## What changes were proposed in this pull request?
The path was recently changed in https://github.com/apache/spark/pull/19946, but the dockerfile was not updated.
This is a trivial 1 line fix.
## How was this patch tested?
`./sbin/build-push-docker-images.sh -r spark-repo -t latest build`
cc/ vanzin mridulm rxin jiangxb1987 liyinan926
Author: Anirudh Ramanathan <ramanathana@google.com>
Author: foxish <ramanathana@google.com>
Closes#20051 from foxish/patch-1.
## What changes were proposed in this pull request?
Fixing configuration that was taking an int which should take time. Discussion in https://github.com/apache/spark/pull/19946#discussion_r156682354
Made the granularity milliseconds as opposed to seconds since there's a use-case for sub-second reactions to scale-up rapidly especially with dynamic allocation.
## How was this patch tested?
TODO: manual run of integration tests against this PR.
PTAL
cc/ mccheah liyinan926 kimoonkim vanzin mridulm jiangxb1987 ueshin
Author: foxish <ramanathana@google.com>
Closes#20032 from foxish/fix-time-conf.
# What changes were proposed in this pull request?
1. entrypoint.sh for Kubernetes spark-base image is marked as executable (644 -> 755)
2. make-distribution script will now create kubernetes/dockerfiles directory when Kubernetes support is compiled.
## How was this patch tested?
Manual testing
cc/ ueshin jiangxb1987 mridulm vanzin rxin liyinan926
Author: foxish <ramanathana@google.com>
Closes#20007 from foxish/fix-dockerfiles.
## What changes were proposed in this pull request?
Changes discussed in https://github.com/apache/spark/pull/19946#discussion_r157063535
docker -> container, since with CRI, we are not limited to running only docker images.
## How was this patch tested?
Manual testing
Author: foxish <ramanathana@google.com>
Closes#19995 from foxish/make-docker-container.
## What changes were proposed in this pull request?
This PR added the missing service metadata for `KubernetesClusterManager`. Without the metadata, the service loader couldn't load `KubernetesClusterManager`, and caused the driver to fail to create a `ExternalClusterManager`, as being reported in SPARK-22778. The PR also changed the `k8s:` prefix used to `k8s://`, which is what existing Spark on k8s users are familiar and used to.
## How was this patch tested?
Manual testing verified that the fix resolved the issue in SPARK-22778.
/cc vanzin felixcheung jiangxb1987
Author: Yinan Li <liyinan926@gmail.com>
Closes#19972 from liyinan926/fix-22778.
## What changes were proposed in this pull request?
PR closed with all the comments -> https://github.com/apache/spark/pull/19793
It solves the problem when submitting a wrong CreateSubmissionRequest to Spark Dispatcher was causing a bad state of Dispatcher and making it inactive as a Mesos framework.
https://issues.apache.org/jira/browse/SPARK-22574
## How was this patch tested?
All spark test passed successfully.
It was tested sending a wrong request (without appArgs) before and after the change. The point is easy, check if the value is null before being accessed.
This was before the change, leaving the dispatcher inactive:
```
Exception in thread "Thread-22" java.lang.NullPointerException
at org.apache.spark.scheduler.cluster.mesos.MesosClusterScheduler.getDriverCommandValue(MesosClusterScheduler.scala:444)
at org.apache.spark.scheduler.cluster.mesos.MesosClusterScheduler.buildDriverCommand(MesosClusterScheduler.scala:451)
at org.apache.spark.scheduler.cluster.mesos.MesosClusterScheduler.org$apache$spark$scheduler$cluster$mesos$MesosClusterScheduler$$createTaskInfo(MesosClusterScheduler.scala:538)
at org.apache.spark.scheduler.cluster.mesos.MesosClusterScheduler$$anonfun$scheduleTasks$1.apply(MesosClusterScheduler.scala:570)
at org.apache.spark.scheduler.cluster.mesos.MesosClusterScheduler$$anonfun$scheduleTasks$1.apply(MesosClusterScheduler.scala:555)
at scala.collection.mutable.ResizableArray$class.foreach(ResizableArray.scala:59)
at scala.collection.mutable.ArrayBuffer.foreach(ArrayBuffer.scala:48)
at org.apache.spark.scheduler.cluster.mesos.MesosClusterScheduler.scheduleTasks(MesosClusterScheduler.scala:555)
at org.apache.spark.scheduler.cluster.mesos.MesosClusterScheduler.resourceOffers(MesosClusterScheduler.scala:621)
```
And after:
```
"message" : "Malformed request: org.apache.spark.deploy.rest.SubmitRestProtocolException: Validation of message CreateSubmissionRequest failed!\n\torg.apache.spark.deploy.rest.SubmitRestProtocolMessage.validate(SubmitRestProtocolMessage.scala:70)\n\torg.apache.spark.deploy.rest.SubmitRequestServlet.doPost(RestSubmissionServer.scala:272)\n\tjavax.servlet.http.HttpServlet.service(HttpServlet.java:707)\n\tjavax.servlet.http.HttpServlet.service(HttpServlet.java:790)\n\torg.spark_project.jetty.servlet.ServletHolder.handle(ServletHolder.java:845)\n\torg.spark_project.jetty.servlet.ServletHandler.doHandle(ServletHandler.java:583)\n\torg.spark_project.jetty.server.handler.ContextHandler.doHandle(ContextHandler.java:1180)\n\torg.spark_project.jetty.servlet.ServletHandler.doScope(ServletHandler.java:511)\n\torg.spark_project.jetty.server.handler.ContextHandler.doScope(ContextHandler.java:1112)\n\torg.spark_project.jetty.server.handler.ScopedHandler.handle(ScopedHandler.java:141)\n\torg.spark_project.jetty.server.handler.HandlerWrapper.handle(HandlerWrapper.java:134)\n\torg.spark_project.jetty.server.Server.handle(Server.java:524)\n\torg.spark_project.jetty.server.HttpChannel.handle(HttpChannel.java:319)\n\torg.spark_project.jetty.server.HttpConnection.onFillable(HttpConnection.java:253)\n\torg.spark_project.jetty.io.AbstractConnection$ReadCallback.succeeded(AbstractConnection.java:273)\n\torg.spark_project.jetty.io.FillInterest.fillable(FillInterest.java:95)\n\torg.spark_project.jetty.io.SelectChannelEndPoint$2.run(SelectChannelEndPoint.java:93)\n\torg.spark_project.jetty.util.thread.strategy.ExecuteProduceConsume.executeProduceConsume(ExecuteProduceConsume.java:303)\n\torg.spark_project.jetty.util.thread.strategy.ExecuteProduceConsume.produceConsume(ExecuteProduceConsume.java:148)\n\torg.spark_project.jetty.util.thread.strategy.ExecuteProduceConsume.run(ExecuteProduceConsume.java:136)\n\torg.spark_project.jetty.util.thread.QueuedThreadPool.runJob(QueuedThreadPool.java:671)\n\torg.spark_project.jetty.util.thread.QueuedThreadPool$2.run(QueuedThreadPool.java:589)\n\tjava.lang.Thread.run(Thread.java:745)"
```
Author: German Schiavon <germanschiavon@gmail.com>
Closes#19966 from Gschiavon/fix-submission-request.
## What changes were proposed in this pull request?
It solves the problem when submitting a wrong CreateSubmissionRequest to Spark Dispatcher was causing a bad state of Dispatcher and making it inactive as a Mesos framework.
https://issues.apache.org/jira/browse/SPARK-22574
## How was this patch tested?
All spark test passed successfully.
It was tested sending a wrong request (without appArgs) before and after the change. The point is easy, check if the value is null before being accessed.
