4075 commits
Author | SHA1 | Message | Date | |
---|---|---|---|---|
|
82f25f5855 |
[SPARK-30507][SQL] TableCalalog reserved properties shoudn't be changed via options or tblpropeties
### What changes were proposed in this pull request? TableCatalog reserves some properties, e,g `provider`, `location` for internal usage. Some of them are static once create, some of them need specific syntax to modify. Instead of using `OPTIONS (k='v')` or TBLPROPERTIES (k='v'), if k is a reserved TableCatalog property, we should use its specific syntax to add/modify/delete it. e.g. `provider` is a reserved property, we should use the `USING` clause to specify it, and should not allow `ALTER TABLE ... UNSET TBLPROPERTIES('provider')` to delete it. Also, there are two paths for v1/v2 catalog tables to resolve these properties, e.g. the v1 session catalog tables will only use the `USING` clause to decide `provider` but v2 tables will also lookup OPTION/TBLPROPERTIES(although there is a bug prohibit it). Additionally, 'path' is not reserved but holds special meaning for `LOCATION` and it is used in `CREATE/REPLACE TABLE`'s `OPTIONS` sub-clause. Now for the session catalog tables, the `path` is case-insensitive, but for the non-session catalog tables, it is case-sensitive, we should make it both case insensitive for disambiguation. ### Why are the changes needed? prevent reserved properties from being modified unexpectedly unify the property resolution for v1/v2. fix some bugs. ### Does this PR introduce any user-facing change? yes 1 . `location` and `provider` (case sensitive) cannot be used in `CREATE/REPLACE TABLE ... OPTIONS/TBLPROPETIES` and `ALTER TABLE ... SET TBLPROPERTIES (...)`, if legacy on, they will be ignored to let the command success without having side effects 3. Once `path` in `CREATE/REPLACE TABLE ... OPTIONS` is case insensitive for v1 but sensitive for v2, but now we change it case insensitive for both kinds of tables, then v2 tables will also fail if `LOCATION` and `OPTIONS('PaTh' ='abc')` are both specified or will pick `PaTh`'s value as table location if `LOCATION` is missing. 4. Now we will detect if there are two different case `path` keys or more in `CREATE/REPLACE TABLE ... OPTIONS`, once it is a kind of unexpected last-win policy for v1, and v2 is case sensitive. ### How was this patch tested? add ut Closes #27197 from yaooqinn/SPARK-30507. Authored-by: Kent Yao <yaooqinn@hotmail.com> Signed-off-by: Wenchen Fan <wenchen@databricks.com> |
||
|
4e50f0291f |
[SPARK-30323][SQL] Support filters pushdown in CSV datasource
### What changes were proposed in this pull request? In the PR, I propose to support pushed down filters in CSV datasource. The reason of pushing a filter up to `UnivocityParser` is to apply the filter as soon as all its attributes become available i.e. converted from CSV fields to desired values according to the schema. This allows to skip conversions of other values if the filter returns `false`. This can improve performance when pushed filters are highly selective and conversion of CSV string fields to desired values are comparably expensive ( for example, conversion to `TIMESTAMP` values). Here are details of the implementation: - `UnivocityParser.convert()` converts parsed CSV tokens one-by-one sequentially starting from index 0 up to `parsedSchema.length - 1`. At current index `i`, it applies filters that refer to attributes at row fields indexes `0..i`. If any filter returns `false`, it skips conversions of other input tokens. - Pushed filters are converted to expressions. The expressions are bound to row positions according to `requiredSchema`. The expressions are compiled to predicates via generating Java code. - To be able to apply predicates to partially initialized rows, the predicates are grouped, and combined via the `And` expression. Final predicate at index `N` can refer to row fields at the positions `0..N`, and can be applied to a row even if other fields at the positions `N+1..requiredSchema.lenght-1` are not set. ### Why are the changes needed? The changes improve performance on synthetic benchmarks more **than 9 times** (on JDK 8 & 11): ``` OpenJDK 64-Bit Server VM 11.0.5+10 on Mac OS X 10.15.2 Intel(R) Core(TM) i7-4850HQ CPU 2.30GHz Filters pushdown: Best Time(ms) Avg Time(ms) Stdev(ms) Rate(M/s) Per Row(ns) Relative ------------------------------------------------------------------------------------------------------------------------ w/o filters 11889 11945 52 0.0 118893.1 1.0X pushdown disabled 11790 11860 115 0.0 117902.3 1.0X w/ filters 1240 1278 33 0.1 12400.8 9.6X ``` ### Does this PR introduce any user-facing change? No ### How was this patch tested? - Added new test suite `CSVFiltersSuite` - Added tests to `CSVSuite` and `UnivocityParserSuite` Closes #26973 from MaxGekk/csv-filters-pushdown. Authored-by: Maxim Gekk <max.gekk@gmail.com> Signed-off-by: HyukjinKwon <gurwls223@apache.org> |
||
|
be4d825872 |
[SPARK-30312][SQL][FOLLOWUP] Rename conf by adding .enabled
### What changes were proposed in this pull request? Based on the [comment](https://github.com/apache/spark/pull/26956#discussion_r366680558), this patch changes the SQL config name from `spark.sql.truncateTable.ignorePermissionAcl` to `spark.sql.truncateTable.ignorePermissionAcl.enabled`. ### Why are the changes needed? Make this config consistent other SQL configs. ### Does this PR introduce any user-facing change? No. ### How was this patch tested? Unit test. Closes #27210 from viirya/truncate-table-permission-followup. Authored-by: Liang-Chi Hsieh <viirya@gmail.com> Signed-off-by: Dongjoon Hyun <dhyun@apple.com> |
||
|
5a55a5a0d0 |
[SPARK-30518][SQL] Precision and scale should be same for values between -1.0 and 1.0 in Decimal
### What changes were proposed in this pull request? For decimal values between -1.0 and 1.0, it should has same precision and scale in `Decimal`, in order to make it be consistent with `DecimalType`. ### Why are the changes needed? Currently, for values between -1.0 and 1.0, precision and scale is inconsistent between `Decimal` and `DecimalType`. For example, for numbers like 0.3, it will have (precision, scale) as (2, 1) in `Decimal`, but (1, 1) in `DecimalType`: ``` scala> Literal(new BigDecimal("0.3")).dataType.asInstanceOf[DecimalType].precision res3: Int = 1 scala> Literal(new BigDecimal("0.3")).value.asInstanceOf[Decimal].precision res4: Int = 2 ``` We should make `Decimal` be consistent with `DecimalType`. And, here, we change it to only count precision digits after dot for values between -1.0 and 1.0 as other DBMS does, like hive: ``` hive> create table testrel as select 0.3; hive> describe testrel; OK _c0 decimal(1,1) ``` This could bring larger scale for values between -1.0 and 1.0. ### Does this PR introduce any user-facing change? No. ### How was this patch tested? Updated existed tests. Closes #27217 from Ngone51/set-decimal-from-javadecimal. Authored-by: yi.wu <yi.wu@databricks.com> Signed-off-by: Takeshi Yamamuro <yamamuro@apache.org> |
||
|
a3a42b30d0 |
[SPARK-27986][SQL][FOLLOWUP] Respect filter in sql/toString of AggregateExpression
### What changes were proposed in this pull request? This pr intends to add filter information in the explain output of an aggregate (This is a follow-up of #26656). Without this pr: ``` scala> sql("select k, SUM(v) filter (where v > 3) from t group by k").explain(true) == Parsed Logical Plan == 'Aggregate ['k], ['k, unresolvedalias('SUM('v, ('v > 3)), None)] +- 'UnresolvedRelation [t] == Analyzed Logical Plan == k: int, sum(v): bigint Aggregate [k#0], [k#0, sum(cast(v#1 as bigint)) AS sum(v)#3L] +- SubqueryAlias `default`.`t` +- Relation[k#0,v#1] parquet == Optimized Logical Plan == Aggregate [k#0], [k#0, sum(cast(v#1 as bigint)) AS sum(v)#3L] +- Relation[k#0,v#1] parquet == Physical Plan == HashAggregate(keys=[k#0], functions=[sum(cast(v#1 as bigint))], output=[k#0, sum(v)#3L]) +- Exchange hashpartitioning(k#0, 200), true, [id=#20] +- HashAggregate(keys=[k#0], functions=[partial_sum(cast(v#1 as bigint))], output=[k#0, sum#7L]) +- *(1) ColumnarToRow +- FileScan parquet default.t[k#0,v#1] Batched: true, DataFilters: [], Format: Parquet, Location: InMemoryFileIndex[file:/Users/maropu/Repositories/spark/spark-master/spark-warehouse/t], PartitionFilters: [], PushedFilters: [], ReadSchema: struct<k:int,v:int> scala> sql("select k, SUM(v) filter (where v > 3) from t group by k").show() +---+------+ | k|sum(v)| +---+------+ +---+------+ ``` With this pr: ``` scala> sql("select k, SUM(v) filter (where v > 3) from t group by k").explain(true) == Parsed Logical Plan == 'Aggregate ['k], ['k, unresolvedalias('SUM('v, ('v > 3)), None)] +- 'UnresolvedRelation [t] == Analyzed Logical Plan == k: int, sum(v) FILTER (v > 3): bigint Aggregate [k#0], [k#0, sum(cast(v#1 as bigint)) filter (v#1 > 3) AS sum(v) FILTER (v > 3)#5L] +- SubqueryAlias `default`.`t` +- Relation[k#0,v#1] parquet == Optimized Logical Plan == Aggregate [k#0], [k#0, sum(cast(v#1 as bigint)) filter (v#1 > 3) AS sum(v) FILTER (v > 3)#5L] +- Relation[k#0,v#1] parquet == Physical Plan == HashAggregate(keys=[k#0], functions=[sum(cast(v#1 as bigint))], output=[k#0, sum(v) FILTER (v > 3)#5L]) +- Exchange hashpartitioning(k#0, 200), true, [id=#20] +- HashAggregate(keys=[k#0], functions=[partial_sum(cast(v#1 as bigint)) filter (v#1 > 3)], output=[k#0, sum#9L]) +- *(1) ColumnarToRow +- FileScan parquet default.t[k#0,v#1] Batched: true, DataFilters: [], Format: Parquet, Location: InMemoryFileIndex[file:/Users/maropu/Repositories/spark/spark-master/spark-warehouse/t], PartitionFilters: [], PushedFilters: [], ReadSchema: struct<k:int,v:int> scala> sql("select k, SUM(v) filter (where v > 3) from t group by k").show() +---+---------------------+ | k|sum(v) FILTER (v > 3)| +---+---------------------+ +---+---------------------+ ``` ### Why are the changes needed? For better usability. ### Does this PR introduce any user-facing change? No. ### How was this patch tested? Manually. Closes #27198 from maropu/SPARK-27986-FOLLOWUP. Authored-by: Takeshi Yamamuro <yamamuro@apache.org> Signed-off-by: Takeshi Yamamuro <yamamuro@apache.org> |
||
|
883ae331c3 |
[SPARK-30497][SQL] migrate DESCRIBE TABLE to the new framework
### What changes were proposed in this pull request? Use the new framework to resolve the DESCRIBE TABLE command. The v1 DESCRIBE TABLE command supports both table and view. Checked with Hive and Presto, they don't have DESCRIBE TABLE syntax but only DESCRIBE, which supports both table and view: 1. https://cwiki.apache.org/confluence/display/Hive/LanguageManual+DDL#LanguageManualDDL-DescribeTable/View/MaterializedView/Column 2. https://prestodb.io/docs/current/sql/describe.html We should make it clear that DESCRIBE support both table and view, by renaming the command to `DescribeRelation`. This PR also tunes the framework a little bit to support the case that a command accepts both table and view. ### Why are the changes needed? This is a part of effort to make the relation lookup behavior consistent: SPARK-29900. Note that I make a separate PR here instead of #26921, as I need to update the framework to support a new use case: accept both table and view. ### Does this PR introduce any user-facing change? no ### How was this patch tested? existing tests Closes #27187 from cloud-fan/describe. Authored-by: Wenchen Fan <wenchen@databricks.com> Signed-off-by: Xiao Li <gatorsmile@gmail.com> |
||
|
5f6cd61913 |
[SPARK-29708][SQL] Correct aggregated values when grouping sets are duplicated
