## What changes were proposed in this pull request?
This patch removes the rangeBetween functions introduced in SPARK-21608. As explained in SPARK-25841, these functions are confusing and don't quite work. We will redesign them and introduce better ones in SPARK-25843.
## How was this patch tested?
Removed relevant test cases as well. These test cases will need to be added back in SPARK-25843.
Closes#22870 from rxin/SPARK-25862.
Lead-authored-by: Reynold Xin <rxin@databricks.com>
Co-authored-by: hyukjinkwon <gurwls223@apache.org>
Signed-off-by: gatorsmile <gatorsmile@gmail.com>
## What changes were proposed in this pull request?
When we compare attributes, in general, we should always refer to semantic equality, as the default `equal` method can return false when there are "cosmetic" differences between them, but still they are the same thing; at least we have to consider them so when analyzing/optimizing queries.
The PR focuses on the usage and comparison of the `output` of a `LogicalPlan`, which is a `Seq[Attribute]` in `AliasViewChild`. In this case, using equality implicitly fails to check the semantic equality. This results in the operator failing to stabilize.
## How was this patch tested?
running the tests with the patch provided by maryannxue in https://github.com/apache/spark/pull/22060Closes#22713 from mgaido91/SPARK-25691.
Authored-by: Marco Gaido <marcogaido91@gmail.com>
Signed-off-by: Wenchen Fan <wenchen@databricks.com>
## What changes were proposed in this pull request?
This PR proposes to avoid hardcorded configuration keys in SQLConf's `doc.
## How was this patch tested?
Manually verified.
Closes#22877 from HyukjinKwon/minor-conf-name.
Authored-by: hyukjinkwon <gurwls223@apache.org>
Signed-off-by: hyukjinkwon <gurwls223@apache.org>
## What changes were proposed in this pull request?
These two classes were added for regr_ expression support (SPARK-23907). These have been removed and hence we can remove these base classes and inline the logic in the concrete classes.
## How was this patch tested?
Existing tests.
Closes#22856 from dilipbiswal/average_cleanup.
Authored-by: Dilip Biswal <dbiswal@us.ibm.com>
Signed-off-by: Sean Owen <sean.owen@databricks.com>
## What changes were proposed in this pull request?
Extractors are made of 2 expressions, one of them defines the the value to be extract from (called `child`) and the other defines the way of extraction (called `extraction`). In this term extractors have 2 children so they shouldn't be `UnaryExpression`s.
`ResolveReferences` was changed in this commit: 36b826f5d1 which resulted a regression with nested extractors. An extractor need to define its children as the set of both `child` and `extraction`; and should try to resolve both in `ResolveReferences`.
This PR changes `UnresolvedExtractValue` to a `BinaryExpression`.
## How was this patch tested?
added UT
Closes#22817 from peter-toth/SPARK-25816.
Authored-by: Peter Toth <peter.toth@gmail.com>
Signed-off-by: gatorsmile <gatorsmile@gmail.com>
## What changes were proposed in this pull request?
WindowSpecDefinition checks start < last, but CalendarIntervalType is not comparable, so it would throw the following exception at runtime:
```
scala.MatchError: CalendarIntervalType (of class org.apache.spark.sql.types.CalendarIntervalType$) at
org.apache.spark.sql.catalyst.util.TypeUtils$.getInterpretedOrdering(TypeUtils.scala:58) at
org.apache.spark.sql.catalyst.expressions.BinaryComparison.ordering$lzycompute(predicates.scala:592) at
org.apache.spark.sql.catalyst.expressions.BinaryComparison.ordering(predicates.scala:592) at
org.apache.spark.sql.catalyst.expressions.GreaterThan.nullSafeEval(predicates.scala:797) at org.apache.spark.sql.catalyst.expressions.BinaryExpression.eval(Expression.scala:496) at org.apache.spark.sql.catalyst.expressions.SpecifiedWindowFrame.isGreaterThan(windowExpressions.scala:245) at
org.apache.spark.sql.catalyst.expressions.SpecifiedWindowFrame.checkInputDataTypes(windowExpressions.scala:216) at
org.apache.spark.sql.catalyst.expressions.Expression.resolved$lzycompute(Expression.scala:171) at
org.apache.spark.sql.catalyst.expressions.Expression.resolved(Expression.scala:171) at
org.apache.spark.sql.catalyst.expressions.Expression$$anonfun$childrenResolved$1.apply(Expression.scala:183) at
org.apache.spark.sql.catalyst.expressions.Expression$$anonfun$childrenResolved$1.apply(Expression.scala:183) at
scala.collection.IndexedSeqOptimized$class.prefixLengthImpl(IndexedSeqOptimized.scala:38) at scala.collection.IndexedSeqOptimized$class.forall(IndexedSeqOptimized.scala:43) at scala.collection.mutable.ArrayBuffer.forall(ArrayBuffer.scala:48) at
org.apache.spark.sql.catalyst.expressions.Expression.childrenResolved(Expression.scala:183) at
org.apache.spark.sql.catalyst.expressions.WindowSpecDefinition.resolved$lzycompute(windowExpressions.scala:48) at
org.apache.spark.sql.catalyst.expressions.WindowSpecDefinition.resolved(windowExpressions.scala:48) at
org.apache.spark.sql.catalyst.expressions.Expression$$anonfun$childrenResolved$1.apply(Expression.scala:183) at
org.apache.spark.sql.catalyst.expressions.Expression$$anonfun$childrenResolved$1.apply(Expression.scala:183) at
scala.collection.LinearSeqOptimized$class.forall(LinearSeqOptimized.scala:83)
```
We fix the issue by only perform the check on boundary expressions that are AtomicType.