This was before the change, leaving the dispatcher inactive:
```
Exception in thread "Thread-22" java.lang.NullPointerException
at org.apache.spark.scheduler.cluster.mesos.MesosClusterScheduler.getDriverCommandValue(MesosClusterScheduler.scala:444)
at org.apache.spark.scheduler.cluster.mesos.MesosClusterScheduler.buildDriverCommand(MesosClusterScheduler.scala:451)
at org.apache.spark.scheduler.cluster.mesos.MesosClusterScheduler.org$apache$spark$scheduler$cluster$mesos$MesosClusterScheduler$$createTaskInfo(MesosClusterScheduler.scala:538)
at org.apache.spark.scheduler.cluster.mesos.MesosClusterScheduler$$anonfun$scheduleTasks$1.apply(MesosClusterScheduler.scala:570)
at org.apache.spark.scheduler.cluster.mesos.MesosClusterScheduler$$anonfun$scheduleTasks$1.apply(MesosClusterScheduler.scala:555)
at scala.collection.mutable.ResizableArray$class.foreach(ResizableArray.scala:59)
at scala.collection.mutable.ArrayBuffer.foreach(ArrayBuffer.scala:48)
at org.apache.spark.scheduler.cluster.mesos.MesosClusterScheduler.scheduleTasks(MesosClusterScheduler.scala:555)
at org.apache.spark.scheduler.cluster.mesos.MesosClusterScheduler.resourceOffers(MesosClusterScheduler.scala:621)
```
And after:
```
"message" : "Malformed request: org.apache.spark.deploy.rest.SubmitRestProtocolException: Validation of message CreateSubmissionRequest failed!\n\torg.apache.spark.deploy.rest.SubmitRestProtocolMessage.validate(SubmitRestProtocolMessage.scala:70)\n\torg.apache.spark.deploy.rest.SubmitRequestServlet.doPost(RestSubmissionServer.scala:272)\n\tjavax.servlet.http.HttpServlet.service(HttpServlet.java:707)\n\tjavax.servlet.http.HttpServlet.service(HttpServlet.java:790)\n\torg.spark_project.jetty.servlet.ServletHolder.handle(ServletHolder.java:845)\n\torg.spark_project.jetty.servlet.ServletHandler.doHandle(ServletHandler.java:583)\n\torg.spark_project.jetty.server.handler.ContextHandler.doHandle(ContextHandler.java:1180)\n\torg.spark_project.jetty.servlet.ServletHandler.doScope(ServletHandler.java:511)\n\torg.spark_project.jetty.server.handler.ContextHandler.doScope(ContextHandler.java:1112)\n\torg.spark_project.jetty.server.handler.ScopedHandler.handle(ScopedHandler.java:141)\n\torg.spark_project.jetty.server.handler.HandlerWrapper.handle(HandlerWrapper.java:134)\n\torg.spark_project.jetty.server.Server.handle(Server.java:524)\n\torg.spark_project.jetty.server.HttpChannel.handle(HttpChannel.java:319)\n\torg.spark_project.jetty.server.HttpConnection.onFillable(HttpConnection.java:253)\n\torg.spark_project.jetty.io.AbstractConnection$ReadCallback.succeeded(AbstractConnection.java:273)\n\torg.spark_project.jetty.io.FillInterest.fillable(FillInterest.java:95)\n\torg.spark_project.jetty.io.SelectChannelEndPoint$2.run(SelectChannelEndPoint.java:93)\n\torg.spark_project.jetty.util.thread.strategy.ExecuteProduceConsume.executeProduceConsume(ExecuteProduceConsume.java:303)\n\torg.spark_project.jetty.util.thread.strategy.ExecuteProduceConsume.produceConsume(ExecuteProduceConsume.java:148)\n\torg.spark_project.jetty.util.thread.strategy.ExecuteProduceConsume.run(ExecuteProduceConsume.java:136)\n\torg.spark_project.jetty.util.thread.QueuedThreadPool.runJob(QueuedThreadPool.java:671)\n\torg.spark_project.jetty.util.thread.QueuedThreadPool$2.run(QueuedThreadPool.java:589)\n\tjava.lang.Thread.run(Thread.java:745)"
```
Author: German Schiavon <germanschiavon@gmail.com>
Closes#19793 from Gschiavon/fix-submission-request.
This PR contains implementation of the basic submission client for the cluster mode of Spark on Kubernetes. It's step 2 from the step-wise plan documented [here](https://github.com/apache-spark-on-k8s/spark/issues/441#issuecomment-330802935).
This addition is covered by the [SPIP](http://apache-spark-developers-list.1001551.n3.nabble.com/SPIP-Spark-on-Kubernetes-td22147.html) vote which passed on Aug 31.
This PR and #19468 together form a MVP of Spark on Kubernetes that allows users to run Spark applications that use resources locally within the driver and executor containers on Kubernetes 1.6 and up. Some changes on pom and build/test setup are copied over from #19468 to make this PR self contained and testable.
The submission client is mainly responsible for creating the Kubernetes pod that runs the Spark driver. It follows a step-based approach to construct the driver pod, as the code under the `submit.steps` package shows. The steps are orchestrated by `DriverConfigurationStepsOrchestrator`. `Client` creates the driver pod and waits for the application to complete if it's configured to do so, which is the case by default.
This PR also contains Dockerfiles of the driver and executor images. They are included because some of the environment variables set in the code would not make sense without referring to the Dockerfiles.
* The patch contains unit tests which are passing.
* Manual testing: ./build/mvn -Pkubernetes clean package succeeded.
* It is a subset of the entire changelist hosted at http://github.com/apache-spark-on-k8s/spark which is in active use in several organizations.
* There is integration testing enabled in the fork currently hosted by PepperData which is being moved over to RiseLAB CI.
* Detailed documentation on trying out the patch in its entirety is in: https://apache-spark-on-k8s.github.io/userdocs/running-on-kubernetes.html
cc rxin felixcheung mateiz (shepherd)
k8s-big-data SIG members & contributors: mccheah foxish ash211 ssuchter varunkatta kimoonkim erikerlandson tnachen ifilonenko liyinan926
Author: Yinan Li <liyinan926@gmail.com>
Closes#19717 from liyinan926/spark-kubernetes-4.
## What changes were proposed in this pull request?
I see the two instances where the exception is occurring.
**Instance 1:**
```
17/11/10 15:49:32 ERROR util.Utils: Uncaught exception in thread driver-revive-thread
org.apache.spark.SparkException: Could not find CoarseGrainedScheduler.
at org.apache.spark.rpc.netty.Dispatcher.postMessage(Dispatcher.scala:160)
at org.apache.spark.rpc.netty.Dispatcher.postOneWayMessage(Dispatcher.scala:140)
at org.apache.spark.rpc.netty.NettyRpcEnv.send(NettyRpcEnv.scala:187)
at org.apache.spark.rpc.netty.NettyRpcEndpointRef.send(NettyRpcEnv.scala:521)
at org.apache.spark.scheduler.cluster.CoarseGrainedSchedulerBackend$DriverEndpoint$$anon$1$$anonfun$run$1$$anonfun$apply$mcV$sp$1.apply(CoarseGrainedSchedulerBackend.scala:125)
at org.apache.spark.scheduler.cluster.CoarseGrainedSchedulerBackend$DriverEndpoint$$anon$1$$anonfun$run$1$$anonfun$apply$mcV$sp$1.apply(CoarseGrainedSchedulerBackend.scala:125)
at scala.Option.foreach(Option.scala:257)
at org.apache.spark.scheduler.cluster.CoarseGrainedSchedulerBackend$DriverEndpoint$$anon$1$$anonfun$run$1.apply$mcV$sp(CoarseGrainedSchedulerBackend.scala:125)
at org.apache.spark.util.Utils$.tryLogNonFatalError(Utils.scala:1344)
at org.apache.spark.scheduler.cluster.CoarseGrainedSchedulerBackend$DriverEndpoint$$anon$1.run(CoarseGrainedSchedulerBackend.scala:124)
at java.util.concurrent.Executors$RunnableAdapter.call(Executors.java:511)
at java.util.concurrent.FutureTask.runAndReset(FutureTask.java:308)
at java.util.concurrent.ScheduledThreadPoolExecutor$ScheduledFutureTask.access$301(ScheduledThreadPoolExecutor.java:180)
at java.util.concurrent.ScheduledThreadPoolExecutor$ScheduledFutureTask.run(ScheduledThreadPoolExecutor.java:294)
at java.util.concurrent.ThreadPoolExecutor.runWorker(ThreadPoolExecutor.java:1142)
at java.util.concurrent.ThreadPoolExecutor$Worker.run(ThreadPoolExecutor.java:617)
at java.lang.Thread.run(Thread.java:745)
```
In CoarseGrainedSchedulerBackend.scala, driver-revive-thread starts with DriverEndpoint.onStart() and keeps sending the ReviveOffers messages periodically till it gets shutdown as part DriverEndpoint.onStop(). There is no proper coordination between the driver-revive-thread(shutdown) and the RpcEndpoint unregister, RpcEndpoint unregister happens first and then driver-revive-thread shuts down as part of DriverEndpoint.onStop(), In-between driver-revive-thread may try to send the ReviveOffers message which is leading to the above exception.
