- `percentile_approx(e: Column, percentage: Array[Double], accuracy: Long): Column`
- `percentile_approx(columnName: String, percentage: Array[Double], accuracy: Long): Column`
- `percentile_approx(e: Column, percentage: Double, accuracy: Long): Column`
- `percentile_approx(columnName: String, percentage: Double, accuracy: Long): Column`
- `percentile_approx(e: Column, percentage: Seq[Double], accuracy: Long): Column` (primarily for
Python interop).
- `percentile_approx(columnName: String, percentage: Seq[Double], accuracy: Long): Column`
- Adds `percentile_approx` to `pyspark.sql.functions`.
- Adds `percentile_approx` function to SparkR.
### Why are the changes needed?
Currently we support `percentile_approx` only in SQL expression. It is inconvenient and makes this function relatively unknown.
### Does this PR introduce any user-facing change?
No.
### How was this patch tested?
New unit tests for SparkR an PySpark.
As for now there are no additional tests in Scala API ‒ `ApproximatePercentile` is well tested and Python (including docstrings) and R tests provide additional tests, so it seems unnecessary.
Closes#27278 from zero323/SPARK-30569.
Lead-authored-by: zero323 <mszymkiewicz@gmail.com>
Co-authored-by: HyukjinKwon <gurwls223@apache.org>
Signed-off-by: HyukjinKwon <gurwls223@apache.org>
### What changes were proposed in this pull request?
jira link: https://issues.apache.org/jira/browse/SPARK-30930
Remove ML/MLLIB DeveloperApi annotations.
### Why are the changes needed?
The Developer APIs in ML/MLLIB have been there for a long time. They are stable now and are very unlikely to be changed or removed, so I unmark these Developer APIs in this PR.
### Does this PR introduce any user-facing change?
Yes. DeveloperApi annotations are removed from docs.
### How was this patch tested?
existing tests
Closes#27859 from huaxingao/spark-30930.
Authored-by: Huaxin Gao <huaxing@us.ibm.com>
Signed-off-by: Sean Owen <srowen@gmail.com>
### What changes were proposed in this pull request?
In Spark version 2.4 and earlier, datetime parsing, formatting and conversion are performed by using the hybrid calendar (Julian + Gregorian).
Since the Proleptic Gregorian calendar is de-facto calendar worldwide, as well as the chosen one in ANSI SQL standard, Spark 3.0 switches to it by using Java 8 API classes (the java.time packages that are based on ISO chronology ). The switching job is completed in SPARK-26651.
