## What changes were proposed in this pull request?
Easy fix in the documentation.
## How was this patch tested?
N/A
Closes#20948
Author: Daniel Sakuma <dsakuma@gmail.com>
Closes#20928 from dsakuma/fix_typo_configuration_docs.
## What changes were proposed in this pull request?
This PR is to finish https://github.com/apache/spark/pull/17272
This JIRA is a follow up work after SPARK-19583
As we discussed in that PR
The following DDL for a managed table with an existed default location should throw an exception:
CREATE TABLE ... (PARTITIONED BY ...) AS SELECT ...
CREATE TABLE ... (PARTITIONED BY ...)
Currently there are some situations which are not consist with above logic:
CREATE TABLE ... (PARTITIONED BY ...) succeed with an existed default location
situation: for both hive/datasource(with HiveExternalCatalog/InMemoryCatalog)
CREATE TABLE ... (PARTITIONED BY ...) AS SELECT ...
situation: hive table succeed with an existed default location
This PR is going to make above two situations consist with the logic that it should throw an exception
with an existed default location.
## How was this patch tested?
unit test added
Author: Gengliang Wang <gengliang.wang@databricks.com>
Closes#20886 from gengliangwang/pr-17272.
Fixes https://issues.apache.org/jira/browse/SPARK-23823
Keep origin for all the methods using transformExpression
## What changes were proposed in this pull request?
Keep origin in transformExpression
## How was this patch tested?
Manually tested that this fixes https://issues.apache.org/jira/browse/SPARK-23823 and columns have correct origins after Analyzer.analyze
Author: JiahuiJiang <jjiang@palantir.com>
Author: Jiahui Jiang <jjiang@palantir.com>
Closes#20961 from JiahuiJiang/jj/keep-origin.
## What changes were proposed in this pull request?
This PR allows us to use one of several types of `MemoryBlock`, such as byte array, int array, long array, or `java.nio.DirectByteBuffer`. To use `java.nio.DirectByteBuffer` allows to have off heap memory which is automatically deallocated by JVM. `MemoryBlock` class has primitive accessors like `Platform.getInt()`, `Platform.putint()`, or `Platform.copyMemory()`.
This PR uses `MemoryBlock` for `OffHeapColumnVector`, `UTF8String`, and other places. This PR can improve performance of operations involving memory accesses (e.g. `UTF8String.trim`) by 1.8x.
For now, this PR does not use `MemoryBlock` for `BufferHolder` based on cloud-fan's [suggestion](https://github.com/apache/spark/pull/11494#issuecomment-309694290).
Since this PR is a successor of #11494, close#11494. Many codes were ported from #11494. Many efforts were put here. **I think this PR should credit to yzotov.**
This PR can achieve **1.1-1.4x performance improvements** for operations in `UTF8String` or `Murmur3_x86_32`. Other operations are almost comparable performances.
Without this PR
```
OpenJDK 64-Bit Server VM 1.8.0_121-8u121-b13-0ubuntu1.16.04.2-b13 on Linux 4.4.0-22-generic
Intel(R) Xeon(R) CPU E5-2667 v3 3.20GHz
OpenJDK 64-Bit Server VM 1.8.0_121-8u121-b13-0ubuntu1.16.04.2-b13 on Linux 4.4.0-22-generic
Intel(R) Xeon(R) CPU E5-2667 v3 3.20GHz
Hash byte arrays with length 268435487: Best/Avg Time(ms) Rate(M/s) Per Row(ns) Relative
------------------------------------------------------------------------------------------------
Murmur3_x86_32 526 / 536 0.0 131399881.5 1.0X
UTF8String benchmark: Best/Avg Time(ms) Rate(M/s) Per Row(ns) Relative
------------------------------------------------------------------------------------------------
hashCode 525 / 552 1022.6 1.0 1.0X
substring 414 / 423 1298.0 0.8 1.3X
```
With this PR
```
OpenJDK 64-Bit Server VM 1.8.0_121-8u121-b13-0ubuntu1.16.04.2-b13 on Linux 4.4.0-22-generic
Intel(R) Xeon(R) CPU E5-2667 v3 3.20GHz
Hash byte arrays with length 268435487: Best/Avg Time(ms) Rate(M/s) Per Row(ns) Relative
------------------------------------------------------------------------------------------------
Murmur3_x86_32 474 / 488 0.0 118552232.0 1.0X
UTF8String benchmark: Best/Avg Time(ms) Rate(M/s) Per Row(ns) Relative
------------------------------------------------------------------------------------------------
hashCode 476 / 480 1127.3 0.9 1.0X
substring 287 / 291 1869.9 0.5 1.7X
```
Benchmark program
```
test("benchmark Murmur3_x86_32") {
val length = 8192 * 32768 + 31
val seed = 42L
val iters = 1 << 2
val random = new Random(seed)
val arrays = Array.fill[MemoryBlock](numArrays) {
val bytes = new Array[Byte](length)
random.nextBytes(bytes)
new ByteArrayMemoryBlock(bytes, Platform.BYTE_ARRAY_OFFSET, length)
}
val benchmark = new Benchmark("Hash byte arrays with length " + length,
iters * numArrays, minNumIters = 20)
benchmark.addCase("HiveHasher") { _: Int =>
var sum = 0L
for (_ <- 0L until iters) {
sum += HiveHasher.hashUnsafeBytesBlock(
arrays(i), Platform.BYTE_ARRAY_OFFSET, length)
}
}
benchmark.run()
}
test("benchmark UTF8String") {
val N = 512 * 1024 * 1024
val iters = 2
val benchmark = new Benchmark("UTF8String benchmark", N, minNumIters = 20)
val str0 = new java.io.StringWriter() { { for (i <- 0 until N) { write(" ") } } }.toString
val s0 = UTF8String.fromString(str0)
benchmark.addCase("hashCode") { _: Int =>
var h: Int = 0
for (_ <- 0L until iters) { h += s0.hashCode }
}
benchmark.addCase("substring") { _: Int =>
var s: UTF8String = null
for (_ <- 0L until iters) { s = s0.substring(N / 2 - 5, N / 2 + 5) }
}
benchmark.run()
}
```
I run [this benchmark program](https://gist.github.com/kiszk/94f75b506c93a663bbbc372ffe8f05de) using [the commit](ee5a79861c). I got the following results:
```
OpenJDK 64-Bit Server VM 1.8.0_151-8u151-b12-0ubuntu0.16.04.2-b12 on Linux 4.4.0-66-generic
Intel(R) Xeon(R) CPU E5-2667 v3 3.20GHz
Memory access benchmarks: Best/Avg Time(ms) Rate(M/s) Per Row(ns) Relative
------------------------------------------------------------------------------------------------
ByteArrayMemoryBlock get/putInt() 220 / 221 609.3 1.6 1.0X
Platform get/putInt(byte[]) 220 / 236 610.9 1.6 1.0X
Platform get/putInt(Object) 492 / 494 272.8 3.7 0.4X
OnHeapMemoryBlock get/putLong() 322 / 323 416.5 2.4 0.7X
long[] 221 / 221 608.0 1.6 1.0X
Platform get/putLong(long[]) 321 / 321 418.7 2.4 0.7X
Platform get/putLong(Object) 561 / 563 239.2 4.2 0.4X
```
I also run [this benchmark program](https://gist.github.com/kiszk/5fdb4e03733a5d110421177e289d1fb5) for comparing performance of `Platform.copyMemory()`.
```
OpenJDK 64-Bit Server VM 1.8.0_151-8u151-b12-0ubuntu0.16.04.2-b12 on Linux 4.4.0-66-generic
Intel(R) Xeon(R) CPU E5-2667 v3 3.20GHz
Platform copyMemory: Best/Avg Time(ms) Rate(M/s) Per Row(ns) Relative
------------------------------------------------------------------------------------------------
Object to Object 1961 / 1967 8.6 116.9 1.0X
System.arraycopy Object to Object 1917 / 1921 8.8 114.3 1.0X
byte array to byte array 1961 / 1968 8.6 116.9 1.0X
System.arraycopy byte array to byte array 1909 / 1937 8.8 113.8 1.0X
int array to int array 1921 / 1990 8.7 114.5 1.0X
double array to double array 1918 / 1923 8.7 114.3 1.0X
Object to byte array 1961 / 1967 8.6 116.9 1.0X
Object to short array 1965 / 1972 8.5 117.1 1.0X
Object to int array 1910 / 1915 8.8 113.9 1.0X
Object to float array 1971 / 1978 8.5 117.5 1.0X
Object to double array 1919 / 1944 8.7 114.4 1.0X
byte array to Object 1959 / 1967 8.6 116.8 1.0X
int array to Object 1961 / 1970 8.6 116.9 1.0X
double array to Object 1917 / 1924 8.8 114.3 1.0X
```
These results show three facts:
1. According to the second/third or sixth/seventh results in the first experiment, if we use `Platform.get/putInt(Object)`, we achieve more than 2x worse performance than `Platform.get/putInt(byte[])` with concrete type (i.e. `byte[]`).
2. According to the second/third or fourth/fifth/sixth results in the first experiment, the fastest way to access an array element on Java heap is `array[]`. **Cons of `array[]` is that it is not possible to support unaligned-8byte access.**
3. According to the first/second/third or fourth/sixth/seventh results in the first experiment, `getInt()/putInt() or getLong()/putLong()` in subclasses of `MemoryBlock` can achieve comparable performance to `Platform.get/putInt()` or `Platform.get/putLong()` with concrete type (second or sixth result). There is no overhead regarding virtual call.
4. According to results in the second experiment, for `Platform.copy()`, to pass `Object` can achieve the same performance as to pass any type of primitive array as source or destination.
5. According to second/fourth results in the second experiment, `Platform.copy()` can achieve the same performance as `System.arrayCopy`. **It would be good to use `Platform.copy()` since `Platform.copy()` can take any types for src and dst.**
We are incrementally replace `Platform.get/putXXX` with `MemoryBlock.get/putXXX`. This is because we have two advantages.
1) Achieve better performance due to having a concrete type for an array.
2) Use simple OO design instead of passing `Object`
It is easy to use `MemoryBlock` in `InternalRow`, `BufferHolder`, `TaskMemoryManager`, and others that are already abstracted. It is not easy to use `MemoryBlock` in utility classes related to hashing or others.
Other candidates are
- UnsafeRow, UnsafeArrayData, UnsafeMapData, SpecificUnsafeRowJoiner
- UTF8StringBuffer
- BufferHolder
- TaskMemoryManager
- OnHeapColumnVector
- BytesToBytesMap
- CachedBatch
- classes for hash
- others.
## How was this patch tested?
Added `UnsafeMemoryAllocator`
Author: Kazuaki Ishizaki <ishizaki@jp.ibm.com>
Closes#19222 from kiszk/SPARK-10399.
## What changes were proposed in this pull request?
Add interpreted execution for `InitializeJavaBean` expression.
## How was this patch tested?
Added unit test.
Author: Liang-Chi Hsieh <viirya@gmail.com>
Closes#20985 from viirya/SPARK-23593-2.
## What changes were proposed in this pull request?
This pr added interpreted execution for `StaticInvoke`.
## How was this patch tested?
Added tests in `ObjectExpressionsSuite`.
Author: Kazuaki Ishizaki <ishizaki@jp.ibm.com>
Closes#20753 from kiszk/SPARK-23582.
## What changes were proposed in this pull request?
Add interpreted execution for `InitializeJavaBean` expression.
## How was this patch tested?
Added unit test.
Author: Liang-Chi Hsieh <viirya@gmail.com>
Closes#20756 from viirya/SPARK-23593.
## What changes were proposed in this pull request?
This pr added interpreted execution for `Invoke`.
## How was this patch tested?
Added tests in `ObjectExpressionsSuite`.
Author: Kazuaki Ishizaki <ishizaki@jp.ibm.com>
Closes#20797 from kiszk/SPARK-28583.
## What changes were proposed in this pull request?
In TestHive, the base spark session does this in getOrCreate(), we emulate that behavior for tests.
## How was this patch tested?
N/A
Author: gatorsmile <gatorsmile@gmail.com>
Closes#20969 from gatorsmile/setDefault.
## What changes were proposed in this pull request?
Add cast to nulls introduced by PropagateEmptyRelation so in cases they're part of coalesce they will not break its type checking rules
## How was this patch tested?
Added unit test
Author: Robert Kruszewski <robertk@palantir.com>
Closes#20914 from robert3005/rk/propagate-empty-fix.
## What changes were proposed in this pull request?
Currently, the active spark session is set inconsistently (e.g., in createDataFrame, prior to query execution). Many places in spark also incorrectly query active session when they should be calling activeSession.getOrElse(defaultSession) and so might get None even if a Spark session exists.
The semantics here can be cleaned up if we also set the active session when the default session is set.
Related: https://github.com/apache/spark/pull/20926/files
## How was this patch tested?
Unit test, existing test. Note that if https://github.com/apache/spark/pull/20926 merges first we should also update the tests there.
Author: Eric Liang <ekl@databricks.com>
Closes#20927 from ericl/active-session-cleanup.
## What changes were proposed in this pull request?
Add interpreted execution for `MapObjects` expression.
## How was this patch tested?
Added unit test.
Author: Liang-Chi Hsieh <viirya@gmail.com>
Closes#20771 from viirya/SPARK-23587.