## How was this patch tested?
Add new test case in `DataFrameWindowFramesSuite`
Closes#22853 from jiangxb1987/windowBoundary.
Authored-by: Xingbo Jiang <xingbo.jiang@databricks.com>
Signed-off-by: Xingbo Jiang <xingbo.jiang@databricks.com>
## What changes were proposed in this pull request?
After https://github.com/apache/spark/pull/22745 , Dataset encoder supports the combination of java bean and map type. This PR is to fix the Scala side.
The reason why it didn't work before is, `CatalystToExternalMap` tries to get the data type of the input map expression, while it can be unresolved and its data type is known. To fix it, we can follow `UnresolvedMapObjects`, to create a `UnresolvedCatalystToExternalMap`, and only create `CatalystToExternalMap` when the input map expression is resolved and the data type is known.
## How was this patch tested?
enable a old test case
Closes#22812 from cloud-fan/map.
Authored-by: Wenchen Fan <wenchen@databricks.com>
Signed-off-by: Wenchen Fan <wenchen@databricks.com>
## What changes were proposed in this pull request?
Implements Every, Some, Any aggregates in SQL. These new aggregate expressions are analyzed in normal way and rewritten to equivalent existing aggregate expressions in the optimizer.
Every(x) => Min(x) where x is boolean.
Some(x) => Max(x) where x is boolean.
Any is a synonym for Some.
SQL
```
explain extended select every(v) from test_agg group by k;
```
Plan :
```
== Parsed Logical Plan ==
'Aggregate ['k], [unresolvedalias('every('v), None)]
+- 'UnresolvedRelation `test_agg`
== Analyzed Logical Plan ==
every(v): boolean
Aggregate [k#0], [every(v#1) AS every(v)#5]
+- SubqueryAlias `test_agg`
+- Project [k#0, v#1]
+- SubqueryAlias `test_agg`
+- LocalRelation [k#0, v#1]
== Optimized Logical Plan ==
Aggregate [k#0], [min(v#1) AS every(v)#5]
+- LocalRelation [k#0, v#1]
== Physical Plan ==
*(2) HashAggregate(keys=[k#0], functions=[min(v#1)], output=[every(v)#5])
+- Exchange hashpartitioning(k#0, 200)
+- *(1) HashAggregate(keys=[k#0], functions=[partial_min(v#1)], output=[k#0, min#7])
+- LocalTableScan [k#0, v#1]
Time taken: 0.512 seconds, Fetched 1 row(s)
```
## How was this patch tested?
Added tests in SQLQueryTestSuite, DataframeAggregateSuite
Closes#22809 from dilipbiswal/SPARK-19851-specific-rewrite.
Authored-by: Dilip Biswal <dbiswal@us.ibm.com>
Signed-off-by: Wenchen Fan <wenchen@databricks.com>
## What changes were proposed in this pull request?
The main purpose of `schema_of_json` is the usage of combination with `from_json` (to make up the leak of schema inference) which takes its schema only as literal; however, currently `schema_of_json` allows JSON input as non-literal expressions (e.g, column).
This was mistakenly allowed - we don't have to take other usages rather then the main purpose into account for now.
This PR makes a followup to only allow literals for `schema_of_json`'s JSON input. We can allow non literal expressions later when it's needed or there are some usecase for it.
## How was this patch tested?
Unit tests were added.
Closes#22775 from HyukjinKwon/SPARK-25447-followup.
Lead-authored-by: hyukjinkwon <gurwls223@apache.org>
Co-authored-by: Hyukjin Kwon <gurwls223@apache.org>
Signed-off-by: Wenchen Fan <wenchen@databricks.com>
## What changes were proposed in this pull request?
In https://github.com/apache/spark/pull/22745 we introduced the `GetArrayFromMap` expression. Later on I realized this is duplicated as we already have `MapKeys` and `MapValues`.
This PR removes `GetArrayFromMap`
## How was this patch tested?
existing tests
Closes#22825 from cloud-fan/minor.
Authored-by: Wenchen Fan <wenchen@databricks.com>
Signed-off-by: Wenchen Fan <wenchen@databricks.com>
## What changes were proposed in this pull request?
This is inspired during implementing #21732. For now `ScalaReflection` needs to consider how `ExpressionEncoder` uses generated serializers and deserializers. And `ExpressionEncoder` has a weird `flat` flag. After discussion with cloud-fan, it seems to be better to refactor `ExpressionEncoder`. It should make SPARK-24762 easier to do.