But after the switching, there are some patterns not compatible between Java 8 and Java 7, Spark needs its own definition on the patterns rather than depends on Java API.
In this PR, we achieve this by writing the document and shadow the incompatible letters. See more details in [SPARK-31030](https://issues.apache.org/jira/browse/SPARK-31030)
### Why are the changes needed?
For backward compatibility.
### Does this PR introduce any user-facing change?
No.
After we define our own datetime parsing and formatting patterns, it's same to old Spark version.
### How was this patch tested?
Existing and new added UT.
Locally document test:
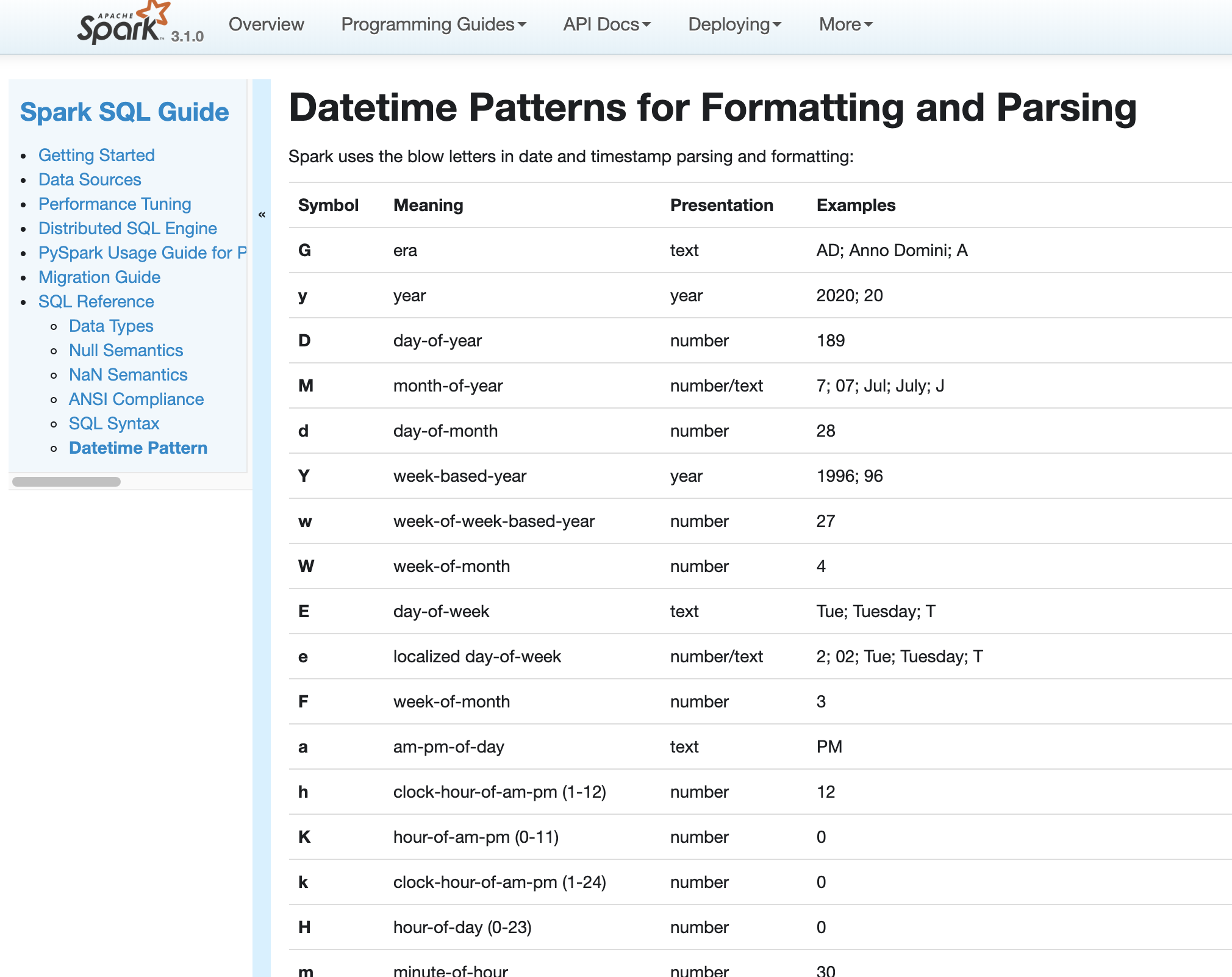
Closes#27830 from xuanyuanking/SPARK-31030.
Authored-by: Yuanjian Li <xyliyuanjian@gmail.com>
Signed-off-by: Wenchen Fan <wenchen@databricks.com>
### What changes were proposed in this pull request?
Adding a note to document `Row.asDict` behavior when there are duplicate fields.
### Why are the changes needed?
When a row contains duplicate fields, `asDict` and `_get_item_` behaves differently. We should document it to let users know the difference explicitly.
### Does this PR introduce any user-facing change?
No. Only document change.
### How was this patch tested?
Existing test.
Closes#27853 from viirya/SPARK-30941.
Authored-by: Liang-Chi Hsieh <viirya@gmail.com>
Signed-off-by: Dongjoon Hyun <dongjoon@apache.org>
### What changes were proposed in this pull request?
Updating ML docs for 3.0 changes
### Why are the changes needed?
I am auditing 3.0 ML changes, found some docs are missing or not updated. Need to update these.
### Does this PR introduce any user-facing change?
Yes, doc changes
### How was this patch tested?
Manually build and check
Closes#27762 from huaxingao/spark-doc.
Authored-by: Huaxin Gao <huaxing@us.ibm.com>
Signed-off-by: Sean Owen <srowen@gmail.com>
### What changes were proposed in this pull request?
Implement common base ML classes (`Predictor`, `PredictionModel`, `Classifier`, `ClasssificationModel` `ProbabilisticClassifier`, `ProbabilisticClasssificationModel`, `Regressor`, `RegrssionModel`) for non-Java backends.
Note
- `Predictor` and `JavaClassifier` should be abstract as `_fit` method is not implemented.
- `PredictionModel` should be abstract as `_transform` is not implemented.
