## What changes were proposed in this pull request?
This is follow-up of #21732. This patch inlines `isOptionType` method.
## How was this patch tested?
Existing tests.
Closes#23143 from viirya/SPARK-24762-followup.
Authored-by: Liang-Chi Hsieh <viirya@gmail.com>
Signed-off-by: Wenchen Fan <wenchen@databricks.com>
## What changes were proposed in this pull request?
This PR is to improve the code comments to document some common traits and traps about the expression.
## How was this patch tested?
N/A
Closes#23135 from gatorsmile/addcomments.
Authored-by: gatorsmile <gatorsmile@gmail.com>
Signed-off-by: Wenchen Fan <wenchen@databricks.com>
## What changes were proposed in this pull request?
Create a new suite DataFrameSetOperationsSuite for the test cases of DataFrame/Dataset's set operations.
Also, add test cases of NULL handling for Array Except and Array Intersect.
## How was this patch tested?
N/A
Closes#23137 from gatorsmile/setOpsTest.
Authored-by: gatorsmile <gatorsmile@gmail.com>
Signed-off-by: Wenchen Fan <wenchen@databricks.com>
## What changes were proposed in this pull request?
SparkSQL doesn't support to encode `Option[Product]` as a top-level row now, because in SparkSQL entire top-level row can't be null.
However for use cases like Aggregator, it is reasonable to use `Option[Product]` as buffer and output column types. Due to above limitation, we don't do it for now.
This patch proposes to encode `Option[Product]` at top-level as single struct column. So we can work around the issue that entire top-level row can't be null.
To summarize encoding of `Product` and `Option[Product]`.
For `Product`, 1. at root level, the schema is all fields are flatten it into multiple columns. The `Product ` can't be null, otherwise it throws an exception.
```scala
val df = Seq((1 -> "a"), (2 -> "b")).toDF()
df.printSchema()
root
|-- _1: integer (nullable = false)
|-- _2: string (nullable = true)
```
2. At non-root level, `Product` is a struct type column.
```scala
val df = Seq((1, (1 -> "a")), (2, (2 -> "b")), (3, null)).toDF()
df.printSchema()
root
|-- _1: integer (nullable = false)
|-- _2: struct (nullable = true)
| |-- _1: integer (nullable = false)
| |-- _2: string (nullable = true)
```
For `Option[Product]`, 1. it was not supported at root level. After this change, it is a struct type column.
```scala
val df = Seq(Some(1 -> "a"), Some(2 -> "b"), None).toDF()
df.printSchema
root
|-- value: struct (nullable = true)
| |-- _1: integer (nullable = false)
| |-- _2: string (nullable = true)
```
2. At non-root level, it is also a struct type column.
```scala
val df = Seq((1, Some(1 -> "a")), (2, Some(2 -> "b")), (3, None)).toDF()
df.printSchema
root
|-- _1: integer (nullable = false)
|-- _2: struct (nullable = true)
| |-- _1: integer (nullable = false)
| |-- _2: string (nullable = true)
```
3. For use case like Aggregator, it was not supported too. After this change, we support to use `Option[Product]` as buffer/output column type.
```scala
val df = Seq(
OptionBooleanIntData("bob", Some((true, 1))),
OptionBooleanIntData("bob", Some((false, 2))),
OptionBooleanIntData("bob", None)).toDF()
val group = df
.groupBy("name")
.agg(OptionBooleanIntAggregator("isGood").toColumn.alias("isGood"))
group.printSchema
root
|-- name: string (nullable = true)
|-- isGood: struct (nullable = true)
| |-- _1: boolean (nullable = false)
| |-- _2: integer (nullable = false)
```
The buffer and output type of `OptionBooleanIntAggregator` is both `Option[(Boolean, Int)`.
## How was this patch tested?
Added test.
Closes#21732 from viirya/SPARK-24762.
Authored-by: Liang-Chi Hsieh <viirya@gmail.com>
Signed-off-by: Wenchen Fan <wenchen@databricks.com>
## What changes were proposed in this pull request?
The DOI foundation recommends [this new resolver](https://www.doi.org/doi_handbook/3_Resolution.html#3.8). Accordingly, this PR re`sed`s all static DOI links ;-)
## How was this patch tested?
It wasn't, since it seems as safe as a "[typo fix](https://spark.apache.org/contributing.html)".
In case any of the files is included from other projects, and should be updated there, please let me know.
Closes#23129 from katrinleinweber/resolve-DOIs-securely.
Authored-by: Katrin Leinweber <9948149+katrinleinweber@users.noreply.github.com>
Signed-off-by: Sean Owen <sean.owen@databricks.com>
## What changes were proposed in this pull request?
Fix Decimal `toScalaBigInt` and `toJavaBigInteger` used to only work for decimals not fitting long.
## How was this patch tested?
Added test to DecimalSuite.
Closes#23022 from juliuszsompolski/SPARK-26038.
Authored-by: Juliusz Sompolski <julek@databricks.com>
Signed-off-by: Herman van Hovell <hvanhovell@databricks.com>
## What changes were proposed in this pull request?
In the PR, I propose new options for CSV datasource - `lineSep` similar to Text and JSON datasource. The option allows to specify custom line separator of maximum length of 2 characters (because of a restriction in `uniVocity` parser). New option can be used in reading and writing CSV files.
## How was this patch tested?
Added a few tests with custom `lineSep` for enabled/disabled `multiLine` in read as well as tests in write. Also I added roundtrip tests.
Closes#23080 from MaxGekk/csv-line-sep.
Lead-authored-by: Maxim Gekk <max.gekk@gmail.com>
Co-authored-by: Maxim Gekk <maxim.gekk@databricks.com>
Signed-off-by: hyukjinkwon <gurwls223@apache.org>
## What changes were proposed in this pull request?
the pr #20014 which introduced `SparkOutOfMemoryError` to avoid killing the entire executor when an `OutOfMemoryError `is thrown.
so apply for memory using `MemoryConsumer. allocatePage `when catch exception, use `SparkOutOfMemoryError `instead of `OutOfMemoryError`
## How was this patch tested?
N / A
Closes#23084 from heary-cao/SparkOutOfMemoryError.
Authored-by: caoxuewen <cao.xuewen@zte.com.cn>
Signed-off-by: Wenchen Fan <wenchen@databricks.com>
GROUP BY treats -0.0 and 0.0 as different values which is unlike hive's behavior.
In addition current behavior with codegen is unpredictable (see example in JIRA ticket).
## What changes were proposed in this pull request?
In Platform.putDouble/Float() checking if the value is -0.0, and if so replacing with 0.0.
This is used by UnsafeRow so it won't have -0.0 values.
## How was this patch tested?
Added tests
Closes#23043 from adoron/adoron-spark-26021-replace-minus-zero-with-zero.
Authored-by: Alon Doron <adoron@palantir.com>
Signed-off-by: Wenchen Fan <wenchen@databricks.com>
## What changes were proposed in this pull request?
This is an addendum patch for SPARK-26129 that defines the edge case behavior for QueryPlanningTracker.topRulesByTime.
## How was this patch tested?
Added unit tests for each behavior.
Closes#23110 from rxin/SPARK-26129-1.
Authored-by: Reynold Xin <rxin@databricks.com>
Signed-off-by: Dongjoon Hyun <dongjoon@apache.org>
## What changes were proposed in this pull request?
The corrupt column specified via JSON/CSV option *columnNameOfCorruptRecord* must have the `string` type and be `nullable`. This has been already checked in `DataFrameReader`.`csv`/`json` and in `Json`/`CsvFileFormat` but not in `from_json`/`from_csv`. The PR adds such checks inside functions as well.
## How was this patch tested?
Added tests to `Json`/`CsvExpressionSuite` for checking type of the corrupt column. They don't check the `nullable` property because `schema` is forcibly casted to nullable.
Closes#23070 from MaxGekk/verify-corrupt-column-csv-json.
Authored-by: Maxim Gekk <max.gekk@gmail.com>
Signed-off-by: hyukjinkwon <gurwls223@apache.org>
## What changes were proposed in this pull request?
When doing typed aggregation on a Dataset, for struct key type, the key attribute is named as "key". But for non-struct type, the key attribute is named as "value". This key attribute should also be named as "key" for non-struct type.
## How was this patch tested?
Added test.
Closes#23054 from viirya/SPARK-26085.
Authored-by: Liang-Chi Hsieh <viirya@gmail.com>
Signed-off-by: Wenchen Fan <wenchen@databricks.com>
## What changes were proposed in this pull request?
An input without valid JSON tokens on the root level will be treated as a bad record, and handled according to `mode`. Previously such input was converted to `null`. After the changes, the input is converted to a row with `null`s in the `PERMISSIVE` mode according the schema. This allows to remove a code in the `from_json` function which can produce `null` as result rows.
## How was this patch tested?
It was tested by existing test suites. Some of them I have to modify (`JsonSuite` for example) because previously bad input was just silently ignored. For now such input is handled according to specified `mode`.
Closes#22938 from MaxGekk/json-nulls.
Lead-authored-by: Maxim Gekk <max.gekk@gmail.com>
Co-authored-by: Maxim Gekk <maxim.gekk@databricks.com>
Signed-off-by: Wenchen Fan <wenchen@databricks.com>
## What changes were proposed in this pull request?
In the PR, I propose:
- new SQL config `spark.sql.debug.maxToStringFields` to control maximum number fields up to which `truncatedString` cuts its input sequences.
- Moving `truncatedString` out of `core` to `sql/catalyst` because it is used only in the `sql/catalyst` packages for restricting number of fields converted to strings from `TreeNode` and expressions of`StructType`.
## How was this patch tested?
Added a test to `QueryExecutionSuite` to check that `spark.sql.debug.maxToStringFields` impacts to behavior of `truncatedString`.
Closes#23039 from MaxGekk/truncated-string-catalyst.
Lead-authored-by: Maxim Gekk <maxim.gekk@databricks.com>
Co-authored-by: Maxim Gekk <max.gekk@gmail.com>
Signed-off-by: Dongjoon Hyun <dongjoon@apache.org>
## What changes were proposed in this pull request?
We currently don't have good visibility into query planning time (analysis vs optimization vs physical planning). This patch adds a simple utility to track the runtime of various rules and various planning phases.
## How was this patch tested?
Added unit tests and end-to-end integration tests.
Closes#23096 from rxin/SPARK-26129.
Authored-by: Reynold Xin <rxin@databricks.com>
Signed-off-by: Reynold Xin <rxin@databricks.com>
## What changes were proposed in this pull request?
This change fixes a particular scenario where default spark SQL can't encode (thrift) types that are generated by twitter scrooge. These types are a trait that extends `scala.ProductX` with a constructor defined only in a companion object, rather than a actual case class. The actual case class used is child class, but that type is almost never referred to in code. The type has no corresponding constructor symbol and causes an exception. For all other purposes, these classes act just like case classes, so it is unfortunate that spark SQL can't serialize them nicely as it can actual case classes. For an full example of a scrooge codegen class, see https://gist.github.com/anonymous/ba13d4b612396ca72725eaa989900314.
This change catches the case where the type has no constructor but does have an `apply` method on the type's companion object. This allows for thrift types to be serialized/deserialized with implicit encoders the same way as normal case classes. This fix had to be done in three places where the constructor is assumed to be an actual constructor:
1) In serializing, determining the schema for the dataframe relies on inspecting its constructor (`ScalaReflection.constructParams`). Here we fall back to using the companion constructor arguments.
2) In deserializing or evaluating, in the java codegen ( `NewInstance.doGenCode`), the type couldn't be constructed with the new keyword. If there is no constructor, we change the constructor call to try the companion constructor.
3) In deserializing or evaluating, without codegen, the constructor is directly invoked (`NewInstance.constructor`). This was fixed with scala reflection to get the actual companion apply method.
The return type of `findConstructor` was changed because the companion apply method constructor can't be represented as a `java.lang.reflect.Constructor`.
There might be situations in which this approach would also fail in a new way, but it does at a minimum work for the specific scrooge example and will not impact cases that were already succeeding prior to this change
Note: this fix does not enable using scrooge thrift enums, additional work for this is necessary. With this patch, it seems like you could patch `com.twitter.scrooge.ThriftEnum` to extend `_root_.scala.Product1[Int]` with `def _1 = value` to get spark's implicit encoders to handle enums, but I've yet to use this method myself.
Note: I previously opened a PR for this issue, but only was able to fix case 1) there: https://github.com/apache/spark/pull/18766
## How was this patch tested?
I've fixed all 3 cases and added two tests that use a case class that is similar to scrooge generated one. The test in ScalaReflectionSuite checks 1), and the additional asserting in ObjectExpressionsSuite checks 2) and 3).
Closes#23062 from drewrobb/SPARK-8288.
Authored-by: Drew Robb <drewrobb@gmail.com>
Signed-off-by: Sean Owen <sean.owen@databricks.com>
## What changes were proposed in this pull request?
In the PR, I propose to pass the CSV option `encoding`/`charset` to `uniVocity` parser to allow parsing CSV files in different encodings when `multiLine` is enabled. The value of the option is passed to the `beginParsing` method of `CSVParser`.
## How was this patch tested?
Added new test to `CSVSuite` for different encodings and enabled/disabled header.
Closes#23091 from MaxGekk/csv-miltiline-encoding.
Authored-by: Maxim Gekk <maxim.gekk@databricks.com>
Signed-off-by: hyukjinkwon <gurwls223@apache.org>
## What changes were proposed in this pull request?
This PR fixes an exception in `AggregateExpression.references` called on unresolved expressions. It implements the solution proposed in [SPARK-26084](https://issues.apache.org/jira/browse/SPARK-26084), a minor refactoring that removes the unnecessary dependence on `AttributeSet.toSeq`, which requires expression IDs and, therefore, can only execute successfully for resolved expressions.
The refactored implementation is both simpler and faster, eliminating the conversion of a `Set` to a
`Seq` and back to `Set`.
## How was this patch tested?
Added a new test based on the failing case in [SPARK-26084](https://issues.apache.org/jira/browse/SPARK-26084).
hvanhovell
Closes#23075 from ssimeonov/ss_SPARK-26084.
Authored-by: Simeon Simeonov <sim@fastignite.com>
Signed-off-by: Herman van Hovell <hvanhovell@databricks.com>
## What changes were proposed in this pull request?
Extend the `ReplaceNullWithFalse` optimizer rule introduced in SPARK-25860 (https://github.com/apache/spark/pull/22857) to also support optimizing predicates in higher-order functions of `ArrayExists`, `ArrayFilter`, `MapFilter`.
Also rename the rule to `ReplaceNullWithFalseInPredicate` to better reflect its intent.
