### What changes were proposed in this pull request?
This patch is to bump the master branch version to 3.1.0-SNAPSHOT.
### Why are the changes needed?
N/A
### Does this PR introduce any user-facing change?
N/A
### How was this patch tested?
N/A
Closes#27698 from gatorsmile/updateVersion.
Authored-by: gatorsmile <gatorsmile@gmail.com>
Signed-off-by: Dongjoon Hyun <dhyun@apple.com>
Fix for #27395
### What changes were proposed in this pull request?
The `allGather` method is added to the `BarrierTaskContext`. This method contains the same functionality as the `BarrierTaskContext.barrier` method; it blocks the task until all tasks make the call, at which time they may continue execution. In addition, the `allGather` method takes an input message. Upon returning from the `allGather` the task receives a list of all the messages sent by all the tasks that made the `allGather` call.
### Why are the changes needed?
There are many situations where having the tasks communicate in a synchronized way is useful. One simple example is if each task needs to start a server to serve requests from one another; first the tasks must find a free port (the result of which is undetermined beforehand) and then start making requests, but to do so they each must know the port chosen by the other task. An `allGather` method would allow them to inform each other of the port they will run on.
### Does this PR introduce any user-facing change?
Yes, an `BarrierTaskContext.allGather` method will be available through the Scala, Java, and Python APIs.
### How was this patch tested?
Most of the code path is already covered by tests to the `barrier` method, since this PR includes a refactor so that much code is shared by the `barrier` and `allGather` methods. However, a test is added to assert that an all gather on each tasks partition ID will return a list of every partition ID.
An example through the Python API:
```python
>>> from pyspark import BarrierTaskContext
>>>
>>> def f(iterator):
... context = BarrierTaskContext.get()
... return [context.allGather('{}'.format(context.partitionId()))]
...
>>> sc.parallelize(range(4), 4).barrier().mapPartitions(f).collect()[0]
[u'3', u'1', u'0', u'2']
```
Closes#27640 from sarthfrey/master.
Lead-authored-by: sarthfrey-db <sarth.frey@databricks.com>
Co-authored-by: sarthfrey <sarth.frey@gmail.com>
Signed-off-by: Xingbo Jiang <xingbo.jiang@databricks.com>
### What changes were proposed in this pull request?
This PR proposes to throw exception by default when user use untyped UDF(a.k.a `org.apache.spark.sql.functions.udf(AnyRef, DataType)`).
And user could still use it by setting `spark.sql.legacy.useUnTypedUdf.enabled` to `true`.
### Why are the changes needed?
According to #23498, since Spark 3.0, the untyped UDF will return the default value of the Java type if the input value is null. For example, `val f = udf((x: Int) => x, IntegerType)`, `f($"x")` will return 0 in Spark 3.0 but null in Spark 2.4. And the behavior change is introduced due to Spark3.0 is built with Scala 2.12 by default.
As a result, this might change data silently and may cause correctness issue if user still expect `null` in some cases. Thus, we'd better to encourage user to use typed UDF to avoid this problem.
### Does this PR introduce any user-facing change?
Yeah. User will hit exception now when use untyped UDF.
### How was this patch tested?
Added test and updated some tests.
Closes#27488 from Ngone51/spark_26580_followup.
Lead-authored-by: yi.wu <yi.wu@databricks.com>
Co-authored-by: wuyi <yi.wu@databricks.com>
Signed-off-by: Wenchen Fan <wenchen@databricks.com>
### What changes were proposed in this pull request?
This PR adds two pages to Web UI for Structured Streaming:
- "/streamingquery": Streaming Query Page, providing some aggregate information for running/completed streaming queries.
- "/streamingquery/statistics": Streaming Query Statistics Page, providing detailed information for streaming query, including `Input Rate`, `Process Rate`, `Input Rows`, `Batch Duration` and `Operation Duration`
