## What changes were proposed in this pull request?
LEGACY_DRIVER_IDENTIFIER and its reference are removed.
corresponding references test are updated.
## How was this patch tested?
tested UT test cases
Closes#24026 from shivusondur/newjira2.
Authored-by: shivusondur <shivusondur@gmail.com>
Signed-off-by: Sean Owen <sean.owen@databricks.com>
## What changes were proposed in this pull request?
The change just use variable(_taskScheduler) instead of function(taskScheduler) to keep the format uniform in different situation.
## How was this patch tested?
Please review http://spark.apache.org/contributing.html before opening a pull request.
Closes#24048 from hddong/Use-variable-instead-of-function.
Authored-by: hongdongdong <hongdongdong@cmss.chinamobile.com>
Signed-off-by: Sean Owen <sean.owen@databricks.com>
## What changes were proposed in this pull request?
This PR proposes to have one base R runner.
In the high level,
Previously, it had `ArrowRRunner` and it inherited `RRunner`:
```
└── RRunner
└── ArrowRRunner
```
After this PR, now it has a `BaseRRunner`, and `ArrowRRunner` and `RRunner` inherit `BaseRRunner`:
```
└── BaseRRunner
├── ArrowRRunner
└── RRunner
```
This way is consistent with Python's.
In more details, see below:
```scala
class BaseRRunner[IN, OUT] {
def compute: Iterator[OUT] = {
...
newWriterThread(...).start()
...
newReaderIterator(...)
...
}
// Make a thread that writes data from JVM to R process
abstract protected def newWriterThread(..., iter: Iterator[IN], ...): WriterThread
// Make an iterator that reads data from the R process to JVM
abstract protected def newReaderIterator(...): ReaderIterator
abstract class WriterThread(..., iter: Iterator[IN], ...) extends Thread {
override def run(): Unit {
...
writeIteratorToStream(...)
...
}
// Actually writing logic to the socket stream.
abstract protected def writeIteratorToStream(dataOut: DataOutputStream): Unit
}
abstract class ReaderIterator extends Iterator[OUT] {
override def hasNext(): Boolean = {
...
read(...)
...
}
override def next(): OUT = {
...
hasNext()
...
}
// Actually reading logic from the socket stream.
abstract protected def read(...): OUT
}
}
```
```scala
case [Arrow]RRunner extends BaseRRunner {
override def newWriterThread(...) {
new WriterThread(...) {
override def writeIteratorToStream(...) {
...
}
}
}
override def newReaderIterator(...) {
new ReaderIterator(...) {
override def read(...) {
...
}
}
}
}
```
## How was this patch tested?
Manually tested and existing tests should cover.
Closes#23977 from HyukjinKwon/SPARK-26923.
Authored-by: Hyukjin Kwon <gurwls223@apache.org>
Signed-off-by: Hyukjin Kwon <gurwls223@apache.org>
This code is from the era when Spark used an HTTP server to distribute
dependencies, which is long gone. Nowadays it only causes problems when
someone is using dependencies from an HTTP server with Spark auth on.
Closes#24033 from vanzin/SPARK-27004.
Authored-by: Marcelo Vanzin <vanzin@cloudera.com>
Signed-off-by: Marcelo Vanzin <vanzin@cloudera.com>
## What changes were proposed in this pull request?
Environment tab in SparkUI do not have Hadoop Configuration sorted. All other tables in the same page like Spark Configrations, System Configuration etc are sorted by keys by default
## How was this patch tested?
Manually tested on SparkUI
Closes#24038 from ajithme/sqluisort.
Authored-by: Ajith <ajith2489@gmail.com>
Signed-off-by: Sean Owen <sean.owen@databricks.com>
## What changes were proposed in this pull request?
Web UI URLs are pointing to `http://` targets even if SSL is enabled. In this PR I've changed the code to point to `https://` URLs.
## How was this patch tested?
Existing unit tests + manually by starting standalone master/worker/spark-shell. Please see jira.
Closes#23991 from gaborgsomogyi/SPARK-24621.
Authored-by: Gabor Somogyi <gabor.g.somogyi@gmail.com>
Signed-off-by: Sean Owen <sean.owen@databricks.com>
## What changes were proposed in this pull request?
Currently, R's Scala codes happened to refer Python's Scala codes for code deduplications. It's a bit odd. For instance, when we face an exception from R, it shows python related code path, which makes confusing to debug. It should rather have one code base and R's and Python's should share.
This PR proposes:
1. Make a `SocketAuthServer` and move `PythonServer` so that `PythonRDD` and `RRDD` can share it.
2. Move `readRDDFromFile` and `readRDDFromInputStream` into `JavaRDD`.
3. Reuse `RAuthHelper` and remove `RSocketAuthHelper` in `RRDD`.
4. Rename `getEncryptionEnabled` to `isEncryptionEnabled` while I am here.
So, now, the places below:
- `sql/core/src/main/scala/org/apache/spark/sql/api/r`
- `core/src/main/scala/org/apache/spark/api/r`
- `mllib/src/main/scala/org/apache/spark/ml/r`
don't refer Python's Scala codes.
## How was this patch tested?
Existing tests should cover this.
Closes#24023 from HyukjinKwon/SPARK-27102.
Authored-by: Hyukjin Kwon <gurwls223@apache.org>
Signed-off-by: Hyukjin Kwon <gurwls223@apache.org>
## What changes were proposed in this pull request?
Spark SQL performs whole-stage code generation to speed up query execution. There are two steps to it:
- Java source code is generated from the physical query plan on the driver. A single version of the source code is generated from a query plan, and sent to all executors.
- It's compiled to bytecode on the driver to catch compilation errors before sending to executors, but currently only the generated source code gets sent to the executors. The bytecode compilation is for fail-fast only.
- Executors receive the generated source code and compile to bytecode, then the query runs like a hand-written Java program.
In this model, there's an implicit assumption about the driver and executors being run on similar platforms. Some code paths accidentally embedded platform-dependent object layout information into the generated code, such as:
```java
Platform.putLong(buffer, /* offset */ 24, /* value */ 1);
```
This code expects a field to be at offset +24 of the `buffer` object, and sets a value to that field.
But whole-stage code generation generally uses platform-dependent information from the driver. If the object layout is significantly different on the driver and executors, the generated code can be reading/writing to wrong offsets on the executors, causing all kinds of data corruption.
One code pattern that leads to such problem is the use of `Platform.XXX` constants in generated code, e.g. `Platform.BYTE_ARRAY_OFFSET`.
