## What changes were proposed in this pull request?
`pyspark.sql.tests.SQLTests2` doesn't stop newly created spark context in the test and it might affect the following tests.
This pr makes `pyspark.sql.tests.SQLTests2` stop `SparkContext`.
## How was this patch tested?
Existing tests.
Author: Takuya UESHIN <ueshin@databricks.com>
Closes#19158 from ueshin/issues/SPARK-21950.
## Problem Description
When pyspark is listed as a dependency of another package, installing
the other package will cause an install failure in pyspark. When the
other package is being installed, pyspark's setup_requires requirements
are installed including pypandoc. Thus, the exception handling on
setup.py:152 does not work because the pypandoc module is indeed
available. However, the pypandoc.convert() function fails if pandoc
itself is not installed (in our use cases it is not). This raises an
OSError that is not handled, and setup fails.
The following is a sample failure:
```
$ which pandoc
$ pip freeze | grep pypandoc
pypandoc==1.4
$ pip install pyspark
Collecting pyspark
Downloading pyspark-2.2.0.post0.tar.gz (188.3MB)
100% |████████████████████████████████| 188.3MB 16.8MB/s
Complete output from command python setup.py egg_info:
Maybe try:
sudo apt-get install pandoc
See http://johnmacfarlane.net/pandoc/installing.html
for installation options
---------------------------------------------------------------
Traceback (most recent call last):
File "<string>", line 1, in <module>
File "/tmp/pip-build-mfnizcwa/pyspark/setup.py", line 151, in <module>
long_description = pypandoc.convert('README.md', 'rst')
File "/home/tbeck/.virtualenvs/cem/lib/python3.5/site-packages/pypandoc/__init__.py", line 69, in convert
outputfile=outputfile, filters=filters)
File "/home/tbeck/.virtualenvs/cem/lib/python3.5/site-packages/pypandoc/__init__.py", line 260, in _convert_input
_ensure_pandoc_path()
File "/home/tbeck/.virtualenvs/cem/lib/python3.5/site-packages/pypandoc/__init__.py", line 544, in _ensure_pandoc_path
raise OSError("No pandoc was found: either install pandoc and add it\n"
OSError: No pandoc was found: either install pandoc and add it
to your PATH or or call pypandoc.download_pandoc(...) or
install pypandoc wheels with included pandoc.
----------------------------------------
Command "python setup.py egg_info" failed with error code 1 in /tmp/pip-build-mfnizcwa/pyspark/
```
## What changes were proposed in this pull request?
This change simply adds an additional exception handler for the OSError
that is raised. This allows pyspark to be installed client-side without requiring pandoc to be installed.
## How was this patch tested?
I tested this by building a wheel package of pyspark with the change applied. Then, in a clean virtual environment with pypandoc installed but pandoc not available on the system, I installed pyspark from the wheel.
Here is the output
```
$ pip freeze | grep pypandoc
pypandoc==1.4
$ which pandoc
$ pip install --no-cache-dir ../spark/python/dist/pyspark-2.3.0.dev0-py2.py3-none-any.whl
Processing /home/tbeck/work/spark/python/dist/pyspark-2.3.0.dev0-py2.py3-none-any.whl
Requirement already satisfied: py4j==0.10.6 in /home/tbeck/.virtualenvs/cem/lib/python3.5/site-packages (from pyspark==2.3.0.dev0)
Installing collected packages: pyspark
Successfully installed pyspark-2.3.0.dev0
```
Author: Tucker Beck <tucker.beck@rentrakmail.com>
Closes#18981 from dusktreader/dusktreader/fix-pandoc-dependency-issue-in-setup_py.
## What changes were proposed in this pull request?
This PR proposes to add a wrapper for `unionByName` API to R and Python as well.
**Python**
```python
df1 = spark.createDataFrame([[1, 2, 3]], ["col0", "col1", "col2"])
df2 = spark.createDataFrame([[4, 5, 6]], ["col1", "col2", "col0"])
df1.unionByName(df2).show()
```
```
+----+----+----+
|col0|col1|col3|
+----+----+----+
| 1| 2| 3|
| 6| 4| 5|
+----+----+----+
```
**R**
```R
df1 <- select(createDataFrame(mtcars), "carb", "am", "gear")
df2 <- select(createDataFrame(mtcars), "am", "gear", "carb")
head(unionByName(limit(df1, 2), limit(df2, 2)))
```
```
carb am gear
1 4 1 4
2 4 1 4
3 4 1 4
4 4 1 4
```
## How was this patch tested?
Doctests for Python and unit test added in `test_sparkSQL.R` for R.
Author: hyukjinkwon <gurwls223@gmail.com>
Closes#19105 from HyukjinKwon/unionByName-r-python.
…build; fix some things that will be warnings or errors in 2.12; restore Scala 2.12 profile infrastructure
## What changes were proposed in this pull request?
This change adds back the infrastructure for a Scala 2.12 build, but does not enable it in the release or Python test scripts.
In order to make that meaningful, it also resolves compile errors that the code hits in 2.12 only, in a way that still works with 2.11.
It also updates dependencies to the earliest minor release of dependencies whose current version does not yet support Scala 2.12. This is in a sense covered by other JIRAs under the main umbrella, but implemented here. The versions below still work with 2.11, and are the _latest_ maintenance release in the _earliest_ viable minor release.
- Scalatest 2.x -> 3.0.3
- Chill 0.8.0 -> 0.8.4
- Clapper 1.0.x -> 1.1.2
- json4s 3.2.x -> 3.4.2
- Jackson 2.6.x -> 2.7.9 (required by json4s)
This change does _not_ fully enable a Scala 2.12 build:
- It will also require dropping support for Kafka before 0.10. Easy enough, just didn't do it yet here
- It will require recreating `SparkILoop` and `Main` for REPL 2.12, which is SPARK-14650. Possible to do here too.
What it does do is make changes that resolve much of the remaining gap without affecting the current 2.11 build.
## How was this patch tested?
Existing tests and build. Manually tested with `./dev/change-scala-version.sh 2.12` to verify it compiles, modulo the exceptions above.
Author: Sean Owen <sowen@cloudera.com>
Closes#18645 from srowen/SPARK-14280.