### Why are the changes needed?
To provide extensions points for non-JVM algorithms, as well as a public (as opposed to `Java*` variants, which are commonly described in docstrings as private) hierarchy which can be used to distinguish between different classes of predictors.
For longer discussion see [SPARK-29212](https://issues.apache.org/jira/browse/SPARK-29212) and / or https://github.com/apache/spark/pull/25776.
### Does this PR introduce any user-facing change?
It adds new base classes as listed above, but effective interfaces (method resolution order notwithstanding) stay the same.
Additionally "private" `Java*` classes in`ml.regression` and `ml.classification` have been renamed to follow PEP-8 conventions (added leading underscore).
It is for discussion if the same should be done to equivalent classes from `ml.wrapper`.
If we take `JavaClassifier` as an example, type hierarchy will change from
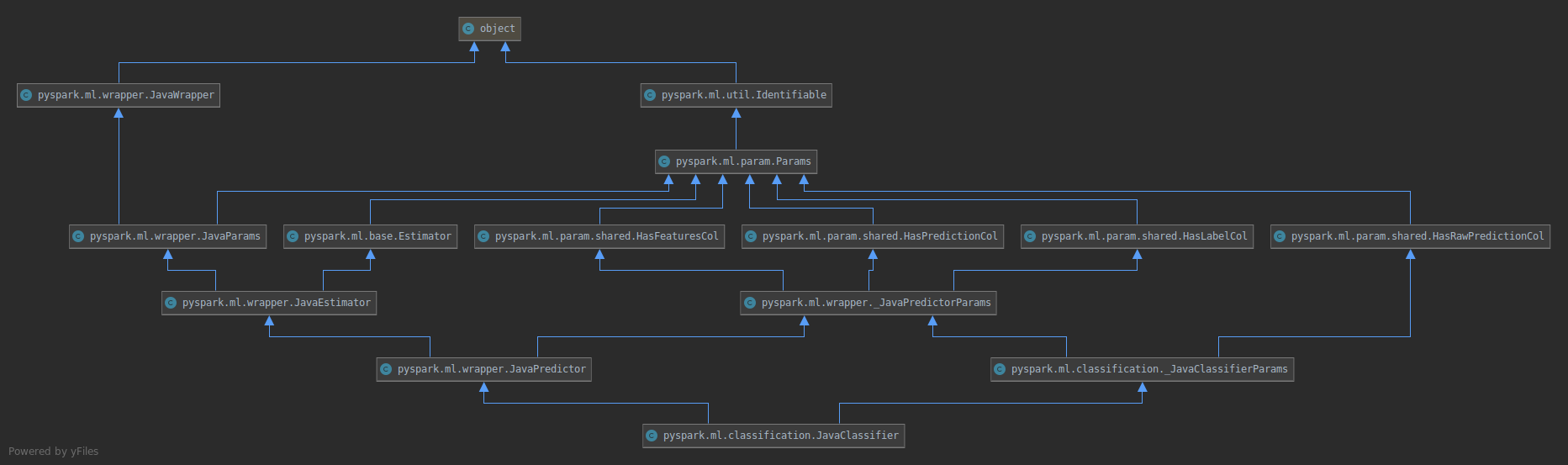
to
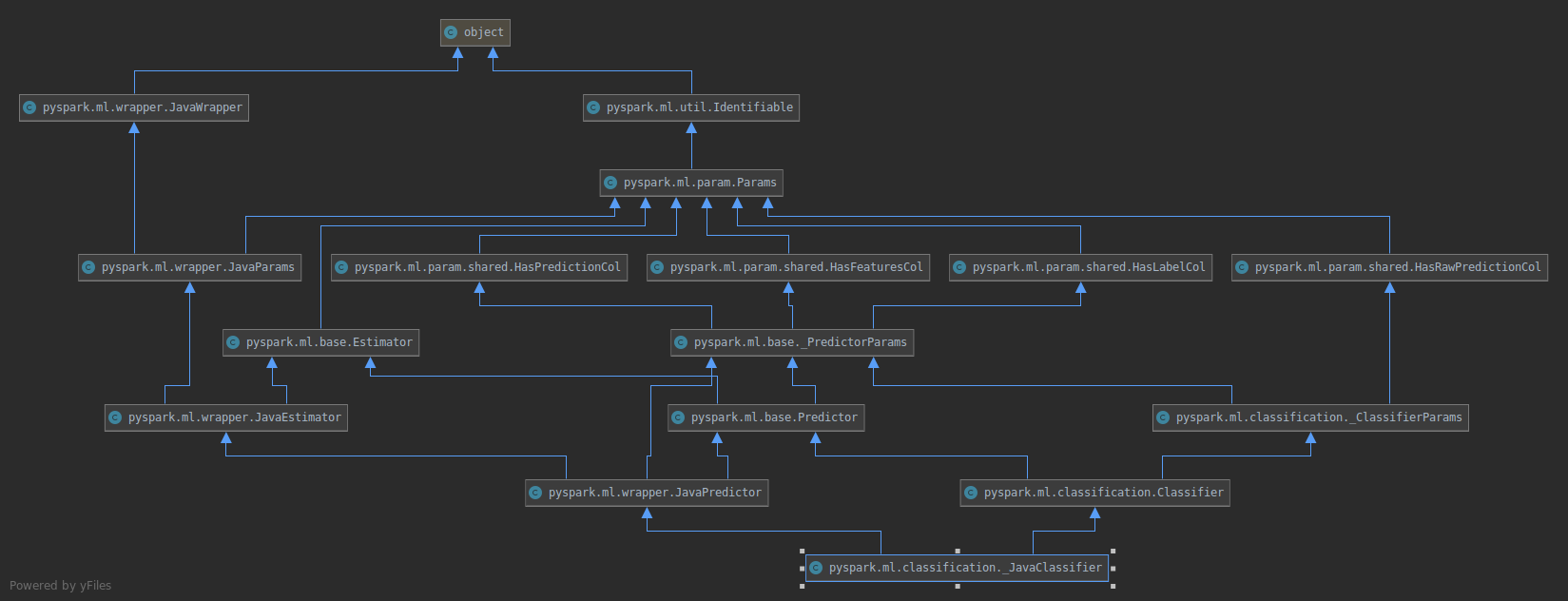
Similarly the old model
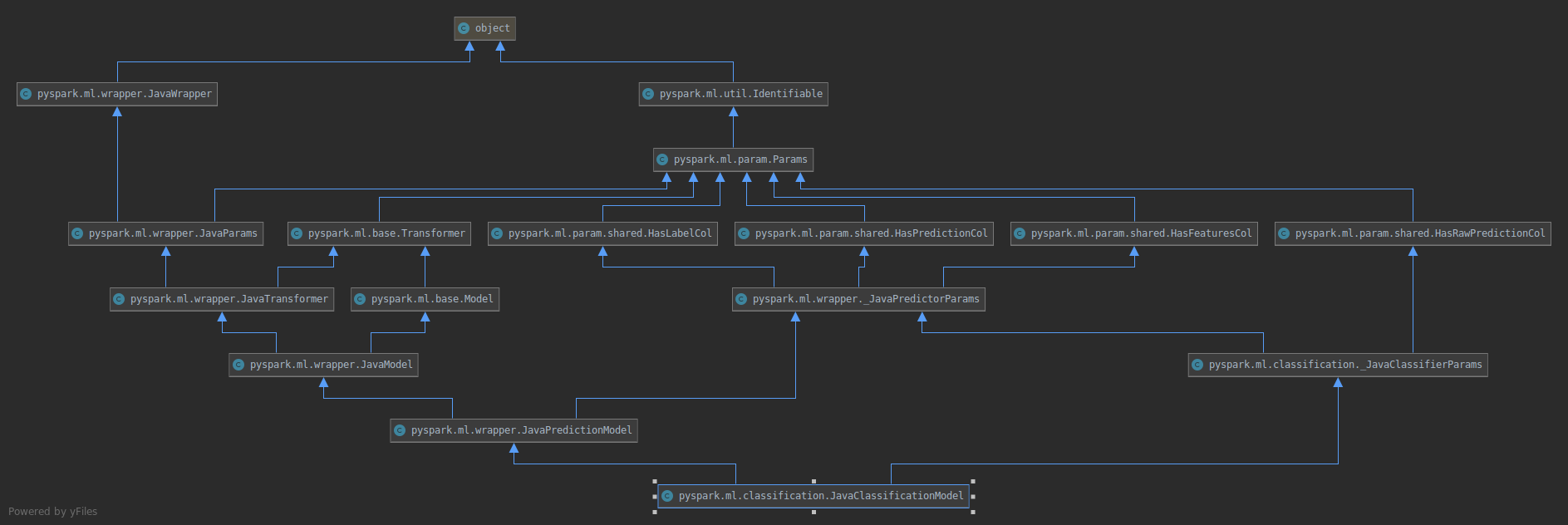
will become
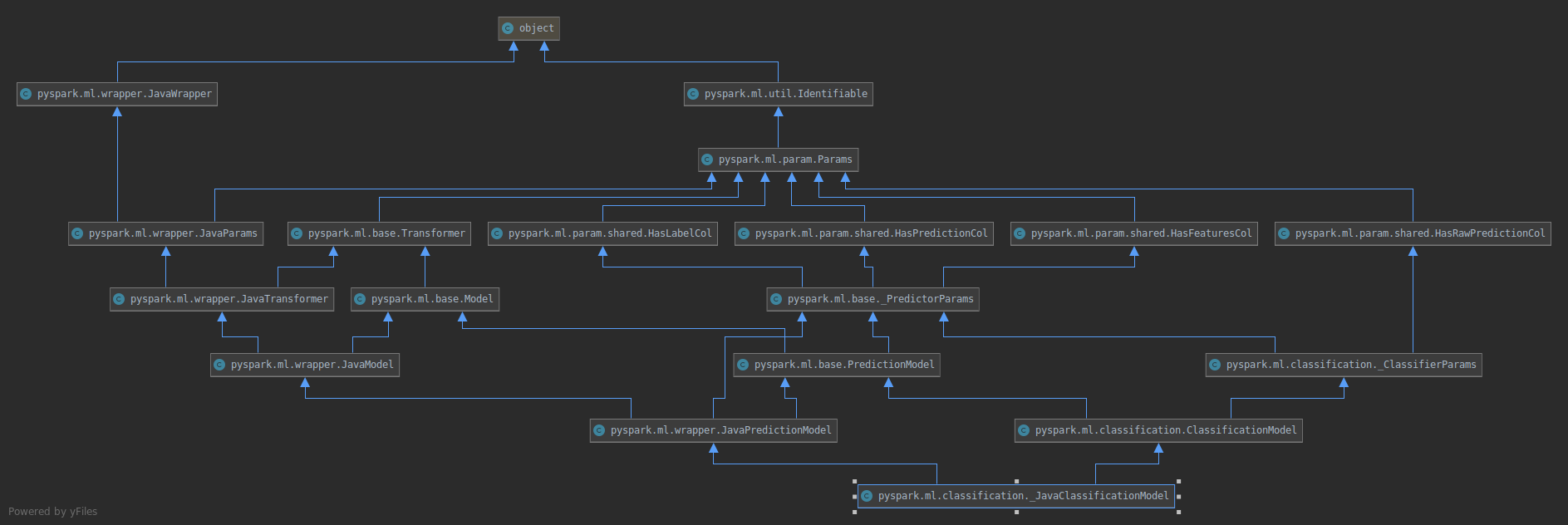
### How was this patch tested?
Existing unit tests.
Closes#27245 from zero323/SPARK-29212.
Authored-by: zero323 <mszymkiewicz@gmail.com>
Signed-off-by: zhengruifeng <ruifengz@foxmail.com>
### What changes were proposed in this pull request?
Current impl needs to convert ml.Vector to breeze.Vector, which can be skipped.
### Why are the changes needed?
avoid unnecessary vector conversions
### Does this PR introduce any user-facing change?
No
### How was this patch tested?
existing testsuites
Closes#27519 from zhengruifeng/gmm_transform_opt.
Authored-by: zhengruifeng <ruifengz@foxmail.com>
Signed-off-by: zhengruifeng <ruifengz@foxmail.com>
### What changes were proposed in this pull request?
Remove automatically resource coordination support from Standalone.
### Why are the changes needed?
Resource coordination is mainly designed for the scenario where multiple workers launched on the same host. However, it's, actually, a non-existed scenario for today's Spark. Because, Spark now can start multiple executors in a single Worker, while it only allow one executor per Worker at very beginning. So, now, it really help nothing for user to launch multiple workers on the same host. Thus, it's not worth for us to bring over complicated implementation and potential high maintain cost for such an impossible scenario.