### What changes were proposed in this pull request? This pr intends to fix wrong aggregated values in `GROUPING SETS` when there are duplicated grouping sets in a query (e.g., `GROUPING SETS ((k1),(k1))`). For example; ``` scala> spark.table("t").show() +---+---+---+ | k1| k2| v| +---+---+---+ | 0| 0| 3| +---+---+---+ scala> sql("""select grouping_id(), k1, k2, sum(v) from t group by grouping sets ((k1),(k1,k2),(k2,k1),(k1,k2))""").show() +-------------+---+----+------+ |grouping_id()| k1| k2|sum(v)| +-------------+---+----+------+ | 0| 0| 0| 9| <---- wrong aggregate value and the correct answer is `3` | 1| 0|null| 3| +-------------+---+----+------+ // PostgreSQL case postgres=# select k1, k2, sum(v) from t group by grouping sets ((k1),(k1,k2),(k2,k1),(k1,k2)); k1 | k2 | sum ----+------+----- 0 | 0 | 3 0 | 0 | 3 0 | 0 | 3 0 | NULL | 3 (4 rows) // Hive case hive> select GROUPING__ID, k1, k2, sum(v) from t group by k1, k2 grouping sets ((k1),(k1,k2),(k2,k1),(k1,k2)); 1 0 NULL 3 0 0 0 3 ``` [MS SQL Server has the same behaviour with PostgreSQL](https://github.com/apache/spark/pull/26961#issuecomment-573638442). This pr follows the behaviour of PostgreSQL/SQL server; it adds one more virtual attribute in `Expand` for avoiding wrongly grouping rows with the same grouping ID. ### Why are the changes needed? To fix bugs. ### Does this PR introduce any user-facing change? No. ### How was this patch tested? The existing tests. Closes #26961 from maropu/SPARK-29708. Authored-by: Takeshi Yamamuro <yamamuro@apache.org> Signed-off-by: Takeshi Yamamuro <yamamuro@apache.org> |
||
|
240840fe92 |
[SPARK-30515][SQL] Refactor SimplifyBinaryComparison to reduce the time complexity
### What changes were proposed in this pull request? The changes in the rule `SimplifyBinaryComparison` from https://github.com/apache/spark/pull/27008 could bring performance regression in the optimizer when there are a large set of filter conditions. We need to improve the implementation and reduce the time complexity. ### Why are the changes needed? Need to fix the potential performance regression in the optimizer. ### Does this PR introduce any user-facing change? No ### How was this patch tested? Existing unit tests. Also run a micor benchmark in `BinaryComparisonSimplificationSuite` ``` object Optimize extends RuleExecutor[LogicalPlan] { val batches = Batch("Constant Folding", FixedPoint(50), SimplifyBinaryComparison) :: Nil } test("benchmark") { val a = Symbol("a") val condition = (1 to 500).map(i => EqualTo(a, a)).reduceLeft(And) val finalCondition = And(condition, IsNotNull(a)) val plan = nullableRelation.where(finalCondition).analyze val start = System.nanoTime() Optimize.execute(plan) println((System.nanoTime() - start) /1000000) } ``` Before the changes: 2507ms After the changes: 3ms Closes #27212 from gengliangwang/SimplifyBinaryComparison. Authored-by: Gengliang Wang <gengliang.wang@databricks.com> Signed-off-by: Takeshi Yamamuro <yamamuro@apache.org> |
||
|
a2aa966ef6 |
[SPARK-29544][SQL] optimize skewed partition based on data size
### What changes were proposed in this pull request? Skew Join is common and can severely downgrade performance of queries, especially those with joins. This PR aim to optimization the skew join based on the runtime Map output statistics by adding "OptimizeSkewedPartitions" rule. And The details design doc is [here](https://docs.google.com/document/d/1NkXN-ck8jUOS0COz3f8LUW5xzF8j9HFjoZXWGGX2HAg/edit). Currently we can support "Inner, Cross, LeftSemi, LeftAnti, LeftOuter, RightOuter" join type. ### Why are the changes needed? To optimize the skewed partition in runtime based on AQE ### Does this PR introduce any user-facing change? No ### How was this patch tested? UT Closes #26434 from JkSelf/skewedPartitionBasedSize. Lead-authored-by: jiake <ke.a.jia@intel.com> Co-authored-by: Wenchen Fan <wenchen@databricks.com> Co-authored-by: JiaKe <ke.a.jia@intel.com> Signed-off-by: Wenchen Fan <wenchen@databricks.com> |
||
|
e0efd213eb |
[SPARK-30292][SQL] Throw Exception when invalid string is cast to numeric type in ANSI mode
### What changes were proposed in this pull request? If spark.sql.ansi.enabled is set, throw exception when cast to any numeric type do not follow the ANSI SQL standards. ### Why are the changes needed? ANSI SQL standards do not allow invalid strings to get casted into numeric types and throw exception for that. Currently spark sql gives NULL in such cases. Before: `select cast('str' as decimal) => NULL` After : `select cast('str' as decimal) => invalid input syntax for type numeric: str` These results are after setting `spark.sql.ansi.enabled=true` ### Does this PR introduce any user-facing change? Yes. Now when ansi mode is on users will get arithmetic exception for invalid strings. ### How was this patch tested? Unit Tests Added. Closes #26933 from iRakson/castDecimalANSI. Lead-authored-by: root1 <raksonrakesh@gmail.com> Co-authored-by: iRakson <raksonrakesh@gmail.com> Signed-off-by: Wenchen Fan <wenchen@databricks.com> |
||
|
88fc8dbc09 |
[SPARK-30482][SQL][CORE][TESTS] Add sub-class of AppenderSkeleton reusable in tests
### What changes were proposed in this pull request? In the PR, I propose to define a sub-class of `AppenderSkeleton` in `SparkFunSuite` and reuse it from other tests. The class stores incoming `LoggingEvent` in an array which is available to tests for future analysis of logged events. ### Why are the changes needed? This eliminates code duplication in tests. ### Does this PR introduce any user-facing change? No ### How was this patch tested? By existing test suites - `CSVSuite`, `OptimizerLoggingSuite`, `JoinHintSuite`, `CodeGenerationSuite` and `SQLConfSuite`. Closes #27166 from MaxGekk/dedup-appender-skeleton. Authored-by: Maxim Gekk <max.gekk@gmail.com> Signed-off-by: Takeshi Yamamuro <yamamuro@apache.org> |
||
|
1846b0261b |
[SPARK-30500][SPARK-30501][SQL] Remove SQL configs deprecated in Spark 2.1 and 2.3
### What changes were proposed in this pull request? In the PR, I propose to remove already deprecated SQL configs: - `spark.sql.variable.substitute.depth` deprecated in Spark 2.1 - `spark.sql.parquet.int64AsTimestampMillis` deprecated in Spark 2.3 Also I moved `removedSQLConfigs` closer to `deprecatedSQLConfigs`. This will allow to have references to other config entries. ### Why are the changes needed? To improve code maintainability. ### Does this PR introduce any user-facing change? Yes. ### How was this patch tested? By existing test suites `ParquetQuerySuite` and `SQLConfSuite`. Closes #27169 from MaxGekk/remove-deprecated-conf-2.4. Authored-by: Maxim Gekk <max.gekk@gmail.com> Signed-off-by: HyukjinKwon <gurwls223@apache.org> |
||
|
28fc0437ce |
[SPARK-28152][SQL][FOLLOWUP] Add a legacy conf for old MsSqlServerDialect numeric mapping
### What changes were proposed in this pull request? This is a follow-up for https://github.com/apache/spark/pull/25248 . ### Why are the changes needed? The new behavior cannot access the existing table which is created by old behavior. This PR provides a way to avoid new behavior for the existing users. ### Does this PR introduce any user-facing change? Yes. This will fix the broken behavior on the existing tables. ### How was this patch tested? Pass the Jenkins and manually run JDBC integration test. ``` build/mvn install -DskipTests build/mvn -Pdocker-integration-tests -pl :spark-docker-integration-tests_2.12 test ``` Closes #27184 from dongjoon-hyun/SPARK-28152-CONF. Authored-by: Dongjoon Hyun <dhyun@apple.com> Signed-off-by: Dongjoon Hyun <dhyun@apple.com> |
||
|
8ce7962931 |
[SPARK-30245][SQL] Add cache for Like and RLike when pattern is not static
### What changes were proposed in this pull request? Add cache for Like and RLike when pattern is not static ### Why are the changes needed? When pattern is not static, we should avoid compile pattern every time if some pattern is same. Here is perf numbers, include 3 test groups and use `range` to make it easy. ``` // --------------------- // 10,000 rows and 10 partitions val df1 = spark.range(0, 10000, 1, 10).withColumnRenamed("id", "id1") val df2 = spark.range(0, 10000, 1, 10).withColumnRenamed("id", "id2") val start = System.currentTimeMillis df1.join(df2).where("id2 like id1").count() // before 16939 // after 6352 println(System.currentTimeMillis - start) // --------------------- // 10,000 rows and 100 partitions val df1 = spark.range(0, 10000, 1, 100).withColumnRenamed("id", "id1") val df2 = spark.range(0, 10000, 1, 100).withColumnRenamed("id", "id2") val start = System.currentTimeMillis df1.join(df2).where("id2 like id1").count() // before 11070 // after 4680 println(System.currentTimeMillis - start) // --------------------- // 20,000 rows and 10 partitions val df1 = spark.range(0, 20000, 1, 10).withColumnRenamed("id", "id1") val df2 = spark.range(0, 20000, 1, 10).withColumnRenamed("id", "id2") val start = System.currentTimeMillis df1.join(df2).where("id2 like id1").count() // before 66962 // after 29934 println(System.currentTimeMillis - start) ``` ### Does this PR introduce any user-facing change? No. ### How was this patch tested? Closes #26875 from ulysses-you/SPARK-30245. Authored-by: ulysses <youxiduo@weidian.com> Signed-off-by: HyukjinKwon <gurwls223@apache.org> |
||
|
823e3d309c |
[SPARK-30353][SQL] Add IsNotNull check in SimplifyBinaryComparison optimization
### What changes were proposed in this pull request? Now Spark can propagate constraint during sql optimization when `spark.sql.constraintPropagation.enabled` is true, then `where c = 1` will convert to `where c = 1 and c is not null`. We also can use constraint in `SimplifyBinaryComparison`. `SimplifyBinaryComparison` will simplify expression which is not nullable and semanticEquals. And we also can simplify if one expression is infered `IsNotNull`. ### Why are the changes needed? Simplify SQL. ``` create table test (c1 string); explain extended select c1 from test where c1 = c1 limit 10; -- before GlobalLimit 10 +- LocalLimit 10 +- Filter (isnotnull(c1#20) AND (c1#20 = c1#20)) +- Relation[c1#20] -- after GlobalLimit 10 +- LocalLimit 10 +- Filter (isnotnull(c1#20) +- Relation[c1#20] explain extended select c1 from test where c1 > c1 limit 10; -- before GlobalLimit 10 +- LocalLimit 10 +- Filter (isnotnull(c1#20) && (c1#20 > c1#20)) +- Relation[c1#20] -- after LocalRelation <empty>, [c1#20] ``` ### Does this PR introduce any user-facing change? No. ### How was this patch tested? Add UT. Closes #27008 from ulysses-you/SPARK-30353. Authored-by: ulysses <youxiduo@weidian.com> Signed-off-by: Wenchen Fan <wenchen@databricks.com> |
||
|
b5bc3e12a6 |
[SPARK-30312][SQL] Preserve path permission and acl when truncate table
### What changes were proposed in this pull request? This patch proposes to preserve existing permission/acls of paths when truncate table/partition. ### Why are the changes needed? When Spark SQL truncates table, it deletes the paths of table/partitions, then re-create new ones. If permission/acls were set on the paths, the existing permission/acls will be deleted. We should preserve the permission/acls if possible. ### Does this PR introduce any user-facing change? Yes. When truncate table/partition, Spark will keep permission/acls of paths. ### How was this patch tested? Unit test. Manual test: 1. Create a table. 2. Manually change it permission/acl 3. Truncate table 4. Check permission/acl ```scala val df = Seq(1, 2, 3).toDF df.write.mode("overwrite").saveAsTable("test.test_truncate_table") val testTable = spark.table("test.test_truncate_table") testTable.show() +-----+ |value| +-----+ | 1| | 2| | 3| +-----+ // hdfs dfs -setfacl ... // hdfs dfs -getfacl ... sql("truncate table test.test_truncate_table") // hdfs dfs -getfacl ... val testTable2 = spark.table("test.test_truncate_table") testTable2.show() +-----+ |value| +-----+ +-----+ ``` 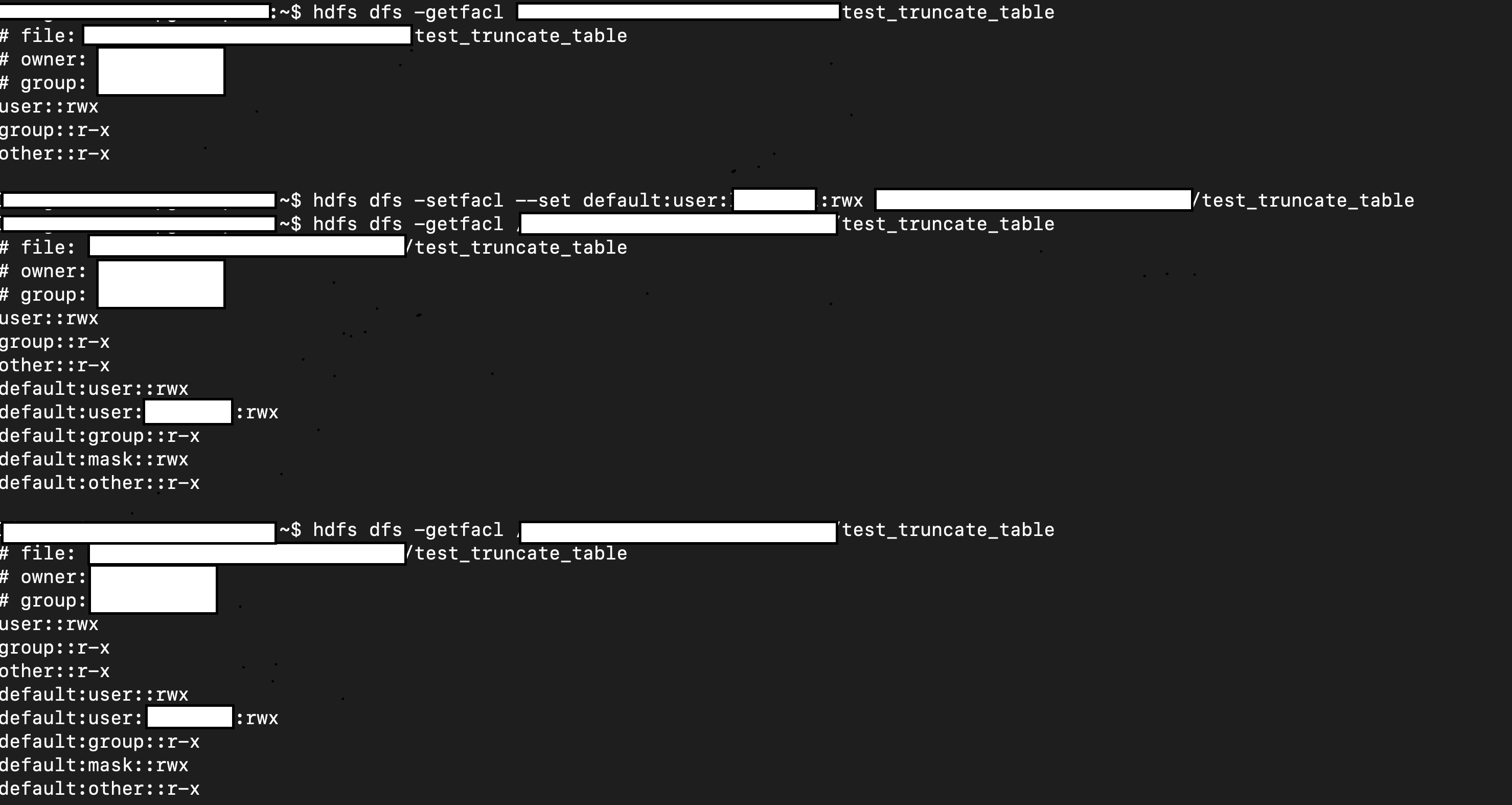 Closes #26956 from viirya/truncate-table-permission. Lead-authored-by: Liang-Chi Hsieh <liangchi@uber.com> Co-authored-by: Liang-Chi Hsieh <viirya@gmail.com> Signed-off-by: Dongjoon Hyun <dhyun@apple.com> |
||