To fix this issue, this PR moves the shutting down of driver-revive-thread to CoarseGrainedSchedulerBackend.stop() which executes before the DriverEndpoint unregister.
**Instance 2:**
```
17/11/10 16:31:38 ERROR cluster.YarnSchedulerBackend$YarnSchedulerEndpoint: Error requesting driver to remove executor 1 for reason Executor for container container_1508535467865_0226_01_000002 exited because of a YARN event (e.g., pre-emption) and not because of an error in the running job.
org.apache.spark.SparkException: Could not find CoarseGrainedScheduler.
at org.apache.spark.rpc.netty.Dispatcher.postMessage(Dispatcher.scala:160)
at org.apache.spark.rpc.netty.Dispatcher.postLocalMessage(Dispatcher.scala:135)
at org.apache.spark.rpc.netty.NettyRpcEnv.ask(NettyRpcEnv.scala:229)
at org.apache.spark.rpc.netty.NettyRpcEndpointRef.ask(NettyRpcEnv.scala:516)
at org.apache.spark.rpc.RpcEndpointRef.ask(RpcEndpointRef.scala:63)
at org.apache.spark.scheduler.cluster.YarnSchedulerBackend$YarnSchedulerEndpoint$$anonfun$receive$1.applyOrElse(YarnSchedulerBackend.scala:269)
at org.apache.spark.rpc.netty.Inbox$$anonfun$process$1.apply$mcV$sp(Inbox.scala:117)
at org.apache.spark.rpc.netty.Inbox.safelyCall(Inbox.scala:205)
at org.apache.spark.rpc.netty.Inbox.process(Inbox.scala:101)
at org.apache.spark.rpc.netty.Dispatcher$MessageLoop.run(Dispatcher.scala:221)
at java.util.concurrent.ThreadPoolExecutor.runWorker(ThreadPoolExecutor.java:1142)
at java.util.concurrent.ThreadPoolExecutor$Worker.run(ThreadPoolExecutor.java:617)
at java.lang.Thread.run(Thread.java:745)
```
Here YarnDriverEndpoint tries to send remove executor messages after the Yarn scheduler backend service stop, which is leading to the above exception. To avoid the above exception,
1) We may add a condition(which checks whether service has stopped or not) before sending executor remove message
2) Add a warn log message in onFailure case when the service is already stopped
In this PR, chosen the 2) option which adds a log message in the case of onFailure without the exception stack trace since the option 1) would need to to go through for every remove executor message.
## How was this patch tested?
I verified it manually, I don't see these exceptions with the PR changes.
Author: Devaraj K <devaraj@apache.org>
Closes#19741 from devaraj-kavali/SPARK-14228.
The main goal of this change is to allow multiple cluster-mode
submissions from the same JVM, without having them end up with
mixed configuration. That is done by extending the SparkApplication
trait, and doing so was reasonably trivial for standalone and
mesos modes.
For YARN mode, there was a complication. YARN used a "SPARK_YARN_MODE"
system property to control behavior indirectly in a whole bunch of
places, mainly in the SparkHadoopUtil / YarnSparkHadoopUtil classes.
Most of the changes here are removing that.
Since we removed support for Hadoop 1.x, some methods that lived in
YarnSparkHadoopUtil can now live in SparkHadoopUtil. The remaining
methods don't need to be part of the class, and can be called directly
from the YarnSparkHadoopUtil object, so now there's a single
implementation of SparkHadoopUtil.
There were two places in the code that relied on SPARK_YARN_MODE to
make decisions about YARN-specific functionality, and now explicitly check
the master from the configuration for that instead:
* fetching the external shuffle service port, which can come from the YARN
configuration.
* propagation of the authentication secret using Hadoop credentials. This also
was cleaned up a little to not need so many methods in `SparkHadoopUtil`.
With those out of the way, actually changing the YARN client
to extend SparkApplication was easy.
Tested with existing unit tests, and also by running YARN apps
with auth and kerberos both on and off in a real cluster.
Author: Marcelo Vanzin <vanzin@cloudera.com>
Closes#19631 from vanzin/SPARK-22372.
## What changes were proposed in this pull request?
- Solves the issue described in the ticket by preserving reservation and allocation info in all cases (port handling included).
- upgrades to 1.4
- Adds extra debug level logging to make debugging easier in the future, for example we add reservation info when applicable.
```
17/09/29 14:53:07 DEBUG MesosCoarseGrainedSchedulerBackend: Accepting offer: f20de49b-dee3-45dd-a3c1-73418b7de891-O32 with attributes: Map() allocation info: role: "spark-prive"
reservation info: name: "ports"
type: RANGES
ranges {
range {
begin: 31000
end: 32000
}
}
role: "spark-prive"
reservation {
principal: "test"
}
allocation_info {
role: "spark-prive"
}
```
- Some style cleanup.
## How was this patch tested?
Manually by running the example in the ticket with and without a principal. Specifically I tested it on a dc/os 1.10 cluster with 7 nodes and played with reservations. From the master node in order to reserve resources I executed:
```for i in 0 1 2 3 4 5 6
do
curl -i \
-d slaveId=90ec65ea-1f7b-479f-a824-35d2527d6d26-S$i \
-d resources='[
{
"name": "cpus",
"type": "SCALAR",
"scalar": { "value": 2 },
"role": "spark-role",
"reservation": {
"principal": ""
}
},
{
"name": "mem",
"type": "SCALAR",
"scalar": { "value": 8026 },
"role": "spark-role",
"reservation": {
"principal": ""
}
}
]' \
-X POST http://master.mesos:5050/master/reserve
done
```
Nodes had 4 cpus (m3.xlarge instances) and I reserved either 2 or 4 cpus (all for a role).
I verified it launches tasks on nodes with reserved resources under `spark-role` role only if
a) there are remaining resources for (*) default role and the spark driver has no role assigned to it.
b) the spark driver has a role assigned to it and it is the same role used in reservations.
I also tested this locally on my machine.
Author: Stavros Kontopoulos <st.kontopoulos@gmail.com>
Closes#19390 from skonto/fix_dynamic_reservation.
## What changes were proposed in this pull request?
This is a stripped down version of the `KubernetesClusterSchedulerBackend` for Spark with the following components:
- Static Allocation of Executors
- Executor Pod Factory
- Executor Recovery Semantics
It's step 1 from the step-wise plan documented [here](https://github.com/apache-spark-on-k8s/spark/issues/441#issuecomment-330802935).
This addition is covered by the [SPIP vote](http://apache-spark-developers-list.1001551.n3.nabble.com/SPIP-Spark-on-Kubernetes-td22147.html) which passed on Aug 31 .