## What changes were proposed in this pull request?
Migrate foreach sink to DataSourceV2.
Since the previous attempt at this PR #20552, we've changed and strictly defined the lifecycle of writer components. This means we no longer need the complicated lifecycle shim from that PR; it just naturally works.
## How was this patch tested?
existing tests
Author: Jose Torres <torres.joseph.f+github@gmail.com>
Closes#20951 from jose-torres/foreach.
## What changes were proposed in this pull request?
This PR implemented the following cleanups related to `UnsafeWriter` class:
- Remove code duplication between `UnsafeRowWriter` and `UnsafeArrayWriter`
- Make `BufferHolder` class internal by delegating its accessor methods to `UnsafeWriter`
- Replace `UnsafeRow.setTotalSize(...)` with `UnsafeRowWriter.setTotalSize()`
## How was this patch tested?
Tested by existing UTs
Author: Kazuaki Ishizaki <ishizaki@jp.ibm.com>
Closes#20850 from kiszk/SPARK-23713.
## What changes were proposed in this pull request?
Currently, the requiredChildDistribution does not specify the partitions. This can cause the weird corner cases where the child's distribution is `SinglePartition` which satisfies the required distribution of `ClusterDistribution(no-num-partition-requirement)`, thus eliminating the shuffle needed to repartition input data into the required number of partitions (i.e. same as state stores). That can lead to "file not found" errors on the state store delta files as the micro-batch-with-no-shuffle will not run certain tasks and therefore not generate the expected state store delta files.
This PR adds the required constraint on the number of partitions.
## How was this patch tested?
Modified test harness to always check that ANY stateful operator should have a constraint on the number of partitions. As part of that, the existing opt-in checks on child output partitioning were removed, as they are redundant.
Author: Tathagata Das <tathagata.das1565@gmail.com>
Closes#20941 from tdas/SPARK-23827.
## What changes were proposed in this pull request?
This PR is to improve the test coverage of the original PR https://github.com/apache/spark/pull/20687
## How was this patch tested?
N/A
Author: gatorsmile <gatorsmile@gmail.com>
Closes#20911 from gatorsmile/addTests.
## What changes were proposed in this pull request?
Roll forward c68ec4e (#20688).
There are two minor test changes required:
* An error which used to be TreeNodeException[ArithmeticException] is no longer wrapped and is now just ArithmeticException.
* The test framework simply does not set the active Spark session. (Or rather, it doesn't do so early enough - I think it only happens when a query is analyzed.) I've added the required logic to SQLTestUtils.
## How was this patch tested?
existing tests
Author: Jose Torres <torres.joseph.f+github@gmail.com>
Author: jerryshao <sshao@hortonworks.com>
Closes#20922 from jose-torres/ratefix.
## What changes were proposed in this pull request?
This PR supports for pushing down filters for DateType in parquet
## How was this patch tested?
Added UT and tested in local.
Author: yucai <yyu1@ebay.com>
Closes#20851 from yucai/SPARK-23727.
## What changes were proposed in this pull request?
isSharedClass returns if some classes can/should be shared or not. It checks if the classes names have some keywords or start with some names. Following the logic, it can occur unintended behaviors when a custom package has `slf4j` inside the package or class name. As I guess, the first intention seems to figure out the class containing `org.slf4j`. It would be better to change the comparison logic to `name.startsWith("org.slf4j")`
## How was this patch tested?
This patch should pass all of the current tests and keep all of the current behaviors. In my case, I'm using ProtobufDeserializer to get a table schema from hive tables. Thus some Protobuf packages and names have `slf4j` inside. Without this patch, it cannot be resolved because of ClassCastException from different classloaders.
Author: Jongyoul Lee <jongyoul@gmail.com>
Closes#20860 from jongyoul/SPARK-23743.
## What changes were proposed in this pull request?
Set default Spark session in the TestSparkSession and TestHiveSparkSession constructors.
## How was this patch tested?
new unit tests
Author: Jose Torres <torres.joseph.f+github@gmail.com>
Closes#20926 from jose-torres/test3.
## What changes were proposed in this pull request?
In SparkSQLCLI, SessionState generates before SparkContext instantiating. When we use --proxy-user to impersonate, it's unable to initializing a metastore client to talk to the secured metastore for no kerberos ticket.
This PR use real user ugi to obtain token for owner before talking to kerberized metastore.
## How was this patch tested?
Manually verified with kerberized hive metasotre / hdfs.
Author: Kent Yao <yaooqinn@hotmail.com>
Closes#20784 from yaooqinn/SPARK-23639.
## What changes were proposed in this pull request?
This PR proposes to add lineSep option for a configurable line separator in text datasource.
It supports this option by using `LineRecordReader`'s functionality with passing it to the constructor.
The approach is similar with https://github.com/apache/spark/pull/20727; however, one main difference is, it uses text datasource's `lineSep` option to parse line by line in JSON's schema inference.
## How was this patch tested?
Manually tested and unit tests were added.
Author: hyukjinkwon <gurwls223@apache.org>
Author: hyukjinkwon <gurwls223@gmail.com>
Closes#20877 from HyukjinKwon/linesep-json.
## What changes were proposed in this pull request?
This PR migrate micro batch rate source to V2 API and rewrite UTs to suite V2 test.
## How was this patch tested?
UTs.
Author: jerryshao <sshao@hortonworks.com>
Closes#20688 from jerryshao/SPARK-23096.
## What changes were proposed in this pull request?
The UUID() expression is stateful and should implement the `Stateful` trait instead of the `Nondeterministic` trait.
## How was this patch tested?
Added test.
Author: Liang-Chi Hsieh <viirya@gmail.com>
Closes#20912 from viirya/SPARK-23794.
## What changes were proposed in this pull request?
This PR fixes an incorrect comparison in SQL between timestamp and date. This is because both of them are casted to `string` and then are compared lexicographically. This implementation shows `false` regarding this query `spark.sql("select cast('2017-03-01 00:00:00' as timestamp) between cast('2017-02-28' as date) and cast('2017-03-01' as date)").show`.
This PR shows `true` for this query by casting `date("2017-03-01")` to `timestamp("2017-03-01 00:00:00")`.
(Please fill in changes proposed in this fix)
## How was this patch tested?
Added new UTs to `TypeCoercionSuite`.
Author: Kazuaki Ishizaki <ishizaki@jp.ibm.com>
Closes#20774 from kiszk/SPARK-23549.
## What changes were proposed in this pull request?
This pr added TPCDS v2.7 (latest) queries in `TPCDSQuerySuite` because the current `TPCDSQuerySuite` tests older one (v1.4) and some queries are different from v1.4 and v2.7. Since the original v2.7 queries have the syntaxes that Spark cannot parse, I changed these queries in a following way:
- [date] + 14 days -> date + `INTERVAL` 14 days
- [column name] as "30 days" -> [column name] as \`30 days\`
- Fix some syntax errors, e.g., missing brackets
## How was this patch tested?
Added tests in `TPCDSQuerySuite`.
Author: Takeshi Yamamuro <yamamuro@apache.org>
Closes#20343 from maropu/TPCDSV2_7.
## What changes were proposed in this pull request?
The serializability test uses the same MemoryStream instance for 3 different queries. If any of those queries ask it to commit before the others have run, the rest will see empty dataframes. This can fail the test if q3 is affected.
We should use one instance per query instead.
## How was this patch tested?
Existing unit test. If I move q2.processAllAvailable() before starting q3, the test always fails without the fix.
Author: Jose Torres <torres.joseph.f+github@gmail.com>
Closes#20896 from jose-torres/fixrace.
## What changes were proposed in this pull request?
We should provide customized canonicalize plan for `InMemoryRelation` and `InMemoryTableScanExec`. Otherwise, we can wrongly treat two different cached plans as same result. It causes wrongly reused exchange then.
For a test query like this:
```scala
val cached = spark.createDataset(Seq(TestDataUnion(1, 2, 3), TestDataUnion(4, 5, 6))).cache()
val group1 = cached.groupBy("x").agg(min(col("y")) as "value")
val group2 = cached.groupBy("x").agg(min(col("z")) as "value")
group1.union(group2)
```
Canonicalized plans before:
First exchange:
```
Exchange hashpartitioning(none#0, 5)
+- *(1) HashAggregate(keys=[none#0], functions=[partial_min(none#1)], output=[none#0, none#4])
+- *(1) InMemoryTableScan [none#0, none#1]
+- InMemoryRelation [x#4253, y#4254, z#4255], true, 10000, StorageLevel(disk, memory, deserialized, 1 replicas)
+- LocalTableScan [x#4253, y#4254, z#4255]
```
Second exchange:
```
Exchange hashpartitioning(none#0, 5)
+- *(3) HashAggregate(keys=[none#0], functions=[partial_min(none#1)], output=[none#0, none#4])
+- *(3) InMemoryTableScan [none#0, none#1]
+- InMemoryRelation [x#4253, y#4254, z#4255], true, 10000, StorageLevel(disk, memory, deserialized, 1 replicas)
+- LocalTableScan [x#4253, y#4254, z#4255]
```
You can find that they have the canonicalized plans are the same, although we use different columns in two `InMemoryTableScan`s.
Canonicalized plan after:
First exchange:
```
Exchange hashpartitioning(none#0, 5)
+- *(1) HashAggregate(keys=[none#0], functions=[partial_min(none#1)], output=[none#0, none#4])
+- *(1) InMemoryTableScan [none#0, none#1]
+- InMemoryRelation [none#0, none#1, none#2], true, 10000, StorageLevel(memory, 1 replicas)
+- LocalTableScan [none#0, none#1, none#2]
```
Second exchange:
```
Exchange hashpartitioning(none#0, 5)
+- *(3) HashAggregate(keys=[none#0], functions=[partial_min(none#1)], output=[none#0, none#4])
+- *(3) InMemoryTableScan [none#0, none#2]
+- InMemoryRelation [none#0, none#1, none#2], true, 10000, StorageLevel(memory, 1 replicas)
+- LocalTableScan [none#0, none#1, none#2]
```
## How was this patch tested?
Added unit test.
Author: Liang-Chi Hsieh <viirya@gmail.com>
Closes#20831 from viirya/SPARK-23614.
## What changes were proposed in this pull request?
As stated in Jira, there are problems with current `Uuid` expression which uses `java.util.UUID.randomUUID` for UUID generation.
This patch uses the newly added `RandomUUIDGenerator` for UUID generation. So we can make `Uuid` deterministic between retries.
## How was this patch tested?
Added unit tests.
Author: Liang-Chi Hsieh <viirya@gmail.com>
Closes#20861 from viirya/SPARK-23599-2.
## What changes were proposed in this pull request?
Currently we allow writing data frames with empty schema into a file based datasource for certain file formats such as JSON, ORC etc. For formats such as Parquet and Text, we raise error at different times of execution. For text format, we return error from the driver early on in processing where as for format such as parquet, the error is raised from executor.
**Example**
spark.emptyDataFrame.write.format("parquet").mode("overwrite").save(path)
**Results in**
``` SQL
org.apache.parquet.schema.InvalidSchemaException: Cannot write a schema with an empty group: message spark_schema {
}
at org.apache.parquet.schema.TypeUtil$1.visit(TypeUtil.java:27)
at org.apache.parquet.schema.TypeUtil$1.visit(TypeUtil.java:37)
at org.apache.parquet.schema.MessageType.accept(MessageType.java:58)
at org.apache.parquet.schema.TypeUtil.checkValidWriteSchema(TypeUtil.java:23)
at org.apache.parquet.hadoop.ParquetFileWriter.<init>(ParquetFileWriter.java:225)
at org.apache.parquet.hadoop.ParquetOutputFormat.getRecordWriter(ParquetOutputFormat.java:342)
at org.apache.parquet.hadoop.ParquetOutputFormat.getRecordWriter(ParquetOutputFormat.java:302)
at org.apache.spark.sql.execution.datasources.parquet.ParquetOutputWriter.<init>(ParquetOutputWriter.scala:37)
at org.apache.spark.sql.execution.datasources.parquet.ParquetFileFormat$$anon$1.newInstance(ParquetFileFormat.scala:151)
at org.apache.spark.sql.execution.datasources.FileFormatWriter$SingleDirectoryWriteTask.newOutputWriter(FileFormatWriter.scala:376)
at org.apache.spark.sql.execution.datasources.FileFormatWriter$SingleDirectoryWriteTask.execute(FileFormatWriter.scala:387)
at org.apache.spark.sql.execution.datasources.FileFormatWriter$$anonfun$org$apache$spark$sql$execution$datasources$FileFormatWriter$$executeTask$3.apply(FileFormatWriter.scala:278)
at org.apache.spark.sql.execution.datasources.FileFormatWriter$$anonfun$org$apache$spark$sql$execution$datasources$FileFormatWriter$$executeTask$3.apply(FileFormatWriter.scala:276)
at org.apache.spark.util.Utils$.tryWithSafeFinallyAndFailureCallbacks(Utils.scala:1411)
at org.apache.spark.sql.execution.datasources.FileFormatWriter$.org$apache$spark$sql$execution$datasources$FileFormatWriter$$executeTask(FileFormatWriter.scala:281)
at org.apache.spark.sql.execution.datasources.FileFormatWriter$$anonfun$write$1.apply(FileFormatWriter.scala:206)
at org.apache.spark.sql.execution.datasources.FileFormatWriter$$anonfun$write$1.apply(FileFormatWriter.scala:205)
at org.apache.spark.scheduler.ResultTask.runTask(ResultTask.scala:87)
at org.apache.spark.scheduler.Task.run(Task.scala:109)
at org.apache.spark.executor.Executor$TaskRunner.run(Executor.scala:345)
at java.util.concurrent.ThreadPoolExecutor.runWorker(ThreadPoolExecutor.java:1142)
at java.util.concurrent.ThreadPoolExecutor$Worker.run(ThreadPoolExecutor.java:617)
at java.lang.Thread.run(Thread.