|
b942832bd3 |
[SPARK-30343][SQL] Skip unnecessary checks in RewriteDistinctAggregates
### What changes were proposed in this pull request? This pr intends to skip the unnecessary checks that most aggregate quries don't need in RewriteDistinctAggregates. ### Why are the changes needed? For minor optimization. ### Does this PR introduce any user-facing change? No. ### How was this patch tested? Existing tests. Closes #26997 from maropu/OptDistinctAggRewrite. Authored-by: Takeshi Yamamuro <yamamuro@apache.org> Signed-off-by: Takeshi Yamamuro <yamamuro@apache.org> |
||
|
2a629e5d10 |
[SPARK-30234][SQL] ADD FILE cannot add directories from sql CLI
### What changes were proposed in this pull request? Now users can add directories from sql CLI as well using ADD FILE command and setting spark.sql.addDirectory.recursive to true. ### Why are the changes needed? In SPARK-4687, support was added for adding directories as resources. But sql users cannot use that feature from CLI. `ADD FILE /path/to/folder` gives the following error: `org.apache.spark.SparkException: Added file /path/to/folder is a directory and recursive is not turned on.` Users need to turn on `recursive` for adding directories. Thus a configuration was required which will allow users to turn on `recursive`. Also Hive allow users to add directories from their shell. ### Does this PR introduce any user-facing change? Yes. Users can set recursive using `spark.sql.addDirectory.recursive`. ### How was this patch tested? Manually. Will add test cases soon. SPARK SCREENSHOTS When `spark.sql.addDirectory.recursive` is not turned on. 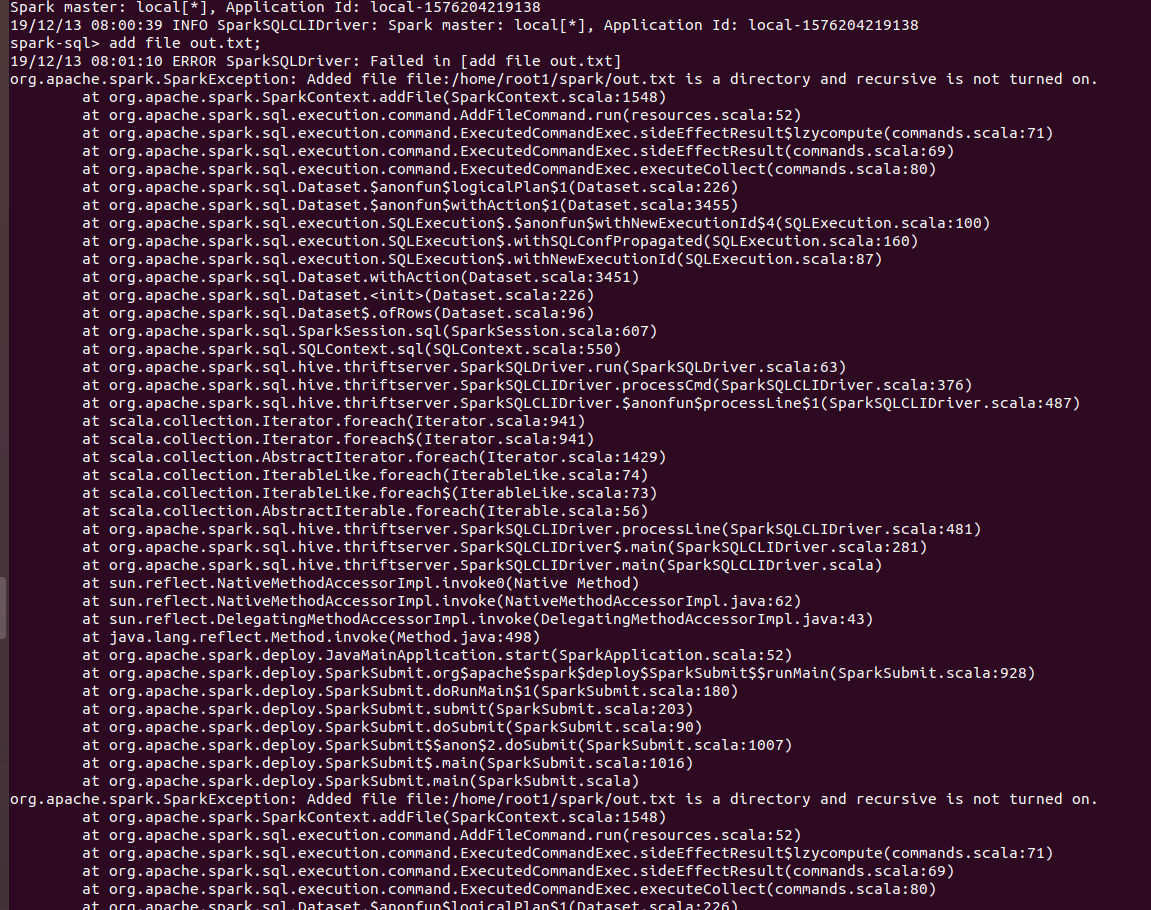 After setting `spark.sql.addDirectory.recursive` to true.  HIVE SCREENSHOT  `RELEASE_NOTES.txt` is text file while `dummy` is a directory. Closes #26863 from iRakson/SPARK-30234. Lead-authored-by: root1 <raksonrakesh@gmail.com> Co-authored-by: iRakson <raksonrakesh@gmail.com> Signed-off-by: HyukjinKwon <gurwls223@apache.org> |
||
|
418f7dc973 |
[SPARK-30447][SQL] Constant propagation nullability issue
## What changes were proposed in this pull request? This PR fixes `ConstantPropagation` rule as the current implementation produce incorrect results in some cases. E.g. ``` SELECT * FROM t WHERE NOT(c = 1 AND c + 1 = 1) ``` returns those rows where `c` is null due to `1 + 1 = 1` propagation but it shouldn't. ## Why are the changes needed? To fix a bug. ## Does this PR introduce any user-facing change? Yes, fixes a bug. ## How was this patch tested? New UTs. Closes #27119 from peter-toth/SPARK-30447. Authored-by: Peter Toth <peter.toth@gmail.com> Signed-off-by: Takeshi Yamamuro <yamamuro@apache.org> |
||
|
bcf07cbf5f |
[SPARK-30018][SQL] Support ALTER DATABASE SET OWNER syntax
### What changes were proposed in this pull request? In this pull request, we are going to support `SET OWNER` syntax for databases and namespaces, ```sql ALTER (DATABASE|SCHEME|NAMESPACE) database_name SET OWNER [USER|ROLE|GROUP] user_or_role_group; ``` Before this commit |
||
|
0ec0355611 |
[SPARK-30439][SQL] Support non-nullable column in CREATE TABLE, ADD COLUMN and ALTER TABLE
### What changes were proposed in this pull request? Allow users to specify NOT NULL in CREATE TABLE and ADD COLUMN column definition, and add a new SQL syntax to alter column nullability: ALTER TABLE ... ALTER COLUMN SET/DROP NOT NULL. This is a SQL standard syntax: ``` <alter column definition> ::= ALTER [ COLUMN ] <column name> <alter column action> <alter column action> ::= <set column default clause> | <drop column default clause> | <set column not null clause> | <drop column not null clause> | ... <set column not null clause> ::= SET NOT NULL <drop column not null clause> ::= DROP NOT NULL ``` ### Why are the changes needed? Previously we don't support it because the table schema in hive catalog are always nullable. Since we have catalog plugin now, it makes more sense to support NOT NULL at spark side, and let catalog implementations to decide if they support it or not. ### Does this PR introduce any user-facing change? Yes, this is a new feature ### How was this patch tested? new tests Closes #27110 from cloud-fan/nullable. Authored-by: Wenchen Fan <wenchen@databricks.com> Signed-off-by: HyukjinKwon <gurwls223@apache.org> |
||
|
1ffa627ffb |
[SPARK-30416][SQL] Log a warning for deprecated SQL config in set() and unset()
### What changes were proposed in this pull request? 1. Put all deprecated SQL configs the map `SQLConf.deprecatedSQLConfigs` with extra info about when configs were deprecated and additional comments that explain why a config was deprecated, what an user can use instead of it. Here is the list of already deprecated configs: - spark.sql.hive.verifyPartitionPath - spark.sql.execution.pandas.respectSessionTimeZone - spark.sql.legacy.execution.pandas.groupedMap.assignColumnsByName - spark.sql.parquet.int64AsTimestampMillis - spark.sql.variable.substitute.depth - spark.sql.execution.arrow.enabled - spark.sql.execution.arrow.fallback.enabled 2. Output warning in `set()` and `unset()` about deprecated SQL configs ### Why are the changes needed? This should improve UX with Spark SQL and notify users about already deprecated SQL configs. ### Does this PR introduce any user-facing change? Yes, before: ``` spark-sql> set spark.sql.hive.verifyPartitionPath=true; spark.sql.hive.verifyPartitionPath true ``` After: ``` spark-sql> set spark.sql.hive.verifyPartitionPath=true; 20/01/03 21:28:17 WARN RuntimeConfig: The SQL config 'spark.sql.hive.verifyPartitionPath' has been deprecated in Spark v3.0.0 and may be removed in the future. This config is replaced by spark.files.ignoreMissingFiles. spark.sql.hive.verifyPartitionPath true ``` ### How was this patch tested? Add new test which registers new log appender and catches all logging to check that `set()` and `unset()` log any warning. Closes #27092 from MaxGekk/group-deprecated-sql-configs. Authored-by: Maxim Gekk <max.gekk@gmail.com> Signed-off-by: HyukjinKwon <gurwls223@apache.org> |
||
|
f8d59572b0 |
[SPARK-29219][SQL] Introduce SupportsCatalogOptions for TableProvider
### What changes were proposed in this pull request? This PR introduces `SupportsCatalogOptions` as an interface for `TableProvider`. Through `SupportsCatalogOptions`, V2 DataSources can implement the two methods `extractIdentifier` and `extractCatalog` to support the creation, and existence check of tables without requiring a formal TableCatalog implementation. We currently don't support all SaveModes for DataSourceV2 in DataFrameWriter.save. The idea here is that eventually File based tables can be written with `DataFrameWriter.save(path)` will create a PathIdentifier where the name is `path`, and the V2SessionCatalog will be able to perform FileSystem checks at `path` to support ErrorIfExists and Ignore SaveModes. ### Why are the changes needed? To support all Save modes for V2 data sources with DataFrameWriter. Since we can now support table creation, we will be able to provide partitioning information when first creating the table as well. ### Does this PR introduce any user-facing change? Introduces a new interface ### How was this patch tested? Will add tests once interface is vetted. Closes #26913 from brkyvz/catalogOptions. Lead-authored-by: Burak Yavuz <brkyvz@gmail.com> Co-authored-by: Burak Yavuz <burak@databricks.com> Signed-off-by: Burak Yavuz <brkyvz@gmail.com> |
||
|
c37312342e |
[SPARK-30183][SQL] Disallow to specify reserved properties in CREATE/ALTER NAMESPACE syntax
### What changes were proposed in this pull request? Currently, COMMENT and LOCATION are reserved properties for Datasource v2 namespaces. They can be set via specific clauses and via properties. And the ones specified in clauses take precede of properties. Since they are reserved, which means they are not able to visit directly. They should be used in COMMENT/LOCATION clauses ONLY. ### Why are the changes needed? make reserved properties be reserved. ### Does this PR introduce any user-facing change? yes, 'location', 'comment' are not allowed use in db properties ### How was this patch tested? UNIT tests. Closes #26806 from yaooqinn/SPARK-30183. Authored-by: Kent Yao <yaooqinn@hotmail.com> Signed-off-by: Wenchen Fan <wenchen@databricks.com> |
||
|
a93b996635 |
[MINOR][ML][INT] Array.fill(0) -> Array.ofDim; Array.empty -> Array.emptyIntArray
### What changes were proposed in this pull request? 1, for primitive types `Array.fill(n)(0)` -> `Array.ofDim(n)`; 2, for `AnyRef` types `Array.fill(n)(null)` -> `Array.ofDim(n)`; 3, for primitive types `Array.empty[XXX]` -> `Array.emptyXXXArray` ### Why are the changes needed? `Array.ofDim` avoid assignments; `Array.emptyXXXArray` avoid create new object; ### Does this PR introduce any user-facing change? No ### How was this patch tested? existing testsuites Closes #27133 from zhengruifeng/minor_fill_ofDim. Authored-by: zhengruifeng <ruifengz@foxmail.com> Signed-off-by: HyukjinKwon <gurwls223@apache.org> |
||
|
b2ed6d0b88 |
[SPARK-30214][SQL][FOLLOWUP] Remove statement logical plans for namespace commands
### What changes were proposed in this pull request? This is a follow-up to address the following comment: https://github.com/apache/spark/pull/27095#discussion_r363152180 Currently, a SQL command string is parsed to a "statement" logical plan, converted to a logical plan with catalog/namespace, then finally converted to a physical plan. With the new resolution framework, there is no need to create a "statement" logical plan; a logical plan can contain `UnresolvedNamespace` which will be resolved to a `ResolvedNamespace`. This should simply the code base and make it a bit easier to add a new command. ### Why are the changes needed? Clean up codebase. ### Does this PR introduce any user-facing change? No ### How was this patch tested? Existing tests should cover the changes. Closes #27125 from imback82/SPARK-30214-followup. Authored-by: Terry Kim <yuminkim@gmail.com> Signed-off-by: Wenchen Fan <wenchen@databricks.com> |
||
|
1160457eed |
[SPARK-30429][SQL] Optimize catalogString and usage in ValidateExternalType.errMsg to avoid OOM
### What changes were proposed in this pull request?
This patch proposes:
1. Fix OOM at WideSchemaBenchmark: make `ValidateExternalType.errMsg` lazy variable, i.e. not to initiate it in the constructor
2. Truncate `errMsg`: Replacing `catalogString` with `simpleString` which is truncated
3. Optimizing `override def catalogString` in `StructType`: Make `catalogString` more efficient in string generation by using `StringConcat`
### Why are the changes needed?
In the JIRA, it is found that WideSchemaBenchmark fails with OOM, like:
```
[error] Exception in thread "main" org.apache.spark.sql.catalyst.errors.package$TreeNodeException: makeCopy, tree: validateexternaltype(getexternalrowfield(input[0, org.apac
he.spark.sql.Row, true], 0, a), StructField(b,StructType(StructField(c,StructType(StructField(value_1,LongType,true), StructField(value_10,LongType,true), StructField(value_
100,LongType,true), StructField(value_1000,LongType,true), StructField(value_1001,LongType,true), StructField(value_1002,LongType,true), StructField(value_1003,LongType,true
), StructField(value_1004,LongType,true), StructField(value_1005,LongType,true), StructField(value_1006,LongType,true), StructField(value_1007,LongType,true), StructField(va
lue_1008,LongType,true), StructField(value_1009,LongType,true), StructField(value_101,LongType,true), StructField(value_1010,LongType,true), StructField(value_1011,LongType,
...
