## What changes were proposed in this pull request?
Currently the `AttributeReference.withMetadata` method have return type `Attribute`, the rest of with methods in the `AttributeReference` return type are `AttributeReference`, as the [spark-25892](https://issues.apache.org/jira/browse/SPARK-25892?jql=project%20%3D%20SPARK%20AND%20component%20in%20(ML%2C%20PySpark%2C%20SQL)) mentioned.
This PR will change `AttributeReference.withMetadata` method's return type from `Attribute` to `AttributeReference`.
## How was this patch tested?
Run all `sql/test,` `catalyst/test` and `org.apache.spark.sql.execution.streaming.*`
Closes#22918 from kevinyu98/spark-25892.
Authored-by: Kevin Yu <qyu@us.ibm.com>
Signed-off-by: Hyukjin Kwon <gurwls223@apache.org>
## What changes were proposed in this pull request?
In the PR, I propose to add `maxFields` parameter to all functions involved in creation of textual representation of spark plans such as `simpleString` and `verboseString`. New parameter restricts number of fields converted to truncated strings. Any elements beyond the limit will be dropped and replaced by a `"... N more fields"` placeholder. The threshold is bumped up to `Int.MaxValue` for `toFile()`.
## How was this patch tested?
Added a test to `QueryExecutionSuite` which checks `maxFields` impacts on number of truncated fields in `LocalRelation`.
Closes#23159 from MaxGekk/to-file-max-fields.
Lead-authored-by: Maxim Gekk <max.gekk@gmail.com>
Co-authored-by: Maxim Gekk <maxim.gekk@databricks.com>
Signed-off-by: Herman van Hovell <hvanhovell@databricks.com>
## What changes were proposed in this pull request?
Spark SQL doesn't support creating partitioned table using Hive CTAS in SQL syntax. However it is supported by using DataFrameWriter API.
```scala
val df = Seq(("a", 1)).toDF("part", "id")
df.write.format("hive").partitionBy("part").saveAsTable("t")
```
Hive begins to support this syntax in newer version: https://issues.apache.org/jira/browse/HIVE-20241:
```
CREATE TABLE t PARTITIONED BY (part) AS SELECT 1 as id, "a" as part
```
This patch adds this support to SQL syntax.
## How was this patch tested?
Added tests.
Closes#23376 from viirya/hive-ctas-partitioned-table.
Authored-by: Liang-Chi Hsieh <viirya@gmail.com>
Signed-off-by: Wenchen Fan <wenchen@databricks.com>
## What changes were proposed in this pull request?
In the PR, I propose to switch the `DateFormatClass`, `ToUnixTimestamp`, `FromUnixTime`, `UnixTime` on java.time API for parsing/formatting dates and timestamps. The API has been already implemented by the `Timestamp`/`DateFormatter` classes. One of benefit is those classes support parsing timestamps with microsecond precision. Old behaviour can be switched on via SQL config: `spark.sql.legacy.timeParser.enabled` (`false` by default).
## How was this patch tested?
It was tested by existing test suites - `DateFunctionsSuite`, `DateExpressionsSuite`, `JsonSuite`, `CsvSuite`, `SQLQueryTestSuite` as well as PySpark tests.
Closes#23358 from MaxGekk/new-time-cast.
Lead-authored-by: Maxim Gekk <maxim.gekk@databricks.com>
Co-authored-by: Maxim Gekk <max.gekk@gmail.com>
Signed-off-by: Wenchen Fan <wenchen@databricks.com>
## What changes were proposed in this pull request?
fix ExpresionInfo assert error in windows operation system, when running unit tests.
## How was this patch tested?
unit tests
Closes#23363 from yanlin-Lynn/unit-test-windows.
Authored-by: wangyanlin01 <wangyanlin01@baidu.com>
Signed-off-by: Hyukjin Kwon <gurwls223@apache.org>
## What changes were proposed in this pull request?
Variation of https://github.com/apache/spark/pull/20500
I cheated by not referencing fields or columns at all as this exception propagates in contexts where both would be applicable.
## How was this patch tested?
Existing tests
Closes#23373 from srowen/SPARK-14023.2.
Authored-by: Sean Owen <sean.owen@databricks.com>
Signed-off-by: Dongjoon Hyun <dongjoon@apache.org>
## What changes were proposed in this pull request?
The PRs #23150 and #23196 switched JSON and CSV datasources on new formatter for dates/timestamps which is based on `DateTimeFormatter`. In this PR, I replaced `SimpleDateFormat` by `DateTimeFormatter` to reflect the changes.
Closes#23374 from MaxGekk/java-time-docs.
Authored-by: Maxim Gekk <max.gekk@gmail.com>
Signed-off-by: Hyukjin Kwon <gurwls223@apache.org>
## What changes were proposed in this pull request?
GetStructField with different optional names should be semantically equal. We will use this as building block to compare the nested fields used in the plans to be optimized by catalyst optimizer.
This PR also fixes a bug below that accessing nested fields with different cases in case insensitive mode will result `AnalysisException`.
```
sql("create table t (s struct<i: Int>) using json")
sql("select s.I from t group by s.i")
```
which is currently failing
```
org.apache.spark.sql.AnalysisException: expression 'default.t.`s`' is neither present in the group by, nor is it an aggregate function
```
as cloud-fan pointed out.
## How was this patch tested?
New tests are added.
Closes#23353 from dbtsai/nestedEqual.
Lead-authored-by: DB Tsai <d_tsai@apple.com>
Co-authored-by: DB Tsai <dbtsai@dbtsai.com>
Signed-off-by: Dongjoon Hyun <dongjoon@apache.org>
## What changes were proposed in this pull request?
This patch adds explanation of `why "spark.sql.shuffle.partitions" keeps unchanged in structured streaming`, which couple of users already wondered and some of them even thought it as a bug.
This patch would help other end users to know about such behavior before they find by theirselves and being wondered.
## How was this patch tested?
No need to test because this is a simple addition on guide doc with markdown editor.
Closes#22238 from HeartSaVioR/SPARK-25245.
Lead-authored-by: Jungtaek Lim <kabhwan@gmail.com>
Co-authored-by: Jungtaek Lim (HeartSaVioR) <kabhwan@gmail.com>
Signed-off-by: Sean Owen <sean.owen@databricks.com>
## What changes were proposed in this pull request?
Use of `ProcessingTime` class was deprecated in favor of `Trigger.ProcessingTime` in Spark 2.2. And, [SPARK-21464](https://issues.apache.org/jira/browse/SPARK-21464) minimized it at 2.2.1. Recently, it grows again in test suites. This PR aims to clean up newly introduced deprecation warnings for Spark 3.0.
## How was this patch tested?
Pass the Jenkins with existing tests and manually check the warnings.
Closes#23367 from dongjoon-hyun/SPARK-26428.
Authored-by: Dongjoon Hyun <dongjoon@apache.org>
Signed-off-by: Dongjoon Hyun <dongjoon@apache.org>
## What changes were proposed in this pull request?
A followup of https://github.com/apache/spark/pull/23178 , to keep binary compability by using abstract class.
## How was this patch tested?
Manual test. I created a simple app with Spark 2.4
```
object TryUDF {
def main(args: Array[String]): Unit = {
val spark = SparkSession.builder().appName("test").master("local[*]").getOrCreate()
import spark.implicits._
val f1 = udf((i: Int) => i + 1)
println(f1.deterministic)
spark.range(10).select(f1.asNonNullable().apply($"id")).show()
spark.stop()
}
}
```
When I run it with current master, it fails with
```
java.lang.IncompatibleClassChangeError: Found interface org.apache.spark.sql.expressions.UserDefinedFunction, but class was expected
```
When I run it with this PR, it works
Closes#23351 from cloud-fan/minor.
Authored-by: Wenchen Fan <wenchen@databricks.com>
Signed-off-by: Wenchen Fan <wenchen@databricks.com>
## What changes were proposed in this pull request?
Currently, even if I explicitly disable Hive support in SparkR session as below:
```r
sparkSession <- sparkR.session("local[4]", "SparkR", Sys.getenv("SPARK_HOME"),
enableHiveSupport = FALSE)
```
produces when the Hadoop version is not supported by our Hive fork:
```
java.lang.reflect.InvocationTargetException
...
Caused by: java.lang.IllegalArgumentException: Unrecognized Hadoop major version number: 3.1.1.3.1.0.0-78
at org.apache.hadoop.hive.shims.ShimLoader.getMajorVersion(ShimLoader.java:174)
at org.apache.hadoop.hive.shims.ShimLoader.loadShims(ShimLoader.java:139)
at org.apache.hadoop.hive.shims.ShimLoader.getHadoopShims(ShimLoader.java:100)
at org.apache.hadoop.hive.conf.HiveConf$ConfVars.<clinit>(HiveConf.java:368)
... 43 more
Error in handleErrors(returnStatus, conn) :
java.lang.ExceptionInInitializerError
at org.apache.hadoop.hive.conf.HiveConf.<clinit>(HiveConf.java:105)
at java.lang.Class.forName0(Native Method)
at java.lang.Class.forName(Class.java:348)
at org.apache.spark.util.Utils$.classForName(Utils.scala:193)
at org.apache.spark.sql.SparkSession$.hiveClassesArePresent(SparkSession.scala:1116)
at org.apache.spark.sql.api.r.SQLUtils$.getOrCreateSparkSession(SQLUtils.scala:52)
at org.apache.spark.sql.api.r.SQLUtils.getOrCreateSparkSession(SQLUtils.scala)
at sun.reflect.NativeMethodAccessorImpl.invoke0(Native Method)
```
The root cause is that:
```
SparkSession.hiveClassesArePresent
```
check if the class is loadable or not to check if that's in classpath but `org.apache.hadoop.hive.conf.HiveConf` has a check for Hadoop version as static logic which is executed right away. This throws an `IllegalArgumentException` and that's not caught:
36edbac1c8/sql/core/src/main/scala/org/apache/spark/sql/SparkSession.scala (L1113-L1121)
So, currently, if users have a Hive built-in Spark with unsupported Hadoop version by our fork (namely 3+), there's no way to use SparkR even though it could work.