## How was this patch tested?
- The patch contains unit tests which are passing.
- Manual testing: `./build/mvn -Pkubernetes clean package` succeeded.
- It is a **subset** of the entire changelist hosted in http://github.com/apache-spark-on-k8s/spark which is in active use in several organizations.
- There is integration testing enabled in the fork currently [hosted by PepperData](spark-k8s-jenkins.pepperdata.org:8080) which is being moved over to RiseLAB CI.
- Detailed documentation on trying out the patch in its entirety is in: https://apache-spark-on-k8s.github.io/userdocs/running-on-kubernetes.html
cc rxin felixcheung mateiz (shepherd)
k8s-big-data SIG members & contributors: mccheah ash211 ssuchter varunkatta kimoonkim erikerlandson liyinan926 tnachen ifilonenko
Author: Yinan Li <liyinan926@gmail.com>
Author: foxish <ramanathana@google.com>
Author: mcheah <mcheah@palantir.com>
Closes#19468 from foxish/spark-kubernetes-3.
The first scheduled renewal time is is set to the exact expiration time,
and all subsequent renewal times are 75% of the renewal time. This makes
it so that the inital renewal time is also 75%.
## What changes were proposed in this pull request?
Set the initial renewal time to be 75% of renewal time.
## How was this patch tested?
Tested locally in a test HDFS cluster, checking various renewal times.
Author: Kalvin Chau <kalvin.chau@viasat.com>
Closes#19798 from kalvinnchau/fix-inital-renewal-time.
## What changes were proposed in this pull request?
tl;dr: Add a class, `MesosHadoopDelegationTokenManager` that updates delegation tokens on a schedule on the behalf of Spark Drivers. Broadcast renewed credentials to the executors.
## The problem
We recently added Kerberos support to Mesos-based Spark jobs as well as Secrets support to the Mesos Dispatcher (SPARK-16742, SPARK-20812, respectively). However the delegation tokens have a defined expiration. This poses a problem for long running Spark jobs (e.g. Spark Streaming applications). YARN has a solution for this where a thread is scheduled to renew the tokens they reach 75% of their way to expiration. It then writes the tokens to HDFS for the executors to find (uses a monotonically increasing suffix).
## This solution
We replace the current method in `CoarseGrainedSchedulerBackend` which used to discard the token renewal time with a protected method `fetchHadoopDelegationTokens`. Now the individual cluster backends are responsible for overriding this method to fetch and manage token renewal. The delegation tokens themselves, are still part of the `CoarseGrainedSchedulerBackend` as before.
In the case of Mesos renewed Credentials are broadcasted to the executors. This maintains all transfer of Credentials within Spark (as opposed to Spark-to-HDFS). It also does not require any writing of Credentials to disk. It also does not require any GC of old files.
## How was this patch tested?
Manually against a Kerberized HDFS cluster.
Thank you for the reviews.
Author: ArtRand <arand@soe.ucsc.edu>
Closes#19272 from ArtRand/spark-21842-450-kerberos-ticket-renewal.
## What changes were proposed in this pull request?
Removed the unnecessary stagingDirPath null check in ApplicationMaster.cleanupStagingDir().
## How was this patch tested?
I verified with the existing test cases.
Author: Devaraj K <devaraj@apache.org>
Closes#19749 from devaraj-kavali/SPARK-22519.
## What changes were proposed in this pull request?
A discussed in SPARK-19606, the addition of a new config property named "spark.mesos.constraints.driver" for constraining drivers running on a Mesos cluster
## How was this patch tested?
Corresponding unit test added also tested locally on a Mesos cluster
Please review http://spark.apache.org/contributing.html before opening a pull request.
Author: Paul Mackles <pmackles@adobe.com>
Closes#19543 from pmackles/SPARK-19606.
## What changes were proposed in this pull request?
When I ran self contained sql apps, such as
```scala
import org.apache.spark.sql.SparkSession
object ShowHiveTables {
def main(args: Array[String]): Unit = {
val spark = SparkSession
.builder()
.appName("Show Hive Tables")
.enableHiveSupport()
.getOrCreate()
spark.sql("show tables").show()
spark.stop()
}
}
```
with **yarn cluster** mode and `hive-site.xml` correctly within `$SPARK_HOME/conf`,they failed to connect the right hive metestore for not seeing hive-site.xml in AM/Driver's classpath.
Although submitting them with `--files/--jars local/path/to/hive-site.xml` or puting it to `$HADOOP_CONF_DIR/YARN_CONF_DIR` can make these apps works well in cluster mode as client mode, according to the official doc, see http://spark.apache.org/docs/latest/sql-programming-guide.html#hive-tables
> Configuration of Hive is done by placing your hive-site.xml, core-site.xml (for security configuration), and hdfs-site.xml (for HDFS configuration) file in conf/.
We may respect these configuration files too or modify the doc for hive-tables in cluster mode.
## How was this patch tested?
cc cloud-fan gatorsmile
Author: Kent Yao <yaooqinn@hotmail.com>
Closes#19663 from yaooqinn/SPARK-21888.
## Background
In #18837 , ArtRand added Mesos secrets support to the dispatcher. **This PR is to add the same secrets support to the drivers.** This means if the secret configs are set, the driver will launch executors that have access to either env or file-based secrets.
One use case for this is to support TLS in the driver <=> executor communication.
## What changes were proposed in this pull request?
Most of the changes are a refactor of the dispatcher secrets support (#18837) - moving it to a common place that can be used by both the dispatcher and drivers. The same goes for the unit tests.
## How was this patch tested?
There are four config combinations: [env or file-based] x [value or reference secret]. For each combination:
- Added a unit test.
- Tested in DC/OS.
Author: Susan X. Huynh <xhuynh@mesosphere.com>
Closes#19437 from susanxhuynh/sh-mesos-driver-secret.
The bug was introduced in SPARK-22290, which changed how the app's user
is impersonated in the AM. The changed missed an initialization function
that needs to be run as the app owner (who has the right credentials to
read from HDFS).
Author: Marcelo Vanzin <vanzin@cloudera.com>
Closes#19566 from vanzin/SPARK-22341.
Hive delegation tokens are only needed when the Spark driver has no access
to the kerberos TGT. That happens only in two situations:
- when using a proxy user
- when using cluster mode without a keytab
This change modifies the Hive provider so that it only generates delegation
tokens in those situations, and tweaks the YARN AM so that it makes the proper
user visible to the Hive code when running with keytabs, so that the TGT
can be used instead of a delegation token.
The effect of this change is that now it's possible to initialize multiple,
non-concurrent SparkContext instances in the same JVM. Before, the second
invocation would fail to fetch a new Hive delegation token, which then could
make the second (or third or...) application fail once the token expired.
With this change, the TGT will be used to authenticate to the HMS instead.
This change also avoids polluting the current logged in user's credentials
when launching applications. The credentials are copied only when running
applications as a proxy user. This makes it possible to implement SPARK-11035
later, where multiple threads might be launching applications, and each app
should have its own set of credentials.
Tested by verifying HDFS and Hive access in following scenarios:
- client and cluster mode
- client and cluster mode with proxy user
- client and cluster mode with principal / keytab
- long-running cluster app with principal / keytab
- pyspark app that creates (and stops) multiple SparkContext instances
through its lifetime
Author: Marcelo Vanzin <vanzin@cloudera.com>
Closes#19509 from vanzin/SPARK-22290.
## What changes were proposed in this pull request?
Added Launcher support for monitoring Mesos apps in Client mode. SPARK-11033 can handle the support for Mesos/Cluster mode since the Standalone/Cluster and Mesos/Cluster modes use the same code at client side.
## How was this patch tested?
I verified it manually by running launcher application, able to launch, stop and kill the mesos applications and also can invoke other launcher API's.
