## What changes were proposed in this pull request?
Remove spark.memory.useLegacyMode and StaticMemoryManager. Update tests that used the StaticMemoryManager to equivalent use of UnifiedMemoryManager.
## How was this patch tested?
Existing tests, with modifications to make them work with a different mem manager.
Closes#23457 from srowen/SPARK-26539.
Authored-by: Sean Owen <sean.owen@databricks.com>
Signed-off-by: Sean Owen <sean.owen@databricks.com>
## What changes were proposed in this pull request?
This is a followup of https://github.com/apache/spark/pull/18576
The newly added rule `UpdateNullabilityInAttributeReferences` does the same thing the `FixNullability` does, we only need to keep one of them.
This PR removes `UpdateNullabilityInAttributeReferences`, and use `FixNullability` to replace it. Also rename it to `UpdateAttributeNullability`
## How was this patch tested?
existing tests
Closes#23390 from cloud-fan/nullable.
Authored-by: Wenchen Fan <wenchen@databricks.com>
Signed-off-by: Takeshi Yamamuro <yamamuro@apache.org>
## What changes were proposed in this pull request?
Added a cache for java.time.format.DateTimeFormatter instances with keys consist of pattern and locale. This should allow to avoid parsing of timestamp/date patterns each time when new instance of `TimestampFormatter`/`DateFormatter` is created.
## How was this patch tested?
By existing test suites `TimestampFormatterSuite`/`DateFormatterSuite` and `JsonFunctionsSuite`/`JsonSuite`.
Closes#23462 from MaxGekk/time-formatter-caching.
Lead-authored-by: Maxim Gekk <max.gekk@gmail.com>
Co-authored-by: Maxim Gekk <maxim.gekk@databricks.com>
Signed-off-by: Hyukjin Kwon <gurwls223@apache.org>
## What changes were proposed in this pull request?
Allow multiple spark.sql.extensions to be specified in the
configuration.
## How was this patch tested?
New tests are added.
Closes#23398 from jamisonbennett/SPARK-26493.
Authored-by: Jamison Bennett <jamison.bennett@gmail.com>
Signed-off-by: Hyukjin Kwon <gurwls223@apache.org>
## What changes were proposed in this pull request?
This is to fix a bug in https://github.com/apache/spark/pull/23036, which would lead to an exception in case of two consecutive hints.
## How was this patch tested?
Added a new test.
Closes#23501 from maryannxue/query-hint-followup.
Authored-by: maryannxue <maryannxue@apache.org>
Signed-off-by: gatorsmile <gatorsmile@gmail.com>
## What changes were proposed in this pull request?
In https://github.com/apache/spark/pull/23043 , we introduced a behavior change: Spark users are not able to distinguish 0.0 and -0.0 anymore.
This PR proposes an alternative fix to the original bug, to retain the difference between 0.0 and -0.0 inside Spark.
The idea is, we can rewrite the window partition key, join key and grouping key during logical phase, to normalize the special floating numbers. Thus only operators care about special floating numbers need to pay the perf overhead, and end users can distinguish -0.0.
## How was this patch tested?
existing test
Closes#23388 from cloud-fan/minor.
Authored-by: Wenchen Fan <wenchen@databricks.com>
Signed-off-by: gatorsmile <gatorsmile@gmail.com>
## What changes were proposed in this pull request?
Refactor ExternalAppendOnlyUnsafeRowArrayBenchmark to use main method.
## How was this patch tested?
Manually tested and regenerated results.
Please note that `spark.memory.debugFill` setting has a huge impact on this benchmark. Since it is set to true by default when running the benchmark from SBT, we need to disable it:
```
SPARK_GENERATE_BENCHMARK_FILES=1 build/sbt ";project sql;set javaOptions in Test += \"-Dspark.memory.debugFill=false\";test:runMain org.apache.spark.sql.execution.ExternalAppendOnlyUnsafeRowArrayBenchmark"
```
Closes#22617 from peter-toth/SPARK-25484.
Lead-authored-by: Peter Toth <peter.toth@gmail.com>
Co-authored-by: Peter Toth <ptoth@hortonworks.com>
Co-authored-by: Dongjoon Hyun <dongjoon@apache.org>
Signed-off-by: Dongjoon Hyun <dongjoon@apache.org>
## What changes were proposed in this pull request?
Currently Spark table maintains Hive catalog storage format, so that Hive client can read it. In `HiveSerDe.scala`, Spark uses a mapping from its data source to HiveSerde. The mapping is old, we need to update with latest canonical name of Parquet and Orc FileFormat.
Otherwise the following queries will result in wrong Serde value in Hive table(default value `org.apache.hadoop.mapred.SequenceFileInputFormat`), and Hive client will fail to read the output table:
```
df.write.format("org.apache.spark.sql.execution.datasources.parquet.ParquetFileFormat").saveAsTable(..)
```
```
df.write.format("org.apache.spark.sql.execution.datasources.orc.OrcFileFormat").saveAsTable(..)
```
This minor PR is to fix the mapping.
## How was this patch tested?
Unit test.
Closes#23491 from gengliangwang/fixHiveSerdeMap.
Authored-by: Gengliang Wang <gengliang.wang@databricks.com>
Signed-off-by: Wenchen Fan <wenchen@databricks.com>
Currently there is code scattered in a bunch of places to do different
things related to HTTP security, such as access control, setting
security-related headers, and filtering out bad content. This makes it
really easy to miss these things when writing new UI code.
This change creates a new filter that does all of those things, and
makes sure that all servlet handlers that are attached to the UI get
the new filter and any user-defined filters consistently. The extent
of the actual features should be the same as before.
The new filter is added at the end of the filter chain, because authentication
is done by custom filters and thus needs to happen first. This means that
custom filters see unfiltered HTTP requests - which is actually the current
behavior anyway.
As a side-effect of some of the code refactoring, handlers added after
the initial set also get wrapped with a GzipHandler, which didn't happen
before.
Tested with added unit tests and in a history server with SPNEGO auth
configured.
Closes#23302 from vanzin/SPARK-24522.
Authored-by: Marcelo Vanzin <vanzin@cloudera.com>
Signed-off-by: Imran Rashid <irashid@cloudera.com>
## What changes were proposed in this pull request?
Fixing leap year calculations for date operators (year/month/dayOfYear) where the Julian calendars are used (before 1582-10-04). In a Julian calendar every years which are multiples of 4 are leap years (there is no extra exception for years multiples of 100).
## How was this patch tested?
With a unit test ("SPARK-26002: correct day of year calculations for Julian calendar years") which focuses to these corner cases.
Manually:
```
scala> sql("select year('1500-01-01')").show()
+------------------------------+
|year(CAST(1500-01-01 AS DATE))|
+------------------------------+
| 1500|
+------------------------------+
scala> sql("select dayOfYear('1100-01-01')").show()
+-----------------------------------+
|dayofyear(CAST(1100-01-01 AS DATE))|
+-----------------------------------+
| 1|
+-----------------------------------+
```
Closes#23000 from attilapiros/julianOffByDays.
Authored-by: “attilapiros” <piros.attila.zsolt@gmail.com>
Signed-off-by: Wenchen Fan <wenchen@databricks.com>
## What changes were proposed in this pull request?
For Scala UDF, when checking input nullability, we will skip inputs with type `Any`, and only check the inputs that provide nullability info.
We should do the same for checking input types.
## How was this patch tested?
new tests
Closes#23275 from cloud-fan/udf.
Authored-by: Wenchen Fan <wenchen@databricks.com>
Signed-off-by: Hyukjin Kwon <gurwls223@apache.org>
## What changes were proposed in this pull request?
The PR https://github.com/apache/spark/pull/23446 happened to introduce a behaviour change - empty dataframes can't be read anymore from underscore files. It looks controversial to allow or disallow this case so this PR targets to fix to issue warning instead of throwing an exception to be more conservative.
**Before**
```scala
scala> spark.read.schema("a int").parquet("_tmp*").show()
org.apache.spark.sql.AnalysisException: All paths were ignored:
file:/.../_tmp
file:/.../_tmp1;
at org.apache.spark.sql.execution.datasources.DataSource.checkAndGlobPathIfNecessary(DataSource.scala:570)
at org.apache.spark.sql.execution.datasources.DataSource.resolveRelation(DataSource.scala:360)
at org.apache.spark.sql.DataFrameReader.loadV1Source(DataFrameReader.scala:231)
at org.apache.spark.sql.DataFrameReader.load(DataFrameReader.scala:219)
at org.apache.spark.sql.DataFrameReader.parquet(DataFrameReader.scala:651)
at org.apache.spark.sql.DataFrameReader.parquet(DataFrameReader.scala:635)
... 49 elided
scala> spark.read.text("_tmp*").show()
org.apache.spark.sql.AnalysisException: All paths were ignored:
file:/.../_tmp
file:/.../_tmp1;
at org.apache.spark.sql.execution.datasources.DataSource.checkAndGlobPathIfNecessary(DataSource.scala:570)
at org.apache.spark.sql.execution.datasources.DataSource.resolveRelation(DataSource.scala:360)
at org.apache.spark.sql.DataFrameReader.loadV1Source(DataFrameReader.scala:231)
at org.apache.spark.sql.DataFrameReader.load(DataFrameReader.scala:219)
at org.apache.spark.sql.DataFrameReader.text(DataFrameReader.scala:723)
at org.apache.spark.sql.DataFrameReader.text(DataFrameReader.scala:695)
... 49 elided
```
**After**
```scala
scala> spark.read.schema("a int").parquet("_tmp*").show()
19/01/07 15:14:43 WARN DataSource: All paths were ignored:
file:/.../_tmp
file:/.../_tmp1
+---+
| a|
+---+
+---+
scala> spark.read.text("_tmp*").show()
19/01/07 15:14:51 WARN DataSource: All paths were ignored:
file:/.../_tmp
file:/.../_tmp1
+-----+
|value|
+-----+
+-----+
```
## How was this patch tested?
Manually tested as above.
Closes#23481 from HyukjinKwon/SPARK-26339.
Authored-by: Hyukjin Kwon <gurwls223@apache.org>
Signed-off-by: gatorsmile <gatorsmile@gmail.com>
## What changes were proposed in this pull request?
The PR makes hardcoded `spark.test` and `spark.testing` configs to use `ConfigEntry` and put them in the config package.
## How was this patch tested?
existing UTs
Closes#23413 from mgaido91/SPARK-26491.
Authored-by: Marco Gaido <marcogaido91@gmail.com>
Signed-off-by: Marcelo Vanzin <vanzin@cloudera.com>
## What changes were proposed in this pull request?
The existing query hint implementation relies on a logical plan node `ResolvedHint` to store query hints in logical plans, and on `Statistics` in physical plans. Since `ResolvedHint` is not really a logical operator and can break the pattern matching for existing and future optimization rules, it is a issue to the Optimizer as the old `AnalysisBarrier` was to the Analyzer.
Given the fact that all our query hints are either 1) a join hint, i.e., broadcast hint; or 2) a re-partition hint, which is indeed an operator, we only need to add a hint field on the Join plan and that will be a good enough solution for the current hint usage.
This PR is to let `Join` node have a hint for its left sub-tree and another hint for its right sub-tree and each hint is a merged result of all the effective hints specified in the corresponding sub-tree. The "effectiveness" of a hint, i.e., whether that hint should be propagated to the `Join` node, is currently consistent with the hint propagation rules originally implemented in the `Statistics` approach. Note that the `ResolvedHint` node still has to live through the analysis stage because of the `Dataset` interface, but it will be got rid of and moved to the `Join` node in the "pre-optimization" stage.
This PR also introduces a change in how hints work with join reordering. Before this PR, hints would stop join reordering. For example, in "a.join(b).join(c).hint("broadcast").join(d)", the broadcast hint would stop d from participating in the cost-based join reordering while still allowing reordering from under the hint node. After this PR, though, the broadcast hint will not interfere with join reordering at all, and after reordering if a relation associated with a hint stays unchanged or equivalent to the original relation, the hint will be retained, otherwise will be discarded. For example, the original plan is like "a.join(b).hint("broadcast").join(c).hint("broadcast").join(d)", thus the join order is "a JOIN b JOIN c JOIN d". So if after reordering the join order becomes "a JOIN b JOIN (c JOIN d)", the plan will be like "a.join(b).hint("broadcast").join(c.join(d))"; but if after reordering the join order becomes "a JOIN c JOIN b JOIN d", the plan will be like "a.join(c).join(b).hint("broadcast").join(d)".
## How was this patch tested?
Added new tests.
Closes#23036 from maryannxue/query-hint.
Authored-by: maryannxue <maryannxue@apache.org>
Signed-off-by: gatorsmile <gatorsmile@gmail.com>
### What changes were proposed in this pull request?
When passing wrong url to jdbc then It would throw IllegalArgumentException instead of NPE.
### How was this patch tested?
Adding test case to Existing tests in JDBCSuite
Closes#23464 from ayudovin/fixing-npe.
Authored-by: ayudovin <a.yudovin6695@gmail.com>
Signed-off-by: Sean Owen <sean.owen@databricks.com>
## What changes were proposed in this pull request?
`StreamingReadSupport` is designed to be a `package` interface. Mockito seems to complain during `Maven` testing. This doesn't fail in `sbt` and IntelliJ. For mock-testing purpose, this PR makes it `public` interface and adds explicit comments like `public interface ReadSupport`
```scala
EpochCoordinatorSuite:
*** RUN ABORTED ***
java.lang.IllegalAccessError: tried to
access class org.apache.spark.sql.sources.v2.reader.streaming.StreamingReadSupport
from class org.apache.spark.sql.sources.v2.reader.streaming.ContinuousReadSupport$MockitoMock$58628338
at org.apache.spark.sql.sources.v2.reader.streaming.ContinuousReadSupport$MockitoMock$58628338.<clinit>(Unknown Source)
at sun.reflect.GeneratedSerializationConstructorAccessor632.newInstance(Unknown Source)
at java.lang.reflect.Constructor.newInstance(Constructor.java:423)
at org.objenesis.instantiator.sun.SunReflectionFactoryInstantiator.newInstance(SunReflectionFactoryInstantiator.java:48)
at org.objenesis.ObjenesisBase.newInstance(ObjenesisBase.java:73)
at org.mockito.internal.creation.instance.ObjenesisInstantiator.newInstance(ObjenesisInstantiator.java:19)
at org.mockito.internal.creation.bytebuddy.SubclassByteBuddyMockMaker.createMock(SubclassByteBuddyMockMaker.java:47)
at org.mockito.internal.creation.bytebuddy.ByteBuddyMockMaker.createMock(ByteBuddyMockMaker.java:25)
at org.mockito.internal.util.MockUtil.createMock(MockUtil.java:35)
at org.mockito.internal.MockitoCore.mock(MockitoCore.java:69)
```
## How was this patch tested?
Pass the Jenkins with Maven build
Closes#23463 from dongjoon-hyun/SPARK-26536-2.
Authored-by: Dongjoon Hyun <dongjoon@apache.org>
Signed-off-by: Dongjoon Hyun <dongjoon@apache.org>
## What changes were proposed in this pull request?
The `toHiveString()` and `toHiveStructString` methods were removed from `HiveUtils` because they have been already implemented in `HiveResult`. One related test was moved to `HiveResultSuite`.
## How was this patch tested?
By tests from `hive-thriftserver`.
Closes#23466 from MaxGekk/dedup-hive-result-string.
Authored-by: Maxim Gekk <max.gekk@gmail.com>
Signed-off-by: Dongjoon Hyun <dongjoon@apache.org>
## What changes were proposed in this pull request?
My pull request #23288 was resolved and merged to master, but it turned out later that my change breaks another regression test. Because we cannot reopen pull request, I create a new pull request here.
Commit 92934b4 is only change after pull request #23288.
`CheckFileExist` was avoided at 239cfa4 after discussing #23288 (comment).
But, that change turned out to be wrong because we should not check if argument checkFileExist is false.
Test 27e42c1de5/sql/core/src/test/scala/org/apache/spark/sql/SQLQuerySuite.scala (L2555)
failed when we avoided checkFileExist, but now successed after commit 92934b4 .
## How was this patch tested?
Both of below tests were passed.
```
testOnly org.apache.spark.sql.execution.datasources.csv.CSVSuite
testOnly org.apache.spark.sql.SQLQuerySuite
```
Closes#23446 from KeiichiHirobe/SPARK-26339.
Authored-by: Hirobe Keiichi <keiichi_hirobe@forcia.com>
Signed-off-by: Sean Owen <sean.owen@databricks.com>
## What changes were proposed in this pull request?
Address SPARK-26548, in Spark 2.4.0, the CacheManager holds a write lock while computing the executedPlan for a cached logicalPlan. In some cases with very large query plans this can be an expensive operation, taking minutes to run. The entire cache is blocked during this time. This PR changes that so the writeLock is only obtained after the executedPlan is generated, this reduces the time the lock is held to just the necessary time when the shared data structure is being updated.
gatorsmile and cloud-fan - You can committed patches in this area before. This is a small incremental change.
## How was this patch tested?
Has been tested on a live system where the blocking was causing major issues and it is working well.
CacheManager has no explicit unit test but is used in many places internally as part of the SharedState.
Closes#23469 from DaveDeCaprio/optimizer-unblocked.
Lead-authored-by: Dave DeCaprio <daved@alum.mit.edu>
Co-authored-by: David DeCaprio <daved@alum.mit.edu>
Signed-off-by: gatorsmile <gatorsmile@gmail.com>
## What changes were proposed in this pull request?
The truth table comment in EqualNullSafe incorrectly marked FALSE results as UNKNOWN.
## How was this patch tested?
N/A
Closes#23461 from rednaxelafx/fix-typo.
Authored-by: Kris Mok <kris.mok@databricks.com>
Signed-off-by: gatorsmile <gatorsmile@gmail.com>
## What changes were proposed in this pull request?
Added new JSON option `inferTimestamp` (`true` by default) to control inferring of `TimestampType` from string values.
## How was this patch tested?
Add new UT to `JsonInferSchemaSuite`.
Closes#23455 from MaxGekk/json-infer-time-followup.
Authored-by: Maxim Gekk <maxim.gekk@databricks.com>
Signed-off-by: Hyukjin Kwon <gurwls223@apache.org>
## What changes were proposed in this pull request?
While backporting the patch to 2.4/2.3, I realized that the patch introduces unneeded imports (probably leftovers from intermediate changes). This PR removes the useless import.
## How was this patch tested?
NA
Closes#23451 from mgaido91/SPARK-26078_FOLLOWUP.
Authored-by: Marco Gaido <marcogaido91@gmail.com>
Signed-off-by: Dongjoon Hyun <dongjoon@apache.org>
## What changes were proposed in this pull request?
This PR upgrades Mockito from 1.10.19 to 2.23.4. The following changes are required.
- Replace `org.mockito.Matchers` with `org.mockito.ArgumentMatchers`
- Replace `anyObject` with `any`
- Replace `getArgumentAt` with `getArgument` and add type annotation.
- Use `isNull` matcher in case of `null` is invoked.
```scala
saslHandler.channelInactive(null);
- verify(handler).channelInactive(any(TransportClient.class));
+ verify(handler).channelInactive(isNull());
```
- Make and use `doReturn` wrapper to avoid [SI-4775](https://issues.scala-lang.org/browse/SI-4775)
```scala
private def doReturn(value: Any) = org.mockito.Mockito.doReturn(value, Seq.empty: _*)
```
## How was this patch tested?
Pass the Jenkins with the existing tests.
Closes#23452 from dongjoon-hyun/SPARK-26536.
Authored-by: Dongjoon Hyun <dongjoon@apache.org>
Signed-off-by: Dongjoon Hyun <dongjoon@apache.org>
## What changes were proposed in this pull request?
Increase test memory to avoid OOM in TimSort-related tests.
## How was this patch tested?
Existing tests.
Closes#23425 from srowen/SPARK-26306.
Authored-by: Sean Owen <sean.owen@databricks.com>
Signed-off-by: Sean Owen <sean.owen@databricks.com>
## What changes were proposed in this pull request?
Test case in SPARK-10316 is used to make sure non-deterministic `Filter` won't be pushed through `Project`
But in current code base this test case can't cover this purpose.
Change LogicalRDD to HadoopFsRelation can fix this issue.
## How was this patch tested?
Modified test pass.
Closes#23440 from LinhongLiu/fix-test.
Authored-by: Liu,Linhong <liulinhong@baidu.com>
Signed-off-by: Wenchen Fan <wenchen@databricks.com>
## What changes were proposed in this pull request?
Currently OrcColumnarBatchReader returns all the partition column values in the batch read.
In data source V2, we can improve it by returning the required partition column values only.
This PR is part of https://github.com/apache/spark/pull/23383 . As cloud-fan suggested, create a new PR to make review easier.
Also, this PR doesn't improve `OrcFileFormat`, since in the method `buildReaderWithPartitionValues`, the `requiredSchema` filter out all the partition columns, so we can't know which partition column is required.
## How was this patch tested?
Unit test
Closes#23387 from gengliangwang/refactorOrcColumnarBatch.
Lead-authored-by: Gengliang Wang <gengliang.wang@databricks.com>
Co-authored-by: Gengliang Wang <ltnwgl@gmail.com>
Co-authored-by: Dongjoon Hyun <dongjoon@apache.org>
Signed-off-by: Wenchen Fan <wenchen@databricks.com>
## What changes were proposed in this pull request?
`testExactCaseQueryPruning` and `testMixedCaseQueryPruning` don't need to set up `PARQUET_VECTORIZED_READER_ENABLED` config. Because `withMixedCaseData` will run against both Spark vectorized reader and Parquet-mr reader.
## How was this patch tested?
Existing test.
Closes#23427 from viirya/fix-parquet-schema-pruning-test.
Authored-by: Liang-Chi Hsieh <viirya@gmail.com>
Signed-off-by: Sean Owen <sean.owen@databricks.com>
## What changes were proposed in this pull request?
In the PR, I propose to move `hiveResultString()` out of `QueryExecution` and put it to a separate object.
Closes#23409 from MaxGekk/hive-result-string.
Lead-authored-by: Maxim Gekk <maxim.gekk@databricks.com>
Co-authored-by: Maxim Gekk <max.gekk@gmail.com>
Signed-off-by: Herman van Hovell <hvanhovell@databricks.com>
## What changes were proposed in this pull request?
This PR fixes `pivot(Column)` can accepts `collection.mutable.WrappedArray`.
Note that we return `collection.mutable.WrappedArray` from `ArrayType`, and `Literal.apply` doesn't support this.
We can unwrap the array and use it for type dispatch.
```scala
val df = Seq(
(2, Seq.empty[String]),
(2, Seq("a", "x")),
(3, Seq.empty[String]),
(3, Seq("a", "x"))).toDF("x", "s")
df.groupBy("x").pivot("s").count().show()
```
Before:
```
Unsupported literal type class scala.collection.mutable.WrappedArray$ofRef WrappedArray()
java.lang.RuntimeException: Unsupported literal type class scala.collection.mutable.WrappedArray$ofRef WrappedArray()
at org.apache.spark.sql.catalyst.expressions.Literal$.apply(literals.scala:80)
at org.apache.spark.sql.RelationalGroupedDataset.$anonfun$pivot$2(RelationalGroupedDataset.scala:427)
at scala.collection.TraversableLike.$anonfun$map$1(TraversableLike.scala:237)
at scala.collection.IndexedSeqOptimized.foreach(IndexedSeqOptimized.scala:36)
at scala.collection.IndexedSeqOptimized.foreach$(IndexedSeqOptimized.scala:33)
at scala.collection.mutable.WrappedArray.foreach(WrappedArray.scala:39)
at scala.collection.TraversableLike.map(TraversableLike.scala:237)
at scala.collection.TraversableLike.map$(TraversableLike.scala:230)
at scala.collection.AbstractTraversable.map(Traversable.scala:108)
at org.apache.spark.sql.RelationalGroupedDataset.pivot(RelationalGroupedDataset.scala:425)
at org.apache.spark.sql.RelationalGroupedDataset.pivot(RelationalGroupedDataset.scala:406)
at org.apache.spark.sql.RelationalGroupedDataset.pivot(RelationalGroupedDataset.scala:317)
at org.apache.spark.sql.DataFramePivotSuite.$anonfun$new$1(DataFramePivotSuite.scala:341)
at scala.runtime.java8.JFunction0$mcV$sp.apply(JFunction0$mcV$sp.java:23)
```
After:
```
+---+---+------+
| x| []|[a, x]|
+---+---+------+
| 3| 1| 1|
| 2| 1| 1|
+---+---+------+
```
## How was this patch tested?
Manually tested and unittests were added.
Closes#23349 from HyukjinKwon/SPARK-26403.
Authored-by: Hyukjin Kwon <gurwls223@apache.org>
Signed-off-by: Hyukjin Kwon <gurwls223@apache.org>
## What changes were proposed in this pull request?
`DataSourceScanExec` overrides "wrong" `treeString` method without `append`. In the PR, I propose to make `treeString`s **final** to prevent such mistakes in the future. And removed the `treeString` and `verboseString` since they both use `simpleString` with reduction.
## How was this patch tested?
It was tested by `DataSourceScanExecRedactionSuite`
Closes#23431 from MaxGekk/datasource-scan-exec-followup.
Authored-by: Maxim Gekk <maxim.gekk@databricks.com>
Signed-off-by: gatorsmile <gatorsmile@gmail.com>
## What changes were proposed in this pull request?
In `org.apache.spark.sql.execution.metric.SQLMetricsSuite`, there's a test case named "WholeStageCodegen metrics". However, it is executed with whole-stage codegen disabled. This PR fixes this by enable whole-stage codegen for this test case.
## How was this patch tested?
Tested locally using exiting test cases.
Closes#23224 from seancxmao/codegen-metrics.
Authored-by: seancxmao <seancxmao@gmail.com>
Signed-off-by: Sean Owen <sean.owen@databricks.com>
## What changes were proposed in this pull request?
This PR addresses warning messages in Java files reported at [lgtm.com](https://lgtm.com).
[lgtm.com](https://lgtm.com) provides automated code review of Java/Python/JavaScript files for OSS projects. [Here](https://lgtm.com/projects/g/apache/spark/alerts/?mode=list&severity=warning) are warning messages regarding Apache Spark project.
This PR addresses the following warnings:
- Result of multiplication cast to wider type
- Implicit narrowing conversion in compound assignment
- Boxed variable is never null
- Useless null check
NOTE: `Potential input resource leak` looks false positive for now.
## How was this patch tested?
Existing UTs
Closes#23420 from kiszk/SPARK-26508.
Authored-by: Kazuaki Ishizaki <ishizaki@jp.ibm.com>
Signed-off-by: Sean Owen <sean.owen@databricks.com>
## What changes were proposed in this pull request?
Default timestamp pattern defined in `JSONOptions` doesn't allow saving/loading timestamps with time zones of seconds precision. Because of that, the round trip test failed for timestamps before 1582. In the PR, I propose to extend zone offset section from `XXX` to `XXXXX` which should allow to save/load zone offsets like `-07:52:48`.
## How was this patch tested?
It was tested by `JsonHadoopFsRelationSuite` and `TimestampFormatterSuite`.
Closes#23417 from MaxGekk/hadoopfsrelationtest-new-formatter.
Lead-authored-by: Maxim Gekk <max.gekk@gmail.com>
Co-authored-by: Maxim Gekk <maxim.gekk@databricks.com>
Signed-off-by: Hyukjin Kwon <gurwls223@apache.org>
## What changes were proposed in this pull request?
When we operate as below:
`
0: jdbc:hive2://xxx/> create function funnel_analysis as 'com.xxx.hive.extend.udf.UapFunnelAnalysis';
`
`
0: jdbc:hive2://xxx/> select funnel_analysis(1,",",1,'');
Error: org.apache.spark.sql.AnalysisException: Undefined function: 'funnel_analysis'. This function is neither a registered temporary function nor a permanent function registered in the database 'xxx'.; line 1 pos 7 (state=,code=0)
`
`
0: jdbc:hive2://xxx/> describe function funnel_analysis;
+-----------------------------------------------------------+--+
| function_desc |
+-----------------------------------------------------------+--+
| Function: xxx.funnel_analysis |
| Class: com.xxx.hive.extend.udf.UapFunnelAnalysis |
| Usage: N/A. |
+-----------------------------------------------------------+--+
`
We can see describe funtion will get right information,but when we actually use this funtion,we will get an undefined exception.
Which is really misleading,the real cause is below:
`
No handler for Hive UDF 'com.xxx.xxx.hive.extend.udf.UapFunnelAnalysis': java.lang.IllegalStateException: Should not be called directly;
at org.apache.hadoop.hive.ql.udf.generic.GenericUDTF.initialize(GenericUDTF.java:72)
at org.apache.spark.sql.hive.HiveGenericUDTF.outputInspector$lzycompute(hiveUDFs.scala:204)
at org.apache.spark.sql.hive.HiveGenericUDTF.outputInspector(hiveUDFs.scala:204)
at org.apache.spark.sql.hive.HiveGenericUDTF.elementSchema$lzycompute(hiveUDFs.scala:212)
at org.apache.spark.sql.hive.HiveGenericUDTF.elementSchema(hiveUDFs.scala:212)
`
This patch print the actual failure for quick debugging.
## How was this patch tested?
UT
Closes#21790 from caneGuy/zhoukang/print-warning1.
Authored-by: zhoukang <zhoukang199191@gmail.com>
Signed-off-by: Sean Owen <sean.owen@databricks.com>
…Type
## What changes were proposed in this pull request?
Modifed JdbcUtils.makeGetter to handle ByteType.
## How was this patch tested?
Added a new test to JDBCSuite that maps ```TINYINT``` to ```ByteType```.
Closes#23400 from twdsilva/tiny_int_support.
Authored-by: Thomas D'Silva <tdsilva@apache.org>
Signed-off-by: Hyukjin Kwon <gurwls223@apache.org>
## What changes were proposed in this pull request?
The current `SelectedField` extractor is somewhat complicated and it seems to be handling cases that should be handled automatically:
- `GetArrayItem(child: GetStructFieldObject())`
- `GetArrayStructFields(child: GetArrayStructFields())`
- `GetMap(value: GetStructFieldObject())`
This PR removes those cases and simplifies the extractor by passing down the data type instead of a field.
## How was this patch tested?
Existing tests.
Closes#23397 from hvanhovell/SPARK-26495.
Authored-by: Herman van Hovell <hvanhovell@databricks.com>
Signed-off-by: Herman van Hovell <hvanhovell@databricks.com>
## What changes were proposed in this pull request?
As the description in SPARK-26339, spark.read behavior is very confusing when reading files that start with underscore, fix this by throwing exception which message is "Path does not exist".
## How was this patch tested?
manual tests.
Both of codes below throws exception which message is "Path does not exist".
```
spark.read.csv("/home/forcia/work/spark/_test.csv")
spark.read.schema("test STRING, number INT").csv("/home/forcia/work/spark/_test.csv")
```
Closes#23288 from KeiichiHirobe/SPARK-26339.
Authored-by: Hirobe Keiichi <keiichi_hirobe@forcia.com>
Signed-off-by: Sean Owen <sean.owen@databricks.com>
## What changes were proposed in this pull request?
Proposed new class `StringConcat` for converting a sequence of strings to string with one memory allocation in the `toString` method. `StringConcat` replaces `StringBuilderWriter` in methods of dumping of Spark plans and codegen to strings.
All `Writer` arguments are replaced by `String => Unit` in methods related to Spark plans stringification.
## How was this patch tested?
It was tested by existing suites `QueryExecutionSuite`, `DebuggingSuite` as well as new tests for `StringConcat` in `StringUtilsSuite`.
Closes#23406 from MaxGekk/rope-plan.
Authored-by: Maxim Gekk <maxim.gekk@databricks.com>
Signed-off-by: Herman van Hovell <hvanhovell@databricks.com>
## What changes were proposed in this pull request?
#20560/[SPARK-23375](https://issues.apache.org/jira/browse/SPARK-23375) introduced an optimizer rule to eliminate redundant Sort. For a test case named "Sort metrics" in `SQLMetricsSuite`, because range is already sorted, sort is removed by the `RemoveRedundantSorts`, which makes this test case meaningless.
This PR modifies the query for testing Sort metrics and checks Sort exists in the plan.
## How was this patch tested?
Modify the existing test case.
Closes#23258 from seancxmao/sort-metrics.
Authored-by: seancxmao <seancxmao@gmail.com>
Signed-off-by: Sean Owen <sean.owen@databricks.com>
## What changes were proposed in this pull request?
Similar with https://github.com/apache/spark/pull/21446. Looks random string is not quite safe as a directory name.
```scala
scala> val prefix = Random.nextString(10); val dir = new File("/tmp", "del_" + prefix + "-" + UUID.randomUUID.toString); dir.mkdirs()
prefix: String = 窽텘⒘駖ⵚ駢⡞Ρ닋
dir: java.io.File = /tmp/del_窽텘⒘駖ⵚ駢⡞Ρ닋-a3f99855-c429-47a0-a108-47bca6905745
res40: Boolean = false // nope, didn't like this one
```
## How was this patch tested?
Unit test was added, and manually.
Closes#23405 from HyukjinKwon/SPARK-26496.
Authored-by: Hyukjin Kwon <gurwls223@apache.org>
Signed-off-by: Dongjoon Hyun <dongjoon@apache.org>
## What changes were proposed in this pull request?
This PR fixes the codegen bug introduced by #23358 .
- https://amplab.cs.berkeley.edu/jenkins/view/Spark%20QA%20Test%20(Dashboard)/job/spark-master-test-maven-hadoop-2.7-ubuntu-scala-2.11/158/
```
Line 44, Column 93: A method named "apply" is not declared in any enclosing class
nor any supertype, nor through a static import
```
## How was this patch tested?
Manual. `DateExpressionsSuite` should be passed with Scala-2.11.
Closes#23394 from dongjoon-hyun/SPARK-26424.
Authored-by: Dongjoon Hyun <dongjoon@apache.org>
Signed-off-by: Dongjoon Hyun <dongjoon@apache.org>
## What changes were proposed in this pull request?
Currently the `AttributeReference.withMetadata` method have return type `Attribute`, the rest of with methods in the `AttributeReference` return type are `AttributeReference`, as the [spark-25892](https://issues.apache.org/jira/browse/SPARK-25892?jql=project%20%3D%20SPARK%20AND%20component%20in%20(ML%2C%20PySpark%2C%20SQL)) mentioned.
This PR will change `AttributeReference.withMetadata` method's return type from `Attribute` to `AttributeReference`.
## How was this patch tested?
Run all `sql/test,` `catalyst/test` and `org.apache.spark.sql.execution.streaming.*`
Closes#22918 from kevinyu98/spark-25892.
Authored-by: Kevin Yu <qyu@us.ibm.com>
Signed-off-by: Hyukjin Kwon <gurwls223@apache.org>
## What changes were proposed in this pull request?
In the PR, I propose to add `maxFields` parameter to all functions involved in creation of textual representation of spark plans such as `simpleString` and `verboseString`. New parameter restricts number of fields converted to truncated strings. Any elements beyond the limit will be dropped and replaced by a `"... N more fields"` placeholder. The threshold is bumped up to `Int.MaxValue` for `toFile()`.
## How was this patch tested?
Added a test to `QueryExecutionSuite` which checks `maxFields` impacts on number of truncated fields in `LocalRelation`.
Closes#23159 from MaxGekk/to-file-max-fields.
Lead-authored-by: Maxim Gekk <max.gekk@gmail.com>
Co-authored-by: Maxim Gekk <maxim.gekk@databricks.com>
Signed-off-by: Herman van Hovell <hvanhovell@databricks.com>
## What changes were proposed in this pull request?
Spark SQL doesn't support creating partitioned table using Hive CTAS in SQL syntax. However it is supported by using DataFrameWriter API.
```scala
val df = Seq(("a", 1)).toDF("part", "id")
df.write.format("hive").partitionBy("part").saveAsTable("t")
```
Hive begins to support this syntax in newer version: https://issues.apache.org/jira/browse/HIVE-20241:
```
CREATE TABLE t PARTITIONED BY (part) AS SELECT 1 as id, "a" as part
```
This patch adds this support to SQL syntax.
## How was this patch tested?
Added tests.
Closes#23376 from viirya/hive-ctas-partitioned-table.
Authored-by: Liang-Chi Hsieh <viirya@gmail.com>
Signed-off-by: Wenchen Fan <wenchen@databricks.com>
## What changes were proposed in this pull request?
In the PR, I propose to switch the `DateFormatClass`, `ToUnixTimestamp`, `FromUnixTime`, `UnixTime` on java.time API for parsing/formatting dates and timestamps. The API has been already implemented by the `Timestamp`/`DateFormatter` classes. One of benefit is those classes support parsing timestamps with microsecond precision. Old behaviour can be switched on via SQL config: `spark.sql.legacy.timeParser.enabled` (`false` by default).
## How was this patch tested?
It was tested by existing test suites - `DateFunctionsSuite`, `DateExpressionsSuite`, `JsonSuite`, `CsvSuite`, `SQLQueryTestSuite` as well as PySpark tests.
Closes#23358 from MaxGekk/new-time-cast.
Lead-authored-by: Maxim Gekk <maxim.gekk@databricks.com>
Co-authored-by: Maxim Gekk <max.gekk@gmail.com>
Signed-off-by: Wenchen Fan <wenchen@databricks.com>
## What changes were proposed in this pull request?
fix ExpresionInfo assert error in windows operation system, when running unit tests.
## How was this patch tested?
unit tests
Closes#23363 from yanlin-Lynn/unit-test-windows.
Authored-by: wangyanlin01 <wangyanlin01@baidu.com>
Signed-off-by: Hyukjin Kwon <gurwls223@apache.org>
## What changes were proposed in this pull request?
Variation of https://github.com/apache/spark/pull/20500
I cheated by not referencing fields or columns at all as this exception propagates in contexts where both would be applicable.
## How was this patch tested?
Existing tests
Closes#23373 from srowen/SPARK-14023.2.
Authored-by: Sean Owen <sean.owen@databricks.com>
Signed-off-by: Dongjoon Hyun <dongjoon@apache.org>
## What changes were proposed in this pull request?
The PRs #23150 and #23196 switched JSON and CSV datasources on new formatter for dates/timestamps which is based on `DateTimeFormatter`. In this PR, I replaced `SimpleDateFormat` by `DateTimeFormatter` to reflect the changes.
Closes#23374 from MaxGekk/java-time-docs.
Authored-by: Maxim Gekk <max.gekk@gmail.com>
Signed-off-by: Hyukjin Kwon <gurwls223@apache.org>
## What changes were proposed in this pull request?
GetStructField with different optional names should be semantically equal. We will use this as building block to compare the nested fields used in the plans to be optimized by catalyst optimizer.
This PR also fixes a bug below that accessing nested fields with different cases in case insensitive mode will result `AnalysisException`.
```
sql("create table t (s struct<i: Int>) using json")
sql("select s.I from t group by s.i")
```
which is currently failing
```
org.apache.spark.sql.AnalysisException: expression 'default.t.`s`' is neither present in the group by, nor is it an aggregate function
```
as cloud-fan pointed out.
## How was this patch tested?
New tests are added.
Closes#23353 from dbtsai/nestedEqual.
Lead-authored-by: DB Tsai <d_tsai@apple.com>
Co-authored-by: DB Tsai <dbtsai@dbtsai.com>
Signed-off-by: Dongjoon Hyun <dongjoon@apache.org>
## What changes were proposed in this pull request?
This patch adds explanation of `why "spark.sql.shuffle.partitions" keeps unchanged in structured streaming`, which couple of users already wondered and some of them even thought it as a bug.
This patch would help other end users to know about such behavior before they find by theirselves and being wondered.
## How was this patch tested?
No need to test because this is a simple addition on guide doc with markdown editor.
Closes#22238 from HeartSaVioR/SPARK-25245.
Lead-authored-by: Jungtaek Lim <kabhwan@gmail.com>
Co-authored-by: Jungtaek Lim (HeartSaVioR) <kabhwan@gmail.com>
Signed-off-by: Sean Owen <sean.owen@databricks.com>
## What changes were proposed in this pull request?
Use of `ProcessingTime` class was deprecated in favor of `Trigger.ProcessingTime` in Spark 2.2. And, [SPARK-21464](https://issues.apache.org/jira/browse/SPARK-21464) minimized it at 2.2.1. Recently, it grows again in test suites. This PR aims to clean up newly introduced deprecation warnings for Spark 3.0.
## How was this patch tested?
Pass the Jenkins with existing tests and manually check the warnings.
Closes#23367 from dongjoon-hyun/SPARK-26428.
Authored-by: Dongjoon Hyun <dongjoon@apache.org>
Signed-off-by: Dongjoon Hyun <dongjoon@apache.org>
## What changes were proposed in this pull request?
A followup of https://github.com/apache/spark/pull/23178 , to keep binary compability by using abstract class.
## How was this patch tested?
Manual test. I created a simple app with Spark 2.4
```
object TryUDF {
def main(args: Array[String]): Unit = {
val spark = SparkSession.builder().appName("test").master("local[*]").getOrCreate()
import spark.implicits._
val f1 = udf((i: Int) => i + 1)
println(f1.deterministic)
spark.range(10).select(f1.asNonNullable().apply($"id")).show()
spark.stop()
}
}
```
When I run it with current master, it fails with
```
java.lang.IncompatibleClassChangeError: Found interface org.apache.spark.sql.expressions.UserDefinedFunction, but class was expected
```
When I run it with this PR, it works
Closes#23351 from cloud-fan/minor.
Authored-by: Wenchen Fan <wenchen@databricks.com>
Signed-off-by: Wenchen Fan <wenchen@databricks.com>
## What changes were proposed in this pull request?
Currently, even if I explicitly disable Hive support in SparkR session as below:
```r
sparkSession <- sparkR.session("local[4]", "SparkR", Sys.getenv("SPARK_HOME"),
enableHiveSupport = FALSE)
```
produces when the Hadoop version is not supported by our Hive fork:
```
java.lang.reflect.InvocationTargetException
...
Caused by: java.lang.IllegalArgumentException: Unrecognized Hadoop major version number: 3.1.1.3.1.0.0-78
at org.apache.hadoop.hive.shims.ShimLoader.getMajorVersion(ShimLoader.java:174)
at org.apache.hadoop.hive.shims.ShimLoader.loadShims(ShimLoader.java:139)
at org.apache.hadoop.hive.shims.ShimLoader.getHadoopShims(ShimLoader.java:100)
at org.apache.hadoop.hive.conf.HiveConf$ConfVars.<clinit>(HiveConf.java:368)
... 43 more
Error in handleErrors(returnStatus, conn) :
java.lang.ExceptionInInitializerError
at org.apache.hadoop.hive.conf.HiveConf.<clinit>(HiveConf.java:105)
at java.lang.Class.forName0(Native Method)
at java.lang.Class.forName(Class.java:348)
at org.apache.spark.util.Utils$.classForName(Utils.scala:193)
at org.apache.spark.sql.SparkSession$.hiveClassesArePresent(SparkSession.scala:1116)
at org.apache.spark.sql.api.r.SQLUtils$.getOrCreateSparkSession(SQLUtils.scala:52)
at org.apache.spark.sql.api.r.SQLUtils.getOrCreateSparkSession(SQLUtils.scala)
at sun.reflect.NativeMethodAccessorImpl.invoke0(Native Method)
```
The root cause is that:
```
SparkSession.hiveClassesArePresent
```
check if the class is loadable or not to check if that's in classpath but `org.apache.hadoop.hive.conf.HiveConf` has a check for Hadoop version as static logic which is executed right away. This throws an `IllegalArgumentException` and that's not caught:
36edbac1c8/sql/core/src/main/scala/org/apache/spark/sql/SparkSession.scala (L1113-L1121)
So, currently, if users have a Hive built-in Spark with unsupported Hadoop version by our fork (namely 3+), there's no way to use SparkR even though it could work.
This PR just propose to change the order of bool comparison so that we can don't execute `SparkSession.hiveClassesArePresent` when:
1. `enableHiveSupport` is explicitly disabled
2. `spark.sql.catalogImplementation` is `in-memory`
so that we **only** check `SparkSession.hiveClassesArePresent` when Hive support is explicitly enabled by short circuiting.
## How was this patch tested?
It's difficult to write a test since we don't run tests against Hadoop 3 yet. See https://github.com/apache/spark/pull/21588. Manually tested.
Closes#23356 from HyukjinKwon/SPARK-26422.
Authored-by: Hyukjin Kwon <gurwls223@apache.org>
Signed-off-by: Hyukjin Kwon <gurwls223@apache.org>
## What changes were proposed in this pull request?
`HadoopFileWholeTextReader` and `HadoopFileLinesReader` will be eventually called in `FileSourceScanExec`.
In fact, locality has been implemented in `FileScanRDD`, even if we implement it in `HadoopFileWholeTextReader ` and `HadoopFileLinesReader`, it would be useless.
So I think these `TODO` can be removed.
## How was this patch tested?
N/A
Closes#23339 from 10110346/noneededtodo.
Authored-by: liuxian <liu.xian3@zte.com.cn>
Signed-off-by: Wenchen Fan <wenchen@databricks.com>
## What changes were proposed in this pull request?
`SQLConf` is supposed to be serializable. However, currently it is not serializable in `WithTestConf`. `WithTestConf` uses the method `overrideConfs` in closure, while the classes which implements it (`TestHiveSessionStateBuilder` and `TestSQLSessionStateBuilder`) are not serializable.
This PR is to use a local variable to fix it.
## How was this patch tested?
Add unit test.
Closes#23352 from gengliangwang/serializableSQLConf.
Authored-by: Gengliang Wang <gengliang.wang@databricks.com>
Signed-off-by: gatorsmile <gatorsmile@gmail.com>
## What changes were proposed in this pull request?
Currently, when we infer the schema for scala/java decimals, we return as data type the `SYSTEM_DEFAULT` implementation, ie. the decimal type with precision 38 and scale 18. But this is not right, as we know nothing about the right precision and scale and these values can be not enough to store the data. This problem arises in particular with UDF, where we cast all the input of type `DecimalType` to a `DecimalType(38, 18)`: in case this is not enough, null is returned as input for the UDF.
The PR defines a custom handling for casting to the expected data types for ScalaUDF: the decimal precision and scale is picked from the input, so no casting to different and maybe wrong percision and scale happens.
## How was this patch tested?
added UTs
Closes#23308 from mgaido91/SPARK-26308.
Authored-by: Marco Gaido <marcogaido91@gmail.com>
Signed-off-by: Wenchen Fan <wenchen@databricks.com>
## What changes were proposed in this pull request?
Deprecate Row.merge
## How was this patch tested?
N/A
Closes#23271 from KyleLi1985/master.
Authored-by: 李亮 <liang.li.work@outlook.com>
Signed-off-by: Hyukjin Kwon <gurwls223@apache.org>
## What changes were proposed in this pull request?
In Spark 2.3.0 and previous versions, Hive CTAS command will convert to use data source to write data into the table when the table is convertible. This behavior is controlled by the configs like HiveUtils.CONVERT_METASTORE_ORC and HiveUtils.CONVERT_METASTORE_PARQUET.
In 2.3.1, we drop this optimization by mistake in the PR [SPARK-22977](https://github.com/apache/spark/pull/20521/files#r217254430). Since that Hive CTAS command only uses Hive Serde to write data.
This patch adds this optimization back to Hive CTAS command. This patch adds OptimizedCreateHiveTableAsSelectCommand which uses data source to write data.
## How was this patch tested?
Added test.
Closes#22514 from viirya/SPARK-25271-2.
Authored-by: Liang-Chi Hsieh <viirya@gmail.com>
Signed-off-by: Wenchen Fan <wenchen@databricks.com>
## What changes were proposed in this pull request?
For better test coverage, this pr proposed to use the 4 mixed config sets of `WHOLESTAGE_CODEGEN_ENABLED` and `CODEGEN_FACTORY_MODE` when running `SQLQueryTestSuite`:
1. WHOLESTAGE_CODEGEN_ENABLED=true, CODEGEN_FACTORY_MODE=CODEGEN_ONLY
2. WHOLESTAGE_CODEGEN_ENABLED=false, CODEGEN_FACTORY_MODE=CODEGEN_ONLY
3. WHOLESTAGE_CODEGEN_ENABLED=true, CODEGEN_FACTORY_MODE=NO_CODEGEN
4. WHOLESTAGE_CODEGEN_ENABLED=false, CODEGEN_FACTORY_MODE=NO_CODEGEN
This pr also moved some existing tests into `ExplainSuite` because explain output results are different between codegen and interpreter modes.
## How was this patch tested?
Existing tests.
Closes#23213 from maropu/InterpreterModeTest.
Authored-by: Takeshi Yamamuro <yamamuro@apache.org>
Signed-off-by: Wenchen Fan <wenchen@databricks.com>
## What changes were proposed in this pull request?
This is a small clean up.
By design catalyst rules should be orthogonal: each rule should have its own responsibility. However, the `ColumnPruning` rule does not only do column pruning, but also remove no-op project and window.
This PR updates the `RemoveRedundantProject` rule to remove no-op window as well, and clean up the `ColumnPruning` rule to only do column pruning.
## How was this patch tested?
existing tests
Closes#23343 from cloud-fan/column-pruning.
Authored-by: Wenchen Fan <wenchen@databricks.com>
Signed-off-by: Dongjoon Hyun <dongjoon@apache.org>
## What changes were proposed in this pull request?
In `ReplaceExceptWithFilter` we do not consider properly the case in which the condition returns NULL. Indeed, in that case, since negating NULL still returns NULL, so it is not true the assumption that negating the condition returns all the rows which didn't satisfy it, rows returning NULL may not be returned. This happens when constraints inferred by `InferFiltersFromConstraints` are not enough, as it happens with `OR` conditions.
The rule had also problems with non-deterministic conditions: in such a scenario, this rule would change the probability of the output.
The PR fixes these problem by:
- returning False for the condition when it is Null (in this way we do return all the rows which didn't satisfy it);
- avoiding any transformation when the condition is non-deterministic.
## How was this patch tested?
added UTs
Closes#23315 from mgaido91/SPARK-26366.
Authored-by: Marco Gaido <marcogaido91@gmail.com>
Signed-off-by: gatorsmile <gatorsmile@gmail.com>
## What changes were proposed in this pull request?
Currently, SQL configs are not propagated to executors while schema inferring in CSV datasource. For example, changing of `spark.sql.legacy.timeParser.enabled` does not impact on inferring timestamp types. In the PR, I propose to fix the issue by wrapping schema inferring action using `SQLExecution.withSQLConfPropagated`.
## How was this patch tested?
Added logging to `TimestampFormatter`:
```patch
-object TimestampFormatter {
+object TimestampFormatter extends Logging {
def apply(format: String, timeZone: TimeZone, locale: Locale): TimestampFormatter = {
if (SQLConf.get.legacyTimeParserEnabled) {
+ logError("LegacyFallbackTimestampFormatter is being used")
new LegacyFallbackTimestampFormatter(format, timeZone, locale)
} else {
+ logError("Iso8601TimestampFormatter is being used")
new Iso8601TimestampFormatter(format, timeZone, locale)
}
}
```
and run the command in `spark-shell`:
```shell
$ ./bin/spark-shell --conf spark.sql.legacy.timeParser.enabled=true
```
```scala
scala> Seq("2010|10|10").toDF.repartition(1).write.mode("overwrite").text("/tmp/foo")
scala> spark.read.option("inferSchema", "true").option("header", "false").option("timestampFormat", "yyyy|MM|dd").csv("/tmp/foo").printSchema()
18/12/18 10:47:27 ERROR TimestampFormatter: LegacyFallbackTimestampFormatter is being used
root
|-- _c0: timestamp (nullable = true)
```
Closes#23345 from MaxGekk/csv-schema-infer-propagate-configs.
Authored-by: Maxim Gekk <maxim.gekk@databricks.com>
Signed-off-by: Hyukjin Kwon <gurwls223@apache.org>
## What changes were proposed in this pull request?
This PR proposes to use foreach instead of misuse of map (for Unit). This could cause some weird errors potentially and it's not a good practice anyway. See also SPARK-16694
## How was this patch tested?
N/A
Closes#23341 from HyukjinKwon/followup-SPARK-26081.
Authored-by: Hyukjin Kwon <gurwls223@apache.org>
Signed-off-by: Hyukjin Kwon <gurwls223@apache.org>
## What changes were proposed in this pull request?
The `JsonInferSchema` class is extended to support `TimestampType` inferring from string fields in JSON input:
- If the `prefersDecimal` option is set to `true`, it tries to infer decimal type from the string field.
- If decimal type inference fails or `prefersDecimal` is disabled, `JsonInferSchema` tries to infer `TimestampType`.
- If timestamp type inference fails, `StringType` is returned as the inferred type.
## How was this patch tested?
Added new test suite - `JsonInferSchemaSuite` to check date and timestamp types inferring from JSON using `JsonInferSchema` directly. A few tests were added `JsonSuite` to check type merging and roundtrip tests. This changes was tested by `JsonSuite`, `JsonExpressionsSuite` and `JsonFunctionsSuite` as well.
Closes#23201 from MaxGekk/json-infer-time.
Lead-authored-by: Maxim Gekk <maxim.gekk@databricks.com>
Co-authored-by: Maxim Gekk <max.gekk@gmail.com>
Signed-off-by: Hyukjin Kwon <gurwls223@apache.org>
## What changes were proposed in this pull request?
This PR implements a new feature - window aggregation Pandas UDF for bounded window.
#### Doc:
https://docs.google.com/document/d/14EjeY5z4-NC27-SmIP9CsMPCANeTcvxN44a7SIJtZPc/edit#heading=h.c87w44wcj3wj
#### Example:
```
from pyspark.sql.functions import pandas_udf, PandasUDFType
from pyspark.sql.window import Window
df = spark.range(0, 10, 2).toDF('v')
w1 = Window.partitionBy().orderBy('v').rangeBetween(-2, 4)
w2 = Window.partitionBy().orderBy('v').rowsBetween(-2, 2)
pandas_udf('double', PandasUDFType.GROUPED_AGG)
def avg(v):
return v.mean()
df.withColumn('v_mean', avg(df['v']).over(w1)).show()
# +---+------+
# | v|v_mean|
# +---+------+
# | 0| 1.0|
# | 2| 2.0|
# | 4| 4.0|
# | 6| 6.0|
# | 8| 7.0|
# +---+------+
df.withColumn('v_mean', avg(df['v']).over(w2)).show()
# +---+------+
# | v|v_mean|
# +---+------+
# | 0| 2.0|
# | 2| 3.0|
# | 4| 4.0|
# | 6| 5.0|
# | 8| 6.0|
# +---+------+
```
#### High level changes:
This PR modifies the existing WindowInPandasExec physical node to deal with unbounded (growing, shrinking and sliding) windows.
* `WindowInPandasExec` now share the same base class as `WindowExec` and share utility functions. See `WindowExecBase`
* `WindowFunctionFrame` now has two new functions `currentLowerBound` and `currentUpperBound` - to return the lower and upper window bound for the current output row. It is also modified to allow `AggregateProcessor` == null. Null aggregator processor is used for `WindowInPandasExec` where we don't have an aggregator and only uses lower and upper bound functions from `WindowFunctionFrame`
* The biggest change is in `WindowInPandasExec`, where it is modified to take `currentLowerBound` and `currentUpperBound` and write those values together with the input data to the python process for rolling window aggregation. See `WindowInPandasExec` for more details.
#### Discussion
In benchmarking, I found numpy variant of the rolling window UDF is much faster than the pandas version:
Spark SQL window function: 20s
Pandas variant: ~80s
Numpy variant: 10s
Numpy variant with numba: 4s
Allowing numpy variant of the vectorized UDFs is something I want to discuss because of the performance improvement, but doesn't have to be in this PR.
## How was this patch tested?
New tests
Closes#22305 from icexelloss/SPARK-24561-bounded-window-udf.
Authored-by: Li Jin <ice.xelloss@gmail.com>
Signed-off-by: Hyukjin Kwon <gurwls223@apache.org>
## What changes were proposed in this pull request?
SinkProgress should report similar properties like SourceProgress as long as they are available for given Sink. Count of written rows is metric availble for all Sinks. Since relevant progress information is with respect to commited rows, ideal object to carry this info is WriterCommitMessage. For brevity the implementation will focus only on Sinks with API V2 and on Micro Batch mode. Implemention for Continuous mode will be provided at later date.
### Before
```
{"description":"org.apache.spark.sql.kafka010.KafkaSourceProvider3c0bd317"}
```
### After
```
{"description":"org.apache.spark.sql.kafka010.KafkaSourceProvider3c0bd317","numOutputRows":5000}
```
### This PR is related to:
- https://issues.apache.org/jira/browse/SPARK-24647
- https://issues.apache.org/jira/browse/SPARK-21313
## How was this patch tested?
Existing and new unit tests.
Please review http://spark.apache.org/contributing.html before opening a pull request.
Closes#21919 from vackosar/feature/SPARK-24933-numOutputRows.
Lead-authored-by: Vaclav Kosar <admin@vaclavkosar.com>
Co-authored-by: Kosar, Vaclav: Functions Transformation <Vaclav.Kosar@barclayscapital.com>
Signed-off-by: gatorsmile <gatorsmile@gmail.com>
## What changes were proposed in this pull request?
1. rename `FormatterUtils` to `DateTimeFormatterHelper`, and move it to a separated file
2. move `DateFormatter` and its implementation to a separated file
3. mark some methods as private
4. add `override` to some methods
## How was this patch tested?
existing tests
Closes#23329 from cloud-fan/minor.
Authored-by: Wenchen Fan <wenchen@databricks.com>
Signed-off-by: Hyukjin Kwon <gurwls223@apache.org>
## What changes were proposed in this pull request?
This PR is a follow-up of the PR https://github.com/apache/spark/pull/17899. It is to add the rule TransposeWindow the optimizer batch.
## How was this patch tested?
The existing tests.
Closes#23222 from gatorsmile/followupSPARK-20636.
Authored-by: gatorsmile <gatorsmile@gmail.com>
Signed-off-by: gatorsmile <gatorsmile@gmail.com>
## What changes were proposed in this pull request?
- The original comment about `updateDriverMetrics` is not right.
- Refactor the code to ensure `selectedPartitions ` has been set before sending the driver-side metrics.
- Restore the original name, which is more general and extendable.
## How was this patch tested?
The existing tests.
Closes#23328 from gatorsmile/followupSpark-26142.
Authored-by: gatorsmile <gatorsmile@gmail.com>
Signed-off-by: gatorsmile <gatorsmile@gmail.com>
## What changes were proposed in this pull request?
The optimizer rule `org.apache.spark.sql.catalyst.optimizer.ReorderJoin` performs join reordering on inner joins. This was introduced from SPARK-12032 (https://github.com/apache/spark/pull/10073) in 2015-12.
After it had reordered the joins, though, it didn't check whether or not the output attribute order is still the same as before. Thus, it's possible to have a mismatch between the reordered output attributes order vs the schema that a DataFrame thinks it has.
The same problem exists in the CBO version of join reordering (`CostBasedJoinReorder`) too.
This can be demonstrated with the example:
```scala
spark.sql("create table table_a (x int, y int) using parquet")
spark.sql("create table table_b (i int, j int) using parquet")
spark.sql("create table table_c (a int, b int) using parquet")
val df = spark.sql("""
with df1 as (select * from table_a cross join table_b)
select * from df1 join table_c on a = x and b = i
""")
```
here's what the DataFrame thinks:
```
scala> df.printSchema
root
|-- x: integer (nullable = true)
|-- y: integer (nullable = true)
|-- i: integer (nullable = true)
|-- j: integer (nullable = true)
|-- a: integer (nullable = true)
|-- b: integer (nullable = true)
```
here's what the optimized plan thinks, after join reordering:
```
scala> df.queryExecution.optimizedPlan.output.foreach(a => println(s"|-- ${a.name}: ${a.dataType.typeName}"))
|-- x: integer
|-- y: integer
|-- a: integer
|-- b: integer
|-- i: integer
|-- j: integer
```
If we exclude the `ReorderJoin` rule (using Spark 2.4's optimizer rule exclusion feature), it's back to normal:
```
scala> spark.conf.set("spark.sql.optimizer.excludedRules", "org.apache.spark.sql.catalyst.optimizer.ReorderJoin")
scala> val df = spark.sql("with df1 as (select * from table_a cross join table_b) select * from df1 join table_c on a = x and b = i")
df: org.apache.spark.sql.DataFrame = [x: int, y: int ... 4 more fields]
scala> df.queryExecution.optimizedPlan.output.foreach(a => println(s"|-- ${a.name}: ${a.dataType.typeName}"))
|-- x: integer
|-- y: integer
|-- i: integer
|-- j: integer
|-- a: integer
|-- b: integer
```
Note that this output attribute ordering problem leads to data corruption, and can manifest itself in various symptoms:
* Silently corrupting data, if the reordered columns happen to either have matching types or have sufficiently-compatible types (e.g. all fixed length primitive types are considered as "sufficiently compatible" in an `UnsafeRow`), then only the resulting data is going to be wrong but it might not trigger any alarms immediately. Or
* Weird Java-level exceptions like `java.lang.NegativeArraySizeException`, or even SIGSEGVs.
## How was this patch tested?
Added new unit test in `JoinReorderSuite` and new end-to-end test in `JoinSuite`.
Also made `JoinReorderSuite` and `StarJoinReorderSuite` assert more strongly on maintaining output attribute order.
Closes#23303 from rednaxelafx/fix-join-reorder.
Authored-by: Kris Mok <rednaxelafx@gmail.com>
Signed-off-by: Wenchen Fan <wenchen@databricks.com>
## What changes were proposed in this pull request?
The `CSVInferSchema` class is extended to support inferring of `DateType` from CSV input. The attempt to infer `DateType` is performed after inferring `TimestampType`.
## How was this patch tested?
Added new test for inferring date types from CSV . It was also tested by existing suites like `CSVInferSchemaSuite`, `CsvExpressionsSuite`, `CsvFunctionsSuite` and `CsvSuite`.
Closes#23202 from MaxGekk/csv-date-inferring.
Lead-authored-by: Maxim Gekk <max.gekk@gmail.com>
Co-authored-by: Maxim Gekk <maxim.gekk@databricks.com>
Signed-off-by: Wenchen Fan <wenchen@databricks.com>
## What changes were proposed in this pull request?
CSV parsing accidentally uses the previous good value for a bad input field. See example in Jira.
This PR ensures that the associated column is set to null when an input field cannot be converted.
## How was this patch tested?
Added new test.
Ran all SQL unit tests (testOnly org.apache.spark.sql.*).
Ran pyspark tests for pyspark-sql
Closes#23323 from bersprockets/csv-bad-field.
Authored-by: Bruce Robbins <bersprockets@gmail.com>
Signed-off-by: Hyukjin Kwon <gurwls223@apache.org>
## What changes were proposed in this pull request?
When there is a self-join as result of a IN subquery, the join condition may be invalid, resulting in trivially true predicates and return wrong results.
The PR deduplicates the subquery output in order to avoid the issue.
## How was this patch tested?
added UT
Closes#23057 from mgaido91/SPARK-26078.
Authored-by: Marco Gaido <marcogaido91@gmail.com>
Signed-off-by: Wenchen Fan <wenchen@databricks.com>
## What changes were proposed in this pull request?
In the PR, I propose to switch on **java.time API** for parsing timestamps and dates from JSON inputs with microseconds precision. The SQL config `spark.sql.legacy.timeParser.enabled` allow to switch back to previous behavior with using `java.text.SimpleDateFormat`/`FastDateFormat` for parsing/generating timestamps/dates.
## How was this patch tested?
It was tested by `JsonExpressionsSuite`, `JsonFunctionsSuite` and `JsonSuite`.
Closes#23196 from MaxGekk/json-time-parser.
Lead-authored-by: Maxim Gekk <maxim.gekk@databricks.com>
Co-authored-by: Maxim Gekk <max.gekk@gmail.com>
Signed-off-by: Wenchen Fan <wenchen@databricks.com>
## What changes were proposed in this pull request?
Multiple SparkContexts are discouraged and it has been warning for last 4 years, see SPARK-4180. It could cause arbitrary and mysterious error cases, see SPARK-2243.
Honestly, I didn't even know Spark still allows it, which looks never officially supported, see SPARK-2243.
I believe It should be good timing now to remove this configuration.
## How was this patch tested?
Each doc was manually checked and manually tested:
```
$ ./bin/spark-shell --conf=spark.driver.allowMultipleContexts=true
...
scala> new SparkContext()
org.apache.spark.SparkException: Only one SparkContext should be running in this JVM (see SPARK-2243).The currently running SparkContext was created at:
org.apache.spark.sql.SparkSession$Builder.getOrCreate(SparkSession.scala:939)
...
org.apache.spark.SparkContext$.$anonfun$assertNoOtherContextIsRunning$2(SparkContext.scala:2435)
at scala.Option.foreach(Option.scala:274)
at org.apache.spark.SparkContext$.assertNoOtherContextIsRunning(SparkContext.scala:2432)
at org.apache.spark.SparkContext$.markPartiallyConstructed(SparkContext.scala:2509)
at org.apache.spark.SparkContext.<init>(SparkContext.scala:80)
at org.apache.spark.SparkContext.<init>(SparkContext.scala:112)
... 49 elided
```
Closes#23311 from HyukjinKwon/SPARK-26362.
Authored-by: Hyukjin Kwon <gurwls223@apache.org>
Signed-off-by: Hyukjin Kwon <gurwls223@apache.org>
## What changes were proposed in this pull request?
When using ordinals to access linked list, the time cost is O(n).
## How was this patch tested?
Existing tests.
Closes#23280 from CarolinePeng/update_Two.
Authored-by: CarolinPeng <00244106@zte.intra>
Signed-off-by: Sean Owen <sean.owen@databricks.com>
## What changes were proposed in this pull request?
When using a higher-order function with the same variable name as the existing columns in `Filter` or something which uses `Analyzer.resolveExpressionBottomUp` during the resolution, e.g.,:
```scala
val df = Seq(
(Seq(1, 9, 8, 7), 1, 2),
(Seq(5, 9, 7), 2, 2),
(Seq.empty, 3, 2),
(null, 4, 2)
).toDF("i", "x", "d")
checkAnswer(df.filter("exists(i, x -> x % d == 0)"),
Seq(Row(Seq(1, 9, 8, 7), 1, 2)))
checkAnswer(df.select("x").filter("exists(i, x -> x % d == 0)"),
Seq(Row(1)))
```
the following exception happens:
```
java.lang.ClassCastException: org.apache.spark.sql.catalyst.expressions.BoundReference cannot be cast to org.apache.spark.sql.catalyst.expressions.NamedExpression
at scala.collection.TraversableLike.$anonfun$map$1(TraversableLike.scala:237)
at scala.collection.mutable.ResizableArray.foreach(ResizableArray.scala:62)
at scala.collection.mutable.ResizableArray.foreach$(ResizableArray.scala:55)
at scala.collection.mutable.ArrayBuffer.foreach(ArrayBuffer.scala:49)
at scala.collection.TraversableLike.map(TraversableLike.scala:237)
at scala.collection.TraversableLike.map$(TraversableLike.scala:230)
at scala.collection.AbstractTraversable.map(Traversable.scala:108)
at org.apache.spark.sql.catalyst.expressions.HigherOrderFunction.$anonfun$functionsForEval$1(higherOrderFunctions.scala:147)
at scala.collection.TraversableLike.$anonfun$map$1(TraversableLike.scala:237)
at scala.collection.immutable.List.foreach(List.scala:392)
at scala.collection.TraversableLike.map(TraversableLike.scala:237)
at scala.collection.TraversableLike.map$(TraversableLike.scala:230)
at scala.collection.immutable.List.map(List.scala:298)
at org.apache.spark.sql.catalyst.expressions.HigherOrderFunction.functionsForEval(higherOrderFunctions.scala:145)
at org.apache.spark.sql.catalyst.expressions.HigherOrderFunction.functionsForEval$(higherOrderFunctions.scala:145)
at org.apache.spark.sql.catalyst.expressions.ArrayExists.functionsForEval$lzycompute(higherOrderFunctions.scala:369)
at org.apache.spark.sql.catalyst.expressions.ArrayExists.functionsForEval(higherOrderFunctions.scala:369)
at org.apache.spark.sql.catalyst.expressions.SimpleHigherOrderFunction.functionForEval(higherOrderFunctions.scala:176)
at org.apache.spark.sql.catalyst.expressions.SimpleHigherOrderFunction.functionForEval$(higherOrderFunctions.scala:176)
at org.apache.spark.sql.catalyst.expressions.ArrayExists.functionForEval(higherOrderFunctions.scala:369)
at org.apache.spark.sql.catalyst.expressions.ArrayExists.nullSafeEval(higherOrderFunctions.scala:387)
at org.apache.spark.sql.catalyst.expressions.SimpleHigherOrderFunction.eval(higherOrderFunctions.scala:190)
at org.apache.spark.sql.catalyst.expressions.SimpleHigherOrderFunction.eval$(higherOrderFunctions.scala:185)
at org.apache.spark.sql.catalyst.expressions.ArrayExists.eval(higherOrderFunctions.scala:369)
at org.apache.spark.sql.catalyst.expressions.GeneratedClass$SpecificPredicate.eval(Unknown Source)
at org.apache.spark.sql.execution.FilterExec.$anonfun$doExecute$3(basicPhysicalOperators.scala:216)
at org.apache.spark.sql.execution.FilterExec.$anonfun$doExecute$3$adapted(basicPhysicalOperators.scala:215)
...
```
because the `UnresolvedAttribute`s in `LambdaFunction` are unexpectedly resolved by the rule.
This pr modified to use a placeholder `UnresolvedNamedLambdaVariable` to prevent unexpected resolution.