This PR just propose to change the order of bool comparison so that we can don't execute `SparkSession.hiveClassesArePresent` when:
1. `enableHiveSupport` is explicitly disabled
2. `spark.sql.catalogImplementation` is `in-memory`
so that we **only** check `SparkSession.hiveClassesArePresent` when Hive support is explicitly enabled by short circuiting.
## How was this patch tested?
It's difficult to write a test since we don't run tests against Hadoop 3 yet. See https://github.com/apache/spark/pull/21588. Manually tested.
Closes#23356 from HyukjinKwon/SPARK-26422.
Authored-by: Hyukjin Kwon <gurwls223@apache.org>
Signed-off-by: Hyukjin Kwon <gurwls223@apache.org>
## What changes were proposed in this pull request?
`HadoopFileWholeTextReader` and `HadoopFileLinesReader` will be eventually called in `FileSourceScanExec`.
In fact, locality has been implemented in `FileScanRDD`, even if we implement it in `HadoopFileWholeTextReader ` and `HadoopFileLinesReader`, it would be useless.
So I think these `TODO` can be removed.
## How was this patch tested?
N/A
Closes#23339 from 10110346/noneededtodo.
Authored-by: liuxian <liu.xian3@zte.com.cn>
Signed-off-by: Wenchen Fan <wenchen@databricks.com>
## What changes were proposed in this pull request?
`SQLConf` is supposed to be serializable. However, currently it is not serializable in `WithTestConf`. `WithTestConf` uses the method `overrideConfs` in closure, while the classes which implements it (`TestHiveSessionStateBuilder` and `TestSQLSessionStateBuilder`) are not serializable.
This PR is to use a local variable to fix it.
## How was this patch tested?
Add unit test.
Closes#23352 from gengliangwang/serializableSQLConf.
Authored-by: Gengliang Wang <gengliang.wang@databricks.com>
Signed-off-by: gatorsmile <gatorsmile@gmail.com>
## What changes were proposed in this pull request?
Currently, when we infer the schema for scala/java decimals, we return as data type the `SYSTEM_DEFAULT` implementation, ie. the decimal type with precision 38 and scale 18. But this is not right, as we know nothing about the right precision and scale and these values can be not enough to store the data. This problem arises in particular with UDF, where we cast all the input of type `DecimalType` to a `DecimalType(38, 18)`: in case this is not enough, null is returned as input for the UDF.
The PR defines a custom handling for casting to the expected data types for ScalaUDF: the decimal precision and scale is picked from the input, so no casting to different and maybe wrong percision and scale happens.
## How was this patch tested?
added UTs
Closes#23308 from mgaido91/SPARK-26308.
Authored-by: Marco Gaido <marcogaido91@gmail.com>
Signed-off-by: Wenchen Fan <wenchen@databricks.com>
## What changes were proposed in this pull request?
Deprecate Row.merge
## How was this patch tested?
N/A
Closes#23271 from KyleLi1985/master.
Authored-by: 李亮 <liang.li.work@outlook.com>
Signed-off-by: Hyukjin Kwon <gurwls223@apache.org>
## What changes were proposed in this pull request?
In Spark 2.3.0 and previous versions, Hive CTAS command will convert to use data source to write data into the table when the table is convertible. This behavior is controlled by the configs like HiveUtils.CONVERT_METASTORE_ORC and HiveUtils.CONVERT_METASTORE_PARQUET.
In 2.3.1, we drop this optimization by mistake in the PR [SPARK-22977](https://github.com/apache/spark/pull/20521/files#r217254430). Since that Hive CTAS command only uses Hive Serde to write data.
This patch adds this optimization back to Hive CTAS command. This patch adds OptimizedCreateHiveTableAsSelectCommand which uses data source to write data.
## How was this patch tested?
Added test.
Closes#22514 from viirya/SPARK-25271-2.
Authored-by: Liang-Chi Hsieh <viirya@gmail.com>
Signed-off-by: Wenchen Fan <wenchen@databricks.com>
## What changes were proposed in this pull request?
For better test coverage, this pr proposed to use the 4 mixed config sets of `WHOLESTAGE_CODEGEN_ENABLED` and `CODEGEN_FACTORY_MODE` when running `SQLQueryTestSuite`:
1. WHOLESTAGE_CODEGEN_ENABLED=true, CODEGEN_FACTORY_MODE=CODEGEN_ONLY
2. WHOLESTAGE_CODEGEN_ENABLED=false, CODEGEN_FACTORY_MODE=CODEGEN_ONLY
3. WHOLESTAGE_CODEGEN_ENABLED=true, CODEGEN_FACTORY_MODE=NO_CODEGEN
4. WHOLESTAGE_CODEGEN_ENABLED=false, CODEGEN_FACTORY_MODE=NO_CODEGEN
This pr also moved some existing tests into `ExplainSuite` because explain output results are different between codegen and interpreter modes.
## How was this patch tested?
Existing tests.
Closes#23213 from maropu/InterpreterModeTest.
Authored-by: Takeshi Yamamuro <yamamuro@apache.org>
Signed-off-by: Wenchen Fan <wenchen@databricks.com>
## What changes were proposed in this pull request?
This is a small clean up.
By design catalyst rules should be orthogonal: each rule should have its own responsibility. However, the `ColumnPruning` rule does not only do column pruning, but also remove no-op project and window.
This PR updates the `RemoveRedundantProject` rule to remove no-op window as well, and clean up the `ColumnPruning` rule to only do column pruning.
## How was this patch tested?
existing tests
Closes#23343 from cloud-fan/column-pruning.
Authored-by: Wenchen Fan <wenchen@databricks.com>
Signed-off-by: Dongjoon Hyun <dongjoon@apache.org>
## What changes were proposed in this pull request?
In `ReplaceExceptWithFilter` we do not consider properly the case in which the condition returns NULL. Indeed, in that case, since negating NULL still returns NULL, so it is not true the assumption that negating the condition returns all the rows which didn't satisfy it, rows returning NULL may not be returned. This happens when constraints inferred by `InferFiltersFromConstraints` are not enough, as it happens with `OR` conditions.
The rule had also problems with non-deterministic conditions: in such a scenario, this rule would change the probability of the output.
The PR fixes these problem by:
- returning False for the condition when it is Null (in this way we do return all the rows which didn't satisfy it);
- avoiding any transformation when the condition is non-deterministic.
## How was this patch tested?
added UTs
Closes#23315 from mgaido91/SPARK-26366.
Authored-by: Marco Gaido <marcogaido91@gmail.com>
Signed-off-by: gatorsmile <gatorsmile@gmail.com>
## What changes were proposed in this pull request?
Currently, SQL configs are not propagated to executors while schema inferring in CSV datasource. For example, changing of `spark.sql.legacy.timeParser.enabled` does not impact on inferring timestamp types. In the PR, I propose to fix the issue by wrapping schema inferring action using `SQLExecution.withSQLConfPropagated`.
## How was this patch tested?
Added logging to `TimestampFormatter`:
```patch
-object TimestampFormatter {
+object TimestampFormatter extends Logging {
def apply(format: String, timeZone: TimeZone, locale: Locale): TimestampFormatter = {
if (SQLConf.get.legacyTimeParserEnabled) {
+ logError("LegacyFallbackTimestampFormatter is being used")
new LegacyFallbackTimestampFormatter(format, timeZone, locale)
} else {
+ logError("Iso8601TimestampFormatter is being used")
new Iso8601TimestampFormatter(format, timeZone, locale)
}
}
```
and run the command in `spark-shell`:
```shell
$ ./bin/spark-shell --conf spark.sql.legacy.timeParser.enabled=true
```
```scala
scala> Seq("2010|10|10").toDF.repartition(1).write.mode("overwrite").text("/tmp/foo")
scala> spark.read.option("inferSchema", "true").option("header", "false").option("timestampFormat", "yyyy|MM|dd").csv("/tmp/foo").printSchema()
18/12/18 10:47:27 ERROR TimestampFormatter: LegacyFallbackTimestampFormatter is being used
root
|-- _c0: timestamp (nullable = true)
```
Closes#23345 from MaxGekk/csv-schema-infer-propagate-configs.
Authored-by: Maxim Gekk <maxim.gekk@databricks.com>
Signed-off-by: Hyukjin Kwon <gurwls223@apache.org>
## What changes were proposed in this pull request?
This PR proposes to use foreach instead of misuse of map (for Unit). This could cause some weird errors potentially and it's not a good practice anyway. See also SPARK-16694
## How was this patch tested?
N/A
Closes#23341 from HyukjinKwon/followup-SPARK-26081.
Authored-by: Hyukjin Kwon <gurwls223@apache.org>
Signed-off-by: Hyukjin Kwon <gurwls223@apache.org>
## What changes were proposed in this pull request?
The `JsonInferSchema` class is extended to support `TimestampType` inferring from string fields in JSON input:
- If the `prefersDecimal` option is set to `true`, it tries to infer decimal type from the string field.
- If decimal type inference fails or `prefersDecimal` is disabled, `JsonInferSchema` tries to infer `TimestampType`.
- If timestamp type inference fails, `StringType` is returned as the inferred type.
## How was this patch tested?
Added new test suite - `JsonInferSchemaSuite` to check date and timestamp types inferring from JSON using `JsonInferSchema` directly. A few tests were added `JsonSuite` to check type merging and roundtrip tests. This changes was tested by `JsonSuite`, `JsonExpressionsSuite` and `JsonFunctionsSuite` as well.
Closes#23201 from MaxGekk/json-infer-time.
Lead-authored-by: Maxim Gekk <maxim.gekk@databricks.com>
Co-authored-by: Maxim Gekk <max.gekk@gmail.com>
Signed-off-by: Hyukjin Kwon <gurwls223@apache.org>
## What changes were proposed in this pull request?
This PR implements a new feature - window aggregation Pandas UDF for bounded window.