Example:
```sql
select filter(a, e -> if(e is null, null, true)) as b from (
select array(null, 1, null, 3) as a)
```
The optimized logical plan:
**Before**:
```
== Optimized Logical Plan ==
Project [filter([null,1,null,3], lambdafunction(if (isnull(lambda e#13)) null else true, lambda e#13, false)) AS b#9]
+- OneRowRelation
```
**After**:
```
== Optimized Logical Plan ==
Project [filter([null,1,null,3], lambdafunction(if (isnull(lambda e#13)) false else true, lambda e#13, false)) AS b#9]
+- OneRowRelation
```
## How was this patch tested?
Added new unit test cases to the `ReplaceNullWithFalseInPredicateSuite` (renamed from `ReplaceNullWithFalseSuite`).
Closes#23079 from rednaxelafx/catalyst-master.
Authored-by: Kris Mok <kris.mok@databricks.com>
Signed-off-by: Wenchen Fan <wenchen@databricks.com>
## What changes were proposed in this pull request?
The build has a lot of deprecation warnings. Some are new in Scala 2.12 and Java 11. We've fixed some, but I wanted to take a pass at fixing lots of easy miscellaneous ones here.
They're too numerous and small to list here; see the pull request. Some highlights:
- `BeanInfo` is deprecated in 2.12, and BeanInfo classes are pretty ancient in Java. Instead, case classes can explicitly declare getters
- Eta expansion of zero-arg methods; foo() becomes () => foo() in many cases
- Floating-point Range is inexact and deprecated, like 0.0 to 100.0 by 1.0
- finalize() is finally deprecated (just needs to be suppressed)
- StageInfo.attempId was deprecated and easiest to remove here
I'm not now going to touch some chunks of deprecation warnings:
- Parquet deprecations
- Hive deprecations (particularly serde2 classes)
- Deprecations in generated code (mostly Thriftserver CLI)
- ProcessingTime deprecations (we may need to revive this class as internal)
- many MLlib deprecations because they concern methods that may be removed anyway
- a few Kinesis deprecations I couldn't figure out
- Mesos get/setRole, which I don't know well
- Kafka/ZK deprecations (e.g. poll())
- Kinesis
- a few other ones that will probably resolve by deleting a deprecated method
## How was this patch tested?
Existing tests, including manual testing with the 2.11 build and Java 11.
Closes#23065 from srowen/SPARK-26090.
Authored-by: Sean Owen <sean.owen@databricks.com>
Signed-off-by: Sean Owen <sean.owen@databricks.com>
## What changes were proposed in this pull request?
Due to implementation limitation, currently Spark can't compare or do equality check between map types. As a result, map values can't appear in EQUAL or comparison expressions, can't be grouping key, etc.
The more important thing is, map loop up needs to do equality check of the map key, and thus can't support map as map key when looking up values from a map. Thus it's not useful to have map as map key.
This PR proposes to stop users from creating maps using map type as key. The list of expressions that are updated: `CreateMap`, `MapFromArrays`, `MapFromEntries`, `MapConcat`, `TransformKeys`. I manually checked all the places that create `MapType`, and came up with this list.
Note that, maps with map type key still exist, via reading from parquet files, converting from scala/java map, etc. This PR is not to completely forbid map as map key, but to avoid creating it by Spark itself.
Motivation: when I was trying to fix the duplicate key problem, I found it's impossible to do it with map type map key. I think it's reasonable to avoid map type map key for builtin functions.
## How was this patch tested?
updated test
Closes#23045 from cloud-fan/map-key.
Authored-by: Wenchen Fan <wenchen@databricks.com>
Signed-off-by: Wenchen Fan <wenchen@databricks.com>
## What changes were proposed in this pull request?
The following 5 functions were removed from branch-2.4:
- map_entries
- map_filter
- transform_values
- transform_keys
- map_zip_with
We should update the since version to 3.0.0.
## How was this patch tested?
Existing tests.
Closes#23082 from ueshin/issues/SPARK-26112/since.
Authored-by: Takuya UESHIN <ueshin@databricks.com>
Signed-off-by: Wenchen Fan <wenchen@databricks.com>
## What changes were proposed in this pull request?
This restores scaladoc artifact generation, which got dropped with the Scala 2.12 update. The change looks large, but is almost all due to needing to make the InterfaceStability annotations top-level classes (i.e. `InterfaceStability.Stable` -> `Stable`), unfortunately. A few inner class references had to be qualified too.
Lots of scaladoc warnings now reappear. We can choose to disable generation by default and enable for releases, later.
## How was this patch tested?
N/A; build runs scaladoc now.
Closes#23069 from srowen/SPARK-26026.
Authored-by: Sean Owen <sean.owen@databricks.com>
Signed-off-by: Sean Owen <sean.owen@databricks.com>
## What changes were proposed in this pull request?
remove invalid comment as we don't use it anymore
More details: https://github.com/apache/spark/pull/22976#discussion_r233764857
## How was this patch tested?
N/A
Closes#23044 from heary-cao/followUpOrdering.
Authored-by: caoxuewen <cao.xuewen@zte.com.cn>
Signed-off-by: Wenchen Fan <wenchen@databricks.com>
## What changes were proposed in this pull request?
In SPARK-24865 `AnalysisBarrier` was removed and in order to improve resolution speed, the `analyzed` flag was (re-)introduced in order to process only plans which are not yet analyzed. This should not be the case when performing attribute deduplication as in that case we need to transform also the plans which were already analyzed, otherwise we can miss to rewrite some attributes leading to invalid plans.
## How was this patch tested?
added UT
Please review http://spark.apache.org/contributing.html before opening a pull request.
Closes#23035 from mgaido91/SPARK-26057.
Authored-by: Marco Gaido <marcogaido91@gmail.com>
Signed-off-by: Wenchen Fan <wenchen@databricks.com>
## What changes were proposed in this pull request?
This PR makes Spark's default Scala version as 2.12, and Scala 2.11 will be the alternative version. This implies that Scala 2.12 will be used by our CI builds including pull request builds.
We'll update the Jenkins to include a new compile-only jobs for Scala 2.11 to ensure the code can be still compiled with Scala 2.11.
## How was this patch tested?
existing tests
Closes#22967 from dbtsai/scala2.12.
Authored-by: DB Tsai <d_tsai@apple.com>
Signed-off-by: Dongjoon Hyun <dongjoon@apache.org>
## What changes were proposed in this pull request?
`Dataset.groupByKey` will bring in new attributes from serializer. If key type is the same as original Dataset's object type, they have same serializer output and so the attribute names will conflict.
This won't be a problem at most of cases, if we don't refer conflict attributes:
```scala
val ds: Dataset[(ClassData, Long)] = Seq(ClassData("one", 1), ClassData("two", 2)).toDS()
.map(c => ClassData(c.a, c.b + 1))
.groupByKey(p => p).count()
```
But if we use conflict attributes, `Analyzer` will complain about ambiguous references:
```scala
val ds = Seq(1, 2, 3).toDS()
val agg = ds.groupByKey(_ >= 2).agg(sum("value").as[Long], sum($"value" + 1).as[Long])
```
We have discussed two fixes https://github.com/apache/spark/pull/22944#discussion_r230977212:
1. Implicitly add alias to key attribute:
Works for primitive type. But for product type, we can't implicitly add aliases to key attributes because we might need to access key attributes by names in methods like `mapGroups`.
2. Detect conflict from key attributes and warn users to add alias manually
This might work, but needs to add some hacks to Analyzer or AttributeSeq.resolve.
This patch applies another simpler fix. We resolve aggregate expressions with `AppendColumns`'s children, instead of `AppendColumns`. `AppendColumns`'s output contains its children's output and serializer output, aggregate expressions shouldn't use serializer output.
## How was this patch tested?
Added test.
Closes#22944 from viirya/dataset_agg.
Authored-by: Liang-Chi Hsieh <viirya@gmail.com>
Signed-off-by: Wenchen Fan <wenchen@databricks.com>
## What changes were proposed in this pull request?
In the PR, I propose new method for debugging queries by dumping info about their execution to a file. It saves logical, optimized and physical plan similar to the `explain()` method + generated code. One of the advantages of the method over `explain` is it does not materializes full output as one string in memory which can cause OOMs.
## How was this patch tested?
Added a few tests to `QueryExecutionSuite` to check positive and negative scenarios.
Closes#23018 from MaxGekk/truncated-plan-to-file.
Authored-by: Maxim Gekk <maxim.gekk@databricks.com>
Signed-off-by: Herman van Hovell <hvanhovell@databricks.com>
## What changes were proposed in this pull request?
Passing current value of SQL config `spark.sql.columnNameOfCorruptRecord` to `CSVOptions` inside of `DataFrameReader`.`csv()`.
## How was this patch tested?
Added a test where default value of `spark.sql.columnNameOfCorruptRecord` is changed.
Closes#23006 from MaxGekk/csv-corrupt-sql-config.
Lead-authored-by: Maxim Gekk <maxim.gekk@databricks.com>
Co-authored-by: Dongjoon Hyun <dongjoon@apache.org>
Co-authored-by: Maxim Gekk <max.gekk@gmail.com>
Signed-off-by: hyukjinkwon <gurwls223@apache.org>
## What changes were proposed in this pull request?
As comment in https://github.com/apache/spark/pull/22326#issuecomment-424923967, we test the new added optimizer rule by end-to-end test in python side, need to add suites under `org.apache.spark.sql.catalyst.optimizer` like other optimizer rules.
## How was this patch tested?
new added UT
Closes#22955 from xuanyuanking/SPARK-25949.
Authored-by: Yuanjian Li <xyliyuanjian@gmail.com>
Signed-off-by: Wenchen Fan <wenchen@databricks.com>
## What changes were proposed in this pull request?
Deprecated in Java 11, replace Class.newInstance with Class.getConstructor.getInstance, and primtive wrapper class constructors with valueOf or equivalent
## How was this patch tested?
Existing tests.
Closes#22988 from srowen/SPARK-25984.
Authored-by: Sean Owen <sean.owen@databricks.com>
Signed-off-by: Sean Owen <sean.owen@databricks.com>
## What changes were proposed in this pull request?
Very minor parser bug, but possibly problematic for code-generated queries:
Consider the following two queries:
```
SELECT avg(k) OVER (w) FROM kv WINDOW w AS (PARTITION BY v ORDER BY w) ORDER BY 1
```
and
```
SELECT avg(k) OVER w FROM kv WINDOW w AS (PARTITION BY v ORDER BY w) ORDER BY 1
```
The former, with parens around the OVER condition, fails to parse while the latter, without parens, succeeds:
```
Error in SQL statement: ParseException:
mismatched input '(' expecting {<EOF>, ',', 'FROM', 'WHERE', 'GROUP', 'ORDER', 'HAVING', 'LIMIT', 'LATERAL', 'WINDOW', 'UNION', 'EXCEPT', 'MINUS', 'INTERSECT', 'SORT', 'CLUSTER', 'DISTRIBUTE'}(line 1, pos 19)
== SQL ==
SELECT avg(k) OVER (w) FROM kv WINDOW w AS (PARTITION BY v ORDER BY w) ORDER BY 1
-------------------^^^
```
This was found when running the cockroach DB tests.
I tried PostgreSQL, The SQL with parentheses is also workable.
## How was this patch tested?
Unit test
Closes#22987 from gengliangwang/windowParentheses.
Authored-by: Gengliang Wang <gengliang.wang@databricks.com>
Signed-off-by: gatorsmile <gatorsmile@gmail.com>
## What changes were proposed in this pull request?
When the queries do not use the column names with the same case, users might hit various errors. Below is a typical test failure they can hit.
```
Expected only partition pruning predicates: ArrayBuffer(isnotnull(tdate#237), (cast(tdate#237 as string) >= 2017-08-15));
org.apache.spark.sql.AnalysisException: Expected only partition pruning predicates: ArrayBuffer(isnotnull(tdate#237), (cast(tdate#237 as string) >= 2017-08-15));
at org.apache.spark.sql.catalyst.catalog.ExternalCatalogUtils$.prunePartitionsByFilter(ExternalCatalogUtils.scala:146)
at org.apache.spark.sql.catalyst.catalog.InMemoryCatalog.listPartitionsByFilter(InMemoryCatalog.scala:560)
at org.apache.spark.sql.catalyst.catalog.SessionCatalog.listPartitionsByFilter(SessionCatalog.scala:925)
```
## How was this patch tested?
Added two test cases.
Closes#22990 from gatorsmile/fix1283.
Authored-by: gatorsmile <gatorsmile@gmail.com>
Signed-off-by: gatorsmile <gatorsmile@gmail.com>
## What changes were proposed in this pull request?
In the PR, I propose to add new option `locale` into CSVOptions/JSONOptions to make parsing date/timestamps in local languages possible. Currently the locale is hard coded to `Locale.US`.
## How was this patch tested?
Added two tests for parsing a date from CSV/JSON - `ноя 2018`.
Closes#22951 from MaxGekk/locale.
Authored-by: Maxim Gekk <max.gekk@gmail.com>
Signed-off-by: hyukjinkwon <gurwls223@apache.org>
## What changes were proposed in this pull request?
The PR fixes an issue when the corrupt record column specified via `spark.sql.columnNameOfCorruptRecord` or JSON options `columnNameOfCorruptRecord` is propagated to JacksonParser, and returned row breaks an assumption in `FailureSafeParser` that the row must contain only actual data. The issue is fixed by passing actual schema without the corrupt record field into `JacksonParser`.
## How was this patch tested?
Added a test with the corrupt record column in the middle of user's schema.
Closes#22958 from MaxGekk/from_json-corrupt-record-schema.
Authored-by: Maxim Gekk <max.gekk@gmail.com>
Signed-off-by: hyukjinkwon <gurwls223@apache.org>
## What changes were proposed in this pull request?
- Remove some AccumulableInfo .apply() methods
- Remove non-label-specific multiclass precision/recall/fScore in favor of accuracy
- Remove toDegrees/toRadians in favor of degrees/radians (SparkR: only deprecated)
- Remove approxCountDistinct in favor of approx_count_distinct (SparkR: only deprecated)
- Remove unused Python StorageLevel constants
- Remove Dataset unionAll in favor of union
- Remove unused multiclass option in libsvm parsing
- Remove references to deprecated spark configs like spark.yarn.am.port
- Remove TaskContext.isRunningLocally
- Remove ShuffleMetrics.shuffle* methods
- Remove BaseReadWrite.context in favor of session
- Remove Column.!== in favor of =!=
- Remove Dataset.explode
- Remove Dataset.registerTempTable
- Remove SQLContext.getOrCreate, setActive, clearActive, constructors
Not touched yet
- everything else in MLLib
- HiveContext
- Anything deprecated more recently than 2.0.0, generally
## How was this patch tested?
Existing tests
Closes#22921 from srowen/SPARK-25908.