## What changes were proposed in this pull request?
This PR proposes to remove private functions that look not used in the main codes, `_split_schema_abstract`, `_parse_field_abstract`, `_parse_schema_abstract` and `_infer_schema_type`.
## How was this patch tested?
Existing tests.
Author: hyukjinkwon <gurwls223@gmail.com>
Closes#18647 from HyukjinKwon/remove-abstract.
## What changes were proposed in this pull request?
This PR make `DataFrame.sample(...)` can omit `withReplacement` defaulting `False`, consistently with equivalent Scala / Java API.
In short, the following examples are allowed:
```python
>>> df = spark.range(10)
>>> df.sample(0.5).count()
7
>>> df.sample(fraction=0.5).count()
3
>>> df.sample(0.5, seed=42).count()
5
>>> df.sample(fraction=0.5, seed=42).count()
5
```
In addition, this PR also adds some type checking logics as below:
```python
>>> df = spark.range(10)
>>> df.sample().count()
...
TypeError: withReplacement (optional), fraction (required) and seed (optional) should be a bool, float and number; however, got [].
>>> df.sample(True).count()
...
TypeError: withReplacement (optional), fraction (required) and seed (optional) should be a bool, float and number; however, got [<type 'bool'>].
>>> df.sample(42).count()
...
TypeError: withReplacement (optional), fraction (required) and seed (optional) should be a bool, float and number; however, got [<type 'int'>].
>>> df.sample(fraction=False, seed="a").count()
...
TypeError: withReplacement (optional), fraction (required) and seed (optional) should be a bool, float and number; however, got [<type 'bool'>, <type 'str'>].
>>> df.sample(seed=[1]).count()
...
TypeError: withReplacement (optional), fraction (required) and seed (optional) should be a bool, float and number; however, got [<type 'list'>].
>>> df.sample(withReplacement="a", fraction=0.5, seed=1)
...
TypeError: withReplacement (optional), fraction (required) and seed (optional) should be a bool, float and number; however, got [<type 'str'>, <type 'float'>, <type 'int'>].
```
## How was this patch tested?
Manually tested, unit tests added in doc tests and manually checked the built documentation for Python.
Author: hyukjinkwon <gurwls223@gmail.com>
Closes#18999 from HyukjinKwon/SPARK-21779.
## What changes were proposed in this pull request?
`PickleException` is thrown when creating dataframe from python row with empty bytearray
spark.createDataFrame(spark.sql("select unhex('') as xx").rdd.map(lambda x: {"abc": x.xx})).show()
net.razorvine.pickle.PickleException: invalid pickle data for bytearray; expected 1 or 2 args, got 0
at net.razorvine.pickle.objects.ByteArrayConstructor.construct(ByteArrayConstructor.java
...
`ByteArrayConstructor` doesn't deal with empty byte array pickled by Python3.
## How was this patch tested?
Added test.
Author: Liang-Chi Hsieh <viirya@gmail.com>
Closes#19085 from viirya/SPARK-21534.
## What changes were proposed in this pull request?
This PR aims to support `spark.sql.orc.compression.codec` like Parquet's `spark.sql.parquet.compression.codec`. Users can use SQLConf to control ORC compression, too.
## How was this patch tested?
Pass the Jenkins with new and updated test cases.
Author: Dongjoon Hyun <dongjoon@apache.org>
Closes#19055 from dongjoon-hyun/SPARK-21839.
## What changes were proposed in this pull request?
This patch adds allowUnquotedControlChars option in JSON data source to allow JSON Strings to contain unquoted control characters (ASCII characters with value less than 32, including tab and line feed characters)
## How was this patch tested?
Add new test cases
Author: vinodkc <vinod.kc.in@gmail.com>
Closes#19008 from vinodkc/br_fix_SPARK-21756.
## What changes were proposed in this pull request?
While preparing to take over https://github.com/apache/spark/pull/16537, I realised a (I think) better approach to make the exception handling in one point.
This PR proposes to fix `_to_java_column` in `pyspark.sql.column`, which most of functions in `functions.py` and some other APIs use. This `_to_java_column` basically looks not working with other types than `pyspark.sql.column.Column` or string (`str` and `unicode`).
If this is not `Column`, then it calls `_create_column_from_name` which calls `functions.col` within JVM:
42b9eda80e/sql/core/src/main/scala/org/apache/spark/sql/functions.scala (L76)
And it looks we only have `String` one with `col`.
So, these should work:
```python
>>> from pyspark.sql.column import _to_java_column, Column
>>> _to_java_column("a")
JavaObject id=o28
>>> _to_java_column(u"a")
JavaObject id=o29
>>> _to_java_column(spark.range(1).id)
JavaObject id=o33
```
whereas these do not:
```python
>>> _to_java_column(1)
```
```
...
py4j.protocol.Py4JError: An error occurred while calling z:org.apache.spark.sql.functions.col. Trace:
py4j.Py4JException: Method col([class java.lang.Integer]) does not exist
...
```
```python
>>> _to_java_column([])
```
```
...
py4j.protocol.Py4JError: An error occurred while calling z:org.apache.spark.sql.functions.col. Trace:
py4j.Py4JException: Method col([class java.util.ArrayList]) does not exist
...
```
```python
>>> class A(): pass
>>> _to_java_column(A())
```
```
...
AttributeError: 'A' object has no attribute '_get_object_id'
```
Meaning most of functions using `_to_java_column` such as `udf` or `to_json` or some other APIs throw an exception as below:
```python
>>> from pyspark.sql.functions import udf
>>> udf(lambda x: x)(None)
```
```
...
py4j.protocol.Py4JJavaError: An error occurred while calling z:org.apache.spark.sql.functions.col.
: java.lang.NullPointerException
...
```
```python
>>> from pyspark.sql.functions import to_json
>>> to_json(None)
```
```
...
py4j.protocol.Py4JJavaError: An error occurred while calling z:org.apache.spark.sql.functions.col.
: java.lang.NullPointerException
...
```
**After this PR**:
```python
>>> from pyspark.sql.functions import udf
>>> udf(lambda x: x)(None)
...
```
```
TypeError: Invalid argument, not a string or column: None of type <type 'NoneType'>. For column literals, use 'lit', 'array', 'struct' or 'create_map' functions.