#### Doc:
https://docs.google.com/document/d/14EjeY5z4-NC27-SmIP9CsMPCANeTcvxN44a7SIJtZPc/edit#heading=h.c87w44wcj3wj
#### Example:
```
from pyspark.sql.functions import pandas_udf, PandasUDFType
from pyspark.sql.window import Window
df = spark.range(0, 10, 2).toDF('v')
w1 = Window.partitionBy().orderBy('v').rangeBetween(-2, 4)
w2 = Window.partitionBy().orderBy('v').rowsBetween(-2, 2)
pandas_udf('double', PandasUDFType.GROUPED_AGG)
def avg(v):
return v.mean()
df.withColumn('v_mean', avg(df['v']).over(w1)).show()
# +---+------+
# | v|v_mean|
# +---+------+
# | 0| 1.0|
# | 2| 2.0|
# | 4| 4.0|
# | 6| 6.0|
# | 8| 7.0|
# +---+------+
df.withColumn('v_mean', avg(df['v']).over(w2)).show()
# +---+------+
# | v|v_mean|
# +---+------+
# | 0| 2.0|
# | 2| 3.0|
# | 4| 4.0|
# | 6| 5.0|
# | 8| 6.0|
# +---+------+
```
#### High level changes:
This PR modifies the existing WindowInPandasExec physical node to deal with unbounded (growing, shrinking and sliding) windows.
* `WindowInPandasExec` now share the same base class as `WindowExec` and share utility functions. See `WindowExecBase`
* `WindowFunctionFrame` now has two new functions `currentLowerBound` and `currentUpperBound` - to return the lower and upper window bound for the current output row. It is also modified to allow `AggregateProcessor` == null. Null aggregator processor is used for `WindowInPandasExec` where we don't have an aggregator and only uses lower and upper bound functions from `WindowFunctionFrame`
* The biggest change is in `WindowInPandasExec`, where it is modified to take `currentLowerBound` and `currentUpperBound` and write those values together with the input data to the python process for rolling window aggregation. See `WindowInPandasExec` for more details.
#### Discussion
In benchmarking, I found numpy variant of the rolling window UDF is much faster than the pandas version:
Spark SQL window function: 20s
Pandas variant: ~80s
Numpy variant: 10s
Numpy variant with numba: 4s
Allowing numpy variant of the vectorized UDFs is something I want to discuss because of the performance improvement, but doesn't have to be in this PR.
## How was this patch tested?
New tests
Closes#22305 from icexelloss/SPARK-24561-bounded-window-udf.
Authored-by: Li Jin <ice.xelloss@gmail.com>
Signed-off-by: Hyukjin Kwon <gurwls223@apache.org>
## What changes were proposed in this pull request?
SinkProgress should report similar properties like SourceProgress as long as they are available for given Sink. Count of written rows is metric availble for all Sinks. Since relevant progress information is with respect to commited rows, ideal object to carry this info is WriterCommitMessage. For brevity the implementation will focus only on Sinks with API V2 and on Micro Batch mode. Implemention for Continuous mode will be provided at later date.
### Before
```
{"description":"org.apache.spark.sql.kafka010.KafkaSourceProvider3c0bd317"}
```
### After
```
{"description":"org.apache.spark.sql.kafka010.KafkaSourceProvider3c0bd317","numOutputRows":5000}
```
### This PR is related to:
- https://issues.apache.org/jira/browse/SPARK-24647
- https://issues.apache.org/jira/browse/SPARK-21313
## How was this patch tested?
Existing and new unit tests.
Please review http://spark.apache.org/contributing.html before opening a pull request.
Closes#21919 from vackosar/feature/SPARK-24933-numOutputRows.
Lead-authored-by: Vaclav Kosar <admin@vaclavkosar.com>
Co-authored-by: Kosar, Vaclav: Functions Transformation <Vaclav.Kosar@barclayscapital.com>
Signed-off-by: gatorsmile <gatorsmile@gmail.com>
## What changes were proposed in this pull request?
1. rename `FormatterUtils` to `DateTimeFormatterHelper`, and move it to a separated file
2. move `DateFormatter` and its implementation to a separated file
3. mark some methods as private
4. add `override` to some methods
## How was this patch tested?
existing tests
Closes#23329 from cloud-fan/minor.
Authored-by: Wenchen Fan <wenchen@databricks.com>
Signed-off-by: Hyukjin Kwon <gurwls223@apache.org>
## What changes were proposed in this pull request?
This PR is a follow-up of the PR https://github.com/apache/spark/pull/17899. It is to add the rule TransposeWindow the optimizer batch.
## How was this patch tested?
The existing tests.
Closes#23222 from gatorsmile/followupSPARK-20636.
Authored-by: gatorsmile <gatorsmile@gmail.com>
Signed-off-by: gatorsmile <gatorsmile@gmail.com>
## What changes were proposed in this pull request?
- The original comment about `updateDriverMetrics` is not right.
- Refactor the code to ensure `selectedPartitions ` has been set before sending the driver-side metrics.
- Restore the original name, which is more general and extendable.
## How was this patch tested?
The existing tests.
Closes#23328 from gatorsmile/followupSpark-26142.
Authored-by: gatorsmile <gatorsmile@gmail.com>
Signed-off-by: gatorsmile <gatorsmile@gmail.com>
## What changes were proposed in this pull request?
The optimizer rule `org.apache.spark.sql.catalyst.optimizer.ReorderJoin` performs join reordering on inner joins. This was introduced from SPARK-12032 (https://github.com/apache/spark/pull/10073) in 2015-12.
After it had reordered the joins, though, it didn't check whether or not the output attribute order is still the same as before. Thus, it's possible to have a mismatch between the reordered output attributes order vs the schema that a DataFrame thinks it has.
The same problem exists in the CBO version of join reordering (`CostBasedJoinReorder`) too.
This can be demonstrated with the example:
```scala
spark.sql("create table table_a (x int, y int) using parquet")
spark.sql("create table table_b (i int, j int) using parquet")
spark.sql("create table table_c (a int, b int) using parquet")
val df = spark.sql("""
with df1 as (select * from table_a cross join table_b)
select * from df1 join table_c on a = x and b = i
""")
```
here's what the DataFrame thinks:
```
scala> df.printSchema
root
|-- x: integer (nullable = true)
|-- y: integer (nullable = true)
|-- i: integer (nullable = true)
|-- j: integer (nullable = true)
|-- a: integer (nullable = true)
|-- b: integer (nullable = true)
```
here's what the optimized plan thinks, after join reordering:
```
scala> df.queryExecution.optimizedPlan.output.foreach(a => println(s"|-- ${a.name}: ${a.dataType.typeName}"))
|-- x: integer
|-- y: integer
|-- a: integer
|-- b: integer
|-- i: integer
|-- j: integer
```
If we exclude the `ReorderJoin` rule (using Spark 2.4's optimizer rule exclusion feature), it's back to normal:
```
scala> spark.conf.set("spark.sql.optimizer.excludedRules", "org.apache.spark.sql.catalyst.optimizer.ReorderJoin")
scala> val df = spark.sql("with df1 as (select * from table_a cross join table_b) select * from df1 join table_c on a = x and b = i")
df: org.apache.spark.sql.DataFrame = [x: int, y: int ... 4 more fields]
scala> df.queryExecution.optimizedPlan.output.foreach(a => println(s"|-- ${a.name}: ${a.dataType.typeName}"))
|-- x: integer
|-- y: integer
|-- i: integer
|-- j: integer
|-- a: integer
|-- b: integer
```
Note that this output attribute ordering problem leads to data corruption, and can manifest itself in various symptoms:
* Silently corrupting data, if the reordered columns happen to either have matching types or have sufficiently-compatible types (e.g. all fixed length primitive types are considered as "sufficiently compatible" in an `UnsafeRow`), then only the resulting data is going to be wrong but it might not trigger any alarms immediately. Or
* Weird Java-level exceptions like `java.lang.NegativeArraySizeException`, or even SIGSEGVs.
## How was this patch tested?
Added new unit test in `JoinReorderSuite` and new end-to-end test in `JoinSuite`.
Also made `JoinReorderSuite` and `StarJoinReorderSuite` assert more strongly on maintaining output attribute order.
Closes#23303 from rednaxelafx/fix-join-reorder.
Authored-by: Kris Mok <rednaxelafx@gmail.com>
Signed-off-by: Wenchen Fan <wenchen@databricks.com>
## What changes were proposed in this pull request?
The `CSVInferSchema` class is extended to support inferring of `DateType` from CSV input. The attempt to infer `DateType` is performed after inferring `TimestampType`.
## How was this patch tested?
Added new test for inferring date types from CSV . It was also tested by existing suites like `CSVInferSchemaSuite`, `CsvExpressionsSuite`, `CsvFunctionsSuite` and `CsvSuite`.
Closes#23202 from MaxGekk/csv-date-inferring.
Lead-authored-by: Maxim Gekk <max.gekk@gmail.com>
Co-authored-by: Maxim Gekk <maxim.gekk@databricks.com>
Signed-off-by: Wenchen Fan <wenchen@databricks.com>
## What changes were proposed in this pull request?
CSV parsing accidentally uses the previous good value for a bad input field. See example in Jira.
This PR ensures that the associated column is set to null when an input field cannot be converted.
## How was this patch tested?
Added new test.
Ran all SQL unit tests (testOnly org.apache.spark.sql.*).
Ran pyspark tests for pyspark-sql
Closes#23323 from bersprockets/csv-bad-field.
Authored-by: Bruce Robbins <bersprockets@gmail.com>
Signed-off-by: Hyukjin Kwon <gurwls223@apache.org>
## What changes were proposed in this pull request?
When there is a self-join as result of a IN subquery, the join condition may be invalid, resulting in trivially true predicates and return wrong results.
The PR deduplicates the subquery output in order to avoid the issue.
## How was this patch tested?
added UT
Closes#23057 from mgaido91/SPARK-26078.
Authored-by: Marco Gaido <marcogaido91@gmail.com>
Signed-off-by: Wenchen Fan <wenchen@databricks.com>
## What changes were proposed in this pull request?
In the PR, I propose to switch on **java.time API** for parsing timestamps and dates from JSON inputs with microseconds precision. The SQL config `spark.sql.legacy.timeParser.enabled` allow to switch back to previous behavior with using `java.text.SimpleDateFormat`/`FastDateFormat` for parsing/generating timestamps/dates.