Author: Devaraj K <devaraj@apache.org>
Closes#19385 from devaraj-kavali/SPARK-11034.
## What changes were proposed in this pull request?
Improve the Spark-Mesos coarse-grained scheduler to consider the preferred locations when dynamic allocation is enabled.
## How was this patch tested?
Added a unittest, and performed manual testing on AWS.
Author: Gene Pang <gene.pang@gmail.com>
Closes#18098 from gpang/mesos_data_locality.
## What changes were proposed in this pull request?
Fix a trivial bug with how metrics are registered in the mesos dispatcher. Bug resulted in creating a new registry each time the metricRegistry() method was called.
## How was this patch tested?
Verified manually on local mesos setup
Author: Paul Mackles <pmackles@adobe.com>
Closes#19358 from pmackles/SPARK-22135.
## What changes were proposed in this pull request?
When the libraries temp directory(i.e. __spark_libs__*.zip dir) file system and staging dir(destination) file systems are the same then the __spark_libs__*.zip is not copying to the staging directory. But after making this decision the libraries zip file is getting deleted immediately and becoming unavailable for the Node Manager's localization.
With this change, client copies the files to remote always when the source scheme is "file".
## How was this patch tested?
I have verified it manually in yarn/cluster and yarn/client modes with hdfs and local file systems.
Author: Devaraj K <devaraj@apache.org>
Closes#19141 from devaraj-kavali/SPARK-21384.
The live listener bus now cleans up after itself and releases listeners
after stopping, so code cannot get references to listeners after the
Spark context is stopped.
Author: Marcelo Vanzin <vanzin@cloudera.com>
Closes#19297 from vanzin/SPARK-18838.hotfix.
…build; fix some things that will be warnings or errors in 2.12; restore Scala 2.12 profile infrastructure
## What changes were proposed in this pull request?
This change adds back the infrastructure for a Scala 2.12 build, but does not enable it in the release or Python test scripts.
In order to make that meaningful, it also resolves compile errors that the code hits in 2.12 only, in a way that still works with 2.11.
It also updates dependencies to the earliest minor release of dependencies whose current version does not yet support Scala 2.12. This is in a sense covered by other JIRAs under the main umbrella, but implemented here. The versions below still work with 2.11, and are the _latest_ maintenance release in the _earliest_ viable minor release.
- Scalatest 2.x -> 3.0.3
- Chill 0.8.0 -> 0.8.4
- Clapper 1.0.x -> 1.1.2
- json4s 3.2.x -> 3.4.2
- Jackson 2.6.x -> 2.7.9 (required by json4s)
This change does _not_ fully enable a Scala 2.12 build:
- It will also require dropping support for Kafka before 0.10. Easy enough, just didn't do it yet here
- It will require recreating `SparkILoop` and `Main` for REPL 2.12, which is SPARK-14650. Possible to do here too.
What it does do is make changes that resolve much of the remaining gap without affecting the current 2.11 build.
## How was this patch tested?
Existing tests and build. Manually tested with `./dev/change-scala-version.sh 2.12` to verify it compiles, modulo the exceptions above.
Author: Sean Owen <sowen@cloudera.com>
Closes#18645 from srowen/SPARK-14280.
Mesos has secrets primitives for environment and file-based secrets, this PR adds that functionality to the Spark dispatcher and the appropriate configuration flags.
Unit tested and manually tested against a DC/OS cluster with Mesos 1.4.
Author: ArtRand <arand@soe.ucsc.edu>
Closes#18837 from ArtRand/spark-20812-dispatcher-secrets-and-labels.
In the current code, if NM recovery is not enabled then `YarnShuffleService` will write shuffle metadata to NM local dir-1, if this local dir-1 is on bad disk, then `YarnShuffleService` will be failed to start. So to solve this issue, in Spark side if NM recovery is not enabled, then Spark will not persist data into leveldb, in that case yarn shuffle service can still be served but lose the ability for recovery, (it is fine because the failure of NM will kill the containers as well as applications).
Tested in the local cluster with NM recovery off and on to see if folder is created or not. MiniCluster UT isn't added because in MiniCluster NM will always set port to 0, but NM recovery requires non-ephemeral port.
Author: jerryshao <sshao@hortonworks.com>
Closes#19032 from jerryshao/SPARK-17321.
Change-Id: I8f2fe73d175e2ad2c4e380caede3873e0192d027
JIRA ticket: https://issues.apache.org/jira/browse/SPARK-21694
## What changes were proposed in this pull request?
Spark already supports launching containers attached to a given CNI network by specifying it via the config `spark.mesos.network.name`.
This PR adds support to pass in network labels to CNI plugins via a new config option `spark.mesos.network.labels`. These network labels are key-value pairs that are set in the `NetworkInfo` of both the driver and executor tasks. More details in the related Mesos documentation: http://mesos.apache.org/documentation/latest/cni/#mesos-meta-data-to-cni-plugins
## How was this patch tested?
Unit tests, for both driver and executor tasks.
Manual integration test to submit a job with the `spark.mesos.network.labels` option, hit the mesos/state.json endpoint, and check that the labels are set in the driver and executor tasks.
ArtRand skonto
Author: Susan X. Huynh <xhuynh@mesosphere.com>
Closes#18910 from susanxhuynh/sh-mesos-cni-labels.
## What changes were proposed in this pull request?
Fix typos
## How was this patch tested?
Existing tests
Author: Andrew Ash <andrew@andrewash.com>
Closes#18996 from ash211/patch-2.
## What changes were proposed in this pull request?
Add Kerberos Support to Mesos. This includes kinit and --keytab support, but does not include delegation token renewal.
## How was this patch tested?
Manually against a Secure DC/OS Apache HDFS cluster.
Author: ArtRand <arand@soe.ucsc.edu>
Author: Michael Gummelt <mgummelt@mesosphere.io>
Closes#18519 from mgummelt/SPARK-16742-kerberos.
This version fixes a few issues in the import order checker; it provides
better error messages, and detects more improper ordering (thus the need
to change a lot of files in this patch). The main fix is that it correctly
complains about the order of packages vs. classes.
As part of the above, I moved some "SparkSession" import in ML examples
inside the "$example on$" blocks; that didn't seem consistent across
different source files to start with, and avoids having to add more on/off blocks
around specific imports.
The new scalastyle also seems to have a better header detector, so a few
license headers had to be updated to match the expected indentation.
Author: Marcelo Vanzin <vanzin@cloudera.com>
Closes#18943 from vanzin/SPARK-21731.
## What changes were proposed in this pull request?
Adds a sandbox link per driver in the dispatcher ui with minimal changes after a bug was fixed here:
https://issues.apache.org/jira/browse/MESOS-4992
The sandbox uri has the following format:
http://<proxy_uri>/#/slaves/\<agent-id\>/ frameworks/ \<scheduler-id\>/executors/\<driver-id\>/browse
For dc/os the proxy uri is <dc/os uri>/mesos. For the dc/os deployment scenario and to make things easier I introduced a new config property named `spark.mesos.proxy.baseURL` which should be passed to the dispatcher when launched using --conf. If no such configuration is detected then no sandbox uri is depicted, and there is an empty column with a header (this can be changed so nothing is shown).
Within dc/os the base url must be a property for the dispatcher that we should add in the future here:
9e7c909c3b/repo/packages/S/spark/26/config.json
It is not easy to detect in different environments what is that uri so user should pass it.
## How was this patch tested?
Tested with the mesos test suite here: https://github.com/typesafehub/mesos-spark-integration-tests.
Attached image shows the ui modification where the sandbox header is added.