Lead-authored-by: Sean Owen <sean.owen@databricks.com>
Co-authored-by: hyukjinkwon <gurwls223@apache.org>
Co-authored-by: Sean Owen <srowen@gmail.com>
Signed-off-by: Sean Owen <sean.owen@databricks.com>
JVMs can't allocate arrays of length exactly Int.MaxValue, so ensure we never try to allocate an array that big. This commit changes some defaults & configs to gracefully fallover to something that doesn't require one large array in some cases; in other cases it simply improves an error message for cases which will still fail.
Closes#22818 from squito/SPARK-25827.
Authored-by: Imran Rashid <irashid@cloudera.com>
Signed-off-by: Imran Rashid <irashid@cloudera.com>
## What changes were proposed in this pull request?
Fix for `CsvToStructs` to take into account SQL config `spark.sql.columnNameOfCorruptRecord` similar to `from_json`.
## How was this patch tested?
Added new test where `spark.sql.columnNameOfCorruptRecord` is set to corrupt column name different from default.
Closes#22956 from MaxGekk/csv-tests.
Authored-by: Maxim Gekk <max.gekk@gmail.com>
Signed-off-by: hyukjinkwon <gurwls223@apache.org>
## What changes were proposed in this pull request?
As per the discussion in [#22823](https://github.com/apache/spark/pull/22823/files#r228400706), add a new configuration to make the split threshold for the code generated function configurable.
When the generated Java function source code exceeds `spark.sql.codegen.methodSplitThreshold`, it will be split into multiple small functions.
## How was this patch tested?
manual tests
Closes#22847 from yucai/splitThreshold.
Authored-by: yucai <yyu1@ebay.com>
Signed-off-by: Wenchen Fan <wenchen@databricks.com>
## What changes were proposed in this pull request?
New functions takes a struct and converts it to a CSV strings using passed CSV options. It accepts the same CSV options as CSV data source does.
## How was this patch tested?
Added `CsvExpressionsSuite`, `CsvFunctionsSuite` as well as R, Python and SQL tests similar to tests for `to_json()`
Closes#22626 from MaxGekk/to_csv.
Lead-authored-by: Maxim Gekk <max.gekk@gmail.com>
Co-authored-by: Maxim Gekk <maxim.gekk@databricks.com>
Signed-off-by: hyukjinkwon <gurwls223@apache.org>
## What changes were proposed in this pull request?
a followup of https://github.com/apache/spark/pull/22749.
When we construct the new serializer in `ExpressionEncoder.tuple`, we don't need to add `if(isnull ...)` check for each field. They are either simple expressions that can propagate null correctly(e.g. `GetStructField(GetColumnByOrdinal(0, schema), index)`), or complex expression that already have the isnull check.
## How was this patch tested?
existing tests
Closes#22898 from cloud-fan/minor.
Authored-by: Wenchen Fan <wenchen@databricks.com>
Signed-off-by: Wenchen Fan <wenchen@databricks.com>
## What changes were proposed in this pull request?
In the PR, I propose to add new function - *schema_of_csv()* which infers schema of CSV string literal. The result of the function is a string containing a schema in DDL format. For example:
```sql
select schema_of_csv('1|abc', map('delimiter', '|'))
```
```
struct<_c0:int,_c1:string>
```
## How was this patch tested?
Added new tests to `CsvFunctionsSuite`, `CsvExpressionsSuite` and SQL tests to `csv-functions.sql`
Closes#22666 from MaxGekk/schema_of_csv-function.
Lead-authored-by: hyukjinkwon <gurwls223@apache.org>
Co-authored-by: Maxim Gekk <maxim.gekk@databricks.com>
Signed-off-by: hyukjinkwon <gurwls223@apache.org>
## What changes were proposed in this pull request?
This PR proposes a new optimization rule that replaces `Literal(null, _)` with `FalseLiteral` in conditions in `Join` and `Filter`, predicates in `If`, conditions in `CaseWhen`.
The idea is that some expressions evaluate to `false` if the underlying expression is `null` (as an example see `GeneratePredicate$create` or `doGenCode` and `eval` methods in `If` and `CaseWhen`). Therefore, we can replace `Literal(null, _)` with `FalseLiteral`, which can lead to more optimizations later on.
Let’s consider a few examples.
```
val df = spark.range(1, 100).select($"id".as("l"), ($"id" > 50).as("b"))
df.createOrReplaceTempView("t")
df.createOrReplaceTempView("p")
```
**Case 1**
```
spark.sql("SELECT * FROM t WHERE if(l > 10, false, NULL)").explain(true)
// without the new rule
…
== Optimized Logical Plan ==
Project [id#0L AS l#2L, cast(id#0L as string) AS s#3]
+- Filter if ((id#0L > 10)) false else null
+- Range (1, 100, step=1, splits=Some(12))
== Physical Plan ==
*(1) Project [id#0L AS l#2L, cast(id#0L as string) AS s#3]
+- *(1) Filter if ((id#0L > 10)) false else null
+- *(1) Range (1, 100, step=1, splits=12)
// with the new rule
…
== Optimized Logical Plan ==
LocalRelation <empty>, [l#2L, s#3]
== Physical Plan ==
LocalTableScan <empty>, [l#2L, s#3]
```
**Case 2**
```
spark.sql("SELECT * FROM t WHERE CASE WHEN l < 10 THEN null WHEN l > 40 THEN false ELSE null END”).explain(true)
// without the new rule
...
== Optimized Logical Plan ==
Project [id#0L AS l#2L, cast(id#0L as string) AS s#3]
+- Filter CASE WHEN (id#0L < 10) THEN null WHEN (id#0L > 40) THEN false ELSE null END
+- Range (1, 100, step=1, splits=Some(12))
== Physical Plan ==
*(1) Project [id#0L AS l#2L, cast(id#0L as string) AS s#3]
+- *(1) Filter CASE WHEN (id#0L < 10) THEN null WHEN (id#0L > 40) THEN false ELSE null END
+- *(1) Range (1, 100, step=1, splits=12)
// with the new rule
...
== Optimized Logical Plan ==
LocalRelation <empty>, [l#2L, s#3]
== Physical Plan ==
LocalTableScan <empty>, [l#2L, s#3]
```
**Case 3**
```
spark.sql("SELECT * FROM t JOIN p ON IF(t.l > p.l, null, false)").explain(true)
// without the new rule
...
== Optimized Logical Plan ==
Join Inner, if ((l#2L > l#37L)) null else false
:- Project [id#0L AS l#2L, cast(id#0L as string) AS s#3]
: +- Range (1, 100, step=1, splits=Some(12))
+- Project [id#0L AS l#37L, cast(id#0L as string) AS s#38]
+- Range (1, 100, step=1, splits=Some(12))
== Physical Plan ==
BroadcastNestedLoopJoin BuildRight, Inner, if ((l#2L > l#37L)) null else false
:- *(1) Project [id#0L AS l#2L, cast(id#0L as string) AS s#3]
: +- *(1) Range (1, 100, step=1, splits=12)
+- BroadcastExchange IdentityBroadcastMode
+- *(2) Project [id#0L AS l#37L, cast(id#0L as string) AS s#38]
+- *(2) Range (1, 100, step=1, splits=12)
// with the new rule
...
== Optimized Logical Plan ==
LocalRelation <empty>, [l#2L, s#3, l#37L, s#38]
```
## How was this patch tested?
This PR comes with a set of dedicated tests.
Closes#22857 from aokolnychyi/spark-25860.
Authored-by: Anton Okolnychyi <aokolnychyi@apple.com>
Signed-off-by: DB Tsai <d_tsai@apple.com>
## What changes were proposed in this pull request?
Currently in `FailureSafeParser` and `from_avro`, the exception is created with such code
```
throw new SparkException("Malformed records are detected in record parsing. " +
s"Parse Mode: ${FailFastMode.name}.", e.cause)
```
1. The cause part should be `e` instead of `e.cause`
2. If `e` contains non-null message, it should be shown in `from_json`/`from_csv`/`from_avro`, e.g.
```
com.fasterxml.jackson.core.JsonParseException: Unexpected character ('1' (code 49)): was expecting a colon to separate field name and value
at [Source: (InputStreamReader); line: 1, column: 7]
```
3.Kindly show hint for trying PERMISSIVE in error message.
## How was this patch tested?
Unit test.
Closes#22895 from gengliangwang/improve_error_msg.
Authored-by: Gengliang Wang <gengliang.wang@databricks.com>
Signed-off-by: hyukjinkwon <gurwls223@apache.org>
## What changes were proposed in this pull request?
This patch removes the rangeBetween functions introduced in SPARK-21608. As explained in SPARK-25841, these functions are confusing and don't quite work. We will redesign them and introduce better ones in SPARK-25843.
## How was this patch tested?
Removed relevant test cases as well. These test cases will need to be added back in SPARK-25843.
Closes#22870 from rxin/SPARK-25862.
Lead-authored-by: Reynold Xin <rxin@databricks.com>
Co-authored-by: hyukjinkwon <gurwls223@apache.org>
Signed-off-by: gatorsmile <gatorsmile@gmail.com>
## What changes were proposed in this pull request?
When we compare attributes, in general, we should always refer to semantic equality, as the default `equal` method can return false when there are "cosmetic" differences between them, but still they are the same thing; at least we have to consider them so when analyzing/optimizing queries.
The PR focuses on the usage and comparison of the `output` of a `LogicalPlan`, which is a `Seq[Attribute]` in `AliasViewChild`. In this case, using equality implicitly fails to check the semantic equality. This results in the operator failing to stabilize.
## How was this patch tested?
running the tests with the patch provided by maryannxue in https://github.com/apache/spark/pull/22060Closes#22713 from mgaido91/SPARK-25691.
Authored-by: Marco Gaido <marcogaido91@gmail.com>
Signed-off-by: Wenchen Fan <wenchen@databricks.com>
## What changes were proposed in this pull request?
This PR proposes to avoid hardcorded configuration keys in SQLConf's `doc.
## How was this patch tested?
Manually verified.
Closes#22877 from HyukjinKwon/minor-conf-name.
Authored-by: hyukjinkwon <gurwls223@apache.org>
Signed-off-by: hyukjinkwon <gurwls223@apache.org>
## What changes were proposed in this pull request?
These two classes were added for regr_ expression support (SPARK-23907). These have been removed and hence we can remove these base classes and inline the logic in the concrete classes.
## How was this patch tested?
Existing tests.
Closes#22856 from dilipbiswal/average_cleanup.
Authored-by: Dilip Biswal <dbiswal@us.ibm.com>
Signed-off-by: Sean Owen <sean.owen@databricks.com>
## What changes were proposed in this pull request?
Set main args correctly in BenchmarkBase, to make it accessible for its subclass.
It will benefit:
- BuiltInDataSourceWriteBenchmark
- AvroWriteBenchmark
## How was this patch tested?
manual tests
Closes#22872 from yucai/main_args.
Authored-by: yucai <yyu1@ebay.com>
Signed-off-by: Wenchen Fan <wenchen@databricks.com>
## What changes were proposed in this pull request?
Extractors are made of 2 expressions, one of them defines the the value to be extract from (called `child`) and the other defines the way of extraction (called `extraction`). In this term extractors have 2 children so they shouldn't be `UnaryExpression`s.
`ResolveReferences` was changed in this commit: 36b826f5d1 which resulted a regression with nested extractors. An extractor need to define its children as the set of both `child` and `extraction`; and should try to resolve both in `ResolveReferences`.
This PR changes `UnresolvedExtractValue` to a `BinaryExpression`.
## How was this patch tested?
added UT
Closes#22817 from peter-toth/SPARK-25816.
Authored-by: Peter Toth <peter.toth@gmail.com>
Signed-off-by: gatorsmile <gatorsmile@gmail.com>
## What changes were proposed in this pull request?
WindowSpecDefinition checks start < last, but CalendarIntervalType is not comparable, so it would throw the following exception at runtime:
```
scala.MatchError: CalendarIntervalType (of class org.apache.spark.sql.types.CalendarIntervalType$) at
org.apache.spark.sql.catalyst.util.TypeUtils$.getInterpretedOrdering(TypeUtils.scala:58) at
org.apache.spark.sql.catalyst.expressions.BinaryComparison.ordering$lzycompute(predicates.scala:592) at
org.apache.spark.sql.catalyst.expressions.BinaryComparison.ordering(predicates.scala:592) at
org.apache.spark.sql.catalyst.expressions.GreaterThan.nullSafeEval(predicates.scala:797) at org.apache.spark.sql.catalyst.expressions.BinaryExpression.eval(Expression.scala:496) at org.apache.spark.sql.catalyst.expressions.SpecifiedWindowFrame.isGreaterThan(windowExpressions.scala:245) at
org.apache.spark.sql.catalyst.expressions.SpecifiedWindowFrame.checkInputDataTypes(windowExpressions.scala:216) at
org.apache.spark.sql.catalyst.expressions.Expression.resolved$lzycompute(Expression.scala:171) at
org.apache.spark.sql.catalyst.expressions.Expression.resolved(Expression.scala:171) at
org.apache.spark.sql.catalyst.expressions.Expression$$anonfun$childrenResolved$1.apply(Expression.scala:183) at
org.apache.spark.sql.catalyst.expressions.Expression$$anonfun$childrenResolved$1.apply(Expression.scala:183) at
scala.collection.IndexedSeqOptimized$class.prefixLengthImpl(IndexedSeqOptimized.scala:38) at scala.collection.IndexedSeqOptimized$class.forall(IndexedSeqOptimized.scala:43) at scala.collection.mutable.ArrayBuffer.forall(ArrayBuffer.scala:48) at
org.apache.spark.sql.catalyst.expressions.Expression.childrenResolved(Expression.scala:183) at
org.apache.spark.sql.catalyst.expressions.WindowSpecDefinition.resolved$lzycompute(windowExpressions.scala:48) at
org.apache.spark.sql.catalyst.expressions.WindowSpecDefinition.resolved(windowExpressions.scala:48) at
org.apache.spark.sql.catalyst.expressions.Expression$$anonfun$childrenResolved$1.apply(Expression.scala:183) at
org.apache.spark.sql.catalyst.expressions.Expression$$anonfun$childrenResolved$1.apply(Expression.scala:183) at
scala.collection.LinearSeqOptimized$class.forall(LinearSeqOptimized.scala:83)
```
We fix the issue by only perform the check on boundary expressions that are AtomicType.