## How was this patch tested?
It was tested by `JsonExpressionsSuite`, `JsonFunctionsSuite` and `JsonSuite`.
Closes#23196 from MaxGekk/json-time-parser.
Lead-authored-by: Maxim Gekk <maxim.gekk@databricks.com>
Co-authored-by: Maxim Gekk <max.gekk@gmail.com>
Signed-off-by: Wenchen Fan <wenchen@databricks.com>
## What changes were proposed in this pull request?
Multiple SparkContexts are discouraged and it has been warning for last 4 years, see SPARK-4180. It could cause arbitrary and mysterious error cases, see SPARK-2243.
Honestly, I didn't even know Spark still allows it, which looks never officially supported, see SPARK-2243.
I believe It should be good timing now to remove this configuration.
## How was this patch tested?
Each doc was manually checked and manually tested:
```
$ ./bin/spark-shell --conf=spark.driver.allowMultipleContexts=true
...
scala> new SparkContext()
org.apache.spark.SparkException: Only one SparkContext should be running in this JVM (see SPARK-2243).The currently running SparkContext was created at:
org.apache.spark.sql.SparkSession$Builder.getOrCreate(SparkSession.scala:939)
...
org.apache.spark.SparkContext$.$anonfun$assertNoOtherContextIsRunning$2(SparkContext.scala:2435)
at scala.Option.foreach(Option.scala:274)
at org.apache.spark.SparkContext$.assertNoOtherContextIsRunning(SparkContext.scala:2432)
at org.apache.spark.SparkContext$.markPartiallyConstructed(SparkContext.scala:2509)
at org.apache.spark.SparkContext.<init>(SparkContext.scala:80)
at org.apache.spark.SparkContext.<init>(SparkContext.scala:112)
... 49 elided
```
Closes#23311 from HyukjinKwon/SPARK-26362.
Authored-by: Hyukjin Kwon <gurwls223@apache.org>
Signed-off-by: Hyukjin Kwon <gurwls223@apache.org>
## What changes were proposed in this pull request?
When using ordinals to access linked list, the time cost is O(n).
## How was this patch tested?
Existing tests.
Closes#23280 from CarolinePeng/update_Two.
Authored-by: CarolinPeng <00244106@zte.intra>
Signed-off-by: Sean Owen <sean.owen@databricks.com>
## What changes were proposed in this pull request?
When using a higher-order function with the same variable name as the existing columns in `Filter` or something which uses `Analyzer.resolveExpressionBottomUp` during the resolution, e.g.,:
```scala
val df = Seq(
(Seq(1, 9, 8, 7), 1, 2),
(Seq(5, 9, 7), 2, 2),
(Seq.empty, 3, 2),
(null, 4, 2)
).toDF("i", "x", "d")
checkAnswer(df.filter("exists(i, x -> x % d == 0)"),
Seq(Row(Seq(1, 9, 8, 7), 1, 2)))
checkAnswer(df.select("x").filter("exists(i, x -> x % d == 0)"),
Seq(Row(1)))
```
the following exception happens:
```
java.lang.ClassCastException: org.apache.spark.sql.catalyst.expressions.BoundReference cannot be cast to org.apache.spark.sql.catalyst.expressions.NamedExpression
at scala.collection.TraversableLike.$anonfun$map$1(TraversableLike.scala:237)
at scala.collection.mutable.ResizableArray.foreach(ResizableArray.scala:62)
at scala.collection.mutable.ResizableArray.foreach$(ResizableArray.scala:55)
at scala.collection.mutable.ArrayBuffer.foreach(ArrayBuffer.scala:49)
at scala.collection.TraversableLike.map(TraversableLike.scala:237)
at scala.collection.TraversableLike.map$(TraversableLike.scala:230)
at scala.collection.AbstractTraversable.map(Traversable.scala:108)
at org.apache.spark.sql.catalyst.expressions.HigherOrderFunction.$anonfun$functionsForEval$1(higherOrderFunctions.scala:147)
at scala.collection.TraversableLike.$anonfun$map$1(TraversableLike.scala:237)
at scala.collection.immutable.List.foreach(List.scala:392)
at scala.collection.TraversableLike.map(TraversableLike.scala:237)
at scala.collection.TraversableLike.map$(TraversableLike.scala:230)
at scala.collection.immutable.List.map(List.scala:298)
at org.apache.spark.sql.catalyst.expressions.HigherOrderFunction.functionsForEval(higherOrderFunctions.scala:145)
at org.apache.spark.sql.catalyst.expressions.HigherOrderFunction.functionsForEval$(higherOrderFunctions.scala:145)
at org.apache.spark.sql.catalyst.expressions.ArrayExists.functionsForEval$lzycompute(higherOrderFunctions.scala:369)
at org.apache.spark.sql.catalyst.expressions.ArrayExists.functionsForEval(higherOrderFunctions.scala:369)
at org.apache.spark.sql.catalyst.expressions.SimpleHigherOrderFunction.functionForEval(higherOrderFunctions.scala:176)
at org.apache.spark.sql.catalyst.expressions.SimpleHigherOrderFunction.functionForEval$(higherOrderFunctions.scala:176)
at org.apache.spark.sql.catalyst.expressions.ArrayExists.functionForEval(higherOrderFunctions.scala:369)
at org.apache.spark.sql.catalyst.expressions.ArrayExists.nullSafeEval(higherOrderFunctions.scala:387)
at org.apache.spark.sql.catalyst.expressions.SimpleHigherOrderFunction.eval(higherOrderFunctions.scala:190)
at org.apache.spark.sql.catalyst.expressions.SimpleHigherOrderFunction.eval$(higherOrderFunctions.scala:185)
at org.apache.spark.sql.catalyst.expressions.ArrayExists.eval(higherOrderFunctions.scala:369)
at org.apache.spark.sql.catalyst.expressions.GeneratedClass$SpecificPredicate.eval(Unknown Source)
at org.apache.spark.sql.execution.FilterExec.$anonfun$doExecute$3(basicPhysicalOperators.scala:216)
at org.apache.spark.sql.execution.FilterExec.$anonfun$doExecute$3$adapted(basicPhysicalOperators.scala:215)
...
```
because the `UnresolvedAttribute`s in `LambdaFunction` are unexpectedly resolved by the rule.
This pr modified to use a placeholder `UnresolvedNamedLambdaVariable` to prevent unexpected resolution.
## How was this patch tested?
Added a test and modified some tests.
Closes#23320 from ueshin/issues/SPARK-26370/hof_resolution.
Authored-by: Takuya UESHIN <ueshin@databricks.com>
Signed-off-by: Wenchen Fan <wenchen@databricks.com>
## What changes were proposed in this pull request?
I was looking at the code and it was a bit difficult to see the life cycle of InMemoryFileIndex passed into getOrInferFileFormatSchema, because once it is passed in, and another time it was created in getOrInferFileFormatSchema. It'd be easier to understand the life cycle if we move the creation of it out.
## How was this patch tested?
This is a simple code move and should be covered by existing tests.
Closes#23317 from rxin/SPARK-26368.
Authored-by: Reynold Xin <rxin@databricks.com>
Signed-off-by: gatorsmile <gatorsmile@gmail.com>
## What changes were proposed in this pull request?
Regarding the performance issue of SPARK-26155, it reports the issue on TPC-DS. I think it is better to add a benchmark for `LongToUnsafeRowMap` which is the root cause of performance regression.
It can be easier to show performance difference between different metric implementations in `LongToUnsafeRowMap`.
## How was this patch tested?
Manually run added benchmark.
Closes#23284 from viirya/SPARK-26337.
Authored-by: Liang-Chi Hsieh <viirya@gmail.com>
Signed-off-by: Wenchen Fan <wenchen@databricks.com>
## What changes were proposed in this pull request?
Added query status updates to ContinuousExecution.
## How was this patch tested?
Existing unit tests + added ContinuousQueryStatusAndProgressSuite.
Closes#23095 from gaborgsomogyi/SPARK-23886.
Authored-by: Gabor Somogyi <gabor.g.somogyi@gmail.com>
Signed-off-by: Marcelo Vanzin <vanzin@cloudera.com>
## What changes were proposed in this pull request?
As discussed in https://github.com/apache/spark/pull/23208/files#r239684490 , we should put `newScanBuilder` in read related mix-in traits like `SupportsBatchRead`, to support write-only table.
In the `Append` operator, we should skip schema validation if not necessary. In the future we would introduce a capability API, so that data source can tell Spark that it doesn't want to do validation.
## How was this patch tested?
existing tests.
Closes#23266 from cloud-fan/ds-read.
Authored-by: Wenchen Fan <wenchen@databricks.com>
Signed-off-by: Hyukjin Kwon <gurwls223@apache.org>
## What changes were proposed in this pull request?
There are some comments issues left when `ConvertToLocalRelation` rule was added (see #22205/[SPARK-25212](https://issues.apache.org/jira/browse/SPARK-25212)). This PR fixes those comments issues.
## How was this patch tested?
N/A
Closes#23273 from seancxmao/ConvertToLocalRelation-doc.
Authored-by: seancxmao <seancxmao@gmail.com>
Signed-off-by: Sean Owen <sean.owen@databricks.com>
## What changes were proposed in this pull request?
cleanup some tests to make sure expression is resolved during test.
## How was this patch tested?
test-only PR
Closes#23297 from cloud-fan/test.
Authored-by: Wenchen Fan <wenchen@databricks.com>
Signed-off-by: Wenchen Fan <wenchen@databricks.com>
## What changes were proposed in this pull request?
Some documents of `Distribution/Partitioning` are stale and misleading, this PR fixes them:
1. `Distribution` never have intra-partition requirement
2. `OrderedDistribution` does not require tuples that share the same value being colocated in the same partition.
3. `RangePartitioning` can provide a weaker guarantee for a prefix of its `ordering` expressions.
## How was this patch tested?
comment-only PR.
Closes#23249 from cloud-fan/doc.