```
In this PR, we unify the error processing and raise error on attempt to write empty schema based dataframes into file based datasource (orc, parquet, text , csv, json etc) early on in the processing.
## How was this patch tested?
Unit tests added in FileBasedDatasourceSuite.
Author: Dilip Biswal <dbiswal@us.ibm.com>
Closes#20579 from dilipbiswal/spark-23372.
## What changes were proposed in this pull request?
Fixed `CodegenContext.withSubExprEliminationExprs()` so that it saves/restores CSE state correctly.
## How was this patch tested?
Added new unit test to verify that the old CSE state is indeed saved and restored around the `withSubExprEliminationExprs()` call. Manually verified that this test fails without this patch.
Author: Kris Mok <kris.mok@databricks.com>
Closes#20870 from rednaxelafx/codegen-subexpr-fix.
## What changes were proposed in this pull request?
Output metrics were not filled when parquet sink used.
This PR fixes this problem by passing a `BasicWriteJobStatsTracker` in `FileStreamSink`.
## How was this patch tested?
Additional unit test added.
Author: Gabor Somogyi <gabor.g.somogyi@gmail.com>
Closes#20745 from gaborgsomogyi/SPARK-23288.
## What changes were proposed in this pull request?
To fix `scala.MatchError` in `literals.sql.out`, this pr added an entry for `CalendarIntervalType` in `QueryExecution.toHiveStructString`.
## How was this patch tested?
Existing tests and added tests in `literals.sql`
Author: Takeshi Yamamuro <yamamuro@apache.org>
Closes#20872 from maropu/FixIntervalTests.
## What changes were proposed in this pull request?
This PR proposes to add `lineSep` option for a configurable line separator in text datasource.
It supports this option by using `LineRecordReader`'s functionality with passing it to the constructor.
## How was this patch tested?
Manual tests and unit tests were added.
Author: hyukjinkwon <gurwls223@gmail.com>
Closes#20727 from HyukjinKwon/linesep-text.
## What changes were proposed in this pull request?
To drop `exprId`s for `Alias` in user-facing info., this pr added an entry for `Alias` in `NonSQLExpression.sql`
## How was this patch tested?
Added tests in `UDFSuite`.
Author: Takeshi Yamamuro <yamamuro@apache.org>
Closes#20827 from maropu/SPARK-23666.
## What changes were proposed in this pull request?
Complex type simplification optimizer rules were not applied to the
entire plan, just the expressions reachable from the root node. This
patch fixes the rules to transform the entire plan.
## How was this patch tested?
New unit test + ran sql / core tests.
Author: Henry Robinson <henry@apache.org>
Author: Henry Robinson <henry@cloudera.com>
Closes#20687 from henryr/spark-25000.
## What changes were proposed in this pull request?
Report SinglePartition in DataSourceV2ScanExec when there's exactly 1 data reader factory.
Note that this means reader factories end up being constructed as partitioning is checked; let me know if you think that could be a problem.
## How was this patch tested?
existing unit tests
Author: Jose Torres <jose@databricks.com>
Author: Jose Torres <torres.joseph.f+github@gmail.com>
Closes#20726 from jose-torres/SPARK-23574.
## What changes were proposed in this pull request?
This patch adds a UUID generator from Pseudo-Random Numbers. We can use it later to have deterministic `UUID()` expression.
## How was this patch tested?
Added unit tests.
Author: Liang-Chi Hsieh <viirya@gmail.com>
Closes#20817 from viirya/SPARK-23599.
## What changes were proposed in this pull request?
We currently can only create unsafe rows using code generation. This is a problem for situations in which code generation fails. There is no fallback, and as a result we cannot execute the query.
This PR adds an interpreted version of `UnsafeProjection`. The implementation is modeled after `InterpretedMutableProjection`. It stores the expression results in a `GenericInternalRow`, and it then uses a conversion function to convert the `GenericInternalRow` into an `UnsafeRow`.
This PR does not implement the actual code generated to interpreted fallback logic. This will be done in a follow-up.
## How was this patch tested?
I am piggybacking on exiting `UnsafeProjection` tests, and I have added an interpreted version for each of these.
Author: Herman van Hovell <hvanhovell@databricks.com>
Closes#20750 from hvanhovell/SPARK-23581.
## What changes were proposed in this pull request?
Currently, some tests have an assumption that `spark.sql.sources.default=parquet`. In fact, that is a correct assumption, but that assumption makes it difficult to test new data source format.
This PR aims to
- Improve test suites more robust and makes it easy to test new data sources in the future.
- Test new native ORC data source with the full existing Apache Spark test coverage.
As an example, the PR uses `spark.sql.sources.default=orc` during reviews. The value should be `parquet` when this PR is accepted.
## How was this patch tested?
Pass the Jenkins with updated tests.
Author: Dongjoon Hyun <dongjoon@apache.org>
Closes#20705 from dongjoon-hyun/SPARK-23553.
Clean up SparkPlanGraphWrapper objects from InMemoryStore together with cleaning up SQLExecutionUIData
existing unit test was extended to check also SparkPlanGraphWrapper object count
vanzin
Author: myroslavlisniak <acnipin@gmail.com>
Closes#20813 from myroslavlisniak/master.
## What changes were proposed in this pull request?
As discussion in #20675, we need add a new interface `ContinuousDataReaderFactory` to support the requirements of setting start offset in Continuous Processing.
## How was this patch tested?
Existing UT.
Author: Yuanjian Li <xyliyuanjian@gmail.com>
Closes#20689 from xuanyuanking/SPARK-23533.
## What changes were proposed in this pull request?
This PR fixes a minor issue in `HadoopFsRelationTest`, that you should create table using `dataSourceName` instead of `parquet`. The issue won't affect the correctness, but it will generate wrong error message in case the test fails.
## How was this patch tested?
Exsiting tests.
Author: Xingbo Jiang <xingbo.jiang@databricks.com>
Closes#20780 from jiangxb1987/dataSourceName.
## What changes were proposed in this pull request?
This PR enables assertions in `XXH64Suite.testKnownByteArrayInputs()` on big endian platform, too. The current implementation performs them only on little endian platform. This PR increase test coverage of big endian platform.
## How was this patch tested?
Updated `XXH64Suite`
Tested on big endian platform using JIT compiler or interpreter `-Xint`.
Author: Kazuaki Ishizaki <ishizaki@jp.ibm.com>
Closes#20804 from kiszk/SPARK-23656.
## What changes were proposed in this pull request?
The error message ```s"""Field "$name" does not exist."""``` is thrown when looking up an unknown field in StructType. In the error message, we should also contain the information about which columns/fields exist in this struct.
## How was this patch tested?
Added new unit tests.
Note: I created a new `StructTypeSuite.scala` as I couldn't find an existing suite that's suitable to place these tests. I may be missing something so feel free to propose new locations.
Please review http://spark.apache.org/contributing.html before opening a pull request.
Author: Xiayun Sun <xiayunsun@gmail.com>
Closes#20649 from xysun/SPARK-23462.
## What changes were proposed in this pull request?
Revise doc of method pushFilters in SupportsPushDownFilters/SupportsPushDownCatalystFilters
In `FileSourceStrategy`, except `partitionKeyFilters`(the references of which is subset of partition keys), all filters needs to be evaluated after scanning. Otherwise, Spark will get wrong result from data sources like Orc/Parquet.
This PR is to improve the doc.
Author: Wang Gengliang <gengliang.wang@databricks.com>
Closes#20769 from gengliangwang/revise_pushdown_doc.
## What changes were proposed in this pull request?
The from_json() function accepts an additional parameter, where the user might specify the schema. The issue is that the specified schema might not be compatible with data. In particular, the JSON data might be missing data for fields declared as non-nullable in the schema. The from_json() function does not verify the data against such errors. When data with missing fields is sent to the parquet encoder, there is no verification either. The end results is a corrupt parquet file.
To avoid corruptions, make sure that all fields in the user-specified schema are set to be nullable.
Since this changes the behavior of a public function, we need to include it in release notes.
The behavior can be reverted by setting `spark.sql.fromJsonForceNullableSchema=false`
## How was this patch tested?
Added two new tests.
Author: Michał Świtakowski <michal.switakowski@databricks.com>
Closes#20694 from mswit-databricks/SPARK-23173.
## What changes were proposed in this pull request?
Below are the two cases.
``` SQL
case 1
scala> List.empty[String].toDF().rdd.partitions.length
res18: Int = 1
```
When we write the above data frame as parquet, we create a parquet file containing
just the schema of the data frame.
Case 2
``` SQL
scala> val anySchema = StructType(StructField("anyName", StringType, nullable = false) :: Nil)
anySchema: org.apache.spark.sql.types.StructType = StructType(StructField(anyName,StringType,false))
scala> spark.read.schema(anySchema).csv("/tmp/empty_folder").rdd.partitions.length
res22: Int = 0
```
For the 2nd case, since number of partitions = 0, we don't call the write task (the task has logic to create the empty metadata only parquet file)
The fix is to create a dummy single partition RDD and set up the write task based on it to ensure
the metadata-only file.
## How was this patch tested?
A new test is added to DataframeReaderWriterSuite.
Author: Dilip Biswal <dbiswal@us.ibm.com>
Closes#20525 from dilipbiswal/spark-23271.
## What changes were proposed in this pull request?
`PrintToStderr` was doing what is it supposed to only when code generation is enabled.
The PR adds the same behavior in interpreted mode too.
## How was this patch tested?
added UT
Author: Marco Gaido <marcogaido91@gmail.com>
Closes#20773 from mgaido91/SPARK-23602.
## What changes were proposed in this pull request?
There was a bug in `calculateParamLength` which caused it to return always 1 + the number of expressions. This could lead to Exceptions especially with expressions of type long.
## How was this patch tested?
added UT + fixed previous UT
Author: Marco Gaido <marcogaido91@gmail.com>
Closes#20772 from mgaido91/SPARK-23628.
## What changes were proposed in this pull request?
The PR adds interpreted execution to DecodeUsingSerializer.
## How was this patch tested?
added UT
Please review http://spark.apache.org/contributing.html before opening a pull request.
Author: Marco Gaido <marcogaido91@gmail.com>
Closes#20760 from mgaido91/SPARK-23592.
## What changes were proposed in this pull request?
This PR proposes to support an alternative function from with group aggregate pandas UDF.
The current form:
```
def foo(pdf):
return ...
```
Takes a single arg that is a pandas DataFrame.
With this PR, an alternative form is supported:
```
def foo(key, pdf):
return ...
```
The alternative form takes two argument - a tuple that presents the grouping key, and a pandas DataFrame represents the data.
## How was this patch tested?
GroupbyApplyTests
Author: Li Jin <ice.xelloss@gmail.com>
Closes#20295 from icexelloss/SPARK-23011-groupby-apply-key.
## What changes were proposed in this pull request?
This PR adds a configuration to control the fallback of Arrow optimization for `toPandas` and `createDataFrame` with Pandas DataFrame.
## How was this patch tested?
Manually tested and unit tests added.
You can test this by:
**`createDataFrame`**
```python
spark.conf.set("spark.sql.execution.arrow.enabled", False)
pdf = spark.createDataFrame([[{'a': 1}]]).toPandas()
spark.conf.set("spark.sql.execution.arrow.enabled", True)
spark.conf.set("spark.sql.execution.arrow.fallback.enabled", True)
spark.createDataFrame(pdf, "a: map<string, int>")
```
```python
spark.conf.set("spark.sql.execution.arrow.enabled", False)
pdf = spark.createDataFrame([[{'a': 1}]]).toPandas()
spark.conf.set("spark.sql.execution.arrow.enabled", True)
spark.conf.set("spark.sql.execution.arrow.fallback.enabled", False)
spark.createDataFrame(pdf, "a: map<string, int>")
```
**`toPandas`**
```python
spark.conf.set("spark.sql.execution.arrow.enabled", True)
spark.conf.set("spark.sql.execution.arrow.fallback.enabled", True)
spark.createDataFrame([[{'a': 1}]]).toPandas()
```
```python
spark.conf.set("spark.sql.execution.arrow.enabled", True)
spark.conf.set("spark.sql.execution.arrow.fallback.enabled", False)
spark.createDataFrame([[{'a': 1}]]).toPandas()
```
Author: hyukjinkwon <gurwls223@gmail.com>
Closes#20678 from HyukjinKwon/SPARK-23380-conf.
## What changes were proposed in this pull request?
The following query doesn't work as expected:
```
CREATE EXTERNAL TABLE ext_table(a STRING, b INT, c STRING) PARTITIONED BY (d STRING)
LOCATION 'sql/core/spark-warehouse/ext_table';
ALTER TABLE ext_table CHANGE a a STRING COMMENT "new comment";
DESC ext_table;
```
The comment of column `a` is not updated, that's because `HiveExternalCatalog.doAlterTable` ignores table schema changes. To fix the issue, we should call `doAlterTableDataSchema` instead of `doAlterTable`.