```
```python
>>> from pyspark.sql.functions import to_json
>>> to_json(None)
```
```
...
TypeError: Invalid argument, not a string or column: None of type <type 'NoneType'>. For column literals, use 'lit', 'array', 'struct' or 'create_map' functions.
```
## How was this patch tested?
Unit tests added in `python/pyspark/sql/tests.py` and manual tests.
Author: hyukjinkwon <gurwls223@gmail.com>
Author: zero323 <zero323@users.noreply.github.com>
Closes#19027 from HyukjinKwon/SPARK-19165.
## What changes were proposed in this pull request?
Modify MLP model to inherit `ProbabilisticClassificationModel` and so that it can expose the probability column when transforming data.
## How was this patch tested?
Test added.
Author: WeichenXu <WeichenXu123@outlook.com>
Closes#17373 from WeichenXu123/expose_probability_in_mlp_model.
## What changes were proposed in this pull request?
Added call to copy values of Params from Estimator to Model after fit in PySpark ML. This will copy values for any params that are also defined in the Model. Since currently most Models do not define the same params from the Estimator, also added method to create new Params from looking at the Java object if they do not exist in the Python object. This is a temporary fix that can be removed once the PySpark models properly define the params themselves.
## How was this patch tested?
Refactored the `check_params` test to optionally check if the model params for Python and Java match and added this check to an existing fitted model that shares params between Estimator and Model.
Author: Bryan Cutler <cutlerb@gmail.com>
Closes#17849 from BryanCutler/pyspark-models-own-params-SPARK-10931.
## What changes were proposed in this pull request?
Based on https://github.com/apache/spark/pull/18282 by rgbkrk this PR attempts to update to the current released cloudpickle and minimize the difference between Spark cloudpickle and "stock" cloud pickle with the goal of eventually using the stock cloud pickle.
Some notable changes:
* Import submodules accessed by pickled functions (cloudpipe/cloudpickle#80)
* Support recursive functions inside closures (cloudpipe/cloudpickle#89, cloudpipe/cloudpickle#90)
* Fix ResourceWarnings and DeprecationWarnings (cloudpipe/cloudpickle#88)
* Assume modules with __file__ attribute are not dynamic (cloudpipe/cloudpickle#85)
* Make cloudpickle Python 3.6 compatible (cloudpipe/cloudpickle#72)
* Allow pickling of builtin methods (cloudpipe/cloudpickle#57)
* Add ability to pickle dynamically created modules (cloudpipe/cloudpickle#52)
* Support method descriptor (cloudpipe/cloudpickle#46)
* No more pickling of closed files, was broken on Python 3 (cloudpipe/cloudpickle#32)
* ** Remove non-standard __transient__check (cloudpipe/cloudpickle#110)** -- while we don't use this internally, and have no tests or documentation for its use, downstream code may use __transient__, although it has never been part of the API, if we merge this we should include a note about this in the release notes.
* Support for pickling loggers (yay!) (cloudpipe/cloudpickle#96)
* BUG: Fix crash when pickling dynamic class cycles. (cloudpipe/cloudpickle#102)
## How was this patch tested?
Existing PySpark unit tests + the unit tests from the cloudpickle project on their own.
Author: Holden Karau <holden@us.ibm.com>
Author: Kyle Kelley <rgbkrk@gmail.com>
Closes#18734 from holdenk/holden-rgbkrk-cloudpickle-upgrades.
Add Python API for `FeatureHasher` transformer.
## How was this patch tested?
New doc test.
Author: Nick Pentreath <nickp@za.ibm.com>
Closes#18970 from MLnick/SPARK-21468-pyspark-hasher.
## What changes were proposed in this pull request?
Adds the recently added `summary` method to the python dataframe interface.
## How was this patch tested?
Additional inline doctests.
Author: Andrew Ray <ray.andrew@gmail.com>
Closes#18762 from aray/summary-py.
Proposed changes:
* Clarify the type error that `Column.substr()` gives.
Test plan:
* Tested this manually.
* Test code:
```python
from pyspark.sql.functions import col, lit
spark.createDataFrame([['nick']], schema=['name']).select(col('name').substr(0, lit(1)))
```
* Before:
```
TypeError: Can not mix the type
```
* After:
```
TypeError: startPos and length must be the same type. Got <class 'int'> and
<class 'pyspark.sql.column.Column'>, respectively.
```
Author: Nicholas Chammas <nicholas.chammas@gmail.com>
Closes#18926 from nchammas/SPARK-21712-substr-type-error.
## What changes were proposed in this pull request?
JIRA issue: https://issues.apache.org/jira/browse/SPARK-21658
Add default None for value in `na.replace` since `Dataframe.replace` and `DataframeNaFunctions.replace` are alias.
The default values are the same now.
```
>>> df = sqlContext.createDataFrame([('Alice', 10, 80.0)])
>>> df.replace({"Alice": "a"}).first()
Row(_1=u'a', _2=10, _3=80.0)
>>> df.na.replace({"Alice": "a"}).first()
Row(_1=u'a', _2=10, _3=80.0)
```
## How was this patch tested?
Existing tests.
cc viirya
Author: byakuinss <grace.chinhanyu@gmail.com>
Closes#18895 from byakuinss/SPARK-21658.
## What changes were proposed in this pull request?
Implemented a Python-only persistence framework for pipelines containing stages that cannot be saved using Java.
## How was this patch tested?
Created a custom Python-only UnaryTransformer, included it in a Pipeline, and saved/loaded the pipeline. The loaded pipeline was compared against the original using _compare_pipelines() in tests.py.
Author: Ajay Saini <ajays725@gmail.com>
Closes#18888 from ajaysaini725/PythonPipelines.
## What changes were proposed in this pull request?
Currently `df.na.replace("*", Map[String, String]("NULL" -> null))` will produce exception.
This PR enables passing null/None as value in the replacement map in DataFrame.replace().
Note that the replacement map keys and values should still be the same type, while the values can have a mix of null/None and that type.