Authored-by: Wenchen Fan <wenchen@databricks.com>
Signed-off-by: Wenchen Fan <wenchen@databricks.com>
## What changes were proposed in this pull request?
Follow up pr for #23207, include following changes:
- Rename `SQLShuffleMetricsReporter` to `SQLShuffleReadMetricsReporter` to make it match with write side naming.
- Display text changes for read side for naming consistent.
- Rename function in `ShuffleWriteProcessor`.
- Delete `private[spark]` in execution package.
## How was this patch tested?
Existing tests.
Closes#23286 from xuanyuanking/SPARK-26193-follow.
Authored-by: Yuanjian Li <xyliyuanjian@gmail.com>
Signed-off-by: Wenchen Fan <wenchen@databricks.com>
## What changes were proposed in this pull request?
If `checkForContinuous` is called ( `checkForStreaming` is called in `checkForContinuous` ), the `checkForStreaming` mothod will be called twice in `createQuery` , this is not necessary, and the `checkForStreaming` method has a lot of statements, so it's better to remove one of them.
## How was this patch tested?
Existing unit tests in `StreamingQueryManagerSuite` and `ContinuousAggregationSuite`
Closes#23251 from 10110346/isUnsupportedOperationCheckEnabled.
Authored-by: liuxian <liu.xian3@zte.com.cn>
Signed-off-by: Dongjoon Hyun <dongjoon@apache.org>
## What changes were proposed in this pull request?
In the PR, I propose to return partial results from JSON datasource and JSON functions in the PERMISSIVE mode if some of JSON fields are parsed and converted to desired types successfully. The changes are made only for `StructType`. Whole bad JSON records are placed into the corrupt column specified by the `columnNameOfCorruptRecord` option or SQL config.
Partial results are not returned for malformed JSON input.
## How was this patch tested?
Added new UT which checks converting JSON strings with one invalid and one valid field at the end of the string.
Closes#23253 from MaxGekk/json-bad-record.
Lead-authored-by: Maxim Gekk <max.gekk@gmail.com>
Co-authored-by: Maxim Gekk <maxim.gekk@databricks.com>
Signed-off-by: Hyukjin Kwon <gurwls223@apache.org>
## What changes were proposed in this pull request?
This is a regression introduced by https://github.com/apache/spark/pull/22104 at Spark 2.4.0.
When we have Python UDF in subquery, we will hit an exception
```
Caused by: java.lang.ClassCastException: org.apache.spark.sql.catalyst.expressions.AttributeReference cannot be cast to org.apache.spark.sql.catalyst.expressions.PythonUDF
at scala.collection.immutable.Stream.map(Stream.scala:414)
at org.apache.spark.sql.execution.python.EvalPythonExec.$anonfun$doExecute$2(EvalPythonExec.scala:98)
at org.apache.spark.rdd.RDD.$anonfun$mapPartitions$2(RDD.scala:815)
...
```
https://github.com/apache/spark/pull/22104 turned `ExtractPythonUDFs` from a physical rule to optimizer rule. However, there is a difference between a physical rule and optimizer rule. A physical rule always runs once, an optimizer rule may be applied twice on a query tree even the rule is located in a batch that only runs once.
For a subquery, the `OptimizeSubqueries` rule will execute the entire optimizer on the query plan inside subquery. Later on subquery will be turned to joins, and the optimizer rules will be applied to it again.
Unfortunately, the `ExtractPythonUDFs` rule is not idempotent. When it's applied twice on a query plan inside subquery, it will produce a malformed plan. It extracts Python UDF from Python exec plans.
This PR proposes 2 changes to be double safe:
1. `ExtractPythonUDFs` should skip python exec plans, to make the rule idempotent
2. `ExtractPythonUDFs` should skip subquery
## How was this patch tested?
a new test.
Closes#23248 from cloud-fan/python.
Authored-by: Wenchen Fan <wenchen@databricks.com>
Signed-off-by: Wenchen Fan <wenchen@databricks.com>
## What changes were proposed in this pull request?
`RDDConversions` would get disproportionately slower as the number of columns in the query increased,
for the type of `converters` before is `scala.collection.immutable.::` which is a subtype of list.
This PR removing `RDDConversions` and using `RowEncoder` to convert the Row to InternalRow.
The test of `PrunedScanSuite` for 2000 columns and 20k rows takes 409 seconds before this PR, and 361 seconds after.
## How was this patch tested?
Test case of `PrunedScanSuite`
Closes#23262 from eatoncys/toarray.
Authored-by: 10129659 <chen.yanshan@zte.com.cn>
Signed-off-by: Hyukjin Kwon <gurwls223@apache.org>
… incorrect.
## What changes were proposed in this pull request?
In the reported heartbeat information, the unit of the memory data is bytes, which is converted by the formatBytes() function in the utils.js file before being displayed in the interface. The cardinality of the unit conversion in the formatBytes function is 1000, which should be 1024.
Change the cardinality of the unit conversion in the formatBytes function to 1024.
## How was this patch tested?
manual tests
Please review http://spark.apache.org/contributing.html before opening a pull request.
Closes#22683 from httfighter/SPARK-25696.
Lead-authored-by: 韩田田00222924 <han.tiantian@zte.com.cn>
Co-authored-by: han.tiantian@zte.com.cn <han.tiantian@zte.com.cn>
Signed-off-by: Sean Owen <sean.owen@databricks.com>
## What changes were proposed in this pull request?
Add appendReadColumns Unit Test for HiveShimSuite.
## How was this patch tested?
```
$ build/sbt
> project hive
> testOnly *HiveShimSuite
```
Closes#23268 from sadhen/refactor/hiveshim.
Authored-by: Darcy Shen <sadhen@zoho.com>
Signed-off-by: Hyukjin Kwon <gurwls223@apache.org>
## What changes were proposed in this pull request?
This is a Spark 2.3 regression introduced in https://github.com/apache/spark/pull/20521. We should add the partition info for InsertIntoHiveTable in CreateHiveTableAsSelectCommand. Otherwise, we will hit the following error by running the newly added test case:
```
[info] - CTAS: INSERT a partitioned table using Hive serde *** FAILED *** (829 milliseconds)
[info] org.apache.spark.SparkException: Requested partitioning does not match the tab1 table:
[info] Requested partitions:
[info] Table partitions: part
[info] at org.apache.spark.sql.hive.execution.InsertIntoHiveTable.processInsert(InsertIntoHiveTable.scala:179)
[info] at org.apache.spark.sql.hive.execution.InsertIntoHiveTable.run(InsertIntoHiveTable.scala:107)
```
## How was this patch tested?
Added a test case.
Closes#23255 from gatorsmile/fixCTAS.
Authored-by: gatorsmile <gatorsmile@gmail.com>
Signed-off-by: Wenchen Fan <wenchen@databricks.com>
## What changes were proposed in this pull request?
1. Implement `SQLShuffleWriteMetricsReporter` on the SQL side as the customized `ShuffleWriteMetricsReporter`.
2. Add shuffle write metrics to `ShuffleExchangeExec`, and use these metrics to create corresponding `SQLShuffleWriteMetricsReporter` in shuffle dependency.
3. Rework on `ShuffleMapTask` to add new class named `ShuffleWriteProcessor` which control shuffle write process, we use sql shuffle write metrics by customizing a ShuffleWriteProcessor on SQL side.
## How was this patch tested?
Add UT in SQLMetricsSuite.
Manually test locally, update screen shot to document attached in JIRA.
Closes#23207 from xuanyuanking/SPARK-26193.
Authored-by: Yuanjian Li <xyliyuanjian@gmail.com>
Signed-off-by: Wenchen Fan <wenchen@databricks.com>
## What changes were proposed in this pull request?
A followup of https://github.com/apache/spark/pull/23043
There are 4 places we need to deal with NaN and -0.0:
1. comparison expressions. `-0.0` and `0.0` should be treated as same. Different NaNs should be treated as same.
2. Join keys. `-0.0` and `0.0` should be treated as same. Different NaNs should be treated as same.
3. grouping keys. `-0.0` and `0.0` should be assigned to the same group. Different NaNs should be assigned to the same group.
4. window partition keys. `-0.0` and `0.0` should be treated as same. Different NaNs should be treated as same.
The case 1 is OK. Our comparison already handles NaN and -0.0, and for struct/array/map, we will recursively compare the fields/elements.
Case 2, 3 and 4 are problematic, as they compare `UnsafeRow` binary directly, and different NaNs have different binary representation, and the same thing happens for -0.0 and 0.0.
To fix it, a simple solution is: normalize float/double when building unsafe data (`UnsafeRow`, `UnsafeArrayData`, `UnsafeMapData`). Then we don't need to worry about it anymore.
Following this direction, this PR moves the handling of NaN and -0.0 from `Platform` to `UnsafeWriter`, so that places like `UnsafeRow.setFloat` will not handle them, which reduces the perf overhead. It's also easier to add comments explaining why we do it in `UnsafeWriter`.
## How was this patch tested?
existing tests
Closes#23239 from cloud-fan/minor.
Authored-by: Wenchen Fan <wenchen@databricks.com>
Signed-off-by: Dongjoon Hyun <dongjoon@apache.org>
## What changes were proposed in this pull request?
Parquet files appear to have nullability info when being written, not being read.