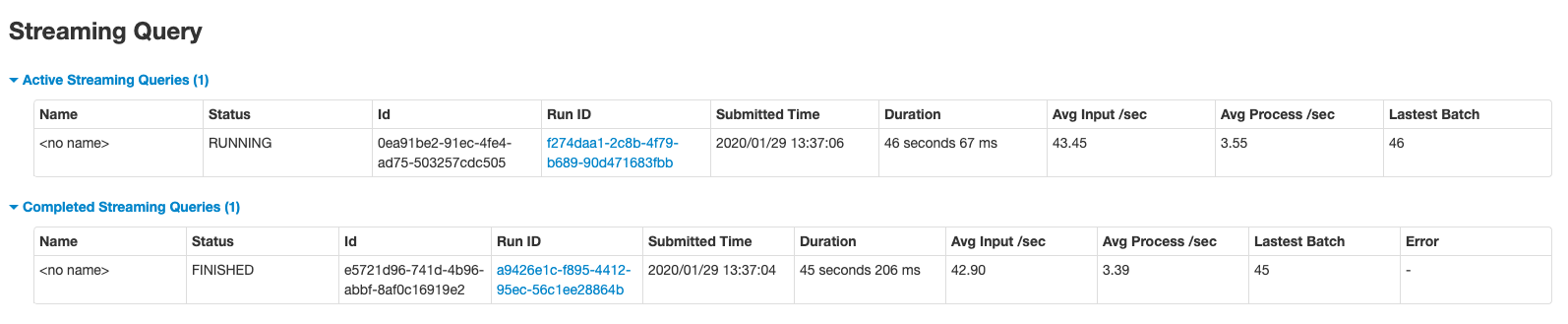
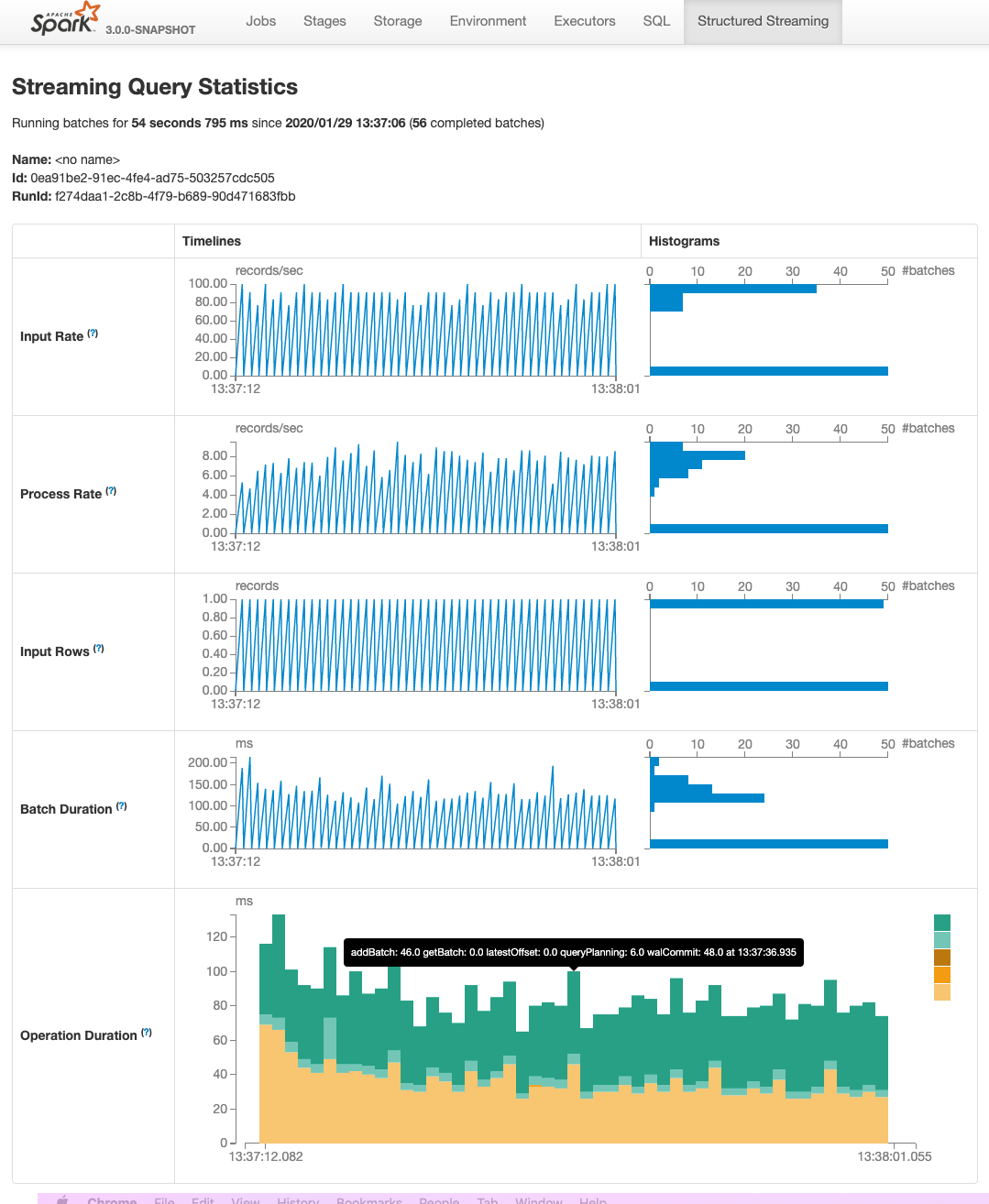
### Why are the changes needed?
It helps users to better monitor Structured Streaming query.
### Does this PR introduce any user-facing change?
No
### How was this patch tested?
- new added and existing UTs
- manual test
Closes#26201 from uncleGen/SPARK-29543.
Lead-authored-by: uncleGen <hustyugm@gmail.com>
Co-authored-by: Yuanjian Li <xyliyuanjian@gmail.com>
Co-authored-by: Genmao Yu <hustyugm@gmail.com>
Signed-off-by: Shixiong Zhu <zsxwing@gmail.com>
### What changes were proposed in this pull request?
Remove ```numTrees``` in GBT in 3.0.0.
### Why are the changes needed?
Currently, GBT has
```
/**
* Number of trees in ensemble
*/
Since("2.0.0")
val getNumTrees: Int = trees.length
```
and
```
/** Number of trees in ensemble */
val numTrees: Int = trees.length
```
I think we should remove one of them. We deprecated it in 2.4.5 via https://github.com/apache/spark/pull/27352.
### Does this PR introduce any user-facing change?
Yes, remove ```numTrees``` in GBT in 3.0.0
### How was this patch tested?
existing tests
Closes#27330 from huaxingao/spark-numTrees.
Authored-by: Huaxin Gao <huaxing@us.ibm.com>
Signed-off-by: Dongjoon Hyun <dhyun@apple.com>
### What changes were proposed in this pull request?
This is the second PR for the Stage Level Scheduling. This is adding in the necessary executor side changes:
1) executors to know what ResourceProfile they should be using
2) handle parsing the resource profile settings - these are not in the global configs
3) then reporting back to the driver what resource profile it was started with.
This PR adds all the piping for YARN to pass the information all the way to executors, but it just uses the default ResourceProfile (which is the global applicatino level configs).
At a high level these changes include:
1) adding a new --resourceProfileId option to the CoarseGrainedExecutorBackend
2) Add the ResourceProfile settings to new internal confs that gets passed into the Executor
3) Executor changes that use the resource profile id passed in to read the corresponding ResourceProfile confs and then parse those requests and discover resources as necessary
4) Executor registers to Driver with the Resource profile id so that the ExecutorMonitor can track how many executor with each profile are running
5) YARN side changes to show that passing the resource profile id and confs actually works. Just uses the DefaultResourceProfile for now.
I also removed a check from the CoarseGrainedExecutorBackend that used to check to make sure there were task requirements before parsing any custom resource executor requests. With the resource profiles this becomes much more expensive because we would then have to pass the task requests to each executor and the check was just a short cut and not really needed. It was much cleaner just to remove it.
Note there were some changes to the ResourceProfile, ExecutorResourceRequests, and TaskResourceRequests in this PR as well because I discovered some issues with things not being immutable. That api now look like:
val rpBuilder = new ResourceProfileBuilder()
val ereq = new ExecutorResourceRequests()
val treq = new TaskResourceRequests()
ereq.cores(2).memory("6g").memoryOverhead("2g").pysparkMemory("2g").resource("gpu", 2, "/home/tgraves/getGpus")
treq.cpus(2).resource("gpu", 2)
val resourceProfile = rpBuilder.require(ereq).require(treq).build
This makes is so that ResourceProfile is immutable and Spark can use it directly without worrying about the user changing it.
### Why are the changes needed?
These changes are needed for the executor to report which ResourceProfile they are using so that ultimately the dynamic allocation manager can use that information to know how many with a profile are running and how many more it needs to request. Its also needed to get the resource profile confs to the executor so that it can run the appropriate discovery script if needed.
### Does this PR introduce any user-facing change?
No
### How was this patch tested?
Unit tests and manually on YARN.
Closes#26682 from tgravescs/SPARK-29306.
Authored-by: Thomas Graves <tgraves@nvidia.com>
Signed-off-by: Thomas Graves <tgraves@apache.org>
### What changes were proposed in this pull request?
Make Regressors extend abstract class Regressor:
```AFTSurvivalRegression extends Estimator => extends Regressor```
```DecisionTreeRegressor extends Predictor => extends Regressor```
```FMRegressor extends Predictor => extends Regressor```
```GBTRegressor extends Predictor => extends Regressor```
```RandomForestRegressor extends Predictor => extends Regressor```
We will not make ```IsotonicRegression``` extend ```Regressor``` because it is tricky to handle both DoubleType and VectorType.
### Why are the changes needed?
Make class hierarchy consistent for all Regressors
### Does this PR introduce any user-facing change?
No
### How was this patch tested?
existing tests
Closes#27168 from huaxingao/spark-30377.
Authored-by: Huaxin Gao <huaxing@us.ibm.com>
Signed-off-by: Sean Owen <srowen@gmail.com>
### What changes were proposed in this pull request?
Make ```MultilayerPerceptronClassificationModel``` extend ```MultilayerPerceptronParams```
### Why are the changes needed?
Make ```MultilayerPerceptronClassificationModel``` extend ```MultilayerPerceptronParams``` to expose the training params, so user can see these params when calling ```extractParamMap```
### Does this PR introduce any user-facing change?
Yes. The ```MultilayerPerceptronParams``` such as ```seed```, ```maxIter``` ... are available in ```MultilayerPerceptronClassificationModel``` now
### How was this patch tested?
Manually tested ```MultilayerPerceptronClassificationModel.extractParamMap()``` to verify all the new params are there.
Closes#26838 from huaxingao/spark-30144.
Authored-by: Huaxin Gao <huaxing@us.ibm.com>
Signed-off-by: Sean Owen <srowen@gmail.com>
### What changes were proposed in this pull request?
1, add new foreach-like methods: foreach/foreachNonZero
2, add iterator: iterator/activeIterator/nonZeroIterator
### Why are the changes needed?
see the [ticke](https://issues.apache.org/jira/browse/SPARK-30329) for details
foreach/foreachNonZero: for both convenience and performace (SparseVector.foreach should be faster than current traversal method)
iterator/activeIterator/nonZeroIterator: add the three iterators, so that we can futuremore add/change some impls based on those iterators for both ml and mllib sides, to avoid vector conversions.