## How was this patch tested?
Added a test and modified some tests.
Closes#23320 from ueshin/issues/SPARK-26370/hof_resolution.
Authored-by: Takuya UESHIN <ueshin@databricks.com>
Signed-off-by: Wenchen Fan <wenchen@databricks.com>
## What changes were proposed in this pull request?
I was looking at the code and it was a bit difficult to see the life cycle of InMemoryFileIndex passed into getOrInferFileFormatSchema, because once it is passed in, and another time it was created in getOrInferFileFormatSchema. It'd be easier to understand the life cycle if we move the creation of it out.
## How was this patch tested?
This is a simple code move and should be covered by existing tests.
Closes#23317 from rxin/SPARK-26368.
Authored-by: Reynold Xin <rxin@databricks.com>
Signed-off-by: gatorsmile <gatorsmile@gmail.com>
## What changes were proposed in this pull request?
Regarding the performance issue of SPARK-26155, it reports the issue on TPC-DS. I think it is better to add a benchmark for `LongToUnsafeRowMap` which is the root cause of performance regression.
It can be easier to show performance difference between different metric implementations in `LongToUnsafeRowMap`.
## How was this patch tested?
Manually run added benchmark.
Closes#23284 from viirya/SPARK-26337.
Authored-by: Liang-Chi Hsieh <viirya@gmail.com>
Signed-off-by: Wenchen Fan <wenchen@databricks.com>
## What changes were proposed in this pull request?
Added query status updates to ContinuousExecution.
## How was this patch tested?
Existing unit tests + added ContinuousQueryStatusAndProgressSuite.
Closes#23095 from gaborgsomogyi/SPARK-23886.
Authored-by: Gabor Somogyi <gabor.g.somogyi@gmail.com>
Signed-off-by: Marcelo Vanzin <vanzin@cloudera.com>
## What changes were proposed in this pull request?
As discussed in https://github.com/apache/spark/pull/23208/files#r239684490 , we should put `newScanBuilder` in read related mix-in traits like `SupportsBatchRead`, to support write-only table.
In the `Append` operator, we should skip schema validation if not necessary. In the future we would introduce a capability API, so that data source can tell Spark that it doesn't want to do validation.
## How was this patch tested?
existing tests.
Closes#23266 from cloud-fan/ds-read.
Authored-by: Wenchen Fan <wenchen@databricks.com>
Signed-off-by: Hyukjin Kwon <gurwls223@apache.org>
## What changes were proposed in this pull request?
There are some comments issues left when `ConvertToLocalRelation` rule was added (see #22205/[SPARK-25212](https://issues.apache.org/jira/browse/SPARK-25212)). This PR fixes those comments issues.
## How was this patch tested?
N/A
Closes#23273 from seancxmao/ConvertToLocalRelation-doc.
Authored-by: seancxmao <seancxmao@gmail.com>
Signed-off-by: Sean Owen <sean.owen@databricks.com>
## What changes were proposed in this pull request?
cleanup some tests to make sure expression is resolved during test.
## How was this patch tested?
test-only PR
Closes#23297 from cloud-fan/test.
Authored-by: Wenchen Fan <wenchen@databricks.com>
Signed-off-by: Wenchen Fan <wenchen@databricks.com>
## What changes were proposed in this pull request?
Some documents of `Distribution/Partitioning` are stale and misleading, this PR fixes them:
1. `Distribution` never have intra-partition requirement
2. `OrderedDistribution` does not require tuples that share the same value being colocated in the same partition.
3. `RangePartitioning` can provide a weaker guarantee for a prefix of its `ordering` expressions.
## How was this patch tested?
comment-only PR.
Closes#23249 from cloud-fan/doc.
Authored-by: Wenchen Fan <wenchen@databricks.com>
Signed-off-by: Wenchen Fan <wenchen@databricks.com>
## What changes were proposed in this pull request?
Follow up pr for #23207, include following changes:
- Rename `SQLShuffleMetricsReporter` to `SQLShuffleReadMetricsReporter` to make it match with write side naming.
- Display text changes for read side for naming consistent.
- Rename function in `ShuffleWriteProcessor`.
- Delete `private[spark]` in execution package.
## How was this patch tested?
Existing tests.
Closes#23286 from xuanyuanking/SPARK-26193-follow.
Authored-by: Yuanjian Li <xyliyuanjian@gmail.com>
Signed-off-by: Wenchen Fan <wenchen@databricks.com>
## What changes were proposed in this pull request?
If `checkForContinuous` is called ( `checkForStreaming` is called in `checkForContinuous` ), the `checkForStreaming` mothod will be called twice in `createQuery` , this is not necessary, and the `checkForStreaming` method has a lot of statements, so it's better to remove one of them.
## How was this patch tested?
Existing unit tests in `StreamingQueryManagerSuite` and `ContinuousAggregationSuite`
Closes#23251 from 10110346/isUnsupportedOperationCheckEnabled.
Authored-by: liuxian <liu.xian3@zte.com.cn>
Signed-off-by: Dongjoon Hyun <dongjoon@apache.org>
## What changes were proposed in this pull request?
In the PR, I propose to return partial results from JSON datasource and JSON functions in the PERMISSIVE mode if some of JSON fields are parsed and converted to desired types successfully. The changes are made only for `StructType`. Whole bad JSON records are placed into the corrupt column specified by the `columnNameOfCorruptRecord` option or SQL config.
Partial results are not returned for malformed JSON input.
## How was this patch tested?
Added new UT which checks converting JSON strings with one invalid and one valid field at the end of the string.
Closes#23253 from MaxGekk/json-bad-record.
Lead-authored-by: Maxim Gekk <max.gekk@gmail.com>
Co-authored-by: Maxim Gekk <maxim.gekk@databricks.com>
Signed-off-by: Hyukjin Kwon <gurwls223@apache.org>
## What changes were proposed in this pull request?
This is a regression introduced by https://github.com/apache/spark/pull/22104 at Spark 2.4.0.
When we have Python UDF in subquery, we will hit an exception
```
Caused by: java.lang.ClassCastException: org.apache.spark.sql.catalyst.expressions.AttributeReference cannot be cast to org.apache.spark.sql.catalyst.expressions.PythonUDF
at scala.collection.immutable.Stream.map(Stream.scala:414)
at org.apache.spark.sql.execution.python.EvalPythonExec.$anonfun$doExecute$2(EvalPythonExec.scala:98)
at org.apache.spark.rdd.RDD.$anonfun$mapPartitions$2(RDD.scala:815)
...
```
https://github.com/apache/spark/pull/22104 turned `ExtractPythonUDFs` from a physical rule to optimizer rule. However, there is a difference between a physical rule and optimizer rule. A physical rule always runs once, an optimizer rule may be applied twice on a query tree even the rule is located in a batch that only runs once.
For a subquery, the `OptimizeSubqueries` rule will execute the entire optimizer on the query plan inside subquery. Later on subquery will be turned to joins, and the optimizer rules will be applied to it again.
Unfortunately, the `ExtractPythonUDFs` rule is not idempotent. When it's applied twice on a query plan inside subquery, it will produce a malformed plan. It extracts Python UDF from Python exec plans.
This PR proposes 2 changes to be double safe:
1. `ExtractPythonUDFs` should skip python exec plans, to make the rule idempotent
2. `ExtractPythonUDFs` should skip subquery
## How was this patch tested?
a new test.
Closes#23248 from cloud-fan/python.
Authored-by: Wenchen Fan <wenchen@databricks.com>
Signed-off-by: Wenchen Fan <wenchen@databricks.com>
## What changes were proposed in this pull request?
`RDDConversions` would get disproportionately slower as the number of columns in the query increased,
for the type of `converters` before is `scala.collection.immutable.::` which is a subtype of list.
This PR removing `RDDConversions` and using `RowEncoder` to convert the Row to InternalRow.
The test of `PrunedScanSuite` for 2000 columns and 20k rows takes 409 seconds before this PR, and 361 seconds after.
## How was this patch tested?
Test case of `PrunedScanSuite`
Closes#23262 from eatoncys/toarray.
Authored-by: 10129659 <chen.yanshan@zte.com.cn>
Signed-off-by: Hyukjin Kwon <gurwls223@apache.org>
… incorrect.
## What changes were proposed in this pull request?
In the reported heartbeat information, the unit of the memory data is bytes, which is converted by the formatBytes() function in the utils.js file before being displayed in the interface. The cardinality of the unit conversion in the formatBytes function is 1000, which should be 1024.
Change the cardinality of the unit conversion in the formatBytes function to 1024.
## How was this patch tested?
manual tests
Please review http://spark.apache.org/contributing.html before opening a pull request.
Closes#22683 from httfighter/SPARK-25696.
Lead-authored-by: 韩田田00222924 <han.tiantian@zte.com.cn>
Co-authored-by: han.tiantian@zte.com.cn <han.tiantian@zte.com.cn>
Signed-off-by: Sean Owen <sean.owen@databricks.com>
## What changes were proposed in this pull request?
Add appendReadColumns Unit Test for HiveShimSuite.
## How was this patch tested?
```
$ build/sbt
> project hive
> testOnly *HiveShimSuite
```
Closes#23268 from sadhen/refactor/hiveshim.
Authored-by: Darcy Shen <sadhen@zoho.com>
Signed-off-by: Hyukjin Kwon <gurwls223@apache.org>
## What changes were proposed in this pull request?
This is a Spark 2.3 regression introduced in https://github.com/apache/spark/pull/20521. We should add the partition info for InsertIntoHiveTable in CreateHiveTableAsSelectCommand. Otherwise, we will hit the following error by running the newly added test case:
```
[info] - CTAS: INSERT a partitioned table using Hive serde *** FAILED *** (829 milliseconds)
[info] org.apache.spark.SparkException: Requested partitioning does not match the tab1 table:
[info] Requested partitions:
[info] Table partitions: part
[info] at org.apache.spark.sql.hive.execution.InsertIntoHiveTable.processInsert(InsertIntoHiveTable.scala:179)
[info] at org.apache.spark.sql.hive.execution.InsertIntoHiveTable.run(InsertIntoHiveTable.scala:107)
```
## How was this patch tested?
Added a test case.
Closes#23255 from gatorsmile/fixCTAS.
Authored-by: gatorsmile <gatorsmile@gmail.com>
Signed-off-by: Wenchen Fan <wenchen@databricks.com>
## What changes were proposed in this pull request?
1. Implement `SQLShuffleWriteMetricsReporter` on the SQL side as the customized `ShuffleWriteMetricsReporter`.
2. Add shuffle write metrics to `ShuffleExchangeExec`, and use these metrics to create corresponding `SQLShuffleWriteMetricsReporter` in shuffle dependency.
3. Rework on `ShuffleMapTask` to add new class named `ShuffleWriteProcessor` which control shuffle write process, we use sql shuffle write metrics by customizing a ShuffleWriteProcessor on SQL side.
## How was this patch tested?
Add UT in SQLMetricsSuite.
Manually test locally, update screen shot to document attached in JIRA.
Closes#23207 from xuanyuanking/SPARK-26193.
Authored-by: Yuanjian Li <xyliyuanjian@gmail.com>
Signed-off-by: Wenchen Fan <wenchen@databricks.com>
## What changes were proposed in this pull request?
A followup of https://github.com/apache/spark/pull/23043
There are 4 places we need to deal with NaN and -0.0:
1. comparison expressions. `-0.0` and `0.0` should be treated as same. Different NaNs should be treated as same.
2. Join keys. `-0.0` and `0.0` should be treated as same. Different NaNs should be treated as same.
3. grouping keys. `-0.0` and `0.0` should be assigned to the same group. Different NaNs should be assigned to the same group.
4. window partition keys. `-0.0` and `0.0` should be treated as same. Different NaNs should be treated as same.
The case 1 is OK. Our comparison already handles NaN and -0.0, and for struct/array/map, we will recursively compare the fields/elements.
Case 2, 3 and 4 are problematic, as they compare `UnsafeRow` binary directly, and different NaNs have different binary representation, and the same thing happens for -0.0 and 0.0.
To fix it, a simple solution is: normalize float/double when building unsafe data (`UnsafeRow`, `UnsafeArrayData`, `UnsafeMapData`). Then we don't need to worry about it anymore.
Following this direction, this PR moves the handling of NaN and -0.0 from `Platform` to `UnsafeWriter`, so that places like `UnsafeRow.setFloat` will not handle them, which reduces the perf overhead. It's also easier to add comments explaining why we do it in `UnsafeWriter`.
## How was this patch tested?
existing tests
Closes#23239 from cloud-fan/minor.
Authored-by: Wenchen Fan <wenchen@databricks.com>
Signed-off-by: Dongjoon Hyun <dongjoon@apache.org>
## What changes were proposed in this pull request?
Parquet files appear to have nullability info when being written, not being read.
## How was this patch tested?
Some test code: (running spark 2.3, but the relevant code in DataSource looks identical on master)
case class NullTest(bo: Boolean, opbol: Option[Boolean])
val testDf = spark.createDataFrame(Seq(NullTest(true, Some(false))))
defined class NullTest
testDf: org.apache.spark.sql.DataFrame = [bo: boolean, opbol: boolean]
testDf.write.parquet("s3://asana-stats/tmp_dima/parquet_check_schema")
spark.read.parquet("s3://asana-stats/tmp_dima/parquet_check_schema/part-00000-b1bf4a19-d9fe-4ece-a2b4-9bbceb490857-c000.snappy.parquet4").printSchema()
root
|-- bo: boolean (nullable = true)
|-- opbol: boolean (nullable = true)
Meanwhile, the parquet file formed does have nullable info:
[]batchprod-report000:/tmp/dimakamalov-batch$ aws s3 ls s3://asana-stats/tmp_dima/parquet_check_schema/
2018-10-17 21:03:52 0 _SUCCESS
2018-10-17 21:03:50 504 part-00000-b1bf4a19-d9fe-4ece-a2b4-9bbceb490857-c000.snappy.parquet
[]batchprod-report000:/tmp/dimakamalov-batch$ aws s3 cp s3://asana-stats/tmp_dima/parquet_check_schema/part-00000-b1bf4a19-d9fe-4ece-a2b4-9bbceb490857-c000.snappy.parquet .
download: s3://asana-stats/tmp_dima/parquet_check_schema/part-00000-b1bf4a19-d9fe-4ece-a2b4-9bbceb490857-c000.snappy.parquet to ./part-00000-b1bf4a19-d9fe-4ece-a2b4-9bbceb490857-c000.snappy.parquet
[]batchprod-report000:/tmp/dimakamalov-batch$ java -jar parquet-tools-1.8.2.jar schema part-00000-b1bf4a19-d9fe-4ece-a2b4-9bbceb490857-c000.snappy.parquet
message spark_schema {
required boolean bo;
optional boolean opbol;
}
Closes#22759 from dima-asana/dima-asana-nullable-parquet-doc.
Authored-by: dima-asana <42555784+dima-asana@users.noreply.github.com>
Signed-off-by: Sean Owen <sean.owen@databricks.com>
## What changes were proposed in this pull request?
This is a follow-up of #23031 to rename the config name to `spark.sql.legacy.setCommandRejectsSparkCoreConfs`.
## How was this patch tested?
Existing tests.
Closes#23245 from ueshin/issues/SPARK-26060/rename_config.
Authored-by: Takuya UESHIN <ueshin@databricks.com>
Signed-off-by: Dongjoon Hyun <dongjoon@apache.org>
## What changes were proposed in this pull request?
Currently if user provides data schema, partition column values are converted as per it. But if the conversion failed, e.g. converting string to int, the column value is null.
This PR proposes to throw exception in such case, instead of converting into null value silently:
1. These null partition column values doesn't make sense to users in most cases. It is better to show the conversion failure, and then users can adjust the schema or ETL jobs to fix it.
2. There are always exceptions on such conversion failure for non-partition data columns. Partition columns should have the same behavior.
We can reproduce the case above as following:
```
/tmp/testDir
├── p=bar
└── p=foo
```
If we run:
```
val schema = StructType(Seq(StructField("p", IntegerType, false)))
spark.read.schema(schema).csv("/tmp/testDir/").show()
```
We will get:
```
+----+
| p|
+----+
|null|
|null|
+----+
```
## How was this patch tested?
Unit test
Closes#23215 from gengliangwang/SPARK-26263.
Authored-by: Gengliang Wang <gengliang.wang@databricks.com>
Signed-off-by: Wenchen Fan <wenchen@databricks.com>
## What changes were proposed in this pull request?
`enablePerfMetrics `was originally designed in `BytesToBytesMap `to control `getNumHashCollisions getTimeSpentResizingNs getAverageProbesPerLookup`.
However, as the Spark version gradual progress. this parameter is only used for `getAverageProbesPerLookup ` and always given to true when using `BytesToBytesMap`.
it is also dangerous to determine whether `getAverageProbesPerLookup `opens and throws an `IllegalStateException `exception.
So this pr will be remove `enablePerfMetrics `parameter from `BytesToBytesMap`. thanks.
## How was this patch tested?
the existed test cases.
Closes#23244 from heary-cao/enablePerfMetrics.
Authored-by: caoxuewen <cao.xuewen@zte.com.cn>
Signed-off-by: Wenchen Fan <wenchen@databricks.com>
## What changes were proposed in this pull request?
When executing `toPandas` with Arrow enabled, partitions that arrive in the JVM out-of-order must be buffered before they can be send to Python. This causes an excess of memory to be used in the driver JVM and increases the time it takes to complete because data must sit in the JVM waiting for preceding partitions to come in.
This change sends un-ordered partitions to Python as soon as they arrive in the JVM, followed by a list of partition indices so that Python can assemble the data in the correct order. This way, data is not buffered at the JVM and there is no waiting on particular partitions so performance will be increased.
Followup to #21546
## How was this patch tested?
Added new test with a large number of batches per partition, and test that forces a small delay in the first partition. These test that partitions are collected out-of-order and then are are put in the correct order in Python.
## Performance Tests - toPandas
Tests run on a 4 node standalone cluster with 32 cores total, 14.04.1-Ubuntu and OpenJDK 8
measured wall clock time to execute `toPandas()` and took the average best time of 5 runs/5 loops each.
Test code
```python
df = spark.range(1 << 25, numPartitions=32).toDF("id").withColumn("x1", rand()).withColumn("x2", rand()).withColumn("x3", rand()).withColumn("x4", rand())
for i in range(5):
start = time.time()
_ = df.toPandas()
elapsed = time.time() - start
```
Spark config
```
spark.driver.memory 5g
spark.executor.memory 5g
spark.driver.maxResultSize 2g
spark.sql.execution.arrow.enabled true
```
Current Master w/ Arrow stream | This PR
---------------------|------------
5.16207 | 4.342533
5.133671 | 4.399408
5.147513 | 4.468471
5.105243 | 4.36524
5.018685 | 4.373791
Avg Master | Avg This PR
------------------|--------------
5.1134364 | 4.3898886
Speedup of **1.164821449**
Closes#22275 from BryanCutler/arrow-toPandas-oo-batches-SPARK-25274.
Authored-by: Bryan Cutler <cutlerb@gmail.com>
Signed-off-by: Bryan Cutler <cutlerb@gmail.com>
## What changes were proposed in this pull request?
this code come from PR: https://github.com/apache/spark/pull/11190,
but this code has never been used, only since PR: https://github.com/apache/spark/pull/14548,
Let's continue fix it. thanks.
## How was this patch tested?
N / A
Closes#23227 from heary-cao/unuseSparkPlan.
Authored-by: caoxuewen <cao.xuewen@zte.com.cn>
Signed-off-by: Wenchen Fan <wenchen@databricks.com>
## What changes were proposed in this pull request?
The PR starts from the [comment](https://github.com/apache/spark/pull/23124#discussion_r236112390) in the main one and it aims at:
- simplifying the code for `MapConcat`;
- be more precise in checking the limit size.
## How was this patch tested?
existing tests
Closes#23217 from mgaido91/SPARK-25829_followup.
Authored-by: Marco Gaido <marcogaido91@gmail.com>
Signed-off-by: Wenchen Fan <wenchen@databricks.com>
## What changes were proposed in this pull request?
When we encode a Decimal from external source we don't check for overflow. That method is useful not only in order to enforce that we can represent the correct value in the specified range, but it also changes the underlying data to the right precision/scale. Since in our code generation we assume that a decimal has exactly the same precision and scale of its data type, missing to enforce it can lead to corrupted output/results when there are subsequent transformations.
## How was this patch tested?
added UT
Closes#23210 from mgaido91/SPARK-26233.
Authored-by: Marco Gaido <marcogaido91@gmail.com>
Signed-off-by: Dongjoon Hyun <dongjoon@apache.org>
## What changes were proposed in this pull request?
In the PR, I propose to use **java.time API** for parsing timestamps and dates from CSV content with microseconds precision. The SQL config `spark.sql.legacy.timeParser.enabled` allow to switch back to previous behaviour with using `java.text.SimpleDateFormat`/`FastDateFormat` for parsing/generating timestamps/dates.
## How was this patch tested?
It was tested by `UnivocityParserSuite`, `CsvExpressionsSuite`, `CsvFunctionsSuite` and `CsvSuite`.
Closes#23150 from MaxGekk/time-parser.
Lead-authored-by: Maxim Gekk <max.gekk@gmail.com>
Co-authored-by: Maxim Gekk <maxim.gekk@databricks.com>
Signed-off-by: Sean Owen <sean.owen@databricks.com>
## What changes were proposed in this pull request?
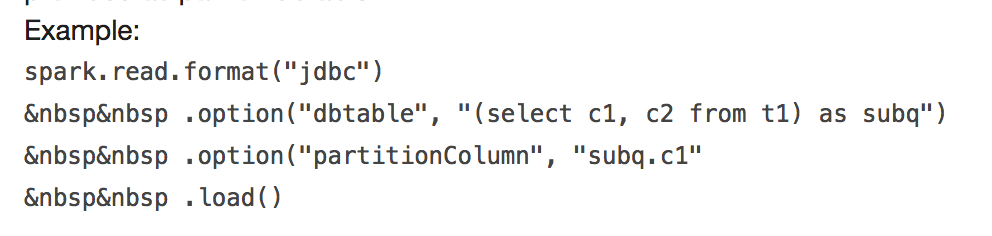
It will throw:
```
requirement failed: When reading JDBC data sources, users need to specify all or none for the following options: 'partitionColumn', 'lowerBound', 'upperBound', and 'numPartitions'
```
and
```
User-defined partition column subq.c1 not found in the JDBC relation ...
```
This PR fix this error example.
## How was this patch tested?
manual tests
Closes#23170 from wangyum/SPARK-24499.
Authored-by: Yuming Wang <yumwang@ebay.com>
Signed-off-by: Sean Owen <sean.owen@databricks.com>
## What changes were proposed in this pull request?
In the DDLSuit, there are four test cases have the same codes , writing a function can combine the same code.
## How was this patch tested?
existing tests.
Closes#23194 from CarolinePeng/Update_temp.
Authored-by: 彭灿00244106 <00244106@zte.intra>
Signed-off-by: Takeshi Yamamuro <yamamuro@apache.org>
## What changes were proposed in this pull request?
In SPARK-23711, we have implemented the expression fallback logic to an interpreted mode. So, this pr fixed code to support the same fallback mode in `SafeProjection` based on `CodeGeneratorWithInterpretedFallback`.
## How was this patch tested?
Add tests in `CodeGeneratorWithInterpretedFallbackSuite` and `UnsafeRowConverterSuite`.
Closes#22468 from maropu/SPARK-25374-3.
Authored-by: Takeshi Yamamuro <yamamuro@apache.org>
Signed-off-by: Wenchen Fan <wenchen@databricks.com>
## What changes were proposed in this pull request?
Currently in the Analyzer, we have two methods 1) Resolve 2)ResolveExpressions that are called at different code paths to resolve attributes, column ordinal and extract value expressions. ~~In this PR, we combine the two into one method to make sure, there is only one method that is tasked with resolving the attributes.~~
Update the description of the methods and use better names to make it easier to know when to make use of one method vs the other.
## How was this patch tested?
Existing tests.
Closes#22899 from dilipbiswal/SPARK-25573-final.
Authored-by: Dilip Biswal <dbiswal@us.ibm.com>
Signed-off-by: gatorsmile <gatorsmile@gmail.com>
## What changes were proposed in this pull request?
This pr is to return an empty config set when regenerating the golden files in `SQLQueryTestSuite`.
This is the follow-up of #22512.
## How was this patch tested?
N/A
Closes#23212 from maropu/SPARK-25498-FOLLOWUP.
Authored-by: Takeshi Yamamuro <yamamuro@apache.org>
Signed-off-by: Wenchen Fan <wenchen@databricks.com>
## What changes were proposed in this pull request?
Since `AggregationIterator` uses `MutableProjection` for `UnsafeRow`, `InterpretedMutableProjection` needs to handle `UnsafeRow` as buffer internally for fixed-length types only.
## How was this patch tested?
Run 'SQLQueryTestSuite' with the interpreted mode.
Closes#22512 from maropu/InterpreterTest.
Authored-by: Takeshi Yamamuro <yamamuro@apache.org>
Signed-off-by: Wenchen Fan <wenchen@databricks.com>
## What changes were proposed in this pull request?
For now the `hasMinMaxStats` will return the same as `hasCountStats`, which is obviously not as expected.
## How was this patch tested?
Existing tests.
Closes#23152 from adrian-wang/minmaxstats.
Authored-by: Daoyuan Wang <me@daoyuan.wang>
Signed-off-by: Wenchen Fan <wenchen@databricks.com>
## What changes were proposed in this pull request?
Partition column name is required to be unique under the same directory. The following paths are invalid partitioned directory:
```
hdfs://host:9000/path/a=1
hdfs://host:9000/path/b=2
```
If case sensitive, the following paths should be invalid too:
```
hdfs://host:9000/path/a=1
hdfs://host:9000/path/A=2
```
Since column 'a' and 'A' are different, and it is wrong to use either one as the column name in partition schema.
Also, there is a `TODO` comment in the code. Currently the Spark doesn't validate such case when `CASE_SENSITIVE` enabled.
This PR is to resolve the problem.
## How was this patch tested?
Add unit test
Closes#23186 from gengliangwang/SPARK-26230.
Authored-by: Gengliang Wang <gengliang.wang@databricks.com>
Signed-off-by: Wenchen Fan <wenchen@databricks.com>
## What changes were proposed in this pull request?
In the PR, I propose to change behaviour of `UnivocityParser` and `FailureSafeParser`, and return all fields that were parsed and converted to expected types successfully instead of just returning a row with all `null`s for a bad input in the `PERMISSIVE` mode. For example, for CSV line `0,2013-111-11 12:13:14` and DDL schema `a int, b timestamp`, new result is `Row(0, null)`.
## How was this patch tested?
It was checked by existing tests from `CsvSuite` and `CsvFunctionsSuite`.
Closes#23120 from MaxGekk/failuresafe-partial-result.
Authored-by: Maxim Gekk <max.gekk@gmail.com>
Signed-off-by: Wenchen Fan <wenchen@databricks.com>
## What changes were proposed in this pull request?
When build hash Map with one row of data and run out of memory, we should throw a SparkOutOfMemoryError exception, which is more accurate than SparkException. this PR fix it.
## How was this patch tested?
N / A
Closes#23190 from heary-cao/throwUnsafeHashedRelation.
Authored-by: caoxuewen <cao.xuewen@zte.com.cn>
Signed-off-by: Wenchen Fan <wenchen@databricks.com>
## What changes were proposed in this pull request?
How to reproduce this issue:
```scala
scala> val meta = new org.apache.spark.sql.types.MetadataBuilder().putNull("key").build().json
java.lang.NullPointerException
at org.apache.spark.sql.types.Metadata$.org$apache$spark$sql$types$Metadata$$toJsonValue(Metadata.scala:196)
at org.apache.spark.sql.types.Metadata$$anonfun$1.apply(Metadata.scala:180)
```
This pr fix `NullPointerException` when `Metadata` serialize `null` values.
## How was this patch tested?
unit tests
Closes#23164 from wangyum/SPARK-26198.
Authored-by: Yuming Wang <yumwang@ebay.com>
Signed-off-by: Sean Owen <sean.owen@databricks.com>
## What changes were proposed in this pull request?
Add headers to empty csv files when header=true, because otherwise these files are invalid when reading.
## How was this patch tested?
Added test for roundtrip of empty dataframe to csv file with headers and back in CSVSuite
Please review http://spark.apache.org/contributing.html before opening a pull request.
Closes#23173 from koertkuipers/feat-empty-csv-with-header.
Authored-by: Koert Kuipers <koert@tresata.com>
Signed-off-by: Hyukjin Kwon <gurwls223@apache.org>
## What changes were proposed in this pull request?
UnsupportedOperationException messages are not the same with method name.This PR correct these messages.
## How was this patch tested?
NA
Closes#23154 from lcqzte10192193/wid-lcq-1127.
Authored-by: lichaoqun <li.chaoqun@zte.com.cn>
Signed-off-by: Wenchen Fan <wenchen@databricks.com>
## What changes were proposed in this pull request?
It's a bad idea to use case class as public API, as it has a very wide surface. For example, the `copy` method, its fields, the companion object, etc.
For a particular case, `UserDefinedFunction`. It has a private constructor, and I believe we only want users to access a few methods:`apply`, `nullable`, `asNonNullable`, etc.
However, all its fields, and `copy` method, and the companion object are public unexpectedly. As a result, we made many tricks to work around the binary compatibility issues.
This PR proposes to only make interfaces public, and hide implementations behind with a private class. Now `UserDefinedFunction` is a pure trait, and the concrete implementation is `SparkUserDefinedFunction`, which is private.
Changing class to interface is not binary compatible(but source compatible), so 3.0 is a good chance to do it.
This is the first PR to go with this direction. If it's accepted, I'll create a umbrella JIRA and fix all the public case classes.
## How was this patch tested?
existing tests.
Closes#23178 from cloud-fan/udf.
Authored-by: Wenchen Fan <wenchen@databricks.com>
Signed-off-by: Wenchen Fan <wenchen@databricks.com>
## What changes were proposed in this pull request?
In the PR, I propose filtering out all empty files inside of `FileSourceScanExec` and exclude them from file splits. It should reduce overhead of opening and reading files without any data, and as consequence datasources will not produce empty partitions for such files.
## How was this patch tested?
Added a test which creates an empty and non-empty files. If empty files are ignored in load, Text datasource in the `wholetext` mode must create only one partition for non-empty file.
Closes#23130 from MaxGekk/ignore-empty-files.
Authored-by: Maxim Gekk <max.gekk@gmail.com>
Signed-off-by: Wenchen Fan <wenchen@databricks.com>
## What changes were proposed in this pull request?
This is a follow pr of #23176.
`In` and `InSet` are semantically equal, so the tests for `In` should pass with `InSet`, and vice versa.
This combines those test cases.
## How was this patch tested?
The combined tests and existing tests.
Closes#23187 from ueshin/issues/SPARK-26211/in_inset_tests.
Authored-by: Takuya UESHIN <ueshin@databricks.com>
Signed-off-by: Wenchen Fan <wenchen@databricks.com>
## What changes were proposed in this pull request?
This is a small change for better debugging: to pass query uuid in IncrementalExecution, when we look at the QueryExecution in isolation to trace back the query.
## How was this patch tested?
N/A - just add some field for better debugging.
Closes#23192 from rxin/SPARK-26241.
Authored-by: Reynold Xin <rxin@databricks.com>
Signed-off-by: gatorsmile <gatorsmile@gmail.com>
## What changes were proposed in this pull request?
In an earlier PR, we missed measuring the optimization phase time for streaming queries. This patch adds it.
## How was this patch tested?
Given this is a debugging feature, and it is very convoluted to add tests to verify the phase is set properly, I am not introducing a streaming specific test.
Closes#23193 from rxin/SPARK-26226-1.
Authored-by: Reynold Xin <rxin@databricks.com>
Signed-off-by: gatorsmile <gatorsmile@gmail.com>
## What changes were proposed in this pull request?
Correct some document description errors.
## How was this patch tested?
N/A
Closes#23162 from 10110346/docerror.
Authored-by: liuxian <liu.xian3@zte.com.cn>
Signed-off-by: Sean Owen <sean.owen@databricks.com>
## What changes were proposed in this pull request?
Currently, the common `withTempDir` function is used in Spark SQL test cases. To handle `val dir = Utils. createTempDir()` and `Utils. deleteRecursively (dir)`. Unfortunately, the `withTempDir` function cannot be used in the Spark Core test case. This PR Sharing `withTempDir` function in Spark Sql and SparkCore to clean up SparkCore test cases. thanks.
## How was this patch tested?
N / A
Closes#23151 from heary-cao/withCreateTempDir.
Authored-by: caoxuewen <cao.xuewen@zte.com.cn>
Signed-off-by: Hyukjin Kwon <gurwls223@apache.org>
## What changes were proposed in this pull request?
This patch changes the query plan tracker added earlier to report phase timeline, rather than just a duration for each phase. This way, we can easily find time that's unaccounted for.
## How was this patch tested?
Updated test cases to reflect that.
Closes#23183 from rxin/SPARK-26226.
Authored-by: Reynold Xin <rxin@databricks.com>
Signed-off-by: gatorsmile <gatorsmile@gmail.com>
## What changes were proposed in this pull request?
This is the first step of the data source v2 API refactor [proposal](https://docs.google.com/document/d/1uUmKCpWLdh9vHxP7AWJ9EgbwB_U6T3EJYNjhISGmiQg/edit?usp=sharing)
It adds the new API for batch read, without removing the old APIs, as they are still needed for streaming sources.
More concretely, it adds
1. `TableProvider`, works like an anonymous catalog
2. `Table`, represents a structured data set.
3. `ScanBuilder` and `Scan`, a logical represents of data source scan
4. `Batch`, a physical representation of data source batch scan.
## How was this patch tested?
existing tests
Closes#23086 from cloud-fan/refactor-batch.
Authored-by: Wenchen Fan <wenchen@databricks.com>
Signed-off-by: gatorsmile <gatorsmile@gmail.com>
## What changes were proposed in this pull request?
This PR is to fix a regression introduced in: https://github.com/apache/spark/pull/21004/files#r236998030
If user specifies schema, Spark don't need to infer data type for of partition columns, otherwise the data type might not match with the one user provided.
E.g. for partition directory `p=4d`, after data type inference the column value will be `4.0`.
See https://issues.apache.org/jira/browse/SPARK-26188 for more details.
Note that user specified schema **might not cover all the data columns**:
```
val schema = new StructType()
.add("id", StringType)
.add("ex", ArrayType(StringType))
val df = spark.read
.schema(schema)
.format("parquet")
.load(src.toString)
assert(df.schema.toList === List(
StructField("ex", ArrayType(StringType)),
StructField("part", IntegerType), // inferred partitionColumn dataType
StructField("id", StringType))) // used user provided partitionColumn dataType
```
For the missing columns in user specified schema, Spark still need to infer their data types if `partitionColumnTypeInferenceEnabled` is enabled.
To implement the partially inference, refactor `PartitioningUtils.parsePartitions` and pass the user specified schema as parameter to cast partition values.
## How was this patch tested?
Add unit test.
Closes#23165 from gengliangwang/fixFileIndex.
Authored-by: Gengliang Wang <gengliang.wang@databricks.com>
Signed-off-by: Wenchen Fan <wenchen@databricks.com>
## What changes were proposed in this pull request?
Currently the `SET` command works without any warnings even if the specified key is for `SparkConf` entries and it has no effect because the command does not update `SparkConf`, but the behavior might confuse users. We should track `SparkConf` entries and make the command reject for such entries.
## How was this patch tested?
Added a test and existing tests.
Closes#23031 from ueshin/issues/SPARK-26060/set_command.
Authored-by: Takuya UESHIN <ueshin@databricks.com>
Signed-off-by: Wenchen Fan <wenchen@databricks.com>
## What changes were proposed in this pull request?
In the PR, I propose using of the locale option to parse decimals from CSV input. After the changes, `UnivocityParser` converts input string to `BigDecimal` and to Spark's Decimal by using `java.text.DecimalFormat`.
## How was this patch tested?
Added a test for the `en-US`, `ko-KR`, `ru-RU`, `de-DE` locales.
Closes#22979 from MaxGekk/decimal-parsing-locale.
Lead-authored-by: Maxim Gekk <maxim.gekk@databricks.com>
Co-authored-by: Maxim Gekk <max.gekk@gmail.com>
Signed-off-by: hyukjinkwon <gurwls223@apache.org>
## What changes were proposed in this pull request?
Follow up for https://github.com/apache/spark/pull/23128, move sql read metrics relatives to `SQLShuffleMetricsReporter`, in order to put sql shuffle read metrics relatives closer and avoid possible problem about forgetting update SQLShuffleMetricsReporter while new metrics added by others.
## How was this patch tested?
Existing tests.
Closes#23175 from xuanyuanking/SPARK-26142-follow.
Authored-by: Yuanjian Li <xyliyuanjian@gmail.com>
Signed-off-by: Reynold Xin <rxin@databricks.com>
## What changes were proposed in this pull request?
In the PR, I propose to postpone creation of `OutputStream`/`Univocity`/`JacksonGenerator` till the first row should be written. This prevents creation of empty files for empty partitions. So, no need to open and to read such files back while loading data from the location.
## How was this patch tested?
Added tests for Text, JSON and CSV datasource where empty dataset is written but should not produce any files.
Closes#23052 from MaxGekk/text-empty-files.
Lead-authored-by: Maxim Gekk <max.gekk@gmail.com>
Co-authored-by: Maxim Gekk <maxim.gekk@databricks.com>
Signed-off-by: Sean Owen <sean.owen@databricks.com>
## What changes were proposed in this pull request?
Currently `InSet` doesn't work properly for binary type, or struct and array type with null value in the set.
Because, as for binary type, the `HashSet` doesn't work properly for `Array[Byte]`, and as for struct and array type with null value in the set, the `ordering` will throw a `NPE`.
## How was this patch tested?
Added a few tests.
Closes#23176 from ueshin/issues/SPARK-26211/inset.
Authored-by: Takuya UESHIN <ueshin@databricks.com>
Signed-off-by: Wenchen Fan <wenchen@databricks.com>
## What changes were proposed in this pull request?
In the PR, I propose using of the locale option to parse (and infer) decimals from JSON input. After the changes, `JacksonParser` converts input string to `BigDecimal` and to Spark's Decimal by using `java.text.DecimalFormat`. New behaviour can be switched off via SQL config `spark.sql.legacy.decimalParsing.enabled`.
## How was this patch tested?
Added 2 tests to `JsonExpressionsSuite` for the `en-US`, `ko-KR`, `ru-RU`, `de-DE` locales:
- Inferring decimal type using locale from JSON field values
- Converting JSON field values to specified decimal type using the locales.
Closes#23132 from MaxGekk/json-decimal-parsing-locale.
Lead-authored-by: Maxim Gekk <max.gekk@gmail.com>
Co-authored-by: Maxim Gekk <maxim.gekk@databricks.com>
Signed-off-by: Wenchen Fan <wenchen@databricks.com>
## What changes were proposed in this pull request?
Currently duplicated map keys are not handled consistently. For example, map look up respects the duplicated key appears first, `Dataset.collect` only keeps the duplicated key appears last, `MapKeys` returns duplicated keys, etc.
This PR proposes to remove duplicated map keys with last wins policy, to follow Java/Scala and Presto. It only applies to built-in functions, as users can create map with duplicated map keys via private APIs anyway.
updated functions: `CreateMap`, `MapFromArrays`, `MapFromEntries`, `StringToMap`, `MapConcat`, `TransformKeys`.
For other places:
1. data source v1 doesn't have this problem, as users need to provide a java/scala map, which can't have duplicated keys.
2. data source v2 may have this problem. I've added a note to `ArrayBasedMapData` to ask the caller to take care of duplicated keys. In the future we should enforce it in the stable data APIs for data source v2.
3. UDF doesn't have this problem, as users need to provide a java/scala map. Same as data source v1.
4. file format. I checked all of them and only parquet does not enforce it. For backward compatibility reasons I change nothing but leave a note saying that the behavior will be undefined if users write map with duplicated keys to parquet files. Maybe we can add a config and fail by default if parquet files have map with duplicated keys. This can be done in followup.
## How was this patch tested?
updated tests and new tests
Closes#23124 from cloud-fan/map.
Authored-by: Wenchen Fan <wenchen@databricks.com>
Signed-off-by: Wenchen Fan <wenchen@databricks.com>
## What changes were proposed in this pull request?
https://github.com/apache/spark/pull/22326 made a mistake that, not all python UDFs are unevaluable in join condition. Only python UDFs that refer to attributes from both join side are unevaluable.
This PR fixes this mistake.
## How was this patch tested?
a new test
Closes#23153 from cloud-fan/join.
Authored-by: Wenchen Fan <wenchen@databricks.com>
Signed-off-by: Wenchen Fan <wenchen@databricks.com>
## What changes were proposed in this pull request?
a followup of https://github.com/apache/spark/pull/23043 . Add a test to show the minor behavior change introduced by #23043 , and add migration guide.
## How was this patch tested?
a new test
Closes#23141 from cloud-fan/follow.
Authored-by: Wenchen Fan <wenchen@databricks.com>
Signed-off-by: Wenchen Fan <wenchen@databricks.com>
## What changes were proposed in this pull request?
Implement codegen for `LocalTableScanExec` and `ExistingRDDExec`. Refactor to share code between `LocalTableScanExec`, `ExistingRDDExec`, `InputAdapter` and `RowDataSourceScanExec`.
The difference in `doProduce` between these four was that `ExistingRDDExec` and `RowDataSourceScanExec` triggered adding an `UnsafeProjection`, while `InputAdapter` and `LocalTableScanExec` did not.
In the new trait `InputRDDCodegen` I added a flag `createUnsafeProjection` which the operators set accordingly.
Note: `LocalTableScanExec` explicitly creates its input as `UnsafeRows`, so it was obvious why it doesn't need an `UnsafeProjection`. But if an `InputAdapter` may take input that is `InternalRows` but not `UnsafeRows`, then I think it doesn't need an unsafe projection just because any other operator that is its parent would do that. That assumes that that any parent operator would always result in some `UnsafeProjection` being eventually added, and hence the output of the `WholeStageCodegen` unit would be `UnsafeRows`. If these assumptions hold, I think `createUnsafeProjection` could be set to `(parent == null)`.
Note: Do not codegen `LocalTableScanExec` when it's the only operator. `LocalTableScanExec` has optimized driver-only `executeCollect` and `executeTake` code paths that are used to return `Command` results without starting Spark Jobs. They can no longer be used if the `LocalTableScanExec` gets optimized.
## How was this patch tested?
Covered and used in existing tests.
Closes#23127 from juliuszsompolski/SPARK-26159.
Authored-by: Juliusz Sompolski <julek@databricks.com>
Signed-off-by: Wenchen Fan <wenchen@databricks.com>
## What changes were proposed in this pull request?
The purpose of this PR is supplement new test cases for a + 1,a + b and Rand in SetOperationSuite.
It comes from the comment of closed PR:#20541, thanks.
## How was this patch tested?
add new test cases
Closes#23138 from heary-cao/UnionPushTestCases.
Authored-by: caoxuewen <cao.xuewen@zte.com.cn>
Signed-off-by: Wenchen Fan <wenchen@databricks.com>
## What changes were proposed in this pull request?
Based on https://github.com/apache/spark/pull/22857 and https://github.com/apache/spark/pull/23079, this PR did a few updates
- Limit the data types of NULL to Boolean.
- Limit the input data type of replaceNullWithFalse to Boolean; throw an exception in the testing mode.
- Create a new file for the rule ReplaceNullWithFalseInPredicate
- Update the description of this rule.
## How was this patch tested?
Added a test case
Closes#23139 from gatorsmile/followupSpark-25860.
Authored-by: gatorsmile <gatorsmile@gmail.com>
Signed-off-by: DB Tsai <d_tsai@apple.com>
## What changes were proposed in this pull request?
This is follow-up of #21732. This patch inlines `isOptionType` method.
## How was this patch tested?
Existing tests.
Closes#23143 from viirya/SPARK-24762-followup.
Authored-by: Liang-Chi Hsieh <viirya@gmail.com>
Signed-off-by: Wenchen Fan <wenchen@databricks.com>
## What changes were proposed in this pull request?
This PR is to improve the code comments to document some common traits and traps about the expression.
## How was this patch tested?
N/A
Closes#23135 from gatorsmile/addcomments.
Authored-by: gatorsmile <gatorsmile@gmail.com>
Signed-off-by: Wenchen Fan <wenchen@databricks.com>
## What changes were proposed in this pull request?
Create a new suite DataFrameSetOperationsSuite for the test cases of DataFrame/Dataset's set operations.
Also, add test cases of NULL handling for Array Except and Array Intersect.
## How was this patch tested?
N/A
Closes#23137 from gatorsmile/setOpsTest.
Authored-by: gatorsmile <gatorsmile@gmail.com>
Signed-off-by: Wenchen Fan <wenchen@databricks.com>
## What changes were proposed in this pull request?
SparkSQL doesn't support to encode `Option[Product]` as a top-level row now, because in SparkSQL entire top-level row can't be null.
However for use cases like Aggregator, it is reasonable to use `Option[Product]` as buffer and output column types. Due to above limitation, we don't do it for now.
This patch proposes to encode `Option[Product]` at top-level as single struct column. So we can work around the issue that entire top-level row can't be null.
To summarize encoding of `Product` and `Option[Product]`.
For `Product`, 1. at root level, the schema is all fields are flatten it into multiple columns. The `Product ` can't be null, otherwise it throws an exception.
```scala
val df = Seq((1 -> "a"), (2 -> "b")).toDF()
df.printSchema()
root
|-- _1: integer (nullable = false)
|-- _2: string (nullable = true)
```
2. At non-root level, `Product` is a struct type column.
```scala
val df = Seq((1, (1 -> "a")), (2, (2 -> "b")), (3, null)).toDF()
df.printSchema()
root
|-- _1: integer (nullable = false)
|-- _2: struct (nullable = true)
| |-- _1: integer (nullable = false)
| |-- _2: string (nullable = true)
```
For `Option[Product]`, 1. it was not supported at root level. After this change, it is a struct type column.
```scala
val df = Seq(Some(1 -> "a"), Some(2 -> "b"), None).toDF()
df.printSchema
root
|-- value: struct (nullable = true)
| |-- _1: integer (nullable = false)
| |-- _2: string (nullable = true)
```
2. At non-root level, it is also a struct type column.
```scala
val df = Seq((1, Some(1 -> "a")), (2, Some(2 -> "b")), (3, None)).toDF()
df.printSchema
root
|-- _1: integer (nullable = false)
|-- _2: struct (nullable = true)
| |-- _1: integer (nullable = false)
| |-- _2: string (nullable = true)
```
3. For use case like Aggregator, it was not supported too. After this change, we support to use `Option[Product]` as buffer/output column type.
```scala
val df = Seq(
OptionBooleanIntData("bob", Some((true, 1))),
OptionBooleanIntData("bob", Some((false, 2))),
OptionBooleanIntData("bob", None)).toDF()
val group = df
.groupBy("name")
.agg(OptionBooleanIntAggregator("isGood").toColumn.alias("isGood"))
group.printSchema
root
|-- name: string (nullable = true)
|-- isGood: struct (nullable = true)
| |-- _1: boolean (nullable = false)
| |-- _2: integer (nullable = false)
```
The buffer and output type of `OptionBooleanIntAggregator` is both `Option[(Boolean, Int)`.
## How was this patch tested?
Added test.
Closes#21732 from viirya/SPARK-24762.
Authored-by: Liang-Chi Hsieh <viirya@gmail.com>
Signed-off-by: Wenchen Fan <wenchen@databricks.com>
## What changes were proposed in this pull request?
This PR is to add back `unionAll`, which is widely used. The name is also consistent with our ANSI SQL. We also have the corresponding `intersectAll` and `exceptAll`, which were introduced in Spark 2.4.
## How was this patch tested?
Added a test case in DataFrameSuite
Closes#23131 from gatorsmile/addBackUnionAll.
Authored-by: gatorsmile <gatorsmile@gmail.com>
Signed-off-by: gatorsmile <gatorsmile@gmail.com>
## What changes were proposed in this pull request?
The DOI foundation recommends [this new resolver](https://www.doi.org/doi_handbook/3_Resolution.html#3.8). Accordingly, this PR re`sed`s all static DOI links ;-)
## How was this patch tested?
It wasn't, since it seems as safe as a "[typo fix](https://spark.apache.org/contributing.html)".
In case any of the files is included from other projects, and should be updated there, please let me know.
Closes#23129 from katrinleinweber/resolve-DOIs-securely.
Authored-by: Katrin Leinweber <9948149+katrinleinweber@users.noreply.github.com>
Signed-off-by: Sean Owen <sean.owen@databricks.com>
## What changes were proposed in this pull request?
This patch defines an internal Spark interface for reporting shuffle metrics and uses that in shuffle reader. Before this patch, shuffle metrics is tied to a specific implementation (using a thread local temporary data structure and accumulators). After this patch, callers that define their own shuffle RDDs can create a custom metrics implementation.
With this patch, we would be able to create a better metrics for the SQL layer, e.g. reporting shuffle metrics in the SQL UI, for each exchange operator.
Note that I'm separating read side and write side implementations, as they are very different, to simplify code review. Write side change is at https://github.com/apache/spark/pull/23106
## How was this patch tested?
No behavior change expected, as it is a straightforward refactoring. Updated all existing test cases.
Closes#23105 from rxin/SPARK-26140.
Authored-by: Reynold Xin <rxin@databricks.com>
Signed-off-by: gatorsmile <gatorsmile@gmail.com>
## What changes were proposed in this pull request?
Fix Decimal `toScalaBigInt` and `toJavaBigInteger` used to only work for decimals not fitting long.
## How was this patch tested?
Added test to DecimalSuite.
Closes#23022 from juliuszsompolski/SPARK-26038.
Authored-by: Juliusz Sompolski <julek@databricks.com>
Signed-off-by: Herman van Hovell <hvanhovell@databricks.com>
## What changes were proposed in this pull request?
In the PR, I propose new options for CSV datasource - `lineSep` similar to Text and JSON datasource. The option allows to specify custom line separator of maximum length of 2 characters (because of a restriction in `uniVocity` parser). New option can be used in reading and writing CSV files.
## How was this patch tested?
Added a few tests with custom `lineSep` for enabled/disabled `multiLine` in read as well as tests in write. Also I added roundtrip tests.
Closes#23080 from MaxGekk/csv-line-sep.
Lead-authored-by: Maxim Gekk <max.gekk@gmail.com>
Co-authored-by: Maxim Gekk <maxim.gekk@databricks.com>
Signed-off-by: hyukjinkwon <gurwls223@apache.org>
## What changes were proposed in this pull request?
the pr #20014 which introduced `SparkOutOfMemoryError` to avoid killing the entire executor when an `OutOfMemoryError `is thrown.
so apply for memory using `MemoryConsumer. allocatePage `when catch exception, use `SparkOutOfMemoryError `instead of `OutOfMemoryError`
## How was this patch tested?
N / A
Closes#23084 from heary-cao/SparkOutOfMemoryError.
Authored-by: caoxuewen <cao.xuewen@zte.com.cn>
Signed-off-by: Wenchen Fan <wenchen@databricks.com>
GROUP BY treats -0.0 and 0.0 as different values which is unlike hive's behavior.
In addition current behavior with codegen is unpredictable (see example in JIRA ticket).
## What changes were proposed in this pull request?
In Platform.putDouble/Float() checking if the value is -0.0, and if so replacing with 0.0.
This is used by UnsafeRow so it won't have -0.0 values.
## How was this patch tested?
Added tests
Closes#23043 from adoron/adoron-spark-26021-replace-minus-zero-with-zero.
Authored-by: Alon Doron <adoron@palantir.com>
Signed-off-by: Wenchen Fan <wenchen@databricks.com>
## What changes were proposed in this pull request?
This is an addendum patch for SPARK-26129 that defines the edge case behavior for QueryPlanningTracker.topRulesByTime.
## How was this patch tested?
Added unit tests for each behavior.
Closes#23110 from rxin/SPARK-26129-1.
Authored-by: Reynold Xin <rxin@databricks.com>
Signed-off-by: Dongjoon Hyun <dongjoon@apache.org>
## What changes were proposed in this pull request?
When I ran spark-shell on JDK11+28(2018-09-25), It failed with the error below.
```
Exception in thread "main" java.lang.ExceptionInInitializerError
at org.apache.hadoop.util.StringUtils.<clinit>(StringUtils.java:80)
at org.apache.hadoop.security.SecurityUtil.getAuthenticationMethod(SecurityUtil.java:611)
at org.apache.hadoop.security.UserGroupInformation.initialize(UserGroupInformation.java:273)
at org.apache.hadoop.security.UserGroupInformation.ensureInitialized(UserGroupInformation.java:261)
at org.apache.hadoop.security.UserGroupInformation.loginUserFromSubject(UserGroupInformation.java:791)
at org.apache.hadoop.security.UserGroupInformation.getLoginUser(UserGroupInformation.java:761)
at org.apache.hadoop.security.UserGroupInformation.getCurrentUser(UserGroupInformation.java:634)
at org.apache.spark.util.Utils$.$anonfun$getCurrentUserName$1(Utils.scala:2427)
at scala.Option.getOrElse(Option.scala:121)
at org.apache.spark.util.Utils$.getCurrentUserName(Utils.scala:2427)
at org.apache.spark.SecurityManager.<init>(SecurityManager.scala:79)
at org.apache.spark.deploy.SparkSubmit.secMgr$lzycompute$1(SparkSubmit.scala:359)
at org.apache.spark.deploy.SparkSubmit.secMgr$1(SparkSubmit.scala:359)
at org.apache.spark.deploy.SparkSubmit.$anonfun$prepareSubmitEnvironment$9(SparkSubmit.scala:367)
at scala.Option.map(Option.scala:146)
at org.apache.spark.deploy.SparkSubmit.prepareSubmitEnvironment(SparkSubmit.scala:367)
at org.apache.spark.deploy.SparkSubmit.submit(SparkSubmit.scala:143)
at org.apache.spark.deploy.SparkSubmit.doSubmit(SparkSubmit.scala:86)
at org.apache.spark.deploy.SparkSubmit$$anon$2.doSubmit(SparkSubmit.scala:927)
at org.apache.spark.deploy.SparkSubmit$.main(SparkSubmit.scala:936)
at org.apache.spark.deploy.SparkSubmit.main(SparkSubmit.scala)
Caused by: java.lang.StringIndexOutOfBoundsException: begin 0, end 3, length 2
at java.base/java.lang.String.checkBoundsBeginEnd(String.java:3319)
at java.base/java.lang.String.substring(String.java:1874)
at org.apache.hadoop.util.Shell.<clinit>(Shell.java:52)
```
This is a Hadoop issue that fails to parse some java.version. It has been fixed from Hadoop-2.7.4(see [HADOOP-14586](https://issues.apache.org/jira/browse/HADOOP-14586)).
Note, Hadoop-2.7.5 or upper have another problem with Spark ([SPARK-25330](https://issues.apache.org/jira/browse/SPARK-25330)). So upgrading to 2.7.4 would be fine for now.
## How was this patch tested?
Existing tests.
Closes#23101 from tasanuma/SPARK-26134.
Authored-by: Takanobu Asanuma <tasanuma@apache.org>
Signed-off-by: Dongjoon Hyun <dongjoon@apache.org>
## What changes were proposed in this pull request?
The corrupt column specified via JSON/CSV option *columnNameOfCorruptRecord* must have the `string` type and be `nullable`. This has been already checked in `DataFrameReader`.`csv`/`json` and in `Json`/`CsvFileFormat` but not in `from_json`/`from_csv`. The PR adds such checks inside functions as well.
## How was this patch tested?
Added tests to `Json`/`CsvExpressionSuite` for checking type of the corrupt column. They don't check the `nullable` property because `schema` is forcibly casted to nullable.
Closes#23070 from MaxGekk/verify-corrupt-column-csv-json.
Authored-by: Maxim Gekk <max.gekk@gmail.com>
Signed-off-by: hyukjinkwon <gurwls223@apache.org>
## What changes were proposed in this pull request?
When doing typed aggregation on a Dataset, for struct key type, the key attribute is named as "key". But for non-struct type, the key attribute is named as "value". This key attribute should also be named as "key" for non-struct type.
## How was this patch tested?
Added test.
Closes#23054 from viirya/SPARK-26085.
Authored-by: Liang-Chi Hsieh <viirya@gmail.com>
Signed-off-by: Wenchen Fan <wenchen@databricks.com>
## What changes were proposed in this pull request?
An input without valid JSON tokens on the root level will be treated as a bad record, and handled according to `mode`. Previously such input was converted to `null`. After the changes, the input is converted to a row with `null`s in the `PERMISSIVE` mode according the schema. This allows to remove a code in the `from_json` function which can produce `null` as result rows.
## How was this patch tested?
It was tested by existing test suites. Some of them I have to modify (`JsonSuite` for example) because previously bad input was just silently ignored. For now such input is handled according to specified `mode`.
Closes#22938 from MaxGekk/json-nulls.
Lead-authored-by: Maxim Gekk <max.gekk@gmail.com>
Co-authored-by: Maxim Gekk <maxim.gekk@databricks.com>
Signed-off-by: Wenchen Fan <wenchen@databricks.com>
## What changes were proposed in this pull request?
In the PR, I propose:
- new SQL config `spark.sql.debug.maxToStringFields` to control maximum number fields up to which `truncatedString` cuts its input sequences.
- Moving `truncatedString` out of `core` to `sql/catalyst` because it is used only in the `sql/catalyst` packages for restricting number of fields converted to strings from `TreeNode` and expressions of`StructType`.
## How was this patch tested?
Added a test to `QueryExecutionSuite` to check that `spark.sql.debug.maxToStringFields` impacts to behavior of `truncatedString`.
Closes#23039 from MaxGekk/truncated-string-catalyst.
Lead-authored-by: Maxim Gekk <maxim.gekk@databricks.com>
Co-authored-by: Maxim Gekk <max.gekk@gmail.com>
Signed-off-by: Dongjoon Hyun <dongjoon@apache.org>
## What changes were proposed in this pull request?
We currently don't have good visibility into query planning time (analysis vs optimization vs physical planning). This patch adds a simple utility to track the runtime of various rules and various planning phases.
## How was this patch tested?
Added unit tests and end-to-end integration tests.
Closes#23096 from rxin/SPARK-26129.
Authored-by: Reynold Xin <rxin@databricks.com>
Signed-off-by: Reynold Xin <rxin@databricks.com>
## What changes were proposed in this pull request?
This change fixes a particular scenario where default spark SQL can't encode (thrift) types that are generated by twitter scrooge. These types are a trait that extends `scala.ProductX` with a constructor defined only in a companion object, rather than a actual case class. The actual case class used is child class, but that type is almost never referred to in code. The type has no corresponding constructor symbol and causes an exception. For all other purposes, these classes act just like case classes, so it is unfortunate that spark SQL can't serialize them nicely as it can actual case classes. For an full example of a scrooge codegen class, see https://gist.github.com/anonymous/ba13d4b612396ca72725eaa989900314.
This change catches the case where the type has no constructor but does have an `apply` method on the type's companion object. This allows for thrift types to be serialized/deserialized with implicit encoders the same way as normal case classes. This fix had to be done in three places where the constructor is assumed to be an actual constructor:
1) In serializing, determining the schema for the dataframe relies on inspecting its constructor (`ScalaReflection.constructParams`). Here we fall back to using the companion constructor arguments.
2) In deserializing or evaluating, in the java codegen ( `NewInstance.doGenCode`), the type couldn't be constructed with the new keyword. If there is no constructor, we change the constructor call to try the companion constructor.
3) In deserializing or evaluating, without codegen, the constructor is directly invoked (`NewInstance.constructor`). This was fixed with scala reflection to get the actual companion apply method.
The return type of `findConstructor` was changed because the companion apply method constructor can't be represented as a `java.lang.reflect.Constructor`.
There might be situations in which this approach would also fail in a new way, but it does at a minimum work for the specific scrooge example and will not impact cases that were already succeeding prior to this change
Note: this fix does not enable using scrooge thrift enums, additional work for this is necessary. With this patch, it seems like you could patch `com.twitter.scrooge.ThriftEnum` to extend `_root_.scala.Product1[Int]` with `def _1 = value` to get spark's implicit encoders to handle enums, but I've yet to use this method myself.
Note: I previously opened a PR for this issue, but only was able to fix case 1) there: https://github.com/apache/spark/pull/18766
## How was this patch tested?
I've fixed all 3 cases and added two tests that use a case class that is similar to scrooge generated one. The test in ScalaReflectionSuite checks 1), and the additional asserting in ObjectExpressionsSuite checks 2) and 3).
Closes#23062 from drewrobb/SPARK-8288.
Authored-by: Drew Robb <drewrobb@gmail.com>
Signed-off-by: Sean Owen <sean.owen@databricks.com>
## What changes were proposed in this pull request?
In the PR, I propose to pass the CSV option `encoding`/`charset` to `uniVocity` parser to allow parsing CSV files in different encodings when `multiLine` is enabled. The value of the option is passed to the `beginParsing` method of `CSVParser`.
## How was this patch tested?
Added new test to `CSVSuite` for different encodings and enabled/disabled header.
Closes#23091 from MaxGekk/csv-miltiline-encoding.
Authored-by: Maxim Gekk <maxim.gekk@databricks.com>
Signed-off-by: hyukjinkwon <gurwls223@apache.org>
## What changes were proposed in this pull request?
This PR fixes an exception in `AggregateExpression.references` called on unresolved expressions. It implements the solution proposed in [SPARK-26084](https://issues.apache.org/jira/browse/SPARK-26084), a minor refactoring that removes the unnecessary dependence on `AttributeSet.toSeq`, which requires expression IDs and, therefore, can only execute successfully for resolved expressions.
The refactored implementation is both simpler and faster, eliminating the conversion of a `Set` to a
`Seq` and back to `Set`.
## How was this patch tested?
Added a new test based on the failing case in [SPARK-26084](https://issues.apache.org/jira/browse/SPARK-26084).
hvanhovell
Closes#23075 from ssimeonov/ss_SPARK-26084.
Authored-by: Simeon Simeonov <sim@fastignite.com>
Signed-off-by: Herman van Hovell <hvanhovell@databricks.com>
## What changes were proposed in this pull request?
Extend the `ReplaceNullWithFalse` optimizer rule introduced in SPARK-25860 (https://github.com/apache/spark/pull/22857) to also support optimizing predicates in higher-order functions of `ArrayExists`, `ArrayFilter`, `MapFilter`.
Also rename the rule to `ReplaceNullWithFalseInPredicate` to better reflect its intent.
Example:
```sql
select filter(a, e -> if(e is null, null, true)) as b from (
select array(null, 1, null, 3) as a)
```
The optimized logical plan:
**Before**:
```
== Optimized Logical Plan ==
Project [filter([null,1,null,3], lambdafunction(if (isnull(lambda e#13)) null else true, lambda e#13, false)) AS b#9]
+- OneRowRelation
```
**After**:
```
== Optimized Logical Plan ==
Project [filter([null,1,null,3], lambdafunction(if (isnull(lambda e#13)) false else true, lambda e#13, false)) AS b#9]
+- OneRowRelation
```
## How was this patch tested?
Added new unit test cases to the `ReplaceNullWithFalseInPredicateSuite` (renamed from `ReplaceNullWithFalseSuite`).
Closes#23079 from rednaxelafx/catalyst-master.
Authored-by: Kris Mok <kris.mok@databricks.com>
Signed-off-by: Wenchen Fan <wenchen@databricks.com>
## What changes were proposed in this pull request?
The build has a lot of deprecation warnings. Some are new in Scala 2.12 and Java 11. We've fixed some, but I wanted to take a pass at fixing lots of easy miscellaneous ones here.
They're too numerous and small to list here; see the pull request. Some highlights:
- `BeanInfo` is deprecated in 2.12, and BeanInfo classes are pretty ancient in Java. Instead, case classes can explicitly declare getters
- Eta expansion of zero-arg methods; foo() becomes () => foo() in many cases
- Floating-point Range is inexact and deprecated, like 0.0 to 100.0 by 1.0
- finalize() is finally deprecated (just needs to be suppressed)
- StageInfo.attempId was deprecated and easiest to remove here
I'm not now going to touch some chunks of deprecation warnings:
- Parquet deprecations
- Hive deprecations (particularly serde2 classes)
- Deprecations in generated code (mostly Thriftserver CLI)
- ProcessingTime deprecations (we may need to revive this class as internal)
- many MLlib deprecations because they concern methods that may be removed anyway
- a few Kinesis deprecations I couldn't figure out
- Mesos get/setRole, which I don't know well
- Kafka/ZK deprecations (e.g. poll())
- Kinesis
- a few other ones that will probably resolve by deleting a deprecated method
## How was this patch tested?
Existing tests, including manual testing with the 2.11 build and Java 11.
Closes#23065 from srowen/SPARK-26090.
Authored-by: Sean Owen <sean.owen@databricks.com>
Signed-off-by: Sean Owen <sean.owen@databricks.com>
## What changes were proposed in this pull request?
Due to implementation limitation, currently Spark can't compare or do equality check between map types. As a result, map values can't appear in EQUAL or comparison expressions, can't be grouping key, etc.
The more important thing is, map loop up needs to do equality check of the map key, and thus can't support map as map key when looking up values from a map. Thus it's not useful to have map as map key.
This PR proposes to stop users from creating maps using map type as key. The list of expressions that are updated: `CreateMap`, `MapFromArrays`, `MapFromEntries`, `MapConcat`, `TransformKeys`. I manually checked all the places that create `MapType`, and came up with this list.
Note that, maps with map type key still exist, via reading from parquet files, converting from scala/java map, etc. This PR is not to completely forbid map as map key, but to avoid creating it by Spark itself.
Motivation: when I was trying to fix the duplicate key problem, I found it's impossible to do it with map type map key. I think it's reasonable to avoid map type map key for builtin functions.
## How was this patch tested?
updated test
Closes#23045 from cloud-fan/map-key.
Authored-by: Wenchen Fan <wenchen@databricks.com>
Signed-off-by: Wenchen Fan <wenchen@databricks.com>
Following [SPARK-26024](https://issues.apache.org/jira/browse/SPARK-26024), I noticed the number of elements in each partition after repartitioning using `df.repartitionByRange` can vary for the same setup:
```scala
// Shuffle numbers from 0 to 1000, and make a DataFrame
val df = Random.shuffle(0.to(1000)).toDF("val")
// Repartition it using 3 partitions
// Sum up number of elements in each partition, and collect it.
// And do it several times
for (i <- 0 to 9) {
var counts = df.repartitionByRange(3, col("val"))
.mapPartitions{part => Iterator(part.size)}
.collect()
println(counts.toList)
}
// -> the number of elements in each partition varies
```
This is expected as for performance reasons this method uses sampling to estimate the ranges (with default size of 100). Hence, the output may not be consistent, since sampling can return different values. But documentation was not mentioning it at all, leading to misunderstanding.
## What changes were proposed in this pull request?
Update the documentation (Spark & PySpark) to mention the impact of `spark.sql.execution.rangeExchange.sampleSizePerPartition` on the resulting partitioned DataFrame.
Closes#23025 from JulienPeloton/SPARK-26024.
Authored-by: Julien <peloton@lal.in2p3.fr>
Signed-off-by: Wenchen Fan <wenchen@databricks.com>
## What changes were proposed in this pull request?
The following 5 functions were removed from branch-2.4:
- map_entries
- map_filter
- transform_values
- transform_keys
- map_zip_with
We should update the since version to 3.0.0.
## How was this patch tested?
Existing tests.
Closes#23082 from ueshin/issues/SPARK-26112/since.
Authored-by: Takuya UESHIN <ueshin@databricks.com>
Signed-off-by: Wenchen Fan <wenchen@databricks.com>
## What changes were proposed in this pull request?
This restores scaladoc artifact generation, which got dropped with the Scala 2.12 update. The change looks large, but is almost all due to needing to make the InterfaceStability annotations top-level classes (i.e. `InterfaceStability.Stable` -> `Stable`), unfortunately. A few inner class references had to be qualified too.
Lots of scaladoc warnings now reappear. We can choose to disable generation by default and enable for releases, later.
## How was this patch tested?
N/A; build runs scaladoc now.
Closes#23069 from srowen/SPARK-26026.
Authored-by: Sean Owen <sean.owen@databricks.com>
Signed-off-by: Sean Owen <sean.owen@databricks.com>
## What changes were proposed in this pull request?
[Hive 2.3.4 is released on Nov. 7th](https://hive.apache.org/downloads.html#7-november-2018-release-234-available). This PR aims to support that version.
## How was this patch tested?
Pass the Jenkins with the updated version
Closes#23059 from dongjoon-hyun/SPARK-26091.
Authored-by: Dongjoon Hyun <dongjoon@apache.org>
Signed-off-by: Dongjoon Hyun <dongjoon@apache.org>
## What changes were proposed in this pull request?
Since [Spark 1.6.0](56d7da14ab (diff-6f38a103058a6e233b7ad80718452387R96)), there was a redundant '}' character in CTAS string plan's database argument string; `default}`. This PR aims to fix it.
**BEFORE**
```scala
scala> sc.version
res1: String = 1.6.0
scala> sql("create table t as select 1").explain
== Physical Plan ==
ExecutedCommand CreateTableAsSelect [Database:default}, TableName: t, InsertIntoHiveTable]
+- Project [1 AS _c0#3]
+- OneRowRelation$
```
**AFTER**
```scala
scala> sql("create table t as select 1").explain
== Physical Plan ==
Execute CreateHiveTableAsSelectCommand CreateHiveTableAsSelectCommand [Database:default, TableName: t, InsertIntoHiveTable]
+- *(1) Project [1 AS 1#4]
+- Scan OneRowRelation[]
```
## How was this patch tested?
Manual.