## How was this patch tested?
Updated `DDLSuite.testChangeColumn`.
Author: Xingbo Jiang <xingbo.jiang@databricks.com>
Closes#20696 from jiangxb1987/alterColumnComment.
A few different things going on:
- Remove unused methods.
- Move JSON methods to the only class that uses them.
- Move test-only methods to TestUtils.
- Make getMaxResultSize() a config constant.
- Reuse functionality from existing libraries (JRE or JavaUtils) where possible.
The change also includes changes to a few tests to call `Utils.createTempFile` correctly,
so that temp dirs are created under the designated top-level temp dir instead of
potentially polluting git index.
Author: Marcelo Vanzin <vanzin@cloudera.com>
Closes#20706 from vanzin/SPARK-23550.
## What changes were proposed in this pull request?
The PR adds interpreted execution to EncodeUsingSerializer.
## How was this patch tested?
added UT
Author: Marco Gaido <marcogaido91@gmail.com>
Closes#20751 from mgaido91/SPARK-23591.
## What changes were proposed in this pull request?
This pr added a helper function in `ExpressionEvalHelper` to check exceptions in all the path of expression evaluation.
## How was this patch tested?
Modified the existing tests.
Author: Takeshi Yamamuro <yamamuro@apache.org>
Closes#20748 from maropu/SPARK-23611.
## What changes were proposed in this pull request?
The PR adds interpreted execution to CreateExternalRow
## How was this patch tested?
added UT
Author: Marco Gaido <marcogaido91@gmail.com>
Closes#20749 from mgaido91/SPARK-23590.
## What changes were proposed in this pull request?
This pr added interpreted execution for `GetExternalRowField`.
## How was this patch tested?
Added tests in `ObjectExpressionsSuite`.
Author: Takeshi Yamamuro <yamamuro@apache.org>
Closes#20746 from maropu/SPARK-23594.
…lValue
## What changes were proposed in this pull request?
Parquet 1.9 will change the semantics of Statistics.isEmpty slightly
to reflect if the null value count has been set. That breaks a
timestamp interoperability test that cares only about whether there
are column values present in the statistics of a written file for an
INT96 column. Fix by using Statistics.hasNonNullValue instead.
## How was this patch tested?
Unit tests continue to pass against Parquet 1.8, and also pass against
a Parquet build including PARQUET-1217.
Author: Henry Robinson <henry@cloudera.com>
Closes#20740 from henryr/spark-23604.
## What changes were proposed in this pull request?
The PR adds interpreted execution to WrapOption.
## How was this patch tested?
added UT
Author: Marco Gaido <marcogaido91@gmail.com>
Closes#20741 from mgaido91/SPARK-23586_2.
## What changes were proposed in this pull request?
Add an epoch ID argument to DataWriterFactory for use in streaming. As a side effect of passing in this value, DataWriter will now have a consistent lifecycle; commit() or abort() ends the lifecycle of a DataWriter instance in any execution mode.
I considered making a separate streaming interface and adding the epoch ID only to that one, but I think it requires a lot of extra work for no real gain. I think it makes sense to define epoch 0 as the one and only epoch of a non-streaming query.
## How was this patch tested?
existing unit tests
Author: Jose Torres <jose@databricks.com>
Closes#20710 from jose-torres/api2.
## What changes were proposed in this pull request?
The PR adds interpreted execution to UnwrapOption.
## How was this patch tested?
added UT
Author: Marco Gaido <marcogaido91@gmail.com>
Closes#20736 from mgaido91/SPARK-23586.
## What changes were proposed in this pull request?
Provide more details in trigonometric function documentations. Referenced `java.lang.Math` for further details in the descriptions.
## How was this patch tested?
Ran full build, checked generated documentation manually
Author: Mihaly Toth <misutoth@gmail.com>
Closes#20618 from misutoth/trigonometric-doc.
## What changes were proposed in this pull request?
A current `CodegenContext` class has immutable value or method without mutable state, too.
This refactoring moves them to `CodeGenerator` object class which can be accessed from anywhere without an instantiated `CodegenContext` in the program.
## How was this patch tested?
Existing tests
Author: Kazuaki Ishizaki <ishizaki@jp.ibm.com>
Closes#20700 from kiszk/SPARK-23546.
## What changes were proposed in this pull request?
It looks like this was incorrectly copied from `XPathFloat` in the class above.
## How was this patch tested?
(Please explain how this patch was tested. E.g. unit tests, integration tests, manual tests)
(If this patch involves UI changes, please attach a screenshot; otherwise, remove this)
Please review http://spark.apache.org/contributing.html before opening a pull request.
Author: Eric Liang <ekhliang@gmail.com>
Closes#20730 from ericl/fix-typo-xpath.
## What changes were proposed in this pull request?
In https://github.com/apache/spark/pull/20679 I missed a few places in SQL tests.
For hygiene, they should also use the sessionState interface where possible.
## How was this patch tested?
Modified existing tests.
Author: Juliusz Sompolski <julek@databricks.com>
Closes#20718 from juliuszsompolski/SPARK-23514-followup.
## What changes were proposed in this pull request?
Add Spark 2.3.0 in HiveExternalCatalogVersionsSuite since Spark 2.3.0 is released for ensuring backward compatibility.
## How was this patch tested?
N/A
Author: gatorsmile <gatorsmile@gmail.com>
Closes#20720 from gatorsmile/add2.3.
## What changes were proposed in this pull request?
This PR moves structured streaming text socket source to V2.
Questions: do we need to remove old "socket" source?
## How was this patch tested?
Unit test and manual verification.
Author: jerryshao <sshao@hortonworks.com>
Closes#20382 from jerryshao/SPARK-23097.
## What changes were proposed in this pull request?
https://github.com/apache/spark/pull/18944 added one patch, which allowed a spark session to be created when the hive metastore server is down. However, it did not allow running any commands with the spark session. This brings troubles to the user who only wants to read / write data frames without metastore setup.
## How was this patch tested?
Added some unit tests to read and write data frames based on the original HiveMetastoreLazyInitializationSuite.
Please review http://spark.apache.org/contributing.html before opening a pull request.
Author: Feng Liu <fengliu@databricks.com>
Closes#20681 from liufengdb/completely-lazy.
## What changes were proposed in this pull request?
(Please fill in changes proposed in this fix)
I run a sql: `select ls.cs_order_number from ls left semi join catalog_sales cs on ls.cs_order_number = cs.cs_order_number`, The `ls` table is a small table ,and the number is one. The `catalog_sales` table is a big table, and the number is 10 billion. The task will be hang up. And i find the many null values of `cs_order_number` in the `catalog_sales` table. I think the null value should be removed in the logical plan.
>== Optimized Logical Plan ==
>Join LeftSemi, (cs_order_number#1 = cs_order_number#22)
>:- Project cs_order_number#1
> : +- Filter isnotnull(cs_order_number#1)
> : +- MetastoreRelation 100t, ls
>+- Project cs_order_number#22
> +- MetastoreRelation 100t, catalog_sales
Now, use this patch, the plan will be:
>== Optimized Logical Plan ==
>Join LeftSemi, (cs_order_number#1 = cs_order_number#22)
>:- Project cs_order_number#1
> : +- Filter isnotnull(cs_order_number#1)
> : +- MetastoreRelation 100t, ls
>+- Project cs_order_number#22
> : **+- Filter isnotnull(cs_order_number#22)**
> :+- MetastoreRelation 100t, catalog_sales
## How was this patch tested?
(Please explain how this patch was tested. E.g. unit tests, integration tests, manual tests)
(If this patch involves UI changes, please attach a screenshot; otherwise, remove this)
Please review http://spark.apache.org/contributing.html before opening a pull request.
Author: KaiXinXiaoLei <584620569@qq.com>
Author: hanghang <584620569@qq.com>
Closes#20670 from KaiXinXiaoLei/Spark-23405.
## What changes were proposed in this pull request?
This is based on https://github.com/apache/spark/pull/20668 for supporting Hive 2.2 and Hive 2.3 metastore.
When we merge the PR, we should give the major credit to wangyum
## How was this patch tested?
Added the test cases
Author: Yuming Wang <yumwang@ebay.com>
Author: gatorsmile <gatorsmile@gmail.com>
Closes#20671 from gatorsmile/pr-20668.
## What changes were proposed in this pull request?
Inside `OptimizeMetadataOnlyQuery.getPartitionAttrs`, avoid using `zip` to generate attribute map.
Also include other minor update of comments and format.
## How was this patch tested?
Existing test cases.
Author: Xingbo Jiang <xingbo.jiang@databricks.com>
Closes#20693 from jiangxb1987/SPARK-23523.
## What changes were proposed in this pull request?
A few places in `spark-sql` were using `sc.hadoopConfiguration` directly. They should be using `sessionState.newHadoopConf()` to blend in configs that were set through `SQLConf`.
Also, for better UX, for these configs blended in from `SQLConf`, we should consider removing the `spark.hadoop` prefix, so that the settings are recognized whether or not they were specified by the user.
## How was this patch tested?
Tested that AlterTableRecoverPartitions now correctly recognizes settings that are passed in to the FileSystem through SQLConf.
Author: Juliusz Sompolski <julek@databricks.com>
Closes#20679 from juliuszsompolski/SPARK-23514.
## What changes were proposed in this pull request?
Clarify JSON and CSV reader behavior in document.
JSON doesn't support partial results for corrupted records.
CSV only supports partial results for the records with more or less tokens.
## How was this patch tested?
Pass existing tests.
Author: Liang-Chi Hsieh <viirya@gmail.com>
Closes#20666 from viirya/SPARK-23448-2.
## What changes were proposed in this pull request?
```Scala
val tablePath = new File(s"${path.getCanonicalPath}/cOl3=c/cOl1=a/cOl5=e")
Seq(("a", "b", "c", "d", "e")).toDF("cOl1", "cOl2", "cOl3", "cOl4", "cOl5")
.write.json(tablePath.getCanonicalPath)
val df = spark.read.json(path.getCanonicalPath).select("CoL1", "CoL5", "CoL3").distinct()
df.show()
```
It generates a wrong result.
```
[c,e,a]
```
We have a bug in the rule `OptimizeMetadataOnlyQuery `. We should respect the attribute order in the original leaf node. This PR is to fix it.
## How was this patch tested?
Added a test case
Author: gatorsmile <gatorsmile@gmail.com>
Closes#20684 from gatorsmile/optimizeMetadataOnly.
## What changes were proposed in this pull request?
Refactor ColumnStat to be more flexible.
* Split `ColumnStat` and `CatalogColumnStat` just like `CatalogStatistics` is split from `Statistics`. This detaches how the statistics are stored from how they are processed in the query plan. `CatalogColumnStat` keeps `min` and `max` as `String`, making it not depend on dataType information.
* For `CatalogColumnStat`, parse column names from property names in the metastore (`KEY_VERSION` property), not from metastore schema. This means that `CatalogColumnStat`s can be created for columns even if the schema itself is not stored in the metastore.
* Make all fields optional. `min`, `max` and `histogram` for columns were optional already. Having them all optional is more consistent, and gives flexibility to e.g. drop some of the fields through transformations if they are difficult / impossible to calculate.
The added flexibility will make it possible to have alternative implementations for stats, and separates stats collection from stats and estimation processing in plans.
## How was this patch tested?
Refactored existing tests to work with refactored `ColumnStat` and `CatalogColumnStat`.
New tests added in `StatisticsSuite` checking that backwards / forwards compatibility is not broken.
Author: Juliusz Sompolski <julek@databricks.com>
Closes#20624 from juliuszsompolski/SPARK-23445.
## What changes were proposed in this pull request?
Remove queryExecutionThread.interrupt() from ContinuousExecution. As detailed in the JIRA, interrupting the thread is only relevant in the microbatch case; for continuous processing the query execution can quickly clean itself up without.
## How was this patch tested?
existing tests
Author: Jose Torres <jose@databricks.com>
Closes#20622 from jose-torres/SPARK-23441.
## What changes were proposed in this pull request?
This PR avoids to print schema internal information when unknown column is specified in partition columns. This PR prints column names in the schema with more readable format.
The following is an example.
Source code
```
test("save with an unknown partition column") {
withTempDir { dir =>
val path = dir.getCanonicalPath
Seq(1L -> "a").toDF("i", "j").write
.format("parquet")
.partitionBy("unknownColumn")
.save(path)
}
```
Output without this PR
```
Partition column unknownColumn not found in schema StructType(StructField(i,LongType,false), StructField(j,StringType,true));
```
Output with this PR
```
Partition column unknownColumn not found in schema struct<i:bigint,j:string>;
```
## How was this patch tested?
Manually tested
Author: Kazuaki Ishizaki <ishizaki@jp.ibm.com>
Closes#20653 from kiszk/SPARK-23459.
**The best way to review this PR is to ignore whitespace/indent changes. Use this link - https://github.com/apache/spark/pull/20650/files?w=1**
## What changes were proposed in this pull request?
The stream-stream join tests add data to multiple sources and expect it all to show up in the next batch. But there's a race condition; the new batch might trigger when only one of the AddData actions has been reached.