### Does this PR introduce any user-facing change?
Yes, new methods are added
### How was this patch tested?
added testsuites
Closes#26982 from zhengruifeng/vector_iter.
Authored-by: zhengruifeng <ruifengz@foxmail.com>
Signed-off-by: zhengruifeng <ruifengz@foxmail.com>
### What changes were proposed in this pull request?
Observable metrics are named arbitrary aggregate functions that can be defined on a query (Dataframe). As soon as the execution of a Dataframe reaches a completion point (e.g. finishes batch query or reaches streaming epoch) a named event is emitted that contains the metrics for the data processed since the last completion point.
A user can observe these metrics by attaching a listener to spark session, it depends on the execution mode which listener to attach:
- Batch: `QueryExecutionListener`. This will be called when the query completes. A user can access the metrics by using the `QueryExecution.observedMetrics` map.
- (Micro-batch) Streaming: `StreamingQueryListener`. This will be called when the streaming query completes an epoch. A user can access the metrics by using the `StreamingQueryProgress.observedMetrics` map. Please note that we currently do not support continuous execution streaming.
### Why are the changes needed?
This enabled observable metrics.
### Does this PR introduce any user-facing change?
Yes. It adds the `observe` method to `Dataset`.
### How was this patch tested?
- Added unit tests for the `CollectMetrics` logical node to the `AnalysisSuite`.
- Added unit tests for `StreamingProgress` JSON serialization to the `StreamingQueryStatusAndProgressSuite`.
- Added integration tests for streaming to the `StreamingQueryListenerSuite`.
- Added integration tests for batch to the `DataFrameCallbackSuite`.
Closes#26127 from hvanhovell/SPARK-29348.
Authored-by: herman <herman@databricks.com>
Signed-off-by: herman <herman@databricks.com>
### What changes were proposed in this pull request?
support `modelType` `gaussian`
### Why are the changes needed?
current modelTypes do not support continuous data
### Does this PR introduce any user-facing change?
yes, add a `modelType` option
### How was this patch tested?
existing testsuites and added ones
Closes#26413 from zhengruifeng/gnb.
Authored-by: zhengruifeng <ruifengz@foxmail.com>
Signed-off-by: zhengruifeng <ruifengz@foxmail.com>
SPARK-29397 added new interfaces for creating driver and executor
plugins. These were added in a new, more isolated package that does
not pollute the main o.a.s package.
The old interface is now redundant. Since it's a DeveloperApi and
we're about to have a new major release, let's remove it instead of
carrying more baggage forward.
Closes#26390 from vanzin/SPARK-29399.
Authored-by: Marcelo Vanzin <vanzin@cloudera.com>
Signed-off-by: HyukjinKwon <gurwls223@apache.org>
### What changes were proposed in this pull request?
1, add shared param `relativeError`
2, `Imputer`/`RobusterScaler`/`QuantileDiscretizer` extend `HasRelativeError`
### Why are the changes needed?
It makes sense to expose RelativeError to end users, since it controls both the precision and memory overhead.
`QuantileDiscretizer` had already added this param, while other algs not yet.
### Does this PR introduce any user-facing change?
yes, new param is added in `Imputer`/`RobusterScaler`
### How was this patch tested?
existing testsutes
Closes#26305 from zhengruifeng/add_relative_err.
Authored-by: zhengruifeng <ruifengz@foxmail.com>
Signed-off-by: zhengruifeng <ruifengz@foxmail.com>
### What changes were proposed in this pull request?
We added a TaskContext.resources() api, but I realized this is returning a scala Map which is not ideal for access from Java. Here I add a resourcesJMap function which returns a java.util.Map to make it easily accessible from Java.
### Why are the changes needed?
Java API access
### Does this PR introduce any user-facing change?
<!--
If yes, please clarify the previous behavior and the change this PR proposes - provide the console output, description and/or an example to show the behavior difference if possible.
If no, write 'No'.
-->
Yes, new TaskContext function to access from Java
### How was this patch tested?
<!--
If tests were added, say they were added here. Please make sure to add some test cases that check the changes thoroughly including negative and positive cases if possible.
If it was tested in a way different from regular unit tests, please clarify how you tested step by step, ideally copy and paste-able, so that other reviewers can test and check, and descendants can verify in the future.
If tests were not added, please describe why they were not added and/or why it was difficult to add.
-->
new unit test
Closes#26083 from tgravescs/SPARK-29417.
Lead-authored-by: Thomas Graves <tgraves@ngvpn01-168-221.dyn.scz.us.nvidia.com>
Co-authored-by: Thomas Graves <tgraves@TGRAVES-MLT.local>
Co-authored-by: Thomas Graves <tgraves@nvidia.com>
Signed-off-by: Xiangrui Meng <meng@databricks.com>
### What changes were proposed in this pull request?
Copy any "spark.hive.foo=bar" spark properties into hadoop conf as "hive.foo=bar"
### Why are the changes needed?
Providing spark side config entry for hive configurations.
### Does this PR introduce any user-facing change?
No.
### How was this patch tested?
UT.
Closes#25661 from WeichenXu123/add_hive_conf.
Authored-by: WeichenXu <weichen.xu@databricks.com>
Signed-off-by: Wenchen Fan <wenchen@databricks.com>
After the newly added shuffle block fetching protocol in #24565, we can keep this work by extending the FetchShuffleBlocks message.
### What changes were proposed in this pull request?
In this patch, we achieve the indeterminate shuffle rerun by reusing the task attempt id(unique id within an application) in shuffle id, so that each shuffle write attempt has a different file name. For the indeterministic stage, when the stage resubmits, we'll clear all existing map status and rerun all partitions.
All changes are summarized as follows:
- Change the mapId to mapTaskAttemptId in shuffle related id.
- Record the mapTaskAttemptId in MapStatus.
- Still keep mapId in ShuffleFetcherIterator for fetch failed scenario.
- Add the determinate flag in Stage and use it in DAGScheduler and the cleaning work for the intermediate stage.
### Why are the changes needed?
This is a follow-up work for #22112's future improvment[1]: `Currently we can't rollback and rerun a shuffle map stage, and just fail.`
Spark will rerun a finished shuffle write stage while meeting fetch failures, currently, the rerun shuffle map stage will only resubmit the task for missing partitions and reuse the output of other partitions. This logic is fine in most scenarios, but for indeterministic operations(like repartition), multiple shuffle write attempts may write different data, only rerun the missing partition will lead a correctness bug. So for the shuffle map stage of indeterministic operations, we need to support rolling back the shuffle map stage and re-generate the shuffle files.
### Does this PR introduce any user-facing change?
Yes, after this PR, the indeterminate stage rerun will be accepted by rerunning the whole stage. The original behavior is aborting the stage and fail the job.
### How was this patch tested?
- UT: Add UT for all changing code and newly added function.
- Manual Test: Also providing a manual test to verify the effect.
```
import scala.sys.process._
import org.apache.spark.TaskContext
val determinateStage0 = sc.parallelize(0 until 1000 * 1000 * 100, 10)
val indeterminateStage1 = determinateStage0.repartition(200)
val indeterminateStage2 = indeterminateStage1.repartition(200)
val indeterminateStage3 = indeterminateStage2.repartition(100)
val indeterminateStage4 = indeterminateStage3.repartition(300)
val fetchFailIndeterminateStage4 = indeterminateStage4.map { x =>
if (TaskContext.get.attemptNumber == 0 && TaskContext.get.partitionId == 190 &&
TaskContext.get.stageAttemptNumber == 0) {
throw new Exception("pkill -f -n java".!!)