This PR enables following operations for example:
`df.na.replace("*", Map[String, String]("NULL" -> null))`(scala)
`df.na.replace("*", Map[Any, Any](60 -> null, 70 -> 80))`(scala)
`df.na.replace('Alice', None)`(python)
`df.na.replace([10, 20])`(python, replacing with None is by default)
One use case could be: I want to replace all the empty strings with null/None because they were incorrectly generated and then drop all null/None data
`df.na.replace("*", Map("" -> null)).na.drop()`(scala)
`df.replace(u'', None).dropna()`(python)
## How was this patch tested?
Scala unit test.
Python doctest and unit test.
Author: bravo-zhang <mzhang1230@gmail.com>
Closes#18820 from bravo-zhang/spark-14932.
## What changes were proposed in this pull request?
This modification increases the timeout for `serveIterator` (which is not dynamically configurable). This fixes timeout issues in pyspark when using `collect` and similar functions, in cases where Python may take more than a couple seconds to connect.
See https://issues.apache.org/jira/browse/SPARK-21551
## How was this patch tested?
Ran the tests.
cc rxin
Author: peay <peay@protonmail.com>
Closes#18752 from peay/spark-21551.
## What changes were proposed in this pull request?
Update breeze to 0.13.1 for an emergency bugfix in strong wolfe line search
https://github.com/scalanlp/breeze/pull/651
## How was this patch tested?
N/A
Author: WeichenXu <WeichenXu123@outlook.com>
Closes#18797 from WeichenXu123/update-breeze.
## What changes were proposed in this pull request?
PySpark GLR ```model.summary``` should return a printable representation by calling Scala ```toString```.
## How was this patch tested?
```
from pyspark.ml.regression import GeneralizedLinearRegression
dataset = spark.read.format("libsvm").load("data/mllib/sample_linear_regression_data.txt")
glr = GeneralizedLinearRegression(family="gaussian", link="identity", maxIter=10, regParam=0.3)
model = glr.fit(dataset)
model.summary
```
Before this PR:

After this PR:
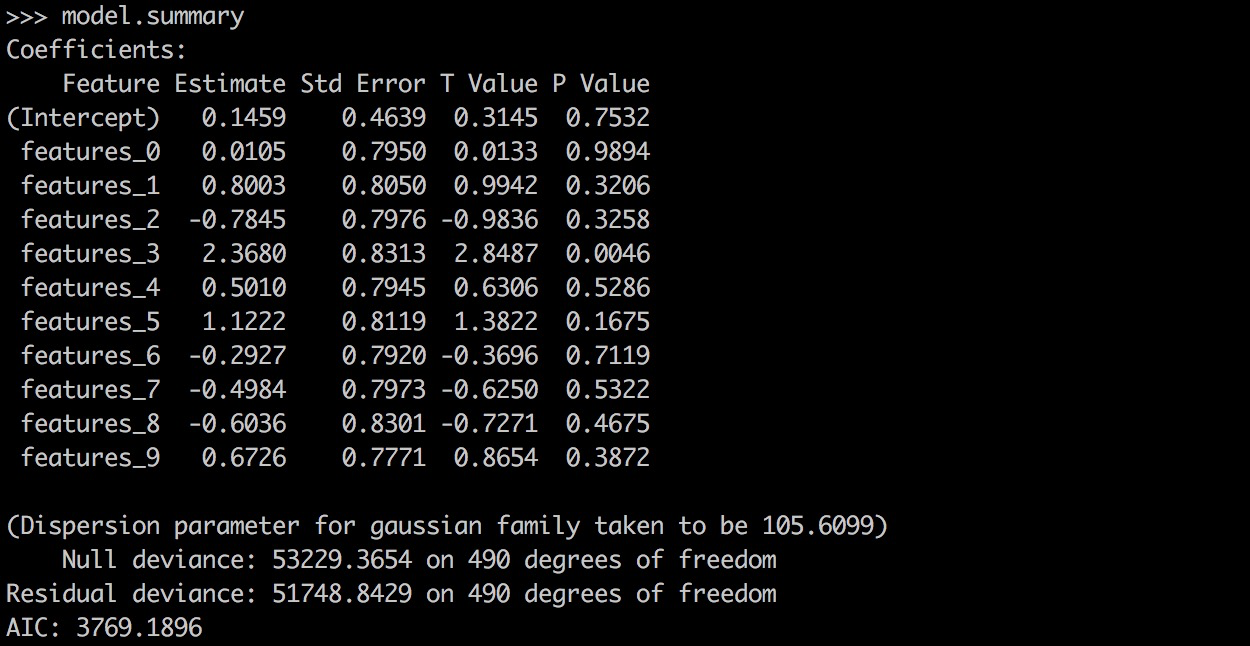
Author: Yanbo Liang <ybliang8@gmail.com>
Closes#18870 from yanboliang/spark-19270.
## What changes were proposed in this pull request?
Added DefaultParamsWriteable, DefaultParamsReadable, DefaultParamsWriter, and DefaultParamsReader to Python to support Python-only persistence of Json-serializable parameters.
## How was this patch tested?
Instantiated an estimator with Json-serializable parameters (ex. LogisticRegression), saved it using the added helper functions, and loaded it back, and compared it to the original instance to make sure it is the same. This test was both done in the Python REPL and implemented in the unit tests.
Note to reviewers: there are a few excess comments that I left in the code for clarity but will remove before the code is merged to master.
Author: Ajay Saini <ajays725@gmail.com>
Closes#18742 from ajaysaini725/PythonPersistenceHelperFunctions.
## What changes were proposed in this pull request?
Enhanced some existing documentation
Please review http://spark.apache.org/contributing.html before opening a pull request.
Author: Mac <maclockard@gmail.com>
Closes#18710 from maclockard/maclockard-patch-1.
## What changes were proposed in this pull request?
Implemented UnaryTransformer in Python.
## How was this patch tested?
This patch was tested by creating a MockUnaryTransformer class in the unit tests that extends UnaryTransformer and testing that the transform function produced correct output.
Author: Ajay Saini <ajays725@gmail.com>
Closes#18746 from ajaysaini725/AddPythonUnaryTransformer.
## What changes were proposed in this pull request?
Python API for Constrained Logistic Regression based on #17922 , thanks for the original contribution from zero323 .
## How was this patch tested?
Unit tests.
Author: zero323 <zero323@users.noreply.github.com>
Author: Yanbo Liang <ybliang8@gmail.com>
Closes#18759 from yanboliang/SPARK-20601.