ue), StructField(value_99,LongType,true), StructField(value_990,LongType,true), StructField(value_991,LongType,true), StructField(value_992,LongType,true), StructField(value
_993,LongType,true), StructField(value_994,LongType,true), StructField(value_995,LongType,true), StructField(value_996,LongType,true), StructField(value_997,LongType,true),
StructField(value_998,LongType,true), StructField(value_999,LongType,true)),true))
[error] at org.apache.spark.sql.catalyst.errors.package$.attachTree(package.scala:56)
[error] at org.apache.spark.sql.catalyst.trees.TreeNode.makeCopy(TreeNode.scala:435)
[error] at org.apache.spark.sql.catalyst.trees.TreeNode.mapChildren(TreeNode.scala:408)
[error] at org.apache.spark.sql.catalyst.trees.TreeNode.mapChildren(TreeNode.scala:327)
[error] at org.apache.spark.sql.catalyst.trees.TreeNode.transformUp(TreeNode.scala:307)
....
[error] at org.apache.spark.sql.catalyst.trees.TreeNode.$anonfun$mapChildren$1(TreeNode.scala:404)
[error] at org.apache.spark.sql.catalyst.trees.TreeNode.mapProductIterator(TreeNode.scala:214)
[error] at org.apache.spark.sql.catalyst.trees.TreeNode.mapChildren(TreeNode.scala:374)
[error] at org.apache.spark.sql.catalyst.trees.TreeNode.mapChildren(TreeNode.scala:327)
[error] at org.apache.spark.sql.catalyst.trees.TreeNode.transformUp(TreeNode.scala:307)
[error] at org.apache.spark.sql.catalyst.trees.TreeNode.$anonfun$transformUp$1(TreeNode.scala:307)
[error] at org.apache.spark.sql.catalyst.trees.TreeNode.$anonfun$mapChildren$1(TreeNode.scala:376)
[error] at org.apache.spark.sql.catalyst.trees.TreeNode.mapProductIterator(TreeNode.scala:214)
[error] at org.apache.spark.sql.catalyst.trees.TreeNode.mapChildren(TreeNode.scala:374)
[error] at org.apache.spark.sql.catalyst.trees.TreeNode.mapChildren(TreeNode.scala:327)
[error] at org.apache.spark.sql.catalyst.trees.TreeNode.transformUp(TreeNode.scala:307)
[error] at org.apache.spark.sql.catalyst.encoders.ExpressionEncoder.<init>(ExpressionEncoder.scala:198)
[error] at org.apache.spark.sql.catalyst.encoders.RowEncoder$.apply(RowEncoder.scala:71)
[error] at org.apache.spark.sql.Dataset$.ofRows(Dataset.scala:88)
[error] at org.apache.spark.sql.SparkSession.internalCreateDataFrame(SparkSession.scala:554)
[error] at org.apache.spark.sql.DataFrameReader.json(DataFrameReader.scala:476)
[error] at org.apache.spark.sql.execution.benchmark.WideSchemaBenchmark$.$anonfun$wideShallowlyNestedStructFieldReadAndWrite$1(WideSchemaBenchmark.scala:126)
...
[error] Caused by: java.lang.OutOfMemoryError: GC overhead limit exceeded
[error] at java.util.Arrays.copyOf(Arrays.java:3332)
[error] at java.lang.AbstractStringBuilder.ensureCapacityInternal(AbstractStringBuilder.java:124)
[error] at java.lang.AbstractStringBuilder.append(AbstractStringBuilder.java:448)
[error] at java.lang.StringBuilder.append(StringBuilder.java:136)
[error] at scala.collection.mutable.StringBuilder.append(StringBuilder.scala:213)
[error] at scala.collection.TraversableOnce.$anonfun$addString$1(TraversableOnce.scala:368)
[error] at scala.collection.TraversableOnce$$Lambda$67/667447085.apply(Unknown Source)
[error] at scala.collection.IndexedSeqOptimized.foreach(IndexedSeqOptimized.scala:36)
[error] at scala.collection.IndexedSeqOptimized.foreach$(IndexedSeqOptimized.scala:33)
[error] at scala.collection.mutable.ArrayOps$ofRef.foreach(ArrayOps.scala:198)
[error] at scala.collection.TraversableOnce.addString(TraversableOnce.scala:362)
[error] at scala.collection.TraversableOnce.addString$(TraversableOnce.scala:358)
[error] at scala.collection.mutable.ArrayOps$ofRef.addString(ArrayOps.scala:198)
[error] at scala.collection.TraversableOnce.mkString(TraversableOnce.scala:328)
[error] at scala.collection.TraversableOnce.mkString$(TraversableOnce.scala:327)
[error] at scala.collection.mutable.ArrayOps$ofRef.mkString(ArrayOps.scala:198)
[error] at scala.collection.TraversableOnce.mkString(TraversableOnce.scala:330)
[error] at scala.collection.TraversableOnce.mkString$(TraversableOnce.scala:330)
[error] at scala.collection.mutable.ArrayOps$ofRef.mkString(ArrayOps.scala:198)
[error] at org.apache.spark.sql.types.StructType.catalogString(StructType.scala:411)
[error] at org.apache.spark.sql.catalyst.expressions.objects.ValidateExternalType.<init>(objects.scala:1695)
[error] at sun.reflect.NativeConstructorAccessorImpl.newInstance0(Native Method)
[error] at sun.reflect.NativeConstructorAccessorImpl.newInstance(NativeConstructorAccessorImpl.java:62)
[error] at sun.reflect.DelegatingConstructorAccessorImpl.newInstance(DelegatingConstructorAccessorImpl.java:45)
[error] at java.lang.reflect.Constructor.newInstance(Constructor.java:423)
[error] at org.apache.spark.sql.catalyst.trees.TreeNode.$anonfun$makeCopy$7(TreeNode.scala:468)
[error] at org.apache.spark.sql.catalyst.trees.TreeNode$$Lambda$934/387827651.apply(Unknown Source)
[error] at org.apache.spark.sql.catalyst.trees.CurrentOrigin$.withOrigin(TreeNode.scala:72)
[error] at org.apache.spark.sql.catalyst.trees.TreeNode.$anonfun$makeCopy$1(TreeNode.scala:467)
[error] at org.apache.spark.sql.catalyst.trees.TreeNode$$Lambda$929/449240381.apply(Unknown Source)
[error] at org.apache.spark.sql.catalyst.errors.package$.attachTree(package.scala:52)
[error] at org.apache.spark.sql.catalyst.trees.TreeNode.makeCopy(TreeNode.scala:435)
```
It is after
|
||
|
9535776e28 |
[SPARK-30302][SQL] Complete info for show create table for views
### What changes were proposed in this pull request? Add table/column comments and table properties to the result of show create table of views. ### Does this PR introduce any user-facing change? When show create table for views, after this patch, the result can contain table/column comments and table properties if they exist. ### How was this patch tested? add new tests Closes #26944 from wzhfy/complete_show_create_view. Authored-by: Zhenhua Wang <wzh_zju@163.com> Signed-off-by: Takeshi Yamamuro <yamamuro@apache.org> |
||
|
9479887ba1 |
[SPARK-30039][SQL] CREATE FUNCTION should do multi-catalog resolution
### What changes were proposed in this pull request? Add CreateFunctionStatement and make CREATE FUNCTION go through the same catalog/table resolution framework of v2 commands. ### Why are the changes needed? It's important to make all the commands have the same table resolution behavior, to avoid confusing CREATE FUNCTION namespace.function ### Does this PR introduce any user-facing change? Yes. When running CREATE FUNCTION namespace.function Spark fails the command if the current catalog is set to a v2 catalog. ### How was this patch tested? Unit tests. Closes #26890 from planga82/feature/SPARK-30039_CreateFunctionV2Command. Authored-by: Pablo Langa <soypab@gmail.com> Signed-off-by: Wenchen Fan <wenchen@databricks.com> |
||
|
8c121b0827 |
[SPARK-30431][SQL] Update SqlBase.g4 to create commentSpec pattern like locationSpec
### What changes were proposed in this pull request? In `SqlBase.g4`, the `comment` clause is used as `COMMENT comment=STRING` and `COMMENT STRING` in many places. While the `location` clause often appears along with the `comment` clause with a pattern defined as ```sql locationSpec : LOCATION STRING ; ``` Then, we have to visit `locationSpec` as a `List` but comment as a single token. We defined `commentSpec` for the comment clause to simplify and unify the grammar and the invocations. ### Why are the changes needed? To simplify the grammar. ### Does this PR introduce any user-facing change? no ### How was this patch tested? existing tests Closes #27102 from yaooqinn/SPARK-30431. Authored-by: Kent Yao <yaooqinn@hotmail.com> Signed-off-by: Wenchen Fan <wenchen@databricks.com> |
||
|
314e70fe23 |
[SPARK-30214][SQL] V2 commands resolves namespaces with new resolution framework
### What changes were proposed in this pull request? #26847 introduced new framework for resolving catalog/namespaces. This PR proposes to integrate commands that need to resolve namespaces into the new framework. ### Why are the changes needed? This is one of the work items for moving into the new resolution framework. Resolving v1/v2 tables with the new framework will be followed up in different PRs. ### Does this PR introduce any user-facing change? No ### How was this patch tested? Existing tests should cover the changes. Closes #27095 from imback82/unresolved_ns. Authored-by: Terry Kim <yuminkim@gmail.com> Signed-off-by: Wenchen Fan <wenchen@databricks.com> |
||
|
866b7df348 |
[SPARK-30335][SQL][DOCS] Add a note first, last, collect_list and collect_set can be non-deterministic in SQL function docs as well
### What changes were proposed in this pull request? This PR adds a note first and last can be non-deterministic in SQL function docs as well. This is already documented in `functions.scala`. ### Why are the changes needed? Some people look reading SQL docs only. ### Does this PR introduce any user-facing change? No ### How was this patch tested? Jenkins will test. Closes #27099 from HyukjinKwon/SPARK-30335. Authored-by: HyukjinKwon <gurwls223@apache.org> Signed-off-by: HyukjinKwon <gurwls223@apache.org> |
||
|
da076153aa |
[SPARK-30433][SQL] Make conflict attributes resolution more scalable in ResolveReferences
### What changes were proposed in this pull request? This PR tries to make conflict attributes resolution in `ResolveReferences` more scalable by doing resolution in batch way. ### Why are the changes needed? Currently, `ResolveReferences` rule only resolves conflict attributes of one single conflict plan pair in one iteration, which can be inefficient when there're many conflicts. ### Does this PR introduce any user-facing change? No. ### How was this patch tested? Covered by existed tests. Closes #27105 from Ngone51/resolve-conflict-columns-in-batch. Authored-by: yi.wu <yi.wu@databricks.com> Signed-off-by: Wenchen Fan <wenchen@databricks.com> |
||
|
604d6799df |
[SPARK-30226][SQL] Remove withXXX functions in WriteBuilder
### What changes were proposed in this pull request? Adding a `LogicalWriteInfo` interface as suggested by cloud-fan in https://github.com/apache/spark/pull/25990#issuecomment-555132991 ### Why are the changes needed? It provides compile-time guarantees where we previously had none, which will make it harder to introduce bugs in the future. ### Does this PR introduce any user-facing change? No ### How was this patch tested? Compiles and passes tests Closes #26678 from edrevo/add-logical-write-info. Lead-authored-by: Ximo Guanter <joaquin.guantergonzalbez@telefonica.com> Co-authored-by: Ximo Guanter Signed-off-by: Wenchen Fan <wenchen@databricks.com> |
||
|
3eade744f8 |
[SPARK-29800][SQL] Rewrite non-correlated EXISTS subquery use ScalaSubquery to optimize perf
### What changes were proposed in this pull request? Current catalyst rewrite non-correlated exists subquery to BroadcastNestLoopJoin, it's performance is not good , now we rewrite non-correlated EXISTS subquery to ScalaSubquery to optimize the performance. We rewrite ``` WHERE EXISTS (SELECT A FROM TABLE B WHERE COL1 > 10) ``` to ``` WHERE (SELECT 1 FROM (SELECT A FROM TABLE B WHERE COL1 > 10) LIMIT 1) IS NOT NULL ``` to avoid build join to solve EXISTS expression. ### Why are the changes needed? Optimize EXISTS performance. ### Does this PR introduce any user-facing change? NO ### How was this patch tested? Manuel Tested Closes #26437 from AngersZhuuuu/SPARK-29800. Lead-authored-by: angerszhu <angers.zhu@gmail.com> Co-authored-by: AngersZhuuuu <angers.zhu@gmail.com> Signed-off-by: Wenchen Fan <wenchen@databricks.com> |
||
|
ebd2fd7e02 |
