### What changes were proposed in this pull request? https://github.com/apache/spark/pull/32015 added a way to run benchmarks much more easily in the same GitHub Actions build. This PR updates the benchmark results by using the way. **NOTE** that looks like GitHub Actions use four types of CPU given my observations: - Intel(R) Xeon(R) Platinum 8171M CPU 2.60GHz - Intel(R) Xeon(R) CPU E5-2673 v4 2.30GHz - Intel(R) Xeon(R) CPU E5-2673 v3 2.40GHz - Intel(R) Xeon(R) Platinum 8272CL CPU 2.60GHz Given my quick research, seems like they perform roughly similarly: 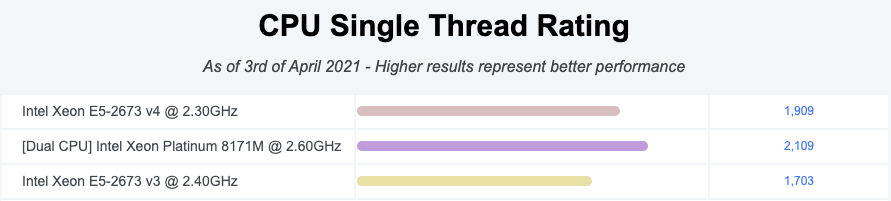 I couldn't find enough information about Intel(R) Xeon(R) Platinum 8272CL CPU 2.60GHz but the performance seems roughly similar given the numbers. So shouldn't be a big deal especially given that this way is much easier, encourages contributors to run more and guarantee the same number of cores and same memory with the same softwares. ### Why are the changes needed? To have a base line of the benchmarks accordingly. ### Does this PR introduce _any_ user-facing change? No, dev-only. ### How was this patch tested? It was generated from: - [Run benchmarks: * (JDK 11)](https://github.com/HyukjinKwon/spark/actions/runs/713575465) - [Run benchmarks: * (JDK 8)](https://github.com/HyukjinKwon/spark/actions/runs/713154337) Closes #32044 from HyukjinKwon/SPARK-34950. Authored-by: HyukjinKwon <gurwls223@apache.org> Signed-off-by: Max Gekk <max.gekk@gmail.com>
68 lines
6.1 KiB
Plaintext
68 lines
6.1 KiB
Plaintext
================================================================================================
|
|
Benchmark to measure CSV read/write performance
|
|
================================================================================================
|
|
|
|
OpenJDK 64-Bit Server VM 11.0.10+9-LTS on Linux 5.4.0-1043-azure
|
|
Intel(R) Xeon(R) CPU E5-2673 v4 @ 2.30GHz
|
|
Parsing quoted values: Best Time(ms) Avg Time(ms) Stdev(ms) Rate(M/s) Per Row(ns) Relative
|
|
------------------------------------------------------------------------------------------------------------------------
|
|
One quoted string 35546 35913 327 0.0 710924.3 1.0X
|
|
|
|
OpenJDK 64-Bit Server VM 11.0.10+9-LTS on Linux 5.4.0-1043-azure
|
|
Intel(R) Xeon(R) CPU E5-2673 v4 @ 2.30GHz
|
|
Wide rows with 1000 columns: Best Time(ms) Avg Time(ms) Stdev(ms) Rate(M/s) Per Row(ns) Relative
|
|
------------------------------------------------------------------------------------------------------------------------
|
|
Select 1000 columns 97613 98458 1123 0.0 97613.1 1.0X
|
|
Select 100 columns 42208 42598 374 0.0 42208.4 2.3X
|
|
Select one column 37602 38233 547 0.0 37601.6 2.6X
|
|
count() 6343 6432 153 0.2 6343.4 15.4X
|
|
Select 100 columns, one bad input field 65577 66403 829 0.0 65577.2 1.5X
|
|
Select 100 columns, corrupt record field 79049 79718 608 0.0 79048.6 1.2X