## How was this patch tested?
Add new test case in `DataFrameWindowFramesSuite`
Closes#22853 from jiangxb1987/windowBoundary.
Authored-by: Xingbo Jiang <xingbo.jiang@databricks.com>
Signed-off-by: Xingbo Jiang <xingbo.jiang@databricks.com>
## What changes were proposed in this pull request?
After https://github.com/apache/spark/pull/22745 , Dataset encoder supports the combination of java bean and map type. This PR is to fix the Scala side.
The reason why it didn't work before is, `CatalystToExternalMap` tries to get the data type of the input map expression, while it can be unresolved and its data type is known. To fix it, we can follow `UnresolvedMapObjects`, to create a `UnresolvedCatalystToExternalMap`, and only create `CatalystToExternalMap` when the input map expression is resolved and the data type is known.
## How was this patch tested?
enable a old test case
Closes#22812 from cloud-fan/map.
Authored-by: Wenchen Fan <wenchen@databricks.com>
Signed-off-by: Wenchen Fan <wenchen@databricks.com>
## What changes were proposed in this pull request?
Implements Every, Some, Any aggregates in SQL. These new aggregate expressions are analyzed in normal way and rewritten to equivalent existing aggregate expressions in the optimizer.
Every(x) => Min(x) where x is boolean.
Some(x) => Max(x) where x is boolean.
Any is a synonym for Some.
SQL
```
explain extended select every(v) from test_agg group by k;
```
Plan :
```
== Parsed Logical Plan ==
'Aggregate ['k], [unresolvedalias('every('v), None)]
+- 'UnresolvedRelation `test_agg`
== Analyzed Logical Plan ==
every(v): boolean
Aggregate [k#0], [every(v#1) AS every(v)#5]
+- SubqueryAlias `test_agg`
+- Project [k#0, v#1]
+- SubqueryAlias `test_agg`
+- LocalRelation [k#0, v#1]
== Optimized Logical Plan ==
Aggregate [k#0], [min(v#1) AS every(v)#5]
+- LocalRelation [k#0, v#1]
== Physical Plan ==
*(2) HashAggregate(keys=[k#0], functions=[min(v#1)], output=[every(v)#5])
+- Exchange hashpartitioning(k#0, 200)
+- *(1) HashAggregate(keys=[k#0], functions=[partial_min(v#1)], output=[k#0, min#7])
+- LocalTableScan [k#0, v#1]
Time taken: 0.512 seconds, Fetched 1 row(s)
```
## How was this patch tested?
Added tests in SQLQueryTestSuite, DataframeAggregateSuite
Closes#22809 from dilipbiswal/SPARK-19851-specific-rewrite.
Authored-by: Dilip Biswal <dbiswal@us.ibm.com>
Signed-off-by: Wenchen Fan <wenchen@databricks.com>
## What changes were proposed in this pull request?
The main purpose of `schema_of_json` is the usage of combination with `from_json` (to make up the leak of schema inference) which takes its schema only as literal; however, currently `schema_of_json` allows JSON input as non-literal expressions (e.g, column).
This was mistakenly allowed - we don't have to take other usages rather then the main purpose into account for now.
This PR makes a followup to only allow literals for `schema_of_json`'s JSON input. We can allow non literal expressions later when it's needed or there are some usecase for it.
## How was this patch tested?
Unit tests were added.
Closes#22775 from HyukjinKwon/SPARK-25447-followup.
Lead-authored-by: hyukjinkwon <gurwls223@apache.org>
Co-authored-by: Hyukjin Kwon <gurwls223@apache.org>
Signed-off-by: Wenchen Fan <wenchen@databricks.com>
## What changes were proposed in this pull request?
In https://github.com/apache/spark/pull/22745 we introduced the `GetArrayFromMap` expression. Later on I realized this is duplicated as we already have `MapKeys` and `MapValues`.
This PR removes `GetArrayFromMap`
## How was this patch tested?
existing tests
Closes#22825 from cloud-fan/minor.
Authored-by: Wenchen Fan <wenchen@databricks.com>
Signed-off-by: Wenchen Fan <wenchen@databricks.com>
## What changes were proposed in this pull request?
This is inspired during implementing #21732. For now `ScalaReflection` needs to consider how `ExpressionEncoder` uses generated serializers and deserializers. And `ExpressionEncoder` has a weird `flat` flag. After discussion with cloud-fan, it seems to be better to refactor `ExpressionEncoder`. It should make SPARK-24762 easier to do.
To summarize the proposed changes:
1. `serializerFor` and `deserializerFor` return expressions for serializing/deserializing an input expression for a given type. They are private and should not be called directly.
2. `serializerForType` and `deserializerForType` returns an expression for serializing/deserializing for an object of type T to/from Spark SQL representation. It assumes the input object/Spark SQL representation is located at ordinal 0 of a row.
So in other words, `serializerForType` and `deserializerForType` return expressions for atomically serializing/deserializing JVM object to/from Spark SQL value.
A serializer returned by `serializerForType` will serialize an object at `row(0)` to a corresponding Spark SQL representation, e.g. primitive type, array, map, struct.
A deserializer returned by `deserializerForType` will deserialize an input field at `row(0)` to an object with given type.
3. The construction of `ExpressionEncoder` takes a pair of serializer and deserializer for type `T`. It uses them to create serializer and deserializer for T <-> row serialization. Now `ExpressionEncoder` dones't need to remember if serializer is flat or not. When we need to construct new `ExpressionEncoder` based on existing ones, we only need to change input location in the atomic serializer and deserializer.
## How was this patch tested?
Existing tests.
Closes#22749 from viirya/SPARK-24762-refactor.
Authored-by: Liang-Chi Hsieh <viirya@gmail.com>
Signed-off-by: Wenchen Fan <wenchen@databricks.com>
## What changes were proposed in this pull request?
Check the `spark.sql.repl.eagerEval.enabled` configuration property in SparkDataFrame `show()` method. If the `SparkSession` has eager execution enabled, the data will be returned to the R client when the data frame is created. So instead of seeing this
```
> df <- createDataFrame(faithful)
> df
SparkDataFrame[eruptions:double, waiting:double]
```
you will see
```
> df <- createDataFrame(faithful)
> df
+---------+-------+
|eruptions|waiting|
+---------+-------+
| 3.6| 79.0|
| 1.8| 54.0|
| 3.333| 74.0|
| 2.283| 62.0|
| 4.533| 85.0|
| 2.883| 55.0|
| 4.7| 88.0|
| 3.6| 85.0|
| 1.95| 51.0|
| 4.35| 85.0|
| 1.833| 54.0|
| 3.917| 84.0|
| 4.2| 78.0|
| 1.75| 47.0|
| 4.7| 83.0|
| 2.167| 52.0|
| 1.75| 62.0|
| 4.8| 84.0|
| 1.6| 52.0|
| 4.25| 79.0|
+---------+-------+
only showing top 20 rows
```
## How was this patch tested?
Manual tests as well as unit tests (one new test case is added).
Author: adrian555 <v2ave10p>
Closes#22455 from adrian555/eager_execution.
## What changes were proposed in this pull request?
In the PR, I propose to switch `from_json` on `FailureSafeParser`, and to make the function compatible to `PERMISSIVE` mode by default, and to support the `FAILFAST` mode as well. The `DROPMALFORMED` mode is not supported by `from_json`.
## How was this patch tested?
It was tested by existing `JsonSuite`/`CSVSuite`, `JsonFunctionsSuite` and `JsonExpressionsSuite` as well as new tests for `from_json` which checks different modes.
Closes#22237 from MaxGekk/from_json-failuresafe.
Lead-authored-by: Maxim Gekk <maxim.gekk@databricks.com>
Co-authored-by: hyukjinkwon <gurwls223@apache.org>
Signed-off-by: Wenchen Fan <wenchen@databricks.com>
This is a follow-up PR for #22708. It considers another case of java beans deserialization: java maps with struct keys/values.
When deserializing values of MapType with struct keys/values in java beans, fields of structs get mixed up. I suggest using struct data types retrieved from resolved input data instead of inferring them from java beans.
## What changes were proposed in this pull request?
Invocations of "keyArray" and "valueArray" functions are used to extract arrays of keys and values. Struct type of keys or values is also inferred from java bean structure and ends up with mixed up field order.
I created a new UnresolvedInvoke expression as a temporary substitution of Invoke expression while no actual data is available. It allows to provide the resulting data type during analysis based on the resolved input data, not on the java bean (similar to UnresolvedMapObjects).
Key and value arrays are then fed to MapObjects expression which I replaced with UnresolvedMapObjects, just like in case of ArrayType.
Finally I added resolution of UnresolvedInvoke expressions in Analyzer.resolveExpression method as an additional pattern matching case.
## How was this patch tested?
Added a test case.
Built complete project on travis.
viirya kiszk cloud-fan michalsenkyr marmbrus liancheng
Closes#22745 from vofque/SPARK-21402-FOLLOWUP.
Lead-authored-by: Vladimir Kuriatkov <vofque@gmail.com>
Co-authored-by: Vladimir Kuriatkov <Vladimir_Kuriatkov@epam.com>
Signed-off-by: Wenchen Fan <wenchen@databricks.com>
## What changes were proposed in this pull request?
The serializers of `RowEncoder` use few `If` Catalyst expression which inherits `ComplexTypeMergingExpression` that will check input data types.
It is possible to generate serializers which fail the check and can't to access the data type of serializers. When producing If expression, we should use the same data type at its input expressions.
## How was this patch tested?
Added test.
Closes#22785 from viirya/SPARK-25791.
Authored-by: Liang-Chi Hsieh <viirya@gmail.com>
Signed-off-by: Wenchen Fan <wenchen@databricks.com>
## What changes were proposed in this pull request?
This takes over original PR at #22019. The original proposal is to have null for float and double types. Later a more reasonable proposal is to disallow empty strings. This patch adds logic to throw exception when finding empty strings for non string types.
## How was this patch tested?
Added test.
Closes#22787 from viirya/SPARK-25040.
Authored-by: Liang-Chi Hsieh <viirya@gmail.com>
Signed-off-by: hyukjinkwon <gurwls223@apache.org>
## What changes were proposed in this pull request?
This PR adds `prettyNames` for `from_json`, `to_json`, `from_csv`, and `schema_of_json` so that appropriate names are used.
## How was this patch tested?
Unit tests
Closes#22773 from HyukjinKwon/minor-prettyNames.
Authored-by: hyukjinkwon <gurwls223@apache.org>
Signed-off-by: hyukjinkwon <gurwls223@apache.org>
## What changes were proposed in this pull request?
SQL interface support specify `StorageLevel` when cache table. The semantic is:
```sql
CACHE TABLE tableName OPTIONS('storageLevel' 'DISK_ONLY');
```
All supported `StorageLevel` are:
eefdf9f9dd/core/src/main/scala/org/apache/spark/storage/StorageLevel.scala (L172-L183)
## How was this patch tested?
unit tests and manual tests.
manual tests configuration:
```
--executor-memory 15G --executor-cores 5 --num-executors 50
```
Data:
Input Size / Records: 1037.7 GB / 11732805788
Result:
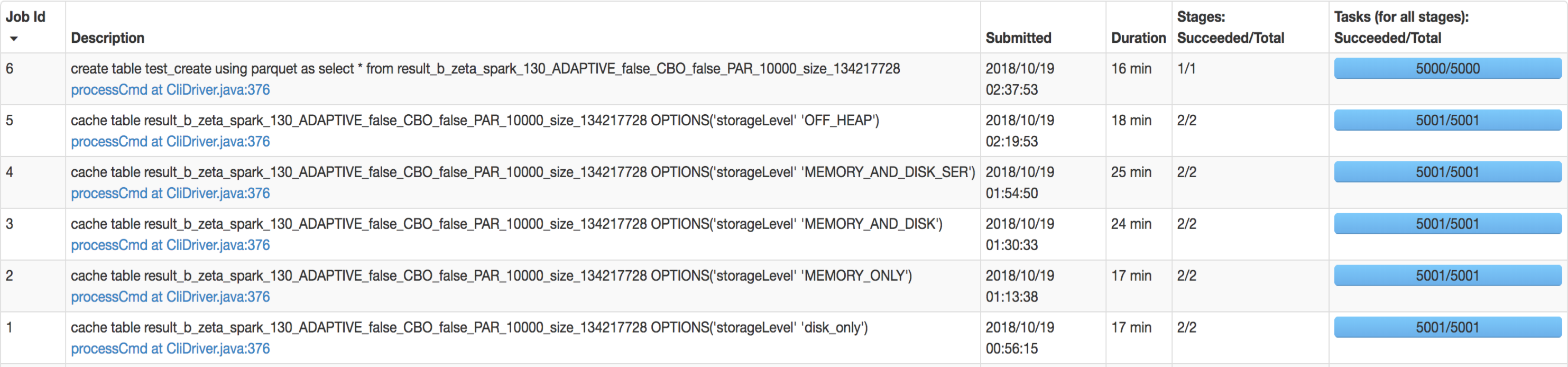
Closes#22263 from wangyum/SPARK-25269.
Authored-by: Yuming Wang <yumwang@ebay.com>
Signed-off-by: Dongjoon Hyun <dongjoon@apache.org>
## What changes were proposed in this pull request?
This is a follow-up PR for #22259. The extra field added in `ScalaUDF` with the original PR was declared optional, but should be indeed required, otherwise callers of `ScalaUDF`'s constructor could ignore this new field and cause the result to be incorrect. This PR makes the new field required and changes its name to `handleNullForInputs`.
#22259 breaks the previous behavior for null-handling of primitive-type input parameters. For example, for `val f = udf({(x: Int, y: Any) => x})`, `f(null, "str")` should return `null` but would return `0` after #22259. In this PR, all UDF methods except `def udf(f: AnyRef, dataType: DataType): UserDefinedFunction` have been restored with the original behavior. The only exception is documented in the Spark SQL migration guide.
In addition, now that we have this extra field indicating if a null-test should be applied on the corresponding input value, we can also make use of this flag to avoid the rule `HandleNullInputsForUDF` being applied infinitely.
## How was this patch tested?
Added UT in UDFSuite
Passed affected existing UTs:
AnalysisSuite
UDFSuite
Closes#22732 from maryannxue/spark-25044-followup.
Lead-authored-by: maryannxue <maryannxue@apache.org>
Co-authored-by: Wenchen Fan <wenchen@databricks.com>
Signed-off-by: Wenchen Fan <wenchen@databricks.com>
## What changes were proposed in this pull request?
CSVs with windows style crlf ('\r\n') don't work in multiline mode. They work fine in single line mode because the line separation is done by Hadoop, which can handle all the different types of line separators. This PR fixes it by enabling Univocity's line separator detection in multiline mode, which will detect '\r\n', '\r', or '\n' automatically as it is done by hadoop in single line mode.