## What changes were proposed in this pull request?
When using PySpark broadcast variables in a multi-threaded environment, `SparkContext._pickled_broadcast_vars` becomes a shared resource. A race condition can occur when broadcast variables that are pickled from one thread get added to the shared ` _pickled_broadcast_vars` and become part of the python command from another thread. This PR introduces a thread-safe pickled registry using thread local storage so that when python command is pickled (causing the broadcast variable to be pickled and added to the registry) each thread will have their own view of the pickle registry to retrieve and clear the broadcast variables used.
## How was this patch tested?
Added a unit test that causes this race condition using another thread.
Author: Bryan Cutler <cutlerb@gmail.com>
Closes#18695 from BryanCutler/pyspark-bcast-threadsafe-SPARK-12717.
## What changes were proposed in this pull request?
GBTs inherit from HasStepSize & LInearSVC/Binarizer from HasThreshold
## How was this patch tested?
existing tests
Author: Zheng RuiFeng <ruifengz@foxmail.com>
Author: Ruifeng Zheng <ruifengz@foxmail.com>
Closes#18612 from zhengruifeng/override_HasXXX.
## What changes were proposed in this pull request?
This PR proposes `StructType.fieldNames` that returns a copy of a field name list rather than a (undocumented) `StructType.names`.
There are two points here:
- API consistency with Scala/Java
- Provide a safe way to get the field names. Manipulating these might cause unexpected behaviour as below:
```python
from pyspark.sql.types import *
struct = StructType([StructField("f1", StringType(), True)])
names = struct.names
del names[0]
spark.createDataFrame([{"f1": 1}], struct).show()
```
```
...
java.lang.IllegalStateException: Input row doesn't have expected number of values required by the schema. 1 fields are required while 0 values are provided.
at org.apache.spark.sql.execution.python.EvaluatePython$.fromJava(EvaluatePython.scala:138)
at org.apache.spark.sql.SparkSession$$anonfun$6.apply(SparkSession.scala:741)
at org.apache.spark.sql.SparkSession$$anonfun$6.apply(SparkSession.scala:741)
...
```
## How was this patch tested?
Added tests in `python/pyspark/sql/tests.py`.
Author: hyukjinkwon <gurwls223@gmail.com>
Closes#18618 from HyukjinKwon/SPARK-20090.
## What changes were proposed in this pull request?
add `setWeightCol` method for OneVsRest.
`weightCol` is ignored if classifier doesn't inherit HasWeightCol trait.
## How was this patch tested?
+ [x] add an unit test.
Author: Yan Facai (颜发才) <facai.yan@gmail.com>
Closes#18554 from facaiy/BUG/oneVsRest_missing_weightCol.
## What changes were proposed in this pull request?
This is a refactoring of `ArrowConverters` and related classes.
1. Refactor `ColumnWriter` as `ArrowWriter`.
2. Add `ArrayType` and `StructType` support.
3. Refactor `ArrowConverters` to skip intermediate `ArrowRecordBatch` creation.
## How was this patch tested?
Added some tests and existing tests.
Author: Takuya UESHIN <ueshin@databricks.com>
Closes#18655 from ueshin/issues/SPARK-21440.
### What changes were proposed in this pull request?
Like [Hive UDFType](https://hive.apache.org/javadocs/r2.0.1/api/org/apache/hadoop/hive/ql/udf/UDFType.html), we should allow users to add the extra flags for ScalaUDF and JavaUDF too. _stateful_/_impliesOrder_ are not applicable to our Scala UDF. Thus, we only add the following two flags.
- deterministic: Certain optimizations should not be applied if UDF is not deterministic. Deterministic UDF returns same result each time it is invoked with a particular input. This determinism just needs to hold within the context of a query.
When the deterministic flag is not correctly set, the results could be wrong.
For ScalaUDF in Dataset APIs, users can call the following extra APIs for `UserDefinedFunction` to make the corresponding changes.
- `nonDeterministic`: Updates UserDefinedFunction to non-deterministic.
Also fixed the Java UDF name loss issue.
Will submit a separate PR for `distinctLike` for UDAF
### How was this patch tested?
Added test cases for both ScalaUDF
Author: gatorsmile <gatorsmile@gmail.com>
Author: Wenchen Fan <cloud0fan@gmail.com>
Closes#17848 from gatorsmile/udfRegister.
## What changes were proposed in this pull request?
After SPARK-12661, I guess we officially dropped Python 2.6 support. It looks there are few places missing this notes.
I grepped "Python 2.6" and "python 2.6" and the results were below:
```
./core/src/main/scala/org/apache/spark/api/python/SerDeUtil.scala: // Unpickle array.array generated by Python 2.6
./docs/index.md:Note that support for Java 7, Python 2.6 and old Hadoop versions before 2.6.5 were removed as of Spark 2.2.0.
./docs/rdd-programming-guide.md:Spark {{site.SPARK_VERSION}} works with Python 2.6+ or Python 3.4+. It can use the standard CPython interpreter,
./docs/rdd-programming-guide.md:Note that support for Python 2.6 is deprecated as of Spark 2.0.0, and may be removed in Spark 2.2.0.
./python/pyspark/context.py: warnings.warn("Support for Python 2.6 is deprecated as of Spark 2.0.0")
./python/pyspark/ml/tests.py: sys.stderr.write('Please install unittest2 to test with Python 2.6 or earlier')
./python/pyspark/mllib/tests.py: sys.stderr.write('Please install unittest2 to test with Python 2.6 or earlier')
./python/pyspark/serializers.py: # On Python 2.6, we can't write bytearrays to streams, so we need to convert them
./python/pyspark/sql/tests.py: sys.stderr.write('Please install unittest2 to test with Python 2.6 or earlier')
./python/pyspark/streaming/tests.py: sys.stderr.write('Please install unittest2 to test with Python 2.6 or earlier')
./python/pyspark/tests.py: sys.stderr.write('Please install unittest2 to test with Python 2.6 or earlier')
./python/pyspark/tests.py: # NOTE: dict is used instead of collections.Counter for Python 2.6
./python/pyspark/tests.py: # NOTE: dict is used instead of collections.Counter for Python 2.6
```
This PR only proposes to change visible changes as below:
```
./docs/rdd-programming-guide.md:Spark {{site.SPARK_VERSION}} works with Python 2.6+ or Python 3.4+. It can use the standard CPython interpreter,
./docs/rdd-programming-guide.md:Note that support for Python 2.6 is deprecated as of Spark 2.0.0, and may be removed in Spark 2.2.0.