Bad:
```scala
val baseOffset = Platform.BYTE_ARRAY_OFFSET
// codegen template:
s"Platform.putLong($buffer, $baseOffset, $value);"
```
This will embed the value of `Platform.BYTE_ARRAY_OFFSET` on the driver into the generated code.
Good:
```scala
val baseOffset = "Platform.BYTE_ARRAY_OFFSET"
// codegen template:
s"Platform.putLong($buffer, $baseOffset, $value);"
```
This will generate the offset symbolically -- `Platform.putLong(buffer, Platform.BYTE_ARRAY_OFFSET, value)`, which will be able to pick up the correct value on the executors.
Caveat: these offset constants are declared as runtime-initialized `static final` in Java, so they're not compile-time constants from the Java language's perspective. It does lead to a slightly increased size of the generated code, but this is necessary for correctness.
NOTE: there can be other patterns that generate platform-dependent code on the driver which is invalid on the executors. e.g. if the endianness is different between the driver and the executors, and if some generated code makes strong assumption about endianness, it would also be problematic.
## How was this patch tested?
Added a new test suite `WholeStageCodegenSparkSubmitSuite`. This test suite needs to set the driver's extraJavaOptions to force the driver and executor use different Java object layouts, so it's run as an actual SparkSubmit job.
Authored-by: Kris Mok <kris.mokdatabricks.com>
Closes#24031 from gatorsmile/cherrypickSPARK-27097.
Lead-authored-by: Kris Mok <kris.mok@databricks.com>
Co-authored-by: gatorsmile <gatorsmile@gmail.com>
Signed-off-by: DB Tsai <d_tsai@apple.com>
## What changes were proposed in this pull request?
This is another attempt to fix the more-than-one-active-task-set-managers bug.
https://github.com/apache/spark/pull/17208 is the first attempt. It marks the TSM as zombie before sending a task completion event to DAGScheduler. This is necessary, because when the DAGScheduler gets the task completion event, and it's for the last partition, then the stage is finished. However, if it's a shuffle stage and it has missing map outputs, DAGScheduler will resubmit it(see the [code](https://github.com/apache/spark/blob/v2.4.0/core/src/main/scala/org/apache/spark/scheduler/DAGScheduler.scala#L1416-L1422)) and create a new TSM for this stage. This leads to more than one active TSM of a stage and fail.
This fix has a hole: Let's say a stage has 10 partitions and 2 task set managers: TSM1(zombie) and TSM2(active). TSM1 has a running task for partition 10 and it completes. TSM2 finishes tasks for partitions 1-9, and thinks he is still active because he hasn't finished partition 10 yet. However, DAGScheduler gets task completion events for all the 10 partitions and thinks the stage is finished. Then the same problem occurs: DAGScheduler may resubmit the stage and cause more than one actice TSM error.
https://github.com/apache/spark/pull/21131 fixed this hole by notifying all the task set managers when a task finishes. For the above case, TSM2 will know that partition 10 is already completed, so he can mark himself as zombie after partitions 1-9 are completed.
However, #21131 still has a hole: TSM2 may be created after the task from TSM1 is completed. Then TSM2 can't get notified about the task completion, and leads to the more than one active TSM error.
#22806 and #23871 are created to fix this hole. However the fix is complicated and there are still ongoing discussions.
This PR proposes a simple fix, which can be easy to backport: mark all existing task set managers as zombie when trying to create a new task set manager.
After this PR, #21131 is still necessary, to avoid launching unnecessary tasks and fix [SPARK-25250](https://issues.apache.org/jira/browse/SPARK-25250 ). #22806 and #23871 are its followups to fix the hole.
## How was this patch tested?
existing tests.
Closes#23927 from cloud-fan/scheduler.
Authored-by: Wenchen Fan <wenchen@databricks.com>
Signed-off-by: Imran Rashid <irashid@cloudera.com>
## What changes were proposed in this pull request?
This is an optional solution for #22806 .
#21131 firstly implement that a previous successful completed task from zombie TaskSetManager could also succeed the active TaskSetManager, which based on an assumption that an active TaskSetManager always exists for that stage when this happen. But that's not always true as an active TaskSetManager may haven't been created when a previous task succeed, and this is the reason why #22806 hit the issue.
This pr extends #21131 's behavior by adding `stageIdToFinishedPartitions` into TaskSchedulerImpl, which recording the finished partition whenever a task(from zombie or active) succeed. Thus, a later created active TaskSetManager could also learn about the finished partition by looking into `stageIdToFinishedPartitions ` and won't launch any duplicate tasks.
## How was this patch tested?
Add.
Closes#23871 from Ngone51/dev-23433-25250.
Lead-authored-by: wuyi <ngone_5451@163.com>
Co-authored-by: Ngone51 <ngone_5451@163.com>
Signed-off-by: Imran Rashid <irashid@cloudera.com>
## What changes were proposed in this pull request?
Spelling mistake: forword -> forward
## How was this patch tested?
This is a private function, there is no place to call this function outside of this file.
Closes#23978 from moqimoqidea/master.
Authored-by: moqimoqidea <39821951+moqimoqidea@users.noreply.github.com>
Signed-off-by: Hyukjin Kwon <gurwls223@apache.org>
## What changes were proposed in this pull request?
Creating an Netty `EventLoopGroup` leads to creating a new Thread pool for handling the events. For stopping the threads of the pool the event loop group should be shut down which is properly done for transport servers and clients by calling for example the `shutdownGracefully()` method (for details see the `close()` method of `TransportClientFactory` and `TransportServer`). But there is a separate event loop group for shuffle chunk fetch requests which is in pipeline for handling fetch request (shared between the client and server) and owned by the `TransportContext` and this was never shut down.
## How was this patch tested?
With existing unittest.
This leak is in the production system too but its effect is spiking in the unittest.
Checking the core unittest logs before the PR:
```
$ grep "LEAK IN SUITE" unit-tests.log | grep -o shuffle-chunk-fetch-handler | wc -l
381
```
And after the PR without whitelisting in thread audit and with an extra `await` after the
` chunkFetchWorkers.shutdownGracefully()`:
```
$ grep "LEAK IN SUITE" unit-tests.log | grep -o shuffle-chunk-fetch-handler | wc -l
0
```
Closes#23930 from attilapiros/SPARK-27021.