Closes#23064 from dongjoon-hyun/SPARK-FIX.
Authored-by: Dongjoon Hyun <dongjoon@apache.org>
Signed-off-by: hyukjinkwon <gurwls223@apache.org>
Events are dispatched on a separate thread, so need to wait for them to be
actually delivered before checking that the listener got them.
Closes#23050 from vanzin/SPARK-26079.
Authored-by: Marcelo Vanzin <vanzin@cloudera.com>
Signed-off-by: hyukjinkwon <gurwls223@apache.org>
## What changes were proposed in this pull request?
Use CheckpointFileManager to write the streaming `metadata` file so that the `metadata` file will never be a partial file.
## How was this patch tested?
Jenkins
Closes#23060 from zsxwing/SPARK-26092.
Authored-by: Shixiong Zhu <zsxwing@gmail.com>
Signed-off-by: Shixiong Zhu <zsxwing@gmail.com>
## What changes were proposed in this pull request?
remove invalid comment as we don't use it anymore
More details: https://github.com/apache/spark/pull/22976#discussion_r233764857
## How was this patch tested?
N/A
Closes#23044 from heary-cao/followUpOrdering.
Authored-by: caoxuewen <cao.xuewen@zte.com.cn>
Signed-off-by: Wenchen Fan <wenchen@databricks.com>
## What changes were proposed in this pull request?
This is a follow-up of #20393.
We should read the conf `"spark.sql.sort.enableRadixSort"` from `SQLConf` instead of `SparkConf`, i.e., use `SQLConf.get.enableRadixSort` instead of `SparkEnv.get.conf.get(SQLConf.RADIX_SORT_ENABLED)`, otherwise the config is never read.
## How was this patch tested?
Existing tests.
Closes#23046 from ueshin/issues/SPARK-23207/conf.
Authored-by: Takuya UESHIN <ueshin@databricks.com>
Signed-off-by: Wenchen Fan <wenchen@databricks.com>
## What changes were proposed in this pull request?
In SPARK-24865 `AnalysisBarrier` was removed and in order to improve resolution speed, the `analyzed` flag was (re-)introduced in order to process only plans which are not yet analyzed. This should not be the case when performing attribute deduplication as in that case we need to transform also the plans which were already analyzed, otherwise we can miss to rewrite some attributes leading to invalid plans.
## How was this patch tested?
added UT
Please review http://spark.apache.org/contributing.html before opening a pull request.
Closes#23035 from mgaido91/SPARK-26057.
Authored-by: Marco Gaido <marcogaido91@gmail.com>
Signed-off-by: Wenchen Fan <wenchen@databricks.com>
## What changes were proposed in this pull request?
This PR makes Spark's default Scala version as 2.12, and Scala 2.11 will be the alternative version. This implies that Scala 2.12 will be used by our CI builds including pull request builds.
We'll update the Jenkins to include a new compile-only jobs for Scala 2.11 to ensure the code can be still compiled with Scala 2.11.
## How was this patch tested?
existing tests
Closes#22967 from dbtsai/scala2.12.
Authored-by: DB Tsai <d_tsai@apple.com>
Signed-off-by: Dongjoon Hyun <dongjoon@apache.org>
## What changes were proposed in this pull request?
Add scala and java lint check rules to ban the usage of `throw new xxxErrors` and fix up all exists instance followed by https://github.com/apache/spark/pull/22989#issuecomment-437939830. See more details in https://github.com/apache/spark/pull/22969.
## How was this patch tested?
Local test with lint-scala and lint-java.
Closes#22989 from xuanyuanking/SPARK-25986.
Authored-by: Yuanjian Li <xyliyuanjian@gmail.com>
Signed-off-by: Sean Owen <sean.owen@databricks.com>
…. Other related changes to get JDK 11 working, to test
## What changes were proposed in this pull request?
- Access `sun.misc.Cleaner` (Java 8) and `jdk.internal.ref.Cleaner` (JDK 9+) by reflection (note: the latter only works if illegal reflective access is allowed)
- Access `sun.misc.Unsafe.invokeCleaner` in Java 9+ instead of `sun.misc.Cleaner` (Java 8)
In order to test anything on JDK 11, I also fixed a few small things, which I include here:
- Fix minor JDK 11 compile issues
- Update scala plugin, Jetty for JDK 11, to facilitate tests too
This doesn't mean JDK 11 tests all pass now, but lots do. Note also that the JDK 9+ solution for the Cleaner has a big caveat.
## How was this patch tested?
Existing tests. Manually tested JDK 11 build and tests, and tests covering this change appear to pass. All Java 8 tests should still pass, but this change alone does not achieve full JDK 11 compatibility.
Closes#22993 from srowen/SPARK-24421.
Authored-by: Sean Owen <sean.owen@databricks.com>
Signed-off-by: Sean Owen <sean.owen@databricks.com>
## What changes were proposed in this pull request?
As initializing lazy vals shares the same lock, a thread is trying to initialize `executedPlan` when `isRDD` is running, this thread will hang forever.
This PR just materializes `executedPlan` so that accessing it when `toRdd` is running doesn't need to wait for a lock
## How was this patch tested?
Jenkins
Closes#23023 from zsxwing/SPARK-26042.
Authored-by: Shixiong Zhu <zsxwing@gmail.com>
Signed-off-by: Shixiong Zhu <zsxwing@gmail.com>
## What changes were proposed in this pull request?
This is the official first attempt to break huge single `tests.py` file - I did it locally before few times and gave up for some reasons. Now, currently it really makes the unittests super hard to read and difficult to check. To me, it even bothers me to to scroll down the big file. It's one single 7000 lines file!
This is not only readability issue. Since one big test takes most of tests time, the tests don't run in parallel fully - although it will costs to start and stop the context.
We could pick up one example and follow. Given my investigation, the current style looks closer to NumPy structure and looks easier to follow. Please see https://github.com/numpy/numpy/tree/master/numpy.
Basically this PR proposes to break down `pyspark/sql/tests.py` into ...:
```bash
pyspark
...
├── sql
...
│ ├── tests # Includes all tests broken down from 'pyspark/sql/tests.py'
│ │ │ # Each matchs to module in 'pyspark/sql'. Additionally, some logical group can
│ │ │ # be added. For instance, 'test_arrow.py', 'test_datasources.py' ...
│ │ ├── __init__.py
│ │ ├── test_appsubmit.py
│ │ ├── test_arrow.py
│ │ ├── test_catalog.py
│ │ ├── test_column.py
│ │ ├── test_conf.py
│ │ ├── test_context.py
│ │ ├── test_dataframe.py
│ │ ├── test_datasources.py
│ │ ├── test_functions.py
│ │ ├── test_group.py
│ │ ├── test_pandas_udf.py
│ │ ├── test_pandas_udf_grouped_agg.py
│ │ ├── test_pandas_udf_grouped_map.py
│ │ ├── test_pandas_udf_scalar.py
│ │ ├── test_pandas_udf_window.py
│ │ ├── test_readwriter.py
│ │ ├── test_serde.py
│ │ ├── test_session.py
│ │ ├── test_streaming.py
│ │ ├── test_types.py
│ │ ├── test_udf.py
│ │ └── test_utils.py
...
├── testing # Includes testing utils that can be used in unittests.
│ ├── __init__.py
│ └── sqlutils.py
...
```
## How was this patch tested?
Existing tests should cover.
`cd python` and `./run-tests-with-coverage`. Manually checked they are actually being ran.
Each test (not officially) can be ran via:
```
SPARK_TESTING=1 ./bin/pyspark pyspark.sql.tests.test_pandas_udf_scalar
```
Note that if you're using Mac and Python 3, you might have to `OBJC_DISABLE_INITIALIZE_FORK_SAFETY=YES`.
Closes#23021 from HyukjinKwon/SPARK-25344.
Authored-by: hyukjinkwon <gurwls223@apache.org>
Signed-off-by: hyukjinkwon <gurwls223@apache.org>
## What changes were proposed in this pull request?
`Dataset.groupByKey` will bring in new attributes from serializer. If key type is the same as original Dataset's object type, they have same serializer output and so the attribute names will conflict.
This won't be a problem at most of cases, if we don't refer conflict attributes:
```scala
val ds: Dataset[(ClassData, Long)] = Seq(ClassData("one", 1), ClassData("two", 2)).toDS()
.map(c => ClassData(c.a, c.b + 1))
.groupByKey(p => p).count()
```
But if we use conflict attributes, `Analyzer` will complain about ambiguous references:
```scala
val ds = Seq(1, 2, 3).toDS()
val agg = ds.groupByKey(_ >= 2).agg(sum("value").as[Long], sum($"value" + 1).as[Long])
```
We have discussed two fixes https://github.com/apache/spark/pull/22944#discussion_r230977212:
1. Implicitly add alias to key attribute:
Works for primitive type. But for product type, we can't implicitly add aliases to key attributes because we might need to access key attributes by names in methods like `mapGroups`.
2. Detect conflict from key attributes and warn users to add alias manually
This might work, but needs to add some hacks to Analyzer or AttributeSeq.resolve.
This patch applies another simpler fix. We resolve aggregate expressions with `AppendColumns`'s children, instead of `AppendColumns`. `AppendColumns`'s output contains its children's output and serializer output, aggregate expressions shouldn't use serializer output.
## How was this patch tested?
Added test.
Closes#22944 from viirya/dataset_agg.
Authored-by: Liang-Chi Hsieh <viirya@gmail.com>
Signed-off-by: Wenchen Fan <wenchen@databricks.com>
## What changes were proposed in this pull request?
An expressions with a subquery can be pushed down as a data source filter. Despite the filter is not actively used, this causes anyway a re-execution of the subquery becuase the `ReuseSubquery` optimization rule is ineffective in this case.
The PR avoids this problem by forbidding the push down of filters containing a subquery.
## How was this patch tested?
added UT
Closes#22518 from mgaido91/SPARK-25482.
Authored-by: Marco Gaido <marcogaido91@gmail.com>
Signed-off-by: Wenchen Fan <wenchen@databricks.com>
## What changes were proposed in this pull request?
When sorting rows, ShuffleExchangeExec uses the entire row instead of just the columns references in SortOrder to create the RangePartitioner. This causes the RangePartitioner to sample entire rows to create rangeBounds and can cause OOM issues on the driver when rows contain large fields.
This change creates a projection and only use columns involved in the SortOrder for the RangePartitioner
## How was this patch tested?
Existing tests in spark-sql.
Plus
Started a local spark-shell with a small spark.driver.maxResultSize:
```
spark-shell --master 'local[16]' --conf spark.driver.maxResultSize=128M --driver-memory 4g
```
and ran the following script:
```
import com.google.common.io.Files
import org.apache.spark.SparkContext
import org.apache.spark.sql.SparkSession
import scala.util.Random
transient val sc = SparkContext.getOrCreate()
transient val spark = SparkSession.builder().getOrCreate()
import spark.implicits._
val path = Files.createTempDir().toString
// this creates a dataset with 1024 entries, each 1MB in size, across 16 partitions
sc.parallelize(0 until (1 << 10), sc.defaultParallelism).
map(_ => Array.fill(1 << 18)(Random.nextInt)).
toDS.
write.mode("overwrite").parquet(path)
spark.read.parquet(path).
orderBy('value (0)).
write.mode("overwrite").parquet(s"$path-sorted")
spark.read.parquet(s"$path-sorted").show
```
execution would fail when initializing RangePartitioner without this change.
execution succeeds and generates a correctly sorted dataset with this change.
Please review http://spark.apache.org/contributing.html before opening a pull request.
Closes#22961 from mu5358271/sort-improvement.
Authored-by: mu5358271 <shuheng.dai@gmail.com>
Signed-off-by: Wenchen Fan <wenchen@databricks.com>
## What changes were proposed in this pull request?
In the PR, I propose new method for debugging queries by dumping info about their execution to a file. It saves logical, optimized and physical plan similar to the `explain()` method + generated code. One of the advantages of the method over `explain` is it does not materializes full output as one string in memory which can cause OOMs.
## How was this patch tested?
Added a few tests to `QueryExecutionSuite` to check positive and negative scenarios.
Closes#23018 from MaxGekk/truncated-plan-to-file.
Authored-by: Maxim Gekk <maxim.gekk@databricks.com>
Signed-off-by: Herman van Hovell <hvanhovell@databricks.com>
## What changes were proposed in this pull request?
In `SQLAppStatusListener.aggregateMetrics`, we use the `metricIds` only to filter the relevant metrics. And this is a Seq which is also sorted. When there are many metrics involved, this can be pretty inefficient. The PR proposes to use a Set for it.
## How was this patch tested?
NA
Closes#23002 from mgaido91/SPARK-26003.
Authored-by: Marco Gaido <marcogaido91@gmail.com>
Signed-off-by: Wenchen Fan <wenchen@databricks.com>
## What changes were proposed in this pull request?
Passing current value of SQL config `spark.sql.columnNameOfCorruptRecord` to `CSVOptions` inside of `DataFrameReader`.`csv()`.
## How was this patch tested?
Added a test where default value of `spark.sql.columnNameOfCorruptRecord` is changed.
Closes#23006 from MaxGekk/csv-corrupt-sql-config.
Lead-authored-by: Maxim Gekk <maxim.gekk@databricks.com>
Co-authored-by: Dongjoon Hyun <dongjoon@apache.org>
Co-authored-by: Maxim Gekk <max.gekk@gmail.com>
Signed-off-by: hyukjinkwon <gurwls223@apache.org>
## What changes were proposed in this pull request?
As comment in https://github.com/apache/spark/pull/22326#issuecomment-424923967, we test the new added optimizer rule by end-to-end test in python side, need to add suites under `org.apache.spark.sql.catalyst.optimizer` like other optimizer rules.
## How was this patch tested?
new added UT
Closes#22955 from xuanyuanking/SPARK-25949.
Authored-by: Yuanjian Li <xyliyuanjian@gmail.com>
Signed-off-by: Wenchen Fan <wenchen@databricks.com>
## What changes were proposed in this pull request?
Deprecated in Java 11, replace Class.newInstance with Class.getConstructor.getInstance, and primtive wrapper class constructors with valueOf or equivalent
## How was this patch tested?
Existing tests.
Closes#22988 from srowen/SPARK-25984.
Authored-by: Sean Owen <sean.owen@databricks.com>
Signed-off-by: Sean Owen <sean.owen@databricks.com>
## What changes were proposed in this pull request?
Currently, Spark writes Spark version number into Hive Table properties with `spark.sql.create.version`.
```
parameters:{
spark.sql.sources.schema.part.0={
"type":"struct",
"fields":[{"name":"a","type":"integer","nullable":true,"metadata":{}}]
},
transient_lastDdlTime=1541142761,
spark.sql.sources.schema.numParts=1,
spark.sql.create.version=2.4.0
}
```
This PR aims to write Spark versions to ORC/Parquet file metadata with `org.apache.spark.sql.create.version` because we used `org.apache.` prefix in Parquet metadata already. It's different from Hive Table property key `spark.sql.create.version`, but it seems that we cannot change Hive Table property for backward compatibility.
After this PR, ORC and Parquet file generated by Spark will have the following metadata.
**ORC (`native` and `hive` implmentation)**
```
$ orc-tools meta /tmp/o
File Version: 0.12 with ...
...
User Metadata:
org.apache.spark.sql.create.version=3.0.0
```
**PARQUET**
```
$ parquet-tools meta /tmp/p
...
creator: parquet-mr version 1.10.0 (build 031a6654009e3b82020012a18434c582bd74c73a)
extra: org.apache.spark.sql.create.version = 3.0.0
extra: org.apache.spark.sql.parquet.row.metadata = {"type":"struct","fields":[{"name":"id","type":"long","nullable":false,"metadata":{}}]}
```
## How was this patch tested?
Pass the Jenkins with newly added test cases.
This closes#22255.
Closes#22932 from dongjoon-hyun/SPARK-25102.
Authored-by: Dongjoon Hyun <dongjoon@apache.org>
Signed-off-by: gatorsmile <gatorsmile@gmail.com>
## What changes were proposed in this pull request?
Very minor parser bug, but possibly problematic for code-generated queries:
Consider the following two queries:
```
SELECT avg(k) OVER (w) FROM kv WINDOW w AS (PARTITION BY v ORDER BY w) ORDER BY 1
```
and
```
SELECT avg(k) OVER w FROM kv WINDOW w AS (PARTITION BY v ORDER BY w) ORDER BY 1
```
The former, with parens around the OVER condition, fails to parse while the latter, without parens, succeeds:
```
Error in SQL statement: ParseException:
mismatched input '(' expecting {<EOF>, ',', 'FROM', 'WHERE', 'GROUP', 'ORDER', 'HAVING', 'LIMIT', 'LATERAL', 'WINDOW', 'UNION', 'EXCEPT', 'MINUS', 'INTERSECT', 'SORT', 'CLUSTER', 'DISTRIBUTE'}(line 1, pos 19)
== SQL ==
SELECT avg(k) OVER (w) FROM kv WINDOW w AS (PARTITION BY v ORDER BY w) ORDER BY 1
-------------------^^^
```
This was found when running the cockroach DB tests.
I tried PostgreSQL, The SQL with parentheses is also workable.
## How was this patch tested?
Unit test
Closes#22987 from gengliangwang/windowParentheses.
Authored-by: Gengliang Wang <gengliang.wang@databricks.com>
Signed-off-by: gatorsmile <gatorsmile@gmail.com>
## What changes were proposed in this pull request?
When the queries do not use the column names with the same case, users might hit various errors. Below is a typical test failure they can hit.
```
Expected only partition pruning predicates: ArrayBuffer(isnotnull(tdate#237), (cast(tdate#237 as string) >= 2017-08-15));
org.apache.spark.sql.AnalysisException: Expected only partition pruning predicates: ArrayBuffer(isnotnull(tdate#237), (cast(tdate#237 as string) >= 2017-08-15));
at org.apache.spark.sql.catalyst.catalog.ExternalCatalogUtils$.prunePartitionsByFilter(ExternalCatalogUtils.scala:146)
at org.apache.spark.sql.catalyst.catalog.InMemoryCatalog.listPartitionsByFilter(InMemoryCatalog.scala:560)
at org.apache.spark.sql.catalyst.catalog.SessionCatalog.listPartitionsByFilter(SessionCatalog.scala:925)
```
## How was this patch tested?
Added two test cases.
Closes#22990 from gatorsmile/fix1283.
Authored-by: gatorsmile <gatorsmile@gmail.com>
Signed-off-by: gatorsmile <gatorsmile@gmail.com>
## What changes were proposed in this pull request?
Remove `BenchmarkWithCodegen` as we don't use it anymore.
More details: https://github.com/apache/spark/pull/22484#discussion_r221397904
## How was this patch tested?
N/A
Closes#22985 from wangyum/SPARK-25510.
Authored-by: Yuming Wang <yumwang@ebay.com>
Signed-off-by: hyukjinkwon <gurwls223@apache.org>
## What changes were proposed in this pull request?
In the PR, I propose to add new option `locale` into CSVOptions/JSONOptions to make parsing date/timestamps in local languages possible. Currently the locale is hard coded to `Locale.US`.
## How was this patch tested?
Added two tests for parsing a date from CSV/JSON - `ноя 2018`.
Closes#22951 from MaxGekk/locale.
Authored-by: Maxim Gekk <max.gekk@gmail.com>
Signed-off-by: hyukjinkwon <gurwls223@apache.org>
## What changes were proposed in this pull request?
Since Spark 2.4.0 is released, we should test it in HiveExternalCatalogVersionsSuite
## How was this patch tested?
N/A
Closes#22984 from cloud-fan/minor.
Authored-by: Wenchen Fan <wenchen@databricks.com>
Signed-off-by: Dongjoon Hyun <dongjoon@apache.org>
## What changes were proposed in this pull request?
1. OrcReadBenchmark is under hive module, so the way to run it should be
```
build/sbt "hive/test:runMain <this class>"
```
2. The benchmark "String with Nulls Scan" should be with case "String with Nulls Scan(5%/50%/95%)", not "(0.05%/0.5%/0.95%)"
3. Add the null value percentages in the test case names of DataSourceReadBenchmark, for the benchmark "String with Nulls Scan" .
## How was this patch tested?
Re-run benchmarks
Closes#22965 from gengliangwang/fixHiveOrcReadBenchmark.
Lead-authored-by: Gengliang Wang <gengliang.wang@databricks.com>
Co-authored-by: Gengliang Wang <ltnwgl@gmail.com>
Co-authored-by: Dongjoon Hyun <dongjoon@apache.org>
Signed-off-by: Dongjoon Hyun <dongjoon@apache.org>
## What changes were proposed in this pull request?
#22970 fixed Scala 2.12 build error, and this PR updates the function according to the review comments.
## How was this patch tested?
This is also manually tested with Scala 2.12 build.
Closes#22978 from dongjoon-hyun/SPARK-25676-3.
Authored-by: Dongjoon Hyun <dongjoon@apache.org>
Signed-off-by: Wenchen Fan <wenchen@databricks.com>
## What changes were proposed in this pull request?
This is a follow-up pr of #20014 which introduced `SparkOutOfMemoryError` to avoid killing the entire executor when an `OutOfMemoryError` is thrown.
We should throw `SparkOutOfMemoryError` in `HashAggregateExec`, too.
## How was this patch tested?
Existing tests.
Closes#22969 from ueshin/issues/SPARK-22827/oome.
Authored-by: Takuya UESHIN <ueshin@databricks.com>
Signed-off-by: Dongjoon Hyun <dongjoon@apache.org>
## What changes were proposed in this pull request?
Currently, `SQLQueryTestSuite` is sensitive in terms of the bytes of parquet files in table partitions. If we change the default file format (from Parquet to ORC) or update the metadata of them, the test case should be changed accordingly. This PR aims to make `SQLQueryTestSuite` more robust by ignoring the partition byte statistics.
```
-Partition Statistics 1144 bytes, 2 rows
+Partition Statistics [not included in comparison] bytes, 2 rows
```
## How was this patch tested?
Pass the Jenkins with the newly updated test cases.
Closes#22972 from dongjoon-hyun/SPARK-25971.
Authored-by: Dongjoon Hyun <dongjoon@apache.org>
Signed-off-by: Dongjoon Hyun <dongjoon@apache.org>
## What changes were proposed in this pull request?
In the PR, I propose to port existing JSON tests from `JsonFunctionsSuite` that are applicable for CSV, and put them to `CsvFunctionsSuite`. In particular:
- roundtrip `from_csv` to `to_csv`, and `to_csv` to `from_csv`
- using `schema_of_csv` in `from_csv`
- Java API `from_csv`
- using `from_csv` and `to_csv` in exprs.
Closes#22960 from MaxGekk/csv-additional-tests.
Authored-by: Maxim Gekk <max.gekk@gmail.com>
Signed-off-by: hyukjinkwon <gurwls223@apache.org>
## What changes were proposed in this pull request?
The PR fixes an issue when the corrupt record column specified via `spark.sql.columnNameOfCorruptRecord` or JSON options `columnNameOfCorruptRecord` is propagated to JacksonParser, and returned row breaks an assumption in `FailureSafeParser` that the row must contain only actual data. The issue is fixed by passing actual schema without the corrupt record field into `JacksonParser`.
## How was this patch tested?
Added a test with the corrupt record column in the middle of user's schema.
Closes#22958 from MaxGekk/from_json-corrupt-record-schema.
Authored-by: Maxim Gekk <max.gekk@gmail.com>
Signed-off-by: hyukjinkwon <gurwls223@apache.org>
## What changes were proposed in this pull request?
This PR fixes the Scala-2.12 build.
## How was this patch tested?
Manual build with Scala-2.12 profile.
Closes#22970 from dongjoon-hyun/SPARK-25676-2.12.
Authored-by: Dongjoon Hyun <dongjoon@apache.org>
Signed-off-by: DB Tsai <d_tsai@apple.com>
## What changes were proposed in this pull request?
- Remove some AccumulableInfo .apply() methods
- Remove non-label-specific multiclass precision/recall/fScore in favor of accuracy
- Remove toDegrees/toRadians in favor of degrees/radians (SparkR: only deprecated)
- Remove approxCountDistinct in favor of approx_count_distinct (SparkR: only deprecated)
- Remove unused Python StorageLevel constants
- Remove Dataset unionAll in favor of union
- Remove unused multiclass option in libsvm parsing
- Remove references to deprecated spark configs like spark.yarn.am.port
- Remove TaskContext.isRunningLocally
- Remove ShuffleMetrics.shuffle* methods
- Remove BaseReadWrite.context in favor of session
- Remove Column.!== in favor of =!=
- Remove Dataset.explode
- Remove Dataset.registerTempTable
- Remove SQLContext.getOrCreate, setActive, clearActive, constructors
Not touched yet
- everything else in MLLib
- HiveContext
- Anything deprecated more recently than 2.0.0, generally
## How was this patch tested?
Existing tests
Closes#22921 from srowen/SPARK-25908.
Lead-authored-by: Sean Owen <sean.owen@databricks.com>
Co-authored-by: hyukjinkwon <gurwls223@apache.org>
Co-authored-by: Sean Owen <srowen@gmail.com>
Signed-off-by: Sean Owen <sean.owen@databricks.com>
JVMs can't allocate arrays of length exactly Int.MaxValue, so ensure we never try to allocate an array that big. This commit changes some defaults & configs to gracefully fallover to something that doesn't require one large array in some cases; in other cases it simply improves an error message for cases which will still fail.
Closes#22818 from squito/SPARK-25827.
Authored-by: Imran Rashid <irashid@cloudera.com>
Signed-off-by: Imran Rashid <irashid@cloudera.com>
## What changes were proposed in this pull request?
Fix for `CsvToStructs` to take into account SQL config `spark.sql.columnNameOfCorruptRecord` similar to `from_json`.
## How was this patch tested?
Added new test where `spark.sql.columnNameOfCorruptRecord` is set to corrupt column name different from default.
Closes#22956 from MaxGekk/csv-tests.
Authored-by: Maxim Gekk <max.gekk@gmail.com>
Signed-off-by: hyukjinkwon <gurwls223@apache.org>
## What changes were proposed in this pull request?
Refactor BenchmarkWideTable to use main method.
Generate benchmark result:
```
SPARK_GENERATE_BENCHMARK_FILES=1 build/sbt "sql/test:runMain org.apache.spark.sql.execution.benchmark.WideTableBenchmark"
```
## How was this patch tested?
manual tests
Closes#22823 from yucai/BenchmarkWideTable.
Lead-authored-by: yucai <yyu1@ebay.com>
Co-authored-by: Yucai Yu <yucai.yu@foxmail.com>
Co-authored-by: Dongjoon Hyun <dongjoon@apache.org>
Signed-off-by: Dongjoon Hyun <dongjoon@apache.org>
## What changes were proposed in this pull request?
Upgrade ASM to 7.x to support JDK11
## How was this patch tested?
Existing tests.
Closes#22953 from dbtsai/asm7.
Authored-by: DB Tsai <d_tsai@apple.com>
Signed-off-by: DB Tsai <d_tsai@apple.com>
## What changes were proposed in this pull request?
Currently definitions of config entries in `core` module are in several files separately. We should move them into `internal/config` to be easy to manage.
## How was this patch tested?
Existing tests.
Closes#22928 from ueshin/issues/SPARK-25926/single_config_file.
Authored-by: Takuya UESHIN <ueshin@databricks.com>
Signed-off-by: Wenchen Fan <wenchen@databricks.com>
## What changes were proposed in this pull request?
Fix typos and misspellings, per https://github.com/apache/spark-website/pull/158#issuecomment-435790366
## How was this patch tested?
Existing tests.
Closes#22950 from srowen/Typos.
Authored-by: Sean Owen <sean.owen@databricks.com>
Signed-off-by: Sean Owen <sean.owen@databricks.com>
## What changes were proposed in this pull request?
As per the discussion in [#22823](https://github.com/apache/spark/pull/22823/files#r228400706), add a new configuration to make the split threshold for the code generated function configurable.
When the generated Java function source code exceeds `spark.sql.codegen.methodSplitThreshold`, it will be split into multiple small functions.
## How was this patch tested?
manual tests
Closes#22847 from yucai/splitThreshold.
Authored-by: yucai <yyu1@ebay.com>
Signed-off-by: Wenchen Fan <wenchen@databricks.com>
## What changes were proposed in this pull request?
This is a follow-up pr of #22892 which moved `sample.json` from hive module to sql module, but we still need the file in hive module.
## How was this patch tested?
Existing tests.
Closes#22942 from ueshin/issues/SPARK-25884/sample.json.
Authored-by: Takuya UESHIN <ueshin@databricks.com>
Signed-off-by: Wenchen Fan <wenchen@databricks.com>
## What changes were proposed in this pull request?
In the PR, I propose to extend `UnaryExecNode` instead of `SparkPlan` by unary nodes.
Closes#22925 from MaxGekk/unary-exec-node.
Authored-by: Maxim Gekk <max.gekk@gmail.com>
Signed-off-by: gatorsmile <gatorsmile@gmail.com>
## What changes were proposed in this pull request?
New functions takes a struct and converts it to a CSV strings using passed CSV options. It accepts the same CSV options as CSV data source does.
## How was this patch tested?
Added `CsvExpressionsSuite`, `CsvFunctionsSuite` as well as R, Python and SQL tests similar to tests for `to_json()`
Closes#22626 from MaxGekk/to_csv.
Lead-authored-by: Maxim Gekk <max.gekk@gmail.com>
Co-authored-by: Maxim Gekk <maxim.gekk@databricks.com>
Signed-off-by: hyukjinkwon <gurwls223@apache.org>
## What changes were proposed in this pull request?
Added new benchmark which forcibly invokes Jackson parser to check overhead of its creation for short and wide JSON strings. Existing benchmarks do not allow to check that due to an optimisation introduced by #21909 for empty schema pushed down to JSON datasource. The `count()` action passes empty schema as required schema to the datasource, and Jackson parser is not created at all in that case.
Besides of new benchmark I also refactored existing benchmarks:
- Added `numIters` to control number of iteration in each benchmark
- Renamed `JSON per-line parsing` -> `count a short column`, `JSON parsing of wide lines` -> `count a wide column`, and `Count a dataset with 10 columns` -> `Select a subset of 10 columns`.
Closes#22920 from MaxGekk/json-benchmark-follow-up.
Lead-authored-by: Maxim Gekk <max.gekk@gmail.com>
Co-authored-by: Dongjoon Hyun <dongjoon@apache.org>
Signed-off-by: Dongjoon Hyun <dongjoon@apache.org>
## What changes were proposed in this pull request?
'refreshInterval' is not used any where in the headerSparkPage method. So, we don't need to pass the parameter while calling the 'headerSparkPage' method.
## How was this patch tested?
Existing tests
Closes#22864 from shahidki31/unusedCode.
Authored-by: Shahid <shahidki31@gmail.com>
Signed-off-by: Sean Owen <sean.owen@databricks.com>
## What changes were proposed in this pull request?
Unfortunately, it seems that we missed this in 2.4.0. In Spark 2.4, if the default file system is not the local file system, `LOAD DATA LOCAL INPATH` only works in case of absolute paths. This PR aims to fix it to support relative paths. This is a regression in 2.4.0.
```scala
$ ls kv1.txt
kv1.txt
scala> spark.sql("LOAD DATA LOCAL INPATH 'kv1.txt' INTO TABLE t")
org.apache.spark.sql.AnalysisException: LOAD DATA input path does not exist: kv1.txt;
```
## How was this patch tested?
Pass the Jenkins
Closes#22927 from dongjoon-hyun/SPARK-LOAD.
Authored-by: Dongjoon Hyun <dongjoon@apache.org>
Signed-off-by: Dongjoon Hyun <dongjoon@apache.org>
## What changes were proposed in this pull request?
a followup of https://github.com/apache/spark/pull/22749.
When we construct the new serializer in `ExpressionEncoder.tuple`, we don't need to add `if(isnull ...)` check for each field. They are either simple expressions that can propagate null correctly(e.g. `GetStructField(GetColumnByOrdinal(0, schema), index)`), or complex expression that already have the isnull check.
## How was this patch tested?
existing tests
Closes#22898 from cloud-fan/minor.
Authored-by: Wenchen Fan <wenchen@databricks.com>
Signed-off-by: Wenchen Fan <wenchen@databricks.com>
## What changes were proposed in this pull request?
When `SHOW CREATE TABLE` for Datasource tables, we are missing `TBLPROPERTIES` and `COMMENT`, and we should use `LOCATION` instead of path in `OPTION`.
## How was this patch tested?
Splitted `ShowCreateTableSuite` to confirm to work with both `InMemoryCatalog` and `HiveExternalCatalog`, and added some tests.
Closes#22892 from ueshin/issues/SPARK-25884/show_create_table.
Authored-by: Takuya UESHIN <ueshin@databricks.com>
Signed-off-by: Wenchen Fan <wenchen@databricks.com>
## What changes were proposed in this pull request?
In the PR, I propose to add new function - *schema_of_csv()* which infers schema of CSV string literal. The result of the function is a string containing a schema in DDL format. For example:
```sql
select schema_of_csv('1|abc', map('delimiter', '|'))
```
```
struct<_c0:int,_c1:string>
```
## How was this patch tested?
Added new tests to `CsvFunctionsSuite`, `CsvExpressionsSuite` and SQL tests to `csv-functions.sql`
Closes#22666 from MaxGekk/schema_of_csv-function.
Lead-authored-by: hyukjinkwon <gurwls223@apache.org>
Co-authored-by: Maxim Gekk <maxim.gekk@databricks.com>
Signed-off-by: hyukjinkwon <gurwls223@apache.org>
## What changes were proposed in this pull request?
This PR proposes a new optimization rule that replaces `Literal(null, _)` with `FalseLiteral` in conditions in `Join` and `Filter`, predicates in `If`, conditions in `CaseWhen`.
The idea is that some expressions evaluate to `false` if the underlying expression is `null` (as an example see `GeneratePredicate$create` or `doGenCode` and `eval` methods in `If` and `CaseWhen`). Therefore, we can replace `Literal(null, _)` with `FalseLiteral`, which can lead to more optimizations later on.
Let’s consider a few examples.
```
val df = spark.range(1, 100).select($"id".as("l"), ($"id" > 50).as("b"))
df.createOrReplaceTempView("t")
df.createOrReplaceTempView("p")
```
**Case 1**
```
spark.sql("SELECT * FROM t WHERE if(l > 10, false, NULL)").explain(true)
// without the new rule
…
== Optimized Logical Plan ==
Project [id#0L AS l#2L, cast(id#0L as string) AS s#3]
+- Filter if ((id#0L > 10)) false else null
+- Range (1, 100, step=1, splits=Some(12))
== Physical Plan ==
*(1) Project [id#0L AS l#2L, cast(id#0L as string) AS s#3]
+- *(1) Filter if ((id#0L > 10)) false else null
+- *(1) Range (1, 100, step=1, splits=12)
// with the new rule
…
== Optimized Logical Plan ==
LocalRelation <empty>, [l#2L, s#3]
== Physical Plan ==
LocalTableScan <empty>, [l#2L, s#3]
```
**Case 2**
```
spark.sql("SELECT * FROM t WHERE CASE WHEN l < 10 THEN null WHEN l > 40 THEN false ELSE null END”).explain(true)
// without the new rule
...
== Optimized Logical Plan ==
Project [id#0L AS l#2L, cast(id#0L as string) AS s#3]
+- Filter CASE WHEN (id#0L < 10) THEN null WHEN (id#0L > 40) THEN false ELSE null END
+- Range (1, 100, step=1, splits=Some(12))
== Physical Plan ==
*(1) Project [id#0L AS l#2L, cast(id#0L as string) AS s#3]
+- *(1) Filter CASE WHEN (id#0L < 10) THEN null WHEN (id#0L > 40) THEN false ELSE null END
+- *(1) Range (1, 100, step=1, splits=12)
// with the new rule
...
== Optimized Logical Plan ==
LocalRelation <empty>, [l#2L, s#3]
== Physical Plan ==
LocalTableScan <empty>, [l#2L, s#3]
```
**Case 3**
```
spark.sql("SELECT * FROM t JOIN p ON IF(t.l > p.l, null, false)").explain(true)
// without the new rule
...
== Optimized Logical Plan ==
Join Inner, if ((l#2L > l#37L)) null else false
:- Project [id#0L AS l#2L, cast(id#0L as string) AS s#3]
: +- Range (1, 100, step=1, splits=Some(12))
+- Project [id#0L AS l#37L, cast(id#0L as string) AS s#38]
+- Range (1, 100, step=1, splits=Some(12))
== Physical Plan ==
BroadcastNestedLoopJoin BuildRight, Inner, if ((l#2L > l#37L)) null else false
:- *(1) Project [id#0L AS l#2L, cast(id#0L as string) AS s#3]
: +- *(1) Range (1, 100, step=1, splits=12)
+- BroadcastExchange IdentityBroadcastMode
+- *(2) Project [id#0L AS l#37L, cast(id#0L as string) AS s#38]
+- *(2) Range (1, 100, step=1, splits=12)
// with the new rule
...
== Optimized Logical Plan ==
LocalRelation <empty>, [l#2L, s#3, l#37L, s#38]
```
## How was this patch tested?
This PR comes with a set of dedicated tests.
Closes#22857 from aokolnychyi/spark-25860.
Authored-by: Anton Okolnychyi <aokolnychyi@apple.com>
Signed-off-by: DB Tsai <d_tsai@apple.com>
## What changes were proposed in this pull request?
When `spark.sql.hive.metastore.version` is misconfigured, we had better give a directional error message.
**BEFORE**
```scala
scala> sql("show databases").show
scala.MatchError: 2.4 (of class java.lang.String)
```
**AFTER**
```scala
scala> sql("show databases").show
java.lang.UnsupportedOperationException: Unsupported Hive Metastore version (2.4).
Please set spark.sql.hive.metastore.version with a valid version.
```
## How was this patch tested?
Manual.
Closes#22902 from dongjoon-hyun/SPARK-25893.
Authored-by: Dongjoon Hyun <dongjoon@apache.org>
Signed-off-by: Dongjoon Hyun <dongjoon@apache.org>
## What changes were proposed in this pull request?
Currently in `FailureSafeParser` and `from_avro`, the exception is created with such code
```
throw new SparkException("Malformed records are detected in record parsing. " +
s"Parse Mode: ${FailFastMode.name}.", e.cause)
```
1. The cause part should be `e` instead of `e.cause`
2. If `e` contains non-null message, it should be shown in `from_json`/`from_csv`/`from_avro`, e.g.
```
com.fasterxml.jackson.core.JsonParseException: Unexpected character ('1' (code 49)): was expecting a colon to separate field name and value
at [Source: (InputStreamReader); line: 1, column: 7]
```
3.Kindly show hint for trying PERMISSIVE in error message.
## How was this patch tested?
Unit test.
Closes#22895 from gengliangwang/improve_error_msg.
Authored-by: Gengliang Wang <gengliang.wang@databricks.com>
Signed-off-by: hyukjinkwon <gurwls223@apache.org>
## What changes were proposed in this pull request?
Refactor BuiltInDataSourceWriteBenchmark, DataSourceWriteBenchmark and AvroWriteBenchmark to use main method.
```
SPARK_GENERATE_BENCHMARK_FILES=1 build/sbt "sql/test:runMain org.apache.spark.sql.execution.benchmark.BuiltInDataSourceWriteBenchmark"
SPARK_GENERATE_BENCHMARK_FILES=1 build/sbt "avro/test:runMain org.apache.spark.sql.execution.benchmark.AvroWriteBenchmark"
```
## How was this patch tested?
manual tests
Closes#22861 from yucai/BuiltInDataSourceWriteBenchmark.
Lead-authored-by: yucai <yyu1@ebay.com>
Co-authored-by: Yucai Yu <yucai.yu@foxmail.com>
Co-authored-by: Dongjoon Hyun <dongjoon@apache.org>
Signed-off-by: Dongjoon Hyun <dongjoon@apache.org>
## What changes were proposed in this pull request?
This patch removes the rangeBetween functions introduced in SPARK-21608. As explained in SPARK-25841, these functions are confusing and don't quite work. We will redesign them and introduce better ones in SPARK-25843.
## How was this patch tested?
Removed relevant test cases as well. These test cases will need to be added back in SPARK-25843.
Closes#22870 from rxin/SPARK-25862.
Lead-authored-by: Reynold Xin <rxin@databricks.com>
Co-authored-by: hyukjinkwon <gurwls223@apache.org>
Signed-off-by: gatorsmile <gatorsmile@gmail.com>
## What changes were proposed in this pull request?
Refactor JSONBenchmark to use main method
use spark-submit:
`bin/spark-submit --class org.apache.spark.sql.execution.datasources.json.JSONBenchmark --jars ./core/target/spark-core_2.11-3.0.0-SNAPSHOT-tests.jar,./sql/catalyst/target/spark-catalyst_2.11-3.0.0-SNAPSHOT-tests.jar ./sql/core/target/spark-sql_2.11-3.0.0-SNAPSHOT-tests.jar`
Generate benchmark result:
`SPARK_GENERATE_BENCHMARK_FILES=1 build/sbt "sql/test:runMain org.apache.spark.sql.execution.datasources.json.JSONBenchmark"`
## How was this patch tested?
manual tests
Closes#22844 from heary-cao/JSONBenchmarks.
Lead-authored-by: caoxuewen <cao.xuewen@zte.com.cn>
Co-authored-by: heary <cao.xuewen@zte.com.cn>
Co-authored-by: Dongjoon Hyun <dongjoon@apache.org>
Signed-off-by: hyukjinkwon <gurwls223@apache.org>
## What changes were proposed in this pull request?
When we compare attributes, in general, we should always refer to semantic equality, as the default `equal` method can return false when there are "cosmetic" differences between them, but still they are the same thing; at least we have to consider them so when analyzing/optimizing queries.
The PR focuses on the usage and comparison of the `output` of a `LogicalPlan`, which is a `Seq[Attribute]` in `AliasViewChild`. In this case, using equality implicitly fails to check the semantic equality. This results in the operator failing to stabilize.
## How was this patch tested?
running the tests with the patch provided by maryannxue in https://github.com/apache/spark/pull/22060Closes#22713 from mgaido91/SPARK-25691.
Authored-by: Marco Gaido <marcogaido91@gmail.com>
Signed-off-by: Wenchen Fan <wenchen@databricks.com>
## What changes were proposed in this pull request?
use spark-submit:
`bin/spark-submit --class org.apache.spark.sql.execution.datasources.csv.CSVBenchmark --jars ./core/target/spark-core_2.11-3.0.0-SNAPSHOT-tests.jar,./sql/catalyst/target/spark-catalyst_2.11-3.0.0-SNAPSHOT-tests.jar ./sql/core/target/spark-sql_2.11-3.0.0-SNAPSHOT-tests.jar`
Generate benchmark result:
`SPARK_GENERATE_BENCHMARK_FILES=1 build/sbt "sql/test:runMain org.apache.spark.sql.execution.datasources.csv.CSVBenchmark"`
## How was this patch tested?
manual tests
Closes#22845 from heary-cao/CSVBenchmarks.
Authored-by: caoxuewen <cao.xuewen@zte.com.cn>
Signed-off-by: Dongjoon Hyun <dongjoon@apache.org>
## What changes were proposed in this pull request?
Currently, the BroadcastHashJoinExec physical plan supports CodeGen and non-codegen, but only CodeGen code is tested in the unit tests of InnerJoinSuite、OuterJoinSuite、ExistenceJoinSuite, and non-codegen code is not tested. This PR supplements this part of the test.
## How was this patch tested?
add new unit tested.
Closes#22755 from heary-cao/AddTestToBroadcastHashJoinExec.
Authored-by: caoxuewen <cao.xuewen@zte.com.cn>
Signed-off-by: Wenchen Fan <wenchen@databricks.com>
## What changes were proposed in this pull request?
This PR proposes to avoid hardcorded configuration keys in SQLConf's `doc.
## How was this patch tested?
Manually verified.
Closes#22877 from HyukjinKwon/minor-conf-name.
Authored-by: hyukjinkwon <gurwls223@apache.org>
Signed-off-by: hyukjinkwon <gurwls223@apache.org>
## What changes were proposed in this pull request?
These two classes were added for regr_ expression support (SPARK-23907). These have been removed and hence we can remove these base classes and inline the logic in the concrete classes.
## How was this patch tested?
Existing tests.
Closes#22856 from dilipbiswal/average_cleanup.
Authored-by: Dilip Biswal <dbiswal@us.ibm.com>
Signed-off-by: Sean Owen <sean.owen@databricks.com>
## What changes were proposed in this pull request?
Code generation is incorrect if `outputVars` parameter of `consume` method in `CodegenSupport` contains a lazily evaluated stream of expressions.
This PR fixes the issue by forcing the evaluation of `inputVars` before generating the code for UnsafeRow.
## How was this patch tested?
Tested with the sample program provided in https://issues.apache.org/jira/browse/SPARK-25767Closes#22789 from peter-toth/SPARK-25767.
Authored-by: Peter Toth <peter.toth@gmail.com>
Signed-off-by: Herman van Hovell <hvanhovell@databricks.com>
## What changes were proposed in this pull request?
Set main args correctly in BenchmarkBase, to make it accessible for its subclass.
It will benefit:
- BuiltInDataSourceWriteBenchmark
- AvroWriteBenchmark
## How was this patch tested?
manual tests
Closes#22872 from yucai/main_args.
Authored-by: yucai <yyu1@ebay.com>
Signed-off-by: Wenchen Fan <wenchen@databricks.com>
## What changes were proposed in this pull request?
Extractors are made of 2 expressions, one of them defines the the value to be extract from (called `child`) and the other defines the way of extraction (called `extraction`). In this term extractors have 2 children so they shouldn't be `UnaryExpression`s.
`ResolveReferences` was changed in this commit: 36b826f5d1 which resulted a regression with nested extractors. An extractor need to define its children as the set of both `child` and `extraction`; and should try to resolve both in `ResolveReferences`.
This PR changes `UnresolvedExtractValue` to a `BinaryExpression`.
## How was this patch tested?
added UT
Closes#22817 from peter-toth/SPARK-25816.
Authored-by: Peter Toth <peter.toth@gmail.com>
Signed-off-by: gatorsmile <gatorsmile@gmail.com>
## What changes were proposed in this pull request?
The instance of `FileSplit` is redundant for `ParquetFileFormat` and `hive\orc\OrcFileFormat` class.
## How was this patch tested?
Existing unit tests in `ParquetQuerySuite.scala` and `HiveOrcQuerySuite.scala`
Closes#22802 from 10110346/FileSplitnotneed.
Authored-by: liuxian <liu.xian3@zte.com.cn>
Signed-off-by: Sean Owen <sean.owen@databricks.com>
## What changes were proposed in this pull request?
WindowSpecDefinition checks start < last, but CalendarIntervalType is not comparable, so it would throw the following exception at runtime:
```
scala.MatchError: CalendarIntervalType (of class org.apache.spark.sql.types.CalendarIntervalType$) at
org.apache.spark.sql.catalyst.util.TypeUtils$.getInterpretedOrdering(TypeUtils.scala:58) at
org.apache.spark.sql.catalyst.expressions.BinaryComparison.ordering$lzycompute(predicates.scala:592) at
org.apache.spark.sql.catalyst.expressions.BinaryComparison.ordering(predicates.scala:592) at
org.apache.spark.sql.catalyst.expressions.GreaterThan.nullSafeEval(predicates.scala:797) at org.apache.spark.sql.catalyst.expressions.BinaryExpression.eval(Expression.scala:496) at org.apache.spark.sql.catalyst.expressions.SpecifiedWindowFrame.isGreaterThan(windowExpressions.scala:245) at
org.apache.spark.sql.catalyst.expressions.SpecifiedWindowFrame.checkInputDataTypes(windowExpressions.scala:216) at
org.apache.spark.sql.catalyst.expressions.Expression.resolved$lzycompute(Expression.scala:171) at
org.apache.spark.sql.catalyst.expressions.Expression.resolved(Expression.scala:171) at
org.apache.spark.sql.catalyst.expressions.Expression$$anonfun$childrenResolved$1.apply(Expression.scala:183) at
org.apache.spark.sql.catalyst.expressions.Expression$$anonfun$childrenResolved$1.apply(Expression.scala:183) at
scala.collection.IndexedSeqOptimized$class.prefixLengthImpl(IndexedSeqOptimized.scala:38) at scala.collection.IndexedSeqOptimized$class.forall(IndexedSeqOptimized.scala:43) at scala.collection.mutable.ArrayBuffer.forall(ArrayBuffer.scala:48) at
org.apache.spark.sql.catalyst.expressions.Expression.childrenResolved(Expression.scala:183) at
org.apache.spark.sql.catalyst.expressions.WindowSpecDefinition.resolved$lzycompute(windowExpressions.scala:48) at
org.apache.spark.sql.catalyst.expressions.WindowSpecDefinition.resolved(windowExpressions.scala:48) at
org.apache.spark.sql.catalyst.expressions.Expression$$anonfun$childrenResolved$1.apply(Expression.scala:183) at
org.apache.spark.sql.catalyst.expressions.Expression$$anonfun$childrenResolved$1.apply(Expression.scala:183) at
scala.collection.LinearSeqOptimized$class.forall(LinearSeqOptimized.scala:83)
```
We fix the issue by only perform the check on boundary expressions that are AtomicType.
## How was this patch tested?
Add new test case in `DataFrameWindowFramesSuite`
Closes#22853 from jiangxb1987/windowBoundary.
Authored-by: Xingbo Jiang <xingbo.jiang@databricks.com>
Signed-off-by: Xingbo Jiang <xingbo.jiang@databricks.com>
## What changes were proposed in this pull request?
After https://github.com/apache/spark/pull/22745 , Dataset encoder supports the combination of java bean and map type. This PR is to fix the Scala side.
The reason why it didn't work before is, `CatalystToExternalMap` tries to get the data type of the input map expression, while it can be unresolved and its data type is known. To fix it, we can follow `UnresolvedMapObjects`, to create a `UnresolvedCatalystToExternalMap`, and only create `CatalystToExternalMap` when the input map expression is resolved and the data type is known.
## How was this patch tested?
enable a old test case
Closes#22812 from cloud-fan/map.
Authored-by: Wenchen Fan <wenchen@databricks.com>
Signed-off-by: Wenchen Fan <wenchen@databricks.com>
## What changes were proposed in this pull request?
Implements Every, Some, Any aggregates in SQL. These new aggregate expressions are analyzed in normal way and rewritten to equivalent existing aggregate expressions in the optimizer.
Every(x) => Min(x) where x is boolean.
Some(x) => Max(x) where x is boolean.
Any is a synonym for Some.
SQL
```
explain extended select every(v) from test_agg group by k;
```
Plan :
```
== Parsed Logical Plan ==
'Aggregate ['k], [unresolvedalias('every('v), None)]
+- 'UnresolvedRelation `test_agg`
== Analyzed Logical Plan ==
every(v): boolean
Aggregate [k#0], [every(v#1) AS every(v)#5]
+- SubqueryAlias `test_agg`
+- Project [k#0, v#1]
+- SubqueryAlias `test_agg`
+- LocalRelation [k#0, v#1]
== Optimized Logical Plan ==
Aggregate [k#0], [min(v#1) AS every(v)#5]
+- LocalRelation [k#0, v#1]
== Physical Plan ==
*(2) HashAggregate(keys=[k#0], functions=[min(v#1)], output=[every(v)#5])
+- Exchange hashpartitioning(k#0, 200)
+- *(1) HashAggregate(keys=[k#0], functions=[partial_min(v#1)], output=[k#0, min#7])
+- LocalTableScan [k#0, v#1]
Time taken: 0.512 seconds, Fetched 1 row(s)
```
## How was this patch tested?
Added tests in SQLQueryTestSuite, DataframeAggregateSuite
Closes#22809 from dilipbiswal/SPARK-19851-specific-rewrite.
Authored-by: Dilip Biswal <dbiswal@us.ibm.com>
Signed-off-by: Wenchen Fan <wenchen@databricks.com>
## What changes were proposed in this pull request?
Change the version number in comment of `HiveUtils.newClientForExecution` from `13` to `1.2.1` .
## How was this patch tested?
N/A
Closes#22850 from laskfla/HiveUtils-Comment.
Authored-by: laskfla <wwlsax11@gmail.com>
Signed-off-by: Sean Owen <sean.owen@databricks.com>
## What changes were proposed in this pull request?
Remove SQLContext methods deprecated in 1.4
## How was this patch tested?
Existing tests.
Closes#22815 from srowen/SPARK-25821.
Authored-by: Sean Owen <sean.owen@databricks.com>
Signed-off-by: Sean Owen <sean.owen@databricks.com>
## What changes were proposed in this pull request?
In https://github.com/apache/spark/pull/21596, Jackson is upgraded to 2.9.6.
There are some deprecated API warnings in SQLListener.
Create a trivial PR to fix them.
```
[warn] SQLListener.scala:92: method uncheckedSimpleType in class TypeFactory is deprecated: see corresponding Javadoc for more information.
[warn] val objectType = typeFactory.uncheckedSimpleType(classOf[Object])
[warn]
[warn] SQLListener.scala:93: method constructSimpleType in class TypeFactory is deprecated: see corresponding Javadoc for more information.
[warn] typeFactory.constructSimpleType(classOf[(_, _)], classOf[(_, _)], Array(objectType, objectType))
[warn]
[warn] SQLListener.scala:97: method uncheckedSimpleType in class TypeFactory is deprecated: see corresponding Javadoc for more information.
[warn] val longType = typeFactory.uncheckedSimpleType(classOf[Long])
[warn]
[warn] SQLListener.scala:98: method constructSimpleType in class TypeFactory is deprecated: see corresponding Javadoc for more information.
[warn] typeFactory.constructSimpleType(classOf[(_, _)], classOf[(_, _)], Array(longType, longType))
```
## How was this patch tested?
Existing unit tests.
Closes#22848 from gengliangwang/fixSQLListenerWarning.
Authored-by: Gengliang Wang <gengliang.wang@databricks.com>
Signed-off-by: Sean Owen <sean.owen@databricks.com>
## What changes were proposed in this pull request?
The main purpose of `schema_of_json` is the usage of combination with `from_json` (to make up the leak of schema inference) which takes its schema only as literal; however, currently `schema_of_json` allows JSON input as non-literal expressions (e.g, column).
This was mistakenly allowed - we don't have to take other usages rather then the main purpose into account for now.
This PR makes a followup to only allow literals for `schema_of_json`'s JSON input. We can allow non literal expressions later when it's needed or there are some usecase for it.
## How was this patch tested?
Unit tests were added.
Closes#22775 from HyukjinKwon/SPARK-25447-followup.
Lead-authored-by: hyukjinkwon <gurwls223@apache.org>
Co-authored-by: Hyukjin Kwon <gurwls223@apache.org>
Signed-off-by: Wenchen Fan <wenchen@databricks.com>
## What changes were proposed in this pull request?
See the detailed information at https://issues.apache.org/jira/browse/SPARK-25841 on why these APIs should be deprecated and redesigned.
This patch also reverts 8acb51f08b which applies to 2.4.
## How was this patch tested?
Only deprecation and doc changes.
Closes#22841 from rxin/SPARK-25842.
Authored-by: Reynold Xin <rxin@databricks.com>
Signed-off-by: Wenchen Fan <wenchen@databricks.com>
## What changes were proposed in this pull request?
There is a race condition when releasing a Python worker. If `ReaderIterator.handleEndOfDataSection` is not running in the task thread, when a task is early terminated (such as `take(N)`), the task completion listener may close the worker but "handleEndOfDataSection" can still put the worker into the worker pool to reuse.
0e07b483d2 is a patch to reproduce this issue.
I also found a user reported this in the mail list: http://mail-archives.apache.org/mod_mbox/spark-user/201610.mbox/%3CCAAUq=H+YLUEpd23nwvq13Ms5hOStkhX3ao4f4zQV6sgO5zM-xAmail.gmail.com%3E
This PR fixes the issue by using `compareAndSet` to make sure we will never return a closed worker to the work pool.
## How was this patch tested?
Jenkins.
Closes#22816 from zsxwing/fix-socket-closed.
Authored-by: Shixiong Zhu <zsxwing@gmail.com>
Signed-off-by: Takuya UESHIN <ueshin@databricks.com>
## What changes were proposed in this pull request?
In https://github.com/apache/spark/pull/22745 we introduced the `GetArrayFromMap` expression. Later on I realized this is duplicated as we already have `MapKeys` and `MapValues`.
This PR removes `GetArrayFromMap`
## How was this patch tested?
existing tests
Closes#22825 from cloud-fan/minor.
Authored-by: Wenchen Fan <wenchen@databricks.com>
Signed-off-by: Wenchen Fan <wenchen@databricks.com>
## What changes were proposed in this pull request?
Refactor ObjectHashAggregateExecBenchmark to use main method
## How was this patch tested?
Manually tested:
```
bin/spark-submit --class org.apache.spark.sql.execution.benchmark.ObjectHashAggregateExecBenchmark --jars sql/catalyst/target/spark-catalyst_2.11-3.0.0-SNAPSHOT-tests.jar,core/target/spark-core_2.11-3.0.0-SNAPSHOT-tests.jar,sql/hive/target/spark-hive_2.11-3.0.0-SNAPSHOT.jar --packages org.spark-project.hive:hive-exec:1.2.1.spark2 sql/hive/target/spark-hive_2.11-3.0.0-SNAPSHOT-tests.jar
```
Generated results with:
```
SPARK_GENERATE_BENCHMARK_FILES=1 build/sbt "hive/test:runMain org.apache.spark.sql.execution.benchmark.ObjectHashAggregateExecBenchmark"
```
Closes#22804 from peter-toth/SPARK-25665.
Lead-authored-by: Peter Toth <peter.toth@gmail.com>
Co-authored-by: Dongjoon Hyun <dongjoon@apache.org>
Signed-off-by: Dongjoon Hyun <dongjoon@apache.org>
## What changes were proposed in this pull request?
This is inspired during implementing #21732. For now `ScalaReflection` needs to consider how `ExpressionEncoder` uses generated serializers and deserializers. And `ExpressionEncoder` has a weird `flat` flag. After discussion with cloud-fan, it seems to be better to refactor `ExpressionEncoder`. It should make SPARK-24762 easier to do.
To summarize the proposed changes:
1. `serializerFor` and `deserializerFor` return expressions for serializing/deserializing an input expression for a given type. They are private and should not be called directly.
2. `serializerForType` and `deserializerForType` returns an expression for serializing/deserializing for an object of type T to/from Spark SQL representation. It assumes the input object/Spark SQL representation is located at ordinal 0 of a row.
So in other words, `serializerForType` and `deserializerForType` return expressions for atomically serializing/deserializing JVM object to/from Spark SQL value.
A serializer returned by `serializerForType` will serialize an object at `row(0)` to a corresponding Spark SQL representation, e.g. primitive type, array, map, struct.
A deserializer returned by `deserializerForType` will deserialize an input field at `row(0)` to an object with given type.
3. The construction of `ExpressionEncoder` takes a pair of serializer and deserializer for type `T`. It uses them to create serializer and deserializer for T <-> row serialization. Now `ExpressionEncoder` dones't need to remember if serializer is flat or not. When we need to construct new `ExpressionEncoder` based on existing ones, we only need to change input location in the atomic serializer and deserializer.
## How was this patch tested?
Existing tests.
Closes#22749 from viirya/SPARK-24762-refactor.
Authored-by: Liang-Chi Hsieh <viirya@gmail.com>
Signed-off-by: Wenchen Fan <wenchen@databricks.com>
## What changes were proposed in this pull request?
Check the `spark.sql.repl.eagerEval.enabled` configuration property in SparkDataFrame `show()` method. If the `SparkSession` has eager execution enabled, the data will be returned to the R client when the data frame is created. So instead of seeing this
```
> df <- createDataFrame(faithful)
> df
SparkDataFrame[eruptions:double, waiting:double]
```
you will see
```
> df <- createDataFrame(faithful)
> df
+---------+-------+
|eruptions|waiting|
+---------+-------+
| 3.6| 79.0|
| 1.8| 54.0|
| 3.333| 74.0|
| 2.283| 62.0|
| 4.533| 85.0|
| 2.883| 55.0|
| 4.7| 88.0|
| 3.6| 85.0|
| 1.95| 51.0|
| 4.35| 85.0|
| 1.833| 54.0|
| 3.917| 84.0|
| 4.2| 78.0|
| 1.75| 47.0|
| 4.7| 83.0|
| 2.167| 52.0|
| 1.75| 62.0|
| 4.8| 84.0|
| 1.6| 52.0|
| 4.25| 79.0|
+---------+-------+
only showing top 20 rows
```
## How was this patch tested?
Manual tests as well as unit tests (one new test case is added).
Author: adrian555 <v2ave10p>
Closes#22455 from adrian555/eager_execution.
## What changes were proposed in this pull request?
In the PR, I propose to switch `from_json` on `FailureSafeParser`, and to make the function compatible to `PERMISSIVE` mode by default, and to support the `FAILFAST` mode as well. The `DROPMALFORMED` mode is not supported by `from_json`.
## How was this patch tested?
It was tested by existing `JsonSuite`/`CSVSuite`, `JsonFunctionsSuite` and `JsonExpressionsSuite` as well as new tests for `from_json` which checks different modes.
Closes#22237 from MaxGekk/from_json-failuresafe.
Lead-authored-by: Maxim Gekk <maxim.gekk@databricks.com>
Co-authored-by: hyukjinkwon <gurwls223@apache.org>
Signed-off-by: Wenchen Fan <wenchen@databricks.com>
This is a follow-up PR for #22708. It considers another case of java beans deserialization: java maps with struct keys/values.
When deserializing values of MapType with struct keys/values in java beans, fields of structs get mixed up. I suggest using struct data types retrieved from resolved input data instead of inferring them from java beans.
## What changes were proposed in this pull request?
Invocations of "keyArray" and "valueArray" functions are used to extract arrays of keys and values. Struct type of keys or values is also inferred from java bean structure and ends up with mixed up field order.
I created a new UnresolvedInvoke expression as a temporary substitution of Invoke expression while no actual data is available. It allows to provide the resulting data type during analysis based on the resolved input data, not on the java bean (similar to UnresolvedMapObjects).
Key and value arrays are then fed to MapObjects expression which I replaced with UnresolvedMapObjects, just like in case of ArrayType.
Finally I added resolution of UnresolvedInvoke expressions in Analyzer.resolveExpression method as an additional pattern matching case.
## How was this patch tested?
Added a test case.
Built complete project on travis.
viirya kiszk cloud-fan michalsenkyr marmbrus liancheng
Closes#22745 from vofque/SPARK-21402-FOLLOWUP.
Lead-authored-by: Vladimir Kuriatkov <vofque@gmail.com>
Co-authored-by: Vladimir Kuriatkov <Vladimir_Kuriatkov@epam.com>
Signed-off-by: Wenchen Fan <wenchen@databricks.com>
## What changes were proposed in this pull request?
The serializers of `RowEncoder` use few `If` Catalyst expression which inherits `ComplexTypeMergingExpression` that will check input data types.
It is possible to generate serializers which fail the check and can't to access the data type of serializers. When producing If expression, we should use the same data type at its input expressions.
## How was this patch tested?
Added test.
Closes#22785 from viirya/SPARK-25791.
Authored-by: Liang-Chi Hsieh <viirya@gmail.com>
Signed-off-by: Wenchen Fan <wenchen@databricks.com>
The original test would sometimes fail if the listener bus did not keep
up, so just wait till the listener bus is empty. Tested by adding a
sleep in the listener, which made the test consistently fail without the
fix, but pass consistently after the fix.
Closes#22799 from squito/SPARK-25805.
Authored-by: Imran Rashid <irashid@cloudera.com>
Signed-off-by: Wenchen Fan <wenchen@databricks.com>
## What changes were proposed in this pull request?
This takes over original PR at #22019. The original proposal is to have null for float and double types. Later a more reasonable proposal is to disallow empty strings. This patch adds logic to throw exception when finding empty strings for non string types.
## How was this patch tested?
Added test.
Closes#22787 from viirya/SPARK-25040.
Authored-by: Liang-Chi Hsieh <viirya@gmail.com>
Signed-off-by: hyukjinkwon <gurwls223@apache.org>
## What changes were proposed in this pull request?
This goes to reduce test time for ContinuousStressSuite - from 8 mins 13 sec to 43 seconds.
The approach taken by this is to reduce the triggers and epochs to wait and to reduce the expected rows accordingly.
## How was this patch tested?
Existing tests.
Closes#22662 from viirya/SPARK-25627.
Authored-by: Liang-Chi Hsieh <viirya@gmail.com>
Signed-off-by: Sean Owen <sean.owen@databricks.com>
Currently, there are some tests testing function descriptions:
```bash
$ grep -ir "describe function" sql/core/src/test/resources/sql-tests/inputs
sql/core/src/test/resources/sql-tests/inputs/json-functions.sql:describe function to_json;
sql/core/src/test/resources/sql-tests/inputs/json-functions.sql:describe function extended to_json;
sql/core/src/test/resources/sql-tests/inputs/json-functions.sql:describe function from_json;
sql/core/src/test/resources/sql-tests/inputs/json-functions.sql:describe function extended from_json;
```
Looks there are not quite good points about testing them since we're not going to test documentation itself.
For `DESCRIBE FCUNTION` functionality itself, they are already being tested here and there.
See the test failures in https://github.com/apache/spark/pull/18749 (where I added examples to function descriptions)
We better remove those tests so that people don't add such tests in the SQL tests.
## How was this patch tested?
Manual.
Closes#22776 from HyukjinKwon/SPARK-25779.
Authored-by: hyukjinkwon <gurwls223@apache.org>
Signed-off-by: Dongjoon Hyun <dongjoon@apache.org>
## What changes were proposed in this pull request?
`needsUnsafeRowConversion` is used in 2 places:
1. `ColumnarBatchScan.produceRows`
2. `FileSourceScanExec.doExecute`
When we hit `ColumnarBatchScan.produceRows`, it means whole stage codegen is on but the vectorized reader is off. The vectorized reader can be off for several reasons:
1. the file format doesn't have a vectorized reader(json, csv, etc.)
2. the vectorized reader config is off
3. the schema is not supported
Anyway when the vectorized reader is off, file format reader will always return unsafe rows, and other `ColumnarBatchScan` implementations also always return unsafe rows, so `ColumnarBatchScan.needsUnsafeRowConversion` is not needed.
When we hit `FileSourceScanExec.doExecute`, it means whole stage codegen is off. For this case, we need the `needsUnsafeRowConversion` to convert `ColumnarRow` to `UnsafeRow`, if the file format reader returns batch.
This PR removes `ColumnarBatchScan.needsUnsafeRowConversion`, and keep this flag only in `FileSourceScanExec`
## How was this patch tested?
existing tests
Closes#22750 from cloud-fan/minor.
Authored-by: Wenchen Fan <wenchen@databricks.com>
Signed-off-by: Dongjoon Hyun <dongjoon@apache.org>
## What changes were proposed in this pull request?
Refactor `WideSchemaBenchmark` to use main method.
1. use `spark-submit`:
```console
bin/spark-submit --class org.apache.spark.sql.execution.benchmark.WideSchemaBenchmark --jars ./core/target/spark-core_2.11-3.0.0-SNAPSHOT-tests.jar ./sql/core/target/spark-sql_2.11-3.0.0-SNAPSHOT-tests.jar
```
2. Generate benchmark result:
```console
SPARK_GENERATE_BENCHMARK_FILES=1 build/sbt "sql/test:runMain org.apache.spark.sql.execution.benchmark.WideSchemaBenchmark"
```
## How was this patch tested?
manual tests
Closes#22501 from wangyum/SPARK-25492.
Lead-authored-by: Yuming Wang <yumwang@ebay.com>
Co-authored-by: Dongjoon Hyun <dongjoon@apache.org>
Signed-off-by: Dongjoon Hyun <dongjoon@apache.org>
## What changes were proposed in this pull request?
This PR adds `prettyNames` for `from_json`, `to_json`, `from_csv`, and `schema_of_json` so that appropriate names are used.
## How was this patch tested?
Unit tests
Closes#22773 from HyukjinKwon/minor-prettyNames.
Authored-by: hyukjinkwon <gurwls223@apache.org>
Signed-off-by: hyukjinkwon <gurwls223@apache.org>
## What changes were proposed in this pull request?
SQL interface support specify `StorageLevel` when cache table. The semantic is:
```sql
CACHE TABLE tableName OPTIONS('storageLevel' 'DISK_ONLY');
```
All supported `StorageLevel` are:
eefdf9f9dd/core/src/main/scala/org/apache/spark/storage/StorageLevel.scala (L172-L183)
## How was this patch tested?
unit tests and manual tests.
manual tests configuration:
```
--executor-memory 15G --executor-cores 5 --num-executors 50
```
Data:
Input Size / Records: 1037.7 GB / 11732805788
Result:
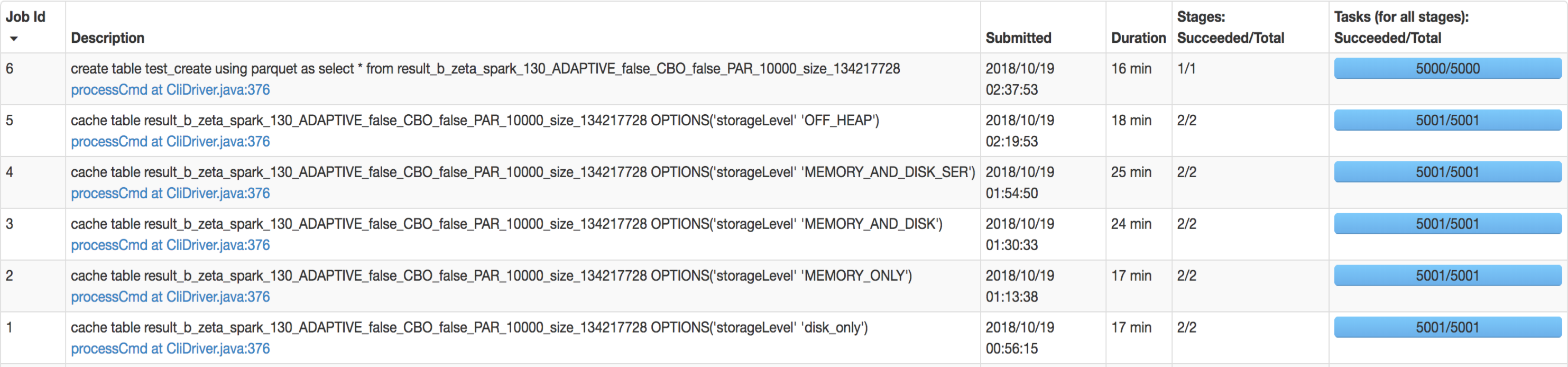
Closes#22263 from wangyum/SPARK-25269.