./python/pyspark/context.py: warnings.warn("Support for Python 2.6 is deprecated as of Spark 2.0.0")
```
This one is already correct:
```
./docs/index.md:Note that support for Java 7, Python 2.6 and old Hadoop versions before 2.6.5 were removed as of Spark 2.2.0.
```
## How was this patch tested?
```bash
grep -r "Python 2.6" .
grep -r "python 2.6" .
```
Author: hyukjinkwon <gurwls223@gmail.com>
Closes#18682 from HyukjinKwon/minor-python.26.
## What changes were proposed in this pull request?
This is the reopen of https://github.com/apache/spark/pull/14198, with merge conflicts resolved.
ueshin Could you please take a look at my code?
Fix bugs about types that result an array of null when creating DataFrame using python.
Python's array.array have richer type than python itself, e.g. we can have `array('f',[1,2,3])` and `array('d',[1,2,3])`. Codes in spark-sql and pyspark didn't take this into consideration which might cause a problem that you get an array of null values when you have `array('f')` in your rows.
A simple code to reproduce this bug is:
```
from pyspark import SparkContext
from pyspark.sql import SQLContext,Row,DataFrame
from array import array
sc = SparkContext()
sqlContext = SQLContext(sc)
row1 = Row(floatarray=array('f',[1,2,3]), doublearray=array('d',[1,2,3]))
rows = sc.parallelize([ row1 ])
df = sqlContext.createDataFrame(rows)
df.show()
```
which have output
```
+---------------+------------------+
| doublearray| floatarray|
+---------------+------------------+
|[1.0, 2.0, 3.0]|[null, null, null]|
+---------------+------------------+
```
## How was this patch tested?
New test case added
Author: Xiang Gao <qasdfgtyuiop@gmail.com>
Author: Gao, Xiang <qasdfgtyuiop@gmail.com>
Author: Takuya UESHIN <ueshin@databricks.com>
Closes#18444 from zasdfgbnm/fix_array_infer.
## What changes were proposed in this pull request?
Added functionality for CrossValidator and TrainValidationSplit to persist nested estimators such as OneVsRest. Also added CrossValidator and TrainValidation split persistence to pyspark.
## How was this patch tested?
Performed both cross validation and train validation split with a one vs. rest estimator and tested read/write functionality of the estimator parameter maps required by these meta-algorithms.
Author: Ajay Saini <ajays725@gmail.com>
Closes#18428 from ajaysaini725/MetaAlgorithmPersistNestedEstimators.
## What changes were proposed in this pull request?
This PR proposes to avoid `__name__` in the tuple naming the attributes assigned directly from the wrapped function to the wrapper function, and use `self._name` (`func.__name__` or `obj.__class__.name__`).
After SPARK-19161, we happened to break callable objects as UDFs in Python as below:
```python
from pyspark.sql import functions
class F(object):
def __call__(self, x):
return x
foo = F()
udf = functions.udf(foo)
```
```
Traceback (most recent call last):
File "<stdin>", line 1, in <module>
File ".../spark/python/pyspark/sql/functions.py", line 2142, in udf
return _udf(f=f, returnType=returnType)
File ".../spark/python/pyspark/sql/functions.py", line 2133, in _udf
return udf_obj._wrapped()
File ".../spark/python/pyspark/sql/functions.py", line 2090, in _wrapped
functools.wraps(self.func)
File "/System/Library/Frameworks/Python.framework/Versions/2.7/lib/python2.7/functools.py", line 33, in update_wrapper
setattr(wrapper, attr, getattr(wrapped, attr))
AttributeError: F instance has no attribute '__name__'
```
This worked in Spark 2.1:
```python
from pyspark.sql import functions
class F(object):
def __call__(self, x):
return x
foo = F()
udf = functions.udf(foo)
spark.range(1).select(udf("id")).show()
```
```
+-----+
|F(id)|
+-----+
| 0|
+-----+
```
**After**
```python
from pyspark.sql import functions
class F(object):
def __call__(self, x):
return x
foo = F()
udf = functions.udf(foo)
spark.range(1).select(udf("id")).show()
```
```
+-----+
|F(id)|
+-----+
| 0|
+-----+
```
_In addition, we also happened to break partial functions as below_:
```python
from pyspark.sql import functions
from functools import partial
partial_func = partial(lambda x: x, x=1)
udf = functions.udf(partial_func)
```
```
Traceback (most recent call last):
File "<stdin>", line 1, in <module>
File ".../spark/python/pyspark/sql/functions.py", line 2154, in udf
return _udf(f=f, returnType=returnType)
File ".../spark/python/pyspark/sql/functions.py", line 2145, in _udf
return udf_obj._wrapped()
File ".../spark/python/pyspark/sql/functions.py", line 2099, in _wrapped
functools.wraps(self.func, assigned=assignments)
File "/System/Library/Frameworks/Python.framework/Versions/2.7/lib/python2.7/functools.py", line 33, in update_wrapper
setattr(wrapper, attr, getattr(wrapped, attr))
AttributeError: 'functools.partial' object has no attribute '__module__'
```
This worked in Spark 2.1:
```python
from pyspark.sql import functions
from functools import partial
partial_func = partial(lambda x: x, x=1)
udf = functions.udf(partial_func)
spark.range(1).select(udf()).show()
```
```
+---------+
|partial()|
+---------+
| 1|
+---------+
```
**After**
```python
from pyspark.sql import functions
from functools import partial
partial_func = partial(lambda x: x, x=1)
udf = functions.udf(partial_func)
spark.range(1).select(udf()).show()
```
```
+---------+
|partial()|
+---------+
| 1|
+---------+
```
## How was this patch tested?
Unit tests in `python/pyspark/sql/tests.py` and manual tests.
Author: hyukjinkwon <gurwls223@gmail.com>
Closes#18615 from HyukjinKwon/callable-object.
## What changes were proposed in this pull request?
```RFormula``` should handle invalid for both features and label column.