Authored-by: “attilapiros” <piros.attila.zsolt@gmail.com>
Signed-off-by: Marcelo Vanzin <vanzin@cloudera.com>
## What changes were proposed in this pull request?
This proposes to add instrumentation for the driver's JVM CPU time via the Spark Dropwizard/Codahale metrics system. It follows directly from previous work SPARK-25228 and shares similar motivations: it is intended as an improvement to be used for Spark performance dashboards and monitoring tools/instrumentation.
Implementation details: this PR takes the code introduced in SPARK-25228 and moves it to a new separate Source JVMCPUSource, which is then used to register the jvmCpuTime gauge metric for both executor and driver.
The registration of the jvmCpuTime metric for the driver is conditional, a new configuration parameter `spark.metrics.cpu.time.driver.enabled` (proposed default: false) is introduced for this purpose.
## How was this patch tested?
Manually tested, using local mode and using YARN.
Closes#23838 from LucaCanali/addCPUTimeMetricDriver.
Authored-by: Luca Canali <luca.canali@cern.ch>
Signed-off-by: Marcelo Vanzin <vanzin@cloudera.com>
## What changes were proposed in this pull request?
After we cache a table, we can see its details in Storage Tab of spark UI. If the executor has shutdown ( graceful shutdown/ Dynamic executor scenario) UI still shows the rdd as cached and when we click the link it throws error. This is because on executor remove event, we fail to adjust rdd partition details org.apache.spark.status.AppStatusListener#onExecutorRemoved
## How was this patch tested?
Have tested this fix in UI manually
Edit: Added UT
Closes#23920 from ajithme/cachestorage.
Authored-by: Ajith <ajith2489@gmail.com>
Signed-off-by: Marcelo Vanzin <vanzin@cloudera.com>
## What changes were proposed in this pull request?
Clarify that text DataSource read/write, and RDD methods that read text, always use UTF-8 as they use Hadoop's implementation underneath. I think these are all the places that this needs a mention in the user-facing docs.
## How was this patch tested?
Doc tests.
Closes#23962 from srowen/SPARK-26016.
Authored-by: Sean Owen <sean.owen@databricks.com>
Signed-off-by: Hyukjin Kwon <gurwls223@apache.org>
## What changes were proposed in this pull request?
This pr makes it get proxy user's delegation token, otherwise throws `AccessControlException`([full log](https://issues.apache.org/jira/browse/SPARK-25689?focusedCommentId=16780609&page=com.atlassian.jira.plugin.system.issuetabpanels%3Acomment-tabpanel#comment-16780609)):
```java
org.apache.hadoop.security.AccessControlException: Client cannot authenticate via:[TOKEN, KERBEROS]
...
at org.apache.spark.scheduler.cluster.YarnClientSchedulerBackend.waitForApplication(YarnClientSchedulerBackend.scala:95)
at org.apache.spark.scheduler.cluster.YarnClientSchedulerBackend.start(YarnClientSchedulerBackend.scala:62)
at org.apache.spark.scheduler.TaskSchedulerImpl.start(TaskSchedulerImpl.scala:185)
```
How to reproduce this issue:
```shell
$ ssh user_admspark-getaway-host1
$ export HADOOP_PROXY_USER=user_a
$ spark-sql --master yarn
```
## How was this patch tested?
Test on our production environment.
Closes#23922 from wangyum/SPARK-25689.
Authored-by: Yuming Wang <yumwang@ebay.com>
Signed-off-by: Marcelo Vanzin <vanzin@cloudera.com>
## What changes were proposed in this pull request?
Only memory usage without GC information could not help us to determinate the proper settings of memory. We need the GC metrics about frequency of major & minor GC. For example, two cases, their configured memory for executor are all 10GB and their usages are all near 10GB. So should we increase or decrease the configured memory for them? This metrics may be helpful. We can increase configured memory for the first one if it has very frequency major GC and decrease the second one if only some minor GC and none major GC.
GC metrics are only useful in entire lifetime of executors instead of separated stages.
## How was this patch tested?
Adding UT.
Closes#22874 from LantaoJin/SPARK-25865.
Authored-by: LantaoJin <jinlantao@gmail.com>
Signed-off-by: Imran Rashid <irashid@cloudera.com>
## What changes were proposed in this pull request?
[SPARK-23155](https://issues.apache.org/jira/browse/SPARK-23155) enables SHS to set up custom executor log URLs. This patch proposes to extend this feature to to Spark UI as well.
Unlike the approach we did for SHS (replace executor log URLs when executor information is requested so it's like a change of view), here this patch replaces executor log URLs while registering executor, which also affects event log as well. In point of SHS's view, it will be treated as original log url when custom log url is applied to Spark UI.
## How was this patch tested?
Added UT.
Closes#23790 from HeartSaVioR/SPARK-26792.
Authored-by: Jungtaek Lim (HeartSaVioR) <kabhwan@gmail.com>
Signed-off-by: Marcelo Vanzin <vanzin@cloudera.com>
## What changes were proposed in this pull request?
Fix warn against subclassing scala.App
## How was this patch tested?
Manual test
Closes#23903 from manuzhang/fix_submit_warning.
Authored-by: manuzhang <owenzhang1990@gmail.com>
Signed-off-by: Sean Owen <sean.owen@databricks.com>
## What changes were proposed in this pull request?
`MetricsSystem` instance creations have a scattered distribution in the project code. So do their names. It may cause some inconvenience for browsing and management.
This PR tries to put them together. In this way, we can have a uniform location for adding or removing them, and have a overall view of `MetircsSystem `instances in current project.
It's also helpful for maintaining user documents by avoiding missing something.
## How was this patch tested?
Existing unit tests.
Closes#23869 from SongYadong/metrics_system_inst_manage.
Lead-authored-by: SongYadong <song.yadong1@zte.com.cn>
Co-authored-by: walter2001 <ydsong2007@163.com>
Signed-off-by: Sean Owen <sean.owen@databricks.com>
## What changes were proposed in this pull request?
Redundant `get` when getting a value from `Map` given a key.
## How was this patch tested?
N/A
Closes#23901 from 10110346/removegetfrommap.
Authored-by: liuxian <liu.xian3@zte.com.cn>
Signed-off-by: Sean Owen <sean.owen@databricks.com>
## What changes were proposed in this pull request?
Add standard deviation to the stats taken during benchmark testing.
## How was this patch tested?
Manually ran a few benchmark tests locally and visually inspected the output
Closes#23914 from yifeih/spark-27009-stdev.
Authored-by: Yifei Huang <yifeih@palantir.com>
Signed-off-by: Dongjoon Hyun <dhyun@apple.com>
A couple of places in TaskSchedulerImpl could use a minor doc update on
threading concerns. There is one bug fix here, but only in
sc.killTaskAttempt() which is probably not used much.