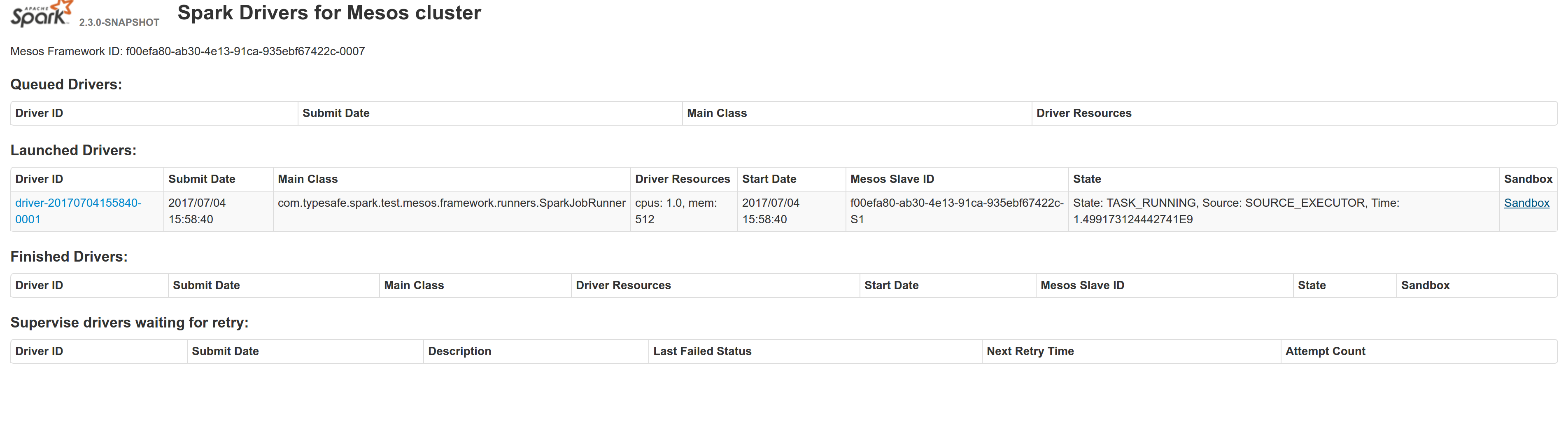
Tested the uri redirection the way it was suggested here:
https://issues.apache.org/jira/browse/MESOS-4992
Built mesos 1.4 from the master branch and started the mesos dispatcher with the command:
`./sbin/start-mesos-dispatcher.sh --conf spark.mesos.proxy.baseURL=http://localhost:5050 -m mesos://127.0.0.1:5050`
Run a spark example:
`./bin/spark-submit --class org.apache.spark.examples.SparkPi --master mesos://10.10.1.79:7078 --deploy-mode cluster --executor-memory 2G --total-executor-cores 2 http://<path>/spark-examples_2.11-2.1.1.jar 10`
Sandbox uri is shown at the bottom of the page:
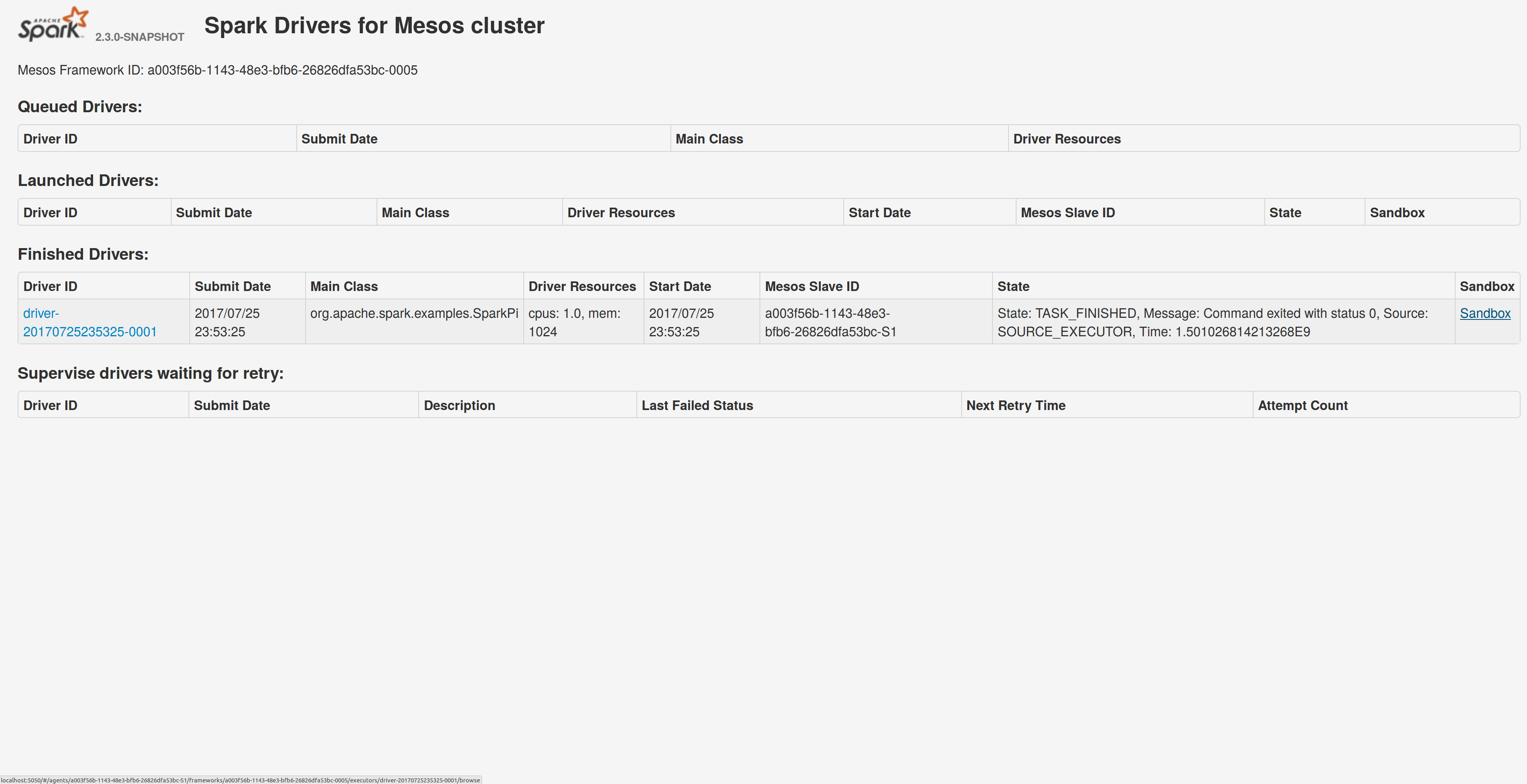
Redirection works as expected:
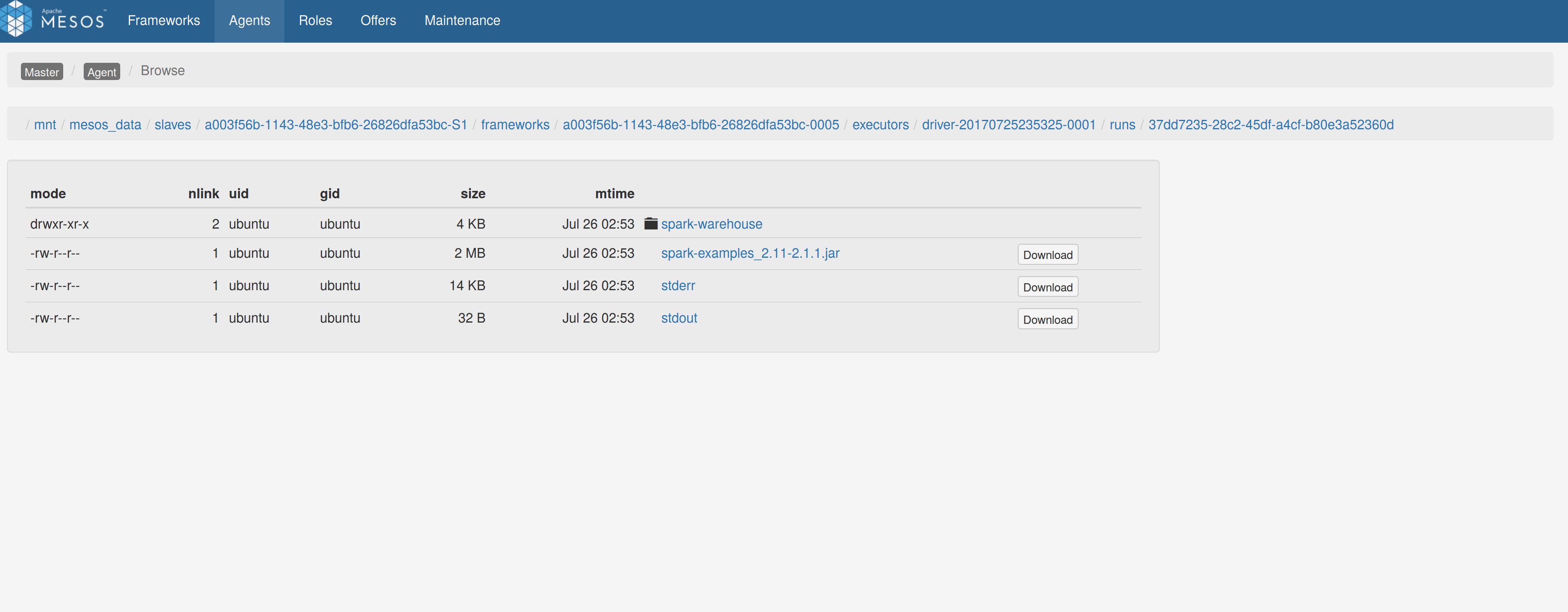
Author: Stavros Kontopoulos <st.kontopoulos@gmail.com>
Closes#18528 from skonto/adds_the_sandbox_uri.