## How was this patch tested?
Unit test with a file with crlf line endings.
Closes#22503 from justinuang/fix-clrf-multiline.
Authored-by: Justin Uang <juang@palantir.com>
Signed-off-by: hyukjinkwon <gurwls223@apache.org>
When deserializing values of ArrayType with struct elements in java beans, fields of structs get mixed up.
I suggest using struct data types retrieved from resolved input data instead of inferring them from java beans.
## What changes were proposed in this pull request?
MapObjects expression is used to map array elements to java beans. Struct type of elements is inferred from java bean structure and ends up with mixed up field order.
I used UnresolvedMapObjects instead of MapObjects, which allows to provide element type for MapObjects during analysis based on the resolved input data, not on the java bean.
## How was this patch tested?
Added a test case.
Built complete project on travis.
michalsenkyr cloud-fan marmbrus liancheng
Closes#22708 from vofque/SPARK-21402.
Lead-authored-by: Vladimir Kuriatkov <vofque@gmail.com>
Co-authored-by: Vladimir Kuriatkov <Vladimir_Kuriatkov@epam.com>
Signed-off-by: Wenchen Fan <wenchen@databricks.com>
## What changes were proposed in this pull request?
this PR correct some comment error:
1. change from "as low a possible" to "as low as possible" in RewriteDistinctAggregates.scala
2. delete redundant word “with” in HiveTableScanExec’s doExecute() method
## How was this patch tested?
Existing unit tests.
Closes#22694 from CarolinePeng/update_comment.
Authored-by: 彭灿00244106 <00244106@zte.intra>
Signed-off-by: hyukjinkwon <gurwls223@apache.org>
## What changes were proposed in this pull request?
`Literal.value` should have a value a value corresponding to `dataType`. This pr added code to verify it and fixed the existing tests to do so.
## How was this patch tested?
Modified the existing tests.
Closes#22724 from maropu/SPARK-25734.
Authored-by: Takeshi Yamamuro <yamamuro@apache.org>
Signed-off-by: Wenchen Fan <wenchen@databricks.com>
## What changes were proposed in this pull request?
The PR adds new function `from_csv()` similar to `from_json()` to parse columns with CSV strings. I added the following methods:
```Scala
def from_csv(e: Column, schema: StructType, options: Map[String, String]): Column
```
and this signature to call it from Python, R and Java:
```Scala
def from_csv(e: Column, schema: String, options: java.util.Map[String, String]): Column
```
## How was this patch tested?
Added new test suites `CsvExpressionsSuite`, `CsvFunctionsSuite` and sql tests.
Closes#22379 from MaxGekk/from_csv.
Lead-authored-by: Maxim Gekk <maxim.gekk@databricks.com>
Co-authored-by: Maxim Gekk <max.gekk@gmail.com>
Co-authored-by: Hyukjin Kwon <gurwls223@gmail.com>
Co-authored-by: hyukjinkwon <gurwls223@apache.org>
Signed-off-by: hyukjinkwon <gurwls223@apache.org>
## What changes were proposed in this pull request?
AFAIK multi-column count is not widely supported by the mainstream databases(postgres doesn't support), and the SQL standard doesn't define it clearly, as near as I can tell.
Since Spark supports it, we should clearly document the current behavior and add tests to verify it.
## How was this patch tested?
N/A
Closes#22728 from cloud-fan/doc.
Authored-by: Wenchen Fan <wenchen@databricks.com>
Signed-off-by: hyukjinkwon <gurwls223@apache.org>
## What changes were proposed in this pull request?
Project logical operator generates valid constraints using two opposite operations. It substracts child constraints from all constraints, than union child constraints again. I think it may be not necessary.
Aggregate operator has the same problem with Project.
This PR try to remove these two opposite collection operations.
## How was this patch tested?
Related unit tests:
ProjectEstimationSuite
CollapseProjectSuite
PushProjectThroughUnionSuite
UnsafeProjectionBenchmark
GeneratedProjectionSuite
CodeGeneratorWithInterpretedFallbackSuite
TakeOrderedAndProjectSuite
GenerateUnsafeProjectionSuite
BucketedRandomProjectionLSHSuite
RemoveRedundantAliasAndProjectSuite
AggregateBenchmark
AggregateOptimizeSuite
AggregateEstimationSuite
DecimalAggregatesSuite
DateFrameAggregateSuite
ObjectHashAggregateSuite
TwoLevelAggregateHashMapSuite
ObjectHashAggregateExecBenchmark
SingleLevelAggregateHaspMapSuite
TypedImperativeAggregateSuite
RewriteDistinctAggregatesSuite
HashAggregationQuerySuite
HashAggregationQueryWithControlledFallbackSuite
TypedImperativeAggregateSuite
TwoLevelAggregateHashMapWithVectorizedMapSuite
Closes#22706 from SongYadong/generate_constraints.
Authored-by: SongYadong <song.yadong1@zte.com.cn>
Signed-off-by: gatorsmile <gatorsmile@gmail.com>
## What changes were proposed in this pull request?
improve the code comment added in https://github.com/apache/spark/pull/22702/files
## How was this patch tested?
N/A
Closes#22711 from cloud-fan/minor.
Authored-by: Wenchen Fan <wenchen@databricks.com>
Signed-off-by: gatorsmile <gatorsmile@gmail.com>
## What changes were proposed in this pull request?
```Scala
val df1 = Seq(("abc", 1), (null, 3)).toDF("col1", "col2")
df1.write.mode(SaveMode.Overwrite).parquet("/tmp/test1")
val df2 = spark.read.parquet("/tmp/test1")
df2.filter("col1 = 'abc' OR (col1 != 'abc' AND col2 == 3)").show()
```
Before the PR, it returns both rows. After the fix, it returns `Row ("abc", 1))`. This is to fix the bug in NULL handling in BooleanSimplification. This is a bug introduced in Spark 1.6 release.
## How was this patch tested?
Added test cases
Closes#22702 from gatorsmile/fixBooleanSimplify2.
Authored-by: gatorsmile <gatorsmile@gmail.com>
Signed-off-by: gatorsmile <gatorsmile@gmail.com>
## What changes were proposed in this pull request?
After the changes, total execution time of `JsonExpressionsSuite.scala` dropped from 12.5 seconds to 3 seconds.
Closes#22657 from MaxGekk/json-timezone-test.
Authored-by: Maxim Gekk <maxim.gekk@databricks.com>
Signed-off-by: Sean Owen <sean.owen@databricks.com>
## What changes were proposed in this pull request?
According to the SQL standard, when a query contains `HAVING`, it indicates an aggregate operator. For more details please refer to https://blog.jooq.org/2014/12/04/do-you-really-understand-sqls-group-by-and-having-clauses/
However, in Spark SQL parser, we treat HAVING as a normal filter when there is no GROUP BY, which breaks SQL semantic and lead to wrong result. This PR fixes the parser.
## How was this patch tested?
new test
Closes#22696 from cloud-fan/having.
Authored-by: Wenchen Fan <wenchen@databricks.com>
Signed-off-by: gatorsmile <gatorsmile@gmail.com>
## What changes were proposed in this pull request?
The HandleNullInputsForUDF rule can generate new If node infinitely, thus causing problems like match of SQL cache missed.
This was fixed in SPARK-24891 and was then broken by SPARK-25044.
The unit test in `AnalysisSuite` added in SPARK-24891 should have failed but didn't because it wasn't properly updated after the `ScalaUDF` constructor signature change. So this PR also updates the test accordingly based on the new `ScalaUDF` constructor.
## How was this patch tested?
Updated the original UT. This should be justified as the original UT became invalid after SPARK-25044.
Closes#22701 from maryannxue/spark-25690.
Authored-by: maryannxue <maryannxue@apache.org>
Signed-off-by: gatorsmile <gatorsmile@gmail.com>
## What changes were proposed in this pull request?
This PR can correctly cause assertion failure when incorrect nullable of DataType in the result is generated by a target function to be tested.
Let us think the following example. In the future, a developer would write incorrect code that returns unexpected result. We have to correctly cause fail in this test since `valueContainsNull=false` while `expr` includes `null`. However, without this PR, this test passes. This PR can correctly cause fail.
```
test("test TARGETFUNCTON") {
val expr = TARGETMAPFUNCTON()
// expr = UnsafeMap(3 -> 6, 7 -> null)
// expr.dataType = (IntegerType, IntegerType, false)
expected = Map(3 -> 6, 7 -> null)
checkEvaluation(expr, expected)
```
In [`checkEvaluationWithUnsafeProjection`](https://github.com/apache/spark/blob/master/sql/catalyst/src/test/scala/org/apache/spark/sql/catalyst/expressions/ExpressionEvalHelper.scala#L208-L235), the results are compared using `UnsafeRow`. When the given `expected` is [converted](https://github.com/apache/spark/blob/master/sql/catalyst/src/test/scala/org/apache/spark/sql/catalyst/expressions/ExpressionEvalHelper.scala#L226-L227)) to `UnsafeRow` using the `DataType` of `expr`.
```
val expectedRow = UnsafeProjection.create(Array(expression.dataType, expression.dataType)).apply(lit)
```
In summary, `expr` is `[0,1800000038,5000000038,18,2,0,700000003,2,0,6,18,2,0,700000003,2,0,6]` with and w/o this PR. `expected` is converted to
* w/o this PR, `[0,1800000038,5000000038,18,2,0,700000003,2,0,6,18,2,0,700000003,2,0,6]`
* with this PR, `[0,1800000038,5000000038,18,2,0,700000003,2,2,6,18,2,0,700000003,2,2,6]`
As a result, w/o this PR, the test unexpectedly passes.
This is because, w/o this PR, based on given `dataType`, generated code of projection for `expected` avoids to set nullbit.
```
// tmpInput_2 is expected
/* 155 */ for (int index_1 = 0; index_1 < numElements_1; index_1++) {
/* 156 */ mutableStateArray_1[1].write(index_1, tmpInput_2.getInt(index_1));
/* 157 */ }
```
With this PR, generated code of projection for `expected` always checks whether nullbit should be set by `isNullAt`
```
// tmpInput_2 is expected
/* 161 */ for (int index_1 = 0; index_1 < numElements_1; index_1++) {
/* 162 */
/* 163 */ if (tmpInput_2.isNullAt(index_1)) {
/* 164 */ mutableStateArray_1[1].setNull4Bytes(index_1);
/* 165 */ } else {
/* 166 */ mutableStateArray_1[1].write(index_1, tmpInput_2.getInt(index_1));
/* 167 */ }
/* 168 */
/* 169 */ }
```
## How was this patch tested?
Existing UTs
Closes#22375 from kiszk/SPARK-25388.
Authored-by: Kazuaki Ishizaki <ishizaki@jp.ibm.com>
Signed-off-by: Wenchen Fan <wenchen@databricks.com>
## What changes were proposed in this pull request?
Try testing timezones in parallel instead in CastSuite, instead of random sampling.
See also #22631
## How was this patch tested?
Existing test.
Closes#22672 from srowen/SPARK-25605.2.
Authored-by: Sean Owen <sean.owen@databricks.com>
Signed-off-by: Sean Owen <sean.owen@databricks.com>
While working on another PR, I noticed that there is quite some legacy Java in there that can be beautified. For example the use of features from Java8, such as:
- Collection libraries
- Try-with-resource blocks
No logic has been changed. I think it is important to have a solid codebase with examples that will inspire next PR's to follow up on the best practices.
What are your thoughts on this?
This makes code easier to read, and using try-with-resource makes is less likely to forget to close something.
## What changes were proposed in this pull request?
No changes in the logic of Spark, but more in the aesthetics of the code.
## How was this patch tested?
Using the existing unit tests. Since no logic is changed, the existing unit tests should pass.
Please review http://spark.apache.org/contributing.html before opening a pull request.
Closes#22637 from Fokko/SPARK-25408.
Authored-by: Fokko Driesprong <fokkodriesprong@godatadriven.com>
Signed-off-by: Sean Owen <sean.owen@databricks.com>
## What changes were proposed in this pull request?
Refactor `HashBenchmark` to use main method.
1. use `spark-submit`:
```console
bin/spark-submit --class org.apache.spark.sql.HashBenchmark --jars ./core/target/spark-core_2.11-3.0.0-SNAPSHOT-tests.jar ./sql/catalyst/target/spark-catalyst_2.11-3.0.0-SNAPSHOT-tests.jar
```
2. Generate benchmark result:
```console
SPARK_GENERATE_BENCHMARK_FILES=1 build/sbt "catalyst/test:runMain org.apache.spark.sql.HashBenchmark"
```
## How was this patch tested?
manual tests
Closes#22651 from wangyum/SPARK-25657.
Lead-authored-by: Yuming Wang <wgyumg@gmail.com>
Co-authored-by: Yuming Wang <yumwang@ebay.com>
Co-authored-by: Dongjoon Hyun <dongjoon@apache.org>
Signed-off-by: Dongjoon Hyun <dongjoon@apache.org>
## What changes were proposed in this pull request?
Refactor `HashByteArrayBenchmark` to use main method.
1. use `spark-submit`:
```console
bin/spark-submit --class org.apache.spark.sql.HashByteArrayBenchmark --jars ./core/target/spark-core_2.11-3.0.0-SNAPSHOT-tests.jar ./sql/catalyst/target/spark-catalyst_2.11-3.0.0-SNAPSHOT-tests.jar
```
2. Generate benchmark result:
```console
SPARK_GENERATE_BENCHMARK_FILES=1 build/sbt "catalyst/test:runMain org.apache.spark.sql.HashByteArrayBenchmark"
```
## How was this patch tested?
manual tests
Closes#22652 from wangyum/SPARK-25658.
Lead-authored-by: Yuming Wang <wgyumg@gmail.com>
Co-authored-by: Yuming Wang <yumwang@ebay.com>
Co-authored-by: Dongjoon Hyun <dongjoon@apache.org>
Signed-off-by: Dongjoon Hyun <dongjoon@apache.org>
## What changes were proposed in this pull request?
Clean up the joinCriteria parsing in the parser by directly using identifierList
## How was this patch tested?
N/A
Closes#22648 from gatorsmile/cleanupJoinCriteria.
Authored-by: gatorsmile <gatorsmile@gmail.com>
Signed-off-by: gatorsmile <gatorsmile@gmail.com>
## What changes were proposed in this pull request?
Adds support for the setting limit in the sql split function
## How was this patch tested?
1. Updated unit tests
2. Tested using Scala spark shell
Please review http://spark.apache.org/contributing.html before opening a pull request.