[SPARK-30415][SQL] Improve Readability of SQLConf Doc
### What changes were proposed in this pull request? SQLCOnf Doc updated. ### Why are the changes needed? Some doc comments were not written properly. Space was missing at many places. This patch updates the doc. ### Does this PR introduce any user-facing change? No. ### How was this patch tested? Documentation update. Closes #27091 from iRakson/SQLConfDoc. Authored-by: root1 <raksonrakesh@gmail.com> Signed-off-by: Sean Owen <srowen@gmail.com> |
||
|
be4faafee4 |
Revert "[SPARK-23264][SQL] Make INTERVAL keyword optional when ANSI enabled"
### What changes were proposed in this pull request? Revert https://github.com/apache/spark/pull/20433 . ### Why are the changes needed? According to the SQL standard, the INTERVAL prefix is required: ``` <interval literal> ::= INTERVAL [ <sign> ] <interval string> <interval qualifier> <interval string> ::= <quote> <unquoted interval string> <quote> ``` ### Does this PR introduce any user-facing change? yes, but omitting the INTERVAL prefix is a new feature in 3.0 ### How was this patch tested? existing tests Closes #27080 from cloud-fan/interval. Authored-by: Wenchen Fan <wenchen@databricks.com> Signed-off-by: Xiao Li <gatorsmile@gmail.com> |
||
|
568ad4e77a |
[SPARK-29947][SQL] Improve ResolveRelations performance
### What changes were proposed in this pull request? It is very common for a SQL query to query a table more than once. For example: ``` == Physical Plan == *(12) HashAggregate(keys=[cmn_mtrc_summ_dt#21, rev_rollup#1279, CASE WHEN (rev_rollup#1319 = rev_rollup#1279) THEN 0 ELSE 1 END#1366, CASE WHEN cast(sap_category_id#24 as decimal(10,0)) IN (5,7,23,41) THEN 0 ELSE 1 END#1367], functions=[sum(coalesce(bid_count#34, 0)), sum(coalesce(ck_trans_count#35, 0)), sum(coalesce(ended_bid_count#36, 0)), sum(coalesce(ended_lstg_count#37, 0)), sum(coalesce(ended_success_lstg_count#38, 0)), sum(coalesce(item_sold_count#39, 0)), sum(coalesce(new_lstg_count#40, 0)), sum(coalesce(gmv_us_amt#41, 0.00)), sum(coalesce(gmv_slr_lc_amt#42, 0.00)), sum(CheckOverflow((promote_precision(cast(coalesce(rvnu_insrtn_fee_us_amt#46, 0.000000) as decimal(19,6))) + promote_precision(cast(coalesce(rvnu_insrtn_crd_us_amt#50, 0.000000) as decimal(19,6)))), DecimalType(19,6), true)), sum(CheckOverflow((promote_precision(cast(coalesce(rvnu_fetr_fee_us_amt#54, 0.000000) as decimal(19,6))) + promote_precision(cast(coalesce(rvnu_fetr_crd_us_amt#58, 0.000000) as decimal(19,6)))), DecimalType(19,6), true)), sum(CheckOverflow((promote_precision(cast(coalesce(rvnu_fv_fee_us_amt#62, 0.000000) as decimal(19,6))) + promote_precision(cast(coalesce(rvnu_fv_crd_us_amt#67, 0.000000) as decimal(19,6)))), DecimalType(19,6), true)), sum(CheckOverflow((promote_precision(cast(coalesce(rvnu_othr_l_fee_us_amt#72, 0.000000) as decimal(19,6))) + promote_precision(cast(coalesce(rvnu_othr_l_crd_us_amt#76, 0.000000) as decimal(19,6)))), DecimalType(19,6), true)), sum(CheckOverflow((promote_precision(cast(coalesce(rvnu_othr_nl_fee_us_amt#80, 0.000000) as decimal(19,6))) + promote_precision(cast(coalesce(rvnu_othr_nl_crd_us_amt#84, 0.000000) as decimal(19,6)))), DecimalType(19,6), true)), sum(CheckOverflow((promote_precision(cast(coalesce(rvnu_slr_tools_fee_us_amt#88, 0.000000) as decimal(19,6))) + promote_precision(cast(coalesce(rvnu_slr_tools_crd_us_amt#92, 0.000000) as decimal(19,6)))), DecimalType(19,6), true)), sum(coalesce(rvnu_unasgnd_us_amt#96, 0.000000)), sum((coalesce(rvnu_transaction_us_amt#112, 0.0) + coalesce(rvnu_transaction_crd_us_amt#115, 0.0))), sum((coalesce(rvnu_total_us_amt#118, 0.0) + coalesce(rvnu_total_crd_us_amt#121, 0.0)))]) +- Exchange hashpartitioning(cmn_mtrc_summ_dt#21, rev_rollup#1279, CASE WHEN (rev_rollup#1319 = rev_rollup#1279) THEN 0 ELSE 1 END#1366, CASE WHEN cast(sap_category_id#24 as decimal(10,0)) IN (5,7,23,41) THEN 0 ELSE 1 END#1367, 200), true, [id=#403] +- *(11) HashAggregate(keys=[cmn_mtrc_summ_dt#21, rev_rollup#1279, CASE WHEN (rev_rollup#1319 = rev_rollup#1279) THEN 0 ELSE 1 END AS CASE WHEN (rev_rollup#1319 = rev_rollup#1279) THEN 0 ELSE 1 END#1366, CASE WHEN cast(sap_category_id#24 as decimal(10,0)) IN (5,7,23,41) THEN 0 ELSE 1 END AS CASE WHEN cast(sap_category_id#24 as decimal(10,0)) IN (5,7,23,41) THEN 0 ELSE 1 END#1367], functions=[partial_sum(coalesce(bid_count#34, 0)), partial_sum(coalesce(ck_trans_count#35, 0)), partial_sum(coalesce(ended_bid_count#36, 0)), partial_sum(coalesce(ended_lstg_count#37, 0)), partial_sum(coalesce(ended_success_lstg_count#38, 0)), partial_sum(coalesce(item_sold_count#39, 0)), partial_sum(coalesce(new_lstg_count#40, 0)), partial_sum(coalesce(gmv_us_amt#41, 0.00)), partial_sum(coalesce(gmv_slr_lc_amt#42, 0.00)), partial_sum(CheckOverflow((promote_precision(cast(coalesce(rvnu_insrtn_fee_us_amt#46, 0.000000) as decimal(19,6))) + promote_precision(cast(coalesce(rvnu_insrtn_crd_us_amt#50, 0.000000) as decimal(19,6)))), DecimalType(19,6), true)), partial_sum(CheckOverflow((promote_precision(cast(coalesce(rvnu_fetr_fee_us_amt#54, 0.000000) as decimal(19,6))) + promote_precision(cast(coalesce(rvnu_fetr_crd_us_amt#58, 0.000000) as decimal(19,6)))), DecimalType(19,6), true)), partial_sum(CheckOverflow((promote_precision(cast(coalesce(rvnu_fv_fee_us_amt#62, 0.000000) as decimal(19,6))) + promote_precision(cast(coalesce(rvnu_fv_crd_us_amt#67, 0.000000) as decimal(19,6)))), DecimalType(19,6), true)), partial_sum(CheckOverflow((promote_precision(cast(coalesce(rvnu_othr_l_fee_us_amt#72, 0.000000) as decimal(19,6))) + promote_precision(cast(coalesce(rvnu_othr_l_crd_us_amt#76, 0.000000) as decimal(19,6)))), DecimalType(19,6), true)), partial_sum(CheckOverflow((promote_precision(cast(coalesce(rvnu_othr_nl_fee_us_amt#80, 0.000000) as decimal(19,6))) + promote_precision(cast(coalesce(rvnu_othr_nl_crd_us_amt#84, 0.000000) as decimal(19,6)))), DecimalType(19,6), true)), partial_sum(CheckOverflow((promote_precision(cast(coalesce(rvnu_slr_tools_fee_us_amt#88, 0.000000) as decimal(19,6))) + promote_precision(cast(coalesce(rvnu_slr_tools_crd_us_amt#92, 0.000000) as decimal(19,6)))), DecimalType(19,6), true)), partial_sum(coalesce(rvnu_unasgnd_us_amt#96, 0.000000)), partial_sum((coalesce(rvnu_transaction_us_amt#112, 0.0) + coalesce(rvnu_transaction_crd_us_amt#115, 0.0))), partial_sum((coalesce(rvnu_total_us_amt#118, 0.0) + coalesce(rvnu_total_crd_us_amt#121, 0.0)))]) +- *(11) Project [cmn_mtrc_summ_dt#21, sap_category_id#24, bid_count#34, ck_trans_count#35, ended_bid_count#36, ended_lstg_count#37, ended_success_lstg_count#38, item_sold_count#39, new_lstg_count#40, gmv_us_amt#41, gmv_slr_lc_amt#42, rvnu_insrtn_fee_us_amt#46, rvnu_insrtn_crd_us_amt#50, rvnu_fetr_fee_us_amt#54, rvnu_fetr_crd_us_amt#58, rvnu_fv_fee_us_amt#62, rvnu_fv_crd_us_amt#67, rvnu_othr_l_fee_us_amt#72, rvnu_othr_l_crd_us_amt#76, rvnu_othr_nl_fee_us_amt#80, rvnu_othr_nl_crd_us_amt#84, rvnu_slr_tools_fee_us_amt#88, rvnu_slr_tools_crd_us_amt#92, rvnu_unasgnd_us_amt#96, ... 6 more fields] +- *(11) BroadcastHashJoin [byr_cntry_id#23], [cntry_id#1309], LeftOuter, BuildRight :- *(11) Project [cmn_mtrc_summ_dt#21, byr_cntry_id#23, sap_category_id#24, bid_count#34, ck_trans_count#35, ended_bid_count#36, ended_lstg_count#37, ended_success_lstg_count#38, item_sold_count#39, new_lstg_count#40, gmv_us_amt#41, gmv_slr_lc_amt#42, rvnu_insrtn_fee_us_amt#46, rvnu_insrtn_crd_us_amt#50, rvnu_fetr_fee_us_amt#54, rvnu_fetr_crd_us_amt#58, rvnu_fv_fee_us_amt#62, rvnu_fv_crd_us_amt#67, rvnu_othr_l_fee_us_amt#72, rvnu_othr_l_crd_us_amt#76, rvnu_othr_nl_fee_us_amt#80, rvnu_othr_nl_crd_us_amt#84, rvnu_slr_tools_fee_us_amt#88, rvnu_slr_tools_crd_us_amt#92, ... 6 more fields] : +- *(11) BroadcastHashJoin [slr_cntry_id#28], [cntry_id#1269], LeftOuter, BuildRight : :- *(11) Project [gen_attr_1#360 AS cmn_mtrc_summ_dt#21, gen_attr_5#267 AS byr_cntry_id#23, gen_attr_7#268 AS sap_category_id#24, gen_attr_15#272 AS slr_cntry_id#28, gen_attr_27#278 AS bid_count#34, gen_attr_29#279 AS ck_trans_count#35, gen_attr_31#280 AS ended_bid_count#36, gen_attr_33#282 AS ended_lstg_count#37, gen_attr_35#283 AS ended_success_lstg_count#38, gen_attr_37#284 AS item_sold_count#39, gen_attr_39#281 AS new_lstg_count#40, gen_attr_41#285 AS gmv_us_amt#41, gen_attr_43#287 AS gmv_slr_lc_amt#42, gen_attr_51#290 AS rvnu_insrtn_fee_us_amt#46, gen_attr_59#294 AS rvnu_insrtn_crd_us_amt#50, gen_attr_67#298 AS rvnu_fetr_fee_us_amt#54, gen_attr_75#302 AS rvnu_fetr_crd_us_amt#58, gen_attr_83#306 AS rvnu_fv_fee_us_amt#62, gen_attr_93#311 AS rvnu_fv_crd_us_amt#67, gen_attr_103#316 AS rvnu_othr_l_fee_us_amt#72, gen_attr_111#320 AS rvnu_othr_l_crd_us_amt#76, gen_attr_119#324 AS rvnu_othr_nl_fee_us_amt#80, gen_attr_127#328 AS rvnu_othr_nl_crd_us_amt#84, gen_attr_135#332 AS rvnu_slr_tools_fee_us_amt#88, ... 6 more fields] : : +- *(11) BroadcastHashJoin [cast(gen_attr_308#777 as decimal(20,0))], [cast(gen_attr_309#803 as decimal(20,0))], LeftOuter, BuildRight : : :- *(11) Project [gen_attr_5#267, gen_attr_7#268, gen_attr_15#272, gen_attr_27#278, gen_attr_29#279, gen_attr_31#280, gen_attr_39#281, gen_attr_33#282, gen_attr_35#283, gen_attr_37#284, gen_attr_41#285, gen_attr_43#287, gen_attr_51#290, gen_attr_59#294, gen_attr_67#298, gen_attr_75#302, gen_attr_83#306, gen_attr_93#311, gen_attr_103#316, gen_attr_111#320, gen_attr_119#324, gen_attr_127#328, gen_attr_135#332, gen_attr_143#336, ... 6 more fields] : : : +- *(11) BroadcastHashJoin [cast(gen_attr_310#674 as int)], [cast(gen_attr_311#774 as int)], LeftOuter, BuildRight : : : :- *(11) Project [gen_attr_5#267, gen_attr_7#268, gen_attr_15#272, gen_attr_27#278, gen_attr_29#279, gen_attr_31#280, gen_attr_39#281, gen_attr_33#282, gen_attr_35#283, gen_attr_37#284, gen_attr_41#285, gen_attr_43#287, gen_attr_51#290, gen_attr_59#294, gen_attr_67#298, gen_attr_75#302, gen_attr_83#306, gen_attr_93#311, gen_attr_103#316, gen_attr_111#320, gen_attr_119#324, gen_attr_127#328, gen_attr_135#332, gen_attr_143#336, ... 6 more fields] : : : : +- *(11) BroadcastHashJoin [cast(gen_attr_5#267 as decimal(20,0))], [cast(gen_attr_312#665 as decimal(20,0))], LeftOuter, BuildRight : : : : :- *(11) Project [gen_attr_5#267, gen_attr_7#268, gen_attr_15#272, gen_attr_27#278, gen_attr_29#279, gen_attr_31#280, gen_attr_39#281, gen_attr_33#282, gen_attr_35#283, gen_attr_37#284, gen_attr_41#285, gen_attr_43#287, gen_attr_51#290, gen_attr_59#294, gen_attr_67#298, gen_attr_75#302, gen_attr_83#306, gen_attr_93#311, gen_attr_103#316, gen_attr_111#320, gen_attr_119#324, gen_attr_127#328, gen_attr_135#332, gen_attr_143#336, ... 5 more fields] : : : : : +- *(11) BroadcastHashJoin [cast(gen_attr_313#565 as decimal(20,0))], [cast(gen_attr_314#591 as decimal(20,0))], LeftOuter, BuildRight : : : : : :- *(11) Project [gen_attr_5#267, gen_attr_7#268, gen_attr_15#272, gen_attr_27#278, gen_attr_29#279, gen_attr_31#280, gen_attr_39#281, gen_attr_33#282, gen_attr_35#283, gen_attr_37#284, gen_attr_41#285, gen_attr_43#287, gen_attr_51#290, gen_attr_59#294, gen_attr_67#298, gen_attr_75#302, gen_attr_83#306, gen_attr_93#311, gen_attr_103#316, gen_attr_111#320, gen_attr_119#324, gen_attr_127#328, gen_attr_135#332, gen_attr_143#336, ... 6 more fields] : : : : : : +- *(11) BroadcastHashJoin [cast(gen_attr_315#462 as int)], [cast(gen_attr_316#562 as int)], LeftOuter, BuildRight : : : : : : :- *(11) Project [gen_attr_5#267, gen_attr_7#268, gen_attr_15#272, gen_attr_27#278, gen_attr_29#279, gen_attr_31#280, gen_attr_39#281, gen_attr_33#282, gen_attr_35#283, gen_attr_37#284, gen_attr_41#285, gen_attr_43#287, gen_attr_51#290, gen_attr_59#294, gen_attr_67#298, gen_attr_75#302, gen_attr_83#306, gen_attr_93#311, gen_attr_103#316, gen_attr_111#320, gen_attr_119#324, gen_attr_127#328, gen_attr_135#332, gen_attr_143#336, ... 