### Does this PR introduce any user-facing change?
No, it's Spark 3.0 feature.
### How was this patch tested?
Pass Jenkins.
Closes#27722 from Ngone51/abandon_coordination.
Authored-by: yi.wu <yi.wu@databricks.com>
Signed-off-by: Xingbo Jiang <xingbo.jiang@databricks.com>
### What changes were proposed in this pull request?
This PR add Python API for invoking following higher functions:
- `transform`
- `exists`
- `forall`
- `filter`
- `aggregate`
- `zip_with`
- `transform_keys`
- `transform_values`
- `map_filter`
- `map_zip_with`
to `pyspark.sql`. Each of these accepts plain Python functions of one of the following types
- `(Column) -> Column: ...`
- `(Column, Column) -> Column: ...`
- `(Column, Column, Column) -> Column: ...`
Internally this proposal piggbacks on objects supporting Scala implementation ([SPARK-27297](https://issues.apache.org/jira/browse/SPARK-27297)) by:
1. Creating required `UnresolvedNamedLambdaVariables` exposing these as PySpark `Columns`
2. Invoking Python function with these columns as arguments.
3. Using the result, and underlying JVM objects from 1., to create `expressions.LambdaFunction` which is passed to desired expression, and repacked as Python `Column`.
### Why are the changes needed?
Currently higher order functions are available only using SQL and Scala API and can use only SQL expressions
```python
df.selectExpr("transform(values, x -> x + 1)")
```
This works reasonably well for simple functions, but can get really ugly with complex functions (complex functions, casts), resulting objects are somewhat verbose and we don't get any IDE support. Additionally DSL used, though very simple, is not documented.
With changes propose here, above query could be rewritten as:
```python
df.select(transform("values", lambda x: x + 1))
```
### Does this PR introduce any user-facing change?
No.
### How was this patch tested?
- For positive cases this PR adds doctest strings covering possible usage patterns.
- For negative cases (unsupported function types) this PR adds unit tests.
### Notes
If approved, the same approach can be used in SparkR.
Closes#27406 from zero323/SPARK-30681.
Authored-by: zero323 <mszymkiewicz@gmail.com>
Signed-off-by: HyukjinKwon <gurwls223@apache.org>
### What changes were proposed in this pull request?
This patch is to bump the master branch version to 3.1.0-SNAPSHOT.
### Why are the changes needed?
N/A
### Does this PR introduce any user-facing change?
N/A
### How was this patch tested?
N/A
Closes#27698 from gatorsmile/updateVersion.
Authored-by: gatorsmile <gatorsmile@gmail.com>
Signed-off-by: Dongjoon Hyun <dhyun@apple.com>
Fix for #27395
### What changes were proposed in this pull request?
The `allGather` method is added to the `BarrierTaskContext`. This method contains the same functionality as the `BarrierTaskContext.barrier` method; it blocks the task until all tasks make the call, at which time they may continue execution. In addition, the `allGather` method takes an input message. Upon returning from the `allGather` the task receives a list of all the messages sent by all the tasks that made the `allGather` call.
### Why are the changes needed?
There are many situations where having the tasks communicate in a synchronized way is useful. One simple example is if each task needs to start a server to serve requests from one another; first the tasks must find a free port (the result of which is undetermined beforehand) and then start making requests, but to do so they each must know the port chosen by the other task. An `allGather` method would allow them to inform each other of the port they will run on.
### Does this PR introduce any user-facing change?
Yes, an `BarrierTaskContext.allGather` method will be available through the Scala, Java, and Python APIs.
### How was this patch tested?
Most of the code path is already covered by tests to the `barrier` method, since this PR includes a refactor so that much code is shared by the `barrier` and `allGather` methods. However, a test is added to assert that an all gather on each tasks partition ID will return a list of every partition ID.
An example through the Python API:
```python
>>> from pyspark import BarrierTaskContext
>>>
>>> def f(iterator):
... context = BarrierTaskContext.get()
... return [context.allGather('{}'.format(context.partitionId()))]
...
>>> sc.parallelize(range(4), 4).barrier().mapPartitions(f).collect()[0]
[u'3', u'1', u'0', u'2']
```
Closes#27640 from sarthfrey/master.