Closes#23874 from squito/SPARK-26774.
Authored-by: Imran Rashid <irashid@cloudera.com>
Signed-off-by: Marcelo Vanzin <vanzin@cloudera.com>
## What changes were proposed in this pull request?
Currently, if I run `spark-shell` in my local, it started to show the logs as below:
```
$ ./bin/spark-shell
...
19/02/28 04:42:43 INFO SecurityManager: Changing view acls to: hkwon
19/02/28 04:42:43 INFO SecurityManager: Changing modify acls to: hkwon
19/02/28 04:42:43 INFO SecurityManager: Changing view acls groups to:
19/02/28 04:42:43 INFO SecurityManager: Changing modify acls groups to:
19/02/28 04:42:43 INFO SecurityManager: SecurityManager: authentication disabled; ui acls disabled; users with view permissions: Set(hkwon); groups with view permissions: Set(); users with modify permissions: Set(hkwon); groups with modify permissions: Set()
19/02/28 04:42:43 INFO SignalUtils: Registered signal handler for INT
19/02/28 04:42:48 INFO SparkContext: Running Spark version 3.0.0-SNAPSHOT
19/02/28 04:42:48 INFO SparkContext: Submitted application: Spark shell
19/02/28 04:42:48 INFO SecurityManager: Changing view acls to: hkwon
```
Seems to be the cause is https://github.com/apache/spark/pull/23806 and `prepareSubmitEnvironment` looks actually reinitializing the logging again.
This PR proposes to uninitializing log later after `prepareSubmitEnvironment`.
## How was this patch tested?
Manually tested.
Closes#23911 from HyukjinKwon/SPARK-26895.
Authored-by: Hyukjin Kwon <gurwls223@apache.org>
Signed-off-by: Marcelo Vanzin <vanzin@cloudera.com>
Before this change, there was some code in the k8s backend to deal
with how to resolve dependencies and make them available to the
Spark application. It turns out that none of that code is necessary,
since spark-submit already handles all that for applications started
in client mode - like the k8s driver that is run inside a Spark-created
pod.
For that reason, specifically for pyspark, there's no need for the
k8s backend to deal with PYTHONPATH; or, in general, to change the URIs
provided by the user at all. spark-submit takes care of that.
For testing, I created a pyspark script that depends on another module
that is shipped with --py-files. Then I used:
- --py-files http://.../dep.pyhttp://.../test.py
- --py-files http://.../dep.ziphttp://.../test.py
- --py-files local:/.../dep.py local:/.../test.py
- --py-files local:/.../dep.zip local:/.../test.py
Without this change, all of the above commands fail. With the change, the
driver is able to see the dependencies in all the above cases; but executors
don't see the dependencies in the last two. That's a bug in shared Spark code
that deals with local: dependencies in pyspark (SPARK-26934).
I also tested a Scala app using the main jar from an http server.
Closes#23793 from vanzin/SPARK-24736.
Authored-by: Marcelo Vanzin <vanzin@cloudera.com>
Signed-off-by: Marcelo Vanzin <vanzin@cloudera.com>
## What changes were proposed in this pull request?
Comparing whether Boolean expression is equal to true is redundant
For example:
The datatype of `a` is boolean.
Before:
if (a == true)
After:
if (a)
## How was this patch tested?
N/A
Closes#23884 from 10110346/simplifyboolean.
Authored-by: liuxian <liu.xian3@zte.com.cn>
Signed-off-by: Sean Owen <sean.owen@databricks.com>
## What changes were proposed in this pull request?
This patch applies redaction to command line arguments before logging them. This applies to two resource managers: standalone cluster and YARN.
This patch only concerns about arguments starting with `-D` since Spark is likely passing the Spark configuration to command line arguments as `-Dspark.blabla=blabla`. More change is necessary if we also want to handle the case of `--conf spark.blabla=blabla`.
## How was this patch tested?
Added UT for redact logic. This patch only touches how to log so not easy to add UT regarding it.
Closes#23820 from HeartSaVioR/MINOR-redact-command-line-args-for-running-driver-executor.
Authored-by: Jungtaek Lim (HeartSaVioR) <kabhwan@gmail.com>
Signed-off-by: Marcelo Vanzin <vanzin@cloudera.com>
## What changes were proposed in this pull request?
In the PR, I propose to refactor existing code related to date/time conversions, and replace constants like `1000` and `1000000` by `DateTimeUtils` constants and transformation functions from `java.util.concurrent.TimeUnit._`.
## How was this patch tested?
The changes are tested by existing test suites.
Closes#23878 from MaxGekk/magic-time-constants.
Lead-authored-by: Maxim Gekk <max.gekk@gmail.com>
Co-authored-by: Maxim Gekk <maxim.gekk@databricks.com>
Signed-off-by: Sean Owen <sean.owen@databricks.com>
Since the yarn module is actually private to Spark, this interface was never
actually "public". Since it has no use inside of Spark, let's avoid adding
a yarn-specific extension that isn't public, and point any potential users
are more general solutions (like using a SparkListener).
Closes#23839 from vanzin/SPARK-26788.
Authored-by: Marcelo Vanzin <vanzin@cloudera.com>
Signed-off-by: Sean Owen <sean.owen@databricks.com>
Before this PR the method `BlockManager#putBlockDataAsStream()` (which is used during block replication where the block data is received as a stream) was reading the whole block content into the memory even at DISK_ONLY storage level.
With this change the received block data (which was temporary stored in a file) is just simply moved into the right location backing the target block. This way a possible OOM error is avoided.
In this implementation to save code duplications the method `doPutBytes` is refactored into a template method called `BlockStoreUpdater` which has a separate implementation to handle byte buffer based and temporary file based block store updates.
With existing unit tests of `DistributedSuite` (the ones dealing with replications):
- caching on disk, replicated (encryption = off) (with replication as stream)
- caching on disk, replicated (encryption = on) (with replication as stream)
- caching in memory, serialized, replicated (encryption = on) (with replication as stream)
- caching in memory, serialized, replicated (encryption = off) (with replication as stream)
- etc.
And with new unit tests testing `putBlockDataAsStream` method directly:
- test putBlockDataAsStream with caching (encryption = off)
- test putBlockDataAsStream with caching (encryption = on)
- test putBlockDataAsStream with caching on disk (encryption = off)
- test putBlockDataAsStream with caching on disk (encryption = on)
Closes#23688 from attilapiros/SPARK-25035.