|
|
|
|
OpenJDK 64-Bit Server VM 11.0.10+9-LTS on Linux 5.4.0-1043-azure
|
|
Intel(R) Xeon(R) CPU E5-2673 v4 @ 2.30GHz
|
|
Count a dataset with 10 columns: Best Time(ms) Avg Time(ms) Stdev(ms) Rate(M/s) Per Row(ns) Relative
|
|
------------------------------------------------------------------------------------------------------------------------
|
|
Select 10 columns + count() 17730 18004 321 0.6 1773.0 1.0X
|
|
Select 1 column + count() 12627 12858 292 0.8 1262.7 1.4X
|
|
count() 4329 4425 130 2.3 432.9 4.1X
|
|
|
|
OpenJDK 64-Bit Server VM 11.0.10+9-LTS on Linux 5.4.0-1043-azure
|
|
Intel(R) Xeon(R) CPU E5-2673 v4 @ 2.30GHz
|
|
Write dates and timestamps: Best Time(ms) Avg Time(ms) Stdev(ms) Rate(M/s) Per Row(ns) Relative
|
|
------------------------------------------------------------------------------------------------------------------------
|
|
Create a dataset of timestamps 1539 1582 47 6.5 153.9 1.0X
|
|
to_csv(timestamp) 12782 12980 192 0.8 1278.2 0.1X
|
|
write timestamps to files 10122 10253 170 1.0 1012.2 0.2X
|
|
Create a dataset of dates 1646 1765 111 6.1 164.6 0.9X
|
|
to_csv(date) 9004 9216 200 1.1 900.4 0.2X
|
|
write dates to files 6519 6615 148 1.5 651.9 0.2X
|
|
|
|
OpenJDK 64-Bit Server VM 11.0.10+9-LTS on Linux 5.4.0-1043-azure
|
|
Intel(R) Xeon(R) CPU E5-2673 v4 @ 2.30GHz
|
|
Read dates and timestamps: Best Time(ms) Avg Time(ms) Stdev(ms) Rate(M/s) Per Row(ns) Relative
|
|
------------------------------------------------------------------------------------------------------------------------
|
|
read timestamp text from files 2364 2428 90 4.2 236.4 1.0X
|
|
read timestamps from files 39799 39885 96 0.3 3979.9 0.1X
|
|
infer timestamps from files 79083 79866 818 0.1 7908.3 0.0X
|
|
read date text from files 2174 2202 37 4.6 217.4 1.1X
|
|
read date from files 17997 18249 218 0.6 1799.7 0.1X
|
|
infer date from files 21635 21893 223 0.5 2163.5 0.1X
|
|
timestamp strings 2686 2719 28 3.7 268.6 0.9X
|
|
parse timestamps from Dataset[String] 40089 41277 1071 0.2 4008.9 0.1X
|
|
infer timestamps from Dataset[String] 78144 78581 524 0.1 7814.4 0.0X
|
|
date strings 2899 2974 74 3.4 289.9 0.8X
|
|
parse dates from Dataset[String] 19762 19875 99 0.5 1976.2 0.1X
|
|
from_csv(timestamp) 39890 40339 725 0.3 3989.0 0.1X
|
|
from_csv(date) 18501 18740 214 0.5 1850.1 0.1X
|
|
|
|
OpenJDK 64-Bit Server VM 11.0.10+9-LTS on Linux 5.4.0-1043-azure
|
|
Intel(R) Xeon(R) CPU E5-2673 v4 @ 2.30GHz
|
|
Filters pushdown: Best Time(ms) Avg Time(ms) Stdev(ms) Rate(M/s) Per Row(ns) Relative
|
|
------------------------------------------------------------------------------------------------------------------------
|
|
w/o filters 25642 25863 202 0.0 256422.1 1.0X
|
|
pushdown disabled 24703 25195 476 0.0 247029.0 1.0X
|
|
w/ filters 1184 1209 27 0.1 11842.4 21.7X
|
|
|
|
|