}
x
}
val indeterminateStage5 = fetchFailIndeterminateStage4.repartition(200)
val finalStage6 = indeterminateStage5.repartition(100).collect().distinct.length
```
It's a simple job with multi indeterminate stage, it will get a wrong answer while using old Spark version like 2.2/2.3, and will be killed after #22112. With this fix, the job can retry all indeterminate stage as below screenshot and get the right result.
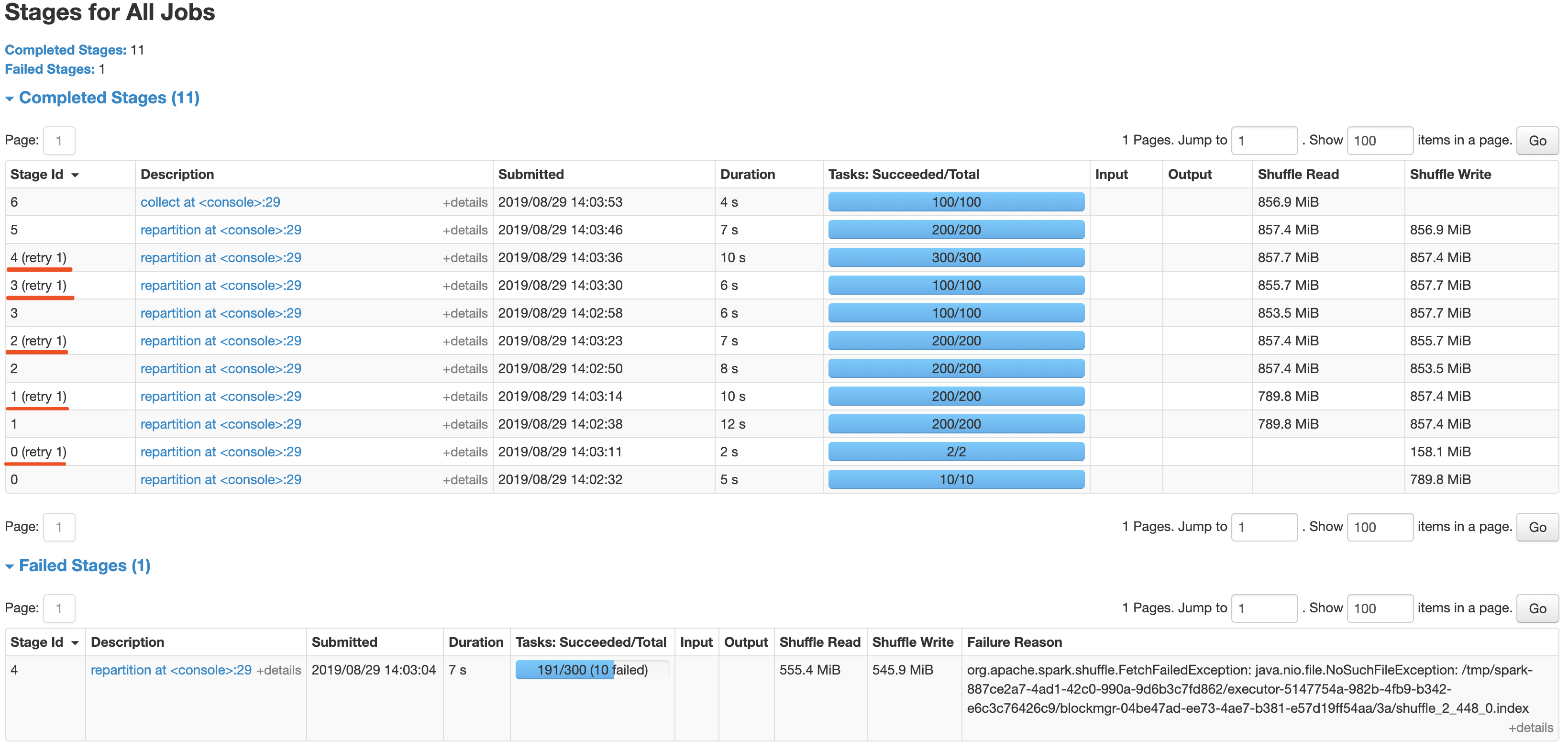
Closes#25620 from xuanyuanking/SPARK-25341-8.27.
Authored-by: Yuanjian Li <xyliyuanjian@gmail.com>
Signed-off-by: Wenchen Fan <wenchen@databricks.com>
## What changes were proposed in this pull request?
This proposes to improve Spark instrumentation by adding a hook for user-defined metrics, extending Spark’s Dropwizard/Codahale metrics system.
The original motivation of this work was to add instrumentation for S3 filesystem access metrics by Spark job. Currently, [[ExecutorSource]] instruments HDFS and local filesystem metrics. Rather than extending the code there, we proposes with this JIRA to add a metrics plugin system which is of more flexible and general use.
Context: The Spark metrics system provides a large variety of metrics, see also , useful to monitor and troubleshoot Spark workloads. A typical workflow is to sink the metrics to a storage system and build dashboards on top of that.
Highlights:
- The metric plugin system makes it easy to implement instrumentation for S3 access by Spark jobs.
- The metrics plugin system allows for easy extensions of how Spark collects HDFS-related workload metrics. This is currently done using the Hadoop Filesystem GetAllStatistics method, which is deprecated in recent versions of Hadoop. Recent versions of Hadoop Filesystem recommend using method GetGlobalStorageStatistics, which also provides several additional metrics. GetGlobalStorageStatistics is not available in Hadoop 2.7 (had been introduced in Hadoop 2.8). Using a metric plugin for Spark would allow an easy way to “opt in” using such new API calls for those deploying suitable Hadoop versions.
- We also have the use case of adding Hadoop filesystem monitoring for a custom Hadoop compliant filesystem in use in our organization (EOS using the XRootD protocol). The metrics plugin infrastructure makes this easy to do. Others may have similar use cases.
- More generally, this method makes it straightforward to plug in Filesystem and other metrics to the Spark monitoring system. Future work on plugin implementation can address extending monitoring to measure usage of external resources (OS, filesystem, network, accelerator cards, etc), that maybe would not normally be considered general enough for inclusion in Apache Spark code, but that can be nevertheless useful for specialized use cases, tests or troubleshooting.
Implementation:
The proposed implementation extends and modifies the work on Executor Plugin of SPARK-24918. Additionally, this is related to recent work on extending Spark executor metrics, such as SPARK-25228.
As discussed during the review, the implementaiton of this feature modifies the Developer API for Executor Plugins, such that the new version is incompatible with the original version in Spark 2.4.
## How was this patch tested?
This modifies existing tests for ExecutorPluginSuite to adapt them to the API changes. In addition, the new funtionality for registering pluginMetrics has been manually tested running Spark on YARN and K8S clusters, in particular for monitoring S3 and for extending HDFS instrumentation with the Hadoop Filesystem “GetGlobalStorageStatistics” metrics. Executor metric plugin example and code used for testing are available, for example at: https://github.com/cerndb/SparkExecutorPluginsCloses#24901 from LucaCanali/executorMetricsPlugin.