Lead-authored-by: sarthfrey-db <sarth.frey@databricks.com>
Co-authored-by: sarthfrey <sarth.frey@gmail.com>
Signed-off-by: Xingbo Jiang <xingbo.jiang@databricks.com>
### What changes were proposed in this pull request?
The style of `EXPLAIN FORMATTED` output needs to be improved. We’ve already got some observations/ideas in
https://github.com/apache/spark/pull/27368#discussion_r376694496https://github.com/apache/spark/pull/27368#discussion_r376927143
Observations/Ideas:
1. Using comma as the separator is not clear, especially commas are used inside the expressions too.
2. Show the column counts first? For example, `Results [4]: …`
3. Currently the attribute names are automatically generated, this need to refined.
4. Add arguments field in common implementations as `EXPLAIN EXTENDED` did by calling `argString` in `TreeNode.simpleString`. This will eliminate most existing minor differences between
`EXPLAIN EXTENDED` and `EXPLAIN FORMATTED`.
5. Another improvement we can do is: the generated alias shouldn't include attribute id. collect_set(val, 0, 0)#123 looks clearer than collect_set(val#456, 0, 0)#123
This PR is currently addressing comments 2 & 4, and open for more discussions on improving readability.
### Why are the changes needed?
The readability of `EXPLAIN FORMATTED` need to be improved, which will help user better understand the query plan.
### Does this PR introduce any user-facing change?
Yes, `EXPLAIN FORMATTED` output style changed.
### How was this patch tested?
Update expect results of test cases in explain.sql
Closes#27509 from Eric5553/ExplainFormattedRefine.
Authored-by: Eric Wu <492960551@qq.com>
Signed-off-by: Wenchen Fan <wenchen@databricks.com>
Split off from #27534.
### What changes were proposed in this pull request?
This PR deletes some unused cruft from the Sphinx Makefiles.
### Why are the changes needed?
To remove dead code and the associated maintenance burden.
### Does this PR introduce any user-facing change?
No.
### How was this patch tested?
Tested locally by building the Python docs and reviewing them in my browser.
Closes#27625 from nchammas/SPARK-30731-makefile-cleanup.
Lead-authored-by: Nicholas Chammas <nicholas.chammas@liveramp.com>
Co-authored-by: Nicholas Chammas <nicholas.chammas@gmail.com>
Signed-off-by: HyukjinKwon <gurwls223@apache.org>
This PR aims to upgrade Py4J to `0.10.9` for better Python 3.7 support in Apache Spark 3.0.0 (master/branch-3.0). This is not for `branch-2.4`.
- Apache Spark 3.0.0 is using `Py4J 0.10.8.1` (released on 2018-10-21) because `0.10.8.1` was the first official release to support Python 3.7.
- https://www.py4j.org/changelog.html#py4j-0-10-8-and-py4j-0-10-8-1
- `Py4J 0.10.9` was released on January 25th 2020 with better Python 3.7 support and `magic_member` bug fix.
- https://github.com/bartdag/py4j/releases/tag/0.10.9
- https://www.py4j.org/changelog.html#py4j-0-10-9
No.
Pass the Jenkins with the existing tests.
Closes#27641 from dongjoon-hyun/SPARK-30884.
Authored-by: Dongjoon Hyun <dhyun@apple.com>
Signed-off-by: Dongjoon Hyun <dhyun@apple.com>
### What changes were proposed in this pull request?
As discussed on the Jira ticket, this change clears the SQLContext._instantiatedContext class attribute when the SparkSession is stopped. That way, the attribute will be reset with a new, usable SQLContext when a new SparkSession is started.
### Why are the changes needed?
When the underlying SQLContext is instantiated for a SparkSession, the instance is saved as a class attribute and returned from subsequent calls to SQLContext.getOrCreate(). If the SparkContext is stopped and a new one started, the SQLContext class attribute is never cleared so any code which calls SQLContext.getOrCreate() will get a SQLContext with a reference to the old, unusable SparkContext.
A similar issue was identified and fixed for SparkSession in [SPARK-19055](https://issues.apache.org/jira/browse/SPARK-19055), but the fix did not change SQLContext as well. I ran into this because mllib still [uses](https://github.com/apache/spark/blob/master/python/pyspark/mllib/common.py#L105) SQLContext.getOrCreate() under the hood.
### Does this PR introduce any user-facing change?
No
### How was this patch tested?
A new test was added. I verified that the test fails without the included change.