6 more fields] : : : : : : : +- *(11) BroadcastHashJoin [cast(gen_attr_15#272 as decimal(20,0))], [cast(gen_attr_317#453 as decimal(20,0))], LeftOuter, BuildRight : : : : : : : :- *(11) Project [gen_attr_5#267, gen_attr_7#268, gen_attr_15#272, gen_attr_27#278, gen_attr_29#279, gen_attr_31#280, gen_attr_39#281, gen_attr_33#282, gen_attr_35#283, gen_attr_37#284, gen_attr_41#285, gen_attr_43#287, gen_attr_51#290, gen_attr_59#294, gen_attr_67#298, gen_attr_75#302, gen_attr_83#306, gen_attr_93#311, gen_attr_103#316, gen_attr_111#320, gen_attr_119#324, gen_attr_127#328, gen_attr_135#332, gen_attr_143#336, ... 5 more fields] : : : : : : : : +- *(11) BroadcastHashJoin [cast(gen_attr_25#277 as decimal(20,0))], [cast(gen_attr_318#379 as decimal(20,0))], LeftOuter, BuildRight : : : : : : : : :- *(11) Project [gen_attr_5#267, gen_attr_7#268, gen_attr_15#272, gen_attr_25#277, gen_attr_27#278, gen_attr_29#279, gen_attr_31#280, gen_attr_39#281, gen_attr_33#282, gen_attr_35#283, gen_attr_37#284, gen_attr_41#285, gen_attr_43#287, gen_attr_51#290, gen_attr_59#294, gen_attr_67#298, gen_attr_75#302, gen_attr_83#306, gen_attr_93#311, gen_attr_103#316, gen_attr_111#320, gen_attr_119#324, gen_attr_127#328, gen_attr_135#332, ... 6 more fields] : : : : : : : : : +- *(11) BroadcastHashJoin [cast(gen_attr_23#276 as decimal(20,0))], [cast(gen_attr_319#367 as decimal(20,0))], LeftOuter, BuildRight : : : : : : : : : :- *(11) Project [byr_cntry_id#1169 AS gen_attr_5#267, sap_category_id#1170 AS gen_attr_7#268, slr_cntry_id#1174 AS gen_attr_15#272, lstg_curncy_id#1178 AS gen_attr_23#276, blng_curncy_id#1179 AS gen_attr_25#277, bid_count#1180 AS gen_attr_27#278, ck_trans_count#1181 AS gen_attr_29#279, ended_bid_count#1182 AS gen_attr_31#280, new_lstg_count#1183 AS gen_attr_39#281, ended_lstg_count#1184 AS gen_attr_33#282, ended_success_lstg_count#1185 AS gen_attr_35#283, item_sold_count#1186 AS gen_attr_37#284, gmv_us_amt#1187 AS gen_attr_41#285, gmv_slr_lc_amt#1189 AS gen_attr_43#287, rvnu_insrtn_fee_us_amt#1192 AS gen_attr_51#290, rvnu_insrtn_crd_us_amt#1196 AS gen_attr_59#294, rvnu_fetr_fee_us_amt#1200 AS gen_attr_67#298, rvnu_fetr_crd_us_amt#1204 AS gen_attr_75#302, rvnu_fv_fee_us_amt#1208 AS gen_attr_83#306, rvnu_fv_crd_us_amt#1213 AS gen_attr_93#311, rvnu_othr_l_fee_us_amt#1218 AS gen_attr_103#316, rvnu_othr_l_crd_us_amt#1222 AS gen_attr_111#320, rvnu_othr_nl_fee_us_amt#1226 AS gen_attr_119#324, rvnu_othr_nl_crd_us_amt#1230 AS gen_attr_127#328, ... 7 more fields] : : : : : : : : : : +- *(11) ColumnarToRow : : : : : : : : : : +- FileScan parquet default.big_table1[byr_cntry_id#1169,sap_category_id#1170,slr_cntry_id#1174,lstg_curncy_id#1178,blng_curncy_id#1179,bid_count#1180,ck_trans_count#1181,ended_bid_count#1182,new_lstg_count#1183,ended_lstg_count#1184,ended_success_lstg_count#1185,item_sold_count#1186,gmv_us_amt#1187,gmv_slr_lc_amt#1189,rvnu_insrtn_fee_us_amt#1192,rvnu_insrtn_crd_us_amt#1196,rvnu_fetr_fee_us_amt#1200,rvnu_fetr_crd_us_amt#1204,rvnu_fv_fee_us_amt#1208,rvnu_fv_crd_us_amt#1213,rvnu_othr_l_fee_us_amt#1218,rvnu_othr_l_crd_us_amt#1222,rvnu_othr_nl_fee_us_amt#1226,rvnu_othr_nl_crd_us_amt#1230,... 7 more fields] Batched: true, DataFilters: [], Format: Parquet, Location: PrunedInMemoryFileIndex[], PartitionFilters: [isnotnull(cmn_mtrc_summ_dt#1262), (cmn_mtrc_summ_dt#1262 >= 18078), (cmn_mtrc_summ_dt#1262 <= 18..., PushedFilters: [], ReadSchema: struct<byr_cntry_id:decimal(4,0),sap_category_id:decimal(9,0),slr_cntry_id:decimal(4,0),lstg_curn... : : : : : : : : : +- BroadcastExchange HashedRelationBroadcastMode(List(cast(input[0, decimal(9,0), true] as decimal(20,0)))), [id=#288] : : : : : : : : : +- *(1) Project [CURNCY_ID#1263 AS gen_attr_319#367] : : : : : : : : : +- *(1) Filter isnotnull(CURNCY_ID#1263) : : : : : : : : : +- *(1) ColumnarToRow : : : : : : : : : +- FileScan parquet default.small_table1[CURNCY_ID#1263] Batched: true, DataFilters: [isnotnull(CURNCY_ID#1263)], Format: Parquet, Location: InMemoryFileIndex[file:/user/hive/warehouse/small_table1], PartitionFilters: [], PushedFilters: [IsNotNull(CURNCY_ID)], ReadSchema: struct<CURNCY_ID:decimal(9,0)>, SelectedBucketsCount: 1 out of 1 : : : : : : : : +- BroadcastExchange HashedRelationBroadcastMode(List(cast(input[0, decimal(9,0), true] as decimal(20,0)))), [id=#297] : : : : : : : : +- *(2) Project [CURNCY_ID#1263 AS gen_attr_318#379] : : : : : : : : +- *(2) Filter isnotnull(CURNCY_ID#1263) : : : : : : : : +- *(2) ColumnarToRow : : : : : : : : +- FileScan parquet default.small_table1[CURNCY_ID#1263] Batched: true, DataFilters: [isnotnull(CURNCY_ID#1263)], Format: Parquet, Location: InMemoryFileIndex[file:/user/hive/warehouse/small_table1], PartitionFilters: [], PushedFilters: [IsNotNull(CURNCY_ID)], ReadSchema: struct<CURNCY_ID:decimal(9,0)>, SelectedBucketsCount: 1 out of 1 : : : : : : : +- BroadcastExchange HashedRelationBroadcastMode(List(cast(input[0, decimal(4,0), true] as decimal(20,0)))), [id=#306] : : : : : : : +- *(3) Project [cntry_id#1269 AS gen_attr_317#453, rev_rollup_id#1278 AS gen_attr_315#462] : : : : : : : +- *(3) Filter isnotnull(cntry_id#1269) : : : : : : : +- *(3) ColumnarToRow : : : : : : : +- FileScan parquet default.small_table2[cntry_id#1269,rev_rollup_id#1278] Batched: true, DataFilters: [isnotnull(cntry_id#1269)], Format: Parquet, Location: InMemoryFileIndex[file:/user/hive/warehouse/small_table2], PartitionFilters: [], PushedFilters: [IsNotNull(cntry_id)], ReadSchema: struct<cntry_id:decimal(4,0),rev_rollup_id:smallint> : : : : : : +- BroadcastExchange HashedRelationBroadcastMode(List(cast(cast(input[0, smallint, true] as int) as bigint))), [id=#315] : : : : : : +- *(4) Project [rev_rollup_id#1286 AS gen_attr_316#562, curncy_id#1289 AS gen_attr_313#565] : : : : : : +- *(4) Filter isnotnull(rev_rollup_id#1286) : : : : : : +- *(4) ColumnarToRow : : : : : : +- FileScan parquet default.small_table3[rev_rollup_id#1286,curncy_id#1289] Batched: true, DataFilters: [isnotnull(rev_rollup_id#1286)], Format: Parquet, Location: InMemoryFileIndex[file:/user/hive/warehouse/small_table3], PartitionFilters: [], PushedFilters: [IsNotNull(rev_rollup_id)], ReadSchema: struct<rev_rollup_id:smallint,curncy_id:decimal(4,0)> : : : : : +- BroadcastExchange HashedRelationBroadcastMode(List(cast(input[0, decimal(9,0), true] as decimal(20,0)))), [id=#324] : : : : : +- *(5) Project [CURNCY_ID#1263 AS gen_attr_314#591] : : : : : +- *(5) Filter isnotnull(CURNCY_ID#1263) : : : : : +- *(5) ColumnarToRow : : : : : +- FileScan parquet default.small_table1[CURNCY_ID#1263] Batched: true, DataFilters: [isnotnull(CURNCY_ID#1263)], Format: Parquet, Location: InMemoryFileIndex[file:/user/hive/warehouse/small_table1], PartitionFilters: [], PushedFilters: [IsNotNull(CURNCY_ID)], ReadSchema: struct<CURNCY_ID:decimal(9,0)>, SelectedBucketsCount: 1 out of 1 : : : : +- BroadcastExchange HashedRelationBroadcastMode(List(cast(input[0, decimal(4,0), true] as decimal(20,0)))), [id=#333] : : : : +- *(6) Project [cntry_id#1269 AS gen_attr_312#665, rev_rollup_id#1278 AS gen_attr_310#674] : : : : +- *(6) Filter isnotnull(cntry_id#1269) : : : : +- *(6) ColumnarToRow : : : : +- FileScan parquet default.small_table2[cntry_id#1269,rev_rollup_id#1278] Batched: true, DataFilters: [isnotnull(cntry_id#1269)], Format: Parquet, Location: InMemoryFileIndex[file:/user/hive/warehouse/small_table2], PartitionFilters: [], PushedFilters: [IsNotNull(cntry_id)], ReadSchema: struct<cntry_id:decimal(4,0),rev_rollup_id:smallint> : : : +- BroadcastExchange HashedRelationBroadcastMode(List(cast(cast(input[0, smallint, true] as int) as bigint))), [id=#342] : : : +- *(7) Project [rev_rollup_id#1286 AS gen_attr_311#774, curncy_id#1289 AS gen_attr_308#777] : : : +- *(7) Filter isnotnull(rev_rollup_id#1286) : : : +- *(7) ColumnarToRow : : : +- FileScan parquet default.small_table3[rev_rollup_id#1286,curncy_id#1289] Batched: true, DataFilters: [isnotnull(rev_rollup_id#1286)], Format: Parquet, Location: InMemoryFileIndex[file:/user/hive/warehouse/small_table3], PartitionFilters: [], PushedFilters: [IsNotNull(rev_rollup_id)], ReadSchema: struct<rev_rollup_id:smallint,curncy_id:decimal(4,0)> : : +- BroadcastExchange HashedRelationBroadcastMode(List(cast(input[0, decimal(9,0), true] as decimal(20,0)))), [id=#351] : : +- *(8) Project [CURNCY_ID#1263 AS gen_attr_309#803] : : +- *(8) Filter isnotnull(CURNCY_ID#1263) : : +- *(8) ColumnarToRow : : +- FileScan parquet default.small_table1[CURNCY_ID#1263] Batched: true, DataFilters: [isnotnull(CURNCY_ID#1263)], Format: Parquet, Location: InMemoryFileIndex[file:/user/hive/warehouse/small_table1], PartitionFilters: [], PushedFilters: [IsNotNull(CURNCY_ID)], ReadSchema: struct<CURNCY_ID:decimal(9,0)>, SelectedBucketsCount: 1 out of 1 : +- BroadcastExchange HashedRelationBroadcastMode(List(input[0, decimal(4,0), true])), [id=#360] : +- *(9) Project [cntry_id#1269, rev_rollup#1279] : +- *(9) Filter isnotnull(cntry_id#1269) : +- *(9) ColumnarToRow : +- FileScan parquet default.small_table2[cntry_id#1269,rev_rollup#1279] Batched: true, DataFilters: [isnotnull(cntry_id#1269)], Format: Parquet, Location: InMemoryFileIndex[file:/user/hive/warehouse/small_table2], PartitionFilters: [], PushedFilters: [IsNotNull(cntry_id)], ReadSchema: struct<cntry_id:decimal(4,0),rev_rollup:string> +- ReusedExchange [cntry_id#1309, rev_rollup#1319], BroadcastExchange HashedRelationBroadcastMode(List(input[0, decimal(4,0), true])), [id=#360] ``` This PR try to improve `ResolveTables` and `ResolveRelations` performance by reducing the connection times to Hive Metastore Server in such case. ### Why are the changes needed? 1. Reduce the connection times to Hive Metastore Server. 2. Improve `ResolveTables` and `ResolveRelations` performance. ### Does this PR introduce any user-facing change? No. ### How was this patch tested? manual test. After [SPARK-29606](https://issues.apache.org/jira/browse/SPARK-29606) and before this PR: ``` === Metrics of Analyzer/Optimizer Rules === Total number of runs: 9323 Total time: 2.687441263 seconds Rule Effective Time / Total Time Effective Runs / Total Runs org.apache.spark.sql.catalyst.analysis.Analyzer$ResolveRelations 929173767 / 930133504 2 / 18 org.apache.spark.sql.catalyst.analysis.Analyzer$ResolveTables 0 / 383363402 0 / 18 org.apache.spark.sql.catalyst.optimizer.EliminateOuterJoin 0 / 99433540 0 / 4 org.apache.spark.sql.catalyst.analysis.DecimalPrecision 41809394 / 83727901 2 / 18 org.apache.spark.sql.execution.datasources.PruneFileSourcePartitions 71372977 / 71372977 1 / 1 org.apache.spark.sql.catalyst.analysis.TypeCoercion$ImplicitTypeCasts 0 / 59071933 0 / 18 org.apache.spark.sql.catalyst.analysis.Analyzer$ResolveReferences 37858325 / 58471776 5 / 18 org.apache.spark.sql.catalyst.analysis.TypeCoercion$PromoteStrings 20889892 / 53229016 1 / 18 org.apache.spark.sql.catalyst.analysis.TypeCoercion$FunctionArgumentConversion 23428968 / 50890815 1 / 18 org.apache.spark.sql.catalyst.analysis.TypeCoercion$InConversion 23230666 / 49182607 1 / 18 org.apache.spark.sql.catalyst.analysis.Analyzer$ExtractGenerator 0 / 43638350 0 / 18 org.apache.spark.sql.catalyst.optimizer.ColumnPruning 17194844 / 42530885 1 / 6 ``` After [SPARK-29606](https://issues.apache.org/jira/browse/SPARK-29606) and after this PR: ``` === Metrics of Analyzer/Optimizer Rules === Total number of runs: 9323 Total time: 2.163765869 seconds Rule Effective Time / Total Time Effective Runs / Total Runs org.apache.spark.sql.catalyst.analysis.Analyzer$ResolveRelations 658905353 / 659829383 2 / 18 org.apache.spark.sql.catalyst.analysis.Analyzer$ResolveTables 0 / 220708715 0 / 18 org.apache.spark.sql.catalyst.optimizer.EliminateOuterJoin 0 / 99606816 0 / 4 org.apache.spark.sql.catalyst.analysis.DecimalPrecision 39616060 / 78215752 2 / 18 org.apache.spark.sql.catalyst.analysis.Analyzer$ResolveReferences 36706549 / 54917789 5 / 18 org.apache.spark.sql.execution.datasources.PruneFileSourcePartitions 53561921 / 53561921 1 / 1 org.apache.spark.sql.catalyst.analysis.TypeCoercion$ImplicitTypeCasts 0 / 52329678 0 / 18 org.apache.spark.sql.catalyst.analysis.TypeCoercion$PromoteStrings 20945755 / 49695998 1 / 18 org.apache.spark.sql.catalyst.analysis.TypeCoercion$FunctionArgumentConversion 20872241 / 46740145 1 / 18 org.apache.spark.sql.catalyst.analysis.TypeCoercion$InConversion 19780298 / 44327227 1 / 18 org.apache.spark.sql.catalyst.analysis.Analyzer$ExtractGenerator 0 / 42312023 0 / 18 org.apache.spark.sql.catalyst.optimizer.ColumnPruning 17197393 / 39501424 1 / 6 ``` Closes #26589 from wangyum/SPARK-29947. Authored-by: Yuming Wang <yumwang@ebay.com> Signed-off-by: Wenchen Fan <wenchen@databricks.com> |
||