Authored-by: Luca Canali <luca.canali@cern.ch>
Signed-off-by: Marcelo Vanzin <vanzin@cloudera.com>
### What changes were proposed in this pull request?
- Remove SQLContext.createExternalTable and Catalog.createExternalTable, deprecated in favor of createTable since 2.2.0, plus tests of deprecated methods
- Remove HiveContext, deprecated in 2.0.0, in favor of `SparkSession.builder.enableHiveSupport`
- Remove deprecated KinesisUtils.createStream methods, plus tests of deprecated methods, deprecate in 2.2.0
- Remove deprecated MLlib (not Spark ML) linear method support, mostly utility constructors and 'train' methods, and associated docs. This includes methods in LinearRegression, LogisticRegression, Lasso, RidgeRegression. These have been deprecated since 2.0.0
- Remove deprecated Pyspark MLlib linear method support, including LogisticRegressionWithSGD, LinearRegressionWithSGD, LassoWithSGD
- Remove 'runs' argument in KMeans.train() method, which has been a no-op since 2.0.0
- Remove deprecated ChiSqSelector isSorted protected method
- Remove deprecated 'yarn-cluster' and 'yarn-client' master argument in favor of 'yarn' and deploy mode 'cluster', etc
Notes:
- I was not able to remove deprecated DataFrameReader.json(RDD) in favor of DataFrameReader.json(Dataset); the former was deprecated in 2.2.0, but, it is still needed to support Pyspark's .json() method, which can't use a Dataset.
- Looks like SQLContext.createExternalTable was not actually deprecated in Pyspark, but, almost certainly was meant to be? Catalog.createExternalTable was.
- I afterwards noted that the toDegrees, toRadians functions were almost removed fully in SPARK-25908, but Felix suggested keeping just the R version as they hadn't been technically deprecated. I'd like to revisit that. Do we really want the inconsistency? I'm not against reverting it again, but then that implies leaving SQLContext.createExternalTable just in Pyspark too, which seems weird.
- I *kept* LogisticRegressionWithSGD, LinearRegressionWithSGD, LassoWithSGD, RidgeRegressionWithSGD in Pyspark, though deprecated, as it is hard to remove them (still used by StreamingLogisticRegressionWithSGD?) and they are not fully removed in Scala. Maybe should not have been deprecated.
### Why are the changes needed?
Deprecated items are easiest to remove in a major release, so we should do so as much as possible for Spark 3. This does not target items deprecated 'recently' as of Spark 2.3, which is still 18 months old.
### Does this PR introduce any user-facing change?
Yes, in that deprecated items are removed from some public APIs.
### How was this patch tested?
Existing tests.
Closes#25684 from srowen/SPARK-28980.
Lead-authored-by: Sean Owen <sean.owen@databricks.com>
Co-authored-by: HyukjinKwon <gurwls223@apache.org>
Signed-off-by: Sean Owen <sean.owen@databricks.com>
### What changes were proposed in this pull request?
Add HasNumFeatures in the scala side, with `1<<18` as the default value
### Why are the changes needed?
HasNumFeatures is already added in the py side, it is reasonable to keep them in sync.
I don't find other similar place.
### Does this PR introduce any user-facing change?
No
### How was this patch tested?
Existing testsuites
Closes#25671 from zhengruifeng/add_HasNumFeatures.
Authored-by: zhengruifeng <ruifengz@foxmail.com>
Signed-off-by: zhengruifeng <ruifengz@foxmail.com>
### What changes were proposed in this pull request?
Delete the incorrect method `def setWeightCol(value: Double): this.type = set(threshold, value)` in `LinearSVCModel`
### Why are the changes needed?
`LinearSVCModel` should not provide this setter, moreover, this method is wrongly defined.
### Does this PR introduce any user-facing change?
yes, a public method is removed
### How was this patch tested?
existing suites
Closes#25510 from zhengruifeng/linearsvc_model_set_weightcol.
Authored-by: zhengruifeng <ruifengz@foxmail.com>
Signed-off-by: Sean Owen <sean.owen@databricks.com>
## What changes were proposed in this pull request?
Currently, PythonBroadcast may delete its data file while a python worker still needs it. This happens because PythonBroadcast overrides the `finalize()` method to delete its data file. So, when GC happens and no references on broadcast variable, it may trigger `finalize()` to delete
data file. That's also means, data under python Broadcast variable couldn't be deleted when `unpersist()`/`destroy()` called but relys on GC.
In this PR, we removed the `finalize()` method, and map the PythonBroadcast data file to a BroadcastBlock(which has the same broadcast id with the broadcast variable who wrapped this PythonBroadcast) when PythonBroadcast is deserializing. As a result, the data file could be deleted just like other pieces of the Broadcast variable when `unpersist()`/`destroy()` called and do not rely on GC any more.
## How was this patch tested?
Added a Python test, and tested manually(verified create/delete the broadcast block).
Closes#25262 from Ngone51/SPARK-28486.
Authored-by: wuyi <ngone_5451@163.com>
Signed-off-by: HyukjinKwon <gurwls223@apache.org>
## What changes were proposed in this pull request?
Prior to this change, in an executor, on each heartbeat, memory metrics are polled and sent in the heartbeat. The heartbeat interval is 10s by default. With this change, in an executor, memory metrics can optionally be polled in a separate poller at a shorter interval.
For each executor, we use a map of (stageId, stageAttemptId) to (count of running tasks, executor metric peaks) to track what stages are active as well as the per-stage memory metric peaks. When polling the executor memory metrics, we attribute the memory to the active stage(s), and update the peaks. In a heartbeat, we send the per-stage peaks (for stages active at that time), and then reset the peaks. The semantics would be that the per-stage peaks sent in each heartbeat are the peaks since the last heartbeat.
We also keep a map of taskId to memory metric peaks. This tracks the metric peaks during the lifetime of the task. The polling thread updates this as well. At end of a task, we send the peak metric values in the task result. In case of task failure, we send the peak metric values in the `TaskFailedReason`.
We continue to do the stage-level aggregation in the EventLoggingListener.
For the driver, we still only poll on heartbeats. What the driver sends will be the current values of the metrics in the driver at the time of the heartbeat. This is semantically the same as before.
## How was this patch tested?
Unit tests. Manually tested applications on an actual system and checked the event logs; the metrics appear in the SparkListenerTaskEnd and SparkListenerStageExecutorMetrics events.
Closes#23767 from wypoon/wypoon_SPARK-26329.
Authored-by: Wing Yew Poon <wypoon@cloudera.com>
Signed-off-by: Imran Rashid <irashid@cloudera.com>
## What changes were proposed in this pull request?
I remove the deprecate `ImageSchema.readImages`.
Move some useful methods from class `ImageSchema` into class `ImageFileFormat`.
In pyspark, I rename `ImageSchema` class to be `ImageUtils`, and keep some useful python methods in it.
## How was this patch tested?
UT.
Please review https://spark.apache.org/contributing.html before opening a pull request.
Closes#25245 from WeichenXu123/remove_image_schema.
Authored-by: WeichenXu <weichen.xu@databricks.com>
Signed-off-by: HyukjinKwon <gurwls223@apache.org>
## What changes were proposed in this pull request?
Right now `Error` is not sent to `QueryExecutionListener.onFailure`. If there is any `Error` (such as `AssertionError`) when running a query, `QueryExecutionListener.onFailure` cannot be triggered.
This PR changes `onFailure` to accept a `Throwable` instead.
## How was this patch tested?
Jenkins
Closes#25292 from zsxwing/fix-QueryExecutionListener.
Authored-by: Shixiong Zhu <zsxwing@gmail.com>
Signed-off-by: HyukjinKwon <gurwls223@apache.org>
## What changes were proposed in this pull request?
This patch proposes moving all Trigger implementations to `Triggers.scala`, to avoid exposing these implementations to the end users and let end users only deal with `Trigger.xxx` static methods. This fits the intention of deprecation of `ProcessingTIme`, and we agree to move others without deprecation as this patch will be shipped in major version (Spark 3.0.0).
## How was this patch tested?
UTs modified to work with newly introduced class.
Closes#24996 from HeartSaVioR/SPARK-28199.