To summarize the proposed changes:
1. `serializerFor` and `deserializerFor` return expressions for serializing/deserializing an input expression for a given type. They are private and should not be called directly.
2. `serializerForType` and `deserializerForType` returns an expression for serializing/deserializing for an object of type T to/from Spark SQL representation. It assumes the input object/Spark SQL representation is located at ordinal 0 of a row.
So in other words, `serializerForType` and `deserializerForType` return expressions for atomically serializing/deserializing JVM object to/from Spark SQL value.
A serializer returned by `serializerForType` will serialize an object at `row(0)` to a corresponding Spark SQL representation, e.g. primitive type, array, map, struct.
A deserializer returned by `deserializerForType` will deserialize an input field at `row(0)` to an object with given type.
3. The construction of `ExpressionEncoder` takes a pair of serializer and deserializer for type `T`. It uses them to create serializer and deserializer for T <-> row serialization. Now `ExpressionEncoder` dones't need to remember if serializer is flat or not. When we need to construct new `ExpressionEncoder` based on existing ones, we only need to change input location in the atomic serializer and deserializer.
## How was this patch tested?
Existing tests.
Closes#22749 from viirya/SPARK-24762-refactor.
Authored-by: Liang-Chi Hsieh <viirya@gmail.com>
Signed-off-by: Wenchen Fan <wenchen@databricks.com>
## What changes were proposed in this pull request?
Check the `spark.sql.repl.eagerEval.enabled` configuration property in SparkDataFrame `show()` method. If the `SparkSession` has eager execution enabled, the data will be returned to the R client when the data frame is created. So instead of seeing this
```
> df <- createDataFrame(faithful)
> df
SparkDataFrame[eruptions:double, waiting:double]
```
you will see
```
> df <- createDataFrame(faithful)
> df
+---------+-------+
|eruptions|waiting|
+---------+-------+
| 3.6| 79.0|
| 1.8| 54.0|
| 3.333| 74.0|
| 2.283| 62.0|
| 4.533| 85.0|
| 2.883| 55.0|
| 4.7| 88.0|
| 3.6| 85.0|
| 1.95| 51.0|
| 4.35| 85.0|
| 1.833| 54.0|
| 3.917| 84.0|
| 4.2| 78.0|
| 1.75| 47.0|
| 4.7| 83.0|
| 2.167| 52.0|
| 1.75| 62.0|
| 4.8| 84.0|
| 1.6| 52.0|
| 4.25| 79.0|
+---------+-------+
only showing top 20 rows
```
## How was this patch tested?
Manual tests as well as unit tests (one new test case is added).
Author: adrian555 <v2ave10p>
Closes#22455 from adrian555/eager_execution.
## What changes were proposed in this pull request?
In the PR, I propose to switch `from_json` on `FailureSafeParser`, and to make the function compatible to `PERMISSIVE` mode by default, and to support the `FAILFAST` mode as well. The `DROPMALFORMED` mode is not supported by `from_json`.
## How was this patch tested?
It was tested by existing `JsonSuite`/`CSVSuite`, `JsonFunctionsSuite` and `JsonExpressionsSuite` as well as new tests for `from_json` which checks different modes.
Closes#22237 from MaxGekk/from_json-failuresafe.
Lead-authored-by: Maxim Gekk <maxim.gekk@databricks.com>
Co-authored-by: hyukjinkwon <gurwls223@apache.org>
Signed-off-by: Wenchen Fan <wenchen@databricks.com>
This is a follow-up PR for #22708. It considers another case of java beans deserialization: java maps with struct keys/values.
When deserializing values of MapType with struct keys/values in java beans, fields of structs get mixed up. I suggest using struct data types retrieved from resolved input data instead of inferring them from java beans.
## What changes were proposed in this pull request?
Invocations of "keyArray" and "valueArray" functions are used to extract arrays of keys and values. Struct type of keys or values is also inferred from java bean structure and ends up with mixed up field order.
I created a new UnresolvedInvoke expression as a temporary substitution of Invoke expression while no actual data is available. It allows to provide the resulting data type during analysis based on the resolved input data, not on the java bean (similar to UnresolvedMapObjects).
Key and value arrays are then fed to MapObjects expression which I replaced with UnresolvedMapObjects, just like in case of ArrayType.
Finally I added resolution of UnresolvedInvoke expressions in Analyzer.resolveExpression method as an additional pattern matching case.
## How was this patch tested?
Added a test case.
Built complete project on travis.
viirya kiszk cloud-fan michalsenkyr marmbrus liancheng
Closes#22745 from vofque/SPARK-21402-FOLLOWUP.
Lead-authored-by: Vladimir Kuriatkov <vofque@gmail.com>
Co-authored-by: Vladimir Kuriatkov <Vladimir_Kuriatkov@epam.com>
Signed-off-by: Wenchen Fan <wenchen@databricks.com>
## What changes were proposed in this pull request?
The serializers of `RowEncoder` use few `If` Catalyst expression which inherits `ComplexTypeMergingExpression` that will check input data types.