Authored-by: Yuming Wang <yumwang@ebay.com>
Signed-off-by: Dongjoon Hyun <dongjoon@apache.org>
## What changes were proposed in this pull request?
Without this PR some UDAFs like `GenericUDAFPercentileApprox` can throw an exception because expecting a constant parameter (object inspector) as a particular argument.
The exception is thrown because `toPrettySQL` call in `ResolveAliases` analyzer rule transforms a `Literal` parameter to a `PrettyAttribute` which is then transformed to an `ObjectInspector` instead of a `ConstantObjectInspector`.
The exception comes from `getEvaluator` method of `GenericUDAFPercentileApprox` that actually shouldn't be called during `toPrettySQL` transformation. The reason why it is called are the non lazy fields in `HiveUDAFFunction`.
This PR makes all fields of `HiveUDAFFunction` lazy.
## How was this patch tested?
added new UT
Closes#22766 from peter-toth/SPARK-25768.
Authored-by: Peter Toth <peter.toth@gmail.com>
Signed-off-by: Wenchen Fan <wenchen@databricks.com>
## What changes were proposed in this pull request?
This is a follow-up PR for #22259. The extra field added in `ScalaUDF` with the original PR was declared optional, but should be indeed required, otherwise callers of `ScalaUDF`'s constructor could ignore this new field and cause the result to be incorrect. This PR makes the new field required and changes its name to `handleNullForInputs`.
#22259 breaks the previous behavior for null-handling of primitive-type input parameters. For example, for `val f = udf({(x: Int, y: Any) => x})`, `f(null, "str")` should return `null` but would return `0` after #22259. In this PR, all UDF methods except `def udf(f: AnyRef, dataType: DataType): UserDefinedFunction` have been restored with the original behavior. The only exception is documented in the Spark SQL migration guide.
In addition, now that we have this extra field indicating if a null-test should be applied on the corresponding input value, we can also make use of this flag to avoid the rule `HandleNullInputsForUDF` being applied infinitely.
## How was this patch tested?
Added UT in UDFSuite
Passed affected existing UTs:
AnalysisSuite
UDFSuite
Closes#22732 from maryannxue/spark-25044-followup.
Lead-authored-by: maryannxue <maryannxue@apache.org>
Co-authored-by: Wenchen Fan <wenchen@databricks.com>
Signed-off-by: Wenchen Fan <wenchen@databricks.com>
This allows an implementer of Spark Session Extensions to utilize a
method "injectFunction" which will add a new function to the default
Spark Session Catalogue.
## What changes were proposed in this pull request?
Adds a new function to SparkSessionExtensions
def injectFunction(functionDescription: FunctionDescription)
Where function description is a new type
type FunctionDescription = (FunctionIdentifier, FunctionBuilder)
The functions are loaded in BaseSessionBuilder when the function registry does not have a parent
function registry to get loaded from.
## How was this patch tested?
New unit tests are added for the extension in SparkSessionExtensionSuite
Closes#22576 from RussellSpitzer/SPARK-25560.
Authored-by: Russell Spitzer <Russell.Spitzer@gmail.com>
Signed-off-by: Herman van Hovell <hvanhovell@databricks.com>
## What changes were proposed in this pull request?
CSVs with windows style crlf ('\r\n') don't work in multiline mode. They work fine in single line mode because the line separation is done by Hadoop, which can handle all the different types of line separators. This PR fixes it by enabling Univocity's line separator detection in multiline mode, which will detect '\r\n', '\r', or '\n' automatically as it is done by hadoop in single line mode.
## How was this patch tested?
Unit test with a file with crlf line endings.
Closes#22503 from justinuang/fix-clrf-multiline.
Authored-by: Justin Uang <juang@palantir.com>
Signed-off-by: hyukjinkwon <gurwls223@apache.org>
## What changes were proposed in this pull request?
Only `AddJarCommand` return `0`, the user will be confused about what it means. This PR sets it to empty.
```sql
spark-sql> add jar /Users/yumwang/spark/sql/hive/src/test/resources/TestUDTF.jar;
ADD JAR /Users/yumwang/spark/sql/hive/src/test/resources/TestUDTF.jar
0
spark-sql>
```
## How was this patch tested?
manual tests
```sql
spark-sql> add jar /Users/yumwang/spark/sql/hive/src/test/resources/TestUDTF.jar;
ADD JAR /Users/yumwang/spark/sql/hive/src/test/resources/TestUDTF.jar
spark-sql>
```
Closes#22747 from wangyum/AddJarCommand.
Authored-by: Yuming Wang <yumwang@ebay.com>
Signed-off-by: Dongjoon Hyun <dongjoon@apache.org>
Master
## What changes were proposed in this pull request?
Previously Pyspark used the private constructor for SparkSession when
building that object. This resulted in a SparkSession without checking
the sql.extensions parameter for additional session extensions. To fix
this we instead use the Session.builder() path as SparkR uses, this
loads the extensions and allows their use in PySpark.
## How was this patch tested?
An integration test was added which mimics the Scala test for the same feature.
Please review http://spark.apache.org/contributing.html before opening a pull request.
Closes#21990 from RussellSpitzer/SPARK-25003-master.
Authored-by: Russell Spitzer <Russell.Spitzer@gmail.com>
Signed-off-by: hyukjinkwon <gurwls223@apache.org>
## What changes were proposed in this pull request?
Currently if we run
```
sh start-thriftserver.sh -h
```
we get
```
...
Thrift server options:
2018-10-15 21:45:39 INFO HiveThriftServer2:54 - Starting SparkContext
2018-10-15 21:45:40 INFO SparkContext:54 - Running Spark version 2.3.2
2018-10-15 21:45:40 WARN NativeCodeLoader:62 - Unable to load native-hadoop library for your platform... using builtin-java classes where applicable
2018-10-15 21:45:40 ERROR SparkContext:91 - Error initializing SparkContext.
org.apache.spark.SparkException: A master URL must be set in your configuration
at org.apache.spark.SparkContext.<init>(SparkContext.scala:367)
at org.apache.spark.SparkContext$.getOrCreate(SparkContext.scala:2493)
at org.apache.spark.sql.SparkSession$Builder$$anonfun$7.apply(SparkSession.scala:934)
at org.apache.spark.sql.SparkSession$Builder$$anonfun$7.apply(SparkSession.scala:925)
at scala.Option.getOrElse(Option.scala:121)
at org.apache.spark.sql.SparkSession$Builder.getOrCreate(SparkSession.scala:925)
at org.apache.spark.sql.hive.thriftserver.SparkSQLEnv$.init(SparkSQLEnv.scala:48)
at org.apache.spark.sql.hive.thriftserver.HiveThriftServer2$.main(HiveThriftServer2.scala:79)
at org.apache.spark.sql.hive.thriftserver.HiveThriftServer2.main(HiveThriftServer2.scala)
2018-10-15 21:45:40 ERROR Utils:91 - Uncaught exception in thread main
```
After fix, the usage output is clean:
```
...
Thrift server options:
--hiveconf <property=value> Use value for given property
```
Also exit with code 1, to follow other scripts(this is the behavior of parsing option `-h` for other linux commands as well).
## How was this patch tested?
Manual test.
Closes#22727 from gengliangwang/stsUsage.
Authored-by: Gengliang Wang <gengliang.wang@databricks.com>
Signed-off-by: Sean Owen <sean.owen@databricks.com>
When deserializing values of ArrayType with struct elements in java beans, fields of structs get mixed up.
I suggest using struct data types retrieved from resolved input data instead of inferring them from java beans.
## What changes were proposed in this pull request?
MapObjects expression is used to map array elements to java beans. Struct type of elements is inferred from java bean structure and ends up with mixed up field order.
I used UnresolvedMapObjects instead of MapObjects, which allows to provide element type for MapObjects during analysis based on the resolved input data, not on the java bean.
## How was this patch tested?
Added a test case.
Built complete project on travis.
michalsenkyr cloud-fan marmbrus liancheng
Closes#22708 from vofque/SPARK-21402.
Lead-authored-by: Vladimir Kuriatkov <vofque@gmail.com>
Co-authored-by: Vladimir Kuriatkov <Vladimir_Kuriatkov@epam.com>
Signed-off-by: Wenchen Fan <wenchen@databricks.com>
## What changes were proposed in this pull request?
The SQL execution listener framework was created from scratch(see https://github.com/apache/spark/pull/9078). It didn't leverage what we already have in the spark listener framework, and one major problem is, the listener runs on the spark execution thread, which means a bad listener can block spark's query processing.
This PR re-implements the SQL execution listener framework. Now `ExecutionListenerManager` is just a normal spark listener, which watches the `SparkListenerSQLExecutionEnd` events and post events to the
user-provided SQL execution listeners.
## How was this patch tested?
existing tests.
Closes#22674 from cloud-fan/listener.
Authored-by: Wenchen Fan <wenchen@databricks.com>
Signed-off-by: Wenchen Fan <wenchen@databricks.com>
## What changes were proposed in this pull request?
this PR correct some comment error:
1. change from "as low a possible" to "as low as possible" in RewriteDistinctAggregates.scala
2. delete redundant word “with” in HiveTableScanExec’s doExecute() method
## How was this patch tested?
Existing unit tests.
Closes#22694 from CarolinePeng/update_comment.
Authored-by: 彭灿00244106 <00244106@zte.intra>
Signed-off-by: hyukjinkwon <gurwls223@apache.org>
## What changes were proposed in this pull request?
`Literal.value` should have a value a value corresponding to `dataType`. This pr added code to verify it and fixed the existing tests to do so.
## How was this patch tested?
Modified the existing tests.
Closes#22724 from maropu/SPARK-25734.
Authored-by: Takeshi Yamamuro <yamamuro@apache.org>
Signed-off-by: Wenchen Fan <wenchen@databricks.com>
## What changes were proposed in this pull request?
The PR adds new function `from_csv()` similar to `from_json()` to parse columns with CSV strings. I added the following methods:
```Scala
def from_csv(e: Column, schema: StructType, options: Map[String, String]): Column
```
and this signature to call it from Python, R and Java:
```Scala
def from_csv(e: Column, schema: String, options: java.util.Map[String, String]): Column
```
## How was this patch tested?
Added new test suites `CsvExpressionsSuite`, `CsvFunctionsSuite` and sql tests.
Closes#22379 from MaxGekk/from_csv.
Lead-authored-by: Maxim Gekk <maxim.gekk@databricks.com>
Co-authored-by: Maxim Gekk <max.gekk@gmail.com>
Co-authored-by: Hyukjin Kwon <gurwls223@gmail.com>
Co-authored-by: hyukjinkwon <gurwls223@apache.org>
Signed-off-by: hyukjinkwon <gurwls223@apache.org>
## What changes were proposed in this pull request?
AFAIK multi-column count is not widely supported by the mainstream databases(postgres doesn't support), and the SQL standard doesn't define it clearly, as near as I can tell.
Since Spark supports it, we should clearly document the current behavior and add tests to verify it.
## How was this patch tested?
N/A
Closes#22728 from cloud-fan/doc.
Authored-by: Wenchen Fan <wenchen@databricks.com>
Signed-off-by: hyukjinkwon <gurwls223@apache.org>
## What changes were proposed in this pull request?
Only test these 4 cases is enough:
be2238fb50/sql/core/src/main/scala/org/apache/spark/sql/execution/datasources/parquet/ParquetWriteSupport.scala (L269-L279)
## How was this patch tested?
Manual tests on my local machine.
before:
```
- filter pushdown - decimal (13 seconds, 683 milliseconds)
```
after:
```
- filter pushdown - decimal (9 seconds, 713 milliseconds)
```
Closes#22636 from wangyum/SPARK-25629.
Authored-by: Yuming Wang <yumwang@ebay.com>
Signed-off-by: hyukjinkwon <gurwls223@apache.org>
## What changes were proposed in this pull request?
LOAD DATA INPATH didn't work if the defaultFS included a port for hdfs.
Handling this just requires a small change to use the correct URI
constructor.
## How was this patch tested?
Added a unit test, ran all tests via jenkins
Closes#22733 from squito/SPARK-25738.
Authored-by: Imran Rashid <irashid@cloudera.com>
Signed-off-by: Marcelo Vanzin <vanzin@cloudera.com>
## What changes were proposed in this pull request?
This PR is a follow-up of https://github.com/apache/spark/pull/22594 . This alternative can avoid the unneeded computation in the hot code path.
- For row-based scan, we keep the original way.
- For the columnar scan, we just need to update the stats after each batch.
## How was this patch tested?
N/A
Closes#22731 from gatorsmile/udpateStatsFileScanRDD.
Authored-by: gatorsmile <gatorsmile@gmail.com>
Signed-off-by: Wenchen Fan <wenchen@databricks.com>
## What changes were proposed in this pull request?
Project logical operator generates valid constraints using two opposite operations. It substracts child constraints from all constraints, than union child constraints again. I think it may be not necessary.
Aggregate operator has the same problem with Project.
This PR try to remove these two opposite collection operations.
## How was this patch tested?
Related unit tests:
ProjectEstimationSuite
CollapseProjectSuite
PushProjectThroughUnionSuite
UnsafeProjectionBenchmark
GeneratedProjectionSuite
CodeGeneratorWithInterpretedFallbackSuite
TakeOrderedAndProjectSuite
GenerateUnsafeProjectionSuite
BucketedRandomProjectionLSHSuite
RemoveRedundantAliasAndProjectSuite
AggregateBenchmark
AggregateOptimizeSuite
AggregateEstimationSuite
DecimalAggregatesSuite
DateFrameAggregateSuite
ObjectHashAggregateSuite
TwoLevelAggregateHashMapSuite
ObjectHashAggregateExecBenchmark
SingleLevelAggregateHaspMapSuite
TypedImperativeAggregateSuite
RewriteDistinctAggregatesSuite
HashAggregationQuerySuite
HashAggregationQueryWithControlledFallbackSuite
TypedImperativeAggregateSuite
TwoLevelAggregateHashMapWithVectorizedMapSuite
Closes#22706 from SongYadong/generate_constraints.
Authored-by: SongYadong <song.yadong1@zte.com.cn>
Signed-off-by: gatorsmile <gatorsmile@gmail.com>
## What changes were proposed in this pull request?
The PR addresses [the comment](https://github.com/apache/spark/pull/22715#discussion_r225024084) in the previous one. `outputOrdering` becomes a field of `InMemoryRelation`.
## How was this patch tested?
existing UTs
Closes#22726 from mgaido91/SPARK-25727_followup.
Authored-by: Marco Gaido <marcogaido91@gmail.com>
Signed-off-by: gatorsmile <gatorsmile@gmail.com>
## What changes were proposed in this pull request?
Add `outputOrdering ` to `otherCopyArgs` in InMemoryRelation so that this field will be copied when we doing the tree transformation.
```
val data = Seq(100).toDF("count").cache()
data.queryExecution.optimizedPlan.toJSON
```
The above code can generate the following error:
```
assertion failed: InMemoryRelation fields: output, cacheBuilder, statsOfPlanToCache, outputOrdering, values: List(count#178), CachedRDDBuilder(true,10000,StorageLevel(disk, memory, deserialized, 1 replicas),*(1) Project [value#176 AS count#178]
+- LocalTableScan [value#176]
,None), Statistics(sizeInBytes=12.0 B, hints=none)
java.lang.AssertionError: assertion failed: InMemoryRelation fields: output, cacheBuilder, statsOfPlanToCache, outputOrdering, values: List(count#178), CachedRDDBuilder(true,10000,StorageLevel(disk, memory, deserialized, 1 replicas),*(1) Project [value#176 AS count#178]
+- LocalTableScan [value#176]
,None), Statistics(sizeInBytes=12.0 B, hints=none)
at scala.Predef$.assert(Predef.scala:170)
at org.apache.spark.sql.catalyst.trees.TreeNode.jsonFields(TreeNode.scala:611)
at org.apache.spark.sql.catalyst.trees.TreeNode.org$apache$spark$sql$catalyst$trees$TreeNode$$collectJsonValue$1(TreeNode.scala:599)
at org.apache.spark.sql.catalyst.trees.TreeNode.jsonValue(TreeNode.scala:604)
at org.apache.spark.sql.catalyst.trees.TreeNode.toJSON(TreeNode.scala:590)
```
## How was this patch tested?
Added a test
Closes#22715 from gatorsmile/copyArgs1.
Authored-by: gatorsmile <gatorsmile@gmail.com>
Signed-off-by: Dongjoon Hyun <dongjoon@apache.org>
## What changes were proposed in this pull request?
[SPARK-22479](https://github.com/apache/spark/pull/19708/files#diff-5c22ac5160d3c9d81225c5dd86265d27R31) adds a test case which sometimes fails because the used password string `123` matches `41230802`. This PR aims to fix the flakiness.
- https://amplab.cs.berkeley.edu/jenkins/job/SparkPullRequestBuilder/97343/consoleFull
```scala
SaveIntoDataSourceCommandSuite:
- simpleString is redacted *** FAILED ***
"SaveIntoDataSourceCommand .org.apache.spark.sql.execution.datasources.jdbc.JdbcRelationProvider41230802, Map(password -> *********(redacted), url -> *********(redacted), driver -> mydriver), ErrorIfExists
+- Range (0, 1, step=1, splits=Some(2))
" contained "123" (SaveIntoDataSourceCommandSuite.scala:42)
```
## How was this patch tested?
Pass the Jenkins with the updated test case
Closes#22716 from dongjoon-hyun/SPARK-25726.
Authored-by: Dongjoon Hyun <dongjoon@apache.org>
Signed-off-by: Dongjoon Hyun <dongjoon@apache.org>
## What changes were proposed in this pull request?
improve the code comment added in https://github.com/apache/spark/pull/22702/files
## How was this patch tested?
N/A
Closes#22711 from cloud-fan/minor.
Authored-by: Wenchen Fan <wenchen@databricks.com>
Signed-off-by: gatorsmile <gatorsmile@gmail.com>
## What changes were proposed in this pull request?
Currently `Range` reports metrics in batch granularity. This is acceptable, but it's better if we can make it row granularity without performance penalty.
Before this PR, the metrics are updated when preparing the batch, which is before we actually consume data. In this PR, the metrics are updated after the data are consumed. There are 2 different cases:
1. The data processing loop has a stop check. The metrics are updated when we need to stop.
2. no stop check. The metrics are updated after the loop.
## How was this patch tested?
existing tests and a new benchmark
Closes#22698 from cloud-fan/range.
Authored-by: Wenchen Fan <wenchen@databricks.com>
Signed-off-by: Wenchen Fan <wenchen@databricks.com>
## What changes were proposed in this pull request?
```Scala
val df1 = Seq(("abc", 1), (null, 3)).toDF("col1", "col2")
df1.write.mode(SaveMode.Overwrite).parquet("/tmp/test1")
val df2 = spark.read.parquet("/tmp/test1")
df2.filter("col1 = 'abc' OR (col1 != 'abc' AND col2 == 3)").show()
```
Before the PR, it returns both rows. After the fix, it returns `Row ("abc", 1))`. This is to fix the bug in NULL handling in BooleanSimplification. This is a bug introduced in Spark 1.6 release.
## How was this patch tested?
Added test cases
Closes#22702 from gatorsmile/fixBooleanSimplify2.
Authored-by: gatorsmile <gatorsmile@gmail.com>
Signed-off-by: gatorsmile <gatorsmile@gmail.com>
## What changes were proposed in this pull request?
Refactor `JoinBenchmark` to use main method.
1. use `spark-submit`:
```console
bin/spark-submit --class org.apache.spark.sql.execution.benchmark.JoinBenchmark --jars ./core/target/spark-core_2.11-3.0.0-SNAPSHOT-tests.jar ./sql/catalyst/target/spark-sql_2.11-3.0.0-SNAPSHOT-tests.jar
```
2. Generate benchmark result:
```console
SPARK_GENERATE_BENCHMARK_FILES=1 build/sbt "sql/test:runMain org.apache.spark.sql.execution.benchmark.JoinBenchmark"
```
## How was this patch tested?
manual tests
Closes#22661 from wangyum/SPARK-25664.
Lead-authored-by: Yuming Wang <yumwang@ebay.com>
Co-authored-by: Yuming Wang <wgyumg@gmail.com>
Co-authored-by: Dongjoon Hyun <dongjoon@apache.org>
Signed-off-by: Dongjoon Hyun <dongjoon@apache.org>
## What changes were proposed in this pull request?
The PR addresses the exception raised on accessing chars out of delimiter string. In particular, the backward slash `\` as the CSV fields delimiter causes the following exception on reading `abc\1`:
```Scala
String index out of range: 1
java.lang.StringIndexOutOfBoundsException: String index out of range: 1
at java.lang.String.charAt(String.java:658)
```
because `str.charAt(1)` tries to access a char out of `str` in `CSVUtils.toChar`
## How was this patch tested?
Added tests for empty string and string containing the backward slash to `CSVUtilsSuite`. Besides of that I added an end-to-end test to check how the backward slash is handled in reading CSV string with it.
Closes#22654 from MaxGekk/csv-slash-delim.
Authored-by: Maxim Gekk <maxim.gekk@databricks.com>
Signed-off-by: gatorsmile <gatorsmile@gmail.com>
## What changes were proposed in this pull request?
After the changes, total execution time of `JsonExpressionsSuite.scala` dropped from 12.5 seconds to 3 seconds.
Closes#22657 from MaxGekk/json-timezone-test.
Authored-by: Maxim Gekk <maxim.gekk@databricks.com>
Signed-off-by: Sean Owen <sean.owen@databricks.com>
## What changes were proposed in this pull request?
Currently SQL tab in the WEBUI doesn't support pagination. Because of that following issues are happening.
1) For large number of executions, SQL page is throwing OOM exception (around 40,000)
2) For large number of executions, loading SQL page is taking time.
3) Difficult to analyse the execution table for large number of execution.
[Note: spark.sql.ui.retainedExecutions = 50000]
All the tabs, Jobs, Stages etc. supports pagination. So, to make it consistent with other tabs
SQL tab also should support pagination.
I have followed the similar flow of the pagination code in the Jobs and Stages page for SQL page.
Also, this patch doesn't make any behavior change for the SQL tab except the pagination support.
## How was this patch tested?
bin/spark-shell --conf spark.sql.ui.retainedExecutions=50000
Run 50,000 sql queries.
**Before this PR**
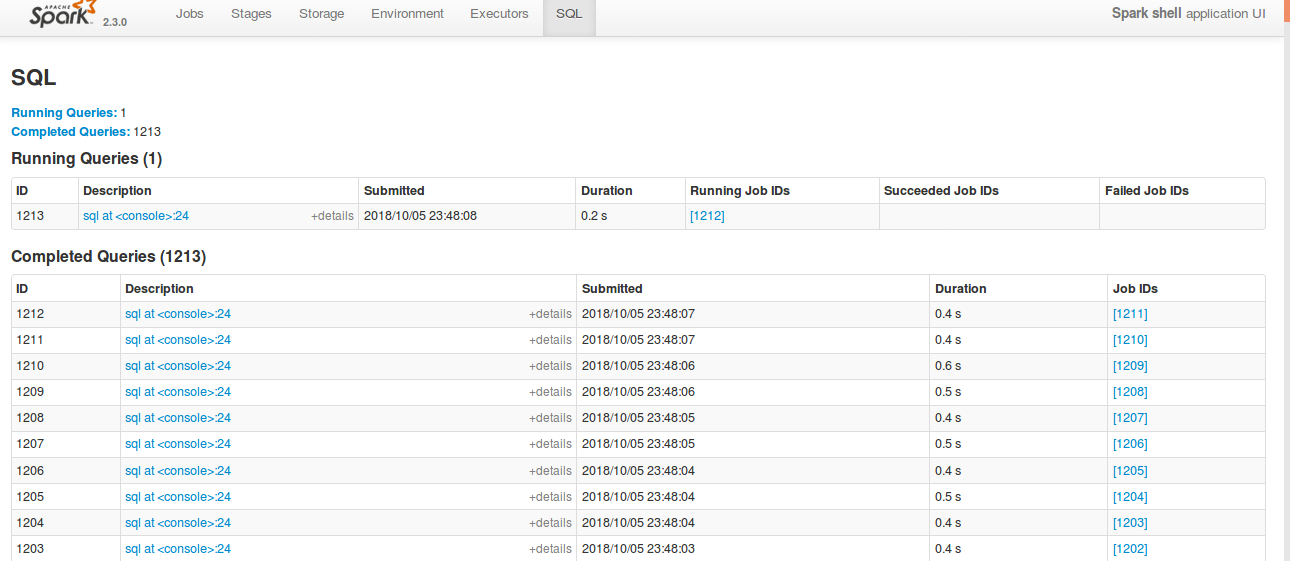
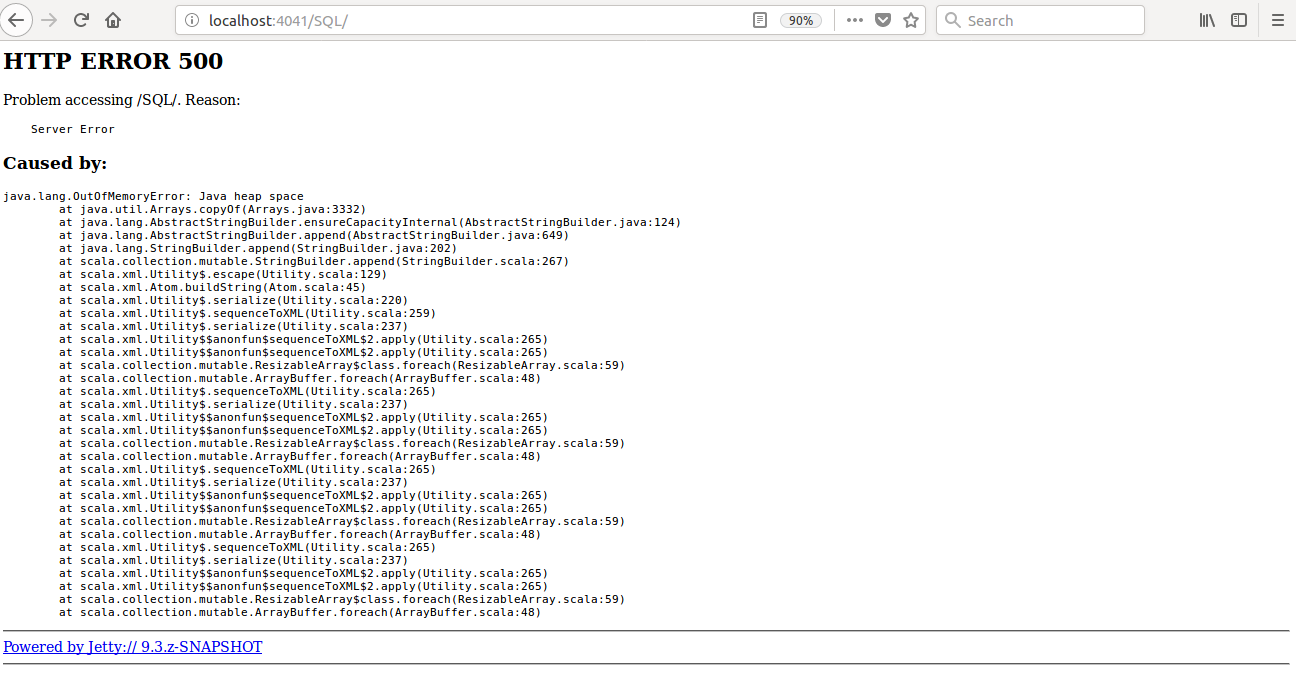
**After this PR**
Loading of the page is faster, and OOM issue doesn't happen.
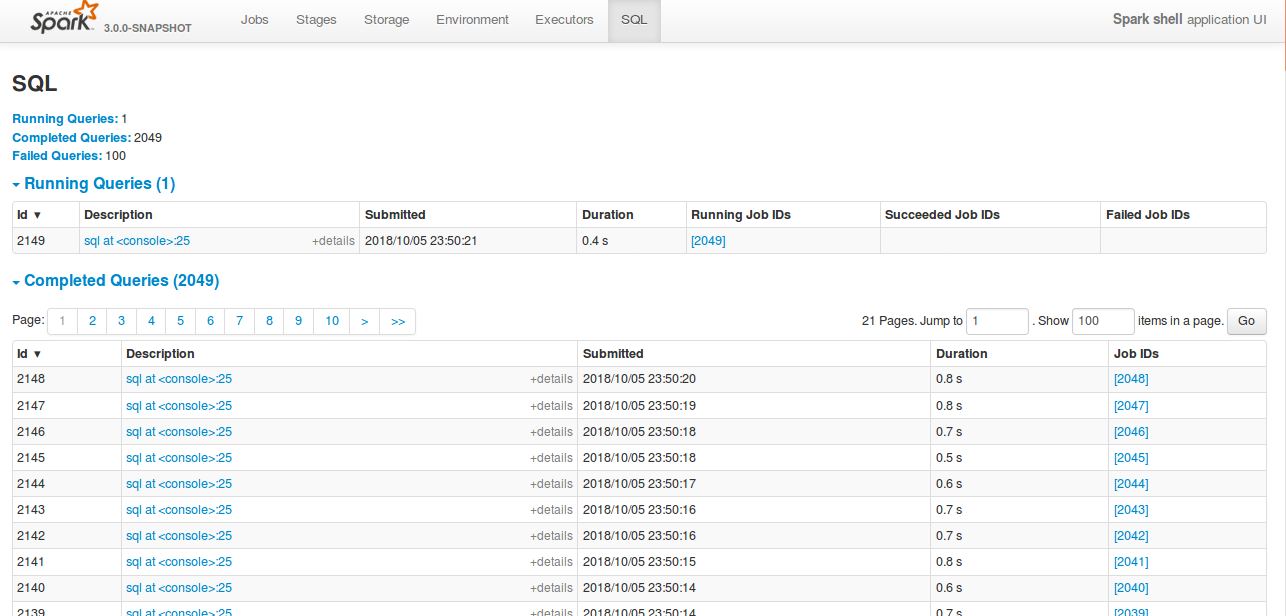
Closes#22645 from shahidki31/SPARK-25566.
Authored-by: Shahid <shahidki31@gmail.com>
Signed-off-by: Sean Owen <sean.owen@databricks.com>
## What changes were proposed in this pull request?
According to the SQL standard, when a query contains `HAVING`, it indicates an aggregate operator. For more details please refer to https://blog.jooq.org/2014/12/04/do-you-really-understand-sqls-group-by-and-having-clauses/
However, in Spark SQL parser, we treat HAVING as a normal filter when there is no GROUP BY, which breaks SQL semantic and lead to wrong result. This PR fixes the parser.
## How was this patch tested?
new test
Closes#22696 from cloud-fan/having.
Authored-by: Wenchen Fan <wenchen@databricks.com>
Signed-off-by: gatorsmile <gatorsmile@gmail.com>
## What changes were proposed in this pull request?
The HandleNullInputsForUDF rule can generate new If node infinitely, thus causing problems like match of SQL cache missed.
This was fixed in SPARK-24891 and was then broken by SPARK-25044.
The unit test in `AnalysisSuite` added in SPARK-24891 should have failed but didn't because it wasn't properly updated after the `ScalaUDF` constructor signature change. So this PR also updates the test accordingly based on the new `ScalaUDF` constructor.
## How was this patch tested?
Updated the original UT. This should be justified as the original UT became invalid after SPARK-25044.
Closes#22701 from maryannxue/spark-25690.
Authored-by: maryannxue <maryannxue@apache.org>
Signed-off-by: gatorsmile <gatorsmile@gmail.com>
## What changes were proposed in this pull request?
This PR can correctly cause assertion failure when incorrect nullable of DataType in the result is generated by a target function to be tested.
Let us think the following example. In the future, a developer would write incorrect code that returns unexpected result. We have to correctly cause fail in this test since `valueContainsNull=false` while `expr` includes `null`. However, without this PR, this test passes. This PR can correctly cause fail.
```
test("test TARGETFUNCTON") {
val expr = TARGETMAPFUNCTON()
// expr = UnsafeMap(3 -> 6, 7 -> null)
// expr.dataType = (IntegerType, IntegerType, false)
expected = Map(3 -> 6, 7 -> null)
checkEvaluation(expr, expected)
```
In [`checkEvaluationWithUnsafeProjection`](https://github.com/apache/spark/blob/master/sql/catalyst/src/test/scala/org/apache/spark/sql/catalyst/expressions/ExpressionEvalHelper.scala#L208-L235), the results are compared using `UnsafeRow`. When the given `expected` is [converted](https://github.com/apache/spark/blob/master/sql/catalyst/src/test/scala/org/apache/spark/sql/catalyst/expressions/ExpressionEvalHelper.scala#L226-L227)) to `UnsafeRow` using the `DataType` of `expr`.
```
val expectedRow = UnsafeProjection.create(Array(expression.dataType, expression.dataType)).apply(lit)
```
In summary, `expr` is `[0,1800000038,5000000038,18,2,0,700000003,2,0,6,18,2,0,700000003,2,0,6]` with and w/o this PR. `expected` is converted to
* w/o this PR, `[0,1800000038,5000000038,18,2,0,700000003,2,0,6,18,2,0,700000003,2,0,6]`
* with this PR, `[0,1800000038,5000000038,18,2,0,700000003,2,2,6,18,2,0,700000003,2,2,6]`
As a result, w/o this PR, the test unexpectedly passes.
This is because, w/o this PR, based on given `dataType`, generated code of projection for `expected` avoids to set nullbit.
```
// tmpInput_2 is expected
/* 155 */ for (int index_1 = 0; index_1 < numElements_1; index_1++) {
/* 156 */ mutableStateArray_1[1].write(index_1, tmpInput_2.getInt(index_1));
/* 157 */ }
```
With this PR, generated code of projection for `expected` always checks whether nullbit should be set by `isNullAt`
```
// tmpInput_2 is expected
/* 161 */ for (int index_1 = 0; index_1 < numElements_1; index_1++) {
/* 162 */
/* 163 */ if (tmpInput_2.isNullAt(index_1)) {
/* 164 */ mutableStateArray_1[1].setNull4Bytes(index_1);
/* 165 */ } else {
/* 166 */ mutableStateArray_1[1].write(index_1, tmpInput_2.getInt(index_1));
/* 167 */ }
/* 168 */
/* 169 */ }
```
## How was this patch tested?
Existing UTs
Closes#22375 from kiszk/SPARK-25388.
Authored-by: Kazuaki Ishizaki <ishizaki@jp.ibm.com>
Signed-off-by: Wenchen Fan <wenchen@databricks.com>
## What changes were proposed in this pull request?
1. Move `CSVDataSource.makeSafeHeader` to `CSVUtils.makeSafeHeader` (as is).
- Historically and at the first place of refactoring (which I did), I intended to put all CSV specific handling (like options), filtering, extracting header, etc.
- See `JsonDataSource`. Now `CSVDataSource` is quite consistent with `JsonDataSource`. Since CSV's code path is quite complicated, we might better match them as possible as we can.
2. Create `CSVHeaderChecker` and put `enforceSchema` logics into that.
- The checking header and column pruning stuff were added (per https://github.com/apache/spark/pull/20894 and https://github.com/apache/spark/pull/21296) but some of codes such as https://github.com/apache/spark/pull/22123 are duplicated
- Also, checking header code is basically here and there. We better put them in a single place, which was quite error-prone. See (https://github.com/apache/spark/pull/22656).
3. Move `CSVDataSource.checkHeaderColumnNames` to `CSVHeaderChecker.checkHeaderColumnNames` (as is).
- Similar reasons above with 1.
## How was this patch tested?
Existing tests should cover this.
Closes#22676 from HyukjinKwon/refactoring-csv.
Authored-by: hyukjinkwon <gurwls223@apache.org>
Signed-off-by: hyukjinkwon <gurwls223@apache.org>
## What changes were proposed in this pull request?
If the records are incremented by more than 1 at a time,the number of bytes might rarely ever get updated,because it might skip over the count that is an exact multiple of UPDATE_INPUT_METRICS_INTERVAL_RECORDS.
This PR just checks whether the increment causes the value to exceed a higher multiple of UPDATE_INPUT_METRICS_INTERVAL_RECORDS.
## How was this patch tested?
existed unit tests
Closes#22594 from 10110346/inputMetrics.
Authored-by: liuxian <liu.xian3@zte.com.cn>
Signed-off-by: Sean Owen <sean.owen@databricks.com>
## What changes were proposed in this pull request?
remove Redundant semicolons in SortMergeJoinExec, thanks.
## How was this patch tested?
N/A
Closes#22695 from heary-cao/RedundantSemicolons.
Authored-by: caoxuewen <cao.xuewen@zte.com.cn>
Signed-off-by: Sean Owen <sean.owen@databricks.com>
## What changes were proposed in this pull request?
1. Refactor DataSourceReadBenchmark
## How was this patch tested?
Manually tested and regenerated results.
```
SPARK_GENERATE_BENCHMARK_FILES=1 build/sbt "sql/test:runMain org.apache.spark.sql.execution.benchmark.DataSourceReadBenchmark"
```
Closes#22664 from peter-toth/SPARK-25662.
Lead-authored-by: Peter Toth <peter.toth@gmail.com>
Co-authored-by: Dongjoon Hyun <dongjoon@apache.org>
Signed-off-by: DB Tsai <d_tsai@apple.com>
## What changes were proposed in this pull request?
Remove Hadoop 2.6 references and make 2.7 the default.
Obviously, this is for master/3.0.0 only.
After this we can also get rid of the separate test jobs for Hadoop 2.6.
## How was this patch tested?
Existing tests
Closes#22615 from srowen/SPARK-25016.
Authored-by: Sean Owen <sean.owen@databricks.com>
Signed-off-by: Sean Owen <sean.owen@databricks.com>
## What changes were proposed in this pull request?
Inspired by https://github.com/apache/spark/pull/22574 .
We can partially push down top level conjunctive predicates to Orc.
This PR improves Orc predicate push down in both SQL and Hive module.
## How was this patch tested?
New unit test.
Closes#22684 from gengliangwang/pushOrcFilters.
Authored-by: Gengliang Wang <gengliang.wang@databricks.com>
Signed-off-by: DB Tsai <d_tsai@apple.com>
## What changes were proposed in this pull request?
Reduced the combination of codecs from 9 to 3 to improve the test runtime.
## How was this patch tested?
This is a test fix.
Closes#22641 from dilipbiswal/SPARK-25611.
Authored-by: Dilip Biswal <dbiswal@us.ibm.com>
Signed-off-by: Sean Owen <sean.owen@databricks.com>
## What changes were proposed in this pull request?
Try testing timezones in parallel instead in CastSuite, instead of random sampling.
See also #22631
## How was this patch tested?
Existing test.
Closes#22672 from srowen/SPARK-25605.2.
Authored-by: Sean Owen <sean.owen@databricks.com>
Signed-off-by: Sean Owen <sean.owen@databricks.com>
## What changes were proposed in this pull request?
This is a follow up of https://github.com/apache/spark/pull/22574. Renamed the parameter and added comments.
## How was this patch tested?
N/A
Closes#22679 from gatorsmile/followupSPARK-25559.
Authored-by: gatorsmile <gatorsmile@gmail.com>
Signed-off-by: DB Tsai <d_tsai@apple.com>
## What changes were proposed in this pull request?
This PR is inspired by https://github.com/apache/spark/pull/22524, but proposes a safer fix.
The current limit whole stage codegen has 2 problems:
1. It's only applied to `InputAdapter`, many leaf nodes can't stop earlier w.r.t. limit.
2. It needs to override a method, which will break if we have more than one limit in the whole-stage.
The first problem is easy to fix, just figure out which nodes can stop earlier w.r.t. limit, and update them. This PR updates `RangeExec`, `ColumnarBatchScan`, `SortExec`, `HashAggregateExec`.
The second problem is hard to fix. This PR proposes to propagate the limit counter variable name upstream, so that the upstream leaf/blocking nodes can check the limit counter and quit the loop earlier.
For better performance, the implementation here follows `CodegenSupport.needStopCheck`, so that we only codegen the check only if there is limit in the query. For columnar node like range, we check the limit counter per-batch instead of per-row, to make the inner loop tight and fast.
Why this is safer?
1. the leaf/blocking nodes don't have to check the limit counter and stop earlier. It's only for performance. (this is same as before)
2. The blocking operators can stop propagating the limit counter name, because the counter of limit after blocking operators will never increase, before blocking operators consume all the data from upstream operators. So the upstream operators don't care about limit after blocking operators. This is also for performance only, it's OK if we forget to do it for some new blocking operators.
## How was this patch tested?
a new test
Closes#22630 from cloud-fan/limit.
Authored-by: Wenchen Fan <wenchen@databricks.com>
Signed-off-by: Kazuaki Ishizaki <ishizaki@jp.ibm.com>
## What changes were proposed in this pull request?
Currently the first row of dataset of CSV strings is compared to field names of user specified or inferred schema independently of presence of CSV header. It causes false-positive error messages. For example, parsing `"1,2"` outputs the error:
```java
java.lang.IllegalArgumentException: CSV header does not conform to the schema.
Header: 1, 2
Schema: _c0, _c1
Expected: _c0 but found: 1
```
In the PR, I propose:
- Checking CSV header only when it exists
- Filter header from the input dataset only if it exists
## How was this patch tested?
Added a test to `CSVSuite` which reproduces the issue.
Closes#22656 from MaxGekk/inferred-header-check.
Authored-by: Maxim Gekk <maxim.gekk@databricks.com>
Signed-off-by: hyukjinkwon <gurwls223@apache.org>
## What changes were proposed in this pull request?
There was 5 suites extends `HadoopFsRelationTest`, for testing "orc"/"parquet"/"text"/"json" data sources.
This PR refactor the base trait `HadoopFsRelationTest`:
1. Rename unnecessary loop for setting parquet conf
2. The test case `SPARK-8406: Avoids name collision while writing files` takes about 14 to 20 seconds. As now all the file format data source are using common code, for creating result files, we can test one data source(Parquet) only to reduce test time.
To run related 5 suites:
```
./build/sbt "hive/testOnly *HadoopFsRelationSuite"
```
The total test run time is reduced from 5 minutes 40 seconds to 3 minutes 50 seconds.
## How was this patch tested?
Unit test
Closes#22643 from gengliangwang/refactorHadoopFsRelationTest.
Authored-by: Gengliang Wang <gengliang.wang@databricks.com>
Signed-off-by: gatorsmile <gatorsmile@gmail.com>
While working on another PR, I noticed that there is quite some legacy Java in there that can be beautified. For example the use of features from Java8, such as:
- Collection libraries
- Try-with-resource blocks
No logic has been changed. I think it is important to have a solid codebase with examples that will inspire next PR's to follow up on the best practices.
What are your thoughts on this?
This makes code easier to read, and using try-with-resource makes is less likely to forget to close something.
## What changes were proposed in this pull request?
No changes in the logic of Spark, but more in the aesthetics of the code.
## How was this patch tested?
Using the existing unit tests. Since no logic is changed, the existing unit tests should pass.
Please review http://spark.apache.org/contributing.html before opening a pull request.
Closes#22637 from Fokko/SPARK-25408.
Authored-by: Fokko Driesprong <fokkodriesprong@godatadriven.com>
Signed-off-by: Sean Owen <sean.owen@databricks.com>
## What changes were proposed in this pull request?
Refactor `HashBenchmark` to use main method.
1. use `spark-submit`:
```console
bin/spark-submit --class org.apache.spark.sql.HashBenchmark --jars ./core/target/spark-core_2.11-3.0.0-SNAPSHOT-tests.jar ./sql/catalyst/target/spark-catalyst_2.11-3.0.0-SNAPSHOT-tests.jar
```
2. Generate benchmark result:
```console
SPARK_GENERATE_BENCHMARK_FILES=1 build/sbt "catalyst/test:runMain org.apache.spark.sql.HashBenchmark"
```
## How was this patch tested?
manual tests
Closes#22651 from wangyum/SPARK-25657.
Lead-authored-by: Yuming Wang <wgyumg@gmail.com>
Co-authored-by: Yuming Wang <yumwang@ebay.com>
Co-authored-by: Dongjoon Hyun <dongjoon@apache.org>
Signed-off-by: Dongjoon Hyun <dongjoon@apache.org>
## What changes were proposed in this pull request?
Refactor `HashByteArrayBenchmark` to use main method.
1. use `spark-submit`:
```console
bin/spark-submit --class org.apache.spark.sql.HashByteArrayBenchmark --jars ./core/target/spark-core_2.11-3.0.0-SNAPSHOT-tests.jar ./sql/catalyst/target/spark-catalyst_2.11-3.0.0-SNAPSHOT-tests.jar
```
2. Generate benchmark result:
```console
SPARK_GENERATE_BENCHMARK_FILES=1 build/sbt "catalyst/test:runMain org.apache.spark.sql.HashByteArrayBenchmark"
```
## How was this patch tested?
manual tests
Closes#22652 from wangyum/SPARK-25658.
Lead-authored-by: Yuming Wang <wgyumg@gmail.com>
Co-authored-by: Yuming Wang <yumwang@ebay.com>
Co-authored-by: Dongjoon Hyun <dongjoon@apache.org>
Signed-off-by: Dongjoon Hyun <dongjoon@apache.org>
## What changes were proposed in this pull request?
`InMemoryFileIndex` contains a cache of `LocatedFileStatus` objects. Each `LocatedFileStatus` object can contain several `BlockLocation`s or some subclass of it. Filling up this cache by listing files happens recursively either on the driver or on the executors, depending on the parallel discovery threshold (`spark.sql.sources.parallelPartitionDiscovery.threshold`). If the listing happens on the executors block location objects are converted to simple `BlockLocation` objects to ensure serialization requirements. If it happens on the driver then there is no conversion and depending on the file system a `BlockLocation` object can be a subclass like `HdfsBlockLocation` and consume more memory. This PR adds the conversion to the latter case and decreases memory consumption.
## How was this patch tested?
Added unit test.
Closes#22603 from peter-toth/SPARK-25062.
Authored-by: Peter Toth <peter.toth@gmail.com>
Signed-off-by: Dongjoon Hyun <dongjoon@apache.org>
## What changes were proposed in this pull request?
This PR fixes the Scala-2.12 build error due to ambiguity in `foreachBatch` test cases.
- https://amplab.cs.berkeley.edu/jenkins/view/Spark%20QA%20Test%20(Dashboard)/job/spark-master-test-maven-hadoop-2.7-ubuntu-scala-2.12/428/console
```scala
[error] /home/jenkins/workspace/spark-master-test-maven-hadoop-2.7-ubuntu-scala-2.12/sql/core/src/test/scala/org/apache/spark/sql/execution/streaming/sources/ForeachBatchSinkSuite.scala:102: ambiguous reference to overloaded definition,
[error] both method foreachBatch in class DataStreamWriter of type (function: org.apache.spark.api.java.function.VoidFunction2[org.apache.spark.sql.Dataset[Int],Long])org.apache.spark.sql.streaming.DataStreamWriter[Int]
[error] and method foreachBatch in class DataStreamWriter of type (function: (org.apache.spark.sql.Dataset[Int], Long) => Unit)org.apache.spark.sql.streaming.DataStreamWriter[Int]
[error] match argument types ((org.apache.spark.sql.Dataset[Int], Any) => Unit)
[error] ds.writeStream.foreachBatch((_, _) => {}).trigger(Trigger.Continuous("1 second")).start()
[error] ^
[error] /home/jenkins/workspace/spark-master-test-maven-hadoop-2.7-ubuntu-scala-2.12/sql/core/src/test/scala/org/apache/spark/sql/execution/streaming/sources/ForeachBatchSinkSuite.scala:106: ambiguous reference to overloaded definition,
[error] both method foreachBatch in class DataStreamWriter of type (function: org.apache.spark.api.java.function.VoidFunction2[org.apache.spark.sql.Dataset[Int],Long])org.apache.spark.sql.streaming.DataStreamWriter[Int]
[error] and method foreachBatch in class DataStreamWriter of type (function: (org.apache.spark.sql.Dataset[Int], Long) => Unit)org.apache.spark.sql.streaming.DataStreamWriter[Int]
[error] match argument types ((org.apache.spark.sql.Dataset[Int], Any) => Unit)
[error] ds.writeStream.foreachBatch((_, _) => {}).partitionBy("value").start()
[error] ^
```
## How was this patch tested?
Manual.
Since this failure occurs in Scala-2.12 profile and test cases, Jenkins will not test this. We need to build with Scala-2.12 and run the tests.
Closes#22649 from dongjoon-hyun/SPARK-SCALA212.
Authored-by: Dongjoon Hyun <dongjoon@apache.org>
Signed-off-by: Dongjoon Hyun <dongjoon@apache.org>
## What changes were proposed in this pull request?
Clean up the joinCriteria parsing in the parser by directly using identifierList
## How was this patch tested?
N/A
Closes#22648 from gatorsmile/cleanupJoinCriteria.
Authored-by: gatorsmile <gatorsmile@gmail.com>
Signed-off-by: gatorsmile <gatorsmile@gmail.com>
## What changes were proposed in this pull request?
Refactor `MiscBenchmark ` to use main method.
Generate benchmark result:
```sh
SPARK_GENERATE_BENCHMARK_FILES=1 build/sbt "sql/test:runMain org.apache.spark.sql.execution.benchmark.MiscBenchmark"
```
## How was this patch tested?
manual tests
Closes#22500 from wangyum/SPARK-25488.
Lead-authored-by: Yuming Wang <yumwang@ebay.com>
Co-authored-by: Yuming Wang <wgyumg@gmail.com>
Co-authored-by: Dongjoon Hyun <dongjoon@apache.org>
Signed-off-by: Dongjoon Hyun <dongjoon@apache.org>
## What changes were proposed in this pull request?
By replacing loops with random possible value.
- `read partitioning bucketed tables with bucket pruning filters` reduce from 55s to 7s
- `read partitioning bucketed tables having composite filters` reduce from 54s to 8s
- total time: reduce from 288s to 192s
## How was this patch tested?
Unit test
Closes#22640 from gengliangwang/fastenBucketedReadSuite.
Authored-by: Gengliang Wang <gengliang.wang@databricks.com>
Signed-off-by: hyukjinkwon <gurwls223@apache.org>
## What changes were proposed in this pull request?
Current the CSV's infer schema code inlines `TypeCoercion.findTightestCommonType`. This is a minor refactor to make use of the common type coercion code when applicable. This way we can take advantage of any improvement to the base method.
Thanks to MaxGekk for finding this while reviewing another PR.
## How was this patch tested?
This is a minor refactor. Existing tests are used to verify the change.
Closes#22619 from dilipbiswal/csv_minor.
Authored-by: Dilip Biswal <dbiswal@us.ibm.com>
Signed-off-by: hyukjinkwon <gurwls223@apache.org>
## What changes were proposed in this pull request?
Adds support for the setting limit in the sql split function
## How was this patch tested?
1. Updated unit tests
2. Tested using Scala spark shell
Please review http://spark.apache.org/contributing.html before opening a pull request.
Closes#22227 from phegstrom/master.
Authored-by: Parker Hegstrom <phegstrom@palantir.com>
Signed-off-by: hyukjinkwon <gurwls223@apache.org>
## What changes were proposed in this pull request?
In this test case, we are verifying that the result of an UDF is cached when the underlying data frame is cached and that the udf is not evaluated again when the cached data frame is used.
To reduce the runtime we do :
1) Use a single partition dataframe, so the total execution time of UDF is more deterministic.
2) Cut down the size of the dataframe from 10 to 2.
3) Reduce the sleep time in the UDF from 5secs to 2secs.
4) Reduce the failafter condition from 3 to 2.
With the above change, it takes about 4 secs to cache the first dataframe. And subsequent check takes a few hundred milliseconds.
The new runtime for 5 consecutive runs of this test is as follows :
```
[info] - cache UDF result correctly (4 seconds, 906 milliseconds)
[info] - cache UDF result correctly (4 seconds, 281 milliseconds)
[info] - cache UDF result correctly (4 seconds, 288 milliseconds)
[info] - cache UDF result correctly (4 seconds, 355 milliseconds)
[info] - cache UDF result correctly (4 seconds, 280 milliseconds)
```
## How was this patch tested?
This is s test fix.
Closes#22638 from dilipbiswal/SPARK-25610.
Authored-by: Dilip Biswal <dbiswal@us.ibm.com>
Signed-off-by: gatorsmile <gatorsmile@gmail.com>
## What changes were proposed in this pull request?
The total run time of `HiveSparkSubmitSuite` is about 10 minutes.
While the related code is stable, add tag `ExtendedHiveTest` for it.
## How was this patch tested?
Unit test.
Closes#22642 from gengliangwang/addTagForHiveSparkSubmitSuite.
Authored-by: Gengliang Wang <gengliang.wang@databricks.com>
Signed-off-by: gatorsmile <gatorsmile@gmail.com>
## What changes were proposed in this pull request?
Before ORC 1.5.3, `orc.dictionary.key.threshold` and `hive.exec.orc.dictionary.key.size.threshold` are applied for all columns. This has been a big huddle to enable dictionary encoding. From ORC 1.5.3, `orc.column.encoding.direct` is added to enforce direct encoding selectively in a column-wise manner. This PR aims to add that feature by upgrading ORC from 1.5.2 to 1.5.3.
The followings are the patches in ORC 1.5.3 and this feature is the only one related to Spark directly.
```
ORC-406: ORC: Char(n) and Varchar(n) writers truncate to n bytes & corrupts multi-byte data (gopalv)
ORC-403: [C++] Add checks to avoid invalid offsets in InputStream
ORC-405: Remove calcite as a dependency from the benchmarks.
ORC-375: Fix libhdfs on gcc7 by adding #include <functional> two places.
ORC-383: Parallel builds fails with ConcurrentModificationException
ORC-382: Apache rat exclusions + add rat check to travis
ORC-401: Fix incorrect quoting in specification.
ORC-385: Change RecordReader to extend Closeable.
ORC-384: [C++] fix memory leak when loading non-ORC files
ORC-391: [c++] parseType does not accept underscore in the field name
ORC-397: Allow selective disabling of dictionary encoding. Original patch was by Mithun Radhakrishnan.
ORC-389: Add ability to not decode Acid metadata columns
```
## How was this patch tested?
Pass the Jenkins with newly added test cases.
Closes#22622 from dongjoon-hyun/SPARK-25635.
Authored-by: Dongjoon Hyun <dongjoon@apache.org>
Signed-off-by: gatorsmile <gatorsmile@gmail.com>
## What changes were proposed in this pull request?
Improve the runtime by reducing the number of partitions created in the test. The number of partitions are reduced from 280 to 60.
Here are the test times for the `getPartitionsByFilter returns all partitions` test on my laptop.
```
[info] - 0.13: getPartitionsByFilter returns all partitions when hive.metastore.try.direct.sql=false (4 seconds, 230 milliseconds)
[info] - 0.14: getPartitionsByFilter returns all partitions when hive.metastore.try.direct.sql=false (3 seconds, 576 milliseconds)
[info] - 1.0: getPartitionsByFilter returns all partitions when hive.metastore.try.direct.sql=false (3 seconds, 495 milliseconds)
[info] - 1.1: getPartitionsByFilter returns all partitions when hive.metastore.try.direct.sql=false (6 seconds, 728 milliseconds)
[info] - 1.2: getPartitionsByFilter returns all partitions when hive.metastore.try.direct.sql=false (7 seconds, 260 milliseconds)
[info] - 2.0: getPartitionsByFilter returns all partitions when hive.metastore.try.direct.sql=false (8 seconds, 270 milliseconds)
[info] - 2.1: getPartitionsByFilter returns all partitions when hive.metastore.try.direct.sql=false (6 seconds, 856 milliseconds)
[info] - 2.2: getPartitionsByFilter returns all partitions when hive.metastore.try.direct.sql=false (7 seconds, 587 milliseconds)
[info] - 2.3: getPartitionsByFilter returns all partitions when hive.metastore.try.direct.sql=false (7 seconds, 230 milliseconds)
## How was this patch tested?
Test only.
Closes#22644 from dilipbiswal/SPARK-25626.
Authored-by: Dilip Biswal <dbiswal@us.ibm.com>
Signed-off-by: gatorsmile <gatorsmile@gmail.com>
## What changes were proposed in this pull request?
The java `foreachBatch` API in `DataStreamWriter` should accept `java.lang.Long` rather `scala.Long`.
## How was this patch tested?
New java test.
Closes#22633 from zsxwing/fix-java-foreachbatch.
Authored-by: Shixiong Zhu <zsxwing@gmail.com>
Signed-off-by: Shixiong Zhu <zsxwing@gmail.com>
## What changes were proposed in this pull request?
When constructing a DataFrame from a Java bean, using nested beans throws an error despite [documentation](http://spark.apache.org/docs/latest/sql-programming-guide.html#inferring-the-schema-using-reflection) stating otherwise. This PR aims to add that support.
This PR does not yet add nested beans support in array or List fields. This can be added later or in another PR.
## How was this patch tested?
Nested bean was added to the appropriate unit test.
Also manually tested in Spark shell on code emulating the referenced JIRA:
```
scala> import scala.beans.BeanProperty
import scala.beans.BeanProperty
scala> class SubCategory(BeanProperty var id: String, BeanProperty var name: String) extends Serializable
defined class SubCategory
scala> class Category(BeanProperty var id: String, BeanProperty var subCategory: SubCategory) extends Serializable
defined class Category
scala> import scala.collection.JavaConverters._
import scala.collection.JavaConverters._
scala> spark.createDataFrame(Seq(new Category("s-111", new SubCategory("sc-111", "Sub-1"))).asJava, classOf[Category])
java.lang.IllegalArgumentException: The value (SubCategory65130cf2) of the type (SubCategory) cannot be converted to struct<id:string,name:string>
at org.apache.spark.sql.catalyst.CatalystTypeConverters$StructConverter.toCatalystImpl(CatalystTypeConverters.scala:262)
at org.apache.spark.sql.catalyst.CatalystTypeConverters$StructConverter.toCatalystImpl(CatalystTypeConverters.scala:238)
at org.apache.spark.sql.catalyst.CatalystTypeConverters$CatalystTypeConverter.toCatalyst(CatalystTypeConverters.scala:103)
at org.apache.spark.sql.catalyst.CatalystTypeConverters$$anonfun$createToCatalystConverter$2.apply(CatalystTypeConverters.scala:396)
at org.apache.spark.sql.SQLContext$$anonfun$beansToRows$1$$anonfun$apply$1.apply(SQLContext.scala:1108)
at org.apache.spark.sql.SQLContext$$anonfun$beansToRows$1$$anonfun$apply$1.apply(SQLContext.scala:1108)
at scala.collection.TraversableLike$$anonfun$map$1.apply(TraversableLike.scala:234)
at scala.collection.TraversableLike$$anonfun$map$1.apply(TraversableLike.scala:234)
at scala.collection.IndexedSeqOptimized$class.foreach(IndexedSeqOptimized.scala:33)
at scala.collection.mutable.ArrayOps$ofRef.foreach(ArrayOps.scala:186)
at scala.collection.TraversableLike$class.map(TraversableLike.scala:234)
at scala.collection.mutable.ArrayOps$ofRef.map(ArrayOps.scala:186)
at org.apache.spark.sql.SQLContext$$anonfun$beansToRows$1.apply(SQLContext.scala:1108)
at org.apache.spark.sql.SQLContext$$anonfun$beansToRows$1.apply(SQLContext.scala:1106)
at scala.collection.Iterator$$anon$11.next(Iterator.scala:410)
at scala.collection.Iterator$class.toStream(Iterator.scala:1320)
at scala.collection.AbstractIterator.toStream(Iterator.scala:1334)
at scala.collection.TraversableOnce$class.toSeq(TraversableOnce.scala:298)
at scala.collection.AbstractIterator.toSeq(Iterator.scala:1334)
at org.apache.spark.sql.SparkSession.createDataFrame(SparkSession.scala:423)
... 51 elided
```
New behavior:
```
scala> spark.createDataFrame(Seq(new Category("s-111", new SubCategory("sc-111", "Sub-1"))).asJava, classOf[Category])
res0: org.apache.spark.sql.DataFrame = [id: string, subCategory: struct<id: string, name: string>]
scala> res0.show()
+-----+---------------+
| id| subCategory|
+-----+---------------+
|s-111|[sc-111, Sub-1]|
+-----+---------------+
```
Closes#22527 from michalsenkyr/SPARK-17952.
Authored-by: Michal Senkyr <mike.senkyr@gmail.com>
Signed-off-by: Takuya UESHIN <ueshin@databricks.com>
Hi all,
Jackson is incompatible with upstream versions, therefore bump the Jackson version to a more recent one. I bumped into some issues with Azure CosmosDB that is using a more recent version of Jackson. This can be fixed by adding exclusions and then it works without any issues. So no breaking changes in the API's.
I would also consider bumping the version of Jackson in Spark. I would suggest to keep up to date with the dependencies, since in the future this issue will pop up more frequently.
## What changes were proposed in this pull request?
Bump Jackson to 2.9.6
## How was this patch tested?
Compiled and tested it locally to see if anything broke.
Please review http://spark.apache.org/contributing.html before opening a pull request.
Closes#21596 from Fokko/fd-bump-jackson.
Authored-by: Fokko Driesprong <fokkodriesprong@godatadriven.com>
Signed-off-by: hyukjinkwon <gurwls223@apache.org>
## What changes were proposed in this pull request?
``As part of insert command in FileFormatWriter, a job context is created for handling the write operation , While initializing the job context using setupJob() API
in HadoopMapReduceCommitProtocol , we set the jobid in the Jobcontext configuration.In FileFormatWriter since we are directly getting the jobId from the map reduce JobContext the job id will come as null while adding the log. As a solution we shall get the jobID from the configuration of the map reduce Jobcontext.``
## How was this patch tested?
Manually, verified the logs after the changes.

Closes#22572 from sujith71955/master_log_issue.
Authored-by: s71955 <sujithchacko.2010@gmail.com>
Signed-off-by: Wenchen Fan <wenchen@databricks.com>
While working on another PR, I noticed that there is quite some legacy Java in there that can be beautified. For example the use og features from Java8, such as:
- Collection libraries
- Try-with-resource blocks
No code has been changed
What are your thoughts on this?
This makes code easier to read, and using try-with-resource makes is less likely to forget to close something.
## What changes were proposed in this pull request?
(Please fill in changes proposed in this fix)
## How was this patch tested?
(Please explain how this patch was tested. E.g. unit tests, integration tests, manual tests)
(If this patch involves UI changes, please attach a screenshot; otherwise, remove this)
Please review http://spark.apache.org/contributing.html before opening a pull request.
Closes#22399 from Fokko/SPARK-25408.
Authored-by: Fokko Driesprong <fokkodriesprong@godatadriven.com>
Signed-off-by: Sean Owen <sean.owen@databricks.com>
## What changes were proposed in this pull request?
The test `cast string to timestamp` used to run for all time zones. So it run for more than 600 times. Running the tests for a significant subset of time zones is probably good enough and doing this in a randomized manner enforces anyway that we are going to test all time zones in different runs.
## How was this patch tested?
the test time reduces to 11 seconds from more than 2 minutes
Closes#22631 from mgaido91/SPARK-25605.
Authored-by: Marco Gaido <marcogaido91@gmail.com>
Signed-off-by: gatorsmile <gatorsmile@gmail.com>
## What changes were proposed in this pull request?
Reduce `DateExpressionsSuite.Hour` test time costs in Jenkins by reduce iteration times.
## How was this patch tested?
Manual tests on my local machine.
before:
```
- Hour (34 seconds, 54 milliseconds)
```
after:
```
- Hour (2 seconds, 697 milliseconds)
```
Closes#22632 from wangyum/SPARK-25606.
Authored-by: Yuming Wang <yumwang@ebay.com>
Signed-off-by: gatorsmile <gatorsmile@gmail.com>
## What changes were proposed in this pull request?
The PR changes the test introduced for SPARK-22226, so that we don't run analysis and optimization on the plan. The scope of the test is code generation and running the above mentioned operation is expensive and useless for the test.
The UT was also moved to the `CodeGenerationSuite` which is a better place given the scope of the test.
## How was this patch tested?
running the UT before SPARK-22226 fails, after it passes. The execution time is about 50% the original one. On my laptop this means that the test now runs in about 23 seconds (instead of 50 seconds).
Closes#22629 from mgaido91/SPARK-25609.
Authored-by: Marco Gaido <marcogaido91@gmail.com>
Signed-off-by: gatorsmile <gatorsmile@gmail.com>
## What changes were proposed in this pull request?
Refactor `DatasetBenchmark` to use main method.
Generate benchmark result:
```sh
SPARK_GENERATE_BENCHMARK_FILES=1 build/sbt "sql/test:runMain org.apache.spark.sql.DatasetBenchmark"
```
## How was this patch tested?
manual tests
Closes#22488 from wangyum/SPARK-25479.
Lead-authored-by: Yuming Wang <yumwang@ebay.com>
Co-authored-by: Dongjoon Hyun <dongjoon@apache.org>
Signed-off-by: Dongjoon Hyun <dongjoon@apache.org>
## What changes were proposed in this pull request?
In `SparkPlan.getByteArrayRdd`, we should only call `it.hasNext` when the limit is not hit, as `iter.hasNext` may produce one row and buffer it, and cause wrong metrics.
## How was this patch tested?
new tests
Closes#22621 from cloud-fan/range.
Authored-by: Wenchen Fan <wenchen@databricks.com>
Signed-off-by: Wenchen Fan <wenchen@databricks.com>
## What changes were proposed in this pull request?
In #20850 when writing non-null decimals, instead of zero-ing all the 16 allocated bytes, we zero-out only the padding bytes. Since we always allocate 16 bytes, if the number of bytes needed for a decimal is lower than 9, then this means that the bytes between 8 and 16 are not zero-ed.
I see 2 solutions here:
- we can zero-out all the bytes in advance as it was done before #20850 (safer solution IMHO);
- we can allocate only the needed bytes (may be a bit more efficient in terms of memory used, but I have not investigated the feasibility of this option).
Hence I propose here the first solution in order to fix the correctness issue. We can eventually switch to the second if we think is more efficient later.
## How was this patch tested?
Running the test attached in the JIRA + added UT
Closes#22602 from mgaido91/SPARK-25582.
Authored-by: Marco Gaido <marcogaido91@gmail.com>
Signed-off-by: Dongjoon Hyun <dongjoon@apache.org>
## What changes were proposed in this pull request?
Refactor `UnsafeArrayDataBenchmark` to use main method.
Generate benchmark result:
```sh
SPARK_GENERATE_BENCHMARK_FILES=1 build/sbt "sql/test:runMain org.apache.spark.sql.execution.benchmark.UnsafeArrayDataBenchmark"
```
## How was this patch tested?
manual tests
Closes#22491 from wangyum/SPARK-25483.
Lead-authored-by: Yuming Wang <yumwang@ebay.com>
Co-authored-by: Dongjoon Hyun <dongjoon@apache.org>
Signed-off-by: Dongjoon Hyun <dongjoon@apache.org>
## What changes were proposed in this pull request?
This PR aims to add `BloomFilterBenchmark`. For ORC data source, Apache Spark has been supporting for a long time. For Parquet data source, it's expected to be added with next Parquet release update.
## How was this patch tested?
Manual.
```scala
SPARK_GENERATE_BENCHMARK_FILES=1 build/sbt "sql/test:runMain org.apache.spark.sql.execution.benchmark.BloomFilterBenchmark"
```
Closes#22605 from dongjoon-hyun/SPARK-25589.
Authored-by: Dongjoon Hyun <dongjoon@apache.org>
Signed-off-by: Dongjoon Hyun <dongjoon@apache.org>
## What changes were proposed in this pull request?
Rename method `benchmark` in `BenchmarkBase` as `runBenchmarkSuite `. Also add comments.
Currently the method name `benchmark` is a bit confusing. Also the name is the same as instances of `Benchmark`:
f246813afb/sql/hive/src/test/scala/org/apache/spark/sql/hive/orc/OrcReadBenchmark.scala (L330-L339)
## How was this patch tested?
Unit test.
Closes#22599 from gengliangwang/renameBenchmarkSuite.
Authored-by: Gengliang Wang <gengliang.wang@databricks.com>
Signed-off-by: Dongjoon Hyun <dongjoon@apache.org>
## What changes were proposed in this pull request?
This patch is to bump the master branch version to 3.0.0-SNAPSHOT.
## How was this patch tested?
N/A
Closes#22606 from gatorsmile/bump3.0.
Authored-by: gatorsmile <gatorsmile@gmail.com>
Signed-off-by: gatorsmile <gatorsmile@gmail.com>
## What changes were proposed in this pull request?
Currently, SQL tab in the WEBUI doesn't support hiding table. Other tabs in the web ui like, Jobs, stages etc supports hiding table (refer SPARK-23024 https://github.com/apache/spark/pull/20216).