Authored-by: Jungtaek Lim (HeartSaVioR) <kabhwan@gmail.com>
Signed-off-by: Sean Owen <sean.owen@databricks.com>
## What changes were proposed in this pull request?
Track tasks separately for each stage attempt (instead of tracking by stage), and do NOT reset the numRunningTasks to 0 on StageCompleted.
In the case of stage retry, the `taskEnd` event from the zombie stage sometimes makes the number of `totalRunningTasks` negative, which will causes the job to get stuck.
Similar problem also exists with `stageIdToTaskIndices` & `stageIdToSpeculativeTaskIndices`.
If it is a failed `taskEnd` event of the zombie stage, this will cause `stageIdToTaskIndices` or `stageIdToSpeculativeTaskIndices` to remove the task index of the active stage, and the number of `totalPendingTasks` will increase unexpectedly.
## How was this patch tested?
unit test properly handle task end events from completed stages
Closes#24497 from cxzl25/fix_stuck_job_follow_up.
Authored-by: sychen <sychen@ctrip.com>
Signed-off-by: Imran Rashid <irashid@cloudera.com>
## What changes were proposed in this pull request?
Currently we are in a strange status that, some data source v2 interfaces(catalog related) are in sql/catalyst, some data source v2 interfaces(Table, ScanBuilder, DataReader, etc.) are in sql/core.
I don't see a reason to keep data source v2 API in 2 modules. If we should pick one module, I think sql/catalyst is the one to go.
Catalyst module already has some user-facing stuff like DataType, Row, etc. And we have to update `Analyzer` and `SessionCatalog` to support the new catalog plugin, which needs to be in the catalyst module.
This PR can solve the problem we have in https://github.com/apache/spark/pull/24246
## How was this patch tested?
existing tests
Closes#24416 from cloud-fan/move.
Authored-by: Wenchen Fan <wenchen@databricks.com>
Signed-off-by: gatorsmile <gatorsmile@gmail.com>
## What changes were proposed in this pull request?
This PR adds support to schedule tasks with extra resource requirements (eg. GPUs) on executors with available resources. It also introduce a new method `TaskContext.resources()` so tasks can access available resource addresses allocated to them.
## How was this patch tested?
* Added new end-to-end test cases in `SparkContextSuite`;
* Added new test case in `CoarseGrainedSchedulerBackendSuite`;
* Added new test case in `CoarseGrainedExecutorBackendSuite`;
* Added new test case in `TaskSchedulerImplSuite`;
* Added new test case in `TaskSetManagerSuite`;
* Updated existing tests.
Closes#24374 from jiangxb1987/gpu.
Authored-by: Xingbo Jiang <xingbo.jiang@databricks.com>
Signed-off-by: Xiangrui Meng <meng@databricks.com>
## What changes were proposed in this pull request?
For the below three thread configuration items applied to both driver and executor,
spark.rpc.io.serverThreads
spark.rpc.io.clientThreads
spark.rpc.netty.dispatcher.numThreads,
we separate them to driver specifics and executor specifics.
spark.driver.rpc.io.serverThreads < - > spark.executor.rpc.io.serverThreads
spark.driver.rpc.io.clientThreads < - > spark.executor.rpc.io.clientThreads
spark.driver.rpc.netty.dispatcher.numThreads < - > spark.executor.rpc.netty.dispatcher.numThreads
Spark reads these specifics first and fall back to the common configurations.
## How was this patch tested?
We ran the SimpleMap app without shuffle for benchmark purpose to test Spark's scalability in HPC with omini-path NIC which has higher bandwidth than normal ethernet NIC.
Spark's base version is 2.4.0.
Spark ran in the Standalone mode. Driver was in a standalone node.
After the separation, the performance is improved a lot in 256 nodes and 512 nodes. see below test results of SimpleMapTask before and after the enhancement. You can view the tables in the [JIRA](https://issues.apache.org/jira/browse/SPARK-26632) too.
ds: spark.driver.rpc.io.serverThreads
dc: spark.driver.rpc.io.clientThreads
dd: spark.driver.rpc.netty.dispatcher.numThreads
ed: spark.executor.rpc.netty.dispatcher.numThreads
time: Overall Time (s)
old time: Overall Time without Separation (s)
**Before:**
nodes | ds | dc | dd | ed | time
-- |-- | -- | -- | -- | --
128 nodes | 8 | 8 | 8 | 8 | 108
256 nodes | 8 | 8 | 8 | 8 | 196
512 nodes | 8 | 8 | 8 | 8 | 377
**After:**
nodes | ds | dc | dd | ed | time | improvement
-- | -- | -- | -- | -- | -- | --
128 nodes | 15 | 15 | 10 | 30 | 107 | 0.9%
256 nodes | 12 | 15 | 10 | 30 | 159 | 18.8%
512 nodes | 12 | 15 | 10 | 30 | 283 | 24.9%
Closes#23560 from zjf2012/thread_conf_separation.
Authored-by: jiafu.zhang@intel.com <jiafu.zhang@intel.com>
Signed-off-by: Marcelo Vanzin <vanzin@cloudera.com>
## What changes were proposed in this pull request?
Fixed the `spark-<version>-yarn-shuffle.jar` artifact packaging to shade the native netty libraries:
- shade the `META-INF/native/libnetty_*` native libraries when packagin
the yarn shuffle service jar. This is required as netty library loader
derives that based on shaded package name.
- updated the `org/spark_project` shade package prefix to `org/sparkproject`
(i.e. removed underscore) as the former breaks the netty native lib loading.
This was causing the yarn external shuffle service to fail
when spark.shuffle.io.mode=EPOLL
## How was this patch tested?
Manual tests
Closes#24502 from amuraru/SPARK-27610_master.
Authored-by: Adi Muraru <amuraru@adobe.com>
Signed-off-by: Marcelo Vanzin <vanzin@cloudera.com>
## What changes were proposed in this pull request?
Remove deprecated / no-op mllib.KMeans getRuns, setRuns
mllib.KMeans has getRuns, setRuns methods which haven't done anything since Spark 2.1. They're deprecated, and no-ops, and should be removed for Spark 3.
## How was this patch tested?
Existing tests.
Closes#24320 from srowen/SPARK-27410.
Authored-by: Sean Owen <sean.owen@databricks.com>
Signed-off-by: Sean Owen <sean.owen@databricks.com>
## What changes were proposed in this pull request?
Remove Scala 2.11 support in build files and docs, and in various parts of code that accommodated 2.11. See some targeted comments below.
## How was this patch tested?
Existing tests.
Closes#23098 from srowen/SPARK-26132.
Authored-by: Sean Owen <sean.owen@databricks.com>
Signed-off-by: Sean Owen <sean.owen@databricks.com>
## What changes were proposed in this pull request?
LEGACY_DRIVER_IDENTIFIER and its reference are removed.
corresponding references test are updated.
## How was this patch tested?
tested UT test cases
Closes#24026 from shivusondur/newjira2.
Authored-by: shivusondur <shivusondur@gmail.com>
Signed-off-by: Sean Owen <sean.owen@databricks.com>
## What changes were proposed in this pull request?
`Saveable` interface introduces `formatVersion` which is protected and it is used nowhere. So the PR proposes to remove it.
## How was this patch tested?
existing tests
Closes#22830 from mgaido91/SPARK-25838.
Authored-by: Marco Gaido <marcogaido91@gmail.com>
Signed-off-by: Sean Owen <sean.owen@databricks.com>
## What changes were proposed in this pull request?
This patch proposes adding a new configuration on SHS: custom executor log URL pattern. This will enable end users to replace executor logs to other than RM provide, like external log service, which enables to serve executor logs when NodeManager becomes unavailable in case of YARN.
End users can build their own of custom executor log URLs with pre-defined patterns which would be vary on each resource manager. This patch adds some patterns to YARN resource manager. (For others, there's even no executor log url available so cannot define patterns as well.)