Authored-by: “attilapiros” <piros.attila.zsolt@gmail.com>
Signed-off-by: Marcelo Vanzin <vanzin@cloudera.com>
## What changes were proposed in this pull request?
In the PR, I propose to test the input showed at the end of the article: https://arxiv.org/pdf/1805.08612.pdf . The difference of the test and paper's test is type of array. This test allocates arrays of bytes instead of array of ints.
## How was this patch tested?
New test is added to `SorterSuite`.
Closes#23856 from MaxGekk/timsort-bug-fix.
Authored-by: Maxim Gekk <maxim.gekk@databricks.com>
Signed-off-by: Sean Owen <sean.owen@databricks.com>
## What changes were proposed in this pull request?
This PR aims to remove references to "Shark", which is a precursor to Spark SQL. I searched the whole project for the text "Shark" (ignore case) and just found a single match. Note that occurrences like nickname or test data are irrelevant.
## How was this patch tested?
N/A. Change comments only.
Closes#23876 from seancxmao/remove-Shark.
Authored-by: seancxmao <seancxmao@gmail.com>
Signed-off-by: Sean Owen <sean.owen@databricks.com>
## What changes were proposed in this pull request?
Don't use inaccessible fields in SizeEstimator, which comes up in Java 9+
## How was this patch tested?
Manually ran tests with Java 11; it causes these tests that failed before to pass.
This ought to pass on Java 8 as there's effectively no change for Java 8.
Closes#23866 from srowen/SPARK-26963.
Authored-by: Sean Owen <sean.owen@databricks.com>
Signed-off-by: Sean Owen <sean.owen@databricks.com>
## What changes were proposed in this pull request?
This PR aims to fix some outdated comments about task schedulers.
1. Change "ClusterScheduler" to "YarnScheduler" in comments of `YarnClusterScheduler`
According to [SPARK-1140 Remove references to ClusterScheduler](https://issues.apache.org/jira/browse/SPARK-1140), ClusterScheduler is not used anymore.
I also searched "ClusterScheduler" within the whole project, no other occurrences are found in comments or test cases. Note classes like `YarnClusterSchedulerBackend` or `MesosClusterScheduler` are not relevant.
2. Update comments about `statusUpdate` from `TaskSetManager`
`statusUpdate` has been moved to `TaskSchedulerImpl`. StatusUpdate event handling is delegated to `handleSuccessfulTask`/`handleFailedTask`.
## How was this patch tested?
N/A. Fix comments only.
Closes#23844 from seancxmao/taskscheduler-comments.
Authored-by: seancxmao <seancxmao@gmail.com>
Signed-off-by: Sean Owen <sean.owen@databricks.com>
## What changes were proposed in this pull request?
`prepareSubmitEnvironment` performs globbing that will fail in the case where a proxy user (`--proxy-user`) doesn't have permission to the file. This is a bug also with 2.3, so we should backport, as currently you can't launch an application that for instance is passing a file under `--archives`, and that file is owned by the target user.
The solution is to call `prepareSubmitEnvironment` within a doAs context if proxying.
## How was this patch tested?
Manual tests running with `--proxy-user` and `--archives`, before and after, showing that the globbing is successful when the resource is owned by the target user.
I've looked at writing unit tests, but I am not sure I can do that cleanly (perhaps with a custom FileSystem). Open to ideas.
Please review http://spark.apache.org/contributing.html before opening a pull request.
Closes#23806 from abellina/SPARK-26895_prepareSubmitEnvironment_from_doAs.
Lead-authored-by: Alessandro Bellina <abellina@gmail.com>
Co-authored-by: Alessandro Bellina <abellina@yahoo-inc.com>
Signed-off-by: Marcelo Vanzin <vanzin@cloudera.com>
## What changes were proposed in this pull request?
Spark's TimSort deviates from JDK 11 TimSort in a couple places:
- `stackLen` was increased in jdk
- additional cases for break in `mergeCollapse`: `n < 0`
In the PR, I propose to align Spark TimSort to jdk implementation.
## How was this patch tested?
By existing test suites, in particular, `SorterSuite`.
Closes#23858 from MaxGekk/timsort-java-alignment.
Authored-by: Maxim Gekk <maxim.gekk@databricks.com>
Signed-off-by: Sean Owen <sean.owen@databricks.com>
## What changes were proposed in this pull request?
Currently, RDD.saveAsTextFile may throw NullPointerException then null row is present.
```
scala> sc.parallelize(Seq(1,null),1).saveAsTextFile("/tmp/foobar.dat")
19/02/15 21:39:17 ERROR Utils: Aborting task
java.lang.NullPointerException
at org.apache.spark.rdd.RDD.$anonfun$saveAsTextFile$3(RDD.scala:1510)
at scala.collection.Iterator$$anon$10.next(Iterator.scala:459)
at org.apache.spark.internal.io.SparkHadoopWriter$.$anonfun$executeTask$1(SparkHadoopWriter.scala:129)
at org.apache.spark.util.Utils$.tryWithSafeFinallyAndFailureCallbacks(Utils.scala:1352)
at org.apache.spark.internal.io.SparkHadoopWriter$.executeTask(SparkHadoopWriter.scala:127)
at org.apache.spark.internal.io.SparkHadoopWriter$.$anonfun$write$1(SparkHadoopWriter.scala:83)
at org.apache.spark.scheduler.ResultTask.runTask(ResultTask.scala:90)
at org.apache.spark.scheduler.Task.run(Task.scala:121)
at org.apache.spark.executor.Executor$TaskRunner.$anonfun$run$3(Executor.scala:425)
at org.apache.spark.util.Utils$.tryWithSafeFinally(Utils.scala:1318)
at org.apache.spark.executor.Executor$TaskRunner.run(Executor.scala:428)
at java.util.concurrent.ThreadPoolExecutor.runWorker(ThreadPoolExecutor.java:1149)
at java.util.concurrent.ThreadPoolExecutor$Worker.run(ThreadPoolExecutor.java:624)
at java.lang.Thread.run(Thread.java:748)
```
This PR write "Null" for null row to avoid NPE and fix it.
## How was this patch tested?
NA
Please review http://spark.apache.org/contributing.html before opening a pull request.
Closes#23799 from liupc/Fix-saveAsTextFile-throws-NullPointerException-when-null-row-present.
Lead-authored-by: liupengcheng <liupengcheng@xiaomi.com>
Co-authored-by: Liupengcheng <liupengcheng@xiaomi.com>
Signed-off-by: Sean Owen <sean.owen@databricks.com>
## What changes were proposed in this pull request?
This PR targets to support Arrow optimization for conversion from Spark DataFrame to R DataFrame.