It is possible to generate serializers which fail the check and can't to access the data type of serializers. When producing If expression, we should use the same data type at its input expressions.
## How was this patch tested?
Added test.
Closes#22785 from viirya/SPARK-25791.
Authored-by: Liang-Chi Hsieh <viirya@gmail.com>
Signed-off-by: Wenchen Fan <wenchen@databricks.com>
## What changes were proposed in this pull request?
This takes over original PR at #22019. The original proposal is to have null for float and double types. Later a more reasonable proposal is to disallow empty strings. This patch adds logic to throw exception when finding empty strings for non string types.
## How was this patch tested?
Added test.
Closes#22787 from viirya/SPARK-25040.
Authored-by: Liang-Chi Hsieh <viirya@gmail.com>
Signed-off-by: hyukjinkwon <gurwls223@apache.org>
## What changes were proposed in this pull request?
This PR adds `prettyNames` for `from_json`, `to_json`, `from_csv`, and `schema_of_json` so that appropriate names are used.
## How was this patch tested?
Unit tests
Closes#22773 from HyukjinKwon/minor-prettyNames.
Authored-by: hyukjinkwon <gurwls223@apache.org>
Signed-off-by: hyukjinkwon <gurwls223@apache.org>
## What changes were proposed in this pull request?
SQL interface support specify `StorageLevel` when cache table. The semantic is:
```sql
CACHE TABLE tableName OPTIONS('storageLevel' 'DISK_ONLY');
```
All supported `StorageLevel` are:
eefdf9f9dd/core/src/main/scala/org/apache/spark/storage/StorageLevel.scala (L172-L183)
## How was this patch tested?
unit tests and manual tests.
manual tests configuration:
```
--executor-memory 15G --executor-cores 5 --num-executors 50
```
Data:
Input Size / Records: 1037.7 GB / 11732805788
Result:
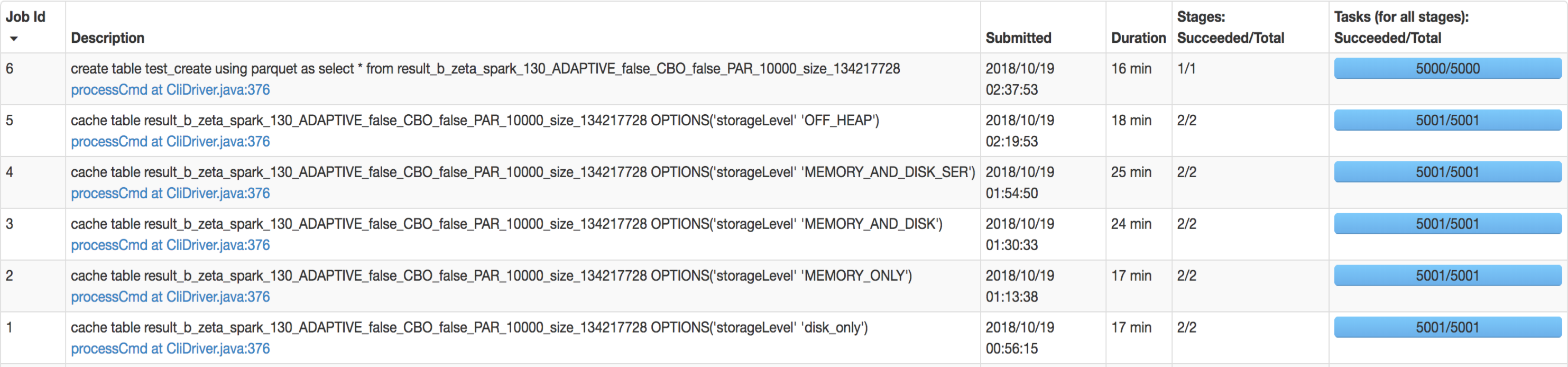
Closes#22263 from wangyum/SPARK-25269.
Authored-by: Yuming Wang <yumwang@ebay.com>
Signed-off-by: Dongjoon Hyun <dongjoon@apache.org>
## What changes were proposed in this pull request?
This is a follow-up PR for #22259. The extra field added in `ScalaUDF` with the original PR was declared optional, but should be indeed required, otherwise callers of `ScalaUDF`'s constructor could ignore this new field and cause the result to be incorrect. This PR makes the new field required and changes its name to `handleNullForInputs`.
#22259 breaks the previous behavior for null-handling of primitive-type input parameters. For example, for `val f = udf({(x: Int, y: Any) => x})`, `f(null, "str")` should return `null` but would return `0` after #22259. In this PR, all UDF methods except `def udf(f: AnyRef, dataType: DataType): UserDefinedFunction` have been restored with the original behavior. The only exception is documented in the Spark SQL migration guide.
In addition, now that we have this extra field indicating if a null-test should be applied on the corresponding input value, we can also make use of this flag to avoid the rule `HandleNullInputsForUDF` being applied infinitely.