Please refer the doc change as well as added UTs in this patch to see how to set up the feature.
## How was this patch tested?
Added UT, as well as manual test with YARN cluster
Closes#23260 from HeartSaVioR/SPARK-26311.
Authored-by: Jungtaek Lim (HeartSaVioR) <kabhwan@gmail.com>
Signed-off-by: Marcelo Vanzin <vanzin@cloudera.com>
## What changes were proposed in this pull request?
This takes over #19621 to add multi-column support to StringIndexer:
1. Supports encoding multiple columns.
2. Previously, when specifying `frequencyDesc` or `frequencyAsc` as `stringOrderType` param in `StringIndexer`, in case of equal frequency, the order of strings is undefined. After this change, the strings with equal frequency are further sorted alphabetically.
## How was this patch tested?
Added tests.
Closes#20146 from viirya/SPARK-11215.
Authored-by: Liang-Chi Hsieh <viirya@gmail.com>
Signed-off-by: Sean Owen <sean.owen@databricks.com>
## What changes were proposed in this pull request?
There are ugly provided dependencies inside core for the following:
* Hive
* Kafka
In this PR I've extracted them out. This PR contains the following:
* Token providers are now loaded with service loader
* Hive token provider moved to hive project
* Kafka token provider extracted into a new project
## How was this patch tested?
Existing + newly added unit tests.
Additionally tested on cluster.
Closes#23499 from gaborgsomogyi/SPARK-26254.
Authored-by: Gabor Somogyi <gabor.g.somogyi@gmail.com>
Signed-off-by: Marcelo Vanzin <vanzin@cloudera.com>
## What changes were proposed in this pull request?
This change exposes the `df` (document frequency) as a public val along with the number of documents (`m`) as part of the IDF model.
* The document frequency is returned as an `Array[Long]`
* If the minimum document frequency is set, this is considered in the df calculation. If the count is less than minDocFreq, the df is 0 for such terms
* numDocs is not very required. But it can be useful, if we plan to provide a provision in future for user to give their own idf function, instead of using a default (log((1+m)/(1+df))). In such cases, the user can provide a function taking input of `m` and `df` and returning the idf value
* Pyspark changes
## How was this patch tested?
The existing test case was edited to also check for the document frequency values.
I am not very good with python or pyspark. I have committed and run tests based on my understanding. Kindly let me know if I have missed anything
Reviewer request: mengxr zjffdu yinxusen
Closes#23549 from purijatin/master.
Authored-by: Jatin Puri <purijatin@gmail.com>
Signed-off-by: Sean Owen <sean.owen@databricks.com>
## What changes were proposed in this pull request?
I know that yarn provided all hadoop configurations. But I guess it may be fine that the historyserver unify all configuration in it. It will be convenient for us to debug some problems.
## How was this patch tested?
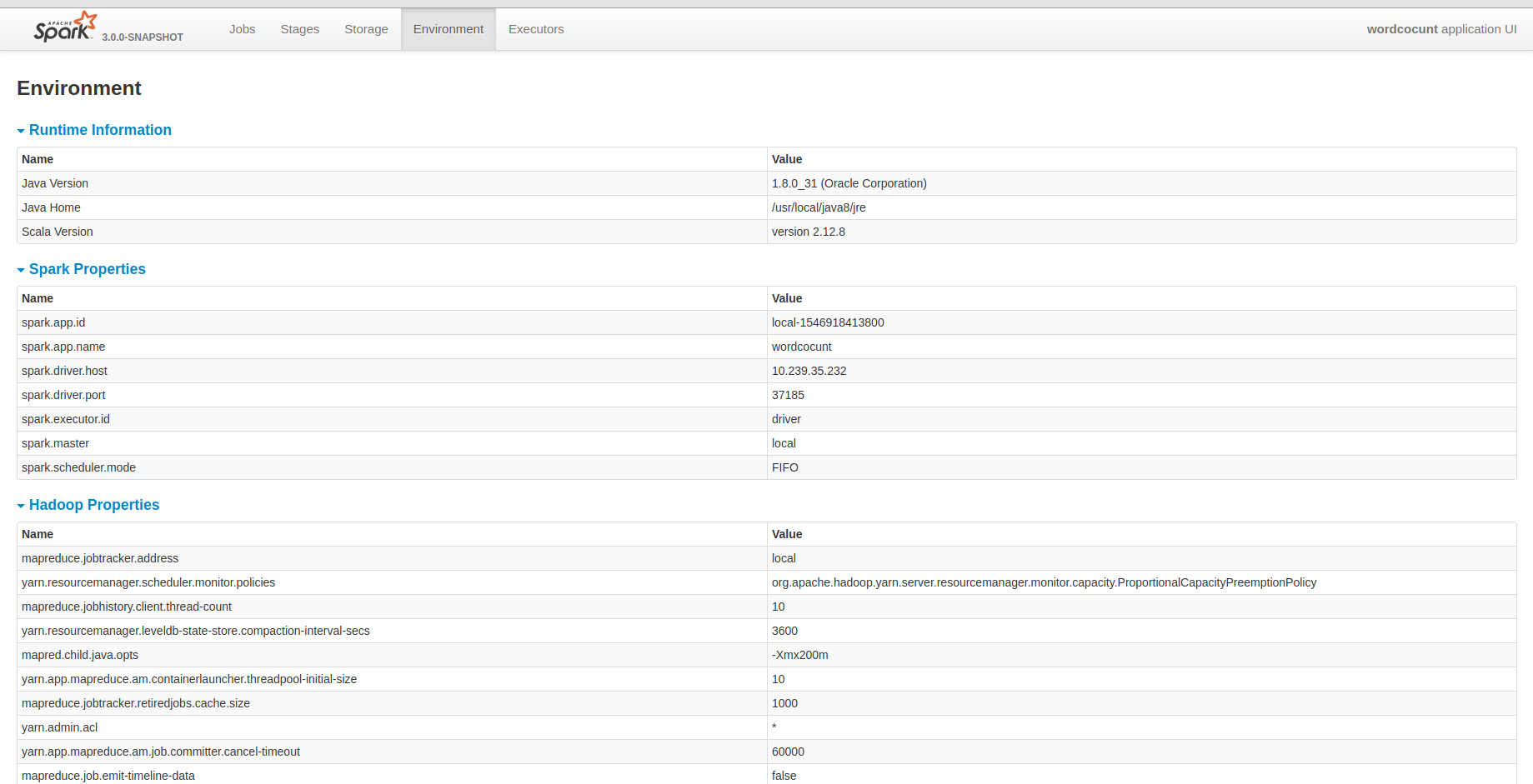
Closes#23486 from deshanxiao/spark-26457.
Lead-authored-by: xiaodeshan <xiaodeshan@xiaomi.com>
Co-authored-by: deshanxiao <42019462+deshanxiao@users.noreply.github.com>
Signed-off-by: Sean Owen <sean.owen@databricks.com>
## What changes were proposed in this pull request?
The PR adds the `trainingCost` value to the `BisectingKMeansSummary`, in order to expose the information retrievable by running `computeCost` on the training dataset. This fills the gap with `KMeans` implementation.
## How was this patch tested?
improved UTs
Closes#22764 from mgaido91/SPARK-25765.
Authored-by: Marco Gaido <marcogaido91@gmail.com>
Signed-off-by: Sean Owen <sean.owen@databricks.com>
## What changes were proposed in this pull request?
A followup of https://github.com/apache/spark/pull/23178 , to keep binary compability by using abstract class.