Prior attempt to solve this issue by jose-torres in #20646 attempted to simultaneously synchronize on all memory sources together when consecutive AddData was found in the actions. However, this carries the risk of deadlock as well as unintended modification of stress tests (see the above PR for a detailed explanation). Instead, this PR attempts the following.
- A new action called `StreamProgressBlockedActions` that allows multiple actions to be executed while the streaming query is blocked from making progress. This allows data to be added to multiple sources that are made visible simultaneously in the next batch.
- An alias of `StreamProgressBlockedActions` called `MultiAddData` is explicitly used in the `Streaming*JoinSuites` to add data to two memory sources simultaneously.
This should avoid unintentional modification of the stress tests (or any other test for that matter) while making sure that the flaky tests are deterministic.
## How was this patch tested?
Modified test cases in `Streaming*JoinSuites` where there are consecutive `AddData` actions.
Author: Tathagata Das <tathagata.das1565@gmail.com>
Closes#20650 from tdas/SPARK-23408.
## What changes were proposed in this pull request?
For CreateTable with Append mode, we should check if `storage.locationUri` is the same with existing table in `PreprocessTableCreation`
In the current code, there is only a simple exception if the `storage.locationUri` is different with existing table:
`org.apache.spark.sql.AnalysisException: Table or view not found:`
which can be improved.
## How was this patch tested?
Unit test
Author: Wang Gengliang <gengliang.wang@databricks.com>
Closes#20660 from gengliangwang/locationUri.
## What changes were proposed in this pull request?
DataSourceV2 initially allowed user-supplied schemas when a source doesn't implement `ReadSupportWithSchema`, as long as the schema was identical to the source's schema. This is confusing behavior because changes to an underlying table can cause a previously working job to fail with an exception that user-supplied schemas are not allowed.
This reverts commit adcb25a0624, which was added to #20387 so that it could be removed in a separate JIRA issue and PR.
## How was this patch tested?
Existing tests.
Author: Ryan Blue <blue@apache.org>
Closes#20603 from rdblue/SPARK-23418-revert-adcb25a0624.
## What changes were proposed in this pull request?
This PR always adds `codegenStageId` in comment of the generated class. This is a replication of #20419 for post-Spark 2.3.
Closes#20419
```
/* 001 */ public Object generate(Object[] references) {
/* 002 */ return new GeneratedIteratorForCodegenStage1(references);
/* 003 */ }
/* 004 */
/* 005 */ // codegenStageId=1
/* 006 */ final class GeneratedIteratorForCodegenStage1 extends org.apache.spark.sql.execution.BufferedRowIterator {
/* 007 */ private Object[] references;
...
```
## How was this patch tested?
Existing tests
Author: Kazuaki Ishizaki <ishizaki@jp.ibm.com>
Closes#20612 from kiszk/SPARK-23424.
## What changes were proposed in this pull request?
In a kerberized cluster, when Spark reads a file path (e.g. `people.json`), it warns with a wrong warning message during looking up `people.json/_spark_metadata`. The root cause of this situation is the difference between `LocalFileSystem` and `DistributedFileSystem`. `LocalFileSystem.exists()` returns `false`, but `DistributedFileSystem.exists` raises `org.apache.hadoop.security.AccessControlException`.
```scala
scala> spark.version
res0: String = 2.4.0-SNAPSHOT
scala> spark.read.json("file:///usr/hdp/current/spark-client/examples/src/main/resources/people.json").show
+----+-------+
| age| name|
+----+-------+
|null|Michael|
| 30| Andy|
| 19| Justin|
+----+-------+
scala> spark.read.json("hdfs:///tmp/people.json")
18/02/15 05:00:48 WARN streaming.FileStreamSink: Error while looking for metadata directory.
18/02/15 05:00:48 WARN streaming.FileStreamSink: Error while looking for metadata directory.
```
After this PR,
```scala
scala> spark.read.json("hdfs:///tmp/people.json").show
+----+-------+
| age| name|
+----+-------+
|null|Michael|
| 30| Andy|
| 19| Justin|
+----+-------+
```
## How was this patch tested?
Manual.
Author: Dongjoon Hyun <dongjoon@apache.org>
Closes#20616 from dongjoon-hyun/SPARK-23434.
## What changes were proposed in this pull request?
Apache Spark 2.3 introduced `native` ORC supports with vectorization and many fixes. However, it's shipped as a not-default option. This PR enables `native` ORC implementation and predicate-pushdown by default for Apache Spark 2.4. We will improve and stabilize ORC data source before Apache Spark 2.4. And, eventually, Apache Spark will drop old Hive-based ORC code.
## How was this patch tested?
Pass the Jenkins with existing tests.
Author: Dongjoon Hyun <dongjoon@apache.org>
Closes#20634 from dongjoon-hyun/SPARK-23456.
## What changes were proposed in this pull request?
SPARK-23203: DataSourceV2 should use immutable catalyst trees instead of wrapping a mutable DataSourceV2Reader. This commit updates DataSourceV2Relation and consolidates much of the DataSourceV2 API requirements for the read path in it. Instead of wrapping a reader that changes, the relation lazily produces a reader from its configuration.
This commit also updates the predicate and projection push-down. Instead of the implementation from SPARK-22197, this reuses the rule matching from the Hive and DataSource read paths (using `PhysicalOperation`) and copies most of the implementation of `SparkPlanner.pruneFilterProject`, with updates for DataSourceV2. By reusing the implementation from other read paths, this should have fewer regressions from other read paths and is less code to maintain.
The new push-down rules also supports the following edge cases:
* The output of DataSourceV2Relation should be what is returned by the reader, in case the reader can only partially satisfy the requested schema projection
* The requested projection passed to the DataSourceV2Reader should include filter columns
* The push-down rule may be run more than once if filters are not pushed through projections
## How was this patch tested?
Existing push-down and read tests.
Author: Ryan Blue <blue@apache.org>
Closes#20387 from rdblue/SPARK-22386-push-down-immutable-trees.
## What changes were proposed in this pull request?
Before the patch, Spark could infer as Date a partition value which cannot be casted to Date (this can happen when there are extra characters after a valid date, like `2018-02-15AAA`).
When this happens and the input format has metadata which define the schema of the table, then `null` is returned as a value for the partition column, because the `cast` operator used in (`PartitioningAwareFileIndex.inferPartitioning`) is unable to convert the value.
The PR checks in the partition inference that values can be casted to Date and Timestamp, in order to infer that datatype to them.
## How was this patch tested?
added UT
Author: Marco Gaido <marcogaido91@gmail.com>
Closes#20621 from mgaido91/SPARK-23436.
## What changes were proposed in this pull request?
ParquetFileFormat leaks opened files in some cases. This PR prevents that by registering task completion listers first before initialization.
- [spark-branch-2.3-test-sbt-hadoop-2.7](https://amplab.cs.berkeley.edu/jenkins/view/Spark%20QA%20Test%20(Dashboard)/job/spark-branch-2.3-test-sbt-hadoop-2.7/205/testReport/org.apache.spark.sql/FileBasedDataSourceSuite/_It_is_not_a_test_it_is_a_sbt_testing_SuiteSelector_/)
- [spark-master-test-sbt-hadoop-2.6](https://amplab.cs.berkeley.edu/jenkins/view/Spark%20QA%20Test%20(Dashboard)/job/spark-master-test-sbt-hadoop-2.6/4228/testReport/junit/org.apache.spark.sql.execution.datasources.parquet/ParquetQuerySuite/_It_is_not_a_test_it_is_a_sbt_testing_SuiteSelector_/)
```
Caused by: sbt.ForkMain$ForkError: java.lang.Throwable: null
at org.apache.spark.DebugFilesystem$.addOpenStream(DebugFilesystem.scala:36)
at org.apache.spark.DebugFilesystem.open(DebugFilesystem.scala:70)
at org.apache.hadoop.fs.FileSystem.open(FileSystem.java:769)
at org.apache.parquet.hadoop.ParquetFileReader.<init>(ParquetFileReader.java:538)
at org.apache.spark.sql.execution.datasources.parquet.SpecificParquetRecordReaderBase.initialize(SpecificParquetRecordReaderBase.java:149)
at org.apache.spark.sql.execution.datasources.parquet.VectorizedParquetRecordReader.initialize(VectorizedParquetRecordReader.java:133)
at org.apache.spark.sql.execution.datasources.parquet.ParquetFileFormat$$anonfun$buildReaderWithPartitionValues$1.apply(ParquetFileFormat.scala:400)
at
```
## How was this patch tested?
Manual. The following test case generates the same leakage.
```scala
test("SPARK-23457 Register task completion listeners first in ParquetFileFormat") {
withSQLConf(SQLConf.PARQUET_VECTORIZED_READER_BATCH_SIZE.key -> s"${Int.MaxValue}") {
withTempDir { dir =>
val basePath = dir.getCanonicalPath
Seq(0).toDF("a").write.format("parquet").save(new Path(basePath, "first").toString)
Seq(1).toDF("a").write.format("parquet").save(new Path(basePath, "second").toString)
val df = spark.read.parquet(
new Path(basePath, "first").toString,
new Path(basePath, "second").toString)
val e = intercept[SparkException] {
df.collect()
}
assert(e.getCause.isInstanceOf[OutOfMemoryError])
}
}
}
```
Author: Dongjoon Hyun <dongjoon@apache.org>
Closes#20619 from dongjoon-hyun/SPARK-23390.
## What changes were proposed in this pull request?
This PR updates Apache ORC dependencies to 1.4.3 released on February 9th. Apache ORC 1.4.2 release removes unnecessary dependencies and 1.4.3 has 5 more patches (https://s.apache.org/Fll8).
Especially, the following ORC-285 is fixed at 1.4.3.
```scala
scala> val df = Seq(Array.empty[Float]).toDF()
scala> df.write.format("orc").save("/tmp/floatarray")
scala> spark.read.orc("/tmp/floatarray")
res1: org.apache.spark.sql.DataFrame = [value: array<float>]
scala> spark.read.orc("/tmp/floatarray").show()
18/02/12 22:09:10 ERROR Executor: Exception in task 0.0 in stage 1.0 (TID 1)
java.io.IOException: Error reading file: file:/tmp/floatarray/part-00000-9c0b461b-4df1-4c23-aac1-3e4f349ac7d6-c000.snappy.orc
at org.apache.orc.impl.RecordReaderImpl.nextBatch(RecordReaderImpl.java:1191)
at org.apache.orc.mapreduce.OrcMapreduceRecordReader.ensureBatch(OrcMapreduceRecordReader.java:78)
...
Caused by: java.io.EOFException: Read past EOF for compressed stream Stream for column 2 kind DATA position: 0 length: 0 range: 0 offset: 0 limit: 0
```
## How was this patch tested?
Pass the Jenkins test.
Author: Dongjoon Hyun <dongjoon@apache.org>
Closes#20511 from dongjoon-hyun/SPARK-23340.
## What changes were proposed in this pull request?
Cleaned up the codegen templates for `Literal`s, to make sure that the `ExprCode` returned from `Literal.doGenCode()` has:
1. an empty `code` field;
2. an `isNull` field of either literal `true` or `false`;
3. a `value` field that is just a simple literal/constant.
Before this PR, there are a couple of paths that would return a non-trivial `code` and all of them are actually unnecessary. The `NaN` and `Infinity` constants for `double` and `float` can be accessed through constants directly available so there's no need to add a reference for them.
Also took the opportunity to add a new util method for ease of creating `ExprCode` for inline-able non-null values.
## How was this patch tested?
Existing tests.
Author: Kris Mok <kris.mok@databricks.com>
Closes#20626 from rednaxelafx/codegen-literal.
## What changes were proposed in this pull request?
Migrating KafkaSource (with data source v1) to KafkaMicroBatchReader (with data source v2).
Performance comparison:
In a unit test with in-process Kafka broker, I tested the read throughput of V1 and V2 using 20M records in a single partition. They were comparable.
## How was this patch tested?
Existing tests, few modified to be better tests than the existing ones.
Author: Tathagata Das <tathagata.das1565@gmail.com>
Closes#20554 from tdas/SPARK-23362.
## What changes were proposed in this pull request?
This replaces `Sparkcurrently` to `Spark currently` in the following error message.
```scala
scala> sql("insert into t2 select * from v1")
org.apache.spark.sql.AnalysisException: Output Hive table `default`.`t2`
is bucketed but Sparkcurrently does NOT populate bucketed ...
```
## How was this patch tested?
Manual.
Author: Dongjoon Hyun <dongjoon@apache.org>
Closes#20617 from dongjoon-hyun/SPARK-ERROR-MSG.
## What changes were proposed in this pull request?
To prevent any regressions, this PR changes ORC implementation to `hive` by default like Spark 2.2.X.
Users can enable `native` ORC. Also, ORC PPD is also restored to `false` like Spark 2.2.X.
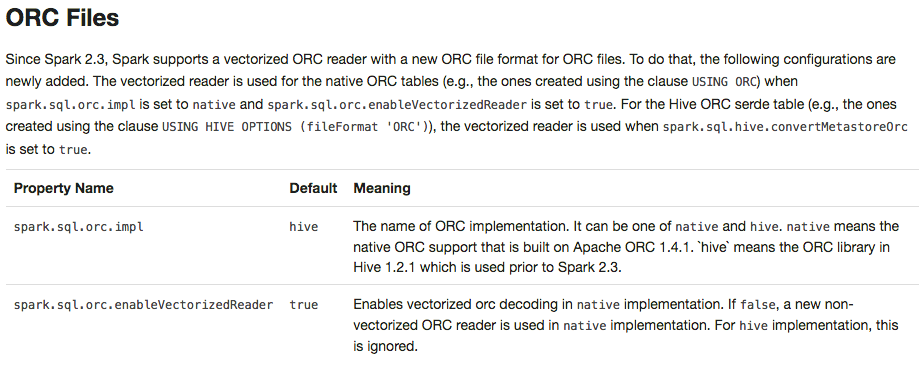
## How was this patch tested?
Pass all test cases.