|
e38964c442 |
[SPARK-29768][SQL][FOLLOW-UP] Improve handling non-deterministic filter of ScanOperation
### What changes were proposed in this pull request? 1. For `ScanOperation`, if it collects more than one filters, then all filters must be deterministic. And filter can be non-deterministic iff there's only one collected filter. 2. `FileSourceStrategy` should filter out non-deterministic filter, as it will hit haven't initialized exception if it's a partition related filter. ### Why are the changes needed? Strictly follow `CombineFilters`'s behavior which doesn't allow combine two filters where non-deterministic predicates exist. And avoid hitting exception for file source. ### Does this PR introduce any user-facing change? No ### How was this patch tested? Test exists. Closes #27073 from Ngone51/SPARK-29768-FOLLOWUP. Authored-by: yi.wu <yi.wu@databricks.com> Signed-off-by: Wenchen Fan <wenchen@databricks.com> |
||
|
c49388a484 |
[SPARK-30214][SQL] A new framework to resolve v2 commands
### What changes were proposed in this pull request? Currently, we have a v2 adapter for v1 catalog (`V2SessionCatalog`), all the table/namespace commands can be implemented via v2 APIs. Usually, a command needs to know which catalog it needs to operate, but different commands have different requirements about what to resolve. A few examples: - `DROP NAMESPACE`: only need to know the name of the namespace. - `DESC NAMESPACE`: need to lookup the namespace and get metadata, but is done during execution - `DROP TABLE`: need to do lookup and make sure it's a table not (temp) view. - `DESC TABLE`: need to lookup the table and get metadata. For namespaces, the analyzer only needs to find the catalog and the namespace name. The command can do lookup during execution if needed. For tables, mostly commands need the analyzer to do lookup. Note that, table and namespace have a difference: `DESC NAMESPACE testcat` works and describes the root namespace under `testcat`, while `DESC TABLE testcat` fails if there is no table `testcat` under the current catalog. It's because namespaces can be named [], but tables can't. The commands should explicitly specify it needs to operate on namespace or table. In this Pull Request, we introduce a new framework to resolve v2 commands: 1. parser creates logical plans or commands with `UnresolvedNamespace`/`UnresolvedTable`/`UnresolvedView`/`UnresolvedRelation`. (CREATE TABLE still keeps Seq[String], as it doesn't need to look up relations) 2. analyzer converts 2.1 `UnresolvedNamespace` to `ResolvesNamespace` (contains catalog and namespace identifier) 2.2 `UnresolvedTable` to `ResolvedTable` (contains catalog, identifier and `Table`) 2.3 `UnresolvedView` to `ResolvedView` (will be added later when we migrate view commands) 2.4 `UnresolvedRelation` to relation. 3. an extra analyzer rule to match commands with `V1Table` and converts them to corresponding v1 commands. This will be added later when we migrate existing commands 4. planner matches commands and converts them to the corresponding physical nodes. We also introduce brand new v2 commands - the `comment` syntaxes to illustrate how to work with the newly added framework. ```sql COMMENT ON (DATABASE|SCHEMA|NAMESPACE) ... IS ... COMMENT ON TABLE ... IS ... ``` Details about the `comment` syntaxes: As the new design of catalog v2, some properties become reserved, e.g. `location`, `comment`. We are going to disable setting reserved properties by dbproperties or tblproperites directly to avoid confliction with their related subClause or specific commands. They are the best practices from PostgreSQL and presto. https://www.postgresql.org/docs/12/sql-comment.html https://prestosql.io/docs/current/sql/comment.html Mostly, the basic thoughts of the new framework came from the discussions bellow with cloud-fan, https://github.com/apache/spark/pull/26847#issuecomment-564510061, ### Why are the changes needed? To make it easier to add new v2 commands, and easier to unify the table relation behavior. ### Does this PR introduce any user-facing change? yes, add new syntax ### How was this patch tested? add uts. Closes #26847 from yaooqinn/SPARK-30214. Authored-by: Kent Yao <yaooqinn@hotmail.com> Signed-off-by: Wenchen Fan <wenchen@databricks.com> |
||
|
a469976e6e |
[SPARK-29930][SQL][FOLLOW-UP] Allow only default value to be set for removed SQL configs
### What changes were proposed in this pull request? In the PR, I propose to throw `AnalysisException` when a removed SQL config is set to non-default value. The following SQL configs removed by #26559 are marked as removed: 1. `spark.sql.fromJsonForceNullableSchema` 2. `spark.sql.legacy.compareDateTimestampInTimestamp` 3. `spark.sql.legacy.allowCreatingManagedTableUsingNonemptyLocation` ### Why are the changes needed? To improve user experience with Spark SQL by notifying of removed SQL configs used by users. ### Does this PR introduce any user-facing change? Yes, before the `set` command was silently ignored: ```sql spark-sql> set spark.sql.fromJsonForceNullableSchema=false; spark.sql.fromJsonForceNullableSchema false ``` after the exception should be raised: ```sql spark-sql> set spark.sql.fromJsonForceNullableSchema=false; Error in query: The SQL config 'spark.sql.fromJsonForceNullableSchema' was removed in the version 3.0.0. It was removed to prevent errors like SPARK-23173 for non-default value.; ``` ### How was this patch tested? Added new tests into `SQLConfSuite` for both cases when removed SQL configs are set to default and non-default values. Closes #27057 from MaxGekk/remove-sql-configs-followup. Authored-by: Maxim Gekk <max.gekk@gmail.com> Signed-off-by: HyukjinKwon <gurwls223@apache.org> |
||
|
e04309cb1f |
[SPARK-30341][SQL] Overflow check for interval arithmetic operations
### What changes were proposed in this pull request? 1. For the interval arithmetic functions, e.g. `add`/`subtract`/`negative`/`multiply`/`divide`, enable overflow check when `ANSI` is on. 2. For `multiply`/`divide`, throw an exception when an overflow happens in spite of `ANSI` is on/off. 3. `add`/`subtract`/`negative` stay the same for backward compatibility. 4. `divide` by 0 throws ArithmeticException whether `ANSI` or not as same as numerics. 5. These behaviors fit the numeric type operations fully when ANSI is on. 6. These behaviors fit the numeric type operations fully when ANSI is off, except 2 and 4. ### Why are the changes needed? 1. bug fix 2. `ANSI` support ### Does this PR introduce any user-facing change? When `ANSI` is on, interval `add`/`subtract`/`negative`/`multiply`/`divide` will overflow if any field overflows ### How was this patch tested? add unit tests Closes #26995 from yaooqinn/SPARK-30341. Authored-by: Kent Yao <yaooqinn@hotmail.com> Signed-off-by: Wenchen Fan <wenchen@databricks.com> |
||
|
68260f5297 |
revert [SPARK-29680][SQL] Remove ALTER TABLE CHANGE COLUMN syntax
### What changes were proposed in this pull request? Revert https://github.com/apache/spark/pull/26338 , as the syntax is actually the [hive style ALTER COLUMN](https://cwiki.apache.org/confluence/display/Hive/LanguageManual+DDL#LanguageManualDDL-ChangeColumnName/Type/Position/Comment). This PR brings it back, and make it support multi-catalog: 1. renaming is not allowed as `AlterTableAlterColumnStatement` can't do renaming. 2. column name should be multi-part ### Why are the changes needed? to not break hive compatibility. ### Does this PR introduce any user-facing change? no, as the removal was merged in 3.0. ### How was this patch tested? new parser tests Closes #27076 from cloud-fan/alter. Authored-by: Wenchen Fan <wenchen@databricks.com> Signed-off-by: Wenchen Fan <wenchen@databricks.com> |
||
|
1743d5be7f |
[SPARK-30284][SQL] CREATE VIEW should keep the current catalog and namespace
### What changes were proposed in this pull request? Update CREATE VIEW command to store the current catalog and namespace instead of current database in view metadata. Also update analyzer to leverage the catalog and namespace in view metastore to resolve relations inside views. Note that, this PR still keeps the way we resolve views, by recursively calling Analyzer. This is necessary because view text may contain CTE, window spec, etc. which needs rules outside of the main resolution batch (e.g. `CTESubstitution`) ### Why are the changes needed? To resolve relations inside view correctly. ### Does this PR introduce any user-facing change? Yes, fix a bug. Now tables referred by a view can be resolved correctly even if the current catalog/namespace has been updated. ### How was this patch tested? a new test Closes #26923 from cloud-fan/view. Authored-by: Wenchen Fan <wenchen@databricks.com> Signed-off-by: Wenchen Fan <wenchen@databricks.com> |
||
|
83d289eef4 |
[SPARK-27638][SQL][FOLLOW-UP] Format config name to follow the other boolean conf naming convention
### What changes were proposed in this pull request? Change config name from `spark.sql.legacy.typeCoercion.datetimeToString` to `spark.sql.legacy.typeCoercion.datetimeToString.enabled`. ### Why are the changes needed? To follow the other boolean conf naming convention. ### Does this PR introduce any user-facing change? No, it's newly added in Spark 3.0. ### How was this patch tested? Pass Jenkins Closes #27065 from Ngone51/SPARK-27638-FOLLOWUP. Authored-by: yi.wu <yi.wu@databricks.com> Signed-off-by: HyukjinKwon <gurwls223@apache.org> |
||
|
90794b617c |
[SPARK-27871][SQL][FOLLOW-UP] Format config name to follow the other boolean conf naming convention
### What changes were proposed in this pull request? Change config name from `spark.sql.optimizer.reassignLambdaVariableID` to `spark.sql.optimizer.reassignLambdaVariableID.enabled`. ### Why are the changes needed? To follow the other boolean conf naming convention. ### Does this PR introduce any user-facing change? No, it's newly added in Spark 3.0. ### How was this patch tested? Pass Jenkins. Closes #27063 from Ngone51/SPARK-27871-FOLLOWUP. Authored-by: yi.wu <yi.wu@databricks.com> Signed-off-by: HyukjinKwon <gurwls223@apache.org> |
||
|
7079e871a7 |
[SPARK-30185][SQL] Implement Dataset.tail API