Closes#27610 from afavaro/restart-sqlcontext.
Authored-by: Alex Favaro <alex.favaro@affirm.com>
Signed-off-by: HyukjinKwon <gurwls223@apache.org>
### What changes were proposed in this pull request?
The `allGather` method is added to the `BarrierTaskContext`. This method contains the same functionality as the `BarrierTaskContext.barrier` method; it blocks the task until all tasks make the call, at which time they may continue execution. In addition, the `allGather` method takes an input message. Upon returning from the `allGather` the task receives a list of all the messages sent by all the tasks that made the `allGather` call.
### Why are the changes needed?
There are many situations where having the tasks communicate in a synchronized way is useful. One simple example is if each task needs to start a server to serve requests from one another; first the tasks must find a free port (the result of which is undetermined beforehand) and then start making requests, but to do so they each must know the port chosen by the other task. An `allGather` method would allow them to inform each other of the port they will run on.
### Does this PR introduce any user-facing change?
Yes, an `BarrierTaskContext.allGather` method will be available through the Scala, Java, and Python APIs.
### How was this patch tested?
Most of the code path is already covered by tests to the `barrier` method, since this PR includes a refactor so that much code is shared by the `barrier` and `allGather` methods. However, a test is added to assert that an all gather on each tasks partition ID will return a list of every partition ID.
An example through the Python API:
```python
>>> from pyspark import BarrierTaskContext
>>>
>>> def f(iterator):
... context = BarrierTaskContext.get()
... return [context.allGather('{}'.format(context.partitionId()))]
...
>>> sc.parallelize(range(4), 4).barrier().mapPartitions(f).collect()[0]
[u'3', u'1', u'0', u'2']
```
Closes#27395 from sarthfrey/master.
Lead-authored-by: sarthfrey-db <sarth.frey@databricks.com>
Co-authored-by: sarthfrey <sarth.frey@gmail.com>
Signed-off-by: Xiangrui Meng <meng@databricks.com>
(cherry picked from commit 57254c9719)
Signed-off-by: Xiangrui Meng <meng@databricks.com>
### What changes were proposed in this pull request?
This PR proposes to deprecate the APIs at `SQLContext` removed in SPARK-25908. We should remove equivalent APIs; however, seems we missed to deprecate.
While I am here, I fix one more issue. After SPARK-25908, `sc._jvm.SQLContext.getOrCreate` dose not exist anymore. So,
```python
from pyspark.sql import SQLContext
from pyspark import SparkContext
sc = SparkContext.getOrCreate()
SQLContext.getOrCreate(sc).range(10).show()
```
throws an exception as below:
```
Traceback (most recent call last):
File "<stdin>", line 1, in <module>
File "/.../spark/python/pyspark/sql/context.py", line 110, in getOrCreate
jsqlContext = sc._jvm.SQLContext.getOrCreate(sc._jsc.sc())
File "/.../spark/python/lib/py4j-0.10.8.1-src.zip/py4j/java_gateway.py", line 1516, in __getattr__
py4j.protocol.Py4JError: org.apache.spark.sql.SQLContext.getOrCreate does not exist in the JVM
```
After this PR:
```
/.../spark/python/pyspark/sql/context.py:113: DeprecationWarning: Deprecated in 3.0.0. Use SparkSession.builder.getOrCreate() instead.
DeprecationWarning)
+---+
| id|
+---+
| 0|
| 1|
| 2|
| 3|
| 4|
| 5|
| 6|
| 7|
| 8|
| 9|
+---+
```
In case of the constructor of `SQLContext`, after this PR:
```python
from pyspark.sql import SQLContext
sc = SparkContext.getOrCreate()
SQLContext(sc)
```
```
/.../spark/python/pyspark/sql/context.py:77: DeprecationWarning: Deprecated in 3.0.0. Use SparkSession.builder.getOrCreate() instead.
DeprecationWarning)
```
### Why are the changes needed?
To promote to use SparkSession, and keep the API party consistent with Scala side.
### Does this PR introduce any user-facing change?
Yes, it will show deprecation warning to users.
### How was this patch tested?
Manually tested as described above. Unittests were also added.
Closes#27614 from HyukjinKwon/SPARK-30861.
Authored-by: HyukjinKwon <gurwls223@apache.org>
Signed-off-by: HyukjinKwon <gurwls223@apache.org>