Author: Dongjoon Hyun <dongjoon@apache.org>
Closes#20610 from dongjoon-hyun/SPARK-ORC-DISABLE.
## What changes were proposed in this pull request?
This PR proposes to add an alias 'names' of 'fieldNames' in Scala. Please see the discussion in [SPARK-20090](https://issues.apache.org/jira/browse/SPARK-20090).
## How was this patch tested?
Unit tests added in `DataTypeSuite.scala`.
Author: hyukjinkwon <gurwls223@gmail.com>
Closes#20545 from HyukjinKwon/SPARK-23359.
## What changes were proposed in this pull request?
Streaming execution has a list of exceptions that means interruption, and handle them specially. `WriteToDataSourceV2Exec` should also respect this list and not wrap them with `SparkException`.
## How was this patch tested?
existing test.
Author: Wenchen Fan <wenchen@databricks.com>
Closes#20605 from cloud-fan/write.
## What changes were proposed in this pull request?
This PR is to revert the PR https://github.com/apache/spark/pull/20302, because it causes a regression.
## How was this patch tested?
N/A
Author: gatorsmile <gatorsmile@gmail.com>
Closes#20614 from gatorsmile/revertJsonFix.
## What changes were proposed in this pull request?
https://github.com/apache/spark/pull/19579 introduces a behavior change. We need to document it in the migration guide.
## How was this patch tested?
Also update the HiveExternalCatalogVersionsSuite to verify it.
Author: gatorsmile <gatorsmile@gmail.com>
Closes#20606 from gatorsmile/addMigrationGuide.
## What changes were proposed in this pull request?
Solved two bugs to enable stream-stream self joins.
### Incorrect analysis due to missing MultiInstanceRelation trait
Streaming leaf nodes did not extend MultiInstanceRelation, which is necessary for the catalyst analyzer to convert the self-join logical plan DAG into a tree (by creating new instances of the leaf relations). This was causing the error `Failure when resolving conflicting references in Join:` (see JIRA for details).
### Incorrect attribute rewrite when splicing batch plans in MicroBatchExecution
When splicing the source's batch plan into the streaming plan (by replacing the StreamingExecutionPlan), we were rewriting the attribute reference in the streaming plan with the new attribute references from the batch plan. This was incorrectly handling the scenario when multiple StreamingExecutionRelation point to the same source, and therefore eventually point to the same batch plan returned by the source. Here is an example query, and its corresponding plan transformations.
```
val df = input.toDF
val join =
df.select('value % 5 as "key", 'value).join(
df.select('value % 5 as "key", 'value), "key")
```
Streaming logical plan before splicing the batch plan
```
Project [key#6, value#1, value#12]
+- Join Inner, (key#6 = key#9)
:- Project [(value#1 % 5) AS key#6, value#1]
: +- StreamingExecutionRelation Memory[#1], value#1
+- Project [(value#12 % 5) AS key#9, value#12]
+- StreamingExecutionRelation Memory[#1], value#12 // two different leaves pointing to same source
```
Batch logical plan after splicing the batch plan and before rewriting
```
Project [key#6, value#1, value#12]
+- Join Inner, (key#6 = key#9)
:- Project [(value#1 % 5) AS key#6, value#1]
: +- LocalRelation [value#66] // replaces StreamingExecutionRelation Memory[#1], value#1
+- Project [(value#12 % 5) AS key#9, value#12]
+- LocalRelation [value#66] // replaces StreamingExecutionRelation Memory[#1], value#12
```
Batch logical plan after rewriting the attributes. Specifically, for spliced, the new output attributes (value#66) replace the earlier output attributes (value#12, and value#1, one for each StreamingExecutionRelation).
```
Project [key#6, value#66, value#66] // both value#1 and value#12 replaces by value#66
+- Join Inner, (key#6 = key#9)
:- Project [(value#66 % 5) AS key#6, value#66]
: +- LocalRelation [value#66]
+- Project [(value#66 % 5) AS key#9, value#66]
+- LocalRelation [value#66]
```
This causes the optimizer to eliminate value#66 from one side of the join.
```
Project [key#6, value#66, value#66]
+- Join Inner, (key#6 = key#9)
:- Project [(value#66 % 5) AS key#6, value#66]
: +- LocalRelation [value#66]
+- Project [(value#66 % 5) AS key#9] // this does not generate value, incorrect join results
+- LocalRelation [value#66]
```
**Solution**: Instead of rewriting attributes, use a Project to introduce aliases between the output attribute references and the new reference generated by the spliced plans. The analyzer and optimizer will take care of the rest.
```
Project [key#6, value#1, value#12]
+- Join Inner, (key#6 = key#9)
:- Project [(value#1 % 5) AS key#6, value#1]
: +- Project [value#66 AS value#1] // solution: project with aliases
: +- LocalRelation [value#66]
+- Project [(value#12 % 5) AS key#9, value#12]
+- Project [value#66 AS value#12] // solution: project with aliases
+- LocalRelation [value#66]
```
## How was this patch tested?
New unit test
Author: Tathagata Das <tathagata.das1565@gmail.com>
Closes#20598 from tdas/SPARK-23406.
## What changes were proposed in this pull request?
This PR aims to resolve an open file leakage issue reported at [SPARK-23390](https://issues.apache.org/jira/browse/SPARK-23390) by moving the listener registration position. Currently, the sequence is like the following.
1. Create `batchReader`
2. `batchReader.initialize` opens a ORC file.
3. `batchReader.initBatch` may take a long time to alloc memory in some environment and cause errors.
4. `Option(TaskContext.get()).foreach(_.addTaskCompletionListener(_ => iter.close()))`
This PR moves 4 before 2 and 3. To sum up, the new sequence is 1 -> 4 -> 2 -> 3.
## How was this patch tested?
Manual. The following test case makes OOM intentionally to cause leaked filesystem connection in the current code base. With this patch, leakage doesn't occurs.
```scala
// This should be tested manually because it raises OOM intentionally
// in order to cause `Leaked filesystem connection`.
test("SPARK-23399 Register a task completion listener first for OrcColumnarBatchReader") {
withSQLConf(SQLConf.ORC_VECTORIZED_READER_BATCH_SIZE.key -> s"${Int.MaxValue}") {
withTempDir { dir =>
val basePath = dir.getCanonicalPath
Seq(0).toDF("a").write.format("orc").save(new Path(basePath, "first").toString)
Seq(1).toDF("a").write.format("orc").save(new Path(basePath, "second").toString)
val df = spark.read.orc(
new Path(basePath, "first").toString,
new Path(basePath, "second").toString)
val e = intercept[SparkException] {
df.collect()
}
assert(e.getCause.isInstanceOf[OutOfMemoryError])
}
}
}
```
Author: Dongjoon Hyun <dongjoon@apache.org>
Closes#20590 from dongjoon-hyun/SPARK-23399.
## What changes were proposed in this pull request?
In this upcoming 2.3 release, we changed the interface of `ScalaUDF`. Unfortunately, some Spark packages (e.g., spark-deep-learning) are using our internal class `ScalaUDF`. In the release 2.3, we added new parameters into this class. The users hit the binary compatibility issues and got the exception:
```
> java.lang.NoSuchMethodError: org.apache.spark.sql.catalyst.expressions.ScalaUDF.<init>(Ljava/lang/Object;Lorg/apache/spark/sql/types/DataType;Lscala/collection/Seq;Lscala/collection/Seq;Lscala/Option;)V
```
This PR is to improve the backward compatibility. However, we definitely should not encourage the external packages to use our internal classes. This might make us hard to maintain/develop the codes in Spark.
## How was this patch tested?
N/A
Author: gatorsmile <gatorsmile@gmail.com>
Closes#20591 from gatorsmile/scalaUDF.
## What changes were proposed in this pull request?
Added flag ignoreNullability to DataType.equalsStructurally.
The previous semantic is for ignoreNullability=false.
When ignoreNullability=true equalsStructurally ignores nullability of contained types (map key types, value types, array element types, structure field types).
In.checkInputTypes calls equalsStructurally to check if the children types match. They should match regardless of nullability (which is just a hint), so it is now called with ignoreNullability=true.
## How was this patch tested?
New test in SubquerySuite
Author: Bogdan Raducanu <bogdan@databricks.com>
Closes#20548 from bogdanrdc/SPARK-23316.
## What changes were proposed in this pull request?
If the target database name is as same as the current database, we should be able to skip one metastore access.
## How was this patch tested?
(Please explain how this patch was tested. E.g. unit tests, integration tests, manual tests)
(If this patch involves UI changes, please attach a screenshot; otherwise, remove this)
Please review http://spark.apache.org/contributing.html before opening a pull request.
Author: Feng Liu <fengliu@databricks.com>
Closes#20565 from liufengdb/remove-redundant.
## What changes were proposed in this pull request?
DataSourceV2 batch writes should use the output commit coordinator if it is required by the data source. This adds a new method, `DataWriterFactory#useCommitCoordinator`, that determines whether the coordinator will be used. If the write factory returns true, `WriteToDataSourceV2` will use the coordinator for batch writes.
## How was this patch tested?
This relies on existing write tests, which now use the commit coordinator.
Author: Ryan Blue <blue@apache.org>
Closes#20490 from rdblue/SPARK-23323-add-commit-coordinator.
When hive.default.fileformat is other kinds of file types, create textfile table cause a serde error.
We should take the default type of textfile and sequencefile both as org.apache.hadoop.hive.serde2.lazy.LazySimpleSerDe.
```
set hive.default.fileformat=orc;
create table tbl( i string ) stored as textfile;
desc formatted tbl;
Serde Library org.apache.hadoop.hive.ql.io.orc.OrcSerde
InputFormat org.apache.hadoop.mapred.TextInputFormat
OutputFormat org.apache.hadoop.hive.ql.io.HiveIgnoreKeyTextOutputFormat
```
Author: sychen <sychen@ctrip.com>
Closes#20406 from cxzl25/default_serde.
## What changes were proposed in this pull request?
This removes the special case that `alterPartitions` call from `HiveExternalCatalog` can reset the current database in the hive client as a side effect.
## How was this patch tested?
(Please explain how this patch was tested. E.g. unit tests, integration tests, manual tests)
(If this patch involves UI changes, please attach a screenshot; otherwise, remove this)
Please review http://spark.apache.org/contributing.html before opening a pull request.
Author: Feng Liu <fengliu@databricks.com>
Closes#20564 from liufengdb/move.
## What changes were proposed in this pull request?
This is a follow-up pr of #19231 which modified the behavior to remove metadata from JDBC table schema.
This pr adds a test to check if the schema doesn't have metadata.
## How was this patch tested?
Added a test and existing tests.
Author: Takuya UESHIN <ueshin@databricks.com>
Closes#20585 from ueshin/issues/SPARK-22002/fup1.
## What changes were proposed in this pull request?
Re-add support for parquet binary DecimalType in VectorizedColumnReader
## How was this patch tested?
Existing test suite
Author: James Thompson <jamesthomp@users.noreply.github.com>
Closes#20580 from jamesthomp/jt/add-back-binary-decimal.
## What changes were proposed in this pull request?
In the `getBlockData`,`blockId.reduceId` is the `Int` type, when it is greater than 2^28, `blockId.reduceId*8` will overflow
In the `decompress0`, `len` and `unitSize` are Int type, so `len * unitSize` may lead to overflow
## How was this patch tested?
N/A
Author: liuxian <liu.xian3@zte.com.cn>
Closes#20581 from 10110346/overflow2.
## What changes were proposed in this pull request?
This is a regression in Spark 2.3.
In Spark 2.2, we have a fragile UI support for SQL data writing commands. We only track the input query plan of `FileFormatWriter` and display its metrics. This is not ideal because we don't know who triggered the writing(can be table insertion, CTAS, etc.), but it's still useful to see the metrics of the input query.
In Spark 2.3, we introduced a new mechanism: `DataWritigCommand`, to fix the UI issue entirely. Now these writing commands have real children, and we don't need to hack into the `FileFormatWriter` for the UI. This also helps with `explain`, now `explain` can show the physical plan of the input query, while in 2.2 the physical writing plan is simply `ExecutedCommandExec` and it has no child.
However there is a regression in CTAS. CTAS commands don't extend `DataWritigCommand`, and we don't have the UI hack in `FileFormatWriter` anymore, so the UI for CTAS is just an empty node. See https://issues.apache.org/jira/browse/SPARK-22977 for more information about this UI issue.
To fix it, we should apply the `DataWritigCommand` mechanism to CTAS commands.
TODO: In the future, we should refactor this part and create some physical layer code pieces for data writing, and reuse them in different writing commands. We should have different logical nodes for different operators, even some of them share some same logic, e.g. CTAS, CREATE TABLE, INSERT TABLE. Internally we can share the same physical logic.
## How was this patch tested?
manually tested.