In this PR, added the support for hide table in the sql tab also.
## How was this patch tested?
bin/spark-shell
```
sql("create table a (id int)")
for(i <- 1 to 100) sql(s"insert into a values ($i)")
```
Open SQL tab in the web UI
**Before fix:**
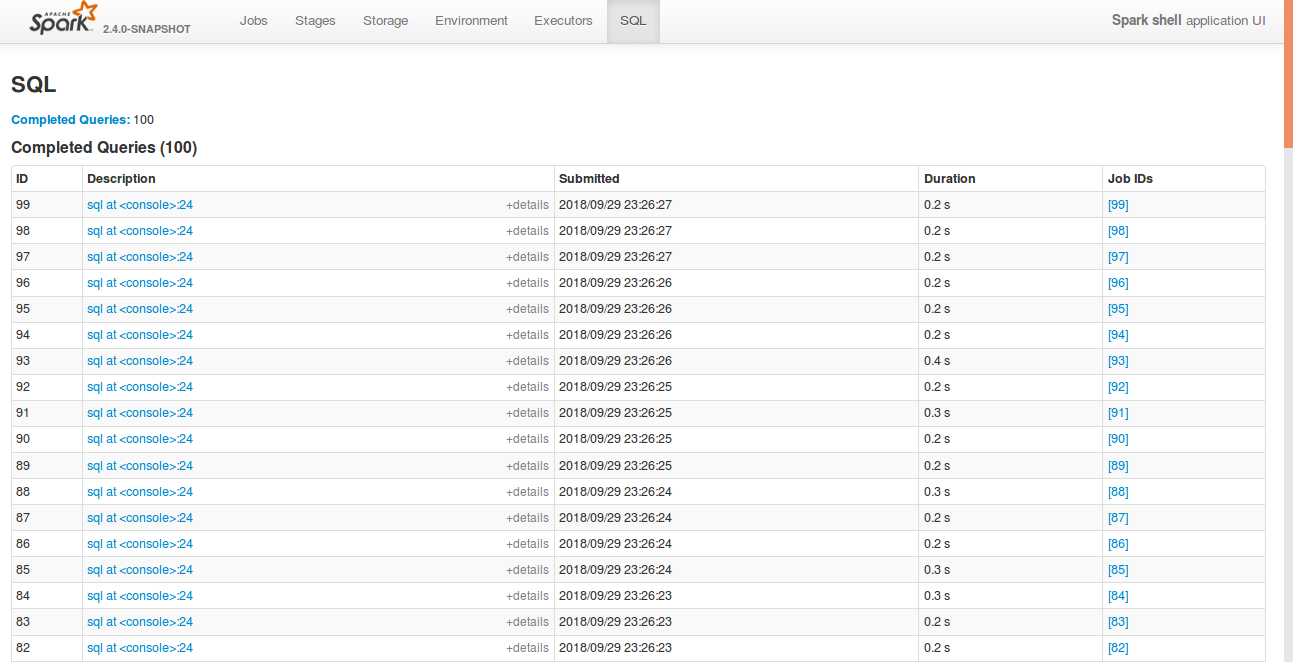
**After fix:** Consistent with the other tabs.

(Please explain how this patch was tested. E.g. unit tests, integration tests, manual tests)
(If this patch involves UI changes, please attach a screenshot; otherwise, remove this)
Please review http://spark.apache.org/contributing.html before opening a pull request.
Closes#22592 from shahidki31/SPARK-25575.
Authored-by: Shahid <shahidki31@gmail.com>
Signed-off-by: Sean Owen <sean.owen@databricks.com>
## What changes were proposed in this pull request?
This PR does 2 things:
1. Add a new trait(`SqlBasedBenchmark`) to better support Dataset and DataFrame API.
2. Refactor `AggregateBenchmark` to use main method. Generate benchmark result:
```
SPARK_GENERATE_BENCHMARK_FILES=1 build/sbt "sql/test:runMain org.apache.spark.sql.execution.benchmark.AggregateBenchmark"
```
## How was this patch tested?
manual tests
Closes#22484 from wangyum/SPARK-25476.
Lead-authored-by: Yuming Wang <yumwang@ebay.com>
Co-authored-by: Dongjoon Hyun <dongjoon@apache.org>
Signed-off-by: Dongjoon Hyun <dongjoon@apache.org>
## What changes were proposed in this pull request?
#22519 introduced a bug when the attributes in the pivot clause are cosmetically different from the output ones (eg. different case). In particular, the problem is that the PR used a `Set[Attribute]` instead of an `AttributeSet`.
## How was this patch tested?
added UT
Closes#22582 from mgaido91/SPARK-25505_followup.
Authored-by: Marco Gaido <marcogaido91@gmail.com>
Signed-off-by: gatorsmile <gatorsmile@gmail.com>
## What changes were proposed in this pull request?
This PR adds a rule to force `.toLowerCase(Locale.ROOT)` or `toUpperCase(Locale.ROOT)`.
It produces an error as below:
```
[error] Are you sure that you want to use toUpperCase or toLowerCase without the root locale? In most cases, you
[error] should use toUpperCase(Locale.ROOT) or toLowerCase(Locale.ROOT) instead.
[error] If you must use toUpperCase or toLowerCase without the root locale, wrap the code block with
[error] // scalastyle:off caselocale
[error] .toUpperCase
[error] .toLowerCase
[error] // scalastyle:on caselocale
```
This PR excludes the cases above for SQL code path for external calls like table name, column name and etc.
For test suites, or when it's clear there's no locale problem like Turkish locale problem, it uses `Locale.ROOT`.
One minor problem is, `UTF8String` has both methods, `toLowerCase` and `toUpperCase`, and the new rule detects them as well. They are ignored.
## How was this patch tested?
Manually tested, and Jenkins tests.
Closes#22581 from HyukjinKwon/SPARK-25565.
Authored-by: hyukjinkwon <gurwls223@apache.org>
Signed-off-by: hyukjinkwon <gurwls223@apache.org>
## What changes were proposed in this pull request?
Refactor OrcReadBenchmark to use main method.
Generate benchmark result:
```
SPARK_GENERATE_BENCHMARK_FILES=1 build/sbt "hive/test:runMain org.apache.spark.sql.hive.orc.OrcReadBenchmark"
```
## How was this patch tested?
manual tests
Closes#22580 from yucai/SPARK-25508.
Lead-authored-by: yucai <yyu1@ebay.com>
Co-authored-by: Yucai Yu <yucai.yu@foxmail.com>
Co-authored-by: Dongjoon Hyun <dongjoon@apache.org>
Signed-off-by: Dongjoon Hyun <dongjoon@apache.org>
## What changes were proposed in this pull request?
In the PR, I propose to extend implementation of existing method:
```
def pivot(pivotColumn: Column, values: Seq[Any]): RelationalGroupedDataset
```
to support values of the struct type. This allows pivoting by multiple columns combined by `struct`:
```
trainingSales
.groupBy($"sales.year")
.pivot(
pivotColumn = struct(lower($"sales.course"), $"training"),
values = Seq(
struct(lit("dotnet"), lit("Experts")),
struct(lit("java"), lit("Dummies")))
).agg(sum($"sales.earnings"))
```
## How was this patch tested?
Added a test for values specified via `struct` in Java and Scala.
Closes#22316 from MaxGekk/pivoting-by-multiple-columns2.
Lead-authored-by: Maxim Gekk <maxim.gekk@databricks.com>
Co-authored-by: Maxim Gekk <max.gekk@gmail.com>
Signed-off-by: hyukjinkwon <gurwls223@apache.org>
## What changes were proposed in this pull request?
In the PR, I propose to extended the `schema_of_json()` function, and accept JSON options since they can impact on schema inferring. Purpose is to support the same options that `from_json` can use during schema inferring.
## How was this patch tested?
Added SQL, Python and Scala tests (`JsonExpressionsSuite` and `JsonFunctionsSuite`) that checks JSON options are used.
Closes#22442 from MaxGekk/schema_of_json-options.
Authored-by: Maxim Gekk <maxim.gekk@databricks.com>
Signed-off-by: hyukjinkwon <gurwls223@apache.org>
## What changes were proposed in this pull request?
This PR aims to prevent test slowdowns at `HiveExternalCatalogVersionsSuite` by using the latest Apache Spark 2.3.2 link because the Apache mirrors will remove the old Spark 2.3.1 binaries eventually. `HiveExternalCatalogVersionsSuite` will not fail because [SPARK-24813](https://issues.apache.org/jira/browse/SPARK-24813) implements a fallback logic. However, it will cause many trials and fallbacks in all builds over `branch-2.3/branch-2.4/master`. We had better fix this issue.
## How was this patch tested?
Pass the Jenkins with the updated version.
Closes#22587 from dongjoon-hyun/SPARK-25570.
Authored-by: Dongjoon Hyun <dongjoon@apache.org>
Signed-off-by: hyukjinkwon <gurwls223@apache.org>
## What changes were proposed in this pull request?
Currently, in `ParquetFilters`, if one of the children predicates is not supported by Parquet, the entire predicates will be thrown away. In fact, if the unsupported predicate is in the top level `And` condition or in the child before hitting `Not` or `Or` condition, it can be safely removed.
## How was this patch tested?
Tests are added.
Closes#22574 from dbtsai/removeUnsupportedPredicatesInParquet.
Lead-authored-by: DB Tsai <d_tsai@apple.com>
Co-authored-by: Dongjoon Hyun <dongjoon@apache.org>
Co-authored-by: DB Tsai <dbtsai@dbtsai.com>
Signed-off-by: Dongjoon Hyun <dongjoon@apache.org>
## What changes were proposed in this pull request?
Use `Set` instead of `Array` to improve `accumulatorIds.contains(acc.id)` performance.
This PR close https://github.com/apache/spark/pull/22420
## How was this patch tested?
manual tests.
Benchmark code:
```scala
def benchmark(func: () => Unit): Long = {
val start = System.currentTimeMillis()
func()
val end = System.currentTimeMillis()
end - start
}
val range = Range(1, 1000000)
val set = range.toSet
val array = range.toArray
for (i <- 0 until 5) {
val setExecutionTime =
benchmark(() => for (i <- 0 until 500) { set.contains(scala.util.Random.nextInt()) })
val arrayExecutionTime =
benchmark(() => for (i <- 0 until 500) { array.contains(scala.util.Random.nextInt()) })
println(s"set execution time: $setExecutionTime, array execution time: $arrayExecutionTime")
}
```
Benchmark result:
```
set execution time: 4, array execution time: 2760
set execution time: 1, array execution time: 1911
set execution time: 3, array execution time: 2043
set execution time: 12, array execution time: 2214
set execution time: 6, array execution time: 1770
```
Closes#22579 from wangyum/SPARK-25429.
Authored-by: Yuming Wang <yumwang@ebay.com>
Signed-off-by: gatorsmile <gatorsmile@gmail.com>
## What changes were proposed in this pull request?
**Description from the JIRA :**
Currently, to collect the statistics of all the columns, users need to specify the names of all the columns when calling the command "ANALYZE TABLE ... FOR COLUMNS...". This is not user friendly. Instead, we can introduce the following SQL command to achieve it without specifying the column names.
```
ANALYZE TABLE [db_name.]tablename COMPUTE STATISTICS FOR ALL COLUMNS;
```
## How was this patch tested?
Added new tests in SparkSqlParserSuite and StatisticsSuite
Closes#22566 from dilipbiswal/SPARK-25458.
Authored-by: Dilip Biswal <dbiswal@us.ibm.com>
Signed-off-by: gatorsmile <gatorsmile@gmail.com>
## What changes were proposed in this pull request?
The grouping columns from a Pivot query are inferred as "input columns - pivot columns - pivot aggregate columns", where input columns are the output of the child relation of Pivot. The grouping columns will be the leading columns in the pivot output and they should preserve the same order as specified by the input. For example,
```
SELECT * FROM (
SELECT course, earnings, "a" as a, "z" as z, "b" as b, "y" as y, "c" as c, "x" as x, "d" as d, "w" as w
FROM courseSales
)
PIVOT (
sum(earnings)
FOR course IN ('dotNET', 'Java')
)
```
The output columns should be "a, z, b, y, c, x, d, w, ..." but now it is "a, b, c, d, w, x, y, z, ..."
The fix is to use the child plan's `output` instead of `outputSet` so that the order can be preserved.
## How was this patch tested?
Added UT.
Closes#22519 from maryannxue/spark-25505.
Authored-by: maryannxue <maryannxue@apache.org>
Signed-off-by: gatorsmile <gatorsmile@gmail.com>
## What changes were proposed in this pull request?
The `show create table` will show a lot of generated attributes for views that created by older Spark version. This PR will basically revert https://issues.apache.org/jira/browse/SPARK-19272 back, so when you `DESC [FORMATTED|EXTENDED] view` will show the original view DDL text.
## How was this patch tested?
Unit test.
Closes#22458 from zheyuan28/testbranch.
Lead-authored-by: Chris Zhao <chris.zhao@databricks.com>
Co-authored-by: Christopher Zhao <chris.zhao@databricks.com>
Co-authored-by: Dongjoon Hyun <dongjoon@apache.org>
Signed-off-by: Dongjoon Hyun <dongjoon@apache.org>
## What changes were proposed in this pull request?
There are 2 places we check for problematic `InSubquery`: the rule `ResolveSubquery` and `InSubquery.checkInputDataTypes`. We should unify them.
## How was this patch tested?
existing tests
Closes#22563 from cloud-fan/followup.
Authored-by: Wenchen Fan <wenchen@databricks.com>
Signed-off-by: Wenchen Fan <wenchen@databricks.com>
## What changes were proposed in this pull request?
As per the discussion in https://github.com/apache/spark/pull/22553#pullrequestreview-159192221,
override `filterKeys` violates the documented semantics.
This PR is to remove it and add documentation.
Also fix one potential non-serializable map in `FileStreamOptions`.
The only one call of `CaseInsensitiveMap`'s `filterKeys` left is
c3c45cbd76/sql/hive/src/main/scala/org/apache/spark/sql/hive/execution/HiveOptions.scala (L88-L90)
But this one is OK.
## How was this patch tested?
Existing unit tests.
Closes#22562 from gengliangwang/SPARK-25541-FOLLOWUP.
Authored-by: Gengliang Wang <gengliang.wang@databricks.com>
Signed-off-by: Wenchen Fan <wenchen@databricks.com>
## What changes were proposed in this pull request?
The PR removes the `InSubquery` expression which was introduced a long time ago and its only usage was removed in 4ce970d714. Hence it is not used anymore.
## How was this patch tested?
existing UTs
Closes#22556 from mgaido91/minor_insubq.
Authored-by: Marco Gaido <marcogaido91@gmail.com>
Signed-off-by: Wenchen Fan <wenchen@databricks.com>
## What changes were proposed in this pull request?
Thanks for bahchis reporting this. It is more like a follow up work for #16581, this PR fix the scenario of Python UDF accessing attributes from both side of join in join condition.
## How was this patch tested?
Add regression tests in PySpark and `BatchEvalPythonExecSuite`.
Closes#22326 from xuanyuanking/SPARK-25314.
Authored-by: Yuanjian Li <xyliyuanjian@gmail.com>
Signed-off-by: Wenchen Fan <wenchen@databricks.com>
## What changes were proposed in this pull request?
In ElementAt, when first argument is MapType, we should coerce the key type and the second argument based on findTightestCommonType. This is not happening currently. We may produce wrong output as we will incorrectly downcast the right hand side double expression to int.
```SQL
spark-sql> select element_at(map(1,"one", 2, "two"), 2.2);
two
```
Also, when the first argument is ArrayType, the second argument should be an integer type or a smaller integral type that can be safely casted to an integer type. Currently we may do an unsafe cast. In the following case, we should fail with an error as 2.2 is not a integer index. But instead we down cast it to int currently and return a result instead.
```SQL
spark-sql> select element_at(array(1,2), 1.24D);
1
```
This PR also supports implicit cast between two MapTypes. I have followed similar logic that exists today to do implicit casts between two array types.
## How was this patch tested?
Added new tests in DataFrameFunctionSuite, TypeCoercionSuite.
Closes#22544 from dilipbiswal/SPARK-25522.
Authored-by: Dilip Biswal <dbiswal@us.ibm.com>
Signed-off-by: Wenchen Fan <wenchen@databricks.com>
## What changes were proposed in this pull request?
We have an agreement that the behavior of `from/to_utc_timestamp` is corrected, although the function itself doesn't make much sense in Spark: https://issues.apache.org/jira/browse/SPARK-23715
This PR improves the document.
## How was this patch tested?
N/A
Closes#22543 from cloud-fan/doc.
Authored-by: Wenchen Fan <wenchen@databricks.com>
Signed-off-by: Wenchen Fan <wenchen@databricks.com>
## What changes were proposed in this pull request?
Refactor `UnsafeProjectionBenchmark` to use main method.
Generate benchmark result:
```
SPARK_GENERATE_BENCHMARK_FILES=1 build/sbt "catalyst/test:runMain org.apache.spark.sql.UnsafeProjectionBenchmark"
```
## How was this patch tested?
manual test
Closes#22493 from yucai/SPARK-25485.
Lead-authored-by: yucai <yyu1@ebay.com>
Co-authored-by: Yucai Yu <yucai.yu@foxmail.com>
Co-authored-by: Dongjoon Hyun <dongjoon@apache.org>
Signed-off-by: Dongjoon Hyun <dongjoon@apache.org>
## What changes were proposed in this pull request?
Refactor `ColumnarBatchBenchmark` to use main method.
Generate benchmark result:
```
SPARK_GENERATE_BENCHMARK_FILES=1 build/sbt "sql/test:runMain org.apache.spark.sql.execution.vectorized.ColumnarBatchBenchmark"
```
## How was this patch tested?
manual tests
Closes#22490 from yucai/SPARK-25481.
Lead-authored-by: yucai <yyu1@ebay.com>
Co-authored-by: Yucai Yu <yucai.yu@foxmail.com>
Co-authored-by: Dongjoon Hyun <dongjoon@apache.org>
Signed-off-by: Dongjoon Hyun <dongjoon@apache.org>
## What changes were proposed in this pull request?
https://github.com/apache/spark/pull/20023 proposed to allow precision lose during decimal operations, to reduce the possibilities of overflow. This is a behavior change and is protected by the DECIMAL_OPERATIONS_ALLOW_PREC_LOSS config. However, that PR introduced another behavior change: pick a minimum precision for integral literals, which is not protected by a config. This PR add a new config for it: `spark.sql.literal.pickMinimumPrecision`.
This can allow users to work around issue in SPARK-25454, which is caused by a long-standing bug of negative scale.
## How was this patch tested?
a new test
Closes#22494 from cloud-fan/decimal.
Authored-by: Wenchen Fan <wenchen@databricks.com>
Signed-off-by: gatorsmile <gatorsmile@gmail.com>
## What changes were proposed in this pull request?
Describe spark.sql.parquet.writeLegacyFormat property in Spark SQL, DataFrames and Datasets Guide.
## How was this patch tested?
N/A
Closes#22453 from seancxmao/SPARK-20937.
Authored-by: seancxmao <seancxmao@gmail.com>
Signed-off-by: hyukjinkwon <gurwls223@apache.org>
## What changes were proposed in this pull request?
This PR contains 3 optimizations:
1) it improves significantly the operation `--` on `AttributeSet`. As a benchmark for the `--` operation, the following code has been run
```
test("AttributeSet -- benchmark") {
val attrSetA = AttributeSet((1 to 100).map { i => AttributeReference(s"c$i", IntegerType)() })
val attrSetB = AttributeSet(attrSetA.take(80).toSeq)
val attrSetC = AttributeSet((1 to 100).map { i => AttributeReference(s"c2_$i", IntegerType)() })
val attrSetD = AttributeSet((attrSetA.take(50) ++ attrSetC.take(50)).toSeq)
val attrSetE = AttributeSet((attrSetC.take(50) ++ attrSetA.take(50)).toSeq)
val n_iter = 1000000
val t0 = System.nanoTime()
(1 to n_iter) foreach { _ =>
val r1 = attrSetA -- attrSetB
val r2 = attrSetA -- attrSetC
val r3 = attrSetA -- attrSetD
val r4 = attrSetA -- attrSetE
}
val t1 = System.nanoTime()
val totalTime = t1 - t0
println(s"Average time: ${totalTime / n_iter} us")
}
```
The results are:
```
Before PR - Average time: 67674 us (100 %)
After PR - Average time: 28827 us (42.6 %)
```
2) In `ColumnPruning`, it replaces the occurrences of `(attributeSet1 -- attributeSet2).nonEmpty` with `attributeSet1.subsetOf(attributeSet2)` which is order of magnitudes more efficient (especially where there are many attributes). Running the previous benchmark replacing `--` with `subsetOf` returns:
```
Average time: 67 us (0.1 %)
```
3) Provides a more efficient way of building `AttributeSet`s, which can greatly improve the performance of the methods `references` and `outputSet` of `Expression` and `QueryPlan`. This basically avoids unneeded operations (eg. creating many `AttributeEqual` wrapper classes which could be avoided)
The overall effect of those optimizations has been tested on `ColumnPruning` with the following benchmark:
```
test("ColumnPruning benchmark") {
val attrSetA = (1 to 100).map { i => AttributeReference(s"c$i", IntegerType)() }
val attrSetB = attrSetA.take(80)
val attrSetC = attrSetA.take(20).map(a => Alias(Add(a, Literal(1)), s"${a.name}_1")())
val input = LocalRelation(attrSetA)
val query1 = Project(attrSetB, Project(attrSetA, input)).analyze
val query2 = Project(attrSetC, Project(attrSetA, input)).analyze
val query3 = Project(attrSetA, Project(attrSetA, input)).analyze
val nIter = 100000
val t0 = System.nanoTime()
(1 to nIter).foreach { _ =>
ColumnPruning(query1)
ColumnPruning(query2)
ColumnPruning(query3)
}
val t1 = System.nanoTime()
val totalTime = t1 - t0
println(s"Average time: ${totalTime / nIter} us")
}
```
The output of the test is:
```
Before PR - Average time: 733471 us (100 %)
After PR - Average time: 362455 us (49.4 %)
```
The performance improvement has been evaluated also on the `SQLQueryTestSuite`'s queries:
```
(before) org.apache.spark.sql.catalyst.optimizer.ColumnPruning 518413198 / 1377707172 2756 / 15717
(after) org.apache.spark.sql.catalyst.optimizer.ColumnPruning 415432579 / 1121147950 2756 / 15717
% Running time 80.1% / 81.3%
```
Also other rules benefit especially from (3), despite the impact is lower, eg:
```
(before) org.apache.spark.sql.catalyst.analysis.Analyzer$ResolveReferences 307341442 / 623436806 2154 / 16480
(after) org.apache.spark.sql.catalyst.analysis.Analyzer$ResolveReferences 290511312 / 560962495 2154 / 16480
% Running time 94.5% / 90.0%
```
The reason why the impact on the `SQLQueryTestSuite`'s queries is lower compared to the other benchmark is that the optimizations are more significant when the number of attributes involved is higher. Since in the tests we often have very few attributes, the effect there is lower.
## How was this patch tested?
run benchmarks + existing UTs
Closes#22364 from mgaido91/SPARK-25379.
Authored-by: Marco Gaido <marcogaido91@gmail.com>
Signed-off-by: Wenchen Fan <wenchen@databricks.com>
## What changes were proposed in this pull request?
`CaseInsensitiveMap` is declared as Serializable. However, it is no serializable after `-` operator or `filterKeys` method.
This PR fix the issue by overriding the operator `-` and method `filterKeys`. So the we can avoid potential `NotSerializableException` on using `CaseInsensitiveMap`.
## How was this patch tested?
New test suite.
Closes#22553 from gengliangwang/fixCaseInsensitiveMap.
Authored-by: Gengliang Wang <gengliang.wang@databricks.com>
Signed-off-by: Wenchen Fan <wenchen@databricks.com>
## What changes were proposed in this pull request?
Currently, Spark has 7 `withTempPath` and 6 `withSQLConf` functions. This PR aims to remove duplicated and inconsistent code and reduce them to the following meaningful implementations.
**withTempPath**
- `SQLHelper.withTempPath`: The one which was used in `SQLTestUtils`.
**withSQLConf**
- `SQLHelper.withSQLConf`: The one which was used in `PlanTest`.
- `ExecutorSideSQLConfSuite.withSQLConf`: The one which doesn't throw `AnalysisException` on StaticConf changes.
- `SQLTestUtils.withSQLConf`: The one which overrides intentionally to change the active session.
```scala
protected override def withSQLConf(pairs: (String, String)*)(f: => Unit): Unit = {
SparkSession.setActiveSession(spark)
super.withSQLConf(pairs: _*)(f)
}
```
## How was this patch tested?
Pass the Jenkins with the existing tests.
Closes#22548 from dongjoon-hyun/SPARK-25534.
Authored-by: Dongjoon Hyun <dongjoon@apache.org>
Signed-off-by: Dongjoon Hyun <dongjoon@apache.org>
## What changes were proposed in this pull request?
The PR introduces new JSON option `pretty` which allows to turn on `DefaultPrettyPrinter` of `Jackson`'s Json generator. New option is useful in exploring of deep nested columns and in converting of JSON columns in more readable representation (look at the added test).
## How was this patch tested?
Added rount trip test which convert an JSON string to pretty representation via `from_json()` and `to_json()`.
Closes#22534 from MaxGekk/pretty-json.
Lead-authored-by: Maxim Gekk <maxim.gekk@databricks.com>
Co-authored-by: Maxim Gekk <max.gekk@gmail.com>
Signed-off-by: hyukjinkwon <gurwls223@apache.org>
## What changes were proposed in this pull request?
Add the legacy prefix for spark.sql.execution.pandas.groupedMap.assignColumnsByPosition and rename it to spark.sql.legacy.execution.pandas.groupedMap.assignColumnsByName
## How was this patch tested?
The existing tests.
Closes#22540 from gatorsmile/renameAssignColumnsByPosition.
Lead-authored-by: gatorsmile <gatorsmile@gmail.com>
Co-authored-by: Hyukjin Kwon <gurwls223@gmail.com>
Signed-off-by: hyukjinkwon <gurwls223@apache.org>
## What changes were proposed in this pull request?
Refactor SortBenchmark to use main method.
Generate benchmark result:
```
SPARK_GENERATE_BENCHMARK_FILES=1 build/sbt "sql/test:runMain org.apache.spark.sql.execution.benchmark.SortBenchmark"
```
## How was this patch tested?
manual tests
Closes#22495 from yucai/SPARK-25486.
Authored-by: yucai <yyu1@ebay.com>
Signed-off-by: Dongjoon Hyun <dongjoon@apache.org>
## What changes were proposed in this pull request?
This patch reverts entirely all the regr_* functions added in SPARK-23907. These were added by mgaido91 (and proposed by gatorsmile) to improve compatibility with other database systems, without any actual use cases. However, they are very rarely used, and in Spark there are much better ways to compute these functions, due to Spark's flexibility in exposing real programming APIs.
I'm going through all the APIs added in Spark 2.4 and I think we should revert these. If there are strong enough demands and more use cases, we can add them back in the future pretty easily.
## How was this patch tested?
Reverted test cases also.
Closes#22541 from rxin/SPARK-23907.
Authored-by: Reynold Xin <rxin@databricks.com>
Signed-off-by: hyukjinkwon <gurwls223@apache.org>
## What changes were proposed in this pull request?
In ArrayRemove, we currently cast the right hand side expression to match the element type of the left hand side Array. This may result in down casting and may return wrong result or questionable result.
Example :
```SQL
spark-sql> select array_remove(array(1,2,3), 1.23D);
[2,3]
```
```SQL
spark-sql> select array_remove(array(1,2,3), 'foo');
NULL
```
We should safely coerce both left and right hand side expressions.
## How was this patch tested?
Added tests in DataFrameFunctionsSuite
Closes#22542 from dilipbiswal/SPARK-25519.
Authored-by: Dilip Biswal <dbiswal@us.ibm.com>
Signed-off-by: Wenchen Fan <wenchen@databricks.com>
## What changes were proposed in this pull request?
In ArrayPosition, we currently cast the right hand side expression to match the element type of the left hand side Array. This may result in down casting and may return wrong result or questionable result.
Example :
```SQL
spark-sql> select array_position(array(1), 1.34);
1
```
```SQL
spark-sql> select array_position(array(1), 'foo');
null
```
We should safely coerce both left and right hand side expressions.
## How was this patch tested?
Added tests in DataFrameFunctionsSuite
Closes#22407 from dilipbiswal/SPARK-25416.
Authored-by: Dilip Biswal <dbiswal@us.ibm.com>
Signed-off-by: Wenchen Fan <wenchen@databricks.com>
## What changes were proposed in this pull request?
The function actually exists in current selected database, and it's failed to init during `lookupFunciton`, but the exception message is:
```
This function is neither a registered temporary function nor a permanent function registered in the database 'default'.
```
This is not conducive to positioning problems. This PR fix the problem.
## How was this patch tested?
new test case + manual tests
Closes#18544 from stanzhai/fix-udf-error-message.
Authored-by: Stan Zhai <mail@stanzhai.site>
Signed-off-by: Wenchen Fan <wenchen@databricks.com>
## What changes were proposed in this pull request?
Refactor `CompressionSchemeBenchmark` to use main method.
Generate benchmark result:
```sh
SPARK_GENERATE_BENCHMARK_FILES=1 build/sbt "sql/test:runMain org.apache.spark.sql.execution.columnar.compression.CompressionSchemeBenchmark"
```
## How was this patch tested?
manual tests
Closes#22486 from wangyum/SPARK-25478.
Lead-authored-by: Yuming Wang <yumwang@ebay.com>
Co-authored-by: Dongjoon Hyun <dongjoon@apache.org>
Signed-off-by: Dongjoon Hyun <dongjoon@apache.org>
## What changes were proposed in this pull request?
Add `Locale.ROOT` when `toUpperCase`.
## How was this patch tested?
manual tests
Closes#22531 from wangyum/SPARK-25415.
Authored-by: Yuming Wang <yumwang@ebay.com>
Signed-off-by: hyukjinkwon <gurwls223@apache.org>
## What changes were proposed in this pull request?
Current the file [parquetSuites.scala](f29c2b5287/sql/hive/src/test/scala/org/apache/spark/sql/hive/parquetSuites.scala) is not recognizable.
When I tried to find test suites for built-in Parquet conversions for Hive serde, I can only find [HiveParquetSuite](f29c2b5287/sql/hive/src/test/scala/org/apache/spark/sql/hive/HiveParquetSuite.scala) in the first few minutes.
This PR is to:
1. Rename `ParquetMetastoreSuite` to `HiveParquetMetastoreSuite`, and create a single file for it.
2. Rename `ParquetSourceSuite` to `HiveParquetSourceSuite`, and create a single file for it.
3. Create a single file for `ParquetPartitioningTest`.
4. Delete `parquetSuites.scala` .
## How was this patch tested?
Unit test
Closes#22467 from gengliangwang/refactor_parquet_suites.
Authored-by: Gengliang Wang <gengliang.wang@databricks.com>
Signed-off-by: gatorsmile <gatorsmile@gmail.com>
One more legacy config to go ...
Closes#22515 from rxin/allowCreatingManagedTableUsingNonemptyLocation.
Authored-by: Reynold Xin <rxin@databricks.com>
Signed-off-by: gatorsmile <gatorsmile@gmail.com>
## What changes were proposed in this pull request?
Currently there are two classes with the same naming BenchmarkBase:
1. `org.apache.spark.util.BenchmarkBase`
2. `org.apache.spark.sql.execution.benchmark.BenchmarkBase`
This is very confusing. And the benchmark object `org.apache.spark.sql.execution.benchmark.FilterPushdownBenchmark` is using the one in `org.apache.spark.util.BenchmarkBase`, while there is another class `BenchmarkBase` in the same package of it...
Here I propose:
1. the package `org.apache.spark.util.BenchmarkBase` should be in test package of core module. Move it to package `org.apache.spark.benchmark` .
2. Move `org.apache.spark.util.Benchmark` to test package of core module. Move it to package `org.apache.spark.benchmark` .
3. Rename the class `org.apache.spark.sql.execution.benchmark.BenchmarkBase` as `BenchmarkWithCodegen`
## How was this patch tested?
Unit test
Closes#22513 from gengliangwang/refactorBenchmarkBase.
Authored-by: Gengliang Wang <gengliang.wang@databricks.com>
Signed-off-by: Wenchen Fan <wenchen@databricks.com>
## What changes were proposed in this pull request?
The PR proposes to avoid usage of pattern matching for each call of ```eval``` method within:
- ```Concat```
- ```Reverse```
- ```ElementAt```
## How was this patch tested?
Run the existing tests for ```Concat```, ```Reverse``` and ```ElementAt``` expression classes.
Closes#22471 from mn-mikke/SPARK-25470.
Authored-by: Marek Novotny <mn.mikke@gmail.com>
Signed-off-by: Takeshi Yamamuro <yamamuro@apache.org>
## What changes were proposed in this pull request?
See title. Makes our legacy backward compatibility configs more consistent.
## How was this patch tested?
Make sure all references have been updated:
```
> git grep compareDateTimestampInTimestamp
docs/sql-programming-guide.md: - Since Spark 2.4, Spark compares a DATE type with a TIMESTAMP type after promotes both sides to TIMESTAMP. To set `false` to `spark.sql.legacy.compareDateTimestampInTimestamp` restores the previous behavior. This option will be removed in Spark 3.0.
sql/catalyst/src/main/scala/org/apache/spark/sql/catalyst/analysis/TypeCoercion.scala: // if conf.compareDateTimestampInTimestamp is true
sql/catalyst/src/main/scala/org/apache/spark/sql/catalyst/analysis/TypeCoercion.scala: => if (conf.compareDateTimestampInTimestamp) Some(TimestampType) else Some(StringType)
sql/catalyst/src/main/scala/org/apache/spark/sql/catalyst/analysis/TypeCoercion.scala: => if (conf.compareDateTimestampInTimestamp) Some(TimestampType) else Some(StringType)
sql/catalyst/src/main/scala/org/apache/spark/sql/internal/SQLConf.scala: buildConf("spark.sql.legacy.compareDateTimestampInTimestamp")
sql/catalyst/src/main/scala/org/apache/spark/sql/internal/SQLConf.scala: def compareDateTimestampInTimestamp : Boolean = getConf(COMPARE_DATE_TIMESTAMP_IN_TIMESTAMP)
sql/catalyst/src/test/scala/org/apache/spark/sql/catalyst/analysis/TypeCoercionSuite.scala: "spark.sql.legacy.compareDateTimestampInTimestamp" -> convertToTS.toString) {
```
Closes#22508 from rxin/SPARK-23549.
Authored-by: Reynold Xin <rxin@databricks.com>
Signed-off-by: Wenchen Fan <wenchen@databricks.com>
See above. This should go into the 2.4 release.
Closes#22509 from rxin/SPARK-25384.
Authored-by: Reynold Xin <rxin@databricks.com>
Signed-off-by: Wenchen Fan <wenchen@databricks.com>
## What changes were proposed in this pull request?
Refactor PrimitiveArrayBenchmark to use main method and print the output as a separate file.
Run blow command to generate benchmark results:
```
SPARK_GENERATE_BENCHMARK_FILES=1 build/sbt "sql/test:runMain org.apache.spark.sql.execution.benchmark.PrimitiveArrayBenchmark"
```
## How was this patch tested?
Manual tests.
Closes#22497 from seancxmao/SPARK-25487.
Authored-by: seancxmao <seancxmao@gmail.com>
Signed-off-by: Kazuaki Ishizaki <ishizaki@jp.ibm.com>
## What changes were proposed in this pull request?
This reverts commit 417ad92502.
We decided to keep the current behaviors unchanged and will consider whether we will deprecate the these functions in 3.0. For more details, see the discussion in https://issues.apache.org/jira/browse/SPARK-23715
## How was this patch tested?
The existing tests.
Closes#22505 from gatorsmile/revertSpark-23715.
Authored-by: gatorsmile <gatorsmile@gmail.com>
Signed-off-by: Wenchen Fan <wenchen@databricks.com>
## What changes were proposed in this pull request?
Refactor `DataSourceWriteBenchmark` and add write benchmark for AVRO.
## How was this patch tested?
Build and run the benchmark.
Closes#22451 from gengliangwang/avroWriteBenchmark.
Authored-by: Gengliang Wang <gengliang.wang@databricks.com>
Signed-off-by: gatorsmile <gatorsmile@gmail.com>
## What changes were proposed in this pull request?
Legitimate stops of streams may actually cause an exception to be captured by stream execution, because the job throws a SparkException regarding job cancellation during a stop. This PR makes the stop more graceful by swallowing this cancellation error.
## How was this patch tested?
This is pretty hard to test. The existing tests should make sure that we're not swallowing other specific SparkExceptions. I've also run the `KafkaSourceStressForDontFailOnDataLossSuite`100 times, and it didn't fail, whereas it used to be flaky.
Closes#22478 from brkyvz/SPARK-25472.
Authored-by: Burak Yavuz <brkyvz@gmail.com>
Signed-off-by: Burak Yavuz <brkyvz@gmail.com>
## What changes were proposed in this pull request?
In the PR, I propose to add an overloaded method for `sampleBy` which accepts the first argument of the `Column` type. This will allow to sample by any complex columns as well as sampling by multiple columns. For example:
```Scala
spark.createDataFrame(Seq(("Bob", 17), ("Alice", 10), ("Nico", 8), ("Bob", 17),
("Alice", 10))).toDF("name", "age")
.stat
.sampleBy(struct($"name", $"age"), Map(Row("Alice", 10) -> 0.3, Row("Nico", 8) -> 1.0), 36L)
.show()
+-----+---+
| name|age|
+-----+---+
| Nico| 8|
|Alice| 10|
+-----+---+
```
## How was this patch tested?
Added new test for sampling by multiple columns for Scala and test for Java, Python to check that `sampleBy` is able to sample by `Column` type argument.
Closes#22365 from MaxGekk/sample-by-column.
Authored-by: Maxim Gekk <maxim.gekk@databricks.com>
Signed-off-by: hyukjinkwon <gurwls223@apache.org>
## What changes were proposed in this pull request?
The problem was cause by the PushProjectThroughUnion rule, which, when creating new Project for each child of Union, uses the same exprId for expressions of the same position. This is wrong because, for each child of Union, the expressions are all independent, and it can lead to a wrong result if other rules like FoldablePropagation kicks in, taking two different expressions as the same.
This fix is to create new expressions in the new Project for each child of Union.
## How was this patch tested?
Added UT.
Closes#22447 from maryannxue/push-project-thru-union-bug.
Authored-by: maryannxue <maryannxue@apache.org>
Signed-off-by: gatorsmile <gatorsmile@gmail.com>
## What changes were proposed in this pull request?
In ArrayContains, we currently cast the right hand side expression to match the element type of the left hand side Array. This may result in down casting and may return wrong result or questionable result.
Example :
```SQL
spark-sql> select array_contains(array(1), 1.34);
true
```
```SQL
spark-sql> select array_contains(array(1), 'foo');
null
```
We should safely coerce both left and right hand side expressions.
## How was this patch tested?
Added tests in DataFrameFunctionsSuite
Closes#22408 from dilipbiswal/SPARK-25417.
Authored-by: Dilip Biswal <dbiswal@us.ibm.com>
Signed-off-by: Wenchen Fan <wenchen@databricks.com>
## What changes were proposed in this pull request?
This PR proposes to respect `SessionConfigSupport` in SS datasources as well. Currently these are only respected in batch sources:
e06da95cd9/sql/core/src/main/scala/org/apache/spark/sql/DataFrameReader.scala (L198-L203)e06da95cd9/sql/core/src/main/scala/org/apache/spark/sql/DataFrameWriter.scala (L244-L249)
If a developer makes a datasource V2 that supports both structured streaming and batch jobs, batch jobs respect a specific configuration, let's say, URL to connect and fetch data (which end users might not be aware of); however, structured streaming ends up with not supporting this (and should explicitly be set into options).
## How was this patch tested?
Unit tests were added.
Closes#22462 from HyukjinKwon/SPARK-25460.
Authored-by: hyukjinkwon <gurwls223@apache.org>
Signed-off-by: Wenchen Fan <wenchen@databricks.com>
## What changes were proposed in this pull request?
This goes to revert sequential PRs based on some discussion and comments at https://github.com/apache/spark/pull/16677#issuecomment-422650759.
#22344#22330#22239#16677
## How was this patch tested?
Existing tests.
Closes#22481 from viirya/revert-SPARK-19355-1.
Authored-by: Liang-Chi Hsieh <viirya@gmail.com>
Signed-off-by: Wenchen Fan <wenchen@databricks.com>
## What changes were proposed in this pull request?
Refactor `FilterPushdownBenchmark` use `main` method. we can use 3 ways to run this test now:
1. bin/spark-submit --class org.apache.spark.sql.execution.benchmark.FilterPushdownBenchmark spark-sql_2.11-2.5.0-SNAPSHOT-tests.jar
2. build/sbt "sql/test:runMain org.apache.spark.sql.execution.benchmark.FilterPushdownBenchmark"
3. SPARK_GENERATE_BENCHMARK_FILES=1 build/sbt "sql/test:runMain org.apache.spark.sql.execution.benchmark.FilterPushdownBenchmark"
The method 2 and the method 3 do not need to compile the `spark-sql_*-tests.jar` package. So these two methods are mainly for developers to quickly do benchmark.
## How was this patch tested?
manual tests
Closes#22443 from wangyum/SPARK-25339.
Authored-by: Yuming Wang <yumwang@ebay.com>
Signed-off-by: Wenchen Fan <wenchen@databricks.com>
## What changes were proposed in this pull request?
This patch adds an "optimizer" prefix to nested schema pruning.
## How was this patch tested?
Should be covered by existing tests.
Closes#22475 from rxin/SPARK-4502.
Authored-by: Reynold Xin <rxin@databricks.com>
Signed-off-by: gatorsmile <gatorsmile@gmail.com>
## What changes were proposed in this pull request?
The PR proposes to return the data type of the operands as a result for the `div` operator. Before the PR, `bigint` is always returned. It introduces also a `spark.sql.legacy.integralDivide.returnBigint` config in order to let the users restore the legacy behavior.
## How was this patch tested?
added UTs
Closes#22465 from mgaido91/SPARK-25457.
Authored-by: Marco Gaido <marcogaido91@gmail.com>
Signed-off-by: Wenchen Fan <wenchen@databricks.com>
## What changes were proposed in this pull request?
This patch changes the config option `spark.sql.streaming.noDataMicroBatchesEnabled` to `spark.sql.streaming.noDataMicroBatches.enabled` to be more consistent with rest of the configs. Unfortunately there is one streaming config called `spark.sql.streaming.metricsEnabled`. For that one we should just use a fallback config and change it in a separate patch.
## How was this patch tested?
Made sure no other references to this config are in the code base:
```
> git grep "noDataMicro"
sql/catalyst/src/main/scala/org/apache/spark/sql/internal/SQLConf.scala: buildConf("spark.sql.streaming.noDataMicroBatches.enabled")
```
Closes#22476 from rxin/SPARK-24157.
Authored-by: Reynold Xin <rxin@databricks.com>
Signed-off-by: Reynold Xin <rxin@databricks.com>
## What changes were proposed in this pull request?
For self-join/self-union, Spark will produce a physical plan which has multiple `DataSourceV2ScanExec` instances referring to the same `ReadSupport` instance. In this case, the streaming source is indeed scanned multiple times, and the `numInputRows` metrics should be counted for each scan.
Actually we already have 2 test cases to verify the behavior:
1. `StreamingQuerySuite.input row calculation with same V2 source used twice in self-join`
2. `KafkaMicroBatchSourceSuiteBase.ensure stream-stream self-join generates only one offset in log and correct metrics`.
However, in these 2 tests, the expected result is different, which is super confusing. It turns out that, the first test doesn't trigger exchange reuse, so the source is scanned twice. The second test triggers exchange reuse, and the source is scanned only once.
This PR proposes to improve these 2 tests, to test with/without exchange reuse.
## How was this patch tested?
test only change
Closes#22402 from cloud-fan/bug.
Authored-by: Wenchen Fan <wenchen@databricks.com>
Signed-off-by: Wenchen Fan <wenchen@databricks.com>
## What changes were proposed in this pull request?
In SPARK-23711, `UnsafeProjection` supports fallback to an interpreted mode. Therefore, this pr fixed code to support the same fallback mode in `MutableProjection` based on `CodeGeneratorWithInterpretedFallback`.
## How was this patch tested?
Added tests in `CodeGeneratorWithInterpretedFallbackSuite`.
Closes#22355 from maropu/SPARK-25358.
Authored-by: Takeshi Yamamuro <yamamuro@apache.org>
Signed-off-by: Wenchen Fan <wenchen@databricks.com>
## What changes were proposed in this pull request?
To be more consistent with other statistics based configs.
## How was this patch tested?
N/A - straightforward rename of config option. Used `git grep` to make sure there are no mention of it.
Closes#22457 from rxin/SPARK-24626.
Authored-by: Reynold Xin <rxin@databricks.com>
Signed-off-by: gatorsmile <gatorsmile@gmail.com>
## What changes were proposed in this pull request?
`spark.sql.fromJsonForceNullableSchema` -> `spark.sql.function.fromJson.forceNullable`
## How was this patch tested?
Made sure there are no more references to `spark.sql.fromJsonForceNullableSchema`.
Closes#22459 from rxin/SPARK-23173.
Authored-by: Reynold Xin <rxin@databricks.com>
Signed-off-by: gatorsmile <gatorsmile@gmail.com>
PythonForeachWriterSuite was failing because RowQueue now needs to have a handle on a SparkEnv with a SerializerManager, so added a mock env with a serializer manager.
Also fixed a typo in the `finally` that was hiding the real exception.
Tested PythonForeachWriterSuite locally, full tests via jenkins.
Closes#22452 from squito/SPARK-25456.
Authored-by: Imran Rashid <irashid@cloudera.com>
Signed-off-by: Imran Rashid <irashid@cloudera.com>
## What changes were proposed in this pull request?
SPARK-22333 introduced a regression in the resolution of `CURRENT_DATE` and `CURRENT_TIMESTAMP`. Before that ticket, these 2 functions were resolved in a case insensitive way. After, this depends on the value of `spark.sql.caseSensitive`.
The PR restores the previous behavior and makes their resolution case insensitive anyhow. The PR takes over #21217, therefore it closes#21217 and credit for this patch should be given to jamesthomp.
## How was this patch tested?
added UT
Closes#22440 from mgaido91/SPARK-24151.
Lead-authored-by: James Thompson <jamesthomp@users.noreply.github.com>
Co-authored-by: Marco Gaido <marcogaido91@gmail.com>
Signed-off-by: gatorsmile <gatorsmile@gmail.com>
## What changes were proposed in this pull request?
This PR makes `GenArrayData.genCodeToCreateArrayData` method simple by using `ArrayData.createArrayData` method.
Before this PR, `genCodeToCreateArrayData` method was complicated
* Generated a temporary Java array to create `ArrayData`
* Had separate code generation path to assign values for `GenericArrayData` and `UnsafeArrayData`
After this PR, the method
* Directly generates `GenericArrayData` or `UnsafeArrayData` without a temporary array
* Has only code generation path to assign values
## How was this patch tested?
Existing UTs
Closes#22439 from kiszk/SPARK-25444.
Authored-by: Kazuaki Ishizaki <ishizaki@jp.ibm.com>
Signed-off-by: Takuya UESHIN <ueshin@databricks.com>
## What changes were proposed in this pull request?
The PR takes over #14036 and it introduces a new expression `IntegralDivide` in order to avoid the several unneded cast added previously.
In order to prove the performance gain, the following benchmark has been run:
```
test("Benchmark IntegralDivide") {
val r = new scala.util.Random(91)
val nData = 1000000
val testDataInt = (1 to nData).map(_ => (r.nextInt(), r.nextInt()))
val testDataLong = (1 to nData).map(_ => (r.nextLong(), r.nextLong()))
val testDataShort = (1 to nData).map(_ => (r.nextInt().toShort, r.nextInt().toShort))
// old code
val oldExprsInt = testDataInt.map(x =>
Cast(Divide(Cast(Literal(x._1), DoubleType), Cast(Literal(x._2), DoubleType)), LongType))
val oldExprsLong = testDataLong.map(x =>
Cast(Divide(Cast(Literal(x._1), DoubleType), Cast(Literal(x._2), DoubleType)), LongType))
val oldExprsShort = testDataShort.map(x =>
Cast(Divide(Cast(Literal(x._1), DoubleType), Cast(Literal(x._2), DoubleType)), LongType))
// new code
val newExprsInt = testDataInt.map(x => IntegralDivide(x._1, x._2))
val newExprsLong = testDataLong.map(x => IntegralDivide(x._1, x._2))
val newExprsShort = testDataShort.map(x => IntegralDivide(x._1, x._2))
Seq(("Long", "old", oldExprsLong),
("Long", "new", newExprsLong),
("Int", "old", oldExprsInt),
("Int", "new", newExprsShort),
("Short", "old", oldExprsShort),
("Short", "new", oldExprsShort)).foreach { case (dt, t, ds) =>
val start = System.nanoTime()
ds.foreach(e => e.eval(EmptyRow))
val endNoCodegen = System.nanoTime()
println(s"Running $nData op with $t code on $dt (no-codegen): ${(endNoCodegen - start) / 1000000} ms")
}
}
```
The results on my laptop are:
```
Running 1000000 op with old code on Long (no-codegen): 600 ms
Running 1000000 op with new code on Long (no-codegen): 112 ms
Running 1000000 op with old code on Int (no-codegen): 560 ms
Running 1000000 op with new code on Int (no-codegen): 135 ms
Running 1000000 op with old code on Short (no-codegen): 317 ms
Running 1000000 op with new code on Short (no-codegen): 153 ms
```
Showing a 2-5X improvement. The benchmark doesn't include code generation as it is pretty hard to test the performance there as for such simple operations the most of the time is spent in the code generation/compilation process.
## How was this patch tested?
added UTs
Closes#22395 from mgaido91/SPARK-16323.
Authored-by: Marco Gaido <marcogaido91@gmail.com>
Signed-off-by: Dongjoon Hyun <dongjoon@apache.org>
## What changes were proposed in this pull request?
Output `dataFilters` in `DataSourceScanExec.metadata`.
## How was this patch tested?
unit tests
Closes#22435 from wangyum/SPARK-25423.
Authored-by: Yuming Wang <yumwang@ebay.com>
Signed-off-by: Dongjoon Hyun <dongjoon@apache.org>
## What changes were proposed in this pull request?
There are some mistakes in examples of newly added functions. Also the format of the example results are not unified. We should fix them.
## How was this patch tested?
Manually executed the examples.
Closes#22437 from ueshin/issues/SPARK-25431/fix_examples_2.
Authored-by: Takuya UESHIN <ueshin@databricks.com>
Signed-off-by: hyukjinkwon <gurwls223@apache.org>
## What changes were proposed in this pull request?
Spark supports BloomFilter creation for ORC files. This PR aims to add test coverages to prevent accidental regressions like [SPARK-12417](https://issues.apache.org/jira/browse/SPARK-12417).
## How was this patch tested?
Pass the Jenkins with newly added test cases.
Closes#22418 from dongjoon-hyun/SPARK-25427.
Authored-by: Dongjoon Hyun <dongjoon@apache.org>
Signed-off-by: Wenchen Fan <wenchen@databricks.com>
What changes were proposed in this pull request
Updated the Migration guide for the behavior changes done in the JIRA issue SPARK-23425.
How was this patch tested?
Manually verified.
Closes#22396 from sujith71955/master_newtest.
Authored-by: s71955 <sujithchacko.2010@gmail.com>
Signed-off-by: Wenchen Fan <wenchen@databricks.com>
## What changes were proposed in this pull request?
Fixes TPCH DDL datatype of `customer.c_nationkey` from `STRING` to `BIGINT` according to spec and `nation.nationkey` in `TPCHQuerySuite.scala`. The rest of the keys are OK.
Note, this will lead to **non-comparable previous results** to new runs involving the customer table.
## How was this patch tested?
Manual tests
Author: npoggi <npmnpm@gmail.com>
Closes#22430 from npoggi/SPARK-25439_Fix-TPCH-customer-c_nationkey.
## What changes were proposed in this pull request?
This PR aims to fix three things in `FilterPushdownBenchmark`.
**1. Use the same memory assumption.**
The following configurations are used in ORC and Parquet.
- Memory buffer for writing
- parquet.block.size (default: 128MB)
- orc.stripe.size (default: 64MB)
- Compression chunk size
- parquet.page.size (default: 1MB)
- orc.compress.size (default: 256KB)
SPARK-24692 used 1MB, the default value of `parquet.page.size`, for `parquet.block.size` and `orc.stripe.size`. But, it missed to match `orc.compress.size`. So, the current benchmark shows the result from ORC with 256KB memory for compression and Parquet with 1MB. To compare correctly, we need to be consistent.
**2. Dictionary encoding should not be enforced for all cases.**
SPARK-24206 enforced dictionary encoding for all test cases. This PR recovers the default behavior in general and enforces dictionary encoding only in case of `prepareStringDictTable`.
**3. Generate test result on AWS r3.xlarge**
SPARK-24206 generated the result on AWS in order to reproduce and compare easily. This PR also aims to update the result on the same machine again in the same reason. Specifically, AWS r3.xlarge with Instance Store is used.
## How was this patch tested?
Manual. Enable the test cases and run `FilterPushdownBenchmark` on `AWS r3.xlarge`. It takes about 4 hours 15 minutes.
Closes#22427 from dongjoon-hyun/SPARK-25438.
Authored-by: Dongjoon Hyun <dongjoon@apache.org>
Signed-off-by: Dongjoon Hyun <dongjoon@apache.org>
## What changes were proposed in this pull request?
In the PR, I propose overriding session options by extra options in DataSource V2. Extra options are more specific and set via `.option()`, and should overwrite more generic session options. Entries from seconds map overwrites entries with the same key from the first map, for example:
```Scala
scala> Map("option" -> false) ++ Map("option" -> true)
res0: scala.collection.immutable.Map[String,Boolean] = Map(option -> true)
```
## How was this patch tested?
Added a test for checking which option is propagated to a data source in `load()`.
Closes#22413 from MaxGekk/session-options.
Lead-authored-by: Maxim Gekk <maxim.gekk@databricks.com>
Co-authored-by: Dongjoon Hyun <dongjoon@apache.org>
Co-authored-by: Maxim Gekk <max.gekk@gmail.com>
Signed-off-by: Dongjoon Hyun <dongjoon@apache.org>
## What changes were proposed in this pull request?
In the dev list, we can still discuss whether the next version is 2.5.0 or 3.0.0. Let us first bump the master branch version to `2.5.0-SNAPSHOT`.
## How was this patch tested?
N/A
Closes#22426 from gatorsmile/bumpVersionMaster.
Authored-by: gatorsmile <gatorsmile@gmail.com>
Signed-off-by: gatorsmile <gatorsmile@gmail.com>
## What changes were proposed in this pull request?
This pr removed the duplicate fallback logic in `UnsafeProjection`.
This pr comes from #22355.
## How was this patch tested?
Added tests in `CodeGeneratorWithInterpretedFallbackSuite`.
Closes#22417 from maropu/SPARK-25426.
Authored-by: Takeshi Yamamuro <yamamuro@apache.org>
Signed-off-by: gatorsmile <gatorsmile@gmail.com>
## What changes were proposed in this pull request?
There are some mistakes in examples of newly added functions. Also the format of the example results are not unified. We should fix and unify them.
## How was this patch tested?
Manually executed the examples.
Closes#22421 from ueshin/issues/SPARK-25431/fix_examples.
Authored-by: Takuya UESHIN <ueshin@databricks.com>
Signed-off-by: gatorsmile <gatorsmile@gmail.com>
## What changes were proposed in this pull request?
When Hive support enabled, Hive catalog puts extra storage properties into table metadata even for DataSource tables, but we should not have them.
## How was this patch tested?
Modified a test.
Closes#22410 from ueshin/issues/SPARK-25418/hive_metadata.
Authored-by: Takuya UESHIN <ueshin@databricks.com>
Signed-off-by: gatorsmile <gatorsmile@gmail.com>
## What changes were proposed in this pull request?
This PR ensures to call `super.afterAll()` in `override afterAll()` method for test suites.
* Some suites did not call `super.afterAll()`
* Some suites may call `super.afterAll()` only under certain condition
* Others never call `super.afterAll()`.
This PR also ensures to call `super.beforeAll()` in `override beforeAll()` for test suites.
## How was this patch tested?
Existing UTs
Closes#22337 from kiszk/SPARK-25338.
Authored-by: Kazuaki Ishizaki <ishizaki@jp.ibm.com>
Signed-off-by: Dongjoon Hyun <dongjoon@apache.org>
(Link to Jira: https://issues.apache.org/jira/browse/SPARK-25406)
## What changes were proposed in this pull request?
The current use of `withSQLConf` in `ParquetSchemaPruningSuite.scala` is incorrect. The desired configuration settings are not being set when running the test cases.
This PR fixes that defective usage and addresses the test failures that were previously masked by that defect.
## How was this patch tested?
I added code to relevant test cases to print the expected SQL configuration settings and found that the settings were not being set as expected. When I changed the order of calls to `test` and `withSQLConf` I found that the configuration settings were being set as expected.
Closes#22394 from mallman/spark-25406-fix_broken_schema_pruning_tests.
Authored-by: Michael Allman <msa@allman.ms>
Signed-off-by: DB Tsai <d_tsai@apple.com>
## What changes were proposed in this pull request?
This follow-up patch addresses [the review comment](https://github.com/apache/spark/pull/22344/files#r217070658) by adding a helper method to simplify code and fixing style issue.
## How was this patch tested?
Existing unit tests.
Author: Liang-Chi Hsieh <viirya@gmail.com>
Closes#22409 from viirya/SPARK-25352-followup.
## What changes were proposed in this pull request?
In RuleExecutor, after applying a rule, if the plan has changed, the before and after plan will be logged using level "trace". At times, however, such information can be very helpful for debugging. Hence, making the log level configurable in SQLConf would allow users to turn on the plan change log independently and save the trouble of tweaking log4j settings. Meanwhile, filtering plan change log for specific rules can also be very useful.
So this PR adds two SQL configurations:
1. spark.sql.optimizer.planChangeLog.level - set a specific log level for logging plan changes after a rule is applied.
2. spark.sql.optimizer.planChangeLog.rules - enable plan change logging only for a set of specified rules, separated by commas.
## How was this patch tested?
Added UT.
Closes#22406 from maryannxue/spark-25415.
Authored-by: maryannxue <maryannxue@apache.org>
Signed-off-by: gatorsmile <gatorsmile@gmail.com>
## What changes were proposed in this pull request?
Field metadata removed from SparkPlanInfo in #18600 . Corresponding, many meta data was also removed from event SparkListenerSQLExecutionStart in Spark event log. If we want to analyze event log to get all input paths, we couldn't get them. Instead, simpleString of SparkPlanInfo JSON only display 100 characters, it won't help.
Before 2.3, the fragment of SparkListenerSQLExecutionStart in event log looks like below (It contains the metadata field which has the intact information):
>{"Event":"org.apache.spark.sql.execution.ui.SparkListenerSQLExecutionStart", Location: InMemoryFileIndex[hdfs://cluster1/sys/edw/test1/test2/test3/test4..., "metadata": {"Location": "InMemoryFileIndex[hdfs://cluster1/sys/edw/test1/test2/test3/test4/test5/snapshot/dt=20180904]","ReadSchema":"struct<snpsht_start_dt:date,snpsht_end_dt:date,am_ntlogin_name:string,am_first_name:string,am_last_name:string,isg_name:string,CRE_DATE:date,CRE_USER:string,UPD_DATE:timestamp,UPD_USER:string>"}
After #18600, metadata field was removed.
>{"Event":"org.apache.spark.sql.execution.ui.SparkListenerSQLExecutionStart", Location: InMemoryFileIndex[hdfs://cluster1/sys/edw/test1/test2/test3/test4...,
So I add this field back to SparkPlanInfo class. Then it will log out the meta data to event log. Intact information in event log is very useful for offline job analysis.
## How was this patch tested?
Unit test
Closes#22353 from LantaoJin/SPARK-25357.
Authored-by: LantaoJin <jinlantao@gmail.com>
Signed-off-by: Wenchen Fan <wenchen@databricks.com>
## What changes were proposed in this pull request?
The PR fixes NPE in `UnivocityParser` caused by malformed CSV input. In some cases, `uniVocity` parser can return `null` for bad input. In the PR, I propose to check result of parsing and not propagate NPE to upper layers.
## How was this patch tested?
I added a test which reproduce the issue and tested by `CSVSuite`.
Closes#22374 from MaxGekk/npe-on-bad-csv.
Lead-authored-by: Maxim Gekk <max.gekk@gmail.com>
Co-authored-by: Maxim Gekk <maxim.gekk@databricks.com>
Signed-off-by: Wenchen Fan <wenchen@databricks.com>
## What changes were proposed in this pull request?
Schema pruning doesn't work if nested column is used in where clause.
For example,
```
sql("select name.first from contacts where name.first = 'David'")
== Physical Plan ==
*(1) Project [name#19.first AS first#40]
+- *(1) Filter (isnotnull(name#19) && (name#19.first = David))
+- *(1) FileScan parquet [name#19] Batched: false, Format: Parquet, PartitionFilters: [],
PushedFilters: [IsNotNull(name)], ReadSchema: struct<name:struct<first:string,middle:string,last:string>>
```
In above query plan, the scan node reads the entire schema of `name` column.
This issue is reported by:
https://github.com/apache/spark/pull/21320#issuecomment-419290197
The cause is that we infer a root field from expression `IsNotNull(name)`. However, for such expression, we don't really use the nested fields of this root field, so we can ignore the unnecessary nested fields.
## How was this patch tested?
Unit tests.
Closes#22357 from viirya/SPARK-25363.
Authored-by: Liang-Chi Hsieh <viirya@gmail.com>
Signed-off-by: DB Tsai <d_tsai@apple.com>
## What changes were proposed in this pull request?
We have optimization on global limit to evenly distribute limit rows across all partitions. This optimization doesn't work for ordered results.
For a query ending with sort + limit, in most cases it is performed by `TakeOrderedAndProjectExec`.
But if limit number is bigger than `SQLConf.TOP_K_SORT_FALLBACK_THRESHOLD`, global limit will be used. At this moment, we need to do ordered global limit.
## How was this patch tested?
Unit tests.
Closes#22344 from viirya/SPARK-25352.
Authored-by: Liang-Chi Hsieh <viirya@gmail.com>
Signed-off-by: Wenchen Fan <wenchen@databricks.com>
## What changes were proposed in this pull request?
This PR is to fix the null handling in BooleanSimplification. In the rule BooleanSimplification, there are two cases that do not properly handle null values. The optimization is not right if either side is null. This PR is to fix them.
## How was this patch tested?
Added test cases
Closes#22390 from gatorsmile/fixBooleanSimplification.
Authored-by: gatorsmile <gatorsmile@gmail.com>
Signed-off-by: Wenchen Fan <wenchen@databricks.com>
## What changes were proposed in this pull request?
The leftover state from running a continuous processing streaming job should not affect later microbatch execution jobs. If a continuous processing job runs and the same thread gets reused for a microbatch execution job in the same environment, the microbatch job could get wrong answers because it can attempt to load the wrong version of the state.
## How was this patch tested?
New and existing unit tests
Closes#22386 from mukulmurthy/25399-streamthread.
Authored-by: Mukul Murthy <mukul.murthy@gmail.com>
Signed-off-by: Tathagata Das <tathagata.das1565@gmail.com>
## What changes were proposed in this pull request?
Correct some comparisons between unrelated types to what they seem to… have been trying to do
## How was this patch tested?
Existing tests.
Closes#22384 from srowen/SPARK-25398.
Authored-by: Sean Owen <sean.owen@databricks.com>
Signed-off-by: Sean Owen <sean.owen@databricks.com>
## What changes were proposed in this pull request?
Like `INSERT OVERWRITE DIRECTORY USING` syntax, `INSERT OVERWRITE DIRECTORY STORED AS` should not generate files with duplicate fields because Spark cannot read those files back.
**INSERT OVERWRITE DIRECTORY USING**
```scala
scala> sql("INSERT OVERWRITE DIRECTORY 'file:///tmp/parquet' USING parquet SELECT 'id', 'id2' id")
... ERROR InsertIntoDataSourceDirCommand: Failed to write to directory ...
org.apache.spark.sql.AnalysisException: Found duplicate column(s) when inserting into file:/tmp/parquet: `id`;
```
**INSERT OVERWRITE DIRECTORY STORED AS**
```scala
scala> sql("INSERT OVERWRITE DIRECTORY 'file:///tmp/parquet' STORED AS parquet SELECT 'id', 'id2' id")
// It generates corrupted files
scala> spark.read.parquet("/tmp/parquet").show
18/09/09 22:09:57 WARN DataSource: Found duplicate column(s) in the data schema and the partition schema: `id`;
```
## How was this patch tested?
Pass the Jenkins with newly added test cases.
Closes#22378 from dongjoon-hyun/SPARK-25389.
Authored-by: Dongjoon Hyun <dongjoon@apache.org>
Signed-off-by: Dongjoon Hyun <dongjoon@apache.org>
## What changes were proposed in this pull request?
In the PR, I propose new CSV option `emptyValue` and an update in the SQL Migration Guide which describes how to revert previous behavior when empty strings were not written at all. Since Spark 2.4, empty strings are saved as `""` to distinguish them from saved `null`s.
Closes#22234Closes#22367
## How was this patch tested?
It was tested by `CSVSuite` and new tests added in the PR #22234Closes#22389 from MaxGekk/csv-empty-value-master.
Lead-authored-by: Mario Molina <mmolimar@gmail.com>
Co-authored-by: Maxim Gekk <maxim.gekk@databricks.com>
Signed-off-by: hyukjinkwon <gurwls223@apache.org>
## What changes were proposed in this pull request?
It turns out it's a bug that a `DataSourceV2ScanExec` instance may be referred to in the execution plan multiple times. This bug is fixed by https://github.com/apache/spark/pull/22284 and now we have corrected SQL metrics for batch queries.
Thus we don't need the hack in `ProgressReporter` anymore, which fixes the same metrics problem for streaming queries.
## How was this patch tested?
existing tests
Closes#22380 from cloud-fan/followup.
Authored-by: Wenchen Fan <wenchen@databricks.com>
Signed-off-by: Wenchen Fan <wenchen@databricks.com>
## What changes were proposed in this pull request?
SPARK-21281 introduced a check for the inputs of `CreateStructLike` to be non-empty. This means that `struct()`, which was previously considered valid, now throws an Exception. This behavior change was introduced in 2.3.0. The change may break users' application on upgrade and it causes `VectorAssembler` to fail when an empty `inputCols` is defined.
The PR removes the added check making `struct()` valid again.
## How was this patch tested?
added UT
Closes#22373 from mgaido91/SPARK-25371.
Authored-by: Marco Gaido <marcogaido91@gmail.com>
Signed-off-by: Wenchen Fan <wenchen@databricks.com>
## What changes were proposed in this pull request?
In the Planner, we collect the placeholder which need to be substituted in the query execution plan and once we plan them, we substitute the placeholder with the effective plan.
In this second phase, we rely on the `==` comparison, ie. the `equals` method. This means that if two placeholder plans - which are different instances - have the same attributes (so that they are equal, according to the equal method) they are both substituted with their corresponding new physical plans. So, in such a situation, the first time we substitute both them with the first of the 2 new generated plan and the second time we substitute nothing.
This is usually of no harm for the execution of the query itself, as the 2 plans are identical. But since they are the same instance, now, the local variables are shared (which is unexpected). This causes issues for the metrics collected, as the same node is executed 2 times, so the metrics are accumulated 2 times, wrongly.
The PR proposes to use the `eq` method in checking which placeholder needs to be substituted,; thus in the previous situation, actually both the two different physical nodes which are created (one for each time the logical plan appears in the query plan) are used and the metrics are collected properly for each of them.
## How was this patch tested?
added UT
Closes#22284 from mgaido91/SPARK-25278.
Authored-by: Marco Gaido <marcogaido91@gmail.com>
Signed-off-by: Wenchen Fan <wenchen@databricks.com>
## What changes were proposed in this pull request?
Add the version number for the new APIs.
## How was this patch tested?
N/A
Closes#22377 from gatorsmile/followup24849.
Authored-by: gatorsmile <gatorsmile@gmail.com>
Signed-off-by: Wenchen Fan <wenchen@databricks.com>
## What changes were proposed in this pull request?
This PR is to solve the CodeGen code generated by fast hash, and there is no need to apply for a block of memory for every new entry, because unsafeRow's memory can be reused.
## How was this patch tested?
the existed test cases.
Closes#21968 from heary-cao/updateNewMemory.
Authored-by: caoxuewen <cao.xuewen@zte.com.cn>
Signed-off-by: Wenchen Fan <wenchen@databricks.com>
## What changes were proposed in this pull request?
How to reproduce:
```scala
spark.sql("CREATE TABLE tbl(id long)")
spark.sql("INSERT OVERWRITE TABLE tbl VALUES 4")
spark.sql("CREATE VIEW view1 AS SELECT id FROM tbl")
spark.sql(s"INSERT OVERWRITE LOCAL DIRECTORY '/tmp/spark/parquet' " +
"STORED AS PARQUET SELECT ID FROM view1")
spark.read.parquet("/tmp/spark/parquet").schema
scala> spark.read.parquet("/tmp/spark/parquet").schema
res10: org.apache.spark.sql.types.StructType = StructType(StructField(id,LongType,true))
```
The schema should be `StructType(StructField(ID,LongType,true))` as we `SELECT ID FROM view1`.