## How was this patch tested?
Some test code: (running spark 2.3, but the relevant code in DataSource looks identical on master)
case class NullTest(bo: Boolean, opbol: Option[Boolean])
val testDf = spark.createDataFrame(Seq(NullTest(true, Some(false))))
defined class NullTest
testDf: org.apache.spark.sql.DataFrame = [bo: boolean, opbol: boolean]
testDf.write.parquet("s3://asana-stats/tmp_dima/parquet_check_schema")
spark.read.parquet("s3://asana-stats/tmp_dima/parquet_check_schema/part-00000-b1bf4a19-d9fe-4ece-a2b4-9bbceb490857-c000.snappy.parquet4").printSchema()
root
|-- bo: boolean (nullable = true)
|-- opbol: boolean (nullable = true)
Meanwhile, the parquet file formed does have nullable info:
[]batchprod-report000:/tmp/dimakamalov-batch$ aws s3 ls s3://asana-stats/tmp_dima/parquet_check_schema/
2018-10-17 21:03:52 0 _SUCCESS
2018-10-17 21:03:50 504 part-00000-b1bf4a19-d9fe-4ece-a2b4-9bbceb490857-c000.snappy.parquet
[]batchprod-report000:/tmp/dimakamalov-batch$ aws s3 cp s3://asana-stats/tmp_dima/parquet_check_schema/part-00000-b1bf4a19-d9fe-4ece-a2b4-9bbceb490857-c000.snappy.parquet .
download: s3://asana-stats/tmp_dima/parquet_check_schema/part-00000-b1bf4a19-d9fe-4ece-a2b4-9bbceb490857-c000.snappy.parquet to ./part-00000-b1bf4a19-d9fe-4ece-a2b4-9bbceb490857-c000.snappy.parquet
[]batchprod-report000:/tmp/dimakamalov-batch$ java -jar parquet-tools-1.8.2.jar schema part-00000-b1bf4a19-d9fe-4ece-a2b4-9bbceb490857-c000.snappy.parquet
message spark_schema {
required boolean bo;
optional boolean opbol;
}
Closes#22759 from dima-asana/dima-asana-nullable-parquet-doc.
Authored-by: dima-asana <42555784+dima-asana@users.noreply.github.com>
Signed-off-by: Sean Owen <sean.owen@databricks.com>
## What changes were proposed in this pull request?
This is a follow-up of #23031 to rename the config name to `spark.sql.legacy.setCommandRejectsSparkCoreConfs`.
## How was this patch tested?
Existing tests.
Closes#23245 from ueshin/issues/SPARK-26060/rename_config.
Authored-by: Takuya UESHIN <ueshin@databricks.com>
Signed-off-by: Dongjoon Hyun <dongjoon@apache.org>
## What changes were proposed in this pull request?
Currently if user provides data schema, partition column values are converted as per it. But if the conversion failed, e.g. converting string to int, the column value is null.
This PR proposes to throw exception in such case, instead of converting into null value silently:
1. These null partition column values doesn't make sense to users in most cases. It is better to show the conversion failure, and then users can adjust the schema or ETL jobs to fix it.
2. There are always exceptions on such conversion failure for non-partition data columns. Partition columns should have the same behavior.
We can reproduce the case above as following:
```
/tmp/testDir
├── p=bar
└── p=foo
```
If we run:
```
val schema = StructType(Seq(StructField("p", IntegerType, false)))
spark.read.schema(schema).csv("/tmp/testDir/").show()
```
We will get:
```
+----+
| p|
+----+
|null|
|null|
+----+
```
## How was this patch tested?
Unit test
Closes#23215 from gengliangwang/SPARK-26263.
Authored-by: Gengliang Wang <gengliang.wang@databricks.com>
Signed-off-by: Wenchen Fan <wenchen@databricks.com>
## What changes were proposed in this pull request?
`enablePerfMetrics `was originally designed in `BytesToBytesMap `to control `getNumHashCollisions getTimeSpentResizingNs getAverageProbesPerLookup`.
However, as the Spark version gradual progress. this parameter is only used for `getAverageProbesPerLookup ` and always given to true when using `BytesToBytesMap`.
it is also dangerous to determine whether `getAverageProbesPerLookup `opens and throws an `IllegalStateException `exception.
So this pr will be remove `enablePerfMetrics `parameter from `BytesToBytesMap`. thanks.
## How was this patch tested?
the existed test cases.
Closes#23244 from heary-cao/enablePerfMetrics.
Authored-by: caoxuewen <cao.xuewen@zte.com.cn>
Signed-off-by: Wenchen Fan <wenchen@databricks.com>
## What changes were proposed in this pull request?
When executing `toPandas` with Arrow enabled, partitions that arrive in the JVM out-of-order must be buffered before they can be send to Python. This causes an excess of memory to be used in the driver JVM and increases the time it takes to complete because data must sit in the JVM waiting for preceding partitions to come in.
This change sends un-ordered partitions to Python as soon as they arrive in the JVM, followed by a list of partition indices so that Python can assemble the data in the correct order. This way, data is not buffered at the JVM and there is no waiting on particular partitions so performance will be increased.
Followup to #21546
## How was this patch tested?
Added new test with a large number of batches per partition, and test that forces a small delay in the first partition. These test that partitions are collected out-of-order and then are are put in the correct order in Python.
## Performance Tests - toPandas
Tests run on a 4 node standalone cluster with 32 cores total, 14.04.1-Ubuntu and OpenJDK 8
measured wall clock time to execute `toPandas()` and took the average best time of 5 runs/5 loops each.
Test code
```python
df = spark.range(1 << 25, numPartitions=32).toDF("id").withColumn("x1", rand()).withColumn("x2", rand()).withColumn("x3", rand()).withColumn("x4", rand())
for i in range(5):
start = time.time()
_ = df.toPandas()
elapsed = time.time() - start
```
Spark config
```
spark.driver.memory 5g
spark.executor.memory 5g
spark.driver.maxResultSize 2g
spark.sql.execution.arrow.enabled true
```
Current Master w/ Arrow stream | This PR
---------------------|------------
5.16207 | 4.342533
5.133671 | 4.399408
5.147513 | 4.468471
5.105243 | 4.36524
5.018685 | 4.373791
Avg Master | Avg This PR
------------------|--------------
5.1134364 | 4.3898886
Speedup of **1.164821449**
Closes#22275 from BryanCutler/arrow-toPandas-oo-batches-SPARK-25274.
Authored-by: Bryan Cutler <cutlerb@gmail.com>
Signed-off-by: Bryan Cutler <cutlerb@gmail.com>
## What changes were proposed in this pull request?
this code come from PR: https://github.com/apache/spark/pull/11190,
but this code has never been used, only since PR: https://github.com/apache/spark/pull/14548,
Let's continue fix it. thanks.
## How was this patch tested?
N / A
Closes#23227 from heary-cao/unuseSparkPlan.
Authored-by: caoxuewen <cao.xuewen@zte.com.cn>
Signed-off-by: Wenchen Fan <wenchen@databricks.com>
## What changes were proposed in this pull request?
The PR starts from the [comment](https://github.com/apache/spark/pull/23124#discussion_r236112390) in the main one and it aims at:
- simplifying the code for `MapConcat`;
- be more precise in checking the limit size.
## How was this patch tested?
existing tests
Closes#23217 from mgaido91/SPARK-25829_followup.
Authored-by: Marco Gaido <marcogaido91@gmail.com>
Signed-off-by: Wenchen Fan <wenchen@databricks.com>
## What changes were proposed in this pull request?
When we encode a Decimal from external source we don't check for overflow. That method is useful not only in order to enforce that we can represent the correct value in the specified range, but it also changes the underlying data to the right precision/scale. Since in our code generation we assume that a decimal has exactly the same precision and scale of its data type, missing to enforce it can lead to corrupted output/results when there are subsequent transformations.
## How was this patch tested?
added UT
Closes#23210 from mgaido91/SPARK-26233.
Authored-by: Marco Gaido <marcogaido91@gmail.com>
Signed-off-by: Dongjoon Hyun <dongjoon@apache.org>
## What changes were proposed in this pull request?
In the PR, I propose to use **java.time API** for parsing timestamps and dates from CSV content with microseconds precision. The SQL config `spark.sql.legacy.timeParser.enabled` allow to switch back to previous behaviour with using `java.text.SimpleDateFormat`/`FastDateFormat` for parsing/generating timestamps/dates.
## How was this patch tested?
It was tested by `UnivocityParserSuite`, `CsvExpressionsSuite`, `CsvFunctionsSuite` and `CsvSuite`.
Closes#23150 from MaxGekk/time-parser.
Lead-authored-by: Maxim Gekk <max.gekk@gmail.com>
Co-authored-by: Maxim Gekk <maxim.gekk@databricks.com>
Signed-off-by: Sean Owen <sean.owen@databricks.com>
## What changes were proposed in this pull request?
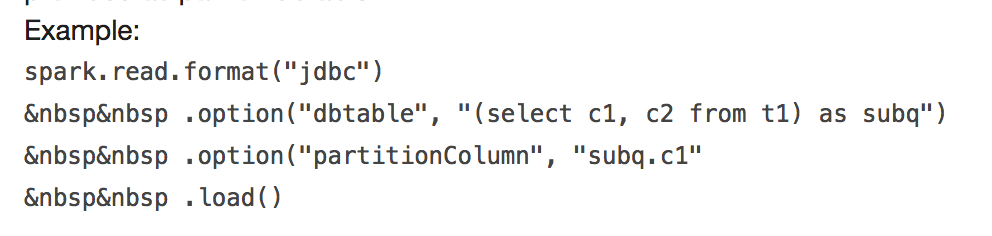
It will throw:
```
requirement failed: When reading JDBC data sources, users need to specify all or none for the following options: 'partitionColumn', 'lowerBound', 'upperBound', and 'numPartitions'
```
and
```
User-defined partition column subq.c1 not found in the JDBC relation ...
```
This PR fix this error example.
## How was this patch tested?
manual tests
Closes#23170 from wangyum/SPARK-24499.
Authored-by: Yuming Wang <yumwang@ebay.com>
Signed-off-by: Sean Owen <sean.owen@databricks.com>
## What changes were proposed in this pull request?
In the DDLSuit, there are four test cases have the same codes , writing a function can combine the same code.
## How was this patch tested?
existing tests.
Closes#23194 from CarolinePeng/Update_temp.
Authored-by: 彭灿00244106 <00244106@zte.intra>
Signed-off-by: Takeshi Yamamuro <yamamuro@apache.org>
## What changes were proposed in this pull request?
In SPARK-23711, we have implemented the expression fallback logic to an interpreted mode. So, this pr fixed code to support the same fallback mode in `SafeProjection` based on `CodeGeneratorWithInterpretedFallback`.
## How was this patch tested?
Add tests in `CodeGeneratorWithInterpretedFallbackSuite` and `UnsafeRowConverterSuite`.
Closes#22468 from maropu/SPARK-25374-3.