Like PySpark side, it falls back to non-optimization code path when it's unable to use Arrow optimization.
This can be tested as below:
```bash
$ ./bin/sparkR --conf spark.sql.execution.arrow.enabled=true
```
```r
collect(createDataFrame(mtcars))
```
### Requirements
- R 3.5.x
- Arrow package 0.12+
```bash
Rscript -e 'remotes::install_github("apache/arrowapache-arrow-0.12.0", subdir = "r")'
```
**Note:** currently, Arrow R package is not in CRAN. Please take a look at ARROW-3204.
**Note:** currently, Arrow R package seems not supporting Windows. Please take a look at ARROW-3204.
### Benchmarks
**Shall**
```bash
sync && sudo purge
./bin/sparkR --conf spark.sql.execution.arrow.enabled=false --driver-memory 4g
```
```bash
sync && sudo purge
./bin/sparkR --conf spark.sql.execution.arrow.enabled=true --driver-memory 4g
```
**R code**
```r
df <- cache(createDataFrame(read.csv("500000.csv")))
count(df)
test <- function() {
options(digits.secs = 6) # milliseconds
start.time <- Sys.time()
collect(df)
end.time <- Sys.time()
time.taken <- end.time - start.time
print(time.taken)
}
test()
```
**Data (350 MB):**
```r
object.size(read.csv("500000.csv"))
350379504 bytes
```
"500000 Records" http://eforexcel.com/wp/downloads-16-sample-csv-files-data-sets-for-testing/
**Results**
```
Time difference of 221.32014 secs
```
```
Time difference of 15.51145 secs
```
The performance improvement was around **1426%**.
### Limitations:
- For now, Arrow optimization with R does not support when the data is `raw`, and when user explicitly gives float type in the schema. They produce corrupt values. In this case, we decide to fall back to non-optimization code path.
- Due to ARROW-4512, it cannot send and receive batch by batch. It has to send all batches in Arrow stream format at once. It needs improvement later.
## How was this patch tested?
Existing tests related with Arrow optimization cover this change. Also, manually tested.
Closes#23760 from HyukjinKwon/SPARK-26762.
Authored-by: Hyukjin Kwon <gurwls223@apache.org>
Signed-off-by: Hyukjin Kwon <gurwls223@apache.org>
## What changes were proposed in this pull request?
`HadoopDelegationTokenProvider` has basically the same functionality just like `ServiceCredentialProvider` so the interfaces can be merged.
`YARNHadoopDelegationTokenManager` now loads `ServiceCredentialProvider`s in one step. The drawback of this if one provider fails all others are not loaded. `HadoopDelegationTokenManager` loads `HadoopDelegationTokenProvider`s independently so it provides more robust behaviour.
In this PR I've I've made the following changes:
* Deleted `YARNHadoopDelegationTokenManager` and `ServiceCredentialProvider`
* Made `HadoopDelegationTokenProvider` a `DeveloperApi`
## How was this patch tested?
Existing unit tests.
Closes#23686 from gaborgsomogyi/SPARK-26772.
Authored-by: Gabor Somogyi <gabor.g.somogyi@gmail.com>
Signed-off-by: Marcelo Vanzin <vanzin@cloudera.com>
## What changes were proposed in this pull request?
This patch proposes to change the approach on extracting log urls as well as attributes from YARN executor:
- AS-IS: extract information from `Container` API and include them to container launch context
- TO-BE: let YARN executor self-extracting information
This approach leads us to populate more attributes like nodemanager's IPC port which can let us configure custom log url to JHS log url directly.
## How was this patch tested?
Existing unit tests.
Closes#23706 from HeartSaVioR/SPARK-26790.
Authored-by: Jungtaek Lim (HeartSaVioR) <kabhwan@gmail.com>
Signed-off-by: Marcelo Vanzin <vanzin@cloudera.com>
Make it a debug message so that it doesn't show up in the vast
majority of cases, where HBase classes are not available.
Closes#23776 from vanzin/SPARK-26650.
Authored-by: Marcelo Vanzin <vanzin@cloudera.com>
Signed-off-by: Hyukjin Kwon <gurwls223@apache.org>
## What changes were proposed in this pull request?
In the PR, I propose to use `System.nanoTime()` instead of `System.currentTimeMillis()` in measurements of time intervals.
`System.currentTimeMillis()` returns current wallclock time and will follow changes to the system clock. Thus, negative wallclock adjustments can cause timeouts to "hang" for a long time (until wallclock time has caught up to its previous value again). This can happen when ntpd does a "step" after the network has been disconnected for some time. The most canonical example is during system bootup when DHCP takes longer than usual. This can lead to failures that are really hard to understand/reproduce. `System.nanoTime()` is guaranteed to be monotonically increasing irrespective of wallclock changes.
## How was this patch tested?
By existing test suites.
Closes#23727 from MaxGekk/system-nanotime.
Lead-authored-by: Maxim Gekk <max.gekk@gmail.com>
Co-authored-by: Maxim Gekk <maxim.gekk@databricks.com>
Signed-off-by: Sean Owen <sean.owen@databricks.com>
## What changes were proposed in this pull request?
This PR targets to add vectorized `gapply()` in R, Arrow optimization.
This can be tested as below:
```bash
$ ./bin/sparkR --conf spark.sql.execution.arrow.enabled=true
```
```r
df <- createDataFrame(mtcars)
collect(gapply(df,
"gear",
function(key, group) {
data.frame(gear = key[[1]], disp = mean(group$disp) > group$disp)
},
structType("gear double, disp boolean")))
```
### Requirements
- R 3.5.x
- Arrow package 0.12+
```bash
Rscript -e 'remotes::install_github("apache/arrowapache-arrow-0.12.0", subdir = "r")'
```
**Note:** currently, Arrow R package is not in CRAN. Please take a look at ARROW-3204.
**Note:** currently, Arrow R package seems not supporting Windows. Please take a look at ARROW-3204.
### Benchmarks
**Shall**
```bash
sync && sudo purge
./bin/sparkR --conf spark.sql.execution.arrow.enabled=false
```
```bash
sync && sudo purge
./bin/sparkR --conf spark.sql.execution.arrow.enabled=true
```
**R code**
```r
rdf <- read.csv("500000.csv")
rdf <- rdf[, c("Month.of.Joining", "Weight.in.Kgs.")] # We're only interested in the key and values to calculate.
df <- cache(createDataFrame(rdf))
count(df)
test <- function() {
options(digits.secs = 6) # milliseconds
start.time <- Sys.time()
count(gapply(df,
"Month_of_Joining",
function(key, group) {
data.frame(Month_of_Joining = key[[1]], Weight_in_Kgs_ = mean(group$Weight_in_Kgs_) > group$Weight_in_Kgs_)
},
structType("Month_of_Joining integer, Weight_in_Kgs_ boolean")))
end.time <- Sys.time()
time.taken <- end.time - start.time
print(time.taken)
}
test()
```
**Data (350 MB):**
```r
object.size(read.csv("500000.csv"))
350379504 bytes
```
"500000 Records" http://eforexcel.com/wp/downloads-16-sample-csv-files-data-sets-for-testing/
**Results**
```
Time difference of 35.67459 secs
```
```
Time difference of 4.301399 secs
```
The performance improvement was around **829%**.