Closes#22227 from phegstrom/master.
Authored-by: Parker Hegstrom <phegstrom@palantir.com>
Signed-off-by: hyukjinkwon <gurwls223@apache.org>
Hi all,
Jackson is incompatible with upstream versions, therefore bump the Jackson version to a more recent one. I bumped into some issues with Azure CosmosDB that is using a more recent version of Jackson. This can be fixed by adding exclusions and then it works without any issues. So no breaking changes in the API's.
I would also consider bumping the version of Jackson in Spark. I would suggest to keep up to date with the dependencies, since in the future this issue will pop up more frequently.
## What changes were proposed in this pull request?
Bump Jackson to 2.9.6
## How was this patch tested?
Compiled and tested it locally to see if anything broke.
Please review http://spark.apache.org/contributing.html before opening a pull request.
Closes#21596 from Fokko/fd-bump-jackson.
Authored-by: Fokko Driesprong <fokkodriesprong@godatadriven.com>
Signed-off-by: hyukjinkwon <gurwls223@apache.org>
While working on another PR, I noticed that there is quite some legacy Java in there that can be beautified. For example the use og features from Java8, such as:
- Collection libraries
- Try-with-resource blocks
No code has been changed
What are your thoughts on this?
This makes code easier to read, and using try-with-resource makes is less likely to forget to close something.
## What changes were proposed in this pull request?
(Please fill in changes proposed in this fix)
## How was this patch tested?
(Please explain how this patch was tested. E.g. unit tests, integration tests, manual tests)
(If this patch involves UI changes, please attach a screenshot; otherwise, remove this)
Please review http://spark.apache.org/contributing.html before opening a pull request.
Closes#22399 from Fokko/SPARK-25408.
Authored-by: Fokko Driesprong <fokkodriesprong@godatadriven.com>
Signed-off-by: Sean Owen <sean.owen@databricks.com>
## What changes were proposed in this pull request?
The test `cast string to timestamp` used to run for all time zones. So it run for more than 600 times. Running the tests for a significant subset of time zones is probably good enough and doing this in a randomized manner enforces anyway that we are going to test all time zones in different runs.
## How was this patch tested?
the test time reduces to 11 seconds from more than 2 minutes
Closes#22631 from mgaido91/SPARK-25605.
Authored-by: Marco Gaido <marcogaido91@gmail.com>
Signed-off-by: gatorsmile <gatorsmile@gmail.com>
## What changes were proposed in this pull request?
Reduce `DateExpressionsSuite.Hour` test time costs in Jenkins by reduce iteration times.
## How was this patch tested?
Manual tests on my local machine.
before:
```
- Hour (34 seconds, 54 milliseconds)
```
after:
```
- Hour (2 seconds, 697 milliseconds)
```
Closes#22632 from wangyum/SPARK-25606.
Authored-by: Yuming Wang <yumwang@ebay.com>
Signed-off-by: gatorsmile <gatorsmile@gmail.com>
## What changes were proposed in this pull request?
The PR changes the test introduced for SPARK-22226, so that we don't run analysis and optimization on the plan. The scope of the test is code generation and running the above mentioned operation is expensive and useless for the test.
The UT was also moved to the `CodeGenerationSuite` which is a better place given the scope of the test.
## How was this patch tested?
running the UT before SPARK-22226 fails, after it passes. The execution time is about 50% the original one. On my laptop this means that the test now runs in about 23 seconds (instead of 50 seconds).
Closes#22629 from mgaido91/SPARK-25609.
Authored-by: Marco Gaido <marcogaido91@gmail.com>
Signed-off-by: gatorsmile <gatorsmile@gmail.com>
## What changes were proposed in this pull request?
In #20850 when writing non-null decimals, instead of zero-ing all the 16 allocated bytes, we zero-out only the padding bytes. Since we always allocate 16 bytes, if the number of bytes needed for a decimal is lower than 9, then this means that the bytes between 8 and 16 are not zero-ed.
I see 2 solutions here:
- we can zero-out all the bytes in advance as it was done before #20850 (safer solution IMHO);
- we can allocate only the needed bytes (may be a bit more efficient in terms of memory used, but I have not investigated the feasibility of this option).
Hence I propose here the first solution in order to fix the correctness issue. We can eventually switch to the second if we think is more efficient later.
## How was this patch tested?
Running the test attached in the JIRA + added UT
Closes#22602 from mgaido91/SPARK-25582.
Authored-by: Marco Gaido <marcogaido91@gmail.com>
Signed-off-by: Dongjoon Hyun <dongjoon@apache.org>
## What changes were proposed in this pull request?
Rename method `benchmark` in `BenchmarkBase` as `runBenchmarkSuite `. Also add comments.
Currently the method name `benchmark` is a bit confusing. Also the name is the same as instances of `Benchmark`:
f246813afb/sql/hive/src/test/scala/org/apache/spark/sql/hive/orc/OrcReadBenchmark.scala (L330-L339)
## How was this patch tested?
Unit test.
Closes#22599 from gengliangwang/renameBenchmarkSuite.
Authored-by: Gengliang Wang <gengliang.wang@databricks.com>
Signed-off-by: Dongjoon Hyun <dongjoon@apache.org>
## What changes were proposed in this pull request?
This patch is to bump the master branch version to 3.0.0-SNAPSHOT.
## How was this patch tested?
N/A
Closes#22606 from gatorsmile/bump3.0.
Authored-by: gatorsmile <gatorsmile@gmail.com>
Signed-off-by: gatorsmile <gatorsmile@gmail.com>
## What changes were proposed in this pull request?
#22519 introduced a bug when the attributes in the pivot clause are cosmetically different from the output ones (eg. different case). In particular, the problem is that the PR used a `Set[Attribute]` instead of an `AttributeSet`.
## How was this patch tested?
added UT
Closes#22582 from mgaido91/SPARK-25505_followup.
Authored-by: Marco Gaido <marcogaido91@gmail.com>
Signed-off-by: gatorsmile <gatorsmile@gmail.com>
## What changes were proposed in this pull request?
This PR adds a rule to force `.toLowerCase(Locale.ROOT)` or `toUpperCase(Locale.ROOT)`.
It produces an error as below:
```
[error] Are you sure that you want to use toUpperCase or toLowerCase without the root locale? In most cases, you
[error] should use toUpperCase(Locale.ROOT) or toLowerCase(Locale.ROOT) instead.
[error] If you must use toUpperCase or toLowerCase without the root locale, wrap the code block with
[error] // scalastyle:off caselocale
[error] .toUpperCase
[error] .toLowerCase
[error] // scalastyle:on caselocale
```
This PR excludes the cases above for SQL code path for external calls like table name, column name and etc.
For test suites, or when it's clear there's no locale problem like Turkish locale problem, it uses `Locale.ROOT`.
One minor problem is, `UTF8String` has both methods, `toLowerCase` and `toUpperCase`, and the new rule detects them as well. They are ignored.
## How was this patch tested?
Manually tested, and Jenkins tests.
Closes#22581 from HyukjinKwon/SPARK-25565.
Authored-by: hyukjinkwon <gurwls223@apache.org>
Signed-off-by: hyukjinkwon <gurwls223@apache.org>
## What changes were proposed in this pull request?
In the PR, I propose to extended the `schema_of_json()` function, and accept JSON options since they can impact on schema inferring. Purpose is to support the same options that `from_json` can use during schema inferring.
## How was this patch tested?
Added SQL, Python and Scala tests (`JsonExpressionsSuite` and `JsonFunctionsSuite`) that checks JSON options are used.
Closes#22442 from MaxGekk/schema_of_json-options.
Authored-by: Maxim Gekk <maxim.gekk@databricks.com>
Signed-off-by: hyukjinkwon <gurwls223@apache.org>
## What changes were proposed in this pull request?
**Description from the JIRA :**
Currently, to collect the statistics of all the columns, users need to specify the names of all the columns when calling the command "ANALYZE TABLE ... FOR COLUMNS...". This is not user friendly. Instead, we can introduce the following SQL command to achieve it without specifying the column names.
```
ANALYZE TABLE [db_name.]tablename COMPUTE STATISTICS FOR ALL COLUMNS;
```
## How was this patch tested?
Added new tests in SparkSqlParserSuite and StatisticsSuite
Closes#22566 from dilipbiswal/SPARK-25458.
Authored-by: Dilip Biswal <dbiswal@us.ibm.com>
Signed-off-by: gatorsmile <gatorsmile@gmail.com>
## What changes were proposed in this pull request?
The grouping columns from a Pivot query are inferred as "input columns - pivot columns - pivot aggregate columns", where input columns are the output of the child relation of Pivot. The grouping columns will be the leading columns in the pivot output and they should preserve the same order as specified by the input. For example,
```
SELECT * FROM (
SELECT course, earnings, "a" as a, "z" as z, "b" as b, "y" as y, "c" as c, "x" as x, "d" as d, "w" as w
FROM courseSales
)
PIVOT (
sum(earnings)
FOR course IN ('dotNET', 'Java')
)
```
The output columns should be "a, z, b, y, c, x, d, w, ..." but now it is "a, b, c, d, w, x, y, z, ..."
The fix is to use the child plan's `output` instead of `outputSet` so that the order can be preserved.
## How was this patch tested?
Added UT.
Closes#22519 from maryannxue/spark-25505.
Authored-by: maryannxue <maryannxue@apache.org>
Signed-off-by: gatorsmile <gatorsmile@gmail.com>
## What changes were proposed in this pull request?
The `show create table` will show a lot of generated attributes for views that created by older Spark version. This PR will basically revert https://issues.apache.org/jira/browse/SPARK-19272 back, so when you `DESC [FORMATTED|EXTENDED] view` will show the original view DDL text.
## How was this patch tested?
Unit test.
Closes#22458 from zheyuan28/testbranch.
Lead-authored-by: Chris Zhao <chris.zhao@databricks.com>
Co-authored-by: Christopher Zhao <chris.zhao@databricks.com>
Co-authored-by: Dongjoon Hyun <dongjoon@apache.org>
Signed-off-by: Dongjoon Hyun <dongjoon@apache.org>
## What changes were proposed in this pull request?
There are 2 places we check for problematic `InSubquery`: the rule `ResolveSubquery` and `InSubquery.checkInputDataTypes`. We should unify them.
## How was this patch tested?
existing tests
Closes#22563 from cloud-fan/followup.
Authored-by: Wenchen Fan <wenchen@databricks.com>
Signed-off-by: Wenchen Fan <wenchen@databricks.com>
## What changes were proposed in this pull request?
As per the discussion in https://github.com/apache/spark/pull/22553#pullrequestreview-159192221,
override `filterKeys` violates the documented semantics.
This PR is to remove it and add documentation.
Also fix one potential non-serializable map in `FileStreamOptions`.
The only one call of `CaseInsensitiveMap`'s `filterKeys` left is
c3c45cbd76/sql/hive/src/main/scala/org/apache/spark/sql/hive/execution/HiveOptions.scala (L88-L90)
But this one is OK.
## How was this patch tested?
Existing unit tests.
Closes#22562 from gengliangwang/SPARK-25541-FOLLOWUP.
Authored-by: Gengliang Wang <gengliang.wang@databricks.com>
Signed-off-by: Wenchen Fan <wenchen@databricks.com>
## What changes were proposed in this pull request?
Thanks for bahchis reporting this. It is more like a follow up work for #16581, this PR fix the scenario of Python UDF accessing attributes from both side of join in join condition.
## How was this patch tested?
Add regression tests in PySpark and `BatchEvalPythonExecSuite`.
Closes#22326 from xuanyuanking/SPARK-25314.
Authored-by: Yuanjian Li <xyliyuanjian@gmail.com>
Signed-off-by: Wenchen Fan <wenchen@databricks.com>
## What changes were proposed in this pull request?
In ElementAt, when first argument is MapType, we should coerce the key type and the second argument based on findTightestCommonType. This is not happening currently. We may produce wrong output as we will incorrectly downcast the right hand side double expression to int.
```SQL
spark-sql> select element_at(map(1,"one", 2, "two"), 2.2);
two
```
Also, when the first argument is ArrayType, the second argument should be an integer type or a smaller integral type that can be safely casted to an integer type. Currently we may do an unsafe cast. In the following case, we should fail with an error as 2.2 is not a integer index. But instead we down cast it to int currently and return a result instead.
```SQL
spark-sql> select element_at(array(1,2), 1.24D);
1
```
This PR also supports implicit cast between two MapTypes. I have followed similar logic that exists today to do implicit casts between two array types.
## How was this patch tested?
Added new tests in DataFrameFunctionSuite, TypeCoercionSuite.
Closes#22544 from dilipbiswal/SPARK-25522.
Authored-by: Dilip Biswal <dbiswal@us.ibm.com>
Signed-off-by: Wenchen Fan <wenchen@databricks.com>
## What changes were proposed in this pull request?
We have an agreement that the behavior of `from/to_utc_timestamp` is corrected, although the function itself doesn't make much sense in Spark: https://issues.apache.org/jira/browse/SPARK-23715
This PR improves the document.
## How was this patch tested?
N/A
Closes#22543 from cloud-fan/doc.
Authored-by: Wenchen Fan <wenchen@databricks.com>
Signed-off-by: Wenchen Fan <wenchen@databricks.com>
## What changes were proposed in this pull request?
Refactor `UnsafeProjectionBenchmark` to use main method.
Generate benchmark result:
```
SPARK_GENERATE_BENCHMARK_FILES=1 build/sbt "catalyst/test:runMain org.apache.spark.sql.UnsafeProjectionBenchmark"
```
## How was this patch tested?
manual test
Closes#22493 from yucai/SPARK-25485.
Lead-authored-by: yucai <yyu1@ebay.com>
Co-authored-by: Yucai Yu <yucai.yu@foxmail.com>
Co-authored-by: Dongjoon Hyun <dongjoon@apache.org>
Signed-off-by: Dongjoon Hyun <dongjoon@apache.org>
## What changes were proposed in this pull request?
https://github.com/apache/spark/pull/20023 proposed to allow precision lose during decimal operations, to reduce the possibilities of overflow. This is a behavior change and is protected by the DECIMAL_OPERATIONS_ALLOW_PREC_LOSS config. However, that PR introduced another behavior change: pick a minimum precision for integral literals, which is not protected by a config. This PR add a new config for it: `spark.sql.literal.pickMinimumPrecision`.
This can allow users to work around issue in SPARK-25454, which is caused by a long-standing bug of negative scale.
## How was this patch tested?
a new test
Closes#22494 from cloud-fan/decimal.