## How was this patch tested?
Added UT in UDFSuite
Passed affected existing UTs:
AnalysisSuite
UDFSuite
Closes#22732 from maryannxue/spark-25044-followup.
Lead-authored-by: maryannxue <maryannxue@apache.org>
Co-authored-by: Wenchen Fan <wenchen@databricks.com>
Signed-off-by: Wenchen Fan <wenchen@databricks.com>
## What changes were proposed in this pull request?
CSVs with windows style crlf ('\r\n') don't work in multiline mode. They work fine in single line mode because the line separation is done by Hadoop, which can handle all the different types of line separators. This PR fixes it by enabling Univocity's line separator detection in multiline mode, which will detect '\r\n', '\r', or '\n' automatically as it is done by hadoop in single line mode.
## How was this patch tested?
Unit test with a file with crlf line endings.
Closes#22503 from justinuang/fix-clrf-multiline.
Authored-by: Justin Uang <juang@palantir.com>
Signed-off-by: hyukjinkwon <gurwls223@apache.org>
When deserializing values of ArrayType with struct elements in java beans, fields of structs get mixed up.
I suggest using struct data types retrieved from resolved input data instead of inferring them from java beans.
## What changes were proposed in this pull request?
MapObjects expression is used to map array elements to java beans. Struct type of elements is inferred from java bean structure and ends up with mixed up field order.
I used UnresolvedMapObjects instead of MapObjects, which allows to provide element type for MapObjects during analysis based on the resolved input data, not on the java bean.
## How was this patch tested?
Added a test case.
Built complete project on travis.
michalsenkyr cloud-fan marmbrus liancheng
Closes#22708 from vofque/SPARK-21402.
Lead-authored-by: Vladimir Kuriatkov <vofque@gmail.com>
Co-authored-by: Vladimir Kuriatkov <Vladimir_Kuriatkov@epam.com>
Signed-off-by: Wenchen Fan <wenchen@databricks.com>
## What changes were proposed in this pull request?
this PR correct some comment error:
1. change from "as low a possible" to "as low as possible" in RewriteDistinctAggregates.scala
2. delete redundant word “with” in HiveTableScanExec’s doExecute() method
## How was this patch tested?
Existing unit tests.
Closes#22694 from CarolinePeng/update_comment.
Authored-by: 彭灿00244106 <00244106@zte.intra>
Signed-off-by: hyukjinkwon <gurwls223@apache.org>
## What changes were proposed in this pull request?
`Literal.value` should have a value a value corresponding to `dataType`. This pr added code to verify it and fixed the existing tests to do so.
## How was this patch tested?
Modified the existing tests.
Closes#22724 from maropu/SPARK-25734.
Authored-by: Takeshi Yamamuro <yamamuro@apache.org>
Signed-off-by: Wenchen Fan <wenchen@databricks.com>
## What changes were proposed in this pull request?
The PR adds new function `from_csv()` similar to `from_json()` to parse columns with CSV strings. I added the following methods:
```Scala
def from_csv(e: Column, schema: StructType, options: Map[String, String]): Column
```
and this signature to call it from Python, R and Java:
```Scala
def from_csv(e: Column, schema: String, options: java.util.Map[String, String]): Column
```
## How was this patch tested?
Added new test suites `CsvExpressionsSuite`, `CsvFunctionsSuite` and sql tests.
Closes#22379 from MaxGekk/from_csv.
Lead-authored-by: Maxim Gekk <maxim.gekk@databricks.com>
Co-authored-by: Maxim Gekk <max.gekk@gmail.com>
Co-authored-by: Hyukjin Kwon <gurwls223@gmail.com>
Co-authored-by: hyukjinkwon <gurwls223@apache.org>
Signed-off-by: hyukjinkwon <gurwls223@apache.org>
## What changes were proposed in this pull request?
AFAIK multi-column count is not widely supported by the mainstream databases(postgres doesn't support), and the SQL standard doesn't define it clearly, as near as I can tell.
Since Spark supports it, we should clearly document the current behavior and add tests to verify it.
## How was this patch tested?
N/A
Closes#22728 from cloud-fan/doc.
Authored-by: Wenchen Fan <wenchen@databricks.com>
Signed-off-by: hyukjinkwon <gurwls223@apache.org>
## What changes were proposed in this pull request?
Project logical operator generates valid constraints using two opposite operations. It substracts child constraints from all constraints, than union child constraints again. I think it may be not necessary.
Aggregate operator has the same problem with Project.
This PR try to remove these two opposite collection operations.