#18496 only handle invalid values in features column. This PR add handling invalid values for label column and test cases.
## How was this patch tested?
Add test cases.
Author: Yanbo Liang <ybliang8@gmail.com>
Closes#18613 from yanboliang/spark-20307.
## What changes were proposed in this pull request?
- Remove Scala 2.10 build profiles and support
- Replace some 2.10 support in scripts with commented placeholders for 2.12 later
- Remove deprecated API calls from 2.10 support
- Remove usages of deprecated context bounds where possible
- Remove Scala 2.10 workarounds like ScalaReflectionLock
- Other minor Scala warning fixes
## How was this patch tested?
Existing tests
Author: Sean Owen <sowen@cloudera.com>
Closes#17150 from srowen/SPARK-19810.
## What changes were proposed in this pull request?
1, HasHandleInvaild support override
2, Make QuantileDiscretizer/Bucketizer/StringIndexer/RFormula inherit from HasHandleInvalid
## How was this patch tested?
existing tests
[JIRA](https://issues.apache.org/jira/browse/SPARK-18619)
Author: Zheng RuiFeng <ruifengz@foxmail.com>
Closes#18582 from zhengruifeng/heritate_HasHandleInvalid.
## What changes were proposed in this pull request?
This PR deals with four points as below:
- Reuse existing DDL parser APIs rather than reimplementing within PySpark
- Support DDL formatted string, `field type, field type`.
- Support case-insensitivity for parsing.
- Support nested data types as below:
**Before**
```
>>> spark.createDataFrame([[[1]]], "struct<a: struct<b: int>>").show()
...
ValueError: The strcut field string format is: 'field_name:field_type', but got: a: struct<b: int>
```
```
>>> spark.createDataFrame([[[1]]], "a: struct<b: int>").show()
...
ValueError: The strcut field string format is: 'field_name:field_type', but got: a: struct<b: int>
```
```
>>> spark.createDataFrame([[1]], "a int").show()
...
ValueError: Could not parse datatype: a int
```
**After**
```
>>> spark.createDataFrame([[[1]]], "struct<a: struct<b: int>>").show()
+---+
| a|
+---+
|[1]|
+---+
```
```
>>> spark.createDataFrame([[[1]]], "a: struct<b: int>").show()
+---+
| a|
+---+
|[1]|
+---+
```
```
>>> spark.createDataFrame([[1]], "a int").show()
+---+
| a|
+---+
| 1|
+---+
```
## How was this patch tested?
Author: hyukjinkwon <gurwls223@gmail.com>
Closes#18590 from HyukjinKwon/deduplicate-python-ddl.
## What changes were proposed in this pull request?
This PR proposes to simply ignore the results in examples that are timezone-dependent in `unix_timestamp` and `from_unixtime`.
```
Failed example:
time_df.select(unix_timestamp('dt', 'yyyy-MM-dd').alias('unix_time')).collect()
Expected:
[Row(unix_time=1428476400)]
Got:unix_timestamp
[Row(unix_time=1428418800)]
```
```
Failed example:
time_df.select(from_unixtime('unix_time').alias('ts')).collect()
Expected:
[Row(ts=u'2015-04-08 00:00:00')]
Got:
[Row(ts=u'2015-04-08 16:00:00')]
```
## How was this patch tested?
Manually tested and `./run-tests --modules pyspark-sql`.
Author: hyukjinkwon <gurwls223@gmail.com>
Closes#18597 from HyukjinKwon/SPARK-20456.
## What changes were proposed in this pull request?
At example of repartitionAndSortWithinPartitions at rdd.py, third argument should be True or False.
I proposed fix of example code.
## How was this patch tested?
* I rename test_repartitionAndSortWithinPartitions to test_repartitionAndSortWIthinPartitions_asc to specify boolean argument.
* I added test_repartitionAndSortWithinPartitions_desc to test False pattern at third argument.
(Please explain how this patch was tested. E.g. unit tests, integration tests, manual tests)
(If this patch involves UI changes, please attach a screenshot; otherwise, remove this)
Please review http://spark.apache.org/contributing.html before opening a pull request.
Author: chie8842 <chie8842@gmail.com>
Closes#18586 from chie8842/SPARK-21358.
## What changes were proposed in this pull request?
Integrate Apache Arrow with Spark to increase performance of `DataFrame.toPandas`. This has been done by using Arrow to convert data partitions on the executor JVM to Arrow payload byte arrays where they are then served to the Python process. The Python DataFrame can then collect the Arrow payloads where they are combined and converted to a Pandas DataFrame. Data types except complex, date, timestamp, and decimal are currently supported, otherwise an `UnsupportedOperation` exception is thrown.
Additions to Spark include a Scala package private method `Dataset.toArrowPayload` that will convert data partitions in the executor JVM to `ArrowPayload`s as byte arrays so they can be easily served. A package private class/object `ArrowConverters` that provide data type mappings and conversion routines. In Python, a private method `DataFrame._collectAsArrow` is added to collect Arrow payloads and a SQLConf "spark.sql.execution.arrow.enable" can be used in `toPandas()` to enable using Arrow (uses the old conversion by default).
## How was this patch tested?
Added a new test suite `ArrowConvertersSuite` that will run tests on conversion of Datasets to Arrow payloads for supported types. The suite will generate a Dataset and matching Arrow JSON data, then the dataset is converted to an Arrow payload and finally validated against the JSON data. This will ensure that the schema and data has been converted correctly.
Added PySpark tests to verify the `toPandas` method is producing equal DataFrames with and without pyarrow. A roundtrip test to ensure the pandas DataFrame produced by pyspark is equal to a one made directly with pandas.
Author: Bryan Cutler <cutlerb@gmail.com>
Author: Li Jin <ice.xelloss@gmail.com>
Author: Li Jin <li.jin@twosigma.com>
Author: Wes McKinney <wes.mckinney@twosigma.com>
Closes#18459 from BryanCutler/toPandas_with_arrow-SPARK-13534.
## What changes were proposed in this pull request?
This PR supports schema in a DDL formatted string for `from_json` in R/Python and `dapply` and `gapply` in R, which are commonly used and/or consistent with Scala APIs.
Additionally, this PR exposes `structType` in R to allow working around in other possible corner cases.