This pr fix this issue.
## How was this patch tested?
unit tests
Closes#22359 from wangyum/SPARK-25313-FOLLOW-UP.
Authored-by: Yuming Wang <yumwang@ebay.com>
Signed-off-by: Wenchen Fan <wenchen@databricks.com>
## What changes were proposed in this pull request?
Apache Spark doesn't create Hive table with duplicated fields in both case-sensitive and case-insensitive mode. However, if Spark creates ORC files in case-sensitive mode first and create Hive table on that location, where it's created. In this situation, field resolution should fail in case-insensitive mode. Otherwise, we don't know which columns will be returned or filtered. Previously, SPARK-25132 fixed the same issue in Parquet.
Here is a simple example:
```
val data = spark.range(5).selectExpr("id as a", "id * 2 as A")
spark.conf.set("spark.sql.caseSensitive", true)
data.write.format("orc").mode("overwrite").save("/user/hive/warehouse/orc_data")
sql("CREATE TABLE orc_data_source (A LONG) USING orc LOCATION '/user/hive/warehouse/orc_data'")
spark.conf.set("spark.sql.caseSensitive", false)
sql("select A from orc_data_source").show
+---+
| A|
+---+
| 3|
| 2|
| 4|
| 1|
| 0|
+---+
```
See #22148 for more details about parquet data source reader.
## How was this patch tested?
Unit tests added.
Closes#22262 from seancxmao/SPARK-25175.
Authored-by: seancxmao <seancxmao@gmail.com>
Signed-off-by: Dongjoon Hyun <dongjoon@apache.org>
## What changes were proposed in this pull request?
How to reproduce:
```scala
val df1 = spark.createDataFrame(Seq(
(1, 1)
)).toDF("a", "b").withColumn("c", lit(null).cast("int"))
val df2 = df1.union(df1).withColumn("d", spark_partition_id).filter($"c".isNotNull)
df2.show
+---+---+----+---+
| a| b| c| d|
+---+---+----+---+
| 1| 1|null| 0|
| 1| 1|null| 1|
+---+---+----+---+
```
`filter($"c".isNotNull)` was transformed to `(null <=> c#10)` before https://github.com/apache/spark/pull/19201, but it is transformed to `(c#10 = null)` since https://github.com/apache/spark/pull/20155. This pr revert it to `(null <=> c#10)` to fix this issue.
## How was this patch tested?
unit tests
Closes#22368 from wangyum/SPARK-25368.
Authored-by: Yuming Wang <yumwang@ebay.com>
Signed-off-by: gatorsmile <gatorsmile@gmail.com>
## What changes were proposed in this pull request?
When running TPC-DS benchmarks on 2.4 release, npoggi and winglungngai saw more than 10% performance regression on the following queries: q67, q24a and q24b. After we applying the PR https://github.com/apache/spark/pull/22338, the performance regression still exists. If we revert the changes in https://github.com/apache/spark/pull/19222, npoggi and winglungngai found the performance regression was resolved. Thus, this PR is to revert the related changes for unblocking the 2.4 release.
In the future release, we still can continue the investigation and find out the root cause of the regression.
## How was this patch tested?
The existing test cases
Closes#22361 from gatorsmile/revertMemoryBlock.
Authored-by: gatorsmile <gatorsmile@gmail.com>
Signed-off-by: Wenchen Fan <wenchen@databricks.com>
## What changes were proposed in this pull request?
Add new optimization rule to eliminate unnecessary shuffling by flipping adjacent Window expressions.
## How was this patch tested?
Tested with unit tests, integration tests, and manual tests.
Closes#17899 from ptkool/adjacent_window_optimization.
Authored-by: ptkool <michael.styles@shopify.com>
Signed-off-by: gatorsmile <gatorsmile@gmail.com>
## What changes were proposed in this pull request?
At Spark 2.0.0, SPARK-14335 adds some [commented-out test coverages](https://github.com/apache/spark/pull/12117/files#diff-dd4b39a56fac28b1ced6184453a47358R177
). This PR enables them because it's supported since 2.0.0.
## How was this patch tested?
Pass the Jenkins with re-enabled test coverage.
Closes#22363 from dongjoon-hyun/SPARK-25375.
Authored-by: Dongjoon Hyun <dongjoon@apache.org>
Signed-off-by: gatorsmile <gatorsmile@gmail.com>
## What changes were proposed in this pull request?
This took me a while to debug and find out. Looks we better at least leave a debug log that SQL text for a view will be used.
Here's how I got there:
**Hive:**
```
CREATE TABLE emp AS SELECT 'user' AS name, 'address' as address;
CREATE DATABASE d100;
CREATE FUNCTION d100.udf100 AS 'org.apache.hadoop.hive.ql.udf.generic.GenericUDFUpper';
CREATE VIEW testview AS SELECT d100.udf100(name) FROM default.emp;
```
**Spark:**
```
sql("SELECT * FROM testview").show()
```
```
scala> sql("SELECT * FROM testview").show()
org.apache.spark.sql.AnalysisException: Undefined function: 'd100.udf100'. This function is neither a registered temporary function nor a permanent function registered in the database 'default'.; line 1 pos 7
```
Under the hood, it actually makes sense since the view is defined as `SELECT d100.udf100(name) FROM default.emp;` and Hive API:
```
org.apache.hadoop.hive.ql.metadata.Table.getViewExpandedText()
```
This returns a wrongly qualified SQL string for the view as below:
```
SELECT `d100.udf100`(`emp`.`name`) FROM `default`.`emp`
```
which works fine in Hive but not in Spark.
## How was this patch tested?
Manually:
```
18/09/06 19:32:48 DEBUG HiveSessionCatalog: 'SELECT `d100.udf100`(`emp`.`name`) FROM `default`.`emp`' will be used for the view(testview).
```
Closes#22351 from HyukjinKwon/minor-debug.
Authored-by: hyukjinkwon <gurwls223@apache.org>
Signed-off-by: hyukjinkwon <gurwls223@apache.org>
## What changes were proposed in this pull request?
Before Apache Spark 2.3, table properties were ignored when writing data to a hive table(created with STORED AS PARQUET/ORC syntax), because the compression configurations were not passed to the FileFormatWriter in hadoopConf. Then it was fixed in #20087. But actually for CTAS with USING PARQUET/ORC syntax, table properties were ignored too when convertMastore, so the test case for CTAS not supported.
Now it has been fixed in #20522 , the test case should be enabled too.
## How was this patch tested?
This only re-enables the test cases of previous PR.
Closes#22302 from fjh100456/compressionCodec.
Authored-by: fjh100456 <fu.jinhua6@zte.com.cn>
Signed-off-by: Dongjoon Hyun <dongjoon@apache.org>
## What changes were proposed in this pull request?
Add test cases for fromString
## How was this patch tested?
N/A
Closes#22345 from gatorsmile/addTest.
Authored-by: Xiao Li <gatorsmile@gmail.com>
Signed-off-by: gatorsmile <gatorsmile@gmail.com>
## What changes were proposed in this pull request?
In SharedSparkSession and TestHive, we need to disable the rule ConvertToLocalRelation for better test case coverage.
## How was this patch tested?
Identify the failures after excluding "ConvertToLocalRelation" rule.
Closes#22270 from dilipbiswal/SPARK-25267-final.
Authored-by: Dilip Biswal <dbiswal@us.ibm.com>
Signed-off-by: gatorsmile <gatorsmile@gmail.com>
## What changes were proposed in this pull request?
This pr removed the method `updateBytesReadWithFileSize` in `FileScanRDD` because it computes input metrics by file size supported in Hadoop 2.5 and earlier. The current Spark does not support the versions, so it causes wrong input metric numbers.
This is rework from #22232.
Closes#22232
## How was this patch tested?
Added tests in `FileBasedDataSourceSuite`.
Closes#22324 from maropu/pr22232-2.
Lead-authored-by: dujunling <dujunling@huawei.com>
Co-authored-by: Takeshi Yamamuro <yamamuro@apache.org>
Signed-off-by: Sean Owen <sean.owen@databricks.com>
## What changes were proposed in this pull request?
This is a follow-up pr of #22200.
When casting to decimal type, if `Cast.canNullSafeCastToDecimal()`, overflow won't happen, so we don't need to check the result of `Decimal.changePrecision()`.
## How was this patch tested?
Existing tests.
Closes#22352 from ueshin/issues/SPARK-25208/reduce_code_size.
Authored-by: Takuya UESHIN <ueshin@databricks.com>
Signed-off-by: Wenchen Fan <wenchen@databricks.com>
This is not a perfect solution. It is designed to minimize complexity on the basis of solving problems.
It is effective for English, Chinese characters, Japanese, Korean and so on.
```scala
before:
+---+---------------------------+-------------+
|id |中国 |s2 |
+---+---------------------------+-------------+
|1 |ab |[a] |
|2 |null |[中国, abc] |
|3 |ab1 |[hello world]|
|4 |か行 きゃ(kya) きゅ(kyu) きょ(kyo) |[“中国] |
|5 |中国(你好)a |[“中(国), 312] |
|6 |中国山(东)服务区 |[“中(国)] |
|7 |中国山东服务区 |[中(国)] |
|8 | |[中国] |
+---+---------------------------+-------------+
after:
+---+-----------------------------------+----------------+
|id |中国 |s2 |
+---+-----------------------------------+----------------+
|1 |ab |[a] |
|2 |null |[中国, abc] |
|3 |ab1 |[hello world] |
|4 |か行 きゃ(kya) きゅ(kyu) きょ(kyo) |[“中国] |
|5 |中国(你好)a |[“中(国), 312]|
|6 |中国山(东)服务区 |[“中(国)] |
|7 |中国山东服务区 |[中(国)] |
|8 | |[中国] |
+---+-----------------------------------+----------------+
```
## What changes were proposed in this pull request?
When there are wide characters such as Chinese characters or Japanese characters in the data, the show method has a alignment problem.
Try to fix this problem.
## How was this patch tested?
(Please explain how this patch was tested. E.g. unit tests, integration tests, manual tests)
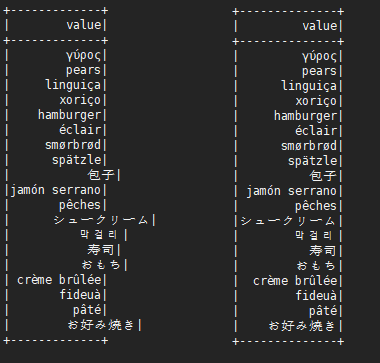
Please review http://spark.apache.org/contributing.html before opening a pull request.
Closes#22048 from xuejianbest/master.
Authored-by: xuejianbest <384329882@qq.com>
Signed-off-by: Sean Owen <sean.owen@databricks.com>
## What changes were proposed in this pull request?
In the PR, I propose to extended `to_json` and support any types as element types of input arrays. It should allow converting arrays of primitive types and arrays of arrays. For example:
```
select to_json(array('1','2','3'))
> ["1","2","3"]
select to_json(array(array(1,2,3),array(4)))
> [[1,2,3],[4]]
```
## How was this patch tested?
Added a couple sql tests for arrays of primitive type and of arrays. Also I added round trip test `from_json` -> `to_json`.
Closes#22226 from MaxGekk/to_json-array.
Authored-by: Maxim Gekk <maxim.gekk@databricks.com>
Signed-off-by: hyukjinkwon <gurwls223@apache.org>
## What changes were proposed in this pull request?
`HiveExternalCatalogVersionsSuite` Scala-2.12 test has been failing due to class path issue. It is marked as `ABORTED` because it fails at `beforeAll` during data population stage.
- https://amplab.cs.berkeley.edu/jenkins/view/Spark%20QA%20Test%20(Dashboard)/job/spark-master-test-maven-hadoop-2.7-ubuntu-scala-2.12/
```
org.apache.spark.sql.hive.HiveExternalCatalogVersionsSuite *** ABORTED ***
Exception encountered when invoking run on a nested suite - spark-submit returned with exit code 1.
```
The root cause of the failure is that `runSparkSubmit` mixes 2.4.0-SNAPSHOT classes and old Spark (2.1.3/2.2.2/2.3.1) together during `spark-submit`. This PR aims to provide `non-test` mode execution mode to `runSparkSubmit` by removing the followings.
- SPARK_TESTING
- SPARK_SQL_TESTING
- SPARK_PREPEND_CLASSES
- SPARK_DIST_CLASSPATH
Previously, in the class path, new Spark classes are behind the old Spark classes. So, new ones are unseen. However, Spark 2.4.0 reveals this bug due to the recent data source class changes.
## How was this patch tested?
Manual test. After merging, it will be tested via Jenkins.
```scala
$ dev/change-scala-version.sh 2.12
$ build/mvn -DskipTests -Phive -Pscala-2.12 clean package
$ build/mvn -Phive -Pscala-2.12 -Dtest=none -DwildcardSuites=org.apache.spark.sql.hive.HiveExternalCatalogVersionsSuite test
...
HiveExternalCatalogVersionsSuite:
- backward compatibility
...
Tests: succeeded 1, failed 0, canceled 0, ignored 0, pending 0
All tests passed.
```
Closes#22340 from dongjoon-hyun/SPARK-25337.
Authored-by: Dongjoon Hyun <dongjoon@apache.org>
Signed-off-by: Sean Owen <sean.owen@databricks.com>
## What changes were proposed in this pull request?
Let's see the follow example:
```
val location = "/tmp/t"
val df = spark.range(10).toDF("id")
df.write.format("parquet").saveAsTable("tbl")
spark.sql("CREATE VIEW view1 AS SELECT id FROM tbl")
spark.sql(s"CREATE TABLE tbl2(ID long) USING parquet location $location")
spark.sql("INSERT OVERWRITE TABLE tbl2 SELECT ID FROM view1")
println(spark.read.parquet(location).schema)
spark.table("tbl2").show()
```
The output column name in schema will be `id` instead of `ID`, thus the last query shows nothing from `tbl2`.
By enabling the debug message we can see that the output naming is changed from `ID` to `id`, and then the `outputColumns` in `InsertIntoHadoopFsRelationCommand` is changed in `RemoveRedundantAliases`.


**To guarantee correctness**, we should change the output columns from `Seq[Attribute]` to `Seq[String]` to avoid its names being replaced by optimizer.
I will fix project elimination related rules in https://github.com/apache/spark/pull/22311 after this one.
## How was this patch tested?
Unit test.
Closes#22320 from gengliangwang/fixOutputSchema.
Authored-by: Gengliang Wang <gengliang.wang@databricks.com>
Signed-off-by: Wenchen Fan <wenchen@databricks.com>
## What changes were proposed in this pull request?
An alternative fix for https://github.com/apache/spark/pull/21698
When Spark rerun tasks for an RDD, there are 3 different behaviors:
1. determinate. Always return the same result with same order when rerun.
2. unordered. Returns same data set in random order when rerun.
3. indeterminate. Returns different result when rerun.
Normally Spark doesn't need to care about it. Spark runs stages one by one, when a task is failed, just rerun it. Although the rerun task may return a different result, users will not be surprised.
However, Spark may rerun a finished stage when seeing fetch failures. When this happens, Spark needs to rerun all the tasks of all the succeeding stages if the RDD output is indeterminate, because the input of the succeeding stages has been changed.
If the RDD output is determinate, we only need to rerun the failed tasks of the succeeding stages, because the input doesn't change.
If the RDD output is unordered, it's same as determinate, because shuffle partitioner is always deterministic(round-robin partitioner is not a shuffle partitioner that extends `org.apache.spark.Partitioner`), so the reducers will still get the same input data set.
This PR fixed the failure handling for `repartition`, to avoid correctness issues.
For `repartition`, it applies a stateful map function to generate a round-robin id, which is order sensitive and makes the RDD's output indeterminate. When the stage contains `repartition` reruns, we must also rerun all the tasks of all the succeeding stages.
**future improvement:**
1. Currently we can't rollback and rerun a shuffle map stage, and just fail. We should fix it later. https://issues.apache.org/jira/browse/SPARK-25341
2. Currently we can't rollback and rerun a result stage, and just fail. We should fix it later. https://issues.apache.org/jira/browse/SPARK-25342
3. We should provide public API to allow users to tag the random level of the RDD's computing function.
## How is this pull request tested?
a new test case
Closes#22112 from cloud-fan/repartition.
Lead-authored-by: Wenchen Fan <wenchen@databricks.com>
Co-authored-by: Xingbo Jiang <xingbo.jiang@databricks.com>
Signed-off-by: Xiao Li <gatorsmile@gmail.com>
## What changes were proposed in this pull request?
This is a follow-up of #22313 and aim to ignore the micro benchmark test which takes over 2 minutes in Jenkins.
- https://amplab.cs.berkeley.edu/jenkins/view/Spark%20QA%20Test%20(Dashboard)/job/spark-master-test-sbt-hadoop-2.6/4939/consoleFull
## How was this patch tested?
The test case should be ignored in Jenkins.
```
[info] FilterPushdownBenchmark:
...
[info] - Pushdown benchmark with many filters !!! IGNORED !!!
```
Closes#22336 from dongjoon-hyun/SPARK-25306-2.
Authored-by: Dongjoon Hyun <dongjoon@apache.org>
Signed-off-by: Xiao Li <gatorsmile@gmail.com>
## What changes were proposed in this pull request?
This is a followup of https://github.com/apache/spark/pull/22259 .
Scala case class has a wide surface: apply, unapply, accessors, copy, etc.
In https://github.com/apache/spark/pull/22259 , we change the type of `UserDefinedFunction.inputTypes` from `Option[Seq[DataType]]` to `Option[Seq[Schema]]`. This breaks backward compatibility.
This PR changes the type back, and use a `var` to keep the new nullable info.
## How was this patch tested?
N/A
Closes#22319 from cloud-fan/revert.
Authored-by: Wenchen Fan <wenchen@databricks.com>
Signed-off-by: Wenchen Fan <wenchen@databricks.com>
## What changes were proposed in this pull request?
Revert SPARK-24863 (#21819) and SPARK-24748 (#21721) as per discussion in #21721. We will revisit them when the data source v2 APIs are out.
## How was this patch tested?
Jenkins
Closes#22334 from zsxwing/revert-SPARK-24863-SPARK-24748.
Authored-by: Shixiong Zhu <zsxwing@gmail.com>
Signed-off-by: Wenchen Fan <wenchen@databricks.com>
## What changes were proposed in this pull request?
In both ORC data sources, `createFilter` function has exponential time complexity due to its skewed filter tree generation. This PR aims to improve it by using new `buildTree` function.
**REPRODUCE**
```scala
// Create and read 1 row table with 1000 columns
sql("set spark.sql.orc.filterPushdown=true")
val selectExpr = (1 to 1000).map(i => s"id c$i")
spark.range(1).selectExpr(selectExpr: _*).write.mode("overwrite").orc("/tmp/orc")
print(s"With 0 filters, ")
spark.time(spark.read.orc("/tmp/orc").count)
// Increase the number of filters
(20 to 30).foreach { width =>
val whereExpr = (1 to width).map(i => s"c$i is not null").mkString(" and ")
print(s"With $width filters, ")
spark.time(spark.read.orc("/tmp/orc").where(whereExpr).count)
}
```
**RESULT**
```scala
With 0 filters, Time taken: 653 ms
With 20 filters, Time taken: 962 ms
With 21 filters, Time taken: 1282 ms
With 22 filters, Time taken: 1982 ms
With 23 filters, Time taken: 3855 ms
With 24 filters, Time taken: 6719 ms
With 25 filters, Time taken: 12669 ms
With 26 filters, Time taken: 25032 ms
With 27 filters, Time taken: 49585 ms
With 28 filters, Time taken: 98980 ms // over 1 min 38 seconds
With 29 filters, Time taken: 198368 ms // over 3 mins
With 30 filters, Time taken: 393744 ms // over 6 mins
```
**AFTER THIS PR**
```scala
With 0 filters, Time taken: 774 ms
With 20 filters, Time taken: 601 ms
With 21 filters, Time taken: 399 ms
With 22 filters, Time taken: 679 ms
With 23 filters, Time taken: 363 ms
With 24 filters, Time taken: 342 ms
With 25 filters, Time taken: 336 ms
With 26 filters, Time taken: 352 ms
With 27 filters, Time taken: 322 ms
With 28 filters, Time taken: 302 ms
With 29 filters, Time taken: 307 ms
With 30 filters, Time taken: 301 ms
```
## How was this patch tested?
Pass the Jenkins with newly added test cases.
Closes#22313 from dongjoon-hyun/SPARK-25306.
Authored-by: Dongjoon Hyun <dongjoon@apache.org>
Signed-off-by: Wenchen Fan <wenchen@databricks.com>
## What changes were proposed in this pull request?
I made one pass over barrier APIs added to Spark 2.4 and updates some scopes and docs. I will update Python docs once Scala doc was reviewed.
One major issue is that `BarrierTaskContext` implements `TaskContextImpl` that exposes some public methods. And internally there were several direct references to `TaskContextImpl` methods instead of `TaskContext`. This PR moved some methods from `TaskContextImpl` to `TaskContext`, remaining package private, and used delegate methods to avoid inheriting `TaskContextImp` and exposing unnecessary APIs.
TODOs:
- [x] scala doc
- [x] python doc (#22261 ).
Closes#22240 from mengxr/SPARK-25248.
Authored-by: Xiangrui Meng <meng@databricks.com>
Signed-off-by: Xiangrui Meng <meng@databricks.com>
## What changes were proposed in this pull request?
Previously in `TakeOrderedAndProjectSuite` the SparkSession will not get recycled when the test suite finishes.
## How was this patch tested?
N/A
Closes#22330 from jiangxb1987/SPARK-19355.
Authored-by: Xingbo Jiang <xingbo.jiang@databricks.com>
Signed-off-by: Xiao Li <gatorsmile@gmail.com>
## What changes were proposed in this pull request?
This PR integrates handling of `UnsafeArrayData` and `GenericArrayData` into one. The current `CodeGenerator.createUnsafeArray` handles only allocation of `UnsafeArrayData`.
This PR introduces a new method `createArrayData` that returns a code to allocate `UnsafeArrayData` or `GenericArrayData` and to assign a value into the allocated array.
This PR also reduce the size of generated code by calling a runtime helper.
This PR replaced `createArrayData` with `createUnsafeArray`. This PR also refactor `ArraySetLike` that can be used for `ArrayDistinct`, too.
This PR also refactors`ArrayDistinct` to use `ArraryBuilder`.
## How was this patch tested?
Existing tests
Closes#21912 from kiszk/SPARK-24962.
Lead-authored-by: Kazuaki Ishizaki <ishizaki@jp.ibm.com>
Co-authored-by: Takuya UESHIN <ueshin@happy-camper.st>
Signed-off-by: Wenchen Fan <wenchen@databricks.com>
## What changes were proposed in this pull request?
This PR fixes a problem that `ArraysOverlap` function throws a `CompilationException` with non-nullable array type.
The following is the stack trace of the original problem:
```
Code generation of arrays_overlap([1,2,3], [4,5,3]) failed:
java.util.concurrent.ExecutionException: org.codehaus.commons.compiler.CompileException: File 'generated.java', Line 56, Column 11: failed to compile: org.codehaus.commons.compiler.CompileException: File 'generated.java', Line 56, Column 11: Expression "isNull_0" is not an rvalue
java.util.concurrent.ExecutionException: org.codehaus.commons.compiler.CompileException: File 'generated.java', Line 56, Column 11: failed to compile: org.codehaus.commons.compiler.CompileException: File 'generated.java', Line 56, Column 11: Expression "isNull_0" is not an rvalue
at com.google.common.util.concurrent.AbstractFuture$Sync.getValue(AbstractFuture.java:306)
at com.google.common.util.concurrent.AbstractFuture$Sync.get(AbstractFuture.java:293)
at com.google.common.util.concurrent.AbstractFuture.get(AbstractFuture.java:116)
at com.google.common.util.concurrent.Uninterruptibles.getUninterruptibly(Uninterruptibles.java:135)
at com.google.common.cache.LocalCache$Segment.getAndRecordStats(LocalCache.java:2410)
at com.google.common.cache.LocalCache$Segment.loadSync(LocalCache.java:2380)
at com.google.common.cache.LocalCache$Segment.lockedGetOrLoad(LocalCache.java:2342)
at com.google.common.cache.LocalCache$Segment.get(LocalCache.java:2257)
at com.google.common.cache.LocalCache.get(LocalCache.java:4000)
at com.google.common.cache.LocalCache.getOrLoad(LocalCache.java:4004)
at com.google.common.cache.LocalCache$LocalLoadingCache.get(LocalCache.java:4874)
at org.apache.spark.sql.catalyst.expressions.codegen.CodeGenerator$.compile(CodeGenerator.scala:1305)
at org.apache.spark.sql.catalyst.expressions.codegen.GenerateMutableProjection$.create(GenerateMutableProjection.scala:143)
at org.apache.spark.sql.catalyst.expressions.codegen.GenerateMutableProjection$.create(GenerateMutableProjection.scala:48)
at org.apache.spark.sql.catalyst.expressions.codegen.GenerateMutableProjection$.create(GenerateMutableProjection.scala:32)
at org.apache.spark.sql.catalyst.expressions.codegen.CodeGenerator.generate(CodeGenerator.scala:1260)
```
## How was this patch tested?
Added test in `CollectionExpressionSuite`.
Closes#22317 from kiszk/SPARK-25310.
Authored-by: Kazuaki Ishizaki <ishizaki@jp.ibm.com>
Signed-off-by: Takuya UESHIN <ueshin@databricks.com>
## What changes were proposed in this pull request?
Invoking ArrayContains function with non nullable array type throws the following error in the code generation phase. Below is the error snippet.
```SQL
Code generation of array_contains([1,2,3], 1) failed:
java.util.concurrent.ExecutionException: org.codehaus.commons.compiler.CompileException: File 'generated.java', Line 40, Column 11: failed to compile: org.codehaus.commons.compiler.CompileException: File 'generated.java', Line 40, Column 11: Expression "isNull_0" is not an rvalue
java.util.concurrent.ExecutionException: org.codehaus.commons.compiler.CompileException: File 'generated.java', Line 40, Column 11: failed to compile: org.codehaus.commons.compiler.CompileException: File 'generated.java', Line 40, Column 11: Expression "isNull_0" is not an rvalue
at com.google.common.util.concurrent.AbstractFuture$Sync.getValue(AbstractFuture.java:306)
at com.google.common.util.concurrent.AbstractFuture$Sync.get(AbstractFuture.java:293)
at com.google.common.util.concurrent.AbstractFuture.get(AbstractFuture.java:116)
at com.google.common.util.concurrent.Uninterruptibles.getUninterruptibly(Uninterruptibles.java:135)
at com.google.common.cache.LocalCache$Segment.getAndRecordStats(LocalCache.java:2410)
at com.google.common.cache.LocalCache$Segment.loadSync(LocalCache.java:2380)
at com.google.common.cache.LocalCache$Segment.lockedGetOrLoad(LocalCache.java:2342)
at com.google.common.cache.LocalCache$Segment.get(LocalCache.java:2257)
at com.google.common.cache.LocalCache.get(LocalCache.java:4000)
at com.google.common.cache.LocalCache.getOrLoad(LocalCache.java:4004)
at com.google.common.cache.LocalCache$LocalLoadingCache.get(LocalCache.java:4874)
at org.apache.spark.sql.catalyst.expressions.codegen.CodeGenerator$.compile(CodeGenerator.scala:1305)
```
## How was this patch tested?
Added test in CollectionExpressionSuite.
Closes#22315 from dilipbiswal/SPARK-25308.
Authored-by: Dilip Biswal <dbiswal@us.ibm.com>
Signed-off-by: Takuya UESHIN <ueshin@databricks.com>
## What changes were proposed in this pull request?
remove test-2.10.jar and add test-2.12.jar.
## How was this patch tested?
```
$ sbt -Dscala-2.12
> ++ 2.12.6
> project hive
> testOnly *HiveSparkSubmitSuite -- -z "8489"
```
Closes#22308 from sadhen/SPARK-8489-FOLLOWUP.
Authored-by: Darcy Shen <sadhen@zoho.com>
Signed-off-by: Sean Owen <sean.owen@databricks.com>
## What changes were proposed in this pull request?
This PR works for one of TODOs in `GenerateUnsafeProjection` "if the nullability of field is correct, we can use it to save null check" to simplify generated code.
When `nullable=false` in `DataType`, `GenerateUnsafeProjection` removed code for null checks in the generated Java code.
## How was this patch tested?
Added new test cases into `GenerateUnsafeProjectionSuite`
Closes#20637 from kiszk/SPARK-23466.
Authored-by: Kazuaki Ishizaki <ishizaki@jp.ibm.com>
Signed-off-by: Takuya UESHIN <ueshin@databricks.com>
## What changes were proposed in this pull request?
Move the output verification of Explain test cases to a new suite ExplainSuite.
## How was this patch tested?
N/A
Closes#22300 from gatorsmile/test3200.
Authored-by: Xiao Li <gatorsmile@gmail.com>
Signed-off-by: Xiao Li <gatorsmile@gmail.com>
## What changes were proposed in this pull request?
Currently, filter pushdown will not work if Parquet schema and Hive metastore schema are in different letter cases even spark.sql.caseSensitive is false.
Like the below case:
```scala
spark.sparkContext.hadoopConfiguration.setInt("parquet.block.size", 8 * 1024 * 1024)
spark.range(1, 40 * 1024 * 1024, 1, 1).sortWithinPartitions("id").write.parquet("/tmp/t")
sql("CREATE TABLE t (ID LONG) USING parquet LOCATION '/tmp/t'")
sql("select * from t where id < 100L").write.csv("/tmp/id")
```
Although filter "ID < 100L" is generated by Spark, it fails to pushdown into parquet actually, Spark still does the full table scan when reading.
This PR provides a case-insensitive field resolution to make it work.
Before - "ID < 100L" fail to pushedown:
<img width="273" alt="screen shot 2018-08-23 at 10 08 26 pm" src="https://user-images.githubusercontent.com/2989575/44530558-40ef8b00-a721-11e8-8abc-7f97671590d3.png">
After - "ID < 100L" pushedown sucessfully:
<img width="267" alt="screen shot 2018-08-23 at 10 08 40 pm" src="https://user-images.githubusercontent.com/2989575/44530567-44831200-a721-11e8-8634-e9f664b33d39.png">
## How was this patch tested?
Added UTs.
Closes#22197 from yucai/SPARK-25207.
Authored-by: yucai <yyu1@ebay.com>
Signed-off-by: Wenchen Fan <wenchen@databricks.com>
## What changes were proposed in this pull request?
Switch `org.apache.hive.service.server.HiveServer2` to register its shutdown callback with Spark's `ShutdownHookManager`, rather than direct with the Java Runtime callback.
This avoids race conditions in shutdown where the filesystem is shutdown before the flush/write/rename of the event log is completed, particularly on object stores where the write and rename can be slow.
## How was this patch tested?
There's no explicit unit for test this, which is consistent with every other shutdown hook in the codebase.
* There's an implicit test when the scalatest process is halted.
* More manual/integration testing is needed.
HADOOP-15679 has added the ability to explicitly execute the hadoop shutdown hook sequence which spark uses; that could be stabilized for testing if desired, after which all the spark hooks could be tested. Until then: external system tests only.
Author: Steve Loughran <stevel@hortonworks.com>
Closes#22186 from steveloughran/BUG/SPARK-25183-shutdown.
## What changes were proposed in this pull request?
### For `SPARK-5775 read array from partitioned_parquet_with_key_and_complextypes`:
scala2.12
```
scala> (1 to 10).toString
res4: String = Range 1 to 10
```
scala2.11
```
scala> (1 to 10).toString
res2: String = Range(1, 2, 3, 4, 5, 6, 7, 8, 9, 10)
```
And
```
def prepareAnswer(answer: Seq[Row], isSorted: Boolean): Seq[Row] = {
val converted: Seq[Row] = answer.map(prepareRow)
if (!isSorted) converted.sortBy(_.toString()) else converted
}
```
sortBy `_.toString` is not a good idea.
### Other failures are caused by
```
Array(Int.box(1)).toSeq == Array(Double.box(1.0)).toSeq
```
It is false in 2.12.2 + and is true in 2.11.x , 2.12.0, 2.12.1
## How was this patch tested?
This is a patch on a specific unit test.
Closes#22264 from sadhen/SPARK25256.
Authored-by: 忍冬 <rendong@wacai.com>
Signed-off-by: Sean Owen <sean.owen@databricks.com>
## What changes were proposed in this pull request?
`JavaColumnExpressionSuite.java` was added and `org.apache.spark.sql.ColumnExpressionSuite#test("isInCollection: Java Collection")` was removed.
It provides native Java tests for the method `org.apache.spark.sql.Column#isInCollection`.
Closes#22253 from aai95/isInCollectionJavaTest.
Authored-by: aai95 <aai95@yandex.ru>
Signed-off-by: DB Tsai <d_tsai@apple.com>
## What changes were proposed in this pull request?
This PR is an follow-up PR of #21087 based on [a discussion thread](https://github.com/apache/spark/pull/21087#discussion_r211080067]. Since #21087 changed a condition of `if` statement, the message in an exception is not consistent of the current behavior.
This PR updates the exception message.
## How was this patch tested?
Existing UTs
Closes#22269 from kiszk/SPARK-23997-followup.
Authored-by: Kazuaki Ishizaki <ishizaki@jp.ibm.com>
Signed-off-by: Sean Owen <sean.owen@databricks.com>
## What changes were proposed in this pull request?
Since https://github.com/apache/spark/pull/21696. Spark uses Parquet schema instead of Hive metastore schema to do pushdown.
That change can avoid wrong records returned when Hive metastore schema and parquet schema are in different letter cases. This pr add a test case for it.
More details:
https://issues.apache.org/jira/browse/SPARK-25206
## How was this patch tested?
unit tests
Closes#22267 from wangyum/SPARK-24716-TESTS.
Authored-by: Yuming Wang <yumwang@ebay.com>
Signed-off-by: Wenchen Fan <wenchen@databricks.com>
## What changes were proposed in this pull request?
Introduced by #21320 and #11744
```
$ sbt
> ++2.12.6
> project sql
> compile
...
[error] [warn] spark/sql/core/src/main/scala/org/apache/spark/sql/execution/ProjectionOverSchema.scala:41: match may not be exhaustive.
[error] It would fail on the following inputs: (_, ArrayType(_, _)), (_, _)
[error] [warn] getProjection(a.child).map(p => (p, p.dataType)).map {
[error] [warn]
[error] [warn] spark/sql/core/src/main/scala/org/apache/spark/sql/execution/ProjectionOverSchema.scala:52: match may not be exhaustive.
[error] It would fail on the following input: (_, _)
[error] [warn] getProjection(child).map(p => (p, p.dataType)).map {
[error] [warn]
...
```
And
```
$ sbt
> ++2.12.6
> project hive
> testOnly *ParquetMetastoreSuite
...
[error] /Users/rendong/wdi/spark/sql/hive/src/test/scala/org/apache/spark/sql/hive/HiveSparkSubmitSuite.scala:22: object tools is not a member of package scala
[error] import scala.tools.nsc.Properties
[error] ^
[error] /Users/rendong/wdi/spark/sql/hive/src/test/scala/org/apache/spark/sql/hive/HiveSparkSubmitSuite.scala:146: not found: value Properties
[error] val version = Properties.versionNumberString match {
[error] ^
[error] two errors found
...
```
## How was this patch tested?
Existing tests.
Closes#22260 from sadhen/fix_exhaustive_match.
Authored-by: 忍冬 <rendong@wacai.com>
Signed-off-by: hyukjinkwon <gurwls223@apache.org>
## What changes were proposed in this pull request?
`fromId` is the child, and `toId` is the parent, see line 127 in `buildSparkPlanGraphNode` above.
The edges in Spark UI also go from child to parent.
## How was this patch tested?
Comment change only. Inspected code above. Inspected how the edges in Spark UI look like.
Closes#22268 from juliuszsompolski/sparkplangraphedgedoc.
Authored-by: Juliusz Sompolski <julek@databricks.com>
Signed-off-by: Xiao Li <gatorsmile@gmail.com>
## What changes were proposed in this pull request?
Alternative take on https://github.com/apache/spark/pull/22063 that does not introduce udfInternal.
Resolve issue with inferring func types in 2.12 by instead using info captured when UDF is registered -- capturing which types are nullable (i.e. not primitive)
## How was this patch tested?
Existing tests.
Closes#22259 from srowen/SPARK-25044.2.
Authored-by: Sean Owen <sean.owen@databricks.com>
Signed-off-by: Wenchen Fan <wenchen@databricks.com>
## What changes were proposed in this pull request?
This changes the calls of `toPandas()` and `createDataFrame()` to use the Arrow stream format, when Arrow is enabled. Previously, Arrow data was written to byte arrays where each chunk is an output of the Arrow file format. This was mainly due to constraints at the time, and caused some overhead by writing the schema/footer on each chunk of data and then having to read multiple Arrow file inputs and concat them together.
Using the Arrow stream format has improved these by increasing performance, lower memory overhead for the average case, and simplified the code. Here are the details of this change:
**toPandas()**
_Before:_
Spark internal rows are converted to Arrow file format, each group of records is a complete Arrow file which contains the schema and other metadata. Next a collect is done and an Array of Arrow files is the result. After that each Arrow file is sent to Python driver which then loads each file and concats them to a single Arrow DataFrame.
_After:_
Spark internal rows are converted to ArrowRecordBatches directly, which is the simplest Arrow component for IPC data transfers. The driver JVM then immediately starts serving data to Python as an Arrow stream, sending the schema first. It then starts a Spark job with a custom handler that sends Arrow RecordBatches to Python. Partitions arriving in order are sent immediately, and out-of-order partitions are buffered until the ones that precede it come in. This improves performance, simplifies memory usage on executors, and improves the average memory usage on the JVM driver. Since the order of partitions must be preserved, the worst case is that the first partition will be the last to arrive all data must be buffered in memory until then. This case is no worse that before when doing a full collect.
**createDataFrame()**
_Before:_
A Pandas DataFrame is split into parts and each part is made into an Arrow file. Then each file is prefixed by the buffer size and written to a temp file. The temp file is read and each Arrow file is parallelized as a byte array.
_After:_
A Pandas DataFrame is split into parts, then an Arrow stream is written to a temp file where each part is an ArrowRecordBatch. The temp file is read as a stream and the Arrow messages are examined. If the message is an ArrowRecordBatch, the data is saved as a byte array. After reading the file, each ArrowRecordBatch is parallelized as a byte array. This has slightly more processing than before because we must look each Arrow message to extract the record batches, but performance ends up a litle better. It is cleaner in the sense that IPC from Python to JVM is done over a single Arrow stream.
## How was this patch tested?
Added new unit tests for the additions to ArrowConverters in Scala, existing tests for Python.
## Performance Tests - toPandas
Tests run on a 4 node standalone cluster with 32 cores total, 14.04.1-Ubuntu and OpenJDK 8
measured wall clock time to execute `toPandas()` and took the average best time of 5 runs/5 loops each.
Test code
```python
df = spark.range(1 << 25, numPartitions=32).toDF("id").withColumn("x1", rand()).withColumn("x2", rand()).withColumn("x3", rand()).withColumn("x4", rand())
for i in range(5):
start = time.time()
_ = df.toPandas()
elapsed = time.time() - start
```
Current Master | This PR
---------------------|------------
5.803557 | 5.16207
5.409119 | 5.133671
5.493509 | 5.147513
5.433107 | 5.105243
5.488757 | 5.018685
Avg Master | Avg This PR
------------------|--------------
5.5256098 | 5.1134364
Speedup of **1.08060595**
## Performance Tests - createDataFrame
Tests run on a 4 node standalone cluster with 32 cores total, 14.04.1-Ubuntu and OpenJDK 8
measured wall clock time to execute `createDataFrame()` and get the first record. Took the average best time of 5 runs/5 loops each.
Test code
```python
def run():
pdf = pd.DataFrame(np.random.rand(10000000, 10))
spark.createDataFrame(pdf).first()
for i in range(6):
start = time.time()
run()
elapsed = time.time() - start
gc.collect()
print("Run %d: %f" % (i, elapsed))
```
Current Master | This PR
--------------------|----------
6.234608 | 5.665641
6.32144 | 5.3475
6.527859 | 5.370803
6.95089 | 5.479151
6.235046 | 5.529167
Avg Master | Avg This PR
---------------|----------------
6.4539686 | 5.4784524
Speedup of **1.178064192**
## Memory Improvements
**toPandas()**
The most significant improvement is reduction of the upper bound space complexity in the JVM driver. Before, the entire dataset was collected in the JVM first before sending it to Python. With this change, as soon as a partition is collected, the result handler immediately sends it to Python, so the upper bound is the size of the largest partition. Also, using the Arrow stream format is more efficient because the schema is written once per stream, followed by record batches. The schema is now only send from driver JVM to Python. Before, multiple Arrow file formats were used that each contained the schema. This duplicated schema was created in the executors, sent to the driver JVM, and then Python where all but the first one received are discarded.
I verified the upper bound limit by running a test that would collect data that would exceed the amount of driver JVM memory available. Using these settings on a standalone cluster:
```
spark.driver.memory 1g
spark.executor.memory 5g
spark.sql.execution.arrow.enabled true
spark.sql.execution.arrow.fallback.enabled false
spark.sql.execution.arrow.maxRecordsPerBatch 0
spark.driver.maxResultSize 2g
```
Test code:
```python
from pyspark.sql.functions import rand
df = spark.range(1 << 25, numPartitions=32).toDF("id").withColumn("x1", rand()).withColumn("x2", rand()).withColumn("x3", rand())
df.toPandas()
```
This makes total data size of 33554432×8×4 = 1073741824
With the current master, it fails with OOM but passes using this PR.
**createDataFrame()**
No significant change in memory except that using the stream format instead of separate file formats avoids duplicated the schema, similar to toPandas above. The process of reading the stream and parallelizing the batches does cause the record batch message metadata to be copied, but it's size is insignificant.
Closes#21546 from BryanCutler/arrow-toPandas-stream-SPARK-23030.
Authored-by: Bryan Cutler <cutlerb@gmail.com>
Signed-off-by: hyukjinkwon <gurwls223@apache.org>
## What changes were proposed in this pull request?
Most of `HigherOrderFunction`s have the same `nullable` definition, ie. they are nullable when one of their arguments is nullable. The PR refactors it in order to avoid code duplication.
## How was this patch tested?
NA
Closes#22243 from mgaido91/MINOR_nullable_hof.
Authored-by: Marco Gaido <marcogaido91@gmail.com>
Signed-off-by: hyukjinkwon <gurwls223@apache.org>
## What changes were proposed in this pull request?
Support Filter in ConvertToLocalRelation, similar to how Project works.
Additionally, in Optimizer, run ConvertToLocalRelation earlier to simplify the plan. This is good for very short queries which often are queries on local relations.
## How was this patch tested?
New test. Manual benchmark.
Author: Bogdan Raducanu <bogdan@databricks.com>
Author: Shixiong Zhu <zsxwing@gmail.com>
Author: Yinan Li <ynli@google.com>
Author: Li Jin <ice.xelloss@gmail.com>
Author: s71955 <sujithchacko.2010@gmail.com>
Author: DB Tsai <d_tsai@apple.com>
Author: jaroslav chládek <mastermism@gmail.com>
Author: Huangweizhe <huangweizhe@bbdservice.com>
Author: Xiangrui Meng <meng@databricks.com>
Author: hyukjinkwon <gurwls223@apache.org>
Author: Kent Yao <yaooqinn@hotmail.com>
Author: caoxuewen <cao.xuewen@zte.com.cn>
Author: liuxian <liu.xian3@zte.com.cn>
Author: Adam Bradbury <abradbury@users.noreply.github.com>
Author: Jose Torres <torres.joseph.f+github@gmail.com>
Author: Yuming Wang <yumwang@ebay.com>
Author: Liang-Chi Hsieh <viirya@gmail.com>
Closes#22205 from bogdanrdc/local-relation-filter.
## What changes were proposed in this pull request?
This adds `spark.executor.pyspark.memory` to configure Python's address space limit, [`resource.RLIMIT_AS`](https://docs.python.org/3/library/resource.html#resource.RLIMIT_AS). Limiting Python's address space allows Python to participate in memory management. In practice, we see fewer cases of Python taking too much memory because it doesn't know to run garbage collection. This results in YARN killing fewer containers. This also improves error messages so users know that Python is consuming too much memory:
```
File "build/bdist.linux-x86_64/egg/package/library.py", line 265, in fe_engineer
fe_eval_rec.update(f(src_rec_prep, mat_rec_prep))
File "build/bdist.linux-x86_64/egg/package/library.py", line 163, in fe_comp
comparisons = EvaluationUtils.leven_list_compare(src_rec_prep.get(item, []), mat_rec_prep.get(item, []))
File "build/bdist.linux-x86_64/egg/package/evaluationutils.py", line 25, in leven_list_compare
permutations = sorted(permutations, reverse=True)
MemoryError
```
The new pyspark memory setting is used to increase requested YARN container memory, instead of sharing overhead memory between python and off-heap JVM activity.
## How was this patch tested?
Tested memory limits in our YARN cluster and verified that MemoryError is thrown.
Author: Ryan Blue <blue@apache.org>
Closes#21977 from rdblue/SPARK-25004-add-python-memory-limit.
## What changes were proposed in this pull request?
In the PR, I propose to not perform recursive parallel listening of files in the `scanPartitions` method because it can cause a deadlock. Instead of that I propose to do `scanPartitions` in parallel for top level partitions only.
## How was this patch tested?
I extended an existing test to trigger the deadlock.
Author: Maxim Gekk <maxim.gekk@databricks.com>
Closes#22233 from MaxGekk/fix-recover-partitions.
## What changes were proposed in this pull request?
This PR implements the possibility of the user to override the maximum number of buckets when saving to a table.
Currently the limit is a hard-coded 100k, which might be insufficient for large workloads.
A new configuration entry is proposed: `spark.sql.bucketing.maxBuckets`, which defaults to the previous 100k.
## How was this patch tested?
Added unit tests in the following spark.sql test suites:
- CreateTableAsSelectSuite
- BucketedWriteSuite
Author: Fernando Pereira <fernando.pereira@epfl.ch>
Closes#21087 from ferdonline/enh/configurable_bucket_limit.
## What changes were proposed in this pull request?
The PR excludes Python UDFs filters in FileSourceStrategy so that they don't ExtractPythonUDF rule to throw exception. It doesn't make sense to pass Python UDF filters in FileSourceStrategy anyway because they cannot be used as push down filters.
## How was this patch tested?
Add a new regression test
Closes#22104 from icexelloss/SPARK-24721-udf-filter.
Authored-by: Li Jin <ice.xelloss@gmail.com>
Signed-off-by: Wenchen Fan <wenchen@databricks.com>
## What changes were proposed in this pull request?
this pr add a configuration parameter to configure the capacity of fast aggregation.
Performance comparison:
```
Java HotSpot(TM) 64-Bit Server VM 1.8.0_60-b27 on Windows 7 6.1
Intel64 Family 6 Model 94 Stepping 3, GenuineIntel
Aggregate w multiple keys: Best/Avg Time(ms) Rate(M/s) Per Row(ns) Relative
------------------------------------------------------------------------------------------------
fasthash = default 5612 / 5882 3.7 267.6 1.0X
fasthash = config 3586 / 3595 5.8 171.0 1.6X
```
## How was this patch tested?
the existed test cases.
Closes#21931 from heary-cao/FastHashCapacity.
Authored-by: caoxuewen <cao.xuewen@zte.com.cn>
Signed-off-by: Wenchen Fan <wenchen@databricks.com>
## What changes were proposed in this pull request?
This is based on the discussion https://github.com/apache/spark/pull/16677/files#r212805327.
As SQL standard doesn't mandate that a nested order by followed by a limit has to respect that ordering clause, this patch removes the `child.outputOrdering` check.
## How was this patch tested?
Unit tests.
Closes#22239 from viirya/improve-global-limit-parallelism-followup.
Authored-by: Liang-Chi Hsieh <viirya@gmail.com>
Signed-off-by: Wenchen Fan <wenchen@databricks.com>
## What changes were proposed in this pull request?
Improved the documentation for the datetime functions in `org.apache.spark.sql.functions` by adding details about the supported column input types, the column return type, behaviour on invalid input, supporting examples and clarifications.
## How was this patch tested?
Manually testing each of the datetime functions with different input to ensure that the corresponding Javadoc/Scaladoc matches the behaviour of the function. Successfully ran the `unidoc` SBT process.
Closes#20901 from abradbury/SPARK-23792.
Authored-by: Adam Bradbury <abradbury@users.noreply.github.com>
Signed-off-by: Sean Owen <sean.owen@databricks.com>
## What changes were proposed in this pull request?
This PR generates the code that to refer a `StructType` generated in the scala code instead of generating `StructType` in Java code.
The original code has two issues.
1. Avoid to used the field name such as `key.name`
1. Support complicated schema (e.g. nested DataType)
At first, [the JIRA entry](https://issues.apache.org/jira/browse/SPARK-25178) proposed to change the generated field name of the keySchema / valueSchema to a dummy name in `RowBasedHashMapGenerator` and `VectorizedHashMapGenerator.scala`. This proposal can addresse issue 1.
Ueshin suggested an approach to refer to a `StructType` generated in the scala code using `ctx.addReferenceObj()`. This approach can address issues 1 and 2. Finally, this PR uses this approach.
## How was this patch tested?
Existing UTs
Closes#22187 from kiszk/SPARK-25178.
Authored-by: Kazuaki Ishizaki <ishizaki@jp.ibm.com>
Signed-off-by: Takuya UESHIN <ueshin@databricks.com>
## What changes were proposed in this pull request?
Update to janino 3.0.9 to address Java 8 + Scala 2.12 incompatibility. The error manifests as test failures like this in `ExpressionEncoderSuite`:
```
- encode/decode for seq of string: List(abc, xyz) *** FAILED ***
java.lang.RuntimeException: Error while encoding: org.codehaus.janino.InternalCompilerException: failed to compile: org.codehaus.janino.InternalCompilerException: Compiling "GeneratedClass": Two non-abstract methods "public int scala.collection.TraversableOnce.size()" have the same parameter types, declaring type and return type
```
It comes up pretty immediately in any generated code that references Scala collections, and virtually always concerning the `size()` method.
## How was this patch tested?
Existing tests
Closes#22203 from srowen/SPARK-25029.
Authored-by: Sean Owen <sean.owen@databricks.com>
Signed-off-by: Xiao Li <gatorsmile@gmail.com>
(Link to Jira: https://issues.apache.org/jira/browse/SPARK-4502)
_N.B. This is a restart of PR #16578 which includes a subset of that code. Relevant review comments from that PR should be considered incorporated by reference. Please avoid duplication in review by reviewing that PR first. The summary below is an edited copy of the summary of the previous PR._
## What changes were proposed in this pull request?
One of the hallmarks of a column-oriented data storage format is the ability to read data from a subset of columns, efficiently skipping reads from other columns. Spark has long had support for pruning unneeded top-level schema fields from the scan of a parquet file. For example, consider a table, `contacts`, backed by parquet with the following Spark SQL schema:
```
root
|-- name: struct
| |-- first: string
| |-- last: string
|-- address: string
```
Parquet stores this table's data in three physical columns: `name.first`, `name.last` and `address`. To answer the query
```SQL
select address from contacts
```
Spark will read only from the `address` column of parquet data. However, to answer the query
```SQL
select name.first from contacts
```
Spark will read `name.first` and `name.last` from parquet.
This PR modifies Spark SQL to support a finer-grain of schema pruning. With this patch, Spark reads only the `name.first` column to answer the previous query.
### Implementation
There are two main components of this patch. First, there is a `ParquetSchemaPruning` optimizer rule for gathering the required schema fields of a `PhysicalOperation` over a parquet file, constructing a new schema based on those required fields and rewriting the plan in terms of that pruned schema. The pruned schema fields are pushed down to the parquet requested read schema. `ParquetSchemaPruning` uses a new `ProjectionOverSchema` extractor for rewriting a catalyst expression in terms of a pruned schema.
Second, the `ParquetRowConverter` has been patched to ensure the ordinals of the parquet columns read are correct for the pruned schema. `ParquetReadSupport` has been patched to address a compatibility mismatch between Spark's built in vectorized reader and the parquet-mr library's reader.
### Limitation
Among the complex Spark SQL data types, this patch supports parquet column pruning of nested sequences of struct fields only.
## How was this patch tested?
Care has been taken to ensure correctness and prevent regressions. A more advanced version of this patch incorporating optimizations for rewriting queries involving aggregations and joins has been running on a production Spark cluster at VideoAmp for several years. In that time, one bug was found and fixed early on, and we added a regression test for that bug.
We forward-ported this patch to Spark master in June 2016 and have been running this patch against Spark 2.x branches on ad-hoc clusters since then.
Closes#21320 from mallman/spark-4502-parquet_column_pruning-foundation.
Lead-authored-by: Michael Allman <msa@allman.ms>
Co-authored-by: Adam Jacques <adam@technowizardry.net>
Co-authored-by: Michael Allman <michael@videoamp.com>
Signed-off-by: Xiao Li <gatorsmile@gmail.com>
## What changes were proposed in this pull request?
Dataset.apply calls dataset.deserializer (to provide an early error) which ends up calling the full Analyzer on the deserializer. This can take tens of milliseconds, depending on how big the plan is.
Since Dataset.apply is called for many Dataset operations such as Dataset.where it can be a significant overhead for short queries.
According to a comment in the PR that introduced this check, we can at least remove this check for DataFrames: https://github.com/apache/spark/pull/20402#discussion_r164338267
## How was this patch tested?
Existing tests + manual benchmark
Author: Bogdan Raducanu <bogdan@databricks.com>
Closes#22201 from bogdanrdc/deserializer-fix.
## What changes were proposed in this pull request?
**Problem statement**
load data command with hdfs file paths consists of wild card strings like * are not working
eg:
"load data inpath 'hdfs://hacluster/user/ext* into table t1"
throws Analysis exception while executing this query

**Analysis -**
Currently fs.exists() API which is used for path validation in load command API cannot resolve the path with wild card pattern, To mitigate this problem i am using globStatus() API another api which can resolve the paths with hdfs supported wildcards like *,? etc(inline with hive wildcard support).
**Improvement identified as part of this issue -**
Currently system wont support wildcard character to be used for folder level path in a local file system. This PR has handled this scenario, the same globStatus API will unify the validation logic of local and non local file systems, this will ensure the behavior consistency between the hdfs and local file path in load command.
with this improvement user will be able to use a wildcard character in folder level path of a local file system in load command inline with hive behaviour, in older versions user can use wildcards only in file path of the local file system if they use in folder path system use to give an error by mentioning that not supported.
eg: load data local inpath '/localfilesystem/folder* into table t1
## How was this patch tested?
a) Manually tested by executing test-cases in HDFS yarn cluster. Reports is been attached in below section.
b) Existing test-case can verify the impact and functionality for local file path scenarios
c) A test-case is been added for verifying the functionality when wild card is been used in folder level path of a local file system
## Test Results
Note: all ip's were updated to localhost for security reasons.
HDFS path details
```
vm1:/opt/ficlient # hadoop fs -ls /user/data/sujith1
Found 2 items
-rw-r--r-- 3 shahid hadoop 4802 2018-03-26 15:45 /user/data/sujith1/typeddata60.txt
-rw-r--r-- 3 shahid hadoop 4883 2018-03-26 15:45 /user/data/sujith1/typeddata61.txt
vm1:/opt/ficlient # hadoop fs -ls /user/data/sujith2
Found 2 items
-rw-r--r-- 3 shahid hadoop 4802 2018-03-26 15:45 /user/data/sujith2/typeddata60.txt
-rw-r--r-- 3 shahid hadoop 4883 2018-03-26 15:45 /user/data/sujith2/typeddata61.txt
```
positive scenario by specifying complete file path to know about record size
```
0: jdbc:hive2://localhost:22550/default> create table wild_spark (time timestamp, name string, isright boolean, datetoday date, num binary, height double, score float, decimaler decimal(10,0), id tinyint, age int, license bigint, length smallint) row format delimited fields terminated by ',';
+---------+--+
| Result |
+---------+--+
+---------+--+
No rows selected (1.217 seconds)
0: jdbc:hive2://localhost:22550/default> load data inpath '/user/data/sujith1/typeddata60.txt' into table wild_spark;
+---------+--+
| Result |
+---------+--+
+---------+--+
No rows selected (4.236 seconds)
0: jdbc:hive2://localhost:22550/default> load data inpath '/user/data/sujith1/typeddata61.txt' into table wild_spark;
+---------+--+
| Result |
+---------+--+
+---------+--+
No rows selected (0.602 seconds)
0: jdbc:hive2://localhost:22550/default> select count(*) from wild_spark;
+-----------+--+
| count(1) |
+-----------+--+
| 121 |
+-----------+--+
1 row selected (18.529 seconds)
0: jdbc:hive2://localhost:22550/default>
```
With wild card character in file path
```
0: jdbc:hive2://localhost:22550/default> create table spark_withWildChar (time timestamp, name string, isright boolean, datetoday date, num binary, height double, score float, decimaler decimal(10,0), id tinyint, age int, license bigint, length smallint) row format delimited fields terminated by ',';
+---------+--+
| Result |
+---------+--+
+---------+--+
No rows selected (0.409 seconds)
0: jdbc:hive2://localhost:22550/default> load data inpath '/user/data/sujith1/type*' into table spark_withWildChar;
+---------+--+
| Result |
+---------+--+
+---------+--+
No rows selected (1.502 seconds)
0: jdbc:hive2://localhost:22550/default> select count(*) from spark_withWildChar;
+-----------+--+
| count(1) |
+-----------+--+
| 121 |
+-----------+--+
```
with ? wild card scenario
```
0: jdbc:hive2://localhost:22550/default> create table spark_withWildChar_DiffChar (time timestamp, name string, isright boolean, datetoday date, num binary, height double, score float, decimaler decimal(10,0), id tinyint, age int, license bigint, length smallint) row format delimited fields terminated by ',';
+---------+--+
| Result |
+---------+--+
+---------+--+
No rows selected (0.489 seconds)
0: jdbc:hive2://localhost:22550/default> load data inpath '/user/data/sujith1/?ypeddata60.txt' into table spark_withWildChar_DiffChar;
+---------+--+
| Result |
+---------+--+
+---------+--+
No rows selected (1.152 seconds)
0: jdbc:hive2://localhost:22550/default> load data inpath '/user/data/sujith1/?ypeddata61.txt' into table spark_withWildChar_DiffChar;
+---------+--+
| Result |
+---------+--+
+---------+--+
No rows selected (0.644 seconds)
0: jdbc:hive2://localhost:22550/default> select count(*) from spark_withWildChar_DiffChar;
+-----------+--+
| count(1) |
+-----------+--+
| 121 |
+-----------+--+
1 row selected (16.078 seconds)
```
with folder level wild card scenario
```
0: jdbc:hive2://localhost:22550/default> create table spark_withWildChar_folderlevel (time timestamp, name string, isright boolean, datetoday date, num binary, height double, score float, decimaler decimal(10,0), id tinyint, age int, license bigint, length smallint) row format delimited fields terminated by ',';
+---------+--+
| Result |
+---------+--+
+---------+--+
No rows selected (0.489 seconds)
0: jdbc:hive2://localhost:22550/default> load data inpath '/user/data/suji*/*' into table spark_withWildChar_folderlevel;
+---------+--+
| Result |
+---------+--+
+---------+--+
No rows selected (1.152 seconds)
0: jdbc:hive2://localhost:22550/default> select count(*) from spark_withWildChar_folderlevel;
+-----------+--+
| count(1) |
+-----------+--+
| 242 |
+-----------+--+
1 row selected (16.078 seconds)
```
Negative scenario invalid path
```
0: jdbc:hive2://localhost:22550/default> load data inpath '/user/data/sujiinvalid*/*' into table spark_withWildChar_folder;
Error: org.apache.spark.sql.AnalysisException: LOAD DATA input path does not exist: /user/data/sujiinvalid*/*; (state=,code=0)
0: jdbc:hive2://localhost:22550/default>
```
Hive Test results- file level
```
0: jdbc:hive2://localhost:21066/> create table hive_withWildChar_files (time timestamp, name string, isright boolean, datetoday date, num binary, height double, score float, decimaler decimal(10,0), id tinyint, age int, license bigint, length smallint) stored as TEXTFILE;
No rows affected (0.723 seconds)
0: jdbc:hive2://localhost:21066/> load data inpath '/user/data/sujith1/type*' into table hive_withWildChar_files;
INFO : Loading data to table default.hive_withwildchar_files from hdfs://hacluster/user/sujith1/type*
No rows affected (0.682 seconds)
0: jdbc:hive2://localhost:21066/> select count(*) from hive_withWildChar_files;
+------+--+
| _c0 |
+------+--+
| 121 |
+------+--+
1 row selected (50.832 seconds)
```
Hive Test results- folder level
```
0: jdbc:hive2://localhost:21066/> create table hive_withWildChar_folder (time timestamp, name string, isright boolean, datetoday date, num binary, height double, score float, decimaler decimal(10,0), id tinyint, age int, license bigint, length smallint) stored as TEXTFILE;
No rows affected (0.459 seconds)
0: jdbc:hive2://localhost:21066/> load data inpath '/user/data/suji*/*' into table hive_withWildChar_folder;
INFO : Loading data to table default.hive_withwildchar_folder from hdfs://hacluster/user/data/suji*/*
No rows affected (0.76 seconds)
0: jdbc:hive2://localhost:21066/> select count(*) from hive_withWildChar_folder;
+------+--+
| _c0 |
+------+--+
| 242 |
+------+--+
1 row selected (46.483 seconds)
```
Closes#20611 from sujith71955/master_wldcardsupport.
Lead-authored-by: s71955 <sujithchacko.2010@gmail.com>
Co-authored-by: sujith71955 <sujithchacko.2010@gmail.com>
Signed-off-by: hyukjinkwon <gurwls223@apache.org>
## What changes were proposed in this pull request?
Fix a race in the rate source tests. We need a better way of testing restart behavior.
## How was this patch tested?
unit test
Closes#22191 from jose-torres/racetest.
Authored-by: Jose Torres <torres.joseph.f+github@gmail.com>
Signed-off-by: Tathagata Das <tathagata.das1565@gmail.com>
## What changes were proposed in this pull request?
Casting to `DecimalType` is not always needed to force nullable.
If the decimal type to cast is wider than original type, or only truncating or precision loss, the casted value won't be `null`.
## How was this patch tested?
Added and modified tests.
Closes#22200 from ueshin/issues/SPARK-25208/cast_nullable_decimal.
Authored-by: Takuya UESHIN <ueshin@databricks.com>
Signed-off-by: Wenchen Fan <wenchen@databricks.com>
## What changes were proposed in this pull request?
[SPARK-25126] (https://issues.apache.org/jira/browse/SPARK-25126)
reports loading a large number of orc files consumes a lot of memory
in both 2.0 and 2.3. The issue is caused by creating a Reader for every
orc file in order to infer the schema.
In OrFileOperator.ReadSchema, a Reader is created for every file
although only the first valid one is used. This uses significant
amount of memory when there `paths` have a lot of files. In 2.3
a different code path (OrcUtils.readSchema) is used for inferring
schema for orc files. This commit changes both functions to create
Reader lazily.
## How was this patch tested?
Pass the Jenkins with a newly added test case by dongjoon-hyun
Closes#22157 from raofu/SPARK-25126.
Lead-authored-by: Rao Fu <rao@coupang.com>
Co-authored-by: Dongjoon Hyun <dongjoon@apache.org>
Co-authored-by: Rao Fu <raofu04@gmail.com>
Signed-off-by: hyukjinkwon <gurwls223@apache.org>
## What changes were proposed in this pull request?
VectorizedParquetRecordReader::initializeInternal rebuilds the column list and path list once for each column. Therefore, it indirectly iterates 2\*colCount\*colCount times for each parquet file.
This inefficiency impacts jobs that read parquet-backed tables with many columns and many files. Jobs that read tables with few columns or few files are not impacted.
This PR changes initializeInternal so that it builds each list only once.
I ran benchmarks on my laptop with 1 worker thread, running this query:
<pre>
sql("select * from parquet_backed_table where id1 = 1").collect
</pre>
There are roughly one matching row for every 425 rows, and the matching rows are sprinkled pretty evenly throughout the table (that is, every page for column <code>id1</code> has at least one matching row).
6000 columns, 1 million rows, 67 32M files:
master | branch | improvement
-------|---------|-----------
10.87 min | 6.09 min | 44%
6000 columns, 1 million rows, 23 98m files:
master | branch | improvement
-------|---------|-----------
7.39 min | 5.80 min | 21%
600 columns 10 million rows, 67 32M files:
master | branch | improvement
-------|---------|-----------
1.95 min | 1.96 min | -0.5%
60 columns, 100 million rows, 67 32M files:
master | branch | improvement
-------|---------|-----------
0.55 min | 0.55 min | 0%
## How was this patch tested?
- sql unit tests
- pyspark-sql tests
Closes#22188 from bersprockets/SPARK-25164.
Authored-by: Bruce Robbins <bersprockets@gmail.com>
Signed-off-by: Wenchen Fan <wenchen@databricks.com>
## What changes were proposed in this pull request?
This pr proposed to show RDD/relation names in RDD/Hive table scan nodes.
This change made these names show up in the webUI and explain results.
For example;
```
scala> sql("CREATE TABLE t(c1 int) USING hive")
scala> sql("INSERT INTO t VALUES(1)")
scala> spark.table("t").explain()
== Physical Plan ==
Scan hive default.t [c1#8], HiveTableRelation `default`.`t`, org.apache.hadoop.hive.serde2.lazy.LazySimpleSerDe, [c1#8]
^^^^^^^^^^^
```
<img width="212" alt="spark-pr-hive" src="https://user-images.githubusercontent.com/692303/44501013-51264c80-a6c6-11e8-94f8-0704aee83bb6.png">
Closes#20226
## How was this patch tested?
Added tests in `DataFrameSuite`, `DatasetSuite`, and `HiveExplainSuite`
Closes#22153 from maropu/pr20226.
Lead-authored-by: Takeshi Yamamuro <yamamuro@apache.org>
Co-authored-by: Tejas Patil <tejasp@fb.com>
Signed-off-by: Wenchen Fan <wenchen@databricks.com>
## What changes were proposed in this pull request?
This is a follow-up pr of #22031 which added `zip_with` function to fix an example.
## How was this patch tested?
Existing tests.
Closes#22194 from ueshin/issues/SPARK-23932/fix_examples.
Authored-by: Takuya UESHIN <ueshin@databricks.com>
Signed-off-by: hyukjinkwon <gurwls223@apache.org>
## What changes were proposed in this pull request?
They depend on internal Expression APIs. Let's see how far we can get without it.
## How was this patch tested?
Just some code removal. There's no existing tests as far as I can tell so it's easy to remove.
Closes#22185 from rxin/SPARK-25127.
Authored-by: Reynold Xin <rxin@databricks.com>
Signed-off-by: Wenchen Fan <wenchen@databricks.com>
## What changes were proposed in this pull request?
The race condition that caused test failure is between 2 threads.
- The MicrobatchExecution thread that processes inputs to produce answers and then generates progress events.
- The test thread that generates some input data, checked the answer and then verified the query generated progress event.
The synchronization structure between these threads is as follows
1. MicrobatchExecution thread, in every batch, does the following in order.
a. Processes batch input to generate answer.
b. Signals `awaitProgressLockCondition` to wake up threads waiting for progress using `awaitOffset`
c. Generates progress event
2. Test execution thread
a. Calls `awaitOffset` to wait for progress, which waits on `awaitProgressLockCondition`.
b. As soon as `awaitProgressLockCondition` is signaled, it would move on the in the test to check answer.
c. Finally, it would verify the last generated progress event.
What can happen is the following sequence of events: 2a -> 1a -> 1b -> 2b -> 2c -> 1c.
In other words, the progress event may be generated after the test tries to verify it.
The solution has two steps.
1. Signal the waiting thread after the progress event has been generated, that is, after `finishTrigger()`.
2. Increase the timeout of `awaitProgressLockCondition.await(100 ms)` to a large value.
This latter is to ensure that test thread for keeps waiting on `awaitProgressLockCondition`until the MicroBatchExecution thread explicitly signals it. With the existing small timeout of 100ms the following sequence can occur.
- MicroBatchExecution thread updates committed offsets
- Test thread waiting on `awaitProgressLockCondition` accidentally times out after 100 ms, finds that the committed offsets have been updated, therefore returns from `awaitOffset` and moves on to the progress event tests.
- MicroBatchExecution thread then generates progress event and signals. But the test thread has already attempted to verify the event and failed.
By increasing the timeout to large (e.g., `streamingTimeoutMs = 60 seconds`, similar to `awaitInitialization`), this above type of race condition is also avoided.
## How was this patch tested?
Ran locally many times.
Closes#22182 from tdas/SPARK-25184.
Authored-by: Tathagata Das <tathagata.das1565@gmail.com>
Signed-off-by: Tathagata Das <tathagata.das1565@gmail.com>
## What changes were proposed in this pull request?
Fix issues arising from the fact that builtins __file__, __long__, __raw_input()__, __unicode__, __xrange()__, etc. were all removed from Python 3. __Undefined names__ have the potential to raise [NameError](https://docs.python.org/3/library/exceptions.html#NameError) at runtime.