Authored-by: Takeshi Yamamuro <yamamuro@apache.org>
Signed-off-by: Wenchen Fan <wenchen@databricks.com>
## What changes were proposed in this pull request?
Currently in the Analyzer, we have two methods 1) Resolve 2)ResolveExpressions that are called at different code paths to resolve attributes, column ordinal and extract value expressions. ~~In this PR, we combine the two into one method to make sure, there is only one method that is tasked with resolving the attributes.~~
Update the description of the methods and use better names to make it easier to know when to make use of one method vs the other.
## How was this patch tested?
Existing tests.
Closes#22899 from dilipbiswal/SPARK-25573-final.
Authored-by: Dilip Biswal <dbiswal@us.ibm.com>
Signed-off-by: gatorsmile <gatorsmile@gmail.com>
## What changes were proposed in this pull request?
This pr is to return an empty config set when regenerating the golden files in `SQLQueryTestSuite`.
This is the follow-up of #22512.
## How was this patch tested?
N/A
Closes#23212 from maropu/SPARK-25498-FOLLOWUP.
Authored-by: Takeshi Yamamuro <yamamuro@apache.org>
Signed-off-by: Wenchen Fan <wenchen@databricks.com>
## What changes were proposed in this pull request?
Since `AggregationIterator` uses `MutableProjection` for `UnsafeRow`, `InterpretedMutableProjection` needs to handle `UnsafeRow` as buffer internally for fixed-length types only.
## How was this patch tested?
Run 'SQLQueryTestSuite' with the interpreted mode.
Closes#22512 from maropu/InterpreterTest.
Authored-by: Takeshi Yamamuro <yamamuro@apache.org>
Signed-off-by: Wenchen Fan <wenchen@databricks.com>
## What changes were proposed in this pull request?
For now the `hasMinMaxStats` will return the same as `hasCountStats`, which is obviously not as expected.
## How was this patch tested?
Existing tests.
Closes#23152 from adrian-wang/minmaxstats.
Authored-by: Daoyuan Wang <me@daoyuan.wang>
Signed-off-by: Wenchen Fan <wenchen@databricks.com>
## What changes were proposed in this pull request?
Partition column name is required to be unique under the same directory. The following paths are invalid partitioned directory:
```
hdfs://host:9000/path/a=1
hdfs://host:9000/path/b=2
```
If case sensitive, the following paths should be invalid too:
```
hdfs://host:9000/path/a=1
hdfs://host:9000/path/A=2
```
Since column 'a' and 'A' are different, and it is wrong to use either one as the column name in partition schema.
Also, there is a `TODO` comment in the code. Currently the Spark doesn't validate such case when `CASE_SENSITIVE` enabled.
This PR is to resolve the problem.
## How was this patch tested?
Add unit test
Closes#23186 from gengliangwang/SPARK-26230.
Authored-by: Gengliang Wang <gengliang.wang@databricks.com>
Signed-off-by: Wenchen Fan <wenchen@databricks.com>
## What changes were proposed in this pull request?
In the PR, I propose to change behaviour of `UnivocityParser` and `FailureSafeParser`, and return all fields that were parsed and converted to expected types successfully instead of just returning a row with all `null`s for a bad input in the `PERMISSIVE` mode. For example, for CSV line `0,2013-111-11 12:13:14` and DDL schema `a int, b timestamp`, new result is `Row(0, null)`.
## How was this patch tested?
It was checked by existing tests from `CsvSuite` and `CsvFunctionsSuite`.
Closes#23120 from MaxGekk/failuresafe-partial-result.
Authored-by: Maxim Gekk <max.gekk@gmail.com>
Signed-off-by: Wenchen Fan <wenchen@databricks.com>
## What changes were proposed in this pull request?
When build hash Map with one row of data and run out of memory, we should throw a SparkOutOfMemoryError exception, which is more accurate than SparkException. this PR fix it.
## How was this patch tested?
N / A
Closes#23190 from heary-cao/throwUnsafeHashedRelation.
Authored-by: caoxuewen <cao.xuewen@zte.com.cn>
Signed-off-by: Wenchen Fan <wenchen@databricks.com>
## What changes were proposed in this pull request?
How to reproduce this issue:
```scala
scala> val meta = new org.apache.spark.sql.types.MetadataBuilder().putNull("key").build().json
java.lang.NullPointerException
at org.apache.spark.sql.types.Metadata$.org$apache$spark$sql$types$Metadata$$toJsonValue(Metadata.scala:196)
at org.apache.spark.sql.types.Metadata$$anonfun$1.apply(Metadata.scala:180)
```
This pr fix `NullPointerException` when `Metadata` serialize `null` values.
## How was this patch tested?
unit tests
Closes#23164 from wangyum/SPARK-26198.
Authored-by: Yuming Wang <yumwang@ebay.com>
Signed-off-by: Sean Owen <sean.owen@databricks.com>
## What changes were proposed in this pull request?
Add headers to empty csv files when header=true, because otherwise these files are invalid when reading.
## How was this patch tested?
Added test for roundtrip of empty dataframe to csv file with headers and back in CSVSuite
Please review http://spark.apache.org/contributing.html before opening a pull request.
Closes#23173 from koertkuipers/feat-empty-csv-with-header.
Authored-by: Koert Kuipers <koert@tresata.com>
Signed-off-by: Hyukjin Kwon <gurwls223@apache.org>
## What changes were proposed in this pull request?
UnsupportedOperationException messages are not the same with method name.This PR correct these messages.
## How was this patch tested?
NA
Closes#23154 from lcqzte10192193/wid-lcq-1127.
Authored-by: lichaoqun <li.chaoqun@zte.com.cn>
Signed-off-by: Wenchen Fan <wenchen@databricks.com>
## What changes were proposed in this pull request?
It's a bad idea to use case class as public API, as it has a very wide surface. For example, the `copy` method, its fields, the companion object, etc.
For a particular case, `UserDefinedFunction`. It has a private constructor, and I believe we only want users to access a few methods:`apply`, `nullable`, `asNonNullable`, etc.
However, all its fields, and `copy` method, and the companion object are public unexpectedly. As a result, we made many tricks to work around the binary compatibility issues.
This PR proposes to only make interfaces public, and hide implementations behind with a private class. Now `UserDefinedFunction` is a pure trait, and the concrete implementation is `SparkUserDefinedFunction`, which is private.
Changing class to interface is not binary compatible(but source compatible), so 3.0 is a good chance to do it.
This is the first PR to go with this direction. If it's accepted, I'll create a umbrella JIRA and fix all the public case classes.
## How was this patch tested?
existing tests.
Closes#23178 from cloud-fan/udf.
Authored-by: Wenchen Fan <wenchen@databricks.com>
Signed-off-by: Wenchen Fan <wenchen@databricks.com>
## What changes were proposed in this pull request?
In the PR, I propose filtering out all empty files inside of `FileSourceScanExec` and exclude them from file splits. It should reduce overhead of opening and reading files without any data, and as consequence datasources will not produce empty partitions for such files.
## How was this patch tested?
Added a test which creates an empty and non-empty files. If empty files are ignored in load, Text datasource in the `wholetext` mode must create only one partition for non-empty file.
Closes#23130 from MaxGekk/ignore-empty-files.
Authored-by: Maxim Gekk <max.gekk@gmail.com>
Signed-off-by: Wenchen Fan <wenchen@databricks.com>
## What changes were proposed in this pull request?
This is a follow pr of #23176.
`In` and `InSet` are semantically equal, so the tests for `In` should pass with `InSet`, and vice versa.
This combines those test cases.
## How was this patch tested?
The combined tests and existing tests.
Closes#23187 from ueshin/issues/SPARK-26211/in_inset_tests.
Authored-by: Takuya UESHIN <ueshin@databricks.com>
Signed-off-by: Wenchen Fan <wenchen@databricks.com>
## What changes were proposed in this pull request?
This is a small change for better debugging: to pass query uuid in IncrementalExecution, when we look at the QueryExecution in isolation to trace back the query.
## How was this patch tested?
N/A - just add some field for better debugging.
Closes#23192 from rxin/SPARK-26241.
Authored-by: Reynold Xin <rxin@databricks.com>
Signed-off-by: gatorsmile <gatorsmile@gmail.com>
## What changes were proposed in this pull request?
In an earlier PR, we missed measuring the optimization phase time for streaming queries. This patch adds it.
## How was this patch tested?
Given this is a debugging feature, and it is very convoluted to add tests to verify the phase is set properly, I am not introducing a streaming specific test.
Closes#23193 from rxin/SPARK-26226-1.
Authored-by: Reynold Xin <rxin@databricks.com>
Signed-off-by: gatorsmile <gatorsmile@gmail.com>
## What changes were proposed in this pull request?
Correct some document description errors.
## How was this patch tested?
N/A
Closes#23162 from 10110346/docerror.
Authored-by: liuxian <liu.xian3@zte.com.cn>
Signed-off-by: Sean Owen <sean.owen@databricks.com>
## What changes were proposed in this pull request?
Currently, the common `withTempDir` function is used in Spark SQL test cases. To handle `val dir = Utils. createTempDir()` and `Utils. deleteRecursively (dir)`. Unfortunately, the `withTempDir` function cannot be used in the Spark Core test case. This PR Sharing `withTempDir` function in Spark Sql and SparkCore to clean up SparkCore test cases. thanks.
## How was this patch tested?
N / A
Closes#23151 from heary-cao/withCreateTempDir.
Authored-by: caoxuewen <cao.xuewen@zte.com.cn>
Signed-off-by: Hyukjin Kwon <gurwls223@apache.org>
## What changes were proposed in this pull request?
This patch changes the query plan tracker added earlier to report phase timeline, rather than just a duration for each phase. This way, we can easily find time that's unaccounted for.
## How was this patch tested?
Updated test cases to reflect that.
Closes#23183 from rxin/SPARK-26226.
Authored-by: Reynold Xin <rxin@databricks.com>
Signed-off-by: gatorsmile <gatorsmile@gmail.com>
## What changes were proposed in this pull request?
This is the first step of the data source v2 API refactor [proposal](https://docs.google.com/document/d/1uUmKCpWLdh9vHxP7AWJ9EgbwB_U6T3EJYNjhISGmiQg/edit?usp=sharing)
It adds the new API for batch read, without removing the old APIs, as they are still needed for streaming sources.