## How was this patch tested?
Manual test. I created a simple app with Spark 2.4
```
object TryUDF {
def main(args: Array[String]): Unit = {
val spark = SparkSession.builder().appName("test").master("local[*]").getOrCreate()
import spark.implicits._
val f1 = udf((i: Int) => i + 1)
println(f1.deterministic)
spark.range(10).select(f1.asNonNullable().apply($"id")).show()
spark.stop()
}
}
```
When I run it with current master, it fails with
```
java.lang.IncompatibleClassChangeError: Found interface org.apache.spark.sql.expressions.UserDefinedFunction, but class was expected
```
When I run it with this PR, it works
Closes#23351 from cloud-fan/minor.
Authored-by: Wenchen Fan <wenchen@databricks.com>
Signed-off-by: Wenchen Fan <wenchen@databricks.com>
## What changes were proposed in this pull request?
Multiple SparkContexts are discouraged and it has been warning for last 4 years, see SPARK-4180. It could cause arbitrary and mysterious error cases, see SPARK-2243.
Honestly, I didn't even know Spark still allows it, which looks never officially supported, see SPARK-2243.
I believe It should be good timing now to remove this configuration.
## How was this patch tested?
Each doc was manually checked and manually tested:
```
$ ./bin/spark-shell --conf=spark.driver.allowMultipleContexts=true
...
scala> new SparkContext()
org.apache.spark.SparkException: Only one SparkContext should be running in this JVM (see SPARK-2243).The currently running SparkContext was created at:
org.apache.spark.sql.SparkSession$Builder.getOrCreate(SparkSession.scala:939)
...
org.apache.spark.SparkContext$.$anonfun$assertNoOtherContextIsRunning$2(SparkContext.scala:2435)
at scala.Option.foreach(Option.scala:274)
at org.apache.spark.SparkContext$.assertNoOtherContextIsRunning(SparkContext.scala:2432)
at org.apache.spark.SparkContext$.markPartiallyConstructed(SparkContext.scala:2509)
at org.apache.spark.SparkContext.<init>(SparkContext.scala:80)
at org.apache.spark.SparkContext.<init>(SparkContext.scala:112)
... 49 elided
```
Closes#23311 from HyukjinKwon/SPARK-26362.
Authored-by: Hyukjin Kwon <gurwls223@apache.org>
Signed-off-by: Hyukjin Kwon <gurwls223@apache.org>
## What changes were proposed in this pull request?
The evaluators BinaryClassificationEvaluator, RegressionEvaluator, and MulticlassClassificationEvaluator and the corresponding metrics classes BinaryClassificationMetrics, RegressionMetrics and MulticlassMetrics should use sample weight data.
I've closed the PR: https://github.com/apache/spark/pull/16557
as recommended in favor of creating three pull requests, one for each of the evaluators (binary/regression/multiclass) to make it easier to review/update.
The updates to the regression metrics were based on (and updated with new changes based on comments):
https://issues.apache.org/jira/browse/SPARK-11520
("RegressionMetrics should support instance weights")
but the pull request was closed as the changes were never checked in.
## How was this patch tested?
I added tests to the metrics class.
Closes#17085 from imatiach-msft/ilmat/regression-evaluate.
Authored-by: Ilya Matiach <ilmat@microsoft.com>
Signed-off-by: Sean Owen <sean.owen@databricks.com>
## What changes were proposed in this pull request?
1. Implement `SQLShuffleWriteMetricsReporter` on the SQL side as the customized `ShuffleWriteMetricsReporter`.
2. Add shuffle write metrics to `ShuffleExchangeExec`, and use these metrics to create corresponding `SQLShuffleWriteMetricsReporter` in shuffle dependency.
3. Rework on `ShuffleMapTask` to add new class named `ShuffleWriteProcessor` which control shuffle write process, we use sql shuffle write metrics by customizing a ShuffleWriteProcessor on SQL side.
## How was this patch tested?
Add UT in SQLMetricsSuite.
Manually test locally, update screen shot to document attached in JIRA.
Closes#23207 from xuanyuanking/SPARK-26193.
Authored-by: Yuanjian Li <xyliyuanjian@gmail.com>
Signed-off-by: Wenchen Fan <wenchen@databricks.com>
Adds a new method to SparkAppHandle called getError which returns
the exception (if present) that caused the underlying Spark app to
fail.
New tests added to SparkLauncherSuite for the new method.
Closes#21849Closes#23221 from vanzin/SPARK-24243.
Signed-off-by: Marcelo Vanzin <vanzin@cloudera.com>
## What changes were proposed in this pull request?
It's a bad idea to use case class as public API, as it has a very wide surface. For example, the `copy` method, its fields, the companion object, etc.
For a particular case, `UserDefinedFunction`. It has a private constructor, and I believe we only want users to access a few methods:`apply`, `nullable`, `asNonNullable`, etc.
However, all its fields, and `copy` method, and the companion object are public unexpectedly. As a result, we made many tricks to work around the binary compatibility issues.
This PR proposes to only make interfaces public, and hide implementations behind with a private class. Now `UserDefinedFunction` is a pure trait, and the concrete implementation is `SparkUserDefinedFunction`, which is private.
Changing class to interface is not binary compatible(but source compatible), so 3.0 is a good chance to do it.
This is the first PR to go with this direction. If it's accepted, I'll create a umbrella JIRA and fix all the public case classes.
## How was this patch tested?
existing tests.
Closes#23178 from cloud-fan/udf.
Authored-by: Wenchen Fan <wenchen@databricks.com>
Signed-off-by: Wenchen Fan <wenchen@databricks.com>
## What changes were proposed in this pull request?
This is the first step of the data source v2 API refactor [proposal](https://docs.google.com/document/d/1uUmKCpWLdh9vHxP7AWJ9EgbwB_U6T3EJYNjhISGmiQg/edit?usp=sharing)
It adds the new API for batch read, without removing the old APIs, as they are still needed for streaming sources.
More concretely, it adds
1. `TableProvider`, works like an anonymous catalog
2. `Table`, represents a structured data set.
3. `ScanBuilder` and `Scan`, a logical represents of data source scan
4. `Batch`, a physical representation of data source batch scan.
## How was this patch tested?
existing tests
Closes#23086 from cloud-fan/refactor-batch.
Authored-by: Wenchen Fan <wenchen@databricks.com>
Signed-off-by: gatorsmile <gatorsmile@gmail.com>
## What changes were proposed in this pull request?
We have deprecated `OneHotEncoder` at Spark 2.3.0 and introduced `OneHotEncoderEstimator`. At 3.0.0, we remove deprecated `OneHotEncoder` and rename `OneHotEncoderEstimator` to `OneHotEncoder`.
TODO: According to ML migration guide, we need to keep `OneHotEncoderEstimator` as an alias after renaming. This is not done at this patch in order to facilitate review.
## How was this patch tested?
Existing tests.
Closes#23100 from viirya/remove_one_hot_encoder.
Authored-by: Liang-Chi Hsieh <viirya@gmail.com>
Signed-off-by: DB Tsai <d_tsai@apple.com>
## What changes were proposed in this pull request?
This is the write side counterpart to https://github.com/apache/spark/pull/23105
## How was this patch tested?
No behavior change expected, as it is a straightforward refactoring. Updated all existing test cases.
Closes#23106 from rxin/SPARK-26141.
Authored-by: Reynold Xin <rxin@databricks.com>
Signed-off-by: Reynold Xin <rxin@databricks.com>
## What changes were proposed in this pull request?
The PR removes the deprecated method `computeCost` of `KMeans`.
## How was this patch tested?
NA
Closes#22875 from mgaido91/SPARK-25867.
Authored-by: Marco Gaido <marcogaido91@gmail.com>
Signed-off-by: Sean Owen <sean.owen@databricks.com>