Authored-by: Wenchen Fan <wenchen@databricks.com>
Signed-off-by: gatorsmile <gatorsmile@gmail.com>
## What changes were proposed in this pull request?
Describe spark.sql.parquet.writeLegacyFormat property in Spark SQL, DataFrames and Datasets Guide.
## How was this patch tested?
N/A
Closes#22453 from seancxmao/SPARK-20937.
Authored-by: seancxmao <seancxmao@gmail.com>
Signed-off-by: hyukjinkwon <gurwls223@apache.org>
## What changes were proposed in this pull request?
This PR contains 3 optimizations:
1) it improves significantly the operation `--` on `AttributeSet`. As a benchmark for the `--` operation, the following code has been run
```
test("AttributeSet -- benchmark") {
val attrSetA = AttributeSet((1 to 100).map { i => AttributeReference(s"c$i", IntegerType)() })
val attrSetB = AttributeSet(attrSetA.take(80).toSeq)
val attrSetC = AttributeSet((1 to 100).map { i => AttributeReference(s"c2_$i", IntegerType)() })
val attrSetD = AttributeSet((attrSetA.take(50) ++ attrSetC.take(50)).toSeq)
val attrSetE = AttributeSet((attrSetC.take(50) ++ attrSetA.take(50)).toSeq)
val n_iter = 1000000
val t0 = System.nanoTime()
(1 to n_iter) foreach { _ =>
val r1 = attrSetA -- attrSetB
val r2 = attrSetA -- attrSetC
val r3 = attrSetA -- attrSetD
val r4 = attrSetA -- attrSetE
}
val t1 = System.nanoTime()
val totalTime = t1 - t0
println(s"Average time: ${totalTime / n_iter} us")
}
```
The results are:
```
Before PR - Average time: 67674 us (100 %)
After PR - Average time: 28827 us (42.6 %)
```
2) In `ColumnPruning`, it replaces the occurrences of `(attributeSet1 -- attributeSet2).nonEmpty` with `attributeSet1.subsetOf(attributeSet2)` which is order of magnitudes more efficient (especially where there are many attributes). Running the previous benchmark replacing `--` with `subsetOf` returns:
```
Average time: 67 us (0.1 %)
```
3) Provides a more efficient way of building `AttributeSet`s, which can greatly improve the performance of the methods `references` and `outputSet` of `Expression` and `QueryPlan`. This basically avoids unneeded operations (eg. creating many `AttributeEqual` wrapper classes which could be avoided)
The overall effect of those optimizations has been tested on `ColumnPruning` with the following benchmark:
```
test("ColumnPruning benchmark") {
val attrSetA = (1 to 100).map { i => AttributeReference(s"c$i", IntegerType)() }
val attrSetB = attrSetA.take(80)
val attrSetC = attrSetA.take(20).map(a => Alias(Add(a, Literal(1)), s"${a.name}_1")())
val input = LocalRelation(attrSetA)
val query1 = Project(attrSetB, Project(attrSetA, input)).analyze
val query2 = Project(attrSetC, Project(attrSetA, input)).analyze
val query3 = Project(attrSetA, Project(attrSetA, input)).analyze
val nIter = 100000
val t0 = System.nanoTime()
(1 to nIter).foreach { _ =>
ColumnPruning(query1)
ColumnPruning(query2)
ColumnPruning(query3)
}
val t1 = System.nanoTime()
val totalTime = t1 - t0
println(s"Average time: ${totalTime / nIter} us")
}
```
The output of the test is:
```
Before PR - Average time: 733471 us (100 %)
After PR - Average time: 362455 us (49.4 %)
```
The performance improvement has been evaluated also on the `SQLQueryTestSuite`'s queries:
```
(before) org.apache.spark.sql.catalyst.optimizer.ColumnPruning 518413198 / 1377707172 2756 / 15717
(after) org.apache.spark.sql.catalyst.optimizer.ColumnPruning 415432579 / 1121147950 2756 / 15717
% Running time 80.1% / 81.3%
```
Also other rules benefit especially from (3), despite the impact is lower, eg:
```
(before) org.apache.spark.sql.catalyst.analysis.Analyzer$ResolveReferences 307341442 / 623436806 2154 / 16480
(after) org.apache.spark.sql.catalyst.analysis.Analyzer$ResolveReferences 290511312 / 560962495 2154 / 16480
% Running time 94.5% / 90.0%
```
The reason why the impact on the `SQLQueryTestSuite`'s queries is lower compared to the other benchmark is that the optimizations are more significant when the number of attributes involved is higher. Since in the tests we often have very few attributes, the effect there is lower.
## How was this patch tested?
run benchmarks + existing UTs
Closes#22364 from mgaido91/SPARK-25379.
Authored-by: Marco Gaido <marcogaido91@gmail.com>
Signed-off-by: Wenchen Fan <wenchen@databricks.com>
## What changes were proposed in this pull request?
`CaseInsensitiveMap` is declared as Serializable. However, it is no serializable after `-` operator or `filterKeys` method.
This PR fix the issue by overriding the operator `-` and method `filterKeys`. So the we can avoid potential `NotSerializableException` on using `CaseInsensitiveMap`.
## How was this patch tested?
New test suite.
Closes#22553 from gengliangwang/fixCaseInsensitiveMap.
Authored-by: Gengliang Wang <gengliang.wang@databricks.com>
Signed-off-by: Wenchen Fan <wenchen@databricks.com>
## What changes were proposed in this pull request?
Currently, Spark has 7 `withTempPath` and 6 `withSQLConf` functions. This PR aims to remove duplicated and inconsistent code and reduce them to the following meaningful implementations.
**withTempPath**
- `SQLHelper.withTempPath`: The one which was used in `SQLTestUtils`.
**withSQLConf**
- `SQLHelper.withSQLConf`: The one which was used in `PlanTest`.
- `ExecutorSideSQLConfSuite.withSQLConf`: The one which doesn't throw `AnalysisException` on StaticConf changes.
- `SQLTestUtils.withSQLConf`: The one which overrides intentionally to change the active session.
```scala
protected override def withSQLConf(pairs: (String, String)*)(f: => Unit): Unit = {
SparkSession.setActiveSession(spark)
super.withSQLConf(pairs: _*)(f)
}
```
## How was this patch tested?
Pass the Jenkins with the existing tests.
Closes#22548 from dongjoon-hyun/SPARK-25534.
Authored-by: Dongjoon Hyun <dongjoon@apache.org>
Signed-off-by: Dongjoon Hyun <dongjoon@apache.org>
## What changes were proposed in this pull request?
The PR introduces new JSON option `pretty` which allows to turn on `DefaultPrettyPrinter` of `Jackson`'s Json generator. New option is useful in exploring of deep nested columns and in converting of JSON columns in more readable representation (look at the added test).
## How was this patch tested?
Added rount trip test which convert an JSON string to pretty representation via `from_json()` and `to_json()`.
Closes#22534 from MaxGekk/pretty-json.
Lead-authored-by: Maxim Gekk <maxim.gekk@databricks.com>
Co-authored-by: Maxim Gekk <max.gekk@gmail.com>
Signed-off-by: hyukjinkwon <gurwls223@apache.org>
## What changes were proposed in this pull request?
Add the legacy prefix for spark.sql.execution.pandas.groupedMap.assignColumnsByPosition and rename it to spark.sql.legacy.execution.pandas.groupedMap.assignColumnsByName
## How was this patch tested?
The existing tests.
Closes#22540 from gatorsmile/renameAssignColumnsByPosition.
Lead-authored-by: gatorsmile <gatorsmile@gmail.com>
Co-authored-by: Hyukjin Kwon <gurwls223@gmail.com>
Signed-off-by: hyukjinkwon <gurwls223@apache.org>
## What changes were proposed in this pull request?
This patch reverts entirely all the regr_* functions added in SPARK-23907. These were added by mgaido91 (and proposed by gatorsmile) to improve compatibility with other database systems, without any actual use cases. However, they are very rarely used, and in Spark there are much better ways to compute these functions, due to Spark's flexibility in exposing real programming APIs.
I'm going through all the APIs added in Spark 2.4 and I think we should revert these. If there are strong enough demands and more use cases, we can add them back in the future pretty easily.
## How was this patch tested?
Reverted test cases also.
Closes#22541 from rxin/SPARK-23907.
Authored-by: Reynold Xin <rxin@databricks.com>
Signed-off-by: hyukjinkwon <gurwls223@apache.org>
## What changes were proposed in this pull request?
In ArrayRemove, we currently cast the right hand side expression to match the element type of the left hand side Array. This may result in down casting and may return wrong result or questionable result.
Example :
```SQL
spark-sql> select array_remove(array(1,2,3), 1.23D);
[2,3]
```
```SQL
spark-sql> select array_remove(array(1,2,3), 'foo');
NULL
```
We should safely coerce both left and right hand side expressions.
## How was this patch tested?
Added tests in DataFrameFunctionsSuite
Closes#22542 from dilipbiswal/SPARK-25519.
Authored-by: Dilip Biswal <dbiswal@us.ibm.com>
Signed-off-by: Wenchen Fan <wenchen@databricks.com>
## What changes were proposed in this pull request?
In ArrayPosition, we currently cast the right hand side expression to match the element type of the left hand side Array. This may result in down casting and may return wrong result or questionable result.
Example :
```SQL
spark-sql> select array_position(array(1), 1.34);
1
```
```SQL
spark-sql> select array_position(array(1), 'foo');
null
```
We should safely coerce both left and right hand side expressions.
## How was this patch tested?
Added tests in DataFrameFunctionsSuite
Closes#22407 from dilipbiswal/SPARK-25416.
Authored-by: Dilip Biswal <dbiswal@us.ibm.com>
Signed-off-by: Wenchen Fan <wenchen@databricks.com>
## What changes were proposed in this pull request?
The function actually exists in current selected database, and it's failed to init during `lookupFunciton`, but the exception message is:
```
This function is neither a registered temporary function nor a permanent function registered in the database 'default'.
```
This is not conducive to positioning problems. This PR fix the problem.
## How was this patch tested?
new test case + manual tests
Closes#18544 from stanzhai/fix-udf-error-message.
Authored-by: Stan Zhai <mail@stanzhai.site>
Signed-off-by: Wenchen Fan <wenchen@databricks.com>
## What changes were proposed in this pull request?
Add `Locale.ROOT` when `toUpperCase`.
## How was this patch tested?
manual tests
Closes#22531 from wangyum/SPARK-25415.
Authored-by: Yuming Wang <yumwang@ebay.com>
Signed-off-by: hyukjinkwon <gurwls223@apache.org>
One more legacy config to go ...
Closes#22515 from rxin/allowCreatingManagedTableUsingNonemptyLocation.
Authored-by: Reynold Xin <rxin@databricks.com>
Signed-off-by: gatorsmile <gatorsmile@gmail.com>
## What changes were proposed in this pull request?
Currently there are two classes with the same naming BenchmarkBase:
1. `org.apache.spark.util.BenchmarkBase`
2. `org.apache.spark.sql.execution.benchmark.BenchmarkBase`
This is very confusing. And the benchmark object `org.apache.spark.sql.execution.benchmark.FilterPushdownBenchmark` is using the one in `org.apache.spark.util.BenchmarkBase`, while there is another class `BenchmarkBase` in the same package of it...
Here I propose:
1. the package `org.apache.spark.util.BenchmarkBase` should be in test package of core module. Move it to package `org.apache.spark.benchmark` .
2. Move `org.apache.spark.util.Benchmark` to test package of core module. Move it to package `org.apache.spark.benchmark` .
3. Rename the class `org.apache.spark.sql.execution.benchmark.BenchmarkBase` as `BenchmarkWithCodegen`
## How was this patch tested?
Unit test
Closes#22513 from gengliangwang/refactorBenchmarkBase.
Authored-by: Gengliang Wang <gengliang.wang@databricks.com>
Signed-off-by: Wenchen Fan <wenchen@databricks.com>
## What changes were proposed in this pull request?
The PR proposes to avoid usage of pattern matching for each call of ```eval``` method within:
- ```Concat```
- ```Reverse```
- ```ElementAt```
## How was this patch tested?
Run the existing tests for ```Concat```, ```Reverse``` and ```ElementAt``` expression classes.
Closes#22471 from mn-mikke/SPARK-25470.
Authored-by: Marek Novotny <mn.mikke@gmail.com>
Signed-off-by: Takeshi Yamamuro <yamamuro@apache.org>
## What changes were proposed in this pull request?
See title. Makes our legacy backward compatibility configs more consistent.
## How was this patch tested?
Make sure all references have been updated:
```
> git grep compareDateTimestampInTimestamp
docs/sql-programming-guide.md: - Since Spark 2.4, Spark compares a DATE type with a TIMESTAMP type after promotes both sides to TIMESTAMP. To set `false` to `spark.sql.legacy.compareDateTimestampInTimestamp` restores the previous behavior. This option will be removed in Spark 3.0.
sql/catalyst/src/main/scala/org/apache/spark/sql/catalyst/analysis/TypeCoercion.scala: // if conf.compareDateTimestampInTimestamp is true
sql/catalyst/src/main/scala/org/apache/spark/sql/catalyst/analysis/TypeCoercion.scala: => if (conf.compareDateTimestampInTimestamp) Some(TimestampType) else Some(StringType)
sql/catalyst/src/main/scala/org/apache/spark/sql/catalyst/analysis/TypeCoercion.scala: => if (conf.compareDateTimestampInTimestamp) Some(TimestampType) else Some(StringType)
sql/catalyst/src/main/scala/org/apache/spark/sql/internal/SQLConf.scala: buildConf("spark.sql.legacy.compareDateTimestampInTimestamp")
sql/catalyst/src/main/scala/org/apache/spark/sql/internal/SQLConf.scala: def compareDateTimestampInTimestamp : Boolean = getConf(COMPARE_DATE_TIMESTAMP_IN_TIMESTAMP)
sql/catalyst/src/test/scala/org/apache/spark/sql/catalyst/analysis/TypeCoercionSuite.scala: "spark.sql.legacy.compareDateTimestampInTimestamp" -> convertToTS.toString) {
```
Closes#22508 from rxin/SPARK-23549.
Authored-by: Reynold Xin <rxin@databricks.com>
Signed-off-by: Wenchen Fan <wenchen@databricks.com>
See above. This should go into the 2.4 release.
Closes#22509 from rxin/SPARK-25384.
Authored-by: Reynold Xin <rxin@databricks.com>
Signed-off-by: Wenchen Fan <wenchen@databricks.com>
## What changes were proposed in this pull request?
This reverts commit 417ad92502.
We decided to keep the current behaviors unchanged and will consider whether we will deprecate the these functions in 3.0. For more details, see the discussion in https://issues.apache.org/jira/browse/SPARK-23715
## How was this patch tested?
The existing tests.