More concretely, it adds
1. `TableProvider`, works like an anonymous catalog
2. `Table`, represents a structured data set.
3. `ScanBuilder` and `Scan`, a logical represents of data source scan
4. `Batch`, a physical representation of data source batch scan.
## How was this patch tested?
existing tests
Closes#23086 from cloud-fan/refactor-batch.
Authored-by: Wenchen Fan <wenchen@databricks.com>
Signed-off-by: gatorsmile <gatorsmile@gmail.com>
## What changes were proposed in this pull request?
This PR is to fix a regression introduced in: https://github.com/apache/spark/pull/21004/files#r236998030
If user specifies schema, Spark don't need to infer data type for of partition columns, otherwise the data type might not match with the one user provided.
E.g. for partition directory `p=4d`, after data type inference the column value will be `4.0`.
See https://issues.apache.org/jira/browse/SPARK-26188 for more details.
Note that user specified schema **might not cover all the data columns**:
```
val schema = new StructType()
.add("id", StringType)
.add("ex", ArrayType(StringType))
val df = spark.read
.schema(schema)
.format("parquet")
.load(src.toString)
assert(df.schema.toList === List(
StructField("ex", ArrayType(StringType)),
StructField("part", IntegerType), // inferred partitionColumn dataType
StructField("id", StringType))) // used user provided partitionColumn dataType
```
For the missing columns in user specified schema, Spark still need to infer their data types if `partitionColumnTypeInferenceEnabled` is enabled.
To implement the partially inference, refactor `PartitioningUtils.parsePartitions` and pass the user specified schema as parameter to cast partition values.
## How was this patch tested?
Add unit test.
Closes#23165 from gengliangwang/fixFileIndex.
Authored-by: Gengliang Wang <gengliang.wang@databricks.com>
Signed-off-by: Wenchen Fan <wenchen@databricks.com>
## What changes were proposed in this pull request?
Currently the `SET` command works without any warnings even if the specified key is for `SparkConf` entries and it has no effect because the command does not update `SparkConf`, but the behavior might confuse users. We should track `SparkConf` entries and make the command reject for such entries.
## How was this patch tested?
Added a test and existing tests.
Closes#23031 from ueshin/issues/SPARK-26060/set_command.
Authored-by: Takuya UESHIN <ueshin@databricks.com>
Signed-off-by: Wenchen Fan <wenchen@databricks.com>
## What changes were proposed in this pull request?
In the PR, I propose using of the locale option to parse decimals from CSV input. After the changes, `UnivocityParser` converts input string to `BigDecimal` and to Spark's Decimal by using `java.text.DecimalFormat`.
## How was this patch tested?
Added a test for the `en-US`, `ko-KR`, `ru-RU`, `de-DE` locales.
Closes#22979 from MaxGekk/decimal-parsing-locale.
Lead-authored-by: Maxim Gekk <maxim.gekk@databricks.com>
Co-authored-by: Maxim Gekk <max.gekk@gmail.com>
Signed-off-by: hyukjinkwon <gurwls223@apache.org>
## What changes were proposed in this pull request?
Follow up for https://github.com/apache/spark/pull/23128, move sql read metrics relatives to `SQLShuffleMetricsReporter`, in order to put sql shuffle read metrics relatives closer and avoid possible problem about forgetting update SQLShuffleMetricsReporter while new metrics added by others.
## How was this patch tested?
Existing tests.
Closes#23175 from xuanyuanking/SPARK-26142-follow.
Authored-by: Yuanjian Li <xyliyuanjian@gmail.com>
Signed-off-by: Reynold Xin <rxin@databricks.com>
## What changes were proposed in this pull request?
In the PR, I propose to postpone creation of `OutputStream`/`Univocity`/`JacksonGenerator` till the first row should be written. This prevents creation of empty files for empty partitions. So, no need to open and to read such files back while loading data from the location.
## How was this patch tested?
Added tests for Text, JSON and CSV datasource where empty dataset is written but should not produce any files.
Closes#23052 from MaxGekk/text-empty-files.
Lead-authored-by: Maxim Gekk <max.gekk@gmail.com>
Co-authored-by: Maxim Gekk <maxim.gekk@databricks.com>
Signed-off-by: Sean Owen <sean.owen@databricks.com>
## What changes were proposed in this pull request?
Currently `InSet` doesn't work properly for binary type, or struct and array type with null value in the set.
Because, as for binary type, the `HashSet` doesn't work properly for `Array[Byte]`, and as for struct and array type with null value in the set, the `ordering` will throw a `NPE`.
## How was this patch tested?
Added a few tests.
Closes#23176 from ueshin/issues/SPARK-26211/inset.
Authored-by: Takuya UESHIN <ueshin@databricks.com>
Signed-off-by: Wenchen Fan <wenchen@databricks.com>
## What changes were proposed in this pull request?
In the PR, I propose using of the locale option to parse (and infer) decimals from JSON input. After the changes, `JacksonParser` converts input string to `BigDecimal` and to Spark's Decimal by using `java.text.DecimalFormat`. New behaviour can be switched off via SQL config `spark.sql.legacy.decimalParsing.enabled`.
## How was this patch tested?
Added 2 tests to `JsonExpressionsSuite` for the `en-US`, `ko-KR`, `ru-RU`, `de-DE` locales:
- Inferring decimal type using locale from JSON field values
- Converting JSON field values to specified decimal type using the locales.
Closes#23132 from MaxGekk/json-decimal-parsing-locale.
Lead-authored-by: Maxim Gekk <max.gekk@gmail.com>
Co-authored-by: Maxim Gekk <maxim.gekk@databricks.com>
Signed-off-by: Wenchen Fan <wenchen@databricks.com>
## What changes were proposed in this pull request?
Currently duplicated map keys are not handled consistently. For example, map look up respects the duplicated key appears first, `Dataset.collect` only keeps the duplicated key appears last, `MapKeys` returns duplicated keys, etc.
This PR proposes to remove duplicated map keys with last wins policy, to follow Java/Scala and Presto. It only applies to built-in functions, as users can create map with duplicated map keys via private APIs anyway.
updated functions: `CreateMap`, `MapFromArrays`, `MapFromEntries`, `StringToMap`, `MapConcat`, `TransformKeys`.
For other places:
1. data source v1 doesn't have this problem, as users need to provide a java/scala map, which can't have duplicated keys.
2. data source v2 may have this problem. I've added a note to `ArrayBasedMapData` to ask the caller to take care of duplicated keys. In the future we should enforce it in the stable data APIs for data source v2.
3. UDF doesn't have this problem, as users need to provide a java/scala map. Same as data source v1.
4. file format. I checked all of them and only parquet does not enforce it. For backward compatibility reasons I change nothing but leave a note saying that the behavior will be undefined if users write map with duplicated keys to parquet files. Maybe we can add a config and fail by default if parquet files have map with duplicated keys. This can be done in followup.
## How was this patch tested?
updated tests and new tests
Closes#23124 from cloud-fan/map.
Authored-by: Wenchen Fan <wenchen@databricks.com>
Signed-off-by: Wenchen Fan <wenchen@databricks.com>
## What changes were proposed in this pull request?
https://github.com/apache/spark/pull/22326 made a mistake that, not all python UDFs are unevaluable in join condition. Only python UDFs that refer to attributes from both join side are unevaluable.
This PR fixes this mistake.
## How was this patch tested?
a new test
Closes#23153 from cloud-fan/join.
Authored-by: Wenchen Fan <wenchen@databricks.com>
Signed-off-by: Wenchen Fan <wenchen@databricks.com>
## What changes were proposed in this pull request?
a followup of https://github.com/apache/spark/pull/23043 . Add a test to show the minor behavior change introduced by #23043 , and add migration guide.
## How was this patch tested?
a new test
Closes#23141 from cloud-fan/follow.
Authored-by: Wenchen Fan <wenchen@databricks.com>
Signed-off-by: Wenchen Fan <wenchen@databricks.com>
## What changes were proposed in this pull request?
Implement codegen for `LocalTableScanExec` and `ExistingRDDExec`. Refactor to share code between `LocalTableScanExec`, `ExistingRDDExec`, `InputAdapter` and `RowDataSourceScanExec`.
The difference in `doProduce` between these four was that `ExistingRDDExec` and `RowDataSourceScanExec` triggered adding an `UnsafeProjection`, while `InputAdapter` and `LocalTableScanExec` did not.
In the new trait `InputRDDCodegen` I added a flag `createUnsafeProjection` which the operators set accordingly.
Note: `LocalTableScanExec` explicitly creates its input as `UnsafeRows`, so it was obvious why it doesn't need an `UnsafeProjection`. But if an `InputAdapter` may take input that is `InternalRows` but not `UnsafeRows`, then I think it doesn't need an unsafe projection just because any other operator that is its parent would do that. That assumes that that any parent operator would always result in some `UnsafeProjection` being eventually added, and hence the output of the `WholeStageCodegen` unit would be `UnsafeRows`. If these assumptions hold, I think `createUnsafeProjection` could be set to `(parent == null)`.
Note: Do not codegen `LocalTableScanExec` when it's the only operator. `LocalTableScanExec` has optimized driver-only `executeCollect` and `executeTake` code paths that are used to return `Command` results without starting Spark Jobs. They can no longer be used if the `LocalTableScanExec` gets optimized.
## How was this patch tested?
Covered and used in existing tests.
Closes#23127 from juliuszsompolski/SPARK-26159.
Authored-by: Juliusz Sompolski <julek@databricks.com>
Signed-off-by: Wenchen Fan <wenchen@databricks.com>
## What changes were proposed in this pull request?
The purpose of this PR is supplement new test cases for a + 1,a + b and Rand in SetOperationSuite.
It comes from the comment of closed PR:#20541, thanks.
## How was this patch tested?
add new test cases
Closes#23138 from heary-cao/UnionPushTestCases.
Authored-by: caoxuewen <cao.xuewen@zte.com.cn>
Signed-off-by: Wenchen Fan <wenchen@databricks.com>