The main goal of this change is to avoid the situation described
in the bug, where an AM restart in the middle of a job may cause
no new executors to be allocated because of faulty logic in the
reset path.
The change does two things:
- fixes the executor alloc manager's reset() so that it does not
stop allocation after a reset() in the middle of a job
- re-orders the initialization of the YarnAllocator class so that
it fetches the current executor ID before triggering the reset()
above.
This ensures both that the new allocator gets new requests for executors,
and that it starts from the correct executor id.
Tested with unit tests and by manually causing AM restarts while
running jobs using spark-shell in YARN mode.
Closes#17882
Author: Marcelo Vanzin <vanzin@cloudera.com>
Author: Guoqiang Li <witgo@qq.com>
Closes#18663 from vanzin/SPARK-20079.
If you run a spark job without creating the SparkSession or SparkContext, the spark job logs says it succeeded but yarn says it fails and retries 3 times. Also, since, Application Master unregisters with Resource Manager and exits successfully, it deletes the spark staging directory, so when yarn makes subsequent retries, it fails to find the staging directory and thus, the retries fail.
Added a flag to check whether user has initialized SparkContext. If it is true, we let Application Master unregister with Resource Manager else, we do not let AM unregister with RM.
## How was this patch tested?
Manually tested the fix.
Before:
<img width="1253" alt="screen shot-before" src="https://user-images.githubusercontent.com/22228190/28647214-69bf81e2-722b-11e7-9ed0-d416d2bf23be.png">
After:
<img width="1319" alt="screen shot-after" src="https://user-images.githubusercontent.com/22228190/28647220-70f9eea2-722b-11e7-85c6-e56276b15614.png">
Please review http://spark.apache.org/contributing.html before opening a pull request.
Author: pgandhi <pgandhi@yahoo-inc.com>
Author: pgandhi999 <parthkgandhi9@gmail.com>
Closes#18741 from pgandhi999/SPARK-21541.
There was some code based on the old SASL handler in the new auth client that
was incorrectly using the SASL user as the user to authenticate against the
external shuffle service. This caused the external service to not be able to
find the correct secret to authenticate the connection, failing the connection.
In the course of debugging, I found that some log messages from the YARN shuffle
service were a little noisy, so I silenced some of them, and also added a couple
of new ones that helped find this issue. On top of that, I found that a check
in the code that records app secrets was wrong, causing more log spam and also
using an O(n) operation instead of an O(1) call.
Also added a new integration suite for the YARN shuffle service with auth on,
and verified it failed before, and passes now.
Author: Marcelo Vanzin <vanzin@cloudera.com>
Closes#18706 from vanzin/SPARK-21494.
When NodeManagers launching Executors,
the `missing` value will exceed the
real value when the launch is slow, this can lead to YARN allocates more resource.
We add the `numExecutorsRunning` when calculate the `missing` to avoid this.
Test by experiment.
Author: DjvuLee <lihu@bytedance.com>
Closes#18651 from djvulee/YarnAllocate.
## What changes were proposed in this pull request?
With supervise enabled for a driver, re-launching it was failing because the driver had the same framework Id. This patch creates a new driver framework id every time we re-launch a driver, but we keep the driver submission id the same since that is the same with the task id the driver was launched with on mesos and retry state and other info within Dispatcher's data structures uses that as a key.
We append a "-retry-%4d" string as a suffix to the framework id passed by the dispatcher to the driver and the same value to the app_id created by each driver, except the first time where we dont need the retry suffix.
The previous format for the frameworkId was 'DispactherFId-DriverSubmissionId'.
We also detect the case where we have multiple spark contexts started from within the same driver and we do set proper names to their corresponding app-ids. The old practice was to unset the framework id passed from the dispatcher after the driver framework was started for the first time and let mesos decide the framework ID for subsequent spark contexts. The decided fId was passed as an appID.
This patch affects heavily the history server. Btw we dont have the issues of the standalone case where driver id must be different since the dispatcher will re-launch a driver(mesos task) only if it gets an update that it is dead and this is verified by mesos implicitly. We also dont fix the fine grained mode which is deprecated and of no use.
## How was this patch tested?
This task was manually tested on dc/os. Launched a driver, stoped its container and verified the expected behavior.