**Note that** I am 100% sure this PR improves more then 829% because I gave up testing it with non-Arrow optimization because it took super super super long when the data size becomes bigger.
### Limitations
- For now, Arrow optimization with R does not support when the data is `raw`, and when user explicitly gives float type in the schema. They produce corrupt values.
- Due to ARROW-4512, it cannot send and receive batch by batch. It has to send all batches in Arrow stream format at once. It needs improvement later.
## How was this patch tested?
Unit tests were added
**TODOs:**
- [x] Draft codes
- [x] make the tests passed
- [x] make the CRAN check pass
- [x] Performance measurement
- [x] Supportability investigation (for instance types)
Closes#23746 from HyukjinKwon/SPARK-26759.
Authored-by: Hyukjin Kwon <gurwls223@apache.org>
Signed-off-by: Hyukjin Kwon <gurwls223@apache.org>
## What changes were proposed in this pull request?
- The benchmark of `XORShiftRandom.nextInt` vis-a-vis `java.util.Random.nextInt` is moved from the `XORShiftRandom` object to `XORShiftRandomBenchmark`.
- Added benchmarks for `nextLong`, `nextDouble` and `nextGaussian` that are used in Spark as well.
- Added a separate benchmark for `XORShiftRandom.hashSeed`.
Closes#23752 from MaxGekk/xorshiftrandom-benchmark.
Lead-authored-by: Maxim Gekk <maxim.gekk@databricks.com>
Co-authored-by: Maxim Gekk <max.gekk@gmail.com>
Co-authored-by: Dongjoon Hyun <dongjoon@apache.org>
Signed-off-by: Dongjoon Hyun <dhyun@apple.com>
## What changes were proposed in this pull request?
Delegation token providers interface now has a parameter `fileSystems` but this is needed only for `HadoopFSDelegationTokenProvider`.
In this PR I've addressed this issue in the following way:
* Removed `fileSystems` parameter from `HadoopDelegationTokenProvider`
* Moved `YarnSparkHadoopUtil.hadoopFSsToAccess` into `HadoopFSDelegationTokenProvider`
* Moved `spark.yarn.stagingDir` into core
* Moved `spark.yarn.access.namenodes` into core and renamed to `spark.kerberos.access.namenodes`
* Moved `spark.yarn.access.hadoopFileSystems` into core and renamed to `spark.kerberos.access.hadoopFileSystems`
## How was this patch tested?
Existing unit tests.
Closes#23698 from gaborgsomogyi/SPARK-26766.
Authored-by: Gabor Somogyi <gabor.g.somogyi@gmail.com>
Signed-off-by: Marcelo Vanzin <vanzin@cloudera.com>
## What changes were proposed in this pull request?
Recently, when I was reading some code of `BlockManager.getBlockData`, I found that there are useless code that would never reach. The related codes is as below:
```
override def getBlockData(blockId: BlockId): ManagedBuffer = {
if (blockId.isShuffle) {
shuffleManager.shuffleBlockResolver.getBlockData(blockId.asInstanceOf[ShuffleBlockId])
} else {
getLocalBytes(blockId) match {
case Some(blockData) =>
new BlockManagerManagedBuffer(blockInfoManager, blockId, blockData, true)
case None =>
// If this block manager receives a request for a block that it doesn't have then it's
// likely that the master has outdated block statuses for this block. Therefore, we send
// an RPC so that this block is marked as being unavailable from this block manager.
reportBlockStatus(blockId, BlockStatus.empty)
throw new BlockNotFoundException(blockId.toString)
}
}
}
```
```
def getLocalBytes(blockId: BlockId): Option[BlockData] = {
logDebug(s"Getting local block $blockId as bytes")
// As an optimization for map output fetches, if the block is for a shuffle, return it
// without acquiring a lock; the disk store never deletes (recent) items so this should work
if (blockId.isShuffle) {
val shuffleBlockResolver = shuffleManager.shuffleBlockResolver
// TODO: This should gracefully handle case where local block is not available. Currently
// downstream code will throw an exception.
val buf = new ChunkedByteBuffer(
shuffleBlockResolver.getBlockData(blockId.asInstanceOf[ShuffleBlockId]).nioByteBuffer())
Some(new ByteBufferBlockData(buf, true))
} else {
blockInfoManager.lockForReading(blockId).map { info => doGetLocalBytes(blockId, info) }
}
}
```
the `blockId.isShuffle` is checked twice, but however it seems that in the method calling hierarchy of `BlockManager.getLocalBytes`, the another callsite of the `BlockManager.getLocalBytes` is at `TorrentBroadcast.readBlocks` where the blockId can never be a `ShuffleBlockId`.
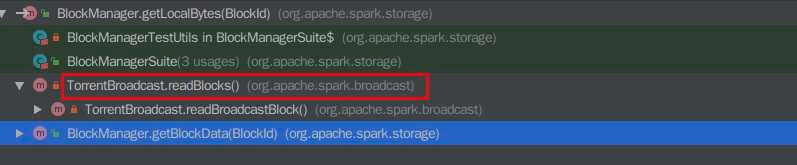
So I think we should remove these useless code for easy reading.
## How was this patch tested?
NA
Please review http://spark.apache.org/contributing.html before opening a pull request.
Closes#23693 from liupc/Remove-useless-code-in-BlockManager.