## How was this patch tested?
Related unit tests:
ProjectEstimationSuite
CollapseProjectSuite
PushProjectThroughUnionSuite
UnsafeProjectionBenchmark
GeneratedProjectionSuite
CodeGeneratorWithInterpretedFallbackSuite
TakeOrderedAndProjectSuite
GenerateUnsafeProjectionSuite
BucketedRandomProjectionLSHSuite
RemoveRedundantAliasAndProjectSuite
AggregateBenchmark
AggregateOptimizeSuite
AggregateEstimationSuite
DecimalAggregatesSuite
DateFrameAggregateSuite
ObjectHashAggregateSuite
TwoLevelAggregateHashMapSuite
ObjectHashAggregateExecBenchmark
SingleLevelAggregateHaspMapSuite
TypedImperativeAggregateSuite
RewriteDistinctAggregatesSuite
HashAggregationQuerySuite
HashAggregationQueryWithControlledFallbackSuite
TypedImperativeAggregateSuite
TwoLevelAggregateHashMapWithVectorizedMapSuite
Closes#22706 from SongYadong/generate_constraints.
Authored-by: SongYadong <song.yadong1@zte.com.cn>
Signed-off-by: gatorsmile <gatorsmile@gmail.com>
## What changes were proposed in this pull request?
improve the code comment added in https://github.com/apache/spark/pull/22702/files
## How was this patch tested?
N/A
Closes#22711 from cloud-fan/minor.
Authored-by: Wenchen Fan <wenchen@databricks.com>
Signed-off-by: gatorsmile <gatorsmile@gmail.com>
## What changes were proposed in this pull request?
```Scala
val df1 = Seq(("abc", 1), (null, 3)).toDF("col1", "col2")
df1.write.mode(SaveMode.Overwrite).parquet("/tmp/test1")
val df2 = spark.read.parquet("/tmp/test1")
df2.filter("col1 = 'abc' OR (col1 != 'abc' AND col2 == 3)").show()
```
Before the PR, it returns both rows. After the fix, it returns `Row ("abc", 1))`. This is to fix the bug in NULL handling in BooleanSimplification. This is a bug introduced in Spark 1.6 release.
## How was this patch tested?
Added test cases
Closes#22702 from gatorsmile/fixBooleanSimplify2.
Authored-by: gatorsmile <gatorsmile@gmail.com>
Signed-off-by: gatorsmile <gatorsmile@gmail.com>
## What changes were proposed in this pull request?
According to the SQL standard, when a query contains `HAVING`, it indicates an aggregate operator. For more details please refer to https://blog.jooq.org/2014/12/04/do-you-really-understand-sqls-group-by-and-having-clauses/
However, in Spark SQL parser, we treat HAVING as a normal filter when there is no GROUP BY, which breaks SQL semantic and lead to wrong result. This PR fixes the parser.
## How was this patch tested?
new test
Closes#22696 from cloud-fan/having.
Authored-by: Wenchen Fan <wenchen@databricks.com>
Signed-off-by: gatorsmile <gatorsmile@gmail.com>
## What changes were proposed in this pull request?
The HandleNullInputsForUDF rule can generate new If node infinitely, thus causing problems like match of SQL cache missed.
This was fixed in SPARK-24891 and was then broken by SPARK-25044.
The unit test in `AnalysisSuite` added in SPARK-24891 should have failed but didn't because it wasn't properly updated after the `ScalaUDF` constructor signature change. So this PR also updates the test accordingly based on the new `ScalaUDF` constructor.
## How was this patch tested?
Updated the original UT. This should be justified as the original UT became invalid after SPARK-25044.
Closes#22701 from maryannxue/spark-25690.
Authored-by: maryannxue <maryannxue@apache.org>
Signed-off-by: gatorsmile <gatorsmile@gmail.com>
While working on another PR, I noticed that there is quite some legacy Java in there that can be beautified. For example the use of features from Java8, such as:
- Collection libraries
- Try-with-resource blocks
No logic has been changed. I think it is important to have a solid codebase with examples that will inspire next PR's to follow up on the best practices.
What are your thoughts on this?
This makes code easier to read, and using try-with-resource makes is less likely to forget to close something.
## What changes were proposed in this pull request?
No changes in the logic of Spark, but more in the aesthetics of the code.
## How was this patch tested?
Using the existing unit tests. Since no logic is changed, the existing unit tests should pass.
Please review http://spark.apache.org/contributing.html before opening a pull request.
Closes#22637 from Fokko/SPARK-25408.
Authored-by: Fokko Driesprong <fokkodriesprong@godatadriven.com>
Signed-off-by: Sean Owen <sean.owen@databricks.com>
## What changes were proposed in this pull request?
Clean up the joinCriteria parsing in the parser by directly using identifierList
## How was this patch tested?
N/A
Closes#22648 from gatorsmile/cleanupJoinCriteria.
Authored-by: gatorsmile <gatorsmile@gmail.com>
Signed-off-by: gatorsmile <gatorsmile@gmail.com>
## What changes were proposed in this pull request?
Adds support for the setting limit in the sql split function
## How was this patch tested?
1. Updated unit tests
2. Tested using Scala spark shell
Please review http://spark.apache.org/contributing.html before opening a pull request.
Closes#22227 from phegstrom/master.
Authored-by: Parker Hegstrom <phegstrom@palantir.com>
Signed-off-by: hyukjinkwon <gurwls223@apache.org>
While working on another PR, I noticed that there is quite some legacy Java in there that can be beautified. For example the use og features from Java8, such as:
- Collection libraries
- Try-with-resource blocks
No code has been changed
What are your thoughts on this?