Closes#22505 from gatorsmile/revertSpark-23715.
Authored-by: gatorsmile <gatorsmile@gmail.com>
Signed-off-by: Wenchen Fan <wenchen@databricks.com>
## What changes were proposed in this pull request?
The problem was cause by the PushProjectThroughUnion rule, which, when creating new Project for each child of Union, uses the same exprId for expressions of the same position. This is wrong because, for each child of Union, the expressions are all independent, and it can lead to a wrong result if other rules like FoldablePropagation kicks in, taking two different expressions as the same.
This fix is to create new expressions in the new Project for each child of Union.
## How was this patch tested?
Added UT.
Closes#22447 from maryannxue/push-project-thru-union-bug.
Authored-by: maryannxue <maryannxue@apache.org>
Signed-off-by: gatorsmile <gatorsmile@gmail.com>
## What changes were proposed in this pull request?
In ArrayContains, we currently cast the right hand side expression to match the element type of the left hand side Array. This may result in down casting and may return wrong result or questionable result.
Example :
```SQL
spark-sql> select array_contains(array(1), 1.34);
true
```
```SQL
spark-sql> select array_contains(array(1), 'foo');
null
```
We should safely coerce both left and right hand side expressions.
## How was this patch tested?
Added tests in DataFrameFunctionsSuite
Closes#22408 from dilipbiswal/SPARK-25417.
Authored-by: Dilip Biswal <dbiswal@us.ibm.com>
Signed-off-by: Wenchen Fan <wenchen@databricks.com>
## What changes were proposed in this pull request?
This goes to revert sequential PRs based on some discussion and comments at https://github.com/apache/spark/pull/16677#issuecomment-422650759.
#22344#22330#22239#16677
## How was this patch tested?
Existing tests.
Closes#22481 from viirya/revert-SPARK-19355-1.
Authored-by: Liang-Chi Hsieh <viirya@gmail.com>
Signed-off-by: Wenchen Fan <wenchen@databricks.com>
## What changes were proposed in this pull request?
This patch adds an "optimizer" prefix to nested schema pruning.
## How was this patch tested?
Should be covered by existing tests.
Closes#22475 from rxin/SPARK-4502.
Authored-by: Reynold Xin <rxin@databricks.com>
Signed-off-by: gatorsmile <gatorsmile@gmail.com>
## What changes were proposed in this pull request?
The PR proposes to return the data type of the operands as a result for the `div` operator. Before the PR, `bigint` is always returned. It introduces also a `spark.sql.legacy.integralDivide.returnBigint` config in order to let the users restore the legacy behavior.
## How was this patch tested?
added UTs
Closes#22465 from mgaido91/SPARK-25457.
Authored-by: Marco Gaido <marcogaido91@gmail.com>
Signed-off-by: Wenchen Fan <wenchen@databricks.com>
## What changes were proposed in this pull request?
This patch changes the config option `spark.sql.streaming.noDataMicroBatchesEnabled` to `spark.sql.streaming.noDataMicroBatches.enabled` to be more consistent with rest of the configs. Unfortunately there is one streaming config called `spark.sql.streaming.metricsEnabled`. For that one we should just use a fallback config and change it in a separate patch.
## How was this patch tested?
Made sure no other references to this config are in the code base:
```
> git grep "noDataMicro"
sql/catalyst/src/main/scala/org/apache/spark/sql/internal/SQLConf.scala: buildConf("spark.sql.streaming.noDataMicroBatches.enabled")
```
Closes#22476 from rxin/SPARK-24157.
Authored-by: Reynold Xin <rxin@databricks.com>
Signed-off-by: Reynold Xin <rxin@databricks.com>
## What changes were proposed in this pull request?
In SPARK-23711, `UnsafeProjection` supports fallback to an interpreted mode. Therefore, this pr fixed code to support the same fallback mode in `MutableProjection` based on `CodeGeneratorWithInterpretedFallback`.
## How was this patch tested?
Added tests in `CodeGeneratorWithInterpretedFallbackSuite`.
Closes#22355 from maropu/SPARK-25358.
Authored-by: Takeshi Yamamuro <yamamuro@apache.org>
Signed-off-by: Wenchen Fan <wenchen@databricks.com>
## What changes were proposed in this pull request?
To be more consistent with other statistics based configs.
## How was this patch tested?
N/A - straightforward rename of config option. Used `git grep` to make sure there are no mention of it.
Closes#22457 from rxin/SPARK-24626.
Authored-by: Reynold Xin <rxin@databricks.com>
Signed-off-by: gatorsmile <gatorsmile@gmail.com>
## What changes were proposed in this pull request?
`spark.sql.fromJsonForceNullableSchema` -> `spark.sql.function.fromJson.forceNullable`
## How was this patch tested?
Made sure there are no more references to `spark.sql.fromJsonForceNullableSchema`.
Closes#22459 from rxin/SPARK-23173.
Authored-by: Reynold Xin <rxin@databricks.com>
Signed-off-by: gatorsmile <gatorsmile@gmail.com>
## What changes were proposed in this pull request?
SPARK-22333 introduced a regression in the resolution of `CURRENT_DATE` and `CURRENT_TIMESTAMP`. Before that ticket, these 2 functions were resolved in a case insensitive way. After, this depends on the value of `spark.sql.caseSensitive`.
The PR restores the previous behavior and makes their resolution case insensitive anyhow. The PR takes over #21217, therefore it closes#21217 and credit for this patch should be given to jamesthomp.
## How was this patch tested?
added UT
Closes#22440 from mgaido91/SPARK-24151.
Lead-authored-by: James Thompson <jamesthomp@users.noreply.github.com>
Co-authored-by: Marco Gaido <marcogaido91@gmail.com>
Signed-off-by: gatorsmile <gatorsmile@gmail.com>
## What changes were proposed in this pull request?
This PR makes `GenArrayData.genCodeToCreateArrayData` method simple by using `ArrayData.createArrayData` method.
Before this PR, `genCodeToCreateArrayData` method was complicated
* Generated a temporary Java array to create `ArrayData`
* Had separate code generation path to assign values for `GenericArrayData` and `UnsafeArrayData`
After this PR, the method
* Directly generates `GenericArrayData` or `UnsafeArrayData` without a temporary array
* Has only code generation path to assign values
## How was this patch tested?
Existing UTs
Closes#22439 from kiszk/SPARK-25444.
Authored-by: Kazuaki Ishizaki <ishizaki@jp.ibm.com>
Signed-off-by: Takuya UESHIN <ueshin@databricks.com>
## What changes were proposed in this pull request?
The PR takes over #14036 and it introduces a new expression `IntegralDivide` in order to avoid the several unneded cast added previously.
In order to prove the performance gain, the following benchmark has been run:
```
test("Benchmark IntegralDivide") {
val r = new scala.util.Random(91)
val nData = 1000000
val testDataInt = (1 to nData).map(_ => (r.nextInt(), r.nextInt()))
val testDataLong = (1 to nData).map(_ => (r.nextLong(), r.nextLong()))
val testDataShort = (1 to nData).map(_ => (r.nextInt().toShort, r.nextInt().toShort))
// old code
val oldExprsInt = testDataInt.map(x =>
Cast(Divide(Cast(Literal(x._1), DoubleType), Cast(Literal(x._2), DoubleType)), LongType))
val oldExprsLong = testDataLong.map(x =>
Cast(Divide(Cast(Literal(x._1), DoubleType), Cast(Literal(x._2), DoubleType)), LongType))
val oldExprsShort = testDataShort.map(x =>
Cast(Divide(Cast(Literal(x._1), DoubleType), Cast(Literal(x._2), DoubleType)), LongType))
// new code
val newExprsInt = testDataInt.map(x => IntegralDivide(x._1, x._2))
val newExprsLong = testDataLong.map(x => IntegralDivide(x._1, x._2))
val newExprsShort = testDataShort.map(x => IntegralDivide(x._1, x._2))
Seq(("Long", "old", oldExprsLong),
("Long", "new", newExprsLong),
("Int", "old", oldExprsInt),
("Int", "new", newExprsShort),
("Short", "old", oldExprsShort),
("Short", "new", oldExprsShort)).foreach { case (dt, t, ds) =>
val start = System.nanoTime()
ds.foreach(e => e.eval(EmptyRow))
val endNoCodegen = System.nanoTime()
println(s"Running $nData op with $t code on $dt (no-codegen): ${(endNoCodegen - start) / 1000000} ms")
}
}
```
The results on my laptop are:
```
Running 1000000 op with old code on Long (no-codegen): 600 ms
Running 1000000 op with new code on Long (no-codegen): 112 ms
Running 1000000 op with old code on Int (no-codegen): 560 ms
Running 1000000 op with new code on Int (no-codegen): 135 ms
Running 1000000 op with old code on Short (no-codegen): 317 ms
Running 1000000 op with new code on Short (no-codegen): 153 ms
```
Showing a 2-5X improvement. The benchmark doesn't include code generation as it is pretty hard to test the performance there as for such simple operations the most of the time is spent in the code generation/compilation process.
## How was this patch tested?
added UTs
Closes#22395 from mgaido91/SPARK-16323.
Authored-by: Marco Gaido <marcogaido91@gmail.com>
Signed-off-by: Dongjoon Hyun <dongjoon@apache.org>
## What changes were proposed in this pull request?
There are some mistakes in examples of newly added functions. Also the format of the example results are not unified. We should fix them.
## How was this patch tested?
Manually executed the examples.
Closes#22437 from ueshin/issues/SPARK-25431/fix_examples_2.
Authored-by: Takuya UESHIN <ueshin@databricks.com>
Signed-off-by: hyukjinkwon <gurwls223@apache.org>
## What changes were proposed in this pull request?
In the dev list, we can still discuss whether the next version is 2.5.0 or 3.0.0. Let us first bump the master branch version to `2.5.0-SNAPSHOT`.
## How was this patch tested?
N/A
Closes#22426 from gatorsmile/bumpVersionMaster.
Authored-by: gatorsmile <gatorsmile@gmail.com>
Signed-off-by: gatorsmile <gatorsmile@gmail.com>
## What changes were proposed in this pull request?
This pr removed the duplicate fallback logic in `UnsafeProjection`.
This pr comes from #22355.
## How was this patch tested?
Added tests in `CodeGeneratorWithInterpretedFallbackSuite`.
Closes#22417 from maropu/SPARK-25426.
Authored-by: Takeshi Yamamuro <yamamuro@apache.org>
Signed-off-by: gatorsmile <gatorsmile@gmail.com>
## What changes were proposed in this pull request?
There are some mistakes in examples of newly added functions. Also the format of the example results are not unified. We should fix and unify them.
## How was this patch tested?
Manually executed the examples.
Closes#22421 from ueshin/issues/SPARK-25431/fix_examples.
Authored-by: Takuya UESHIN <ueshin@databricks.com>
Signed-off-by: gatorsmile <gatorsmile@gmail.com>
## What changes were proposed in this pull request?
In RuleExecutor, after applying a rule, if the plan has changed, the before and after plan will be logged using level "trace". At times, however, such information can be very helpful for debugging. Hence, making the log level configurable in SQLConf would allow users to turn on the plan change log independently and save the trouble of tweaking log4j settings. Meanwhile, filtering plan change log for specific rules can also be very useful.
So this PR adds two SQL configurations:
1. spark.sql.optimizer.planChangeLog.level - set a specific log level for logging plan changes after a rule is applied.
2. spark.sql.optimizer.planChangeLog.rules - enable plan change logging only for a set of specified rules, separated by commas.
## How was this patch tested?
Added UT.
Closes#22406 from maryannxue/spark-25415.
Authored-by: maryannxue <maryannxue@apache.org>
Signed-off-by: gatorsmile <gatorsmile@gmail.com>
## What changes were proposed in this pull request?
This PR is to fix the null handling in BooleanSimplification. In the rule BooleanSimplification, there are two cases that do not properly handle null values. The optimization is not right if either side is null. This PR is to fix them.
## How was this patch tested?
Added test cases
Closes#22390 from gatorsmile/fixBooleanSimplification.
Authored-by: gatorsmile <gatorsmile@gmail.com>
Signed-off-by: Wenchen Fan <wenchen@databricks.com>
## What changes were proposed in this pull request?
Correct some comparisons between unrelated types to what they seem to… have been trying to do
## How was this patch tested?
Existing tests.
Closes#22384 from srowen/SPARK-25398.
Authored-by: Sean Owen <sean.owen@databricks.com>
Signed-off-by: Sean Owen <sean.owen@databricks.com>
## What changes were proposed in this pull request?
SPARK-21281 introduced a check for the inputs of `CreateStructLike` to be non-empty. This means that `struct()`, which was previously considered valid, now throws an Exception. This behavior change was introduced in 2.3.0. The change may break users' application on upgrade and it causes `VectorAssembler` to fail when an empty `inputCols` is defined.
The PR removes the added check making `struct()` valid again.
## How was this patch tested?
added UT
Closes#22373 from mgaido91/SPARK-25371.
Authored-by: Marco Gaido <marcogaido91@gmail.com>
Signed-off-by: Wenchen Fan <wenchen@databricks.com>
## What changes were proposed in this pull request?
In the Planner, we collect the placeholder which need to be substituted in the query execution plan and once we plan them, we substitute the placeholder with the effective plan.
In this second phase, we rely on the `==` comparison, ie. the `equals` method. This means that if two placeholder plans - which are different instances - have the same attributes (so that they are equal, according to the equal method) they are both substituted with their corresponding new physical plans. So, in such a situation, the first time we substitute both them with the first of the 2 new generated plan and the second time we substitute nothing.
This is usually of no harm for the execution of the query itself, as the 2 plans are identical. But since they are the same instance, now, the local variables are shared (which is unexpected). This causes issues for the metrics collected, as the same node is executed 2 times, so the metrics are accumulated 2 times, wrongly.
The PR proposes to use the `eq` method in checking which placeholder needs to be substituted,; thus in the previous situation, actually both the two different physical nodes which are created (one for each time the logical plan appears in the query plan) are used and the metrics are collected properly for each of them.
## How was this patch tested?
added UT
Closes#22284 from mgaido91/SPARK-25278.
Authored-by: Marco Gaido <marcogaido91@gmail.com>
Signed-off-by: Wenchen Fan <wenchen@databricks.com>
## What changes were proposed in this pull request?
Add the version number for the new APIs.
## How was this patch tested?
N/A
Closes#22377 from gatorsmile/followup24849.
Authored-by: gatorsmile <gatorsmile@gmail.com>
Signed-off-by: Wenchen Fan <wenchen@databricks.com>