## How was this patch tested?
* $ __python2 -m flake8 . --count --select=E9,F82 --show-source --statistics__
* $ __python3 -m flake8 . --count --select=E9,F82 --show-source --statistics__
holdenk
flake8 testing of https://github.com/apache/spark on Python 3.6.3
$ __python3 -m flake8 . --count --select=E901,E999,F821,F822,F823 --show-source --statistics__
```
./dev/merge_spark_pr.py:98:14: F821 undefined name 'raw_input'
result = raw_input("\n%s (y/n): " % prompt)
^
./dev/merge_spark_pr.py:136:22: F821 undefined name 'raw_input'
primary_author = raw_input(
^
./dev/merge_spark_pr.py:186:16: F821 undefined name 'raw_input'
pick_ref = raw_input("Enter a branch name [%s]: " % default_branch)
^
./dev/merge_spark_pr.py:233:15: F821 undefined name 'raw_input'
jira_id = raw_input("Enter a JIRA id [%s]: " % default_jira_id)
^
./dev/merge_spark_pr.py:278:20: F821 undefined name 'raw_input'
fix_versions = raw_input("Enter comma-separated fix version(s) [%s]: " % default_fix_versions)
^
./dev/merge_spark_pr.py:317:28: F821 undefined name 'raw_input'
raw_assignee = raw_input(
^
./dev/merge_spark_pr.py:430:14: F821 undefined name 'raw_input'
pr_num = raw_input("Which pull request would you like to merge? (e.g. 34): ")
^
./dev/merge_spark_pr.py:442:18: F821 undefined name 'raw_input'
result = raw_input("Would you like to use the modified title? (y/n): ")
^
./dev/merge_spark_pr.py:493:11: F821 undefined name 'raw_input'
while raw_input("\n%s (y/n): " % pick_prompt).lower() == "y":
^
./dev/create-release/releaseutils.py:58:16: F821 undefined name 'raw_input'
response = raw_input("%s [y/n]: " % msg)
^
./dev/create-release/releaseutils.py:152:38: F821 undefined name 'unicode'
author = unidecode.unidecode(unicode(author, "UTF-8")).strip()
^
./python/setup.py:37:11: F821 undefined name '__version__'
VERSION = __version__
^
./python/pyspark/cloudpickle.py:275:18: F821 undefined name 'buffer'
dispatch[buffer] = save_buffer
^
./python/pyspark/cloudpickle.py:807:18: F821 undefined name 'file'
dispatch[file] = save_file
^
./python/pyspark/sql/conf.py:61:61: F821 undefined name 'unicode'
if not isinstance(obj, str) and not isinstance(obj, unicode):
^
./python/pyspark/sql/streaming.py:25:21: F821 undefined name 'long'
intlike = (int, long)
^
./python/pyspark/streaming/dstream.py:405:35: F821 undefined name 'long'
return self._sc._jvm.Time(long(timestamp * 1000))
^
./sql/hive/src/test/resources/data/scripts/dumpdata_script.py:21:10: F821 undefined name 'xrange'
for i in xrange(50):
^
./sql/hive/src/test/resources/data/scripts/dumpdata_script.py:22:14: F821 undefined name 'xrange'
for j in xrange(5):
^
./sql/hive/src/test/resources/data/scripts/dumpdata_script.py:23:18: F821 undefined name 'xrange'
for k in xrange(20022):
^
20 F821 undefined name 'raw_input'
20
```
Closes#20838 from cclauss/fix-undefined-names.
Authored-by: cclauss <cclauss@bluewin.ch>
Signed-off-by: Bryan Cutler <cutlerb@gmail.com>
## What changes were proposed in this pull request?
Improve the data source v2 API according to the [design doc](https://docs.google.com/document/d/1DDXCTCrup4bKWByTalkXWgavcPdvur8a4eEu8x1BzPM/edit?usp=sharing)
summary of the changes
1. rename `ReadSupport` -> `DataSourceReader` -> `InputPartition` -> `InputPartitionReader` to `BatchReadSupportProvider` -> `BatchReadSupport` -> `InputPartition`/`PartitionReaderFactory` -> `PartitionReader`. Similar renaming also happens at streaming and write APIs.
2. create `ScanConfig` to store query specific information like operator pushdown result, streaming offsets, etc. This makes batch and streaming `ReadSupport`(previouslly named `DataSourceReader`) immutable. All other methods take `ScanConfig` as input, which implies applying operator pushdown and getting streaming offsets happen before all other things(get input partitions, report statistics, etc.).
3. separate `InputPartition` to `InputPartition` and `PartitionReaderFactory`. This is a natural separation, data splitting and reading are orthogonal and we should not mix them in one interfaces. This also makes the naming consistent between read and write API: `PartitionReaderFactory` vs `DataWriterFactory`.
4. separate the batch and streaming interfaces. Sometimes it's painful to force the streaming interface to extend batch interface, as we may need to override some batch methods to return false, or even leak the streaming concept to batch API(e.g. `DataWriterFactory#createWriter(partitionId, taskId, epochId)`)
Some follow-ups we should do after this PR (tracked by https://issues.apache.org/jira/browse/SPARK-25186 ):
1. Revisit the life cycle of `ReadSupport` instances. Currently I keep it same as the previous `DataSourceReader`, i.e. the life cycle is bound to the batch/stream query. This fits streaming very well but may not be perfect for batch source. We can also consider to let `ReadSupport.newScanConfigBuilder` take `DataSourceOptions` as parameter, if we decide to change the life cycle.
2. Add `WriteConfig`. This is similar to `ScanConfig` and makes the write API more flexible. But it's only needed when we add the `replaceWhere` support, and it needs to change the streaming execution engine for this new concept, which I think is better to be done in another PR.
3. Refine the document. This PR adds/changes a lot of document and it's very likely that some people may have better ideas.
4. Figure out the life cycle of `CustomMetrics`. It looks to me that it should be bound to a `ScanConfig`, but we need to change `ProgressReporter` to get the `ScanConfig`. Better to be done in another PR.
5. Better operator pushdown API. This PR keeps the pushdown API as it was, i.e. using the `SupportsPushdownXYZ` traits. We can design a better API using build pattern, but this is a complicated design and deserves an individual JIRA ticket and design doc.
6. Improve the continuous streaming engine to only create a new `ScanConfig` when re-configuring.
7. Remove `SupportsPushdownCatalystFilter`. This is actually not a must-have for file source, we can change the hive partition pruning to use the public `Filter`.
## How was this patch tested?
existing tests.
Closes#22009 from cloud-fan/redesign.
Authored-by: Wenchen Fan <wenchen@databricks.com>
Signed-off-by: Xiao Li <gatorsmile@gmail.com>
## What changes were proposed in this pull request?
The PR moves the compilation of the regexp for code formatting outside the method which is called for each code block when splitting expressions, in order to avoid recompiling the regexp every time.
Credit should be given to Izek Greenfield.
## How was this patch tested?
existing UTs
Closes#22135 from mgaido91/SPARK-25093.
Authored-by: Marco Gaido <marcogaido91@gmail.com>
Signed-off-by: Wenchen Fan <wenchen@databricks.com>
## What changes were proposed in this pull request?
This fixes a perf regression caused by https://github.com/apache/spark/pull/21376 .
We should not use `RDD#toLocalIterator`, which triggers one Spark job per RDD partition. This is very bad for RDDs with a lot of small partitions.
To fix it, this PR introduces a way to access SQLConf in the scheduler event loop thread, so that we don't need to use `RDD#toLocalIterator` anymore in `JsonInferSchema`.
## How was this patch tested?
a new test
Closes#22152 from cloud-fan/conf.
Authored-by: Wenchen Fan <wenchen@databricks.com>
Signed-off-by: Xiao Li <gatorsmile@gmail.com>
## What changes were proposed in this pull request?
This pr is to fix bugs when expr codegen fails; we need to catch `java.util.concurrent.ExecutionException` instead of `InternalCompilerException` and `CompileException` . This handling is the same with the `WholeStageCodegenExec ` one: 60af2501e1/sql/core/src/main/scala/org/apache/spark/sql/execution/WholeStageCodegenExec.scala (L585)
## How was this patch tested?
Added tests in `CodeGeneratorWithInterpretedFallbackSuite`
Closes#22154 from maropu/SPARK-25140.
Authored-by: Takeshi Yamamuro <yamamuro@apache.org>
Signed-off-by: Xiao Li <gatorsmile@gmail.com>
## What changes were proposed in this pull request?
Two back to PRs implicitly conflicted by one PR removing an existing import that the other PR needed. This did not cause explicit conflict as the import already existed, but not used.
https://amplab.cs.berkeley.edu/jenkins/view/Spark%20QA%20Compile/job/spark-master-compile-maven-hadoop-2.7/8226/consoleFull
```
[info] Compiling 342 Scala sources and 97 Java sources to /home/jenkins/workspace/spark-master-compile-maven-hadoop-2.7/sql/core/target/scala-2.11/classes...
[warn] /home/jenkins/workspace/spark-master-compile-maven-hadoop-2.7/sql/core/src/main/scala/org/apache/spark/sql/execution/datasources/parquet/ParquetFileFormat.scala:128: value ENABLE_JOB_SUMMARY in object ParquetOutputFormat is deprecated: see corresponding Javadoc for more information.
[warn] && conf.get(ParquetOutputFormat.ENABLE_JOB_SUMMARY) == null) {
[warn] ^
[error] /home/jenkins/workspace/spark-master-compile-maven-hadoop-2.7/sql/core/src/main/scala/org/apache/spark/sql/execution/streaming/statefulOperators.scala:95: value asJava is not a member of scala.collection.immutable.Map[String,Long]
[error] new java.util.HashMap(customMetrics.mapValues(long2Long).asJava)
[error] ^
[warn] one warning found
[error] one error found
[error] Compile failed at Aug 21, 2018 4:04:35 PM [12.827s]
```
## How was this patch tested?
It compiles!
Closes#22175 from tdas/fix-build.
Authored-by: Tathagata Das <tathagata.das1565@gmail.com>
Signed-off-by: Tathagata Das <tathagata.das1565@gmail.com>
## What changes were proposed in this pull request?
This patch exposes the estimation of size of cache (loadedMaps) in HDFSBackedStateStoreProvider as a custom metric of StateStore.
The rationalize of the patch is that state backed by HDFSBackedStateStoreProvider will consume more memory than the number what we can get from query status due to caching multiple versions of states. The memory footprint to be much larger than query status reports in situations where the state store is getting a lot of updates: while shallow-copying map incurs additional small memory usages due to the size of map entities and references, but row objects will still be shared across the versions. If there're lots of updates between batches, less row objects will be shared and more row objects will exist in memory consuming much memory then what we expect.
While HDFSBackedStateStore refers loadedMaps in HDFSBackedStateStoreProvider directly, there would be only one `StateStoreWriter` which refers a StateStoreProvider, so the value is not exposed as well as being aggregated multiple times. Current state metrics are safe to aggregate for the same reason.
## How was this patch tested?
Tested manually. Below is the snapshot of UI page which is reflected by the patch:
<img width="601" alt="screen shot 2018-06-05 at 10 16 16 pm" src="https://user-images.githubusercontent.com/1317309/40978481-b46ad324-690e-11e8-9b0f-e80528612a62.png">
Please refer "estimated size of states cache in provider total" as well as "count of versions in state cache in provider".
Closes#21469 from HeartSaVioR/SPARK-24441.
Authored-by: Jungtaek Lim <kabhwan@gmail.com>
Signed-off-by: Tathagata Das <tathagata.das1565@gmail.com>
## What changes were proposed in this pull request?
In https://issues.apache.org/jira/browse/SPARK-24924, the data source provider com.databricks.spark.avro is mapped to the new package org.apache.spark.sql.avro .
As per the discussion in the [Jira](https://issues.apache.org/jira/browse/SPARK-24924) and PR #22119, we should make the mapping configurable.
This PR also improve the error message when data source of Avro/Kafka is not found.
## How was this patch tested?
Unit test
Closes#22133 from gengliangwang/configurable_avro_mapping.
Authored-by: Gengliang Wang <gengliang.wang@databricks.com>
Signed-off-by: Xiao Li <gatorsmile@gmail.com>
## What changes were proposed in this pull request?
This patch proposes a new flag option for stateful aggregation: remove redundant key data from value.
Enabling new option runs similar with current, and uses less memory for state according to key/value fields of state operator.
Please refer below link to see detailed perf. test result:
https://issues.apache.org/jira/browse/SPARK-24763?focusedCommentId=16536539&page=com.atlassian.jira.plugin.system.issuetabpanels%3Acomment-tabpanel#comment-16536539
Since the state between enabling the option and disabling the option is not compatible, the option is set to 'disable' by default (to ensure backward compatibility), and OffsetSeqMetadata would prevent modifying the option after executing query.
## How was this patch tested?
Modify unit tests to cover both disabling option and enabling option.
Also did manual tests to see whether propose patch improves state memory usage.
Closes#21733 from HeartSaVioR/SPARK-24763.
Authored-by: Jungtaek Lim <kabhwan@gmail.com>
Signed-off-by: Tathagata Das <tathagata.das1565@gmail.com>
## What changes were proposed in this pull request?
https://github.com/apache/spark/pull/22079#discussion_r209705612 It is possible for two objects to be unequal and yet we consider them as equal with this code, if the long values are separated by Int.MaxValue.
This PR fixes the issue.
## How was this patch tested?
Add new test cases in `RecordBinaryComparatorSuite`.
Closes#22101 from jiangxb1987/fix-rbc.
Authored-by: Xingbo Jiang <xingbo.jiang@databricks.com>
Signed-off-by: Xiao Li <gatorsmile@gmail.com>
## What changes were proposed in this pull request?
Spark SQL returns NULL for a column whose Hive metastore schema and Parquet schema are in different letter cases, regardless of spark.sql.caseSensitive set to true or false. This PR aims to add case-insensitive field resolution for ParquetFileFormat.
* Do case-insensitive resolution only if Spark is in case-insensitive mode.
* Field resolution should fail if there is ambiguity, i.e. more than one field is matched.
## How was this patch tested?
Unit tests added.
Closes#22148 from seancxmao/SPARK-25132-Parquet.
Authored-by: seancxmao <seancxmao@gmail.com>
Signed-off-by: hyukjinkwon <gurwls223@apache.org>
## What changes were proposed in this pull request?
When column pruning is turned on the checking of headers in the csv should only be for the fields in the requiredSchema, not the dataSchema, because column pruning means only requiredSchema is read.
## How was this patch tested?
Added 2 unit tests where column pruning is turned on/off and csv headers are checked againt schema
Please review http://spark.apache.org/contributing.html before opening a pull request.
Closes#22123 from koertkuipers/feat-csv-column-pruning-and-check-header.
Authored-by: Koert Kuipers <koert@tresata.com>
Signed-off-by: hyukjinkwon <gurwls223@apache.org>
## What changes were proposed in this pull request?
[SPARK-25144](https://issues.apache.org/jira/browse/SPARK-25144) reports memory leaks on Apache Spark 2.0.2 ~ 2.3.2-RC5. The bug is already fixed via #21738 as a part of SPARK-21743. This PR only adds a test case to prevent any future regression.
```scala
scala> case class Foo(bar: Option[String])
scala> val ds = List(Foo(Some("bar"))).toDS
scala> val result = ds.flatMap(_.bar).distinct
scala> result.rdd.isEmpty
18/08/19 23:01:54 WARN Executor: Managed memory leak detected; size = 8650752 bytes, TID = 125
res0: Boolean = false
```
## How was this patch tested?
Pass the Jenkins with a new added test case.
Closes#22155 from dongjoon-hyun/SPARK-25144-2.
Authored-by: Dongjoon Hyun <dongjoon@apache.org>
Signed-off-by: hyukjinkwon <gurwls223@apache.org>
## What changes were proposed in this pull request?
In the PR for supporting logical timestamp types https://github.com/apache/spark/pull/21935, a SQL configuration spark.sql.avro.outputTimestampType is added, so that user can specify the output timestamp precision they want.
With PR https://github.com/apache/spark/pull/21847, the output file can be written with user specified types.
So there is no need to have such trivial configuration. Otherwise to make it consistent we need to add configuration for all the Catalyst types that can be converted into different Avro types.
This PR also add a test case for user specified output schema with different timestamp types.
## How was this patch tested?
Unit test
Closes#22151 from gengliangwang/removeOutputTimestampType.
Authored-by: Gengliang Wang <gengliang.wang@databricks.com>
Signed-off-by: hyukjinkwon <gurwls223@apache.org>
## What changes were proposed in this pull request?
We should also check `HigherOrderFunction.bind` method passes expected parameters.
This pr modifies tests for higher-order functions to check `bind` method.
## How was this patch tested?
Modified tests.
Closes#22131 from ueshin/issues/SPARK-25141/bind_test.
Authored-by: Takuya UESHIN <ueshin@databricks.com>
Signed-off-by: Takuya UESHIN <ueshin@databricks.com>
## What changes were proposed in this pull request?
In the PR, I propose to skip invoking of the CSV/JSON parser per each line in the case if the required schema is empty. Added benchmarks for `count()` shows performance improvement up to **3.5 times**.
Before:
```
Count a dataset with 10 columns: Best/Avg Time(ms) Rate(M/s) Per Row(ns)
--------------------------------------------------------------------------------------
JSON count() 7676 / 7715 1.3 767.6
CSV count() 3309 / 3363 3.0 330.9
```
After:
```
Count a dataset with 10 columns: Best/Avg Time(ms) Rate(M/s) Per Row(ns)
--------------------------------------------------------------------------------------
JSON count() 2104 / 2156 4.8 210.4
CSV count() 2332 / 2386 4.3 233.2
```
## How was this patch tested?
It was tested by `CSVSuite` and `JSONSuite` as well as on added benchmarks.
Author: Maxim Gekk <maxim.gekk@databricks.com>
Author: Maxim Gekk <max.gekk@gmail.com>
Closes#21909 from MaxGekk/empty-schema-optimization.
## What changes were proposed in this pull request?
Put annotation args in one line, or API doc generation will fail.
~~~
[error] /Users/meng/src/spark/sql/catalyst/src/main/scala/org/apache/spark/sql/catalyst/expressions/stringExpressions.scala:1559: annotation argument needs to be a constant; found: "_FUNC_(expr) - Returns the character length of string data or number of bytes of ".+("binary data. The length of string data includes the trailing spaces. The length of binary ").+("data includes binary zeros.")
[error] "binary data. The length of string data includes the trailing spaces. The length of binary " +
[error] ^
[info] No documentation generated with unsuccessful compiler run
[error] one error found
[error] (catalyst/compile:doc) Scaladoc generation failed
[error] Total time: 27 s, completed Aug 17, 2018 3:20:08 PM
~~~
## How was this patch tested?
sbt catalyst/compile:doc passed
Closes#22137 from mengxr/minor-doc-fix.
Authored-by: Xiangrui Meng <meng@databricks.com>
Signed-off-by: hyukjinkwon <gurwls223@apache.org>
## What changes were proposed in this pull request?
This is a follow-up pr of #22017 which added `map_zip_with` function.
In the test, when creating a lambda function, we use the `valueContainsNull` values for the nullabilities of the value arguments, but we should've used `true` as the same as `bind` method because the values might be `null` if the keys don't match.
## How was this patch tested?
Added small tests and existing tests.
Closes#22126 from ueshin/issues/SPARK-23938/fix_tests.
Authored-by: Takuya UESHIN <ueshin@databricks.com>
Signed-off-by: Takuya UESHIN <ueshin@databricks.com>
## What changes were proposed in this pull request?
The method ```*supportEquals``` determining whether elements of a data type could be used as items in a hash set or as keys in a hash map is duplicated across multiple collection and higher-order functions.
This PR suggests to deduplicate the method.
## How was this patch tested?
Run tests in:
- DataFrameFunctionsSuite
- CollectionExpressionsSuite
- HigherOrderExpressionsSuite
Closes#22110 from mn-mikke/SPARK-25122.
Authored-by: Marek Novotny <mn.mikke@gmail.com>
Signed-off-by: Wenchen Fan <wenchen@databricks.com>
## What changes were proposed in this pull request?
This pr adds `transform_values` function which applies the function to each entry of the map and transforms the values.
```javascript
> SELECT transform_values(map(array(1, 2, 3), array(1, 2, 3)), (k,v) -> v + 1);
map(1->2, 2->3, 3->4)
> SELECT transform_values(map(array(1, 2, 3), array(1, 2, 3)), (k,v) -> k + v);
map(1->2, 2->4, 3->6)
```
## How was this patch tested?
New Tests added to
`DataFrameFunctionsSuite`
`HigherOrderFunctionsSuite`
`SQLQueryTestSuite`
Closes#22045 from codeatri/SPARK-23940.
Authored-by: codeatri <nehapatil6@gmail.com>
Signed-off-by: Takuya UESHIN <ueshin@databricks.com>
## What changes were proposed in this pull request?
Add RewriteCorrelatedScalarSubquery in the list of nonExcludableRules since its used to transform correlated scalar subqueries to joins.
## How was this patch tested?
Added test in OptimizerRuleExclusionSuite
Author: Dilip Biswal <dbiswal@us.ibm.com>
Closes#22108 from dilipbiswal/scalar_exclusion.
## What changes were proposed in this pull request?
Merges the two given arrays, element-wise, into a single array using function. If one array is shorter, nulls are appended at the end to match the length of the longer array, before applying function:
```
SELECT zip_with(ARRAY[1, 3, 5], ARRAY['a', 'b', 'c'], (x, y) -> (y, x)); -- [ROW('a', 1), ROW('b', 3), ROW('c', 5)]
SELECT zip_with(ARRAY[1, 2], ARRAY[3, 4], (x, y) -> x + y); -- [4, 6]
SELECT zip_with(ARRAY['a', 'b', 'c'], ARRAY['d', 'e', 'f'], (x, y) -> concat(x, y)); -- ['ad', 'be', 'cf']
SELECT zip_with(ARRAY['a'], ARRAY['d', null, 'f'], (x, y) -> coalesce(x, y)); -- ['a', null, 'f']
```
## How was this patch tested?
Added tests
Closes#22031 from techaddict/SPARK-23932.
Authored-by: Sandeep Singh <sandeep@techaddict.me>
Signed-off-by: Takuya UESHIN <ueshin@databricks.com>
## What changes were proposed in this pull request?
This pr adds transform_keys function which applies the function to each entry of the map and transforms the keys.
```javascript
> SELECT transform_keys(map(array(1, 2, 3), array(1, 2, 3)), (k,v) -> k + 1);
map(2->1, 3->2, 4->3)
> SELECT transform_keys(map(array(1, 2, 3), array(1, 2, 3)), (k,v) -> k + v);
map(2->1, 4->2, 6->3)
```
## How was this patch tested?
Added tests.
Closes#22013 from codeatri/SPARK-23939.
Authored-by: codeatri <nehapatil6@gmail.com>
Signed-off-by: Takuya UESHIN <ueshin@databricks.com>
## What changes were proposed in this pull request?
Correct the javadoc for expm1() function.
## How was this patch tested?
None. It is a minor issue.
Closes#22115 from bomeng/25082.
Authored-by: Bo Meng <bo.meng@jd.com>
Signed-off-by: hyukjinkwon <gurwls223@apache.org>
## What changes were proposed in this pull request?
This is split from #21520. This includes changes of `BoundAttribute` and `Cast`.
This patch also adds few convenient APIs:
```scala
CodeGenerator.freshVariable(name: String, dt: DataType): VariableValue
CodeGenerator.freshVariable(name: String, javaClass: Class[_]): VariableValue
JavaCode.javaType(javaClass: Class[_]): Inline
JavaCode.javaType(dataType: DataType): Inline
JavaCode.boxedType(dataType: DataType): Inline
```
## How was this patch tested?
Existing tests.
Closes#21537 from viirya/SPARK-24505-1.
Authored-by: Liang-Chi Hsieh <viirya@gmail.com>
Signed-off-by: hyukjinkwon <gurwls223@apache.org>
## What changes were proposed in this pull request?
Upgrade Apache Arrow to 0.10.0
Version 0.10.0 has a number of bug fixes and improvements with the following pertaining directly to usage in Spark:
* Allow for adding BinaryType support ARROW-2141
* Bug fix related to array serialization ARROW-1973
* Python2 str will be made into an Arrow string instead of bytes ARROW-2101
* Python bytearrays are supported in as input to pyarrow ARROW-2141
* Java has common interface for reset to cleanup complex vectors in Spark ArrowWriter ARROW-1962
* Cleanup pyarrow type equality checks ARROW-2423
* ArrowStreamWriter should not hold references to ArrowBlocks ARROW-2632, ARROW-2645
* Improved low level handling of messages for RecordBatch ARROW-2704
## How was this patch tested?
existing tests
Author: Bryan Cutler <cutlerb@gmail.com>
Closes#21939 from BryanCutler/arrow-upgrade-010.
## What changes were proposed in this pull request?
Add logging for all generated methods from the `CodeGenerator` whose bytecode size goes above 8000 bytes.
This is to help with gathering stats on how often Spark is generating methods too big to be JIT'd. It covers all codegen scenarios, include whole-stage codegen and also individual expression codegen, e.g. unsafe projection, mutable projection, etc.
## How was this patch tested?
Manually tested that logging did happen when generated method was above 8000 bytes.
Also added a new unit test case to `CodeGenerationSuite` to verify that the logging did happen.
Author: Kris Mok <kris.mok@databricks.com>
Closes#22103 from rednaxelafx/codegen-8k-logging.
## What changes were proposed in this pull request?
A small change to print the master and appId from spark-sql as with logging turned down all the way (`log4j.logger.org.apache.spark.sql.hive.thriftserver.SparkSQLCLIDriver=WARN`), we may not know this information easily. This adds the following string before the `spark-sql>` prompt shows on the screen.
`Spark master: yarn, Application Id: application_123456789_12345`
## How was this patch tested?
I ran spark-sql locally and saw the appId displayed as expected.
Please review http://spark.apache.org/contributing.html before opening a pull request.
Closes#22025 from abellina/SPARK-25043_print_master_and_app_id_from_sparksql.
Lead-authored-by: Alessandro Bellina <abellina@gmail.com>
Co-authored-by: Alessandro Bellina <abellina@yahoo-inc.com>
Signed-off-by: Thomas Graves <tgraves@apache.org>
## What changes were proposed in this pull request?
This PR adds a new SQL function called ```map_zip_with```. It merges the two given maps into a single map by applying function to the pair of values with the same key.
## How was this patch tested?
Added new tests into:
- DataFrameFunctionsSuite.scala
- HigherOrderFunctionsSuite.scala
Closes#22017 from mn-mikke/SPARK-23938.
Authored-by: Marek Novotny <mn.mikke@gmail.com>
Signed-off-by: Takuya UESHIN <ueshin@databricks.com>
## What changes were proposed in this pull request?
This PR fixes the an example for `to_json` in doc and function description.
- http://spark.apache.org/docs/2.3.0/api/sql/#to_json
- `describe function extended`
## How was this patch tested?
Pass the Jenkins with the updated test.
Closes#22096 from dongjoon-hyun/minor_json.
Authored-by: Dongjoon Hyun <dongjoon@apache.org>
Signed-off-by: hyukjinkwon <gurwls223@apache.org>
## What changes were proposed in this pull request?
`ANALYZE TABLE ... PARTITION(...) COMPUTE STATISTICS` can fail with a NPE if a partition column contains a NULL value.
The PR avoids the NPE, replacing the `NULL` values with the default partition placeholder.
## How was this patch tested?
added UT
Closes#22036 from mgaido91/SPARK-25028.
Authored-by: Marco Gaido <marcogaido91@gmail.com>
Signed-off-by: Wenchen Fan <wenchen@databricks.com>
## What changes were proposed in this pull request?
This is a follow-up pr of #21954 to address comments.
- Rename ambiguous name `inputs` to `arguments`.
- Add argument type check and remove hacky workaround.
- Address other small comments.
## How was this patch tested?
Existing tests and some additional tests.
Closes#22075 from ueshin/issues/SPARK-23908/fup1.
Authored-by: Takuya UESHIN <ueshin@databricks.com>
Signed-off-by: Wenchen Fan <wenchen@databricks.com>
## What changes were proposed in this pull request?
The PR removes a restriction for element types of array type which exists in `from_json` for the root type. Currently, the function can handle only arrays of structs. Even array of primitive types is disallowed. The PR allows arrays of any types currently supported by JSON datasource. Here is an example of an array of a primitive type:
```
scala> import org.apache.spark.sql.functions._
scala> val df = Seq("[1, 2, 3]").toDF("a")
scala> val schema = new ArrayType(IntegerType, false)
scala> val arr = df.select(from_json($"a", schema))
scala> arr.printSchema
root
|-- jsontostructs(a): array (nullable = true)
| |-- element: integer (containsNull = true)
```
and result of converting of the json string to the `ArrayType`:
```
scala> arr.show
+----------------+
|jsontostructs(a)|
+----------------+
| [1, 2, 3]|
+----------------+
```
## How was this patch tested?
I added a few positive and negative tests:
- array of primitive types
- array of arrays
- array of structs
- array of maps
Closes#21439 from MaxGekk/from_json-array.
Lead-authored-by: Maxim Gekk <maxim.gekk@databricks.com>
Co-authored-by: Maxim Gekk <max.gekk@gmail.com>
Signed-off-by: hyukjinkwon <gurwls223@apache.org>
## What changes were proposed in this pull request?
In type coercion for complex types, if the found type is force-nullable to cast, we should loosen the nullability to be able to cast. Also for map key type, we can't use the type.
## How was this patch tested?
Added some test.
Closes#22086 from ueshin/issues/SPARK-25096/fix_type_coercion.
Authored-by: Takuya UESHIN <ueshin@databricks.com>
Signed-off-by: hyukjinkwon <gurwls223@apache.org>
## What changes were proposed in this pull request?
Support Avro logical date type:
https://avro.apache.org/docs/1.8.2/spec.html#Decimal
## How was this patch tested?
Unit test
Closes#22037 from gengliangwang/avro_decimal.
Authored-by: Gengliang Wang <gengliang.wang@databricks.com>
Signed-off-by: Wenchen Fan <wenchen@databricks.com>
## What changes were proposed in this pull request?
Fix scaladoc in Column
## How was this patch tested?
None
Closes#22069 from sadhen/fix_doc_minor.
Authored-by: 忍冬 <rendong@wacai.com>
Signed-off-by: Sean Owen <srowen@gmail.com>
## What changes were proposed in this pull request?
This PR adds codes to ``"Test `spark.sql.parquet.compression.codec` config"` in `ParquetCompressionCodecPrecedenceSuite`.
## How was this patch tested?
Existing UTs
Closes#22083 from kiszk/ParquetCompressionCodecPrecedenceSuite.
Authored-by: Kazuaki Ishizaki <ishizaki@jp.ibm.com>
Signed-off-by: hyukjinkwon <gurwls223@apache.org>
## What changes were proposed in this pull request?
Add RewriteExceptAll and RewriteIntersectAll in the list of nonExcludableRules as the rewrites are essential for the functioning of EXCEPT ALL and INTERSECT ALL feature.
## How was this patch tested?
Added test in OptimizerRuleExclusionSuite.
Closes#22080 from dilipbiswal/exceptall_rewrite_exclusion.
Authored-by: Dilip Biswal <dbiswal@us.ibm.com>
Signed-off-by: Xiao Li <gatorsmile@gmail.com>
## What changes were proposed in this pull request?
Fixing typos is sometimes very hard. It's not so easy to visually review them. Recently, I discovered a very useful tool for it, [misspell](https://github.com/client9/misspell).
This pull request fixes minor typos detected by [misspell](https://github.com/client9/misspell) except for the false positives. If you would like me to work on other files as well, let me know.
## How was this patch tested?
### before
```
$ misspell . | grep -v '.js'
R/pkg/R/SQLContext.R:354:43: "definiton" is a misspelling of "definition"
R/pkg/R/SQLContext.R:424:43: "definiton" is a misspelling of "definition"
R/pkg/R/SQLContext.R:445:43: "definiton" is a misspelling of "definition"
R/pkg/R/SQLContext.R:495:43: "definiton" is a misspelling of "definition"
NOTICE-binary:454:16: "containd" is a misspelling of "contained"
R/pkg/R/context.R:46:43: "definiton" is a misspelling of "definition"
R/pkg/R/context.R:74:43: "definiton" is a misspelling of "definition"
R/pkg/R/DataFrame.R:591:48: "persistance" is a misspelling of "persistence"
R/pkg/R/streaming.R:166:44: "occured" is a misspelling of "occurred"
R/pkg/inst/worker/worker.R:65:22: "ouput" is a misspelling of "output"
R/pkg/tests/fulltests/test_utils.R:106:25: "environemnt" is a misspelling of "environment"
common/kvstore/src/test/java/org/apache/spark/util/kvstore/InMemoryStoreSuite.java:38:39: "existant" is a misspelling of "existent"
common/kvstore/src/test/java/org/apache/spark/util/kvstore/LevelDBSuite.java:83:39: "existant" is a misspelling of "existent"
common/network-common/src/main/java/org/apache/spark/network/crypto/TransportCipher.java:243:46: "transfered" is a misspelling of "transferred"
common/network-common/src/main/java/org/apache/spark/network/sasl/SaslEncryption.java:234:19: "transfered" is a misspelling of "transferred"
common/network-common/src/main/java/org/apache/spark/network/sasl/SaslEncryption.java:238:63: "transfered" is a misspelling of "transferred"
common/network-common/src/main/java/org/apache/spark/network/sasl/SaslEncryption.java:244:46: "transfered" is a misspelling of "transferred"
common/network-common/src/main/java/org/apache/spark/network/sasl/SaslEncryption.java:276:39: "transfered" is a misspelling of "transferred"
common/network-common/src/main/java/org/apache/spark/network/util/AbstractFileRegion.java:27:20: "transfered" is a misspelling of "transferred"
common/unsafe/src/test/scala/org/apache/spark/unsafe/types/UTF8StringPropertyCheckSuite.scala:195:15: "orgin" is a misspelling of "origin"
core/src/main/scala/org/apache/spark/api/python/PythonRDD.scala:621:39: "gauranteed" is a misspelling of "guaranteed"
core/src/main/scala/org/apache/spark/status/storeTypes.scala:113:29: "ect" is a misspelling of "etc"
core/src/main/scala/org/apache/spark/storage/DiskStore.scala:282:18: "transfered" is a misspelling of "transferred"
core/src/main/scala/org/apache/spark/util/ListenerBus.scala:64:17: "overriden" is a misspelling of "overridden"
core/src/test/scala/org/apache/spark/ShuffleSuite.scala:211:7: "substracted" is a misspelling of "subtracted"
core/src/test/scala/org/apache/spark/scheduler/DAGSchedulerSuite.scala:1922:49: "agriculteur" is a misspelling of "agriculture"
core/src/test/scala/org/apache/spark/scheduler/DAGSchedulerSuite.scala:2468:84: "truely" is a misspelling of "truly"
core/src/test/scala/org/apache/spark/storage/FlatmapIteratorSuite.scala:25:18: "persistance" is a misspelling of "persistence"
core/src/test/scala/org/apache/spark/storage/FlatmapIteratorSuite.scala:26:69: "persistance" is a misspelling of "persistence"
data/streaming/AFINN-111.txt:1219:0: "humerous" is a misspelling of "humorous"
dev/run-pip-tests:55:28: "enviroments" is a misspelling of "environments"
dev/run-pip-tests:91:37: "virutal" is a misspelling of "virtual"
dev/merge_spark_pr.py:377:72: "accross" is a misspelling of "across"
dev/merge_spark_pr.py:378:66: "accross" is a misspelling of "across"
dev/run-pip-tests:126:25: "enviroments" is a misspelling of "environments"
docs/configuration.md:1830:82: "overriden" is a misspelling of "overridden"
docs/structured-streaming-programming-guide.md:525:45: "processs" is a misspelling of "processes"
docs/structured-streaming-programming-guide.md:1165:61: "BETWEN" is a misspelling of "BETWEEN"
docs/sql-programming-guide.md:1891:810: "behaivor" is a misspelling of "behavior"
examples/src/main/python/sql/arrow.py:98:8: "substract" is a misspelling of "subtract"
examples/src/main/python/sql/arrow.py:103:27: "substract" is a misspelling of "subtract"
licenses/LICENSE-heapq.txt:5:63: "Stichting" is a misspelling of "Stitching"
licenses/LICENSE-heapq.txt:6:2: "Mathematisch" is a misspelling of "Mathematics"
licenses/LICENSE-heapq.txt:262:29: "Stichting" is a misspelling of "Stitching"
licenses/LICENSE-heapq.txt:262:39: "Mathematisch" is a misspelling of "Mathematics"
licenses/LICENSE-heapq.txt:269:49: "Stichting" is a misspelling of "Stitching"
licenses/LICENSE-heapq.txt:269:59: "Mathematisch" is a misspelling of "Mathematics"
licenses/LICENSE-heapq.txt:274:2: "STICHTING" is a misspelling of "STITCHING"
licenses/LICENSE-heapq.txt:274:12: "MATHEMATISCH" is a misspelling of "MATHEMATICS"
licenses/LICENSE-heapq.txt:276:29: "STICHTING" is a misspelling of "STITCHING"
licenses/LICENSE-heapq.txt:276:39: "MATHEMATISCH" is a misspelling of "MATHEMATICS"
licenses-binary/LICENSE-heapq.txt:5:63: "Stichting" is a misspelling of "Stitching"
licenses-binary/LICENSE-heapq.txt:6:2: "Mathematisch" is a misspelling of "Mathematics"
licenses-binary/LICENSE-heapq.txt:262:29: "Stichting" is a misspelling of "Stitching"
licenses-binary/LICENSE-heapq.txt:262:39: "Mathematisch" is a misspelling of "Mathematics"
licenses-binary/LICENSE-heapq.txt:269:49: "Stichting" is a misspelling of "Stitching"
licenses-binary/LICENSE-heapq.txt:269:59: "Mathematisch" is a misspelling of "Mathematics"
licenses-binary/LICENSE-heapq.txt:274:2: "STICHTING" is a misspelling of "STITCHING"
licenses-binary/LICENSE-heapq.txt:274:12: "MATHEMATISCH" is a misspelling of "MATHEMATICS"
licenses-binary/LICENSE-heapq.txt:276:29: "STICHTING" is a misspelling of "STITCHING"
licenses-binary/LICENSE-heapq.txt:276:39: "MATHEMATISCH" is a misspelling of "MATHEMATICS"
mllib/src/main/resources/org/apache/spark/ml/feature/stopwords/hungarian.txt:170:0: "teh" is a misspelling of "the"
mllib/src/main/resources/org/apache/spark/ml/feature/stopwords/portuguese.txt:53:0: "eles" is a misspelling of "eels"
mllib/src/main/scala/org/apache/spark/ml/stat/Summarizer.scala:99:20: "Euclidian" is a misspelling of "Euclidean"
mllib/src/main/scala/org/apache/spark/ml/stat/Summarizer.scala:539:11: "Euclidian" is a misspelling of "Euclidean"
mllib/src/main/scala/org/apache/spark/mllib/clustering/LDAOptimizer.scala:77:36: "Teh" is a misspelling of "The"
mllib/src/main/scala/org/apache/spark/mllib/clustering/StreamingKMeans.scala:230:24: "inital" is a misspelling of "initial"
mllib/src/main/scala/org/apache/spark/mllib/stat/MultivariateOnlineSummarizer.scala:276:9: "Euclidian" is a misspelling of "Euclidean"
mllib/src/test/scala/org/apache/spark/ml/clustering/KMeansSuite.scala:237:26: "descripiton" is a misspelling of "descriptions"
python/pyspark/find_spark_home.py:30:13: "enviroment" is a misspelling of "environment"
python/pyspark/context.py:937:12: "supress" is a misspelling of "suppress"
python/pyspark/context.py:938:12: "supress" is a misspelling of "suppress"
python/pyspark/context.py:939:12: "supress" is a misspelling of "suppress"
python/pyspark/context.py:940:12: "supress" is a misspelling of "suppress"
python/pyspark/heapq3.py:6:63: "Stichting" is a misspelling of "Stitching"
python/pyspark/heapq3.py:7:2: "Mathematisch" is a misspelling of "Mathematics"
python/pyspark/heapq3.py:263:29: "Stichting" is a misspelling of "Stitching"
python/pyspark/heapq3.py:263:39: "Mathematisch" is a misspelling of "Mathematics"
python/pyspark/heapq3.py:270:49: "Stichting" is a misspelling of "Stitching"
python/pyspark/heapq3.py:270:59: "Mathematisch" is a misspelling of "Mathematics"
python/pyspark/heapq3.py:275:2: "STICHTING" is a misspelling of "STITCHING"
python/pyspark/heapq3.py:275:12: "MATHEMATISCH" is a misspelling of "MATHEMATICS"
python/pyspark/heapq3.py:277:29: "STICHTING" is a misspelling of "STITCHING"
python/pyspark/heapq3.py:277:39: "MATHEMATISCH" is a misspelling of "MATHEMATICS"
python/pyspark/heapq3.py:713:8: "probabilty" is a misspelling of "probability"
python/pyspark/ml/clustering.py:1038:8: "Currenlty" is a misspelling of "Currently"
python/pyspark/ml/stat.py:339:23: "Euclidian" is a misspelling of "Euclidean"
python/pyspark/ml/regression.py:1378:20: "paramter" is a misspelling of "parameter"
python/pyspark/mllib/stat/_statistics.py:262:8: "probabilty" is a misspelling of "probability"
python/pyspark/rdd.py:1363:32: "paramter" is a misspelling of "parameter"
python/pyspark/streaming/tests.py:825:42: "retuns" is a misspelling of "returns"
python/pyspark/sql/tests.py:768:29: "initalization" is a misspelling of "initialization"
python/pyspark/sql/tests.py:3616:31: "initalize" is a misspelling of "initialize"
resource-managers/mesos/src/main/scala/org/apache/spark/scheduler/cluster/mesos/MesosSchedulerBackendUtil.scala:120:39: "arbitary" is a misspelling of "arbitrary"
resource-managers/mesos/src/test/scala/org/apache/spark/deploy/mesos/MesosClusterDispatcherArgumentsSuite.scala:26:45: "sucessfully" is a misspelling of "successfully"
resource-managers/mesos/src/main/scala/org/apache/spark/scheduler/cluster/mesos/MesosSchedulerUtils.scala:358:27: "constaints" is a misspelling of "constraints"
resource-managers/yarn/src/test/scala/org/apache/spark/deploy/yarn/YarnClusterSuite.scala:111:24: "senstive" is a misspelling of "sensitive"
sql/catalyst/src/main/scala/org/apache/spark/sql/catalyst/catalog/SessionCatalog.scala:1063:5: "overwirte" is a misspelling of "overwrite"
sql/catalyst/src/main/scala/org/apache/spark/sql/catalyst/expressions/datetimeExpressions.scala:1348:17: "compatability" is a misspelling of "compatibility"
sql/catalyst/src/main/scala/org/apache/spark/sql/catalyst/plans/logical/basicLogicalOperators.scala:77:36: "paramter" is a misspelling of "parameter"
sql/catalyst/src/main/scala/org/apache/spark/sql/internal/SQLConf.scala:1374:22: "precendence" is a misspelling of "precedence"
sql/catalyst/src/test/scala/org/apache/spark/sql/catalyst/analysis/AnalysisSuite.scala:238:27: "unnecassary" is a misspelling of "unnecessary"
sql/catalyst/src/test/scala/org/apache/spark/sql/catalyst/expressions/ConditionalExpressionSuite.scala:212:17: "whn" is a misspelling of "when"
sql/core/src/main/scala/org/apache/spark/sql/execution/streaming/StreamingSymmetricHashJoinHelper.scala:147:60: "timestmap" is a misspelling of "timestamp"
sql/core/src/test/scala/org/apache/spark/sql/TPCDSQuerySuite.scala:150:45: "precentage" is a misspelling of "percentage"
sql/core/src/test/scala/org/apache/spark/sql/execution/datasources/csv/CSVInferSchemaSuite.scala:135:29: "infered" is a misspelling of "inferred"
sql/hive/src/test/resources/golden/udf_instr-1-2e76f819563dbaba4beb51e3a130b922:1:52: "occurance" is a misspelling of "occurrence"
sql/hive/src/test/resources/golden/udf_instr-2-32da357fc754badd6e3898dcc8989182:1:52: "occurance" is a misspelling of "occurrence"
sql/hive/src/test/resources/golden/udf_locate-1-6e41693c9c6dceea4d7fab4c02884e4e:1:63: "occurance" is a misspelling of "occurrence"
sql/hive/src/test/resources/golden/udf_locate-2-d9b5934457931447874d6bb7c13de478:1:63: "occurance" is a misspelling of "occurrence"
sql/hive/src/test/resources/golden/udf_translate-2-f7aa38a33ca0df73b7a1e6b6da4b7fe8:9:79: "occurence" is a misspelling of "occurrence"
sql/hive/src/test/resources/golden/udf_translate-2-f7aa38a33ca0df73b7a1e6b6da4b7fe8:13:110: "occurence" is a misspelling of "occurrence"
sql/hive/src/test/resources/ql/src/test/queries/clientpositive/annotate_stats_join.q:46:105: "distint" is a misspelling of "distinct"
sql/hive/src/test/resources/ql/src/test/queries/clientpositive/auto_sortmerge_join_11.q:29:3: "Currenly" is a misspelling of "Currently"
sql/hive/src/test/resources/ql/src/test/queries/clientpositive/avro_partitioned.q:72:15: "existant" is a misspelling of "existent"
sql/hive/src/test/resources/ql/src/test/queries/clientpositive/decimal_udf.q:25:3: "substraction" is a misspelling of "subtraction"
sql/hive/src/test/resources/ql/src/test/queries/clientpositive/groupby2_map_multi_distinct.q:16:51: "funtion" is a misspelling of "function"
sql/hive/src/test/resources/ql/src/test/queries/clientpositive/groupby_sort_8.q:15:30: "issueing" is a misspelling of "issuing"
sql/hive/src/test/scala/org/apache/spark/sql/sources/HadoopFsRelationTest.scala:669:52: "wiht" is a misspelling of "with"
sql/hive-thriftserver/src/main/java/org/apache/hive/service/cli/session/HiveSessionImpl.java:474:9: "Refering" is a misspelling of "Referring"
```
### after
```
$ misspell . | grep -v '.js'
common/network-common/src/main/java/org/apache/spark/network/util/AbstractFileRegion.java:27:20: "transfered" is a misspelling of "transferred"
core/src/main/scala/org/apache/spark/status/storeTypes.scala:113:29: "ect" is a misspelling of "etc"
core/src/test/scala/org/apache/spark/scheduler/DAGSchedulerSuite.scala:1922:49: "agriculteur" is a misspelling of "agriculture"
data/streaming/AFINN-111.txt:1219:0: "humerous" is a misspelling of "humorous"
licenses/LICENSE-heapq.txt:5:63: "Stichting" is a misspelling of "Stitching"
licenses/LICENSE-heapq.txt:6:2: "Mathematisch" is a misspelling of "Mathematics"
licenses/LICENSE-heapq.txt:262:29: "Stichting" is a misspelling of "Stitching"
licenses/LICENSE-heapq.txt:262:39: "Mathematisch" is a misspelling of "Mathematics"
licenses/LICENSE-heapq.txt:269:49: "Stichting" is a misspelling of "Stitching"
licenses/LICENSE-heapq.txt:269:59: "Mathematisch" is a misspelling of "Mathematics"
licenses/LICENSE-heapq.txt:274:2: "STICHTING" is a misspelling of "STITCHING"
licenses/LICENSE-heapq.txt:274:12: "MATHEMATISCH" is a misspelling of "MATHEMATICS"
licenses/LICENSE-heapq.txt:276:29: "STICHTING" is a misspelling of "STITCHING"
licenses/LICENSE-heapq.txt:276:39: "MATHEMATISCH" is a misspelling of "MATHEMATICS"
licenses-binary/LICENSE-heapq.txt:5:63: "Stichting" is a misspelling of "Stitching"
licenses-binary/LICENSE-heapq.txt:6:2: "Mathematisch" is a misspelling of "Mathematics"
licenses-binary/LICENSE-heapq.txt:262:29: "Stichting" is a misspelling of "Stitching"
licenses-binary/LICENSE-heapq.txt:262:39: "Mathematisch" is a misspelling of "Mathematics"
licenses-binary/LICENSE-heapq.txt:269:49: "Stichting" is a misspelling of "Stitching"
licenses-binary/LICENSE-heapq.txt:269:59: "Mathematisch" is a misspelling of "Mathematics"
licenses-binary/LICENSE-heapq.txt:274:2: "STICHTING" is a misspelling of "STITCHING"
licenses-binary/LICENSE-heapq.txt:274:12: "MATHEMATISCH" is a misspelling of "MATHEMATICS"
licenses-binary/LICENSE-heapq.txt:276:29: "STICHTING" is a misspelling of "STITCHING"
licenses-binary/LICENSE-heapq.txt:276:39: "MATHEMATISCH" is a misspelling of "MATHEMATICS"
mllib/src/main/resources/org/apache/spark/ml/feature/stopwords/hungarian.txt:170:0: "teh" is a misspelling of "the"
mllib/src/main/resources/org/apache/spark/ml/feature/stopwords/portuguese.txt:53:0: "eles" is a misspelling of "eels"
mllib/src/main/scala/org/apache/spark/ml/stat/Summarizer.scala:99:20: "Euclidian" is a misspelling of "Euclidean"
mllib/src/main/scala/org/apache/spark/ml/stat/Summarizer.scala:539:11: "Euclidian" is a misspelling of "Euclidean"
mllib/src/main/scala/org/apache/spark/mllib/clustering/LDAOptimizer.scala:77:36: "Teh" is a misspelling of "The"
mllib/src/main/scala/org/apache/spark/mllib/stat/MultivariateOnlineSummarizer.scala:276:9: "Euclidian" is a misspelling of "Euclidean"
python/pyspark/heapq3.py:6:63: "Stichting" is a misspelling of "Stitching"
python/pyspark/heapq3.py:7:2: "Mathematisch" is a misspelling of "Mathematics"
python/pyspark/heapq3.py:263:29: "Stichting" is a misspelling of "Stitching"
python/pyspark/heapq3.py:263:39: "Mathematisch" is a misspelling of "Mathematics"
python/pyspark/heapq3.py:270:49: "Stichting" is a misspelling of "Stitching"
python/pyspark/heapq3.py:270:59: "Mathematisch" is a misspelling of "Mathematics"
python/pyspark/heapq3.py:275:2: "STICHTING" is a misspelling of "STITCHING"
python/pyspark/heapq3.py:275:12: "MATHEMATISCH" is a misspelling of "MATHEMATICS"
python/pyspark/heapq3.py:277:29: "STICHTING" is a misspelling of "STITCHING"
python/pyspark/heapq3.py:277:39: "MATHEMATISCH" is a misspelling of "MATHEMATICS"
python/pyspark/ml/stat.py:339:23: "Euclidian" is a misspelling of "Euclidean"
```
Closes#22070 from seratch/fix-typo.
Authored-by: Kazuhiro Sera <seratch@gmail.com>
Signed-off-by: Sean Owen <srowen@gmail.com>
## What changes were proposed in this pull request?
"distribute by" on multiple columns (wrap in brackets) may lead to codegen issue.
Simple way to reproduce:
```scala
val df = spark.range(1000)
val columns = (0 until 400).map{ i => s"id as id$i" }
val distributeExprs = (0 until 100).map(c => s"id$c").mkString(",")
df.selectExpr(columns : _*).createTempView("test")
spark.sql(s"select * from test distribute by ($distributeExprs)").count()
```
## How was this patch tested?
Add UT.
Closes#22066 from yucai/SPARK-25084.
Authored-by: yucai <yyu1@ebay.com>
Signed-off-by: Wenchen Fan <wenchen@databricks.com>
## What changes were proposed in this pull request?
Parquet file provides six codecs: "snappy", "gzip", "lzo", "lz4", "brotli", "zstd".
This pr add missing compression codec :"lz4", "brotli", "zstd" .
## How was this patch tested?
N/A
Closes#22068 from 10110346/nosupportlz4.
Authored-by: liuxian <liu.xian3@zte.com.cn>
Signed-off-by: hyukjinkwon <gurwls223@apache.org>
## What changes were proposed in this pull request?
A logical `Limit` is performed physically by two operations `LocalLimit` and `GlobalLimit`.
Most of time, we gather all data into a single partition in order to run `GlobalLimit`. If we use a very big limit number, shuffling data causes performance issue also reduces parallelism.
We can avoid shuffling into single partition if we don't care data ordering. This patch implements this idea by doing a map stage during global limit. It collects the info of row numbers at each partition. For each partition, we locally retrieves limited data without any shuffling to finish this global limit.
For example, we have three partitions with rows (100, 100, 50) respectively. In global limit of 100 rows, we may take (34, 33, 33) rows for each partition locally. After global limit we still have three partitions.
If the data partition has certain ordering, we can't distribute required rows evenly to each partitions because it could change data ordering. But we still can avoid shuffling.
## How was this patch tested?
Jenkins tests.
Author: Liang-Chi Hsieh <viirya@gmail.com>
Closes#16677 from viirya/improve-global-limit-parallelism.
## What changes were proposed in this pull request?
Support for text socket stream in spark structured streaming "continuous" mode. This is roughly based on the idea of ContinuousMemoryStream where the executor queries the data from driver over an RPC endpoint.
This makes it possible to create Structured streaming continuous pipeline to ingest data via "nc" and run examples.
## How was this patch tested?
Unit test and ran spark examples in structured streaming continuous mode.
Please review http://spark.apache.org/contributing.html before opening a pull request.
Closes#21199 from arunmahadevan/SPARK-24127.
Authored-by: Arun Mahadevan <arunm@apache.org>
Signed-off-by: hyukjinkwon <gurwls223@apache.org>
## What changes were proposed in this pull request?
This PR simplified code generation for `ArrayDistinct`. #21966 enabled code generation only if the type can be specialized by the hash set. This PR follows this strategy.
Optimization of null handling will be implemented in #21912.
## How was this patch tested?
Existing UTs
Closes#22044 from kiszk/SPARK-23912-follow.
Authored-by: Kazuaki Ishizaki <ishizaki@jp.ibm.com>
Signed-off-by: Takuya UESHIN <ueshin@databricks.com>
## What changes were proposed in this pull request?
This is a follow-up to #21305 that adds a test suite for AppendData analysis.
This also fixes the following problems uncovered by these tests:
* Incorrect order of data types passed to `canWrite` is fixed
* The field check calls `canWrite` first to ensure all errors are found
* `AppendData#resolved` must check resolution of the query's attributes
* Column names are quoted to show empty names
## How was this patch tested?
This PR adds a test suite for AppendData analysis.
Closes#22043 from rdblue/SPARK-24251-add-append-data-analysis-tests.
Authored-by: Ryan Blue <blue@apache.org>
Signed-off-by: Wenchen Fan <wenchen@databricks.com>
## What changes were proposed in this pull request?
This pr adds `exists` function which tests whether a predicate holds for one or more elements in the array.
```sql
> SELECT exists(array(1, 2, 3), x -> x % 2 == 0);
true
```
## How was this patch tested?
Added tests.
Closes#22052 from ueshin/issues/SPARK-25068/exists.
Authored-by: Takuya UESHIN <ueshin@databricks.com>
Signed-off-by: Xiao Li <gatorsmile@gmail.com>
## What changes were proposed in this pull request?
When a `SparkSession` is stopped, `SQLConf.get` should use the fallback conf to avoid weird issues like
```
sbt.ForkMain$ForkError: java.lang.IllegalStateException: LiveListenerBus is stopped.
at org.apache.spark.scheduler.LiveListenerBus.addToQueue(LiveListenerBus.scala:97)
at org.apache.spark.scheduler.LiveListenerBus.addToStatusQueue(LiveListenerBus.scala:80)
at org.apache.spark.sql.internal.SharedState.<init>(SharedState.scala:93)
at org.apache.spark.sql.SparkSession$$anonfun$sharedState$1.apply(SparkSession.scala:120)
at org.apache.spark.sql.SparkSession$$anonfun$sharedState$1.apply(SparkSession.scala:120)
at scala.Option.getOrElse(Option.scala:121)
...
```
## How was this patch tested?
a new test suite
Closes#22056 from cloud-fan/session.
Authored-by: Wenchen Fan <wenchen@databricks.com>
Signed-off-by: Xiao Li <gatorsmile@gmail.com>
## What changes were proposed in this pull request?
Just delete the unused variable `inputFields` in WindowExec, avoid making others confused while reading the code.
## How was this patch tested?
Existing UT.
Closes#22057 from xuanyuanking/SPARK-25077.
Authored-by: liyuanjian <liyuanjian@baidu.com>
Signed-off-by: Xiao Li <gatorsmile@gmail.com>
## What changes were proposed in this pull request?
Currently, Analyze table calculates table size sequentially for each partition. We can parallelize size calculations over partitions.
Results : Tested on a table with 100 partitions and data stored in S3.
With changes :
- 10.429s
- 10.557s
- 10.439s
- 9.893s
Without changes :
- 110.034s
- 99.510s
- 100.743s
- 99.106s
## How was this patch tested?
Simple unit test.
Closes#21608 from Achuth17/improveAnalyze.
Lead-authored-by: Achuth17 <Achuth.narayan@gmail.com>
Co-authored-by: arajagopal17 <arajagopal@qubole.com>
Signed-off-by: Xiao Li <gatorsmile@gmail.com>
## What changes were proposed in this pull request?
Correct the class name typo checked in through SPARK-24891
## How was this patch tested?
Passed all existing tests.
Closes#22049 from maryannxue/known-not-null.
Authored-by: maryannxue <maryannxue@apache.org>
Signed-off-by: Xiao Li <gatorsmile@gmail.com>
## What changes were proposed in this pull request?
This PR addresses two issues in `BufferHolderSparkSubmitSuite`.
1. While `BufferHolderSparkSubmitSuite` tried to allocate a large object several times, it actually allocated an object once and reused the object.
2. `BufferHolderSparkSubmitSuite` may fail due to timeout
To assign a small object before allocating a large object each time solved issue 1 by avoiding reuse.
To increasing heap size from 4g to 7g solved issue 2. It can also avoid OOM after fixing issue 1.
## How was this patch tested?
Updated existing `BufferHolderSparkSubmitSuite`
Closes#20636 from kiszk/SPARK-23415.
Authored-by: Kazuaki Ishizaki <ishizaki@jp.ibm.com>
Signed-off-by: Wenchen Fan <wenchen@databricks.com>
## What changes were proposed in this pull request?
This PR fixes typo regarding `auxiliary verb + verb[s]`. This is a follow-on of #21956.
## How was this patch tested?
N/A
Closes#22040 from kiszk/spellcheck1.
Authored-by: Kazuaki Ishizaki <ishizaki@jp.ibm.com>
Signed-off-by: hyukjinkwon <gurwls223@apache.org>
## What changes were proposed in this pull request?
We should use `Block.isEmpty/nonEmpty` instead of comparing with empty string to check whether the code is empty or not.
```
[error] [warn] /.../sql/core/src/main/scala/org/apache/spark/sql/execution/WholeStageCodegenExec.scala:278: org.apache.spark.sql.catalyst.expressions.codegen.Block and String are unrelated: they will most likely always compare unequal
[error] [warn] if (ev.code != "" && required.contains(attributes(i))) {
[error] [warn]
[error] [warn] /.../sql/core/src/main/scala/org/apache/spark/sql/execution/joins/BroadcastHashJoinExec.scala:323: org.apache.spark.sql.catalyst.expressions.codegen.Block and String are unrelated: they will most likely never compare equal
[error] [warn] | ${buildVars.filter(_.code == "").map(v => s"${v.isNull} = true;").mkString("\n")}
[error] [warn]
```
## How was this patch tested?
Existing tests.
Closes#22041 from ueshin/issues/SPARK-25058/fix_comparison.
Authored-by: Takuya UESHIN <ueshin@databricks.com>
Signed-off-by: Takuya UESHIN <ueshin@databricks.com>
## What changes were proposed in this pull request?
We have completed a significant subset of the object related Expressions to provide an interpreted fallback. This PR is going to modify the tests to also test the interpreted code paths.
One concern right now is that by testing the interpreted code paths too, we will double current test time or more. Otherwise, we can only choose to test the interpreted code paths for just few test suites such as encoder related.
## How was this patch tested?
Existing tests.
Closes#21535 from viirya/SPARK-23596.
Authored-by: Liang-Chi Hsieh <viirya@gmail.com>
Signed-off-by: hyukjinkwon <gurwls223@apache.org>
## What changes were proposed in this pull request?
This is a follow-up pr of #22014.
We still have some more compilation errors in scala-2.12 with sbt:
```
[error] [warn] /.../sql/core/src/main/scala/org/apache/spark/sql/DataFrameNaFunctions.scala:493: match may not be exhaustive.
[error] It would fail on the following input: (_, _)
[error] [warn] val typeMatches = (targetType, f.dataType) match {
[error] [warn]
[error] [warn] /.../sql/core/src/main/scala/org/apache/spark/sql/execution/streaming/MicroBatchExecution.scala:393: match may not be exhaustive.
[error] It would fail on the following input: (_, _)
[error] [warn] prevBatchOff.get.toStreamProgress(sources).foreach {
[error] [warn]
[error] [warn] /.../sql/core/src/main/scala/org/apache/spark/sql/execution/aggregate/AggUtils.scala:173: match may not be exhaustive.
[error] It would fail on the following input: AggregateExpression(_, _, false, _)
[error] [warn] val rewrittenDistinctFunctions = functionsWithDistinct.map {
[error] [warn]
[error] [warn] /.../sql/core/src/main/scala/org/apache/spark/sql/execution/streaming/state/SymmetricHashJoinStateManager.scala:271: match may not be exhaustive.
[error] It would fail on the following input: (_, _)
[error] [warn] keyWithIndexToValueMetrics.customMetrics.map {
[error] [warn]
[error] [warn] /.../sql/core/src/main/scala/org/apache/spark/sql/execution/command/tables.scala:959: match may not be exhaustive.
[error] It would fail on the following input: CatalogTableType(_)
[error] [warn] val tableTypeString = metadata.tableType match {
[error] [warn]
[error] [warn] /.../sql/hive/src/main/scala/org/apache/spark/sql/hive/client/HiveClientImpl.scala:923: match may not be exhaustive.
[error] It would fail on the following input: CatalogTableType(_)
[error] [warn] hiveTable.setTableType(table.tableType match {
[error] [warn]
```
## How was this patch tested?
Manually build with Scala-2.12.
Closes#22039 from ueshin/issues/SPARK-25036/fix_match.
Authored-by: Takuya UESHIN <ueshin@databricks.com>
Signed-off-by: Sean Owen <srowen@gmail.com>
## What changes were proposed in this pull request?
This pr is a follow-up pr of #21982 and fixes the examples.
## How was this patch tested?
Existing tests.
Closes#22035 from ueshin/issues/SPARK-23911/fup1.
Authored-by: Takuya UESHIN <ueshin@databricks.com>
Signed-off-by: Takuya UESHIN <ueshin@databricks.com>
## What changes were proposed in this pull request?
The PR remove the following compilation error using scala-2.12 with sbt by adding a default case to `match`.
```
/home/ishizaki/Spark/PR/scala212/spark/sql/catalyst/src/main/scala/org/apache/spark/sql/catalyst/plans/logical/statsEstimation/ValueInterval.scala:63: match may not be exhaustive.
[error] It would fail on the following inputs: (NumericValueInterval(_, _), _), (_, NumericValueInterval(_, _)), (_, _)
[error] [warn] def isIntersected(r1: ValueInterval, r2: ValueInterval): Boolean = (r1, r2) match {
[error] [warn]
[error] [warn] /home/ishizaki/Spark/PR/scala212/spark/sql/catalyst/src/main/scala/org/apache/spark/sql/catalyst/plans/logical/statsEstimation/ValueInterval.scala:79: match may not be exhaustive.
[error] It would fail on the following inputs: (NumericValueInterval(_, _), _), (_, NumericValueInterval(_, _)), (_, _)
[error] [warn] (r1, r2) match {
[error] [warn]
[error] [warn] /home/ishizaki/Spark/PR/scala212/spark/sql/catalyst/src/main/scala/org/apache/spark/sql/catalyst/expressions/aggregate/ApproxCountDistinctForIntervals.scala:67: match may not be exhaustive.
[error] It would fail on the following inputs: (ArrayType(_, _), _), (_, ArrayData()), (_, _)
[error] [warn] (endpointsExpression.dataType, endpointsExpression.eval()) match {
[error] [warn]
[error] [warn] /home/ishizaki/Spark/PR/scala212/spark/sql/catalyst/src/main/scala/org/apache/spark/sql/catalyst/expressions/codegen/CodeGenerator.scala:470: match may not be exhaustive.
[error] It would fail on the following inputs: NewFunctionSpec(_, None, Some(_)), NewFunctionSpec(_, Some(_), None)
[error] [warn] newFunction match {
[error] [warn]
[error] [warn] [error] [warn] /home/ishizaki/Spark/PR/scala212/spark/sql/catalyst/src/main/scala/org/apache/spark/sql/catalyst/ScalaReflection.scala:709: match may not be exhaustive.
[error] It would fail on the following input: Schema((x: org.apache.spark.sql.types.DataType forSome x not in org.apache.spark.sql.types.StructType), _)
[error] [warn] def attributesFor[T: TypeTag]: Seq[Attribute] = schemaFor[T] match {
[error] [warn]
```
## How was this patch tested?
Existing UTs with Scala-2.11.
Manually build with Scala-2.12
Closes#22014 from kiszk/SPARK-25036b.
Authored-by: Kazuaki Ishizaki <ishizaki@jp.ibm.com>
Signed-off-by: hyukjinkwon <gurwls223@apache.org>
## What changes were proposed in this pull request?
This PR fixes an exception during the compilation of generated code of `mapEntry`. This error occurs since the current code uses `key` type to store a `value` when `key` and `value` types are primitive type.
```
val mid0 = Literal.create(Map(1 -> 1.1, 2 -> 2.2), MapType(IntegerType, DoubleType))
checkEvaluation(MapEntries(mid0), Seq(r(1, 1.1), r(2, 2.2)))
```
```
[info] Code generation of map_entries(keys: [1,2], values: [1.1,2.2]) failed:
[info] java.util.concurrent.ExecutionException: org.codehaus.commons.compiler.CompileException: File 'generated.java', Line 80, Column 20: failed to compile: org.codehaus.commons.compiler.CompileException: File 'generated.java', Line 80, Column 20: No applicable constructor/method found for actual parameters "int, double"; candidates are: "public void org.apache.spark.sql.catalyst.expressions.UnsafeRow.setInt(int, int)", "public void org.apache.spark.sql.catalyst.InternalRow.setInt(int, int)"
[info] java.util.concurrent.ExecutionException: org.codehaus.commons.compiler.CompileException: File 'generated.java', Line 80, Column 20: failed to compile: org.codehaus.commons.compiler.CompileException: File 'generated.java', Line 80, Column 20: No applicable constructor/method found for actual parameters "int, double"; candidates are: "public void org.apache.spark.sql.catalyst.expressions.UnsafeRow.setInt(int, int)", "public void org.apache.spark.sql.catalyst.InternalRow.setInt(int, int)"
[info] at com.google.common.util.concurrent.AbstractFuture$Sync.getValue(AbstractFuture.java:306)
[info] at com.google.common.util.concurrent.AbstractFuture$Sync.get(AbstractFuture.java:293)
[info] at com.google.common.util.concurrent.AbstractFuture.get(AbstractFuture.java:116)
[info] at com.google.common.util.concurrent.Uninterruptibles.getUninterruptibly(Uninterruptibles.java:135)
[info] at com.google.common.cache.LocalCache$Segment.getAndRecordStats(LocalCache.java:2410)
[info] at com.google.common.cache.LocalCache$Segment.loadSync(LocalCache.java:2380)
[info] at com.google.common.cache.LocalCache$Segment.lockedGetOrLoad(LocalCache.java:2342)
[info] at com.google.common.cache.LocalCache$Segment.get(LocalCache.java:2257)
[info] at com.google.common.cache.LocalCache.get(LocalCache.java:4000)
[info] at com.google.common.cache.LocalCache.getOrLoad(LocalCache.java:4004)
[info] at com.google.common.cache.LocalCache$LocalLoadingCache.get(LocalCache.java:4874)
[info] at org.apache.spark.sql.catalyst.expressions.codegen.CodeGenerator$.compile(CodeGenerator.scala:1290)
...
```
## How was this patch tested?
Added a new test to `CollectionExpressionsSuite`
Closes#22033 from kiszk/SPARK-23935-followup.
Authored-by: Kazuaki Ishizaki <ishizaki@jp.ibm.com>
Signed-off-by: Takuya UESHIN <ueshin@databricks.com>
## What changes were proposed in this pull request?
This is a follow-up pr of #21980.
`Shuffle` can also be `ExpressionWithRandomSeed` to produce different values for each execution in streaming query.
## How was this patch tested?
Added a test.
Closes#22027 from ueshin/issues/SPARK-25010/random_seed.
Authored-by: Takuya UESHIN <ueshin@databricks.com>
Signed-off-by: Wenchen Fan <wenchen@databricks.com>
## What changes were proposed in this pull request?
This adds a new logical plan, AppendData, that was proposed in SPARK-23521: Standardize SQL logical plans.
* DataFrameWriter uses the new AppendData plan for DataSourceV2 appends
* AppendData is resolved if its output columns match the incoming data frame
* A new analyzer rule, ResolveOutputColumns, validates data before it is appended. This rule will add safe casts, rename columns, and checks nullability
## How was this patch tested?
Existing tests for v2 appends. Will add AppendData tests to validate logical plan analysis.
Closes#21305 from rdblue/SPARK-24251-add-append-data.