This makes code easier to read, and using try-with-resource makes is less likely to forget to close something.
## What changes were proposed in this pull request?
(Please fill in changes proposed in this fix)
## How was this patch tested?
(Please explain how this patch was tested. E.g. unit tests, integration tests, manual tests)
(If this patch involves UI changes, please attach a screenshot; otherwise, remove this)
Please review http://spark.apache.org/contributing.html before opening a pull request.
Closes#22399 from Fokko/SPARK-25408.
Authored-by: Fokko Driesprong <fokkodriesprong@godatadriven.com>
Signed-off-by: Sean Owen <sean.owen@databricks.com>
## What changes were proposed in this pull request?
In #20850 when writing non-null decimals, instead of zero-ing all the 16 allocated bytes, we zero-out only the padding bytes. Since we always allocate 16 bytes, if the number of bytes needed for a decimal is lower than 9, then this means that the bytes between 8 and 16 are not zero-ed.
I see 2 solutions here:
- we can zero-out all the bytes in advance as it was done before #20850 (safer solution IMHO);
- we can allocate only the needed bytes (may be a bit more efficient in terms of memory used, but I have not investigated the feasibility of this option).
Hence I propose here the first solution in order to fix the correctness issue. We can eventually switch to the second if we think is more efficient later.
## How was this patch tested?
Running the test attached in the JIRA + added UT
Closes#22602 from mgaido91/SPARK-25582.
Authored-by: Marco Gaido <marcogaido91@gmail.com>
Signed-off-by: Dongjoon Hyun <dongjoon@apache.org>
## What changes were proposed in this pull request?
This patch is to bump the master branch version to 3.0.0-SNAPSHOT.
## How was this patch tested?
N/A
Closes#22606 from gatorsmile/bump3.0.
Authored-by: gatorsmile <gatorsmile@gmail.com>
Signed-off-by: gatorsmile <gatorsmile@gmail.com>
## What changes were proposed in this pull request?
#22519 introduced a bug when the attributes in the pivot clause are cosmetically different from the output ones (eg. different case). In particular, the problem is that the PR used a `Set[Attribute]` instead of an `AttributeSet`.
## How was this patch tested?
added UT
Closes#22582 from mgaido91/SPARK-25505_followup.
Authored-by: Marco Gaido <marcogaido91@gmail.com>
Signed-off-by: gatorsmile <gatorsmile@gmail.com>
## What changes were proposed in this pull request?
This PR adds a rule to force `.toLowerCase(Locale.ROOT)` or `toUpperCase(Locale.ROOT)`.
It produces an error as below:
```
[error] Are you sure that you want to use toUpperCase or toLowerCase without the root locale? In most cases, you
[error] should use toUpperCase(Locale.ROOT) or toLowerCase(Locale.ROOT) instead.
[error] If you must use toUpperCase or toLowerCase without the root locale, wrap the code block with
[error] // scalastyle:off caselocale
[error] .toUpperCase
[error] .toLowerCase
[error] // scalastyle:on caselocale
```
This PR excludes the cases above for SQL code path for external calls like table name, column name and etc.
For test suites, or when it's clear there's no locale problem like Turkish locale problem, it uses `Locale.ROOT`.
One minor problem is, `UTF8String` has both methods, `toLowerCase` and `toUpperCase`, and the new rule detects them as well. They are ignored.
## How was this patch tested?
Manually tested, and Jenkins tests.
Closes#22581 from HyukjinKwon/SPARK-25565.
Authored-by: hyukjinkwon <gurwls223@apache.org>
Signed-off-by: hyukjinkwon <gurwls223@apache.org>
## What changes were proposed in this pull request?
In the PR, I propose to extended the `schema_of_json()` function, and accept JSON options since they can impact on schema inferring. Purpose is to support the same options that `from_json` can use during schema inferring.
## How was this patch tested?
Added SQL, Python and Scala tests (`JsonExpressionsSuite` and `JsonFunctionsSuite`) that checks JSON options are used.
Closes#22442 from MaxGekk/schema_of_json-options.
Authored-by: Maxim Gekk <maxim.gekk@databricks.com>
Signed-off-by: hyukjinkwon <gurwls223@apache.org>
## What changes were proposed in this pull request?
**Description from the JIRA :**
Currently, to collect the statistics of all the columns, users need to specify the names of all the columns when calling the command "ANALYZE TABLE ... FOR COLUMNS...". This is not user friendly. Instead, we can introduce the following SQL command to achieve it without specifying the column names.
```
ANALYZE TABLE [db_name.]tablename COMPUTE STATISTICS FOR ALL COLUMNS;
```
## How was this patch tested?
Added new tests in SparkSqlParserSuite and StatisticsSuite
Closes#22566 from dilipbiswal/SPARK-25458.