Authored-by: Liupengcheng <liupengcheng@xiaomi.com>
Signed-off-by: Sean Owen <sean.owen@databricks.com>
## What changes were proposed in this pull request?
**updateAndSyncNumExecutorsTarget** API should be called after **initializing** flag is unset
## How was this patch tested?
Added UT and also manually tested
After Fix
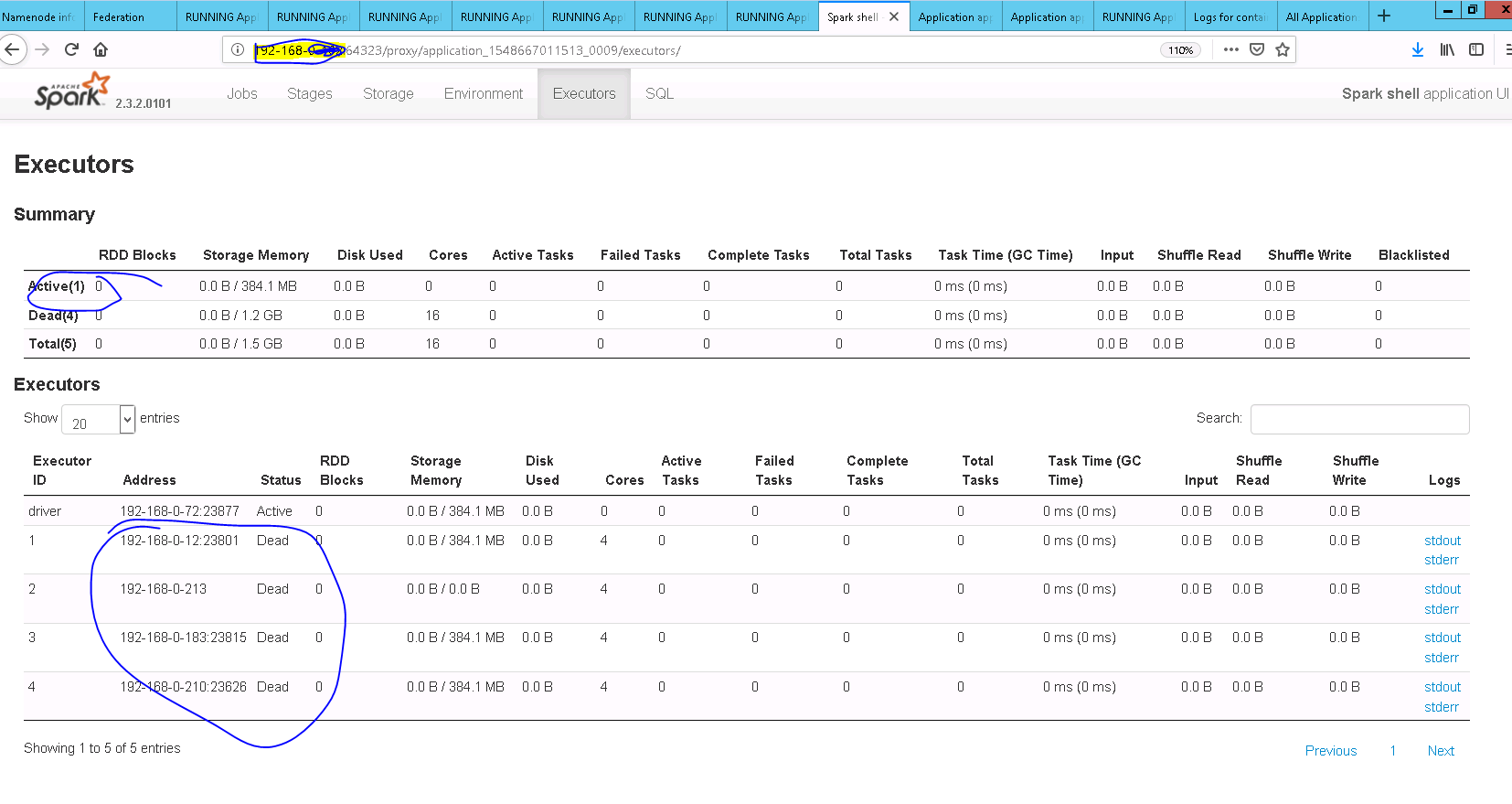
Closes#23697 from sandeep-katta/executorIssue.
Authored-by: sandeep-katta <sandeep.katta2007@gmail.com>
Signed-off-by: Sean Owen <sean.owen@databricks.com>
## What changes were proposed in this pull request?
When the job's partiton is zero, it will still get a jobid but not shown in ui. It's strange. This PR is to show this job in ui.
Example:
In bash:
mkdir -p /home/test/testdir
sc.textFile("/home/test/testdir")
Some logs:
```
19/01/24 17:26:19 INFO FileInputFormat: Total input paths to process : 0
19/01/24 17:26:19 INFO SparkContext: Starting job: collect at WordCount.scala:9
19/01/24 17:26:19 INFO DAGScheduler: Job 0 finished: collect at WordCount.scala:9, took 0.003735 s
```
## How was this patch tested?
UT
Closes#23637 from deshanxiao/spark-26714.
Authored-by: xiaodeshan <xiaodeshan@xiaomi.com>
Signed-off-by: Sean Owen <sean.owen@databricks.com>
## What changes were proposed in this pull request?
Make .unpersist(), .destroy() non-blocking by default and adjust callers to request blocking only where important.
This also adds an optional blocking argument to Pyspark's RDD.unpersist(), which never had one.
## How was this patch tested?
Existing tests.
Closes#23685 from srowen/SPARK-26771.
Authored-by: Sean Owen <sean.owen@databricks.com>
Signed-off-by: Sean Owen <sean.owen@databricks.com>
## What changes were proposed in this pull request?
Currently, spark would not release ShuffleBlockFetcherIterator until the whole task finished.In some conditions, it incurs memory leak.
An example is `rdd.repartition(m).coalesce(n, shuffle = false).save`, each `ShuffleBlockFetcherIterator` contains some metas about mapStatus(`blocksByAddress`) and each resultTask will keep n(max to shuffle partitions) shuffleBlockFetcherIterator and the memory would never released until the task completion, for they are referenced by the completion callbacks of TaskContext. In some case, it may take huge memory and incurs OOM.
Actually, We can release ShuffleBlockFetcherIterator as soon as it's consumed.
This PR is to resolve this problem.
## How was this patch tested?
unittest
Please review http://spark.apache.org/contributing.html before opening a pull request.
Closes#23438 from liupc/Fast-release-shuffleblockfetcheriterator.
Lead-authored-by: Liupengcheng <liupengcheng@xiaomi.com>
Co-authored-by: liupengcheng <liupengcheng@xiaomi.com>
Signed-off-by: Wenchen Fan <wenchen@databricks.com>