Initial retry of the driver, driver in pending state:

Driver re-launched:
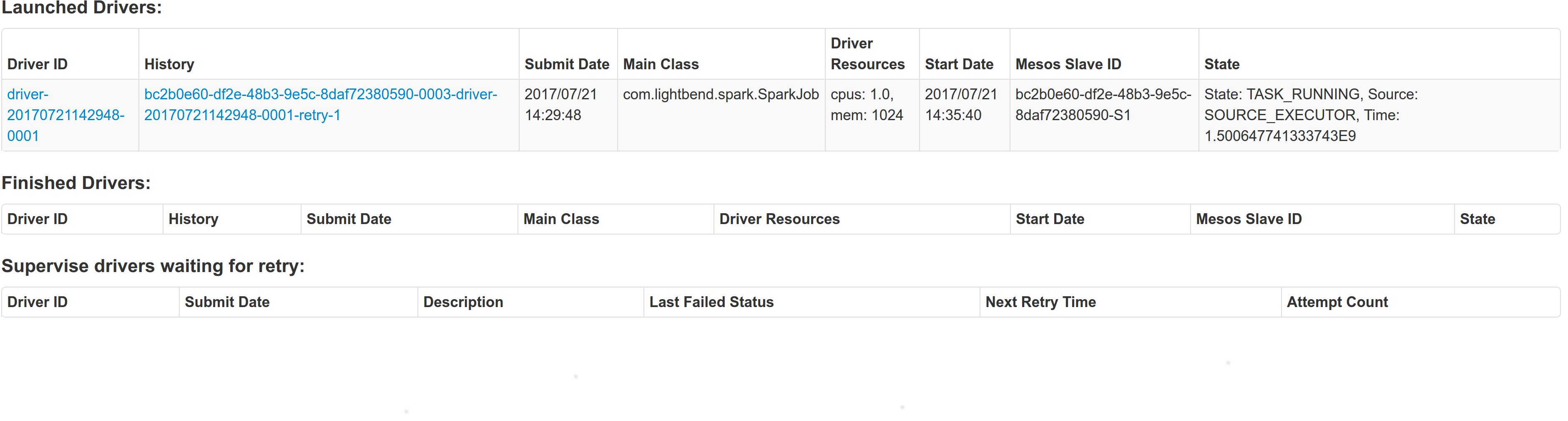
Another re-try:

The resulted entries in history server at the bottom:
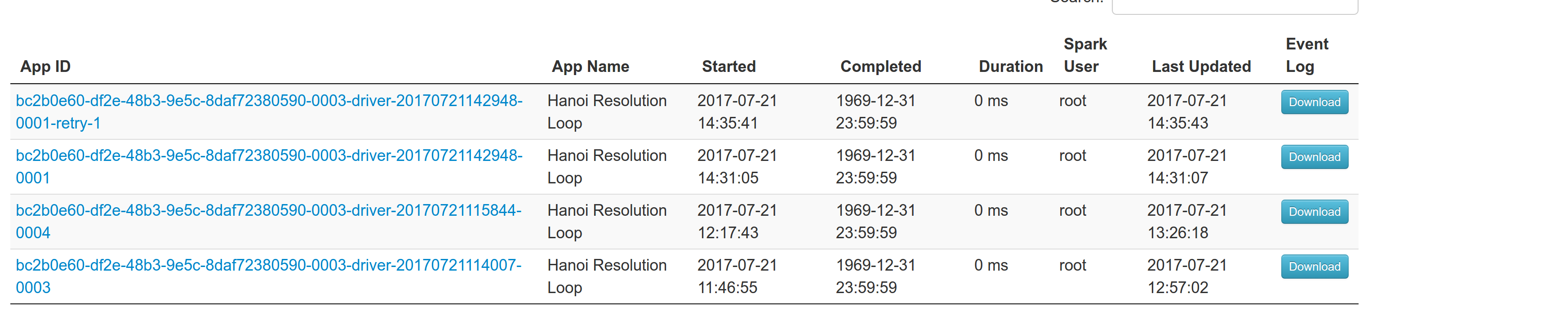
Regarding multiple spark contexts here is the end result regarding the spark history server, for the second spark context we add an increasing number as a suffix:

Author: Stavros Kontopoulos <st.kontopoulos@gmail.com>
Closes#18705 from skonto/fix_supervise_flag.
## What changes were proposed in this pull request?
Current behavior: in Mesos cluster mode, the driver failover_timeout is set to zero. If the driver temporarily loses connectivity with the Mesos master, the framework will be torn down and all executors killed.
Proposed change: make the failover_timeout configurable via a new option, spark.mesos.driver.failoverTimeout. The default value is still zero.
Note: with non-zero failover_timeout, an explicit teardown is needed in some cases. This is captured in https://issues.apache.org/jira/browse/SPARK-21458
## How was this patch tested?
Added a unit test to make sure the config option is set while creating the scheduler driver.
Ran an integration test with mesosphere/spark showing that with a non-zero failover_timeout the Spark job finishes after a driver is disconnected from the master.
Author: Susan X. Huynh <xhuynh@mesosphere.com>
Closes#18674 from susanxhuynh/sh-mesos-failover-timeout.
Instead of using the host's cpu count, use the number of cores allocated
for the Spark process when sizing the RPC dispatch thread pool. This avoids
creating large thread pools on large machines when the number of allocated
cores is small.
Tested by verifying number of threads with spark.executor.cores set
to 1 and 4; same thing for YARN AM.
Author: Marcelo Vanzin <vanzin@cloudera.com>
Closes#18639 from vanzin/SPARK-21408.
## What changes were proposed in this pull request?
In the current `YARNHadoopDelegationTokenManager`, `FileSystem` to which to get tokens are created out of KDC logged UGI, using these `FileSystem` to get new tokens will lead to exception. The main thing is that Spark code trying to get new tokens from the FS created with token auth-ed UGI, but Hadoop can only grant new tokens in kerberized UGI. To fix this issue, we should lazily create these FileSystem within KDC logged UGI.
## How was this patch tested?
Manual verification in secure cluster.
CC vanzin mgummelt please help to review, thanks!
Author: jerryshao <sshao@hortonworks.com>
Closes#18633 from jerryshao/SPARK-21411.
## What changes were proposed in this pull request?
Address scapegoat warnings for:
- BigDecimal double constructor
- Catching NPE
- Finalizer without super
- List.size is O(n)
- Prefer Seq.empty
- Prefer Set.empty
- reverse.map instead of reverseMap
- Type shadowing
- Unnecessary if condition.
- Use .log1p
- Var could be val
In some instances like Seq.empty, I avoided making the change even where valid in test code to keep the scope of the change smaller. Those issues are concerned with performance and it won't matter for tests.
## How was this patch tested?
Existing tests
Author: Sean Owen <sowen@cloudera.com>
Closes#18635 from srowen/Scapegoat1.
## What changes were proposed in this pull request?
In this issue we have a long running Spark application with secure HBase, which requires `HBaseCredentialProvider` to get tokens periodically, we specify HBase related jars with `--packages`, but these dependencies are not added into AM classpath, so when `HBaseCredentialProvider` tries to initialize HBase connections to get tokens, it will be failed.
Currently because jars specified with `--jars` or `--packages` are not added into AM classpath, the only way to extend AM classpath is to use "spark.driver.extraClassPath" which supposed to be used in yarn cluster mode.
So in this fix, we proposed to use/reuse a classloader for `AMCredentialRenewer` to acquire new tokens.
Also in this patch, we fixed AM cannot get tokens from HDFS issue, it is because FileSystem is gotten before kerberos logged, so using this FS to get tokens will throw exception.
## How was this patch tested?
Manual verification.
Author: jerryshao <sshao@hortonworks.com>
Closes#18616 from jerryshao/SPARK-21377.
## What changes were proposed in this pull request?
The current code is very verbose on shutdown.
The changes I propose is to change the log level when the driver is shutting down and the RPC connections are closed (RpcEnvStoppedException).
## How was this patch tested?
Tested with word count(deploy-mode = cluster, master = yarn, num-executors = 4) with 300GB of data.
Author: John Lee <jlee2@yahoo-inc.com>
Closes#18547 from yoonlee95/SPARK-21321.
When localizing the gateway config files in a YARN application, avoid
overwriting final configs by distributing the gateway files to a separate
directory, and explicitly loading them into the Hadoop config, instead
of placing those files before the cluster's files in the classpath.
This is done by saving the gateway's config to a separate XML file
distributed with the rest of the Spark app's config, and loading that
file when creating a new config through `YarnSparkHadoopUtil`.
Tested with existing unit tests, and by verifying the behavior in a YARN
cluster (final values are not overridden, non-final values are).
Author: Marcelo Vanzin <vanzin@cloudera.com>
Closes#18370 from vanzin/SPARK-9825.
## What changes were proposed in this pull request?
This issue happens in long running application with yarn cluster mode, because yarn#client doesn't sync token with AM, so it will always keep the initial token, this token may be expired in the long running scenario, so when yarn#client tries to clean up staging directory after application finished, it will use this expired token and meet token expire issue.
## How was this patch tested?
Manual verification is secure cluster.
Author: jerryshao <sshao@hortonworks.com>
Closes#18617 from jerryshao/SPARK-21376.
## What changes were proposed in this pull request?
Adding the default UncaughtExceptionHandler to the Worker.
## How was this patch tested?
I verified it manually, when any of the worker thread gets uncaught exceptions then the default UncaughtExceptionHandler will handle those exceptions.
Author: Devaraj K <devaraj@apache.org>
Closes#18357 from devaraj-kavali/SPARK-21146.