For data source table
<img width="644" alt="1" src="https://user-images.githubusercontent.com/3182036/35874155-bdffab28-0ba6-11e8-94a8-e32e106ba069.png">
For hive table
<img width="666" alt="2" src="https://user-images.githubusercontent.com/3182036/35874161-c437e2a8-0ba6-11e8-98ed-7930f01432c5.png">
Author: Wenchen Fan <wenchen@databricks.com>
Closes#20521 from cloud-fan/UI.
## What changes were proposed in this pull request?
Currently, we use SBT and MAVN to spark unit test, are affected by the parameters of `spark.testing`. However, when using the IDE test tool, `spark.testing` support is not very good, sometimes need to be manually added to the beforeEach. example: HiveSparkSubmitSuite RPackageUtilsSuite SparkSubmitSuite. The PR unified `spark.testing` parameter extraction to SparkFunSuite, support IDE test tool, and the test code is more compact.
## How was this patch tested?
the existed test cases.
Author: caoxuewen <cao.xuewen@zte.com.cn>
Closes#20582 from heary-cao/sparktesting.
## What changes were proposed in this pull request?
This PR targets to explicitly specify supported types in Pandas UDFs.
The main change here is to add a deduplicated and explicit type checking in `returnType` ahead with documenting this; however, it happened to fix multiple things.
1. Currently, we don't support `BinaryType` in Pandas UDFs, for example, see:
```python
from pyspark.sql.functions import pandas_udf
pudf = pandas_udf(lambda x: x, "binary")
df = spark.createDataFrame([[bytearray(1)]])
df.select(pudf("_1")).show()
```
```
...
TypeError: Unsupported type in conversion to Arrow: BinaryType
```
We can document this behaviour for its guide.
2. Also, the grouped aggregate Pandas UDF fails fast on `ArrayType` but seems we can support this case.
```python
from pyspark.sql.functions import pandas_udf, PandasUDFType
foo = pandas_udf(lambda v: v.mean(), 'array<double>', PandasUDFType.GROUPED_AGG)
df = spark.range(100).selectExpr("id", "array(id) as value")
df.groupBy("id").agg(foo("value")).show()
```
```
...
NotImplementedError: ArrayType, StructType and MapType are not supported with PandasUDFType.GROUPED_AGG
```
3. Since we can check the return type ahead, we can fail fast before actual execution.
```python
# we can fail fast at this stage because we know the schema ahead
pandas_udf(lambda x: x, BinaryType())
```
## How was this patch tested?
Manually tested and unit tests for `BinaryType` and `ArrayType(...)` were added.
Author: hyukjinkwon <gurwls223@gmail.com>
Closes#20531 from HyukjinKwon/pudf-cleanup.
## What changes were proposed in this pull request?
This test only fails with sbt on Hadoop 2.7, I can't reproduce it locally, but here is my speculation by looking at the code:
1. FileSystem.delete doesn't delete the directory entirely, somehow we can still open the file as a 0-length empty file.(just speculation)
2. ORC intentionally allow empty files, and the reader fails during reading without closing the file stream.
This PR improves the test to make sure all files are deleted and can't be opened.
## How was this patch tested?
N/A
Author: Wenchen Fan <wenchen@databricks.com>
Closes#20584 from cloud-fan/flaky-test.
## What changes were proposed in this pull request?
This is a long-standing bug in `UnsafeKVExternalSorter` and was reported in the dev list multiple times.
When creating `UnsafeKVExternalSorter` with `BytesToBytesMap`, we need to create a `UnsafeInMemorySorter` to sort the data in `BytesToBytesMap`. The data format of the sorter and the map is same, so no data movement is required. However, both the sorter and the map need a point array for some bookkeeping work.
There is an optimization in `UnsafeKVExternalSorter`: reuse the point array between the sorter and the map, to avoid an extra memory allocation. This sounds like a reasonable optimization, the length of the `BytesToBytesMap` point array is at least 4 times larger than the number of keys(to avoid hash collision, the hash table size should be at least 2 times larger than the number of keys, and each key occupies 2 slots). `UnsafeInMemorySorter` needs the pointer array size to be 4 times of the number of entries, so we are safe to reuse the point array.
However, the number of keys of the map doesn't equal to the number of entries in the map, because `BytesToBytesMap` supports duplicated keys. This breaks the assumption of the above optimization and we may run out of space when inserting data into the sorter, and hit error
```
java.lang.IllegalStateException: There is no space for new record
at org.apache.spark.util.collection.unsafe.sort.UnsafeInMemorySorter.insertRecord(UnsafeInMemorySorter.java:239)
at org.apache.spark.sql.execution.UnsafeKVExternalSorter.<init>(UnsafeKVExternalSorter.java:149)
...
```
This PR fixes this bug by creating a new point array if the existing one is not big enough.
## How was this patch tested?
a new test
Author: Wenchen Fan <wenchen@databricks.com>
Closes#20561 from cloud-fan/bug.
## What changes were proposed in this pull request?
This is a follow up of https://github.com/apache/spark/pull/20441.
The two lines actually can trigger the hive metastore bug: https://issues.apache.org/jira/browse/HIVE-16844
The two configs are not in the default `ObjectStore` properties, so any run hive commands after these two lines will set the `propsChanged` flag in the `ObjectStore.setConf` and then cause thread leaks.
I don't think the two lines are very useful. They can be removed safely.
## How was this patch tested?
(Please explain how this patch was tested. E.g. unit tests, integration tests, manual tests)
(If this patch involves UI changes, please attach a screenshot; otherwise, remove this)
Please review http://spark.apache.org/contributing.html before opening a pull request.
Author: Feng Liu <fengliu@databricks.com>
Closes#20562 from liufengdb/fix-omm.
## What changes were proposed in this pull request?
Typo fixes (with expanding a Hive property)
## How was this patch tested?
local build. Awaiting Jenkins
Author: Jacek Laskowski <jacek@japila.pl>
Closes#20550 from jaceklaskowski/hiveutils-typos.
## What changes were proposed in this pull request?
This is a followup of https://github.com/apache/spark/pull/20435.
While reorganizing the packages for streaming data source v2, the top level stream read/write support interfaces should not be in the reader/writer package, but should be in the `sources.v2` package, to follow the `ReadSupport`, `WriteSupport`, etc.
## How was this patch tested?
N/A
Author: Wenchen Fan <wenchen@databricks.com>
Closes#20509 from cloud-fan/followup.
## What changes were proposed in this pull request?
For inserting/appending data to an existing table, Spark should adjust the data types of the input query according to the table schema, or fail fast if it's uncastable.
There are several ways to insert/append data: SQL API, `DataFrameWriter.insertInto`, `DataFrameWriter.saveAsTable`. The first 2 ways create `InsertIntoTable` plan, and the last way creates `CreateTable` plan. However, we only adjust input query data types for `InsertIntoTable`, and users may hit weird errors when appending data using `saveAsTable`. See the JIRA for the error case.
This PR fixes this bug by adjusting data types for `CreateTable` too.
## How was this patch tested?
new test.
Author: Wenchen Fan <wenchen@databricks.com>
Closes#20527 from cloud-fan/saveAsTable.
## What changes were proposed in this pull request?
This is to revert the changes made in https://github.com/apache/spark/pull/19499 , because this causes a regression. We should not ignore the table-specific compression conf when the Hive serde tables are converted to the data source tables.
## How was this patch tested?
The existing tests.
Author: gatorsmile <gatorsmile@gmail.com>
Closes#20536 from gatorsmile/revert22279.
## What changes were proposed in this pull request?
This PR migrates the MemoryStream to DataSourceV2 APIs.
One additional change is in the reported keys in StreamingQueryProgress.durationMs. "getOffset" and "getBatch" replaced with "setOffsetRange" and "getEndOffset" as tracking these make more sense. Unit tests changed accordingly.
## How was this patch tested?
Existing unit tests, few updated unit tests.
Author: Tathagata Das <tathagata.das1565@gmail.com>
Author: Burak Yavuz <brkyvz@gmail.com>
Closes#20445 from tdas/SPARK-23092.
## What changes were proposed in this pull request?
When `DebugFilesystem` closes opened stream, if any exception occurs, we still need to remove the open stream record from `DebugFilesystem`. Otherwise, it goes to report leaked filesystem connection.
## How was this patch tested?
Existing tests.
Author: Liang-Chi Hsieh <viirya@gmail.com>
Closes#20524 from viirya/SPARK-23345.
## What changes were proposed in this pull request?
Replace `registerTempTable` by `createOrReplaceTempView`.
## How was this patch tested?
N/A
Author: gatorsmile <gatorsmile@gmail.com>
Closes#20523 from gatorsmile/updateExamples.
## What changes were proposed in this pull request?
Update the description and tests of three external API or functions `createFunction `, `length` and `repartitionByRange `
## How was this patch tested?
N/A
Author: gatorsmile <gatorsmile@gmail.com>
Closes#20495 from gatorsmile/updateFunc.
## What changes were proposed in this pull request?
`DataSourceV2Relation` keeps a `fullOutput` and resolves the real output on demand by column name lookup. i.e.
```
lazy val output: Seq[Attribute] = reader.readSchema().map(_.name).map { name =>
fullOutput.find(_.name == name).get
}
```
This will be broken after we canonicalize the plan, because all attribute names become "None", see https://github.com/apache/spark/blob/v2.3.0-rc1/sql/catalyst/src/main/scala/org/apache/spark/sql/catalyst/expressions/Canonicalize.scala#L42
To fix this, `DataSourceV2Relation` should just keep `output`, and update the `output` when doing column pruning.
## How was this patch tested?
a new test case
Author: Wenchen Fan <wenchen@databricks.com>
Closes#20485 from cloud-fan/canonicalize.
## What changes were proposed in this pull request?
https://github.com/apache/spark/pull/20483 tried to provide a way to turn off the new columnar cache reader, to restore the behavior in 2.2. However even we turn off that config, the behavior is still different than 2.2.
If the output data are rows, we still enable whole stage codegen for the scan node, which is different with 2.2, we should also fix it.
## How was this patch tested?
existing tests.
Author: Wenchen Fan <wenchen@databricks.com>
Closes#20513 from cloud-fan/cache.
## What changes were proposed in this pull request?
Spark SQL executions page throws the following error and the page crashes:
```
HTTP ERROR 500
Problem accessing /SQL/. Reason:
Server Error
Caused by:
java.lang.NullPointerException
at scala.collection.immutable.StringOps$.length$extension(StringOps.scala:47)
at scala.collection.immutable.StringOps.length(StringOps.scala:47)
at scala.collection.IndexedSeqOptimized$class.isEmpty(IndexedSeqOptimized.scala:27)
at scala.collection.immutable.StringOps.isEmpty(StringOps.scala:29)
at scala.collection.TraversableOnce$class.nonEmpty(TraversableOnce.scala:111)
at scala.collection.immutable.StringOps.nonEmpty(StringOps.scala:29)
at org.apache.spark.sql.execution.ui.ExecutionTable.descriptionCell(AllExecutionsPage.scala:182)
at org.apache.spark.sql.execution.ui.ExecutionTable.row(AllExecutionsPage.scala:155)
at org.apache.spark.sql.execution.ui.ExecutionTable$$anonfun$8.apply(AllExecutionsPage.scala:204)
at org.apache.spark.sql.execution.ui.ExecutionTable$$anonfun$8.apply(AllExecutionsPage.scala:204)
at org.apache.spark.ui.UIUtils$$anonfun$listingTable$2.apply(UIUtils.scala:339)
at org.apache.spark.ui.UIUtils$$anonfun$listingTable$2.apply(UIUtils.scala:339)
at scala.collection.TraversableLike$$anonfun$map$1.apply(TraversableLike.scala:234)
at scala.collection.TraversableLike$$anonfun$map$1.apply(TraversableLike.scala:234)
at scala.collection.mutable.ResizableArray$class.foreach(ResizableArray.scala:59)
at scala.collection.mutable.ArrayBuffer.foreach(ArrayBuffer.scala:48)
at scala.collection.TraversableLike$class.map(TraversableLike.scala:234)
at scala.collection.AbstractTraversable.map(Traversable.scala:104)
at org.apache.spark.ui.UIUtils$.listingTable(UIUtils.scala:339)
at org.apache.spark.sql.execution.ui.ExecutionTable.toNodeSeq(AllExecutionsPage.scala:203)
at org.apache.spark.sql.execution.ui.AllExecutionsPage.render(AllExecutionsPage.scala:67)
at org.apache.spark.ui.WebUI$$anonfun$2.apply(WebUI.scala:82)
at org.apache.spark.ui.WebUI$$anonfun$2.apply(WebUI.scala:82)
at org.apache.spark.ui.JettyUtils$$anon$3.doGet(JettyUtils.scala:90)
at javax.servlet.http.HttpServlet.service(HttpServlet.java:687)
at javax.servlet.http.HttpServlet.service(HttpServlet.java:790)
at org.eclipse.jetty.servlet.ServletHolder.handle(ServletHolder.java:848)
at org.eclipse.jetty.servlet.ServletHandler.doHandle(ServletHandler.java:584)
at org.eclipse.jetty.server.handler.ContextHandler.doHandle(ContextHandler.java:1180)
at org.eclipse.jetty.servlet.ServletHandler.doScope(ServletHandler.java:512)
at org.eclipse.jetty.server.handler.ContextHandler.doScope(ContextHandler.java:1112)
at org.eclipse.jetty.server.handler.ScopedHandler.handle(ScopedHandler.java:141)
at org.eclipse.jetty.server.handler.ContextHandlerCollection.handle(ContextHandlerCollection.java:213)
at org.eclipse.jetty.server.handler.HandlerWrapper.handle(HandlerWrapper.java:134)
at org.eclipse.jetty.server.Server.handle(Server.java:534)
at org.eclipse.jetty.server.HttpChannel.handle(HttpChannel.java:320)
at org.eclipse.jetty.server.HttpConnection.onFillable(HttpConnection.java:251)
at org.eclipse.jetty.io.AbstractConnection$ReadCallback.succeeded(AbstractConnection.java:283)
at org.eclipse.jetty.io.FillInterest.fillable(FillInterest.java:108)
at org.eclipse.jetty.io.SelectChannelEndPoint$2.run(SelectChannelEndPoint.java:93)
at org.eclipse.jetty.util.thread.strategy.ExecuteProduceConsume.executeProduceConsume(ExecuteProduceConsume.java:303)
at org.eclipse.jetty.util.thread.strategy.ExecuteProduceConsume.produceConsume(ExecuteProduceConsume.java:148)
at org.eclipse.jetty.util.thread.strategy.ExecuteProduceConsume.run(ExecuteProduceConsume.java:136)
at org.eclipse.jetty.util.thread.QueuedThreadPool.runJob(QueuedThreadPool.java:671)
at org.eclipse.jetty.util.thread.QueuedThreadPool$2.run(QueuedThreadPool.java:589)
at java.lang.Thread.run(Thread.java:748)
```
One of the possible reason that this page fails may be the `SparkListenerSQLExecutionStart` event get dropped before processed, so the execution description and details don't get updated.