Authored-by: Dilip Biswal <dbiswal@us.ibm.com>
Signed-off-by: gatorsmile <gatorsmile@gmail.com>
## What changes were proposed in this pull request?
The grouping columns from a Pivot query are inferred as "input columns - pivot columns - pivot aggregate columns", where input columns are the output of the child relation of Pivot. The grouping columns will be the leading columns in the pivot output and they should preserve the same order as specified by the input. For example,
```
SELECT * FROM (
SELECT course, earnings, "a" as a, "z" as z, "b" as b, "y" as y, "c" as c, "x" as x, "d" as d, "w" as w
FROM courseSales
)
PIVOT (
sum(earnings)
FOR course IN ('dotNET', 'Java')
)
```
The output columns should be "a, z, b, y, c, x, d, w, ..." but now it is "a, b, c, d, w, x, y, z, ..."
The fix is to use the child plan's `output` instead of `outputSet` so that the order can be preserved.
## How was this patch tested?
Added UT.
Closes#22519 from maryannxue/spark-25505.
Authored-by: maryannxue <maryannxue@apache.org>
Signed-off-by: gatorsmile <gatorsmile@gmail.com>
## What changes were proposed in this pull request?
The `show create table` will show a lot of generated attributes for views that created by older Spark version. This PR will basically revert https://issues.apache.org/jira/browse/SPARK-19272 back, so when you `DESC [FORMATTED|EXTENDED] view` will show the original view DDL text.
## How was this patch tested?
Unit test.
Closes#22458 from zheyuan28/testbranch.
Lead-authored-by: Chris Zhao <chris.zhao@databricks.com>
Co-authored-by: Christopher Zhao <chris.zhao@databricks.com>
Co-authored-by: Dongjoon Hyun <dongjoon@apache.org>
Signed-off-by: Dongjoon Hyun <dongjoon@apache.org>
## What changes were proposed in this pull request?
There are 2 places we check for problematic `InSubquery`: the rule `ResolveSubquery` and `InSubquery.checkInputDataTypes`. We should unify them.
## How was this patch tested?
existing tests
Closes#22563 from cloud-fan/followup.
Authored-by: Wenchen Fan <wenchen@databricks.com>
Signed-off-by: Wenchen Fan <wenchen@databricks.com>
## What changes were proposed in this pull request?
As per the discussion in https://github.com/apache/spark/pull/22553#pullrequestreview-159192221,
override `filterKeys` violates the documented semantics.
This PR is to remove it and add documentation.
Also fix one potential non-serializable map in `FileStreamOptions`.
The only one call of `CaseInsensitiveMap`'s `filterKeys` left is
c3c45cbd76/sql/hive/src/main/scala/org/apache/spark/sql/hive/execution/HiveOptions.scala (L88-L90)
But this one is OK.
## How was this patch tested?
Existing unit tests.
Closes#22562 from gengliangwang/SPARK-25541-FOLLOWUP.
Authored-by: Gengliang Wang <gengliang.wang@databricks.com>
Signed-off-by: Wenchen Fan <wenchen@databricks.com>
## What changes were proposed in this pull request?
Thanks for bahchis reporting this. It is more like a follow up work for #16581, this PR fix the scenario of Python UDF accessing attributes from both side of join in join condition.
## How was this patch tested?
Add regression tests in PySpark and `BatchEvalPythonExecSuite`.
Closes#22326 from xuanyuanking/SPARK-25314.
Authored-by: Yuanjian Li <xyliyuanjian@gmail.com>
Signed-off-by: Wenchen Fan <wenchen@databricks.com>
## What changes were proposed in this pull request?
In ElementAt, when first argument is MapType, we should coerce the key type and the second argument based on findTightestCommonType. This is not happening currently. We may produce wrong output as we will incorrectly downcast the right hand side double expression to int.
```SQL
spark-sql> select element_at(map(1,"one", 2, "two"), 2.2);
two
```
Also, when the first argument is ArrayType, the second argument should be an integer type or a smaller integral type that can be safely casted to an integer type. Currently we may do an unsafe cast. In the following case, we should fail with an error as 2.2 is not a integer index. But instead we down cast it to int currently and return a result instead.
```SQL
spark-sql> select element_at(array(1,2), 1.24D);
1
```
This PR also supports implicit cast between two MapTypes. I have followed similar logic that exists today to do implicit casts between two array types.
## How was this patch tested?
Added new tests in DataFrameFunctionSuite, TypeCoercionSuite.
Closes#22544 from dilipbiswal/SPARK-25522.
Authored-by: Dilip Biswal <dbiswal@us.ibm.com>
Signed-off-by: Wenchen Fan <wenchen@databricks.com>
## What changes were proposed in this pull request?
We have an agreement that the behavior of `from/to_utc_timestamp` is corrected, although the function itself doesn't make much sense in Spark: https://issues.apache.org/jira/browse/SPARK-23715
This PR improves the document.
## How was this patch tested?
N/A
Closes#22543 from cloud-fan/doc.
Authored-by: Wenchen Fan <wenchen@databricks.com>
Signed-off-by: Wenchen Fan <wenchen@databricks.com>