### What changes were proposed in this pull request? This PR proposes a `tail` API. Namely, as below: ```scala scala> spark.range(10).head(5) res1: Array[Long] = Array(0, 1, 2, 3, 4) scala> spark.range(10).tail(5) res2: Array[Long] = Array(5, 6, 7, 8, 9) ``` Implementation details will be similar with `head` but it will be reversed: 1. Run the job against the last partition and collect rows. If this is enough, return as is. 2. If this is not enough, calculate the number of partitions to select more based upon `spark.sql.limit.scaleUpFactor` 3. Run more jobs against more partitions (in a reversed order compared to head) as many as the number calculated from 2. 4. Go to 2. **Note that**, we don't guarantee the natural order in DataFrame in general - there are cases when it's deterministic and when it's not. We probably should write down this as a caveat separately. ### Why are the changes needed? Many other systems support the way to take data from the end, for instance, pandas[1] and Python[2][3]. Scala collections APIs also have head and tail On the other hand, in Spark, we only provide a way to take data from the start (e.g., DataFrame.head). This has been requested multiple times here and there in Spark user mailing list[4], StackOverFlow[5][6], JIRA[7] and other third party projects such as Koalas[8]. In addition, this missing API seems explicitly mentioned in comparison to another system[9] time to time. It seems we're missing non-trivial use case in Spark and this motivated me to propose this API. [1] https://pandas.pydata.org/pandas-docs/stable/reference/api/pandas.DataFrame.tail.html?highlight=tail#pandas.DataFrame.tail [2] https://stackoverflow.com/questions/10532473/head-and-tail-in-one-line [3] https://stackoverflow.com/questions/646644/how-to-get-last-items-of-a-list-in-python [4] http://apache-spark-user-list.1001560.n3.nabble.com/RDD-tail-td4217.html [5] https://stackoverflow.com/questions/39544796/how-to-select-last-row-and-also-how-to-access-pyspark-dataframe-by-index [6] https://stackoverflow.com/questions/45406762/how-to-get-the-last-row-from-dataframe [7] https://issues.apache.org/jira/browse/SPARK-26433 [8] https://github.com/databricks/koalas/issues/343 [9] https://medium.com/chris_bour/6-differences-between-pandas-and-spark-dataframes-1380cec394d2 ### Does this PR introduce any user-facing change? No, (new API) ### How was this patch tested? Unit tests were added and manually tested. Closes #26809 from HyukjinKwon/wip-tail. Authored-by: HyukjinKwon <gurwls223@apache.org> Signed-off-by: HyukjinKwon <gurwls223@apache.org> |
||
|
a90ad5bf2a |
[SPARK-30370][SQL] Update SqlBase.g4 to combine namespace and database tokens
### What changes were proposed in this pull request? In `SqlBase.g4`, `database` is defined as ``` database : DATABASE | SCHEMA; ``` and it is being used as `(database | NAMESPACE)` in many places. This PR proposes to define the following and use it as discussed in https://github.com/apache/spark/pull/26847/files#r359754778: ``` namespace : NAMESPACE | DATABASE | SCHEMA; ``` ### Why are the changes needed? To simplify the grammar. ### Does this PR introduce any user-facing change? No. ### How was this patch tested? There is no change in the actual grammar, so the existing tests should be sufficient. Closes #27027 from imback82/sqlbase_namespace. Authored-by: Terry Kim <yuminkim@gmail.com> Signed-off-by: Wenchen Fan <wenchen@databricks.com> |
||
|
919d551ddb |
Revert "[SPARK-29390][SQL] Add the justify_days(), justify_hours() and justif_interval() functions"
This reverts commit
|
||
|
f0bf2eb006 |
[SPARK-30356][SQL] Codegen support for the function str_to_map
### What changes were proposed in this pull request? `str_to_map ` has not implemented with codegen support, which prevents a query that contains this expression from being whole stage codegen-ed. This PR removes `CodegenFallBack` from `StringToMap`, add the codegen support for it. ### Why are the changes needed? improve codegen coverage and gain better perfomance ### Does this PR introduce any user-facing change? no ### How was this patch tested? 1. pass ComplexTypeSuite 2. manually review generated code ```java -- !query 12 explain codegen select v, str_to_map(v) from values ('abc🅰️a,:'), (null), (''), ('1:2') t(v) -- !query 12 schema struct<plan:string> -- !query 12 output Found 1 WholeStageCodegen subtrees. == Subtree 1 / 1 (maxMethodCodeSize:511; maxConstantPoolSize:188(0.29% used); numInnerClasses:0) == *Project [v#x, str_to_map(v#x, ,, :) AS str_to_map(v, ,, :)#x] +- *LocalTableScan [v#x] Generated code: /* 001 */ public Object generate(Object[] references) { /* 002 */ return new GeneratedIteratorForCodegenStage1(references); /* 003 */ } /* 004 */ /* 005 */ // codegenStageId=1 /* 006 */ final class GeneratedIteratorForCodegenStage1 extends org.apache.spark.sql.execution.BufferedRowIterator { /* 007 */ private Object[] references; /* 008 */ private scala.collection.Iterator[] inputs; /* 009 */ private scala.collection.Iterator localtablescan_input_0; /* 010 */ private org.apache.spark.sql.catalyst.expressions.codegen.UnsafeRowWriter[] project_mutableStateArray_0 = new org.apache.spark.sql.catalyst.expressions.codegen.UnsafeRowWriter[1]; /* 011 */ private org.apache.spark.sql.catalyst.expressions.codegen.UnsafeArrayWriter[] project_mutableStateArray_1 = new org.apache.spark.sql.catalyst.expressions.codegen.UnsafeArrayWriter[2]; /* 012 */ /* 013 */ public GeneratedIteratorForCodegenStage1(Object[] references) { /* 014 */ this.references = references; /* 015 */ } /* 016 */ /* 017 */ public void init(int index, scala.collection.Iterator[] inputs) { /* 018 */ partitionIndex = index; /* 019 */ this.inputs = inputs; /* 020 */ localtablescan_input_0 = inputs[0]; /* 021 */ project_mutableStateArray_0[0] = new org.apache.spark.sql.catalyst.expressions.codegen.UnsafeRowWriter(2, 64); /* 022 */ project_mutableStateArray_1[0] = new org.apache.spark.sql.catalyst.expressions.codegen.UnsafeArrayWriter(project_mutableStateArray_0[0], 8); /* 023 */ project_mutableStateArray_1[1] = new org.apache.spark.sql.catalyst.expressions.codegen.UnsafeArrayWriter(project_mutableStateArray_0[0], 8); /* 024 */ /* 025 */ } /* 026 */ /* 027 */ private void project_doConsume_0(InternalRow localtablescan_row_0, UTF8String project_expr_0_0, boolean project_exprIsNull_0_0) throws java.io.IOException { /* 028 */ boolean project_isNull_1 = true; /* 029 */ MapData project_value_1 = null; /* 030 */ /* 031 */ if (!project_exprIsNull_0_0) { /* 032 */ project_isNull_1 = false; // resultCode could change nullability. /* 033 */ /* 034 */ int project_i_0 = 0; /* 035 */ UTF8String[] project_kvs_0 = project_expr_0_0.split(((UTF8String) references[2] /* literal */), -1); /* 036 */ while (project_i_0 < project_kvs_0.length) { /* 037 */ UTF8String[] kv = project_kvs_0[project_i_0].split(((UTF8String) references[3] /* literal */), 2); /* 038 */ UTF8String key = kv[0]; /* 039 */ UTF8String value = null; /* 040 */ if (kv.length == 2) { /* 041 */ value = kv[1]; /* 042 */ } /* 043 */ ((org.apache.spark.sql.catalyst.util.ArrayBasedMapBuilder) references[1] /* mapBuilder */).put(key, value); /* 044 */ project_i_0++; /* 045 */ } /* 046 */ project_value_1 = ((org.apache.spark.sql.catalyst.util.ArrayBasedMapBuilder) references[1] /* mapBuilder */).build(); /* 047 */ /* 048 */ } /* 049 */ project_mutableStateArray_0[0].reset(); /* 050 */ /* 051 */ project_mutableStateArray_0[0].zeroOutNullBytes(); /* 052 */ /* 053 */ if (project_exprIsNull_0_0) { /* 054 */ project_mutableStateArray_0[0].setNullAt(0); /* 055 */ } else { /* 056 */ project_mutableStateArray_0[0].write(0, project_expr_0_0); /* 057 */ } /* 058 */ /* 059 */ if (project_isNull_1) { /* 060 */ project_mutableStateArray_0[0].setNullAt(1); /* 061 */ } else { /* 062 */ final MapData project_tmpInput_0 = project_value_1; /* 063 */ if (project_tmpInput_0 instanceof UnsafeMapData) { /* 064 */ project_mutableStateArray_0[0].write(1, (UnsafeMapData) project_tmpInput_0); /* 065 */ } else { /* 066 */ // Remember the current cursor so that we can calculate how many bytes are /* 067 */ // written later. /* 068 */ final int project_previousCursor_0 = project_mutableStateArray_0[0].cursor(); /* 069 */ /* 070 */ // preserve 8 bytes to write the key array numBytes later. /* 071 */ project_mutableStateArray_0[0].grow(8); /* 072 */ project_mutableStateArray_0[0].increaseCursor(8); /* 073 */ /* 074 */ // Remember the current cursor so that we can write numBytes of key array later. /* 075 */ final int project_tmpCursor_0 = project_mutableStateArray_0[0].cursor(); /* 076 */ /* 077 */ final ArrayData project_tmpInput_1 = project_tmpInput_0.keyArray(); /* 078 */ if (project_tmpInput_1 instanceof UnsafeArrayData) { /* 079 */ project_mutableStateArray_0[0].write((UnsafeArrayData) project_tmpInput_1); /* 080 */ } else { /* 081 */ final int project_numElements_0 = project_tmpInput_1.numElements(); /* 082 */ project_mutableStateArray_1[0].initialize(project_numElements_0); /* 083 */ /* 084 */ for (int project_index_0 = 0; project_index_0 < project_numElements_0; project_index_0++) { /* 085 */ project_mutableStateArray_1[0].write(project_index_0, project_tmpInput_1.getUTF8String(project_index_0)); /* 086 */ } /* 087 */ } /* 088 */ /* 089 */ // Write the numBytes of key array into the first 8 bytes. /* 090 */ Platform.putLong( /* 091 */ project_mutableStateArray_0[0].getBuffer(), /* 092 */ project_tmpCursor_0 - 8, /* 093 */ project_mutableStateArray_0[0].cursor() - project_tmpCursor_0); /* 094 */ /* 095 */ final ArrayData project_tmpInput_2 = project_tmpInput_0.valueArray(); /* 096 */ if (project_tmpInput_2 instanceof UnsafeArrayData) { /* 097 */ project_mutableStateArray_0[0].write((UnsafeArrayData) project_tmpInput_2); /* 098 */ } else { /* 099 */ final int project_numElements_1 = project_tmpInput_2.numElements(); /* 100 */ project_mutableStateArray_1[1].initialize(project_numElements_1); /* 101 */ /* 102 */ for (int project_index_1 = 0; project_index_1 < project_numElements_1; project_index_1++) { /* 103 */ if (project_tmpInput_2.isNullAt(project_index_1)) { /* 104 */ project_mutableStateArray_1[1].setNull8Bytes(project_index_1); /* 105 */ } else { /* 106 */ project_mutableStateArray_1[1].write(project_index_1, project_tmpInput_2.getUTF8String(project_index_1)); /* 107 */ } /* 108 */ /* 109 */ } /* 110 */ } /* 111 */ /* 112 */ project_mutableStateArray_0[0].setOffsetAndSizeFromPreviousCursor(1, project_previousCursor_0); /* 113 */ } /* 114 */ } /* 115 */ append((project_mutableStateArray_0[0].getRow())); /* 116 */ /* 117 */ } /* 118 */ /* 119 */ protected void processNext() throws java.io.IOException { /* 120 */ while ( localtablescan_input_0.hasNext()) { /* 121 */ InternalRow localtablescan_row_0 = (InternalRow) localtablescan_input_0.next(); /* 122 */ ((org.apache.spark.sql.execution.metric.SQLMetric) references[0] /* numOutputRows */).add(1); /* 123 */ boolean localtablescan_isNull_0 = localtablescan_row_0.isNullAt(0); /* 124 */ UTF8String localtablescan_value_0 = localtablescan_isNull_0 ? /* 125 */ null : (localtablescan_row_0.getUTF8String(0)); /* 126 */ /* 127 */ project_doConsume_0(localtablescan_row_0, localtablescan_value_0, localtablescan_isNull_0); /* 128 */ if (shouldStop()) return; /* 129 */ } /* 130 */ } /* 131 */ /* 132 */ } -- !query 13 select v, str_to_map(v) from values ('abc🅰️a,:'), (null), (''), ('1:2') t(v) -- !query 13 schema struct<v:string,str_to_map(v, ,, :):map<string,string>> -- !query 13 output {"":null} 1:2 {"1":"2"} NULL NULL abc🅰️a,: {"":"","abc":"a:a"} ``` Closes #27013 from yaooqinn/SPARK-30356. Authored-by: Kent Yao <yaooqinn@hotmail.com> Signed-off-by: Wenchen Fan <wenchen@databricks.com> |