This was not a issue in 2.2 because it would ignore any job start event that arrives before the corresponding execution start event, which doesn't sound like a good decision.
We shall try to handle the null values in the front page side, that is, try to give a default value when `execution.details` or `execution.description` is null.
Another possible approach is not to spill the `LiveExecutionData` in `SQLAppStatusListener.update(exec: LiveExecutionData)` if `exec.details` is null. This is not ideal because this way you will not see the execution if `SparkListenerSQLExecutionStart` event is lost, because `AllExecutionsPage` only read executions from KVStore.
## How was this patch tested?
After the change, the page shows the following:
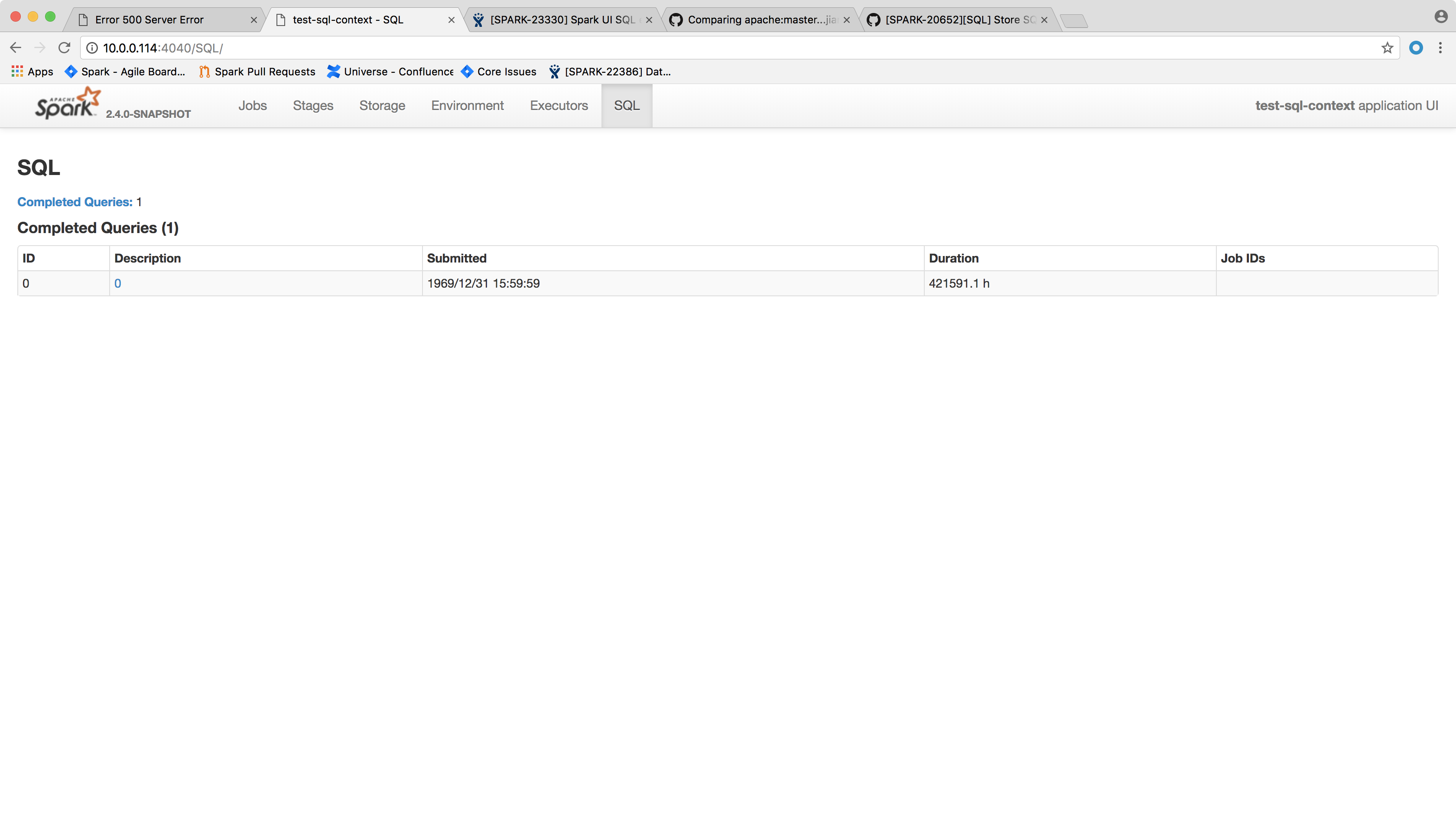
Author: Xingbo Jiang <xingbo.jiang@databricks.com>
Closes#20502 from jiangxb1987/executionPage.
## What changes were proposed in this pull request?
Sort jobs/stages/tasks/queries with the completed timestamp before cleaning up them to make the behavior consistent with 2.2.
## How was this patch tested?
- Jenkins.
- Manually ran the following codes and checked the UI for jobs/stages/tasks/queries.
```
spark.ui.retainedJobs 10
spark.ui.retainedStages 10
spark.sql.ui.retainedExecutions 10
spark.ui.retainedTasks 10
```
```
new Thread() {
override def run() {
spark.range(1, 2).foreach { i =>
Thread.sleep(10000)
}
}
}.start()
Thread.sleep(5000)
for (_ <- 1 to 20) {
new Thread() {
override def run() {
spark.range(1, 2).foreach { i =>
}
}
}.start()
}
Thread.sleep(15000)
spark.range(1, 2).foreach { i =>
}
sc.makeRDD(1 to 100, 100).foreach { i =>
}
```
Author: Shixiong Zhu <zsxwing@gmail.com>
Closes#20481 from zsxwing/SPARK-23307.
## What changes were proposed in this pull request?
Fix decimalArithmeticOperations.sql test
## How was this patch tested?
N/A
Author: Yuming Wang <wgyumg@gmail.com>
Author: wangyum <wgyumg@gmail.com>
Author: Yuming Wang <yumwang@ebay.com>
Closes#20498 from wangyum/SPARK-22036.
## What changes were proposed in this pull request?
Like Parquet, all file-based data source handles `spark.sql.files.ignoreMissingFiles` correctly. We had better have a test coverage for feature parity and in order to prevent future accidental regression for all data sources.
## How was this patch tested?
Pass Jenkins with a newly added test case.
Author: Dongjoon Hyun <dongjoon@apache.org>
Closes#20479 from dongjoon-hyun/SPARK-23305.
## What changes were proposed in this pull request?
In the current test case for CombineTypedFilters, we lack the test of FilterFunction, so let's add it.
In addition, in TypedFilterOptimizationSuite's existing test cases, Let's extract a common LocalRelation.
## How was this patch tested?
add new test cases.
Author: caoxuewen <cao.xuewen@zte.com.cn>
Closes#20482 from heary-cao/TypedFilterOptimizationSuite.
## What changes were proposed in this pull request?
In the document of `ContinuousReader.setOffset`, we say this method is used to specify the start offset. We also have a `ContinuousReader.getStartOffset` to get the value back. I think it makes more sense to rename `ContinuousReader.setOffset` to `setStartOffset`.
## How was this patch tested?
N/A
Author: Wenchen Fan <wenchen@databricks.com>
Closes#20486 from cloud-fan/rename.
## What changes were proposed in this pull request?
This patch adds a small example to the schema string definition of schema function. It isn't obvious how to use it, so an example would be useful.
## How was this patch tested?
N/A - doc only.
Author: Reynold Xin <rxin@databricks.com>
Closes#20491 from rxin/schema-doc.
## What changes were proposed in this pull request?
https://issues.apache.org/jira/browse/SPARK-23309 reported a performance regression about cached table in Spark 2.3. While the investigating is still going on, this PR adds a conf to turn off the vectorized cache reader, to unblock the 2.3 release.
## How was this patch tested?
a new test
Author: Wenchen Fan <wenchen@databricks.com>
Closes#20483 from cloud-fan/cache.
## What changes were proposed in this pull request?
This PR fixes a mistake in the `PushDownOperatorsToDataSource` rule, the column pruning logic is incorrect about `Project`.
## How was this patch tested?
a new test case for column pruning with arbitrary expressions, and improve the existing tests to make sure the `PushDownOperatorsToDataSource` really works.
Author: Wenchen Fan <wenchen@databricks.com>
Closes#20476 from cloud-fan/push-down.
## What changes were proposed in this pull request?
For some ColumnVector get APIs such as getDecimal, getBinary, getStruct, getArray, getInterval, getUTF8String, we should clearly document their behaviors when accessing null slot. They should return null in this case. Then we can remove null checks from the places using above APIs.
For the APIs of primitive values like getInt, getInts, etc., this also documents their behaviors when accessing null slots. Their returning values are undefined and can be anything.
## How was this patch tested?
Added tests into `ColumnarBatchSuite`.
Author: Liang-Chi Hsieh <viirya@gmail.com>
Closes#20455 from viirya/SPARK-23272-followup.
## What changes were proposed in this pull request?
`DataSourceV2Relation` should extend `MultiInstanceRelation`, to take care of self-join.
## How was this patch tested?
a new test
Author: Wenchen Fan <wenchen@databricks.com>
Closes#20466 from cloud-fan/dsv2-selfjoin.
## What changes were proposed in this pull request?
`--hiveconf` and `--hivevar` variables no longer work since Spark 2.0. The `spark-sql` client has fixed by [SPARK-15730](https://issues.apache.org/jira/browse/SPARK-15730) and [SPARK-18086](https://issues.apache.org/jira/browse/SPARK-18086). but `beeline`/[`Spark SQL HiveThriftServer2`](https://github.com/apache/spark/blob/v2.1.1/sql/hive-thriftserver/src/main/scala/org/apache/spark/sql/hive/thriftserver/HiveThriftServer2.scala) is still broken. This pull request fix it.
This pull request works for both `JDBC client` and `beeline`.
## How was this patch tested?
unit tests for `JDBC client`
manual tests for `beeline`:
```
git checkout origin/pr/17886
dev/make-distribution.sh --mvn mvn --tgz -Phive -Phive-thriftserver -Phadoop-2.6 -DskipTests
tar -zxf spark-2.3.0-SNAPSHOT-bin-2.6.5.tgz && cd spark-2.3.0-SNAPSHOT-bin-2.6.5
sbin/start-thriftserver.sh
```
```
cat <<EOF > test.sql
select '\${a}', '\${b}';
EOF
beeline -u jdbc:hive2://localhost:10000 --hiveconf a=avalue --hivevar b=bvalue -f test.sql
```
Author: Yuming Wang <wgyumg@gmail.com>
Closes#17886 from wangyum/SPARK-13983-dev.
## What changes were proposed in this pull request?
The current DataSourceWriter API makes it hard to implement `onTaskCommit(taskCommit: TaskCommitMessage)` in `FileCommitProtocol`.
In general, on receiving commit message, driver can start processing messages(e.g. persist messages into files) before all the messages are collected.
The proposal to add a new API:
`add(WriterCommitMessage message)`: Handles a commit message on receiving from a successful data writer.
This should make the whole API of DataSourceWriter compatible with `FileCommitProtocol`, and more flexible.
There was another radical attempt in #20386. This one should be more reasonable.
## How was this patch tested?
Unit test
Author: Wang Gengliang <ltnwgl@gmail.com>
Closes#20454 from gengliangwang/write_api.
## What changes were proposed in this pull request?
This is a followup pr of #20450.
We should've enabled `MutableColumnarRow.getMap()` as well.
## How was this patch tested?
Existing tests.
Author: Takuya UESHIN <ueshin@databricks.com>
Closes#20471 from ueshin/issues/SPARK-23280/fup2.