**Python**
`from_json`
```python
from pyspark.sql.functions import from_json
data = [(1, '''{"a": 1}''')]
df = spark.createDataFrame(data, ("key", "value"))
df.select(from_json(df.value, "a INT").alias("json")).show()
```
**R**
`from_json`
```R
df <- sql("SELECT named_struct('name', 'Bob') as people")
df <- mutate(df, people_json = to_json(df$people))
head(select(df, from_json(df$people_json, "name STRING")))
```
`structType.character`
```R
structType("a STRING, b INT")
```
`dapply`
```R
dapply(createDataFrame(list(list(1.0)), "a"), function(x) {x}, "a DOUBLE")
```
`gapply`
```R
gapply(createDataFrame(list(list(1.0)), "a"), "a", function(key, x) { x }, "a DOUBLE")
```
## How was this patch tested?
Doc tests for `from_json` in Python and unit tests `test_sparkSQL.R` in R.
Author: hyukjinkwon <gurwls223@gmail.com>
Closes#18498 from HyukjinKwon/SPARK-21266.
## What changes were proposed in this pull request?
This adds documentation to many functions in pyspark.sql.functions.py:
`upper`, `lower`, `reverse`, `unix_timestamp`, `from_unixtime`, `rand`, `randn`, `collect_list`, `collect_set`, `lit`
Add units to the trigonometry functions.
Renames columns in datetime examples to be more informative.
Adds links between some functions.
## How was this patch tested?
`./dev/lint-python`
`python python/pyspark/sql/functions.py`
`./python/run-tests.py --module pyspark-sql`
Author: Michael Patterson <map222@gmail.com>
Closes#17865 from map222/spark-20456.
## What changes were proposed in this pull request?
Currently `ArrayConstructor` handles an array of typecode `'l'` as `int` when converting Python object in Python 2 into Java object, so if the value is larger than `Integer.MAX_VALUE` or smaller than `Integer.MIN_VALUE` then the overflow occurs.
```python
import array
data = [Row(longarray=array.array('l', [-9223372036854775808, 0, 9223372036854775807]))]
df = spark.createDataFrame(data)
df.show(truncate=False)
```
```
+----------+
|longarray |
+----------+
|[0, 0, -1]|
+----------+
```
This should be:
```
+----------------------------------------------+
|longarray |
+----------------------------------------------+
|[-9223372036854775808, 0, 9223372036854775807]|
+----------------------------------------------+
```
## How was this patch tested?
Added a test and existing tests.
Author: Takuya UESHIN <ueshin@databricks.com>
Closes#18553 from ueshin/issues/SPARK-21327.
## What changes were proposed in this pull request?
This PR aims to bump Py4J in order to fix the following float/double bug.
Py4J 0.10.5 fixes this (https://github.com/bartdag/py4j/issues/272) and the latest Py4J is 0.10.6.
**BEFORE**
```
>>> df = spark.range(1)
>>> df.select(df['id'] + 17.133574204226083).show()
+--------------------+
|(id + 17.1335742042)|
+--------------------+
| 17.1335742042|
+--------------------+
```
**AFTER**
```
>>> df = spark.range(1)
>>> df.select(df['id'] + 17.133574204226083).show()
+-------------------------+
|(id + 17.133574204226083)|
+-------------------------+
| 17.133574204226083|
+-------------------------+
```
## How was this patch tested?
Manual.
Author: Dongjoon Hyun <dongjoon@apache.org>
Closes#18546 from dongjoon-hyun/SPARK-21278.
## What changes were proposed in this pull request?
Support register Java UDAFs in PySpark so that user can use Java UDAF in PySpark. Besides that I also add api in `UDFRegistration`
## How was this patch tested?
Unit test is added
Author: Jeff Zhang <zjffdu@apache.org>
Closes#17222 from zjffdu/SPARK-19439.
## What changes were proposed in this pull request?
Add offset to PySpark in GLM as in #16699.
## How was this patch tested?
Python test
Author: actuaryzhang <actuaryzhang10@gmail.com>
Closes#18534 from actuaryzhang/pythonOffset.
## What changes were proposed in this pull request?
**Context**
While reviewing https://github.com/apache/spark/pull/17227, I realised here we type-dispatch per record. The PR itself is fine in terms of performance as is but this prints a prefix, `"obj"` in exception message as below:
```
from pyspark.sql.types import *
schema = StructType([StructField('s', IntegerType(), nullable=False)])
spark.createDataFrame([["1"]], schema)
...
TypeError: obj.s: IntegerType can not accept object '1' in type <type 'str'>
```
I suggested to get rid of this but during investigating this, I realised my approach might bring a performance regression as it is a hot path.
Only for SPARK-19507 and https://github.com/apache/spark/pull/17227, It needs more changes to cleanly get rid of the prefix and I rather decided to fix both issues together.
**Propersal**
This PR tried to
- get rid of per-record type dispatch as we do in many code paths in Scala so that it improves the performance (roughly ~25% improvement) - SPARK-21296
This was tested with a simple code `spark.createDataFrame(range(1000000), "int")`. However, I am quite sure the actual improvement in practice is larger than this, in particular, when the schema is complicated.
- improve error message in exception describing field information as prose - SPARK-19507
## How was this patch tested?
Manually tested and unit tests were added in `python/pyspark/sql/tests.py`.
Benchmark - codes: https://gist.github.com/HyukjinKwon/c3397469c56cb26c2d7dd521ed0bc5a3
Error message - codes: https://gist.github.com/HyukjinKwon/b1b2c7f65865444c4a8836435100e398
**Before**
Benchmark:
- Results: https://gist.github.com/HyukjinKwon/4a291dab45542106301a0c1abcdca924
Error message
- Results: https://gist.github.com/HyukjinKwon/57b1916395794ce924faa32b14a3fe19
**After**
Benchmark
- Results: https://gist.github.com/HyukjinKwon/21496feecc4a920e50c4e455f836266e
Error message
- Results: https://gist.github.com/HyukjinKwon/7a494e4557fe32a652ce1236e504a395Closes#17227
Author: hyukjinkwon <gurwls223@gmail.com>
Author: David Gingrich <david@textio.com>
Closes#18521 from HyukjinKwon/python-type-dispatch.