Lead-authored-by: Ryan Blue <blue@apache.org>
Co-authored-by: Ryan Blue <rdblue@users.noreply.github.com>
Signed-off-by: Wenchen Fan <wenchen@databricks.com>
## What changes were proposed in this pull request?
Alter View can excute sql like "ALTER VIEW ... AS INSERT INTO" . We should throw ParseException(s"Operation not allowed: $message", ctx) as Create View does.
```
override def visitCreateView(ctx: CreateViewContext): LogicalPlan = withOrigin(ctx) {
if (ctx.identifierList != null) {
operationNotAllowed("CREATE VIEW ... PARTITIONED ON", ctx)
} else {
// CREATE VIEW ... AS INSERT INTO is not allowed.
ctx.query.queryNoWith match {
case s: SingleInsertQueryContext if s.insertInto != null =>
operationNotAllowed("CREATE VIEW ... AS INSERT INTO", ctx)
case _: MultiInsertQueryContext =>
operationNotAllowed("CREATE VIEW ... AS FROM ... [INSERT INTO ...]+", ctx)
case _ => // OK
}
```
```
override def visitAlterViewQuery(ctx: AlterViewQueryContext): LogicalPlan = withOrigin(ctx) {
// ALTER VIEW ... AS INSERT INTO is not allowed.
ctx.query.queryNoWith match {
case s: SingleInsertQueryContext if s.insertInto != null =>
operationNotAllowed("ALTER VIEW ... AS INSERT INTO", ctx)
case _: MultiInsertQueryContext =>
operationNotAllowed("ALTER VIEW ... AS FROM ... [INSERT INTO ...]+", ctx)
case _ => // OK
}
AlterViewAsCommand(
name = visitTableIdentifier(ctx.tableIdentifier),
originalText = source(ctx.query),
query = plan(ctx.query))
}
```
## How was this patch tested?
UT has been added in SparkSqlParserSuite
Closes#22028 from sddyljsx/SPARK-25046.
Lead-authored-by: Neal Song <neal_song@126.com>
Co-authored-by: neal <neal_song@126.com>
Signed-off-by: Xiao Li <gatorsmile@gmail.com>
## What changes were proposed in this pull request?
The PR fix the bug in `buildFormattedString` function in `MapType`, which makes the printed schema misleading.
## How was this patch tested?
Added UT
Closes#22006 from invkrh/fix-map-schema-print.
Authored-by: invkrh <invkrh@gmail.com>
Signed-off-by: Xiao Li <gatorsmile@gmail.com>
## What changes were proposed in this pull request?
The PR adds the high order function `map_filter`, which filters the entries of a map and returns a new map which contains only the entries which satisfied the filter function.
## How was this patch tested?
added UTs
Closes#21986 from mgaido91/SPARK-23937.
Authored-by: Marco Gaido <marcogaido91@gmail.com>
Signed-off-by: Takuya UESHIN <ueshin@databricks.com>
## What changes were proposed in this pull request?
This is a followup of https://github.com/apache/spark/pull/21822
Similar to `TreeNode`, `AnalysisHelper` should also provide 3 versions of transformations: `resolveOperatorsUp`, `resolveOperatorsDown` and `resolveOperators`.
This PR adds the missing `resolveOperatorsUp`, and also fixes some code style which is missed in #21822
## How was this patch tested?
existing tests.
Author: Wenchen Fan <wenchen@databricks.com>
Closes#21932 from cloud-fan/follow.
## What changes were proposed in this pull request?
The tests for shuffle can be flaky (eg. https://amplab.cs.berkeley.edu/jenkins/job/SparkPullRequestBuilder/94355/testReport/). This happens because we have not set the seed for `Random`.
## How was this patch tested?
running 10000 times the UT (validated that with a different seed eg. 12345 the test fails).
Closes#22023 from mgaido91/SPARK-23928_followup.
Authored-by: Marco Gaido <marcogaido91@gmail.com>
Signed-off-by: hyukjinkwon <gurwls223@apache.org>
## What changes were proposed in this pull request?
The design details is attached to the JIRA issue [here](https://drive.google.com/file/d/1zKm3aNZ3DpsqIuoMvRsf0kkDkXsAasxH/view)
High level overview of the changes are:
- Enhance the qualifier to be more than one string
- Add support to store the qualifier. Enhance the lookupRelation to keep the qualifier appropriately.
- Enhance the table matching column resolution algorithm to account for qualifier being more than a string.
- Enhance the table matching algorithm in UnresolvedStar.expand
- Ensure that we continue to support select t1.i1 from db1.t1
## How was this patch tested?
- New tests are added.
- Several test scenarios were added in a separate [test pr 17067](https://github.com/apache/spark/pull/17067). The tests that were not supported earlier are marked with TODO markers and those are now supported with the code changes here.
- Existing unit tests ( hive, catalyst and sql) were run successfully.
Closes#17185 from skambha/colResolution.
Authored-by: Sunitha Kambhampati <skambha@us.ibm.com>
Signed-off-by: Wenchen Fan <wenchen@databricks.com>
## What changes were proposed in this pull request?
In the PR, I propose to replace Scala parallel collections by new methods `parmap()`. The methods use futures to transform a sequential collection by applying a lambda function to each element in parallel. The result of `parmap` is another regular (sequential) collection.
The proposed `parmap` method aims to solve the problem of impossibility to interrupt parallel Scala collection. This possibility is needed for reliable task preemption.
## How was this patch tested?
A test was added to `ThreadUtilsSuite`
Closes#21913 from MaxGekk/par-map.
Authored-by: Maxim Gekk <maxim.gekk@databricks.com>
Signed-off-by: Wenchen Fan <wenchen@databricks.com>
## What changes were proposed in this pull request?
Using struct types in subqueries with the `IN` clause can generate invalid plans in `RewritePredicateSubquery`. Indeed, we are not handling clearly the cases when the outer value is a struct or the output of the inner subquery is a struct.
The PR aims to make Spark's behavior the same as the one of the other RDBMS - namely Oracle and Postgres behavior were checked. So we consider valid only queries having the same number of fields in the outer value and in the subquery. This means that:
- `(a, b) IN (select c, d from ...)` is a valid query;
- `(a, b) IN (select (c, d) from ...)` throws an AnalysisException, as in the subquery we have only one field of type struct while in the outer value we have 2 fields;
- `a IN (select (c, d) from ...)` - where `a` is a struct - is a valid query.
## How was this patch tested?
Added UT
Closes#21403 from mgaido91/SPARK-24313.
Authored-by: Marco Gaido <marcogaido91@gmail.com>
Signed-off-by: Wenchen Fan <wenchen@databricks.com>
## What changes were proposed in this pull request?
Like Uuid in SPARK-24896, Rand and Randn expressions now produce the same results for each execution in streaming query. It doesn't make too much sense for streaming queries. We should make them produce different results as Uuid.
In this change, similar to Uuid, we assign new random seeds to Rand/Randn when returning optimized plan from `IncrementalExecution`.
Note: Different to Uuid, Rand/Randn can be created with initial seed. Because we replace this initial seed at `IncrementalExecution`, it doesn't use the initial seed anymore. For now it seems to me not a big issue for streaming query. But need to confirm with others. cc zsxwing cloud-fan
## How was this patch tested?
Added test.
Closes#21980 from viirya/SPARK-25010.
Authored-by: Liang-Chi Hsieh <viirya@gmail.com>
Signed-off-by: Wenchen Fan <wenchen@databricks.com>
## What changes were proposed in this pull request?
This PR refactors `ArrayUnion` based on [this suggestion](https://github.com/apache/spark/pull/21103#discussion_r205668821).
1. Generate optimized code for all of the primitive types except `boolean`
1. Generate code using `ArrayBuilder` or `ArrayBuffer`
1. Leave only a generic path in the interpreted path
## How was this patch tested?
Existing tests
Author: Kazuaki Ishizaki <ishizaki@jp.ibm.com>
Closes#21937 from kiszk/SPARK-23914-follow.
## What changes were proposed in this pull request?
Currently the Structured Streaming sources and sinks does not have a way to report custom metrics. Providing an option to report custom metrics and making it available via Streaming Query progress can enable sources and sinks to report custom progress information (E.g. the lag metrics for Kafka source).
Similar metrics can be reported for Sinks as well, but would like to get initial feedback before proceeding further.
## How was this patch tested?
New and existing unit tests.
Please review http://spark.apache.org/contributing.html before opening a pull request.
Closes#21721 from arunmahadevan/SPARK-24748.
Authored-by: Arun Mahadevan <arunm@apache.org>
Signed-off-by: hyukjinkwon <gurwls223@apache.org>
## What changes were proposed in this pull request?
The patch adds metrics regarding state and watermark to dropwizard metrics, so that watermark and state rows/size can be tracked via time-series manner.
## How was this patch tested?
Manually tested with CSV metric sink.
Closes#21622 from HeartSaVioR/SPARK-24637.
Authored-by: Jungtaek Lim <kabhwan@gmail.com>
Signed-off-by: hyukjinkwon <gurwls223@apache.org>
## What changes were proposed in this pull request?
The PR refactors the aggregate expressions which were not using DSL in order to simplify them.
## How was this patch tested?
NA
Author: Marco Gaido <marcogaido91@gmail.com>
Closes#21970 from mgaido91/SPARK-24996.
## What changes were proposed in this pull request?
This PR fixes a comparison of `ExprValue.isNull` with `String`. `ExprValue.isNull` should be compared with `LiteralTrue` or `LiteralFalse`.
This causes the following compilation error using scala-2.12 with sbt. In addition, this code may also generate incorrect code in Spark 2.3.
```
/home/ishizaki/Spark/PR/scala212/spark/sql/catalyst/src/main/scala/org/apache/spark/sql/catalyst/expressions/stringExpressions.scala:94: org.apache.spark.sql.catalyst.expressions.codegen.ExprValue and String are unrelated: they will most likely always compare unequal
[error] [warn] if (eval.isNull != "true") {
[error] [warn]
[error] [warn] /home/ishizaki/Spark/PR/scala212/spark/sql/catalyst/src/main/scala/org/apache/spark/sql/catalyst/expressions/stringExpressions.scala:126: org.apache.spark.sql.catalyst.expressions.codegen.ExprValue and String are unrelated: they will most likely never compare equal
[error] [warn] if (eval.isNull == "true") {
[error] [warn]
[error] [warn] /home/ishizaki/Spark/PR/scala212/spark/sql/catalyst/src/main/scala/org/apache/spark/sql/catalyst/expressions/stringExpressions.scala:133: org.apache.spark.sql.catalyst.expressions.codegen.ExprValue and String are unrelated: they will most likely never compare equal
[error] [warn] if (eval.isNull == "true") {
[error] [warn]
[error] [warn] /home/ishizaki/Spark/PR/scala212/spark/sql/catalyst/src/main/scala/org/apache/spark/sql/catalyst/expressions/codegen/GenerateUnsafeProjection.scala:90: org.apache.spark.sql.catalyst.expressions.codegen.ExprValue and String are unrelated: they will most likely never compare equal
[error] [warn] if (inputs.map(_.isNull).forall(_ == "false")) {
[error] [warn]
```
## How was this patch tested?
Existing UTs
Author: Kazuaki Ishizaki <ishizaki@jp.ibm.com>
Closes#22012 from kiszk/SPARK-25036a.
## What changes were proposed in this pull request?
Currently, debug package has a implicit class "DebugQuery" which matches Dataset to provide debug features on Dataset class. It doesn't work with structured streaming: it requires query is already started, and the information can be retrieved from StreamingQuery, not Dataset. I guess that's why "explain" had to be placed to StreamingQuery whereas it already exists on Dataset.
This patch adds a new implicit class "DebugStreamQuery" which matches StreamingQuery to provide similar debug features on StreamingQuery class.
## How was this patch tested?
Added relevant unit tests.
Author: Jungtaek Lim <kabhwan@gmail.com>
Closes#21222 from HeartSaVioR/SPARK-24161.
## What changes were proposed in this pull request?
During upgrading Apache ORC to 1.5.2 ([SPARK-24576](https://issues.apache.org/jira/browse/SPARK-24576)), `sql/core` module overrides the exclusion rules of parent pom file and it causes published `spark-sql_2.1X` artifacts have incomplete exclusion rules ([SPARK-25019](https://issues.apache.org/jira/browse/SPARK-25019)). This PR fixes it by moving the newly added exclusion rule to the parent pom. This also fixes the sbt build hack introduced at that time.
## How was this patch tested?
Pass the existing dependency check and the tests.
Author: Dongjoon Hyun <dongjoon@apache.org>
Closes#22003 from dongjoon-hyun/SPARK-25019.
## What changes were proposed in this pull request?
The PR adds the SQL function `array_intersect`. The behavior of the function is based on Presto's one.
This function returns returns an array of the elements in the intersection of array1 and array2.
Note: The order of elements in the result is not defined.
## How was this patch tested?
Added UTs
Author: Kazuaki Ishizaki <ishizaki@jp.ibm.com>
Closes#21102 from kiszk/SPARK-23913.
## What changes were proposed in this pull request?
Having the default value of isAll in the logical plan nodes INTERSECT/EXCEPT could introduce bugs when the callers are not aware of it. This PR removes the default value and makes caller explicitly specify them.
## How was this patch tested?
This is a refactoring change. Existing tests test the functionality already.
Author: Dilip Biswal <dbiswal@us.ibm.com>
Closes#22000 from dilipbiswal/SPARK-25025.
## What changes were proposed in this pull request?
Follow up to fix an unmerged review comment.
## How was this patch tested?
Unit test ResolveHintsSuite.
Author: John Zhuge <jzhuge@apache.org>
Closes#21998 from jzhuge/SPARK-24940.
## What changes were proposed in this pull request?
A follow up of #21118
Since we use `InternalRow` in the read API of data source v2, we should do the same thing for the write API.
## How was this patch tested?
existing tests.
Author: Wenchen Fan <wenchen@databricks.com>
Closes#21948 from cloud-fan/row-write.
## What changes were proposed in this pull request?
This pr adds `aggregate` function which applies a binary operator to an initial state and all elements in the array, and reduces this to a single state. The final state is converted into the final result by applying a finish function.
```sql
> SELECT aggregate(array(1, 2, 3), (acc, x) -> acc + x);
6
> SELECT aggregate(array(1, 2, 3), (acc, x) -> acc + x, acc -> acc * 10);
60
```
## How was this patch tested?
Added tests.
Author: Takuya UESHIN <ueshin@databricks.com>
Closes#21982 from ueshin/issues/SPARK-23911/aggregate.
## What changes were proposed in this pull request?
There are many warnings in the current build (for instance see https://amplab.cs.berkeley.edu/jenkins/job/spark-master-test-sbt-hadoop-2.7/4734/console).
**common**:
```
[warn] /home/jenkins/workspace/spark-master-test-maven-hadoop-2.7/common/kvstore/src/main/java/org/apache/spark/util/kvstore/LevelDB.java:237: warning: [rawtypes] found raw type: LevelDBIterator
[warn] void closeIterator(LevelDBIterator it) throws IOException {
[warn] ^
[warn] missing type arguments for generic class LevelDBIterator<T>
[warn] where T is a type-variable:
[warn] T extends Object declared in class LevelDBIterator
[warn] /home/jenkins/workspace/spark-master-test-maven-hadoop-2.7/common/network-common/src/main/java/org/apache/spark/network/server/TransportServer.java:151: warning: [deprecation] group() in AbstractBootstrap has been deprecated
[warn] if (bootstrap != null && bootstrap.group() != null) {
[warn] ^
[warn] /home/jenkins/workspace/spark-master-test-maven-hadoop-2.7/common/network-common/src/main/java/org/apache/spark/network/server/TransportServer.java:152: warning: [deprecation] group() in AbstractBootstrap has been deprecated
[warn] bootstrap.group().shutdownGracefully();
[warn] ^
[warn] /home/jenkins/workspace/spark-master-test-maven-hadoop-2.7/common/network-common/src/main/java/org/apache/spark/network/server/TransportServer.java:154: warning: [deprecation] childGroup() in ServerBootstrap has been deprecated
[warn] if (bootstrap != null && bootstrap.childGroup() != null) {
[warn] ^
[warn] /home/jenkins/workspace/spark-master-test-maven-hadoop-2.7/common/network-common/src/main/java/org/apache/spark/network/server/TransportServer.java:155: warning: [deprecation] childGroup() in ServerBootstrap has been deprecated
[warn] bootstrap.childGroup().shutdownGracefully();
[warn] ^
[warn] /home/jenkins/workspace/spark-master-test-maven-hadoop-2.7/common/network-common/src/main/java/org/apache/spark/network/util/NettyUtils.java:112: warning: [deprecation] PooledByteBufAllocator(boolean,int,int,int,int,int,int,int) in PooledByteBufAllocator has been deprecated
[warn] return new PooledByteBufAllocator(
[warn] ^
[warn] /home/jenkins/workspace/spark-master-test-maven-hadoop-2.7/common/network-common/src/main/java/org/apache/spark/network/client/TransportClient.java:321: warning: [rawtypes] found raw type: Future
[warn] public void operationComplete(Future future) throws Exception {
[warn] ^
[warn] missing type arguments for generic class Future<V>
[warn] where V is a type-variable:
[warn] V extends Object declared in interface Future
[warn] /home/jenkins/workspace/spark-master-test-maven-hadoop-2.7/common/network-common/src/main/java/org/apache/spark/network/client/TransportResponseHandler.java:215: warning: [rawtypes] found raw type: StreamInterceptor
[warn] StreamInterceptor interceptor = new StreamInterceptor(this, resp.streamId, resp.byteCount,
[warn] ^
[warn] missing type arguments for generic class StreamInterceptor<T>
[warn] where T is a type-variable:
[warn] T extends Message declared in class StreamInterceptor
[warn] /home/jenkins/workspace/spark-master-test-maven-hadoop-2.7/common/network-common/src/main/java/org/apache/spark/network/client/TransportResponseHandler.java:215: warning: [rawtypes] found raw type: StreamInterceptor
[warn] StreamInterceptor interceptor = new StreamInterceptor(this, resp.streamId, resp.byteCount,
[warn] ^
[warn] missing type arguments for generic class StreamInterceptor<T>
[warn] where T is a type-variable:
[warn] T extends Message declared in class StreamInterceptor
[warn] /home/jenkins/workspace/spark-master-test-maven-hadoop-2.7/common/network-common/src/main/java/org/apache/spark/network/client/TransportResponseHandler.java:215: warning: [unchecked] unchecked call to StreamInterceptor(MessageHandler<T>,String,long,StreamCallback) as a member of the raw type StreamInterceptor
[warn] StreamInterceptor interceptor = new StreamInterceptor(this, resp.streamId, resp.byteCount,
[warn] ^
[warn] where T is a type-variable:
[warn] T extends Message declared in class StreamInterceptor
[warn] /home/jenkins/workspace/spark-master-test-maven-hadoop-2.7/common/network-common/src/main/java/org/apache/spark/network/server/TransportRequestHandler.java:255: warning: [rawtypes] found raw type: StreamInterceptor
[warn] StreamInterceptor interceptor = new StreamInterceptor(this, wrappedCallback.getID(),
[warn] ^
[warn] missing type arguments for generic class StreamInterceptor<T>
[warn] where T is a type-variable:
[warn] T extends Message declared in class StreamInterceptor
[warn] /home/jenkins/workspace/spark-master-test-maven-hadoop-2.7/common/network-common/src/main/java/org/apache/spark/network/server/TransportRequestHandler.java:255: warning: [rawtypes] found raw type: StreamInterceptor
[warn] StreamInterceptor interceptor = new StreamInterceptor(this, wrappedCallback.getID(),
[warn] ^
[warn] missing type arguments for generic class StreamInterceptor<T>
[warn] where T is a type-variable:
[warn] T extends Message declared in class StreamInterceptor
[warn] /home/jenkins/workspace/spark-master-test-maven-hadoop-2.7/common/network-common/src/main/java/org/apache/spark/network/server/TransportRequestHandler.java:255: warning: [unchecked] unchecked call to StreamInterceptor(MessageHandler<T>,String,long,StreamCallback) as a member of the raw type StreamInterceptor
[warn] StreamInterceptor interceptor = new StreamInterceptor(this, wrappedCallback.getID(),
[warn] ^
[warn] where T is a type-variable:
[warn] T extends Message declared in class StreamInterceptor
[warn] /home/jenkins/workspace/spark-master-test-maven-hadoop-2.7/common/network-common/src/main/java/org/apache/spark/network/crypto/TransportCipher.java:270: warning: [deprecation] transfered() in FileRegion has been deprecated
[warn] region.transferTo(byteRawChannel, region.transfered());
[warn] ^
[warn] /home/jenkins/workspace/spark-master-test-maven-hadoop-2.7/common/network-common/src/main/java/org/apache/spark/network/sasl/SaslEncryption.java:304: warning: [deprecation] transfered() in FileRegion has been deprecated
[warn] region.transferTo(byteChannel, region.transfered());
[warn] ^
[warn] /home/jenkins/workspace/spark-master-test-maven-hadoop-2.7/common/network-common/src/test/java/org/apache/spark/network/ProtocolSuite.java:119: warning: [deprecation] transfered() in FileRegion has been deprecated
[warn] while (in.transfered() < in.count()) {
[warn] ^
[warn] /home/jenkins/workspace/spark-master-test-maven-hadoop-2.7/common/network-common/src/test/java/org/apache/spark/network/ProtocolSuite.java:120: warning: [deprecation] transfered() in FileRegion has been deprecated
[warn] in.transferTo(channel, in.transfered());
[warn] ^
[warn] /home/jenkins/workspace/spark-master-test-maven-hadoop-2.7/common/unsafe/src/test/java/org/apache/spark/unsafe/hash/Murmur3_x86_32Suite.java:80: warning: [static] static method should be qualified by type name, Murmur3_x86_32, instead of by an expression
[warn] Assert.assertEquals(-300363099, hasher.hashUnsafeWords(bytes, offset, 16, 42));
[warn] ^
[warn] /home/jenkins/workspace/spark-master-test-maven-hadoop-2.7/common/unsafe/src/test/java/org/apache/spark/unsafe/hash/Murmur3_x86_32Suite.java:84: warning: [static] static method should be qualified by type name, Murmur3_x86_32, instead of by an expression
[warn] Assert.assertEquals(-1210324667, hasher.hashUnsafeWords(bytes, offset, 16, 42));
[warn] ^
[warn] /home/jenkins/workspace/spark-master-test-maven-hadoop-2.7/common/unsafe/src/test/java/org/apache/spark/unsafe/hash/Murmur3_x86_32Suite.java:88: warning: [static] static method should be qualified by type name, Murmur3_x86_32, instead of by an expression
[warn] Assert.assertEquals(-634919701, hasher.hashUnsafeWords(bytes, offset, 16, 42));
[warn] ^
```
**launcher**:
```
[warn] Pruning sources from previous analysis, due to incompatible CompileSetup.
[warn] /home/jenkins/workspace/spark-master-test-maven-hadoop-2.7/launcher/src/main/java/org/apache/spark/launcher/AbstractLauncher.java:31: warning: [rawtypes] found raw type: AbstractLauncher
[warn] public abstract class AbstractLauncher<T extends AbstractLauncher> {
[warn] ^
[warn] missing type arguments for generic class AbstractLauncher<T>
[warn] where T is a type-variable:
[warn] T extends AbstractLauncher declared in class AbstractLauncher
```
**core**:
```
[warn] /home/jenkins/workspace/spark-master-test-maven-hadoop-2.7/core/src/main/scala/org/apache/spark/api/r/RBackend.scala:99: method group in class AbstractBootstrap is deprecated: see corresponding Javadoc for more information.
[warn] if (bootstrap != null && bootstrap.group() != null) {
[warn] ^
[warn] /home/jenkins/workspace/spark-master-test-maven-hadoop-2.7/core/src/main/scala/org/apache/spark/api/r/RBackend.scala💯 method group in class AbstractBootstrap is deprecated: see corresponding Javadoc for more information.
[warn] bootstrap.group().shutdownGracefully()
[warn] ^
[warn] /home/jenkins/workspace/spark-master-test-maven-hadoop-2.7/core/src/main/scala/org/apache/spark/api/r/RBackend.scala:102: method childGroup in class ServerBootstrap is deprecated: see corresponding Javadoc for more information.
[warn] if (bootstrap != null && bootstrap.childGroup() != null) {
[warn] ^
[warn] /home/jenkins/workspace/spark-master-test-maven-hadoop-2.7/core/src/main/scala/org/apache/spark/api/r/RBackend.scala:103: method childGroup in class ServerBootstrap is deprecated: see corresponding Javadoc for more information.
[warn] bootstrap.childGroup().shutdownGracefully()
[warn] ^
[warn] /home/jenkins/workspace/spark-master-test-maven-hadoop-2.7/core/src/test/scala/org/apache/spark/util/ClosureCleanerSuite.scala:151: reflective access of structural type member method getData should be enabled
[warn] by making the implicit value scala.language.reflectiveCalls visible.
[warn] This can be achieved by adding the import clause 'import scala.language.reflectiveCalls'
[warn] or by setting the compiler option -language:reflectiveCalls.
[warn] See the Scaladoc for value scala.language.reflectiveCalls for a discussion
[warn] why the feature should be explicitly enabled.
[warn] val rdd = sc.parallelize(1 to 1).map(concreteObject.getData)
[warn] ^
[warn] /home/jenkins/workspace/spark-master-test-maven-hadoop-2.7/core/src/test/scala/org/apache/spark/util/ClosureCleanerSuite.scala:175: reflective access of structural type member value innerObject2 should be enabled
[warn] by making the implicit value scala.language.reflectiveCalls visible.
[warn] val rdd = sc.parallelize(1 to 1).map(concreteObject.innerObject2.getData)
[warn] ^
[warn] /home/jenkins/workspace/spark-master-test-maven-hadoop-2.7/core/src/test/scala/org/apache/spark/util/ClosureCleanerSuite.scala:175: reflective access of structural type member method getData should be enabled
[warn] by making the implicit value scala.language.reflectiveCalls visible.
[warn] val rdd = sc.parallelize(1 to 1).map(concreteObject.innerObject2.getData)
[warn] ^
[warn] /home/jenkins/workspace/spark-master-test-maven-hadoop-2.7/core/src/test/scala/org/apache/spark/LocalSparkContext.scala:32: constructor Slf4JLoggerFactory in class Slf4JLoggerFactory is deprecated: see corresponding Javadoc for more information.
[warn] InternalLoggerFactory.setDefaultFactory(new Slf4JLoggerFactory())
[warn] ^
[warn] /home/jenkins/workspace/spark-master-test-maven-hadoop-2.7/core/src/test/scala/org/apache/spark/status/AppStatusListenerSuite.scala:218: value attemptId in class StageInfo is deprecated: Use attemptNumber instead
[warn] assert(wrapper.stageAttemptId === stages.head.attemptId)
[warn] ^
[warn] /home/jenkins/workspace/spark-master-test-maven-hadoop-2.7/core/src/test/scala/org/apache/spark/status/AppStatusListenerSuite.scala:261: value attemptId in class StageInfo is deprecated: Use attemptNumber instead
[warn] stageAttemptId = stages.head.attemptId))
[warn] ^
[warn] /home/jenkins/workspace/spark-master-test-maven-hadoop-2.7/core/src/test/scala/org/apache/spark/status/AppStatusListenerSuite.scala:287: value attemptId in class StageInfo is deprecated: Use attemptNumber instead
[warn] stageAttemptId = stages.head.attemptId))
[warn] ^
[warn] /home/jenkins/workspace/spark-master-test-maven-hadoop-2.7/core/src/test/scala/org/apache/spark/status/AppStatusListenerSuite.scala:471: value attemptId in class StageInfo is deprecated: Use attemptNumber instead
[warn] stageAttemptId = stages.last.attemptId))
[warn] ^
[warn] /home/jenkins/workspace/spark-master-test-maven-hadoop-2.7/core/src/test/scala/org/apache/spark/status/AppStatusListenerSuite.scala:966: value attemptId in class StageInfo is deprecated: Use attemptNumber instead
[warn] listener.onTaskStart(SparkListenerTaskStart(dropped.stageId, dropped.attemptId, task))
[warn] ^
[warn] /home/jenkins/workspace/spark-master-test-maven-hadoop-2.7/core/src/test/scala/org/apache/spark/status/AppStatusListenerSuite.scala:972: value attemptId in class StageInfo is deprecated: Use attemptNumber instead
[warn] listener.onTaskEnd(SparkListenerTaskEnd(dropped.stageId, dropped.attemptId,
[warn] ^
[warn] /home/jenkins/workspace/spark-master-test-maven-hadoop-2.7/core/src/test/scala/org/apache/spark/status/AppStatusListenerSuite.scala:976: value attemptId in class StageInfo is deprecated: Use attemptNumber instead
[warn] .taskSummary(dropped.stageId, dropped.attemptId, Array(0.25d, 0.50d, 0.75d))
[warn] ^
[warn] /home/jenkins/workspace/spark-master-test-maven-hadoop-2.7/core/src/test/scala/org/apache/spark/status/AppStatusListenerSuite.scala:1146: value attemptId in class StageInfo is deprecated: Use attemptNumber instead
[warn] SparkListenerTaskEnd(stage1.stageId, stage1.attemptId, "taskType", Success, tasks(1), null))
[warn] ^
[warn] /home/jenkins/workspace/spark-master-test-maven-hadoop-2.7/core/src/test/scala/org/apache/spark/status/AppStatusListenerSuite.scala:1150: value attemptId in class StageInfo is deprecated: Use attemptNumber instead
[warn] SparkListenerTaskEnd(stage1.stageId, stage1.attemptId, "taskType", Success, tasks(0), null))
[warn] ^
[warn] /home/jenkins/workspace/spark-master-test-maven-hadoop-2.7/core/src/test/scala/org/apache/spark/storage/DiskStoreSuite.scala:197: method transfered in trait FileRegion is deprecated: see corresponding Javadoc for more information.
[warn] while (region.transfered() < region.count()) {
[warn] ^
[warn] /home/jenkins/workspace/spark-master-test-maven-hadoop-2.7/core/src/test/scala/org/apache/spark/storage/DiskStoreSuite.scala:198: method transfered in trait FileRegion is deprecated: see corresponding Javadoc for more information.
[warn] region.transferTo(byteChannel, region.transfered())
[warn] ^
```
**sql**:
```
[warn] /home/jenkins/workspace/spark-master-test-maven-hadoop-2.7/sql/catalyst/src/test/scala/org/apache/spark/sql/catalyst/analysis/AnalysisSuite.scala:534: abstract type T is unchecked since it is eliminated by erasure
[warn] assert(partitioning.isInstanceOf[T])
[warn] ^
[warn] /home/jenkins/workspace/spark-master-test-maven-hadoop-2.7/sql/catalyst/src/test/scala/org/apache/spark/sql/catalyst/analysis/AnalysisSuite.scala:534: abstract type T is unchecked since it is eliminated by erasure
[warn] assert(partitioning.isInstanceOf[T])
[warn] ^
[warn] /home/jenkins/workspace/spark-master-test-maven-hadoop-2.7/sql/catalyst/src/test/scala/org/apache/spark/sql/catalyst/expressions/ObjectExpressionsSuite.scala:323: inferred existential type Option[Class[_$1]]( forSome { type _$1 }), which cannot be expressed by wildcards, should be enabled
[warn] by making the implicit value scala.language.existentials visible.
[warn] This can be achieved by adding the import clause 'import scala.language.existentials'
[warn] or by setting the compiler option -language:existentials.
[warn] See the Scaladoc for value scala.language.existentials for a discussion
[warn] why the feature should be explicitly enabled.
[warn] val optClass = Option(collectionCls)
[warn] ^
[warn] /home/jenkins/workspace/spark-master-test-maven-hadoop-2.7/sql/core/src/main/java/org/apache/spark/sql/execution/datasources/parquet/SpecificParquetRecordReaderBase.java:226: warning: [deprecation] ParquetFileReader(Configuration,FileMetaData,Path,List<BlockMetaData>,List<ColumnDescriptor>) in ParquetFileReader has been deprecated
[warn] this.reader = new ParquetFileReader(
[warn] ^
[warn] /home/jenkins/workspace/spark-master-test-maven-hadoop-2.7/sql/core/src/main/java/org/apache/spark/sql/execution/datasources/parquet/VectorizedColumnReader.java:178: warning: [deprecation] getType() in ColumnDescriptor has been deprecated
[warn] (descriptor.getType() == PrimitiveType.PrimitiveTypeName.INT32 ||
[warn] ^
[warn] /home/jenkins/workspace/spark-master-test-maven-hadoop-2.7/sql/core/src/main/java/org/apache/spark/sql/execution/datasources/parquet/VectorizedColumnReader.java:179: warning: [deprecation] getType() in ColumnDescriptor has been deprecated
[warn] (descriptor.getType() == PrimitiveType.PrimitiveTypeName.INT64 &&
[warn] ^
[warn] /home/jenkins/workspace/spark-master-test-maven-hadoop-2.7/sql/core/src/main/java/org/apache/spark/sql/execution/datasources/parquet/VectorizedColumnReader.java:181: warning: [deprecation] getType() in ColumnDescriptor has been deprecated
[warn] descriptor.getType() == PrimitiveType.PrimitiveTypeName.FLOAT ||
[warn] ^
[warn] /home/jenkins/workspace/spark-master-test-maven-hadoop-2.7/sql/core/src/main/java/org/apache/spark/sql/execution/datasources/parquet/VectorizedColumnReader.java:182: warning: [deprecation] getType() in ColumnDescriptor has been deprecated
[warn] descriptor.getType() == PrimitiveType.PrimitiveTypeName.DOUBLE ||
[warn] ^
[warn] /home/jenkins/workspace/spark-master-test-maven-hadoop-2.7/sql/core/src/main/java/org/apache/spark/sql/execution/datasources/parquet/VectorizedColumnReader.java:183: warning: [deprecation] getType() in ColumnDescriptor has been deprecated
[warn] descriptor.getType() == PrimitiveType.PrimitiveTypeName.BINARY))) {
[warn] ^
[warn] /home/jenkins/workspace/spark-master-test-maven-hadoop-2.7/sql/core/src/main/java/org/apache/spark/sql/execution/datasources/parquet/VectorizedColumnReader.java:198: warning: [deprecation] getType() in ColumnDescriptor has been deprecated
[warn] switch (descriptor.getType()) {
[warn] ^
[warn] /home/jenkins/workspace/spark-master-test-maven-hadoop-2.7/sql/core/src/main/java/org/apache/spark/sql/execution/datasources/parquet/VectorizedColumnReader.java:221: warning: [deprecation] getTypeLength() in ColumnDescriptor has been deprecated
[warn] readFixedLenByteArrayBatch(rowId, num, column, descriptor.getTypeLength());
[warn] ^
[warn] /home/jenkins/workspace/spark-master-test-maven-hadoop-2.7/sql/core/src/main/java/org/apache/spark/sql/execution/datasources/parquet/VectorizedColumnReader.java:224: warning: [deprecation] getType() in ColumnDescriptor has been deprecated
[warn] throw new IOException("Unsupported type: " + descriptor.getType());
[warn] ^
[warn] /home/jenkins/workspace/spark-master-test-maven-hadoop-2.7/sql/core/src/main/java/org/apache/spark/sql/execution/datasources/parquet/VectorizedColumnReader.java:246: warning: [deprecation] getType() in ColumnDescriptor has been deprecated
[warn] descriptor.getType().toString(),
[warn] ^
[warn] /home/jenkins/workspace/spark-master-test-maven-hadoop-2.7/sql/core/src/main/java/org/apache/spark/sql/execution/datasources/parquet/VectorizedColumnReader.java:258: warning: [deprecation] getType() in ColumnDescriptor has been deprecated
[warn] switch (descriptor.getType()) {
[warn] ^
[warn] /home/jenkins/workspace/spark-master-test-maven-hadoop-2.7/sql/core/src/main/java/org/apache/spark/sql/execution/datasources/parquet/VectorizedColumnReader.java:384: warning: [deprecation] getType() in ColumnDescriptor has been deprecated
[warn] throw new UnsupportedOperationException("Unsupported type: " + descriptor.getType());
[warn] ^
[warn] /home/jenkins/workspace/spark-master-test-maven-hadoop-2.7/sql/core/src/main/java/org/apache/spark/sql/vectorized/ArrowColumnVector.java:458: warning: [static] static variable should be qualified by type name, BaseRepeatedValueVector, instead of by an expression
[warn] int index = rowId * accessor.OFFSET_WIDTH;
[warn] ^
[warn] /home/jenkins/workspace/spark-master-test-maven-hadoop-2.7/sql/core/src/main/java/org/apache/spark/sql/vectorized/ArrowColumnVector.java:460: warning: [static] static variable should be qualified by type name, BaseRepeatedValueVector, instead of by an expression
[warn] int end = offsets.getInt(index + accessor.OFFSET_WIDTH);
[warn] ^
[warn] /home/jenkins/workspace/spark-master-test-maven-hadoop-2.7/sql/core/src/test/scala/org/apache/spark/sql/BenchmarkQueryTest.scala:57: a pure expression does nothing in statement position; you may be omitting necessary parentheses
[warn] case s => s
[warn] ^
[warn] /home/jenkins/workspace/spark-master-test-maven-hadoop-2.7/sql/core/src/test/scala/org/apache/spark/sql/execution/datasources/parquet/ParquetInteroperabilitySuite.scala:182: inferred existential type org.apache.parquet.column.statistics.Statistics[?0]( forSome { type ?0 <: Comparable[?0] }), which cannot be expressed by wildcards, should be enabled
[warn] by making the implicit value scala.language.existentials visible.
[warn] This can be achieved by adding the import clause 'import scala.language.existentials'
[warn] or by setting the compiler option -language:existentials.
[warn] See the Scaladoc for value scala.language.existentials for a discussion
[warn] why the feature should be explicitly enabled.
[warn] val columnStats = oneBlockColumnMeta.getStatistics
[warn] ^
[warn] /home/jenkins/workspace/spark-master-test-maven-hadoop-2.7/sql/core/src/test/scala/org/apache/spark/sql/execution/streaming/sources/ForeachBatchSinkSuite.scala:146: implicit conversion method conv should be enabled
[warn] by making the implicit value scala.language.implicitConversions visible.
[warn] This can be achieved by adding the import clause 'import scala.language.implicitConversions'
[warn] or by setting the compiler option -language:implicitConversions.
[warn] See the Scaladoc for value scala.language.implicitConversions for a discussion
[warn] why the feature should be explicitly enabled.
[warn] implicit def conv(x: (Int, Long)): KV = KV(x._1, x._2)
[warn] ^
[warn] /home/jenkins/workspace/spark-master-test-maven-hadoop-2.7/sql/core/src/test/scala/org/apache/spark/sql/streaming/continuous/shuffle/ContinuousShuffleSuite.scala:48: implicit conversion method unsafeRow should be enabled
[warn] by making the implicit value scala.language.implicitConversions visible.
[warn] private implicit def unsafeRow(value: Int) = {
[warn] ^
[warn] /home/jenkins/workspace/spark-master-test-maven-hadoop-2.7/sql/core/src/test/scala/org/apache/spark/sql/execution/datasources/parquet/ParquetInteroperabilitySuite.scala:178: method getType in class ColumnDescriptor is deprecated: see corresponding Javadoc for more information.
[warn] assert(oneFooter.getFileMetaData.getSchema.getColumns.get(0).getType() ===
[warn] ^
[warn] /home/jenkins/workspace/spark-master-test-maven-hadoop-2.7/sql/core/src/test/scala/org/apache/spark/sql/execution/datasources/parquet/ParquetTest.scala:154: method readAllFootersInParallel in object ParquetFileReader is deprecated: see corresponding Javadoc for more information.
[warn] ParquetFileReader.readAllFootersInParallel(configuration, fs.getFileStatus(path)).asScala.toSeq
[warn] ^
[warn] /home/jenkins/workspace/spark-master-test-maven-hadoop-2.7/sql/hive/src/test/java/org/apache/spark/sql/hive/test/Complex.java:679: warning: [cast] redundant cast to Complex
[warn] Complex typedOther = (Complex)other;
[warn] ^
```
**mllib**:
```
[warn] Pruning sources from previous analysis, due to incompatible CompileSetup.
[warn] /home/jenkins/workspace/spark-master-test-maven-hadoop-2.7/mllib/src/test/scala/org/apache/spark/ml/recommendation/ALSSuite.scala:597: match may not be exhaustive.
[warn] It would fail on the following inputs: None, Some((x: Tuple2[?, ?] forSome x not in (?, ?)))
[warn] val df = dfs.find {
[warn] ^
```
This PR does not target fix all of them since some look pretty tricky to fix and there look too many warnings including false positive (like deprecated API but it's used in its test, etc.)
## How was this patch tested?
Existing tests should cover this.
Author: hyukjinkwon <gurwls223@apache.org>
Closes#21975 from HyukjinKwon/remove-build-warnings.
## What changes were proposed in this pull request?
simplify the codegen:
1. only do real codegen if the type can be specialized by the hash set
2. change the null handling. Before: track the nullElementIndex, and create a new ArrayData to insert the null in the middle. After: track the nullElementIndex, put a null placeholder in the ArrayBuilder, at the end create ArrayData from ArrayBuilder directly.
## How was this patch tested?
existing tests.
Author: Wenchen Fan <wenchen@databricks.com>
Closes#21966 from cloud-fan/minor2.
## What changes were proposed in this pull request?
This pr adds `filter` function which filters the input array using the given predicate.
```sql
> SELECT filter(array(1, 2, 3), x -> x % 2 == 1);
array(1, 3)
```
## How was this patch tested?
Added tests.
Author: Takuya UESHIN <ueshin@databricks.com>
Closes#21965 from ueshin/issues/SPARK-23909/filter.
## What changes were proposed in this pull request?
Many Spark SQL users in my company have asked for a way to control the number of output files in Spark SQL. The users prefer not to use function repartition(n) or coalesce(n, shuffle) that require them to write and deploy Scala/Java/Python code. We propose adding the following Hive-style Coalesce and Repartition Hint to Spark SQL:
```
... SELECT /*+ COALESCE(numPartitions) */ ...
... SELECT /*+ REPARTITION(numPartitions) */ ...
```
Multiple such hints are allowed. Multiple nodes are inserted into the logical plan, and the optimizer will pick the leftmost hint.
```
INSERT INTO s SELECT /*+ REPARTITION(100), COALESCE(500), COALESCE(10) */ * FROM t
== Logical Plan ==
'InsertIntoTable 'UnresolvedRelation `s`, false, false
+- 'UnresolvedHint REPARTITION, [100]
+- 'UnresolvedHint COALESCE, [500]
+- 'UnresolvedHint COALESCE, [10]
+- 'Project [*]
+- 'UnresolvedRelation `t`
== Optimized Logical Plan ==
InsertIntoHadoopFsRelationCommand ...
+- Repartition 100, true
+- HiveTableRelation ...
```
## How was this patch tested?
All unit tests. Manual tests using explain.
Author: John Zhuge <jzhuge@apache.org>
Closes#21911 from jzhuge/SPARK-24940.
## What changes were proposed in this pull request?
In the PR, I propose column-based API for the `pivot()` function. It allows using of any column expressions as the pivot column. Also this makes it consistent with how groupBy() works.
## How was this patch tested?
I added new tests to `DataFramePivotSuite` and updated PySpark examples for the `pivot()` function.
Author: Maxim Gekk <maxim.gekk@databricks.com>
Closes#21699 from MaxGekk/pivot-column.
## What changes were proposed in this pull request?
Enable support for MINUS ALL which was gated at AstBuilder.
## How was this patch tested?
Added tests in SQLQueryTestSuite and modify PlanParserSuite.
Please review http://spark.apache.org/contributing.html before opening a pull request.
Author: Dilip Biswal <dbiswal@us.ibm.com>
Closes#21963 from dilipbiswal/minus-all.
## What changes were proposed in this pull request?
In the current master, `toString` throws an exception when `RelationalGroupedDataset` has unresolved expressions;
```
scala> spark.range(0, 10).groupBy("id")
res4: org.apache.spark.sql.RelationalGroupedDataset = RelationalGroupedDataset: [grouping expressions: [id: bigint], value: [id: bigint], type: GroupBy]
scala> spark.range(0, 10).groupBy('id)
org.apache.spark.sql.catalyst.analysis.UnresolvedException: Invalid call to dataType on unresolved object, tree: 'id
at org.apache.spark.sql.catalyst.analysis.UnresolvedAttribute.dataType(unresolved.scala:105)
at org.apache.spark.sql.RelationalGroupedDataset$$anonfun$12.apply(RelationalGroupedDataset.scala:474)
at org.apache.spark.sql.RelationalGroupedDataset$$anonfun$12.apply(RelationalGroupedDataset.scala:473)
at scala.collection.TraversableLike$$anonfun$map$1.apply(TraversableLike.scala:234)
at scala.collection.TraversableLike$$anonfun$map$1.apply(TraversableLike.scala:234)
at scala.collection.mutable.ResizableArray$class.foreach(ResizableArray.scala:59)
at scala.collection.mutable.ArrayBuffer.foreach(ArrayBuffer.scala:48)
at scala.collection.TraversableLike$class.map(TraversableLike.scala:234)
at scala.collection.AbstractTraversable.map(Traversable.scala:104)
at org.apache.spark.sql.RelationalGroupedDataset.toString(RelationalGroupedDataset.scala:473)
at scala.runtime.ScalaRunTime$.scala$runtime$ScalaRunTime$$inner$1(ScalaRunTime.scala:332)
at scala.runtime.ScalaRunTime$.stringOf(ScalaRunTime.scala:337)
at scala.runtime.ScalaRunTime$.replStringOf(ScalaRunTime.scala:345)
```
This pr fixed code to handle the unresolved case in `RelationalGroupedDataset.toString`.
Closes#21752
## How was this patch tested?
Added tests in `DataFrameAggregateSuite`.
Author: Chris Horn <chorn4033@gmail.com>
Author: Takeshi Yamamuro <yamamuro@apache.org>
Closes#21964 from maropu/SPARK-24788.
## What changes were proposed in this pull request?
Currently the set operations INTERSECT, UNION and EXCEPT are assigned the same precedence. This PR fixes the problem by giving INTERSECT higher precedence than UNION and EXCEPT. UNION and EXCEPT operators are evaluated in the order in which they appear in the query from left to right.
This results in change in behavior because of the change in order of evaluations of set operators in a query. The old behavior is still preserved under a newly added config parameter.
Query `:`
```
SELECT * FROM t1
UNION
SELECT * FROM t2
EXCEPT
SELECT * FROM t3
INTERSECT
SELECT * FROM t4
```
Parsed plan before the change `:`
```
== Parsed Logical Plan ==
'Intersect false
:- 'Except false
: :- 'Distinct
: : +- 'Union
: : :- 'Project [*]
: : : +- 'UnresolvedRelation `t1`
: : +- 'Project [*]
: : +- 'UnresolvedRelation `t2`
: +- 'Project [*]
: +- 'UnresolvedRelation `t3`
+- 'Project [*]
+- 'UnresolvedRelation `t4`
```
Parsed plan after the change `:`
```
== Parsed Logical Plan ==
'Except false
:- 'Distinct
: +- 'Union
: :- 'Project [*]
: : +- 'UnresolvedRelation `t1`
: +- 'Project [*]
: +- 'UnresolvedRelation `t2`
+- 'Intersect false
:- 'Project [*]
: +- 'UnresolvedRelation `t3`
+- 'Project [*]
+- 'UnresolvedRelation `t4`
```
## How was this patch tested?
Added tests in PlanParserSuite, SQLQueryTestSuite.
Please review http://spark.apache.org/contributing.html before opening a pull request.
Author: Dilip Biswal <dbiswal@us.ibm.com>
Closes#21941 from dilipbiswal/SPARK-24966.
## What changes were proposed in this pull request?
Support reading/writing Avro logical timestamp type with different precisions
https://avro.apache.org/docs/1.8.2/spec.html#Timestamp+%28millisecond+precision%29
To specify the output timestamp type, use Dataframe option `outputTimestampType` or SQL config `spark.sql.avro.outputTimestampType`. The supported values are
* `TIMESTAMP_MICROS`
* `TIMESTAMP_MILLIS`
The default output type is `TIMESTAMP_MICROS`
## How was this patch tested?
Unit test
Author: Gengliang Wang <gengliang.wang@databricks.com>
Closes#21935 from gengliangwang/avro_timestamp.
## What changes were proposed in this pull request?
This PR refactors code to get a value for "spark.sql.codegen.comments" by avoiding `SparkEnv.get.conf`. This PR uses `SQLConf.get.codegenComments` since `SQLConf.get` always returns an instance of `SQLConf`.
## How was this patch tested?
Added test case to `DebuggingSuite`
Author: Kazuaki Ishizaki <ishizaki@jp.ibm.com>
Closes#19449 from kiszk/SPARK-22219.
## What changes were proposed in this pull request?
`Uuid`'s results depend on random seed given during analysis. Thus under streaming query, we will have the same uuids in each execution. This seems to be incorrect for streaming query execution.
## How was this patch tested?
Added test.
Author: Liang-Chi Hsieh <viirya@gmail.com>
Closes#21854 from viirya/uuid_in_streaming.
## What changes were proposed in this pull request?
In the current master, `EnsureRequirements` sets the number of exchanges in `ExchangeCoordinator` before `ReuseExchange`. Then, `ReuseExchange` removes some duplicate exchange and the actual number of registered exchanges changes. Finally, the assertion in `ExchangeCoordinator` fails because the logical number of exchanges and the actual number of registered exchanges become different;
https://github.com/apache/spark/blob/master/sql/core/src/main/scala/org/apache/spark/sql/execution/exchange/ExchangeCoordinator.scala#L201
This pr fixed the issue and the code to reproduce this is as follows;
```
scala> sql("SET spark.sql.adaptive.enabled=true")
scala> sql("SET spark.sql.autoBroadcastJoinThreshold=-1")
scala> val df = spark.range(1).selectExpr("id AS key", "id AS value")
scala> val resultDf = df.join(df, "key").join(df, "key")
scala> resultDf.show
...
at org.apache.spark.sql.execution.exchange.ShuffleExchangeExec$$anonfun$doExecute$1.apply(ShuffleExchangeExec.scala:119)
at org.apache.spark.sql.catalyst.errors.package$.attachTree(package.scala:52)
... 101 more
Caused by: java.lang.AssertionError: assertion failed
at scala.Predef$.assert(Predef.scala:156)
at org.apache.spark.sql.execution.exchange.ExchangeCoordinator.doEstimationIfNecessary(ExchangeCoordinator.scala:201)
at org.apache.spark.sql.execution.exchange.ExchangeCoordinator.postShuffleRDD(ExchangeCoordinator.scala:259)
at org.apache.spark.sql.execution.exchange.ShuffleExchangeExec$$anonfun$doExecute$1.apply(ShuffleExchangeExec.scala:124)
at org.apache.spark.sql.execution.exchange.ShuffleExchangeExec$$anonfun$doExecute$1.apply(ShuffleExchangeExec.scala:119)
at org.apache.spark.sql.catalyst.errors.package$.attachTree(package.scala:52)
...
```
## How was this patch tested?
Added tests in `ExchangeCoordinatorSuite`.
Author: Takeshi Yamamuro <yamamuro@apache.org>
Closes#21754 from maropu/SPARK-24705-2.
## What changes were proposed in this pull request?
This pr adds `transform` function which transforms elements in an array using the function.
Optionally we can take the index of each element as the second argument.
```sql
> SELECT transform(array(1, 2, 3), x -> x + 1);
array(2, 3, 4)
> SELECT transform(array(1, 2, 3), (x, i) -> x + i);
array(1, 3, 5)
```
## How was this patch tested?
Added tests.
Author: Takuya UESHIN <ueshin@databricks.com>
Closes#21954 from ueshin/issues/SPARK-23908/transform.
## What changes were proposed in this pull request?
This pull request provides a fix for SPARK-24742: SQL Field MetaData was throwing an Exception in the hashCode method when a "null" Metadata was added via "putNull"
## How was this patch tested?
A new unittest is provided in org/apache/spark/sql/types/MetadataSuite.scala
Author: Kaya Kupferschmidt <k.kupferschmidt@dimajix.de>
Closes#21722 from kupferk/SPARK-24742.
## What changes were proposed in this pull request?
Remove the AnalysisBarrier LogicalPlan node, which is useless now.
## How was this patch tested?
N/A
Author: Xiao Li <gatorsmile@gmail.com>
Closes#21962 from gatorsmile/refactor2.
## What changes were proposed in this pull request?
This PR addresses issues 2,3 in this [document](https://docs.google.com/document/d/1fbkjEL878witxVQpOCbjlvOvadHtVjYXeB-2mgzDTvk).
* We modified the closure cleaner to identify closures that are implemented via the LambdaMetaFactory mechanism (serializedLambdas) (issue2).
* We also fix the issue due to scala/bug#11016. There are two options for solving the Unit issue, either add () at the end of the closure or use the trick described in the doc. Otherwise overloading resolution does not work (we are not going to eliminate either of the methods) here. Compiler tries to adapt to Unit and makes these two methods candidates for overloading, when there is polymorphic overloading there is no ambiguity (that is the workaround implemented). This does not look that good but it serves its purpose as we need to support two different uses for method: `addTaskCompletionListener`. One that passes a TaskCompletionListener and one that passes a closure that is wrapped with a TaskCompletionListener later on (issue3).
Note: regarding issue 1 in the doc the plan is:
> Do Nothing. Don’t try to fix this as this is only a problem for Java users who would want to use 2.11 binaries. In that case they can cast to MapFunction to be able to utilize lambdas. In Spark 3.0.0 the API should be simplified so that this issue is removed.
## How was this patch tested?
This was manually tested:
```./dev/change-scala-version.sh 2.12
./build/mvn -DskipTests -Pscala-2.12 clean package
./build/mvn -Pscala-2.12 clean package -DwildcardSuites=org.apache.spark.serializer.ProactiveClosureSerializationSuite -Dtest=None
./build/mvn -Pscala-2.12 clean package -DwildcardSuites=org.apache.spark.util.ClosureCleanerSuite -Dtest=None
./build/mvn -Pscala-2.12 clean package -DwildcardSuites=org.apache.spark.streaming.DStreamClosureSuite -Dtest=None```
Author: Stavros Kontopoulos <stavros.kontopoulos@lightbend.com>
Closes#21930 from skonto/scala2.12-sup.
## What changes were proposed in this pull request?
This PR is to refactor the code in AVERAGE by dsl.
## How was this patch tested?
N/A
Author: Xiao Li <gatorsmile@gmail.com>
Closes#21951 from gatorsmile/refactor1.
## What changes were proposed in this pull request?
Regarding user-specified schema, data sources may have 3 different behaviors:
1. must have a user-specified schema
2. can't have a user-specified schema
3. can accept the user-specified if it's given, or infer the schema.
I added `ReadSupportWithSchema` to support these behaviors, following data source v1. But it turns out we don't need this extra interface. We can just add a `createReader(schema, options)` to `ReadSupport` and make it call `createReader(options)` by default.
TODO: also fix the streaming API in followup PRs.
## How was this patch tested?
existing tests.
Author: Wenchen Fan <wenchen@databricks.com>
Closes#21946 from cloud-fan/ds-schema.
## What changes were proposed in this pull request?
How to reproduce:
```sql
spark-sql> CREATE TABLE tbl AS SELECT 1;
spark-sql> CREATE TABLE tbl1 (c1 BIGINT, day STRING, hour STRING)
> USING parquet
> PARTITIONED BY (day, hour);
spark-sql> INSERT INTO TABLE tbl1 PARTITION (day = '2018-07-25', hour='01') SELECT * FROM tbl where 1=0;
spark-sql> SHOW PARTITIONS tbl1;
spark-sql> CREATE TABLE tbl2 (c1 BIGINT)
> PARTITIONED BY (day STRING, hour STRING);
spark-sql> INSERT INTO TABLE tbl2 PARTITION (day = '2018-07-25', hour='01') SELECT * FROM tbl where 1=0;
spark-sql> SHOW PARTITIONS tbl2;
day=2018-07-25/hour=01
spark-sql>
```
1. Users will be confused about whether the partition data of `tbl1` is generated.
2. Inconsistent with Hive table behavior.
This pr fix this issues.
## How was this patch tested?
unit tests
Author: Yuming Wang <yumwang@ebay.com>
Closes#21883 from wangyum/SPARK-24937.
## What changes were proposed in this pull request?
The PR adds the SQL function `array_except`. The behavior of the function is based on Presto's one.
This function returns returns an array of the elements in array1 but not in array2.
Note: The order of elements in the result is not defined.
## How was this patch tested?
Added UTs.
Author: Kazuaki Ishizaki <ishizaki@jp.ibm.com>
Closes#21103 from kiszk/SPARK-23915.
## What changes were proposed in this pull request?
This is a follow up of https://github.com/apache/spark/pull/21118 .
In https://github.com/apache/spark/pull/21118 we added `SupportsDeprecatedScanRow`. Ideally data source should produce `InternalRow` instead of `Row` for better performance. We should remove `SupportsDeprecatedScanRow` and encourage data sources to produce `InternalRow`, which is also very easy to build.
## How was this patch tested?
existing tests.
Author: Wenchen Fan <wenchen@databricks.com>
Closes#21921 from cloud-fan/row.
## What changes were proposed in this pull request?
When user calls anUDAF with the wrong number of arguments, Spark previously throws an AssertionError, which is not supposed to be a user-facing exception. This patch updates it to throw AnalysisException instead, so it is consistent with a regular UDF.
## How was this patch tested?
Updated test case udaf.sql.
Author: Reynold Xin <rxin@databricks.com>
Closes#21938 from rxin/SPARK-24982.
## What changes were proposed in this pull request?
Previously TVF resolution could throw IllegalArgumentException if the data type is null type. This patch replaces that exception with AnalysisException, enriched with positional information, to improve error message reporting and to be more consistent with rest of Spark SQL.
## How was this patch tested?
Updated the test case in table-valued-functions.sql.out, which is how I identified this problem in the first place.
Author: Reynold Xin <rxin@databricks.com>
Closes#21934 from rxin/SPARK-24951.
## What changes were proposed in this pull request?
Similar to SPARK-24890, if all the outputs of `CaseWhen` are semantic equivalence, `CaseWhen` can be removed.
## How was this patch tested?
Tests added.
Author: DB Tsai <d_tsai@apple.com>
Closes#21852 from dbtsai/short-circuit-when.
## What changes were proposed in this pull request?
It proposes a version in which nullable expressions are not valid in the limit clause
## How was this patch tested?
It was tested with unit and e2e tests.
Please review http://spark.apache.org/contributing.html before opening a pull request.
Author: Mauro Palsgraaf <mauropalsgraaf@hotmail.com>
Closes#21807 from mauropalsgraaf/SPARK-24536.
## What changes were proposed in this pull request?
When the pivot column is of a complex type, the eval() result will be an UnsafeRow, while the keys of the HashMap for column value matching is a GenericInternalRow. As a result, there will be no match and the result will always be empty.
So for a pivot column of complex-types, we should:
1) If the complex-type is not comparable (orderable), throw an Exception. It cannot be a pivot column.
2) Otherwise, if it goes through the `PivotFirst` code path, `PivotFirst` should use a TreeMap instead of HashMap for such columns.
This PR has also reverted the walk-around in Analyzer that had been introduced to avoid this `PivotFirst` issue.
## How was this patch tested?
Added UT.
Author: maryannxue <maryannxue@apache.org>
Closes#21926 from maryannxue/pivot_followup.
## What changes were proposed in this pull request?
In the PR, I propose to support `LZMA2` (`XZ`) and `BZIP2` compressions by `AVRO` datasource in write since the codecs may have better characteristics like compression ratio and speed comparing to already supported `snappy` and `deflate` codecs.
## How was this patch tested?
It was tested manually and by an existing test which was extended to check the `xz` and `bzip2` compressions.
Author: Maxim Gekk <maxim.gekk@databricks.com>
Closes#21902 from MaxGekk/avro-xz-bzip2.
## What changes were proposed in this pull request?
I didn't want to pollute the diff in the previous PR and left some TODOs. This is a follow-up to address those TODOs.
## How was this patch tested?
Should be covered by existing tests.
Author: Reynold Xin <rxin@databricks.com>
Closes#21896 from rxin/SPARK-24865-addendum.
## What changes were proposed in this pull request?
This pr supported Date/Timestamp in a JDBC partition column (a numeric column is only supported in the master). This pr also modified code to verify a partition column type;
```
val jdbcTable = spark.read
.option("partitionColumn", "text")
.option("lowerBound", "aaa")
.option("upperBound", "zzz")
.option("numPartitions", 2)
.jdbc("jdbc:postgresql:postgres", "t", options)
// with this pr
org.apache.spark.sql.AnalysisException: Partition column type should be numeric, date, or timestamp, but string found.;
at org.apache.spark.sql.execution.datasources.jdbc.JDBCRelation$.verifyAndGetNormalizedPartitionColumn(JDBCRelation.scala:165)
at org.apache.spark.sql.execution.datasources.jdbc.JDBCRelation$.columnPartition(JDBCRelation.scala:85)
at org.apache.spark.sql.execution.datasources.jdbc.JdbcRelationProvider.createRelation(JdbcRelationProvider.scala:36)
at org.apache.spark.sql.execution.datasources.DataSource.resolveRelation(DataSource.scala:317)
// without this pr
java.lang.NumberFormatException: For input string: "aaa"
at java.lang.NumberFormatException.forInputString(NumberFormatException.java:65)
at java.lang.Long.parseLong(Long.java:589)
at java.lang.Long.parseLong(Long.java:631)
at scala.collection.immutable.StringLike$class.toLong(StringLike.scala:277)
```
Closes#19999
## How was this patch tested?
Added tests in `JDBCSuite`.
Author: Takeshi Yamamuro <yamamuro@apache.org>
Closes#21834 from maropu/SPARK-22814.
## What changes were proposed in this pull request?
Upgrade Apache Avro from 1.7.7 to 1.8.2. The major new features:
1. More logical types. From the spec of 1.8.2 https://avro.apache.org/docs/1.8.2/spec.html#Logical+Types we can see comparing to [1.7.7](https://avro.apache.org/docs/1.7.7/spec.html#Logical+Types), the new version support:
- Date
- Time (millisecond precision)
- Time (microsecond precision)
- Timestamp (millisecond precision)
- Timestamp (microsecond precision)
- Duration
2. Single-object encoding: https://avro.apache.org/docs/1.8.2/spec.html#single_object_encoding
This PR aims to update Apache Spark to support these new features.
## How was this patch tested?
Unit test
Author: Gengliang Wang <gengliang.wang@databricks.com>
Closes#21761 from gengliangwang/upgrade_avro_1.8.
## What changes were proposed in this pull request?
When we do an average, the result is computed dividing the sum of the values by their count. In the case the result is a DecimalType, the way we are casting/managing the precision and scale is not really optimized and it is not coherent with what we do normally.
In particular, a problem can happen when the `Divide` operand returns a result which contains a precision and scale different by the ones which are expected as output of the `Divide` operand. In the case reported in the JIRA, for instance, the result of the `Divide` operand is a `Decimal(38, 36)`, while the output data type for `Divide` is 38, 22. This is not an issue when the `Divide` is followed by a `CheckOverflow` or a `Cast` to the right data type, as these operations return a decimal with the defined precision and scale. Despite in the `Average` operator we do have a `Cast`, this may be bypassed if the result of `Divide` is the same type which it is casted to, hence the issue reported in the JIRA may arise.
The PR proposes to use the normal rules/handling of the arithmetic operators with Decimal data type, so we both reuse the existing code (having a single logic for operations between decimals) and we fix this problem as the result is always guarded by `CheckOverflow`.
## How was this patch tested?
added UT
Author: Marco Gaido <marcogaido91@gmail.com>
Closes#21910 from mgaido91/SPARK-24957.
## What changes were proposed in this pull request?
Looks we intentionally set `null` for upper/lower bounds for complex types and don't use it. However, these look used in in-memory partition pruning, which ends up with incorrect results.
This PR proposes to explicitly whitelist the supported types.
```scala
val df = Seq(Array("a", "b"), Array("c", "d")).toDF("arrayCol")
df.cache().filter("arrayCol > array('a', 'b')").show()
```
```scala
val df = sql("select cast('a' as binary) as a")
df.cache().filter("a == cast('a' as binary)").show()
```
**Before:**
```
+--------+
|arrayCol|
+--------+
+--------+
```
```
+---+
| a|
+---+
+---+
```
**After:**
```
+--------+
|arrayCol|
+--------+
| [c, d]|
+--------+
```
```
+----+
| a|
+----+
|[61]|
+----+
```
## How was this patch tested?
Unit tests were added and manually tested.
Author: hyukjinkwon <gurwls223@apache.org>
Closes#21882 from HyukjinKwon/stats-filter.
## What changes were proposed in this pull request?
Implements INTERSECT ALL clause through query rewrites using existing operators in Spark. Please refer to [Link](https://drive.google.com/open?id=1nyW0T0b_ajUduQoPgZLAsyHK8s3_dko3ulQuxaLpUXE) for the design.
Input Query
``` SQL
SELECT c1 FROM ut1 INTERSECT ALL SELECT c1 FROM ut2
```
Rewritten Query
```SQL
SELECT c1
FROM (
SELECT replicate_row(min_count, c1)
FROM (
SELECT c1,
IF (vcol1_cnt > vcol2_cnt, vcol2_cnt, vcol1_cnt) AS min_count
FROM (
SELECT c1, count(vcol1) as vcol1_cnt, count(vcol2) as vcol2_cnt
FROM (
SELECT c1, true as vcol1, null as vcol2 FROM ut1
UNION ALL
SELECT c1, null as vcol1, true as vcol2 FROM ut2
) AS union_all
GROUP BY c1
HAVING vcol1_cnt >= 1 AND vcol2_cnt >= 1
)
)
)
```
## How was this patch tested?
Added test cases in SQLQueryTestSuite, DataFrameSuite, SetOperationSuite
Author: Dilip Biswal <dbiswal@us.ibm.com>
Closes#21886 from dilipbiswal/dkb_intersect_all_final.
## What changes were proposed in this pull request?
This PR propose to address https://github.com/apache/spark/pull/21318#discussion_r187843125 comment.
This is rather a nit but looks we better avoid to update the link for each release since it always points the latest (it doesn't look like worth enough updating release guide on the other hand as well).
## How was this patch tested?
N/A
Author: hyukjinkwon <gurwls223@apache.org>
Closes#21907 from HyukjinKwon/minor-fix.
When join key is long or int in broadcast join, Spark will use `LongToUnsafeRowMap` to store key-values of the table witch will be broadcasted. But, when `LongToUnsafeRowMap` is broadcasted to executors, and it is too big to hold in memory, it will be stored in disk. At that time, because `write` uses a variable `cursor` to determine how many bytes in `page` of `LongToUnsafeRowMap` will be write out and the `cursor` was not restore when deserializing, executor will write out nothing from page into disk.
## What changes were proposed in this pull request?
Restore cursor value when deserializing.
Author: liulijia <liutang123@yeah.net>
Closes#21772 from liutang123/SPARK-24809.
## What changes were proposed in this pull request?
- Update DateTimeUtilsSuite so that when testing roundtripping in daysToMillis and millisToDays multiple skipdates can be specified.
- Updated test so that both new years eve 2014 and new years day 2015 are skipped for kiribati time zones. This is necessary as java versions pre 181-b13 considered new years day 2015 to be skipped while susequent versions corrected this to new years eve.
## How was this patch tested?
Unit tests
Author: Chris Martin <chris@cmartinit.co.uk>
Closes#21901 from d80tb7/SPARK-24950_datetimeUtilsSuite_failures.
## What changes were proposed in this pull request?
Implements EXCEPT ALL clause through query rewrites using existing operators in Spark. In this PR, an internal UDTF (replicate_rows) is added to aid in preserving duplicate rows. Please refer to [Link](https://drive.google.com/open?id=1nyW0T0b_ajUduQoPgZLAsyHK8s3_dko3ulQuxaLpUXE) for the design.
**Note** This proposed UDTF is kept as a internal function that is purely used to aid with this particular rewrite to give us flexibility to change to a more generalized UDTF in future.
Input Query
``` SQL
SELECT c1 FROM ut1 EXCEPT ALL SELECT c1 FROM ut2
```
Rewritten Query
```SQL
SELECT c1
FROM (
SELECT replicate_rows(sum_val, c1)
FROM (
SELECT c1, sum_val
FROM (
SELECT c1, sum(vcol) AS sum_val
FROM (
SELECT 1L as vcol, c1 FROM ut1
UNION ALL
SELECT -1L as vcol, c1 FROM ut2
) AS union_all
GROUP BY union_all.c1
)
WHERE sum_val > 0
)
)
```
## How was this patch tested?
Added test cases in SQLQueryTestSuite, DataFrameSuite and SetOperationSuite
Author: Dilip Biswal <dbiswal@us.ibm.com>
Closes#21857 from dilipbiswal/dkb_except_all_final.
## What changes were proposed in this pull request?
In the PR, I added new option for Avro datasource - `compression`. The option allows to specify compression codec for saved Avro files. This option is similar to `compression` option in another datasources like `JSON` and `CSV`.
Also I added the SQL configs `spark.sql.avro.compression.codec` and `spark.sql.avro.deflate.level`. I put the configs into `SQLConf`. If the `compression` option is not specified by an user, the first SQL config is taken into account.
## How was this patch tested?
I added new test which read meta info from written avro files and checks `avro.codec` property.
Author: Maxim Gekk <maxim.gekk@databricks.com>
Closes#21837 from MaxGekk/avro-compression.
## What changes were proposed in this pull request?
This PR adds a new collection function: shuffle. It generates a random permutation of the given array. This implementation uses the "inside-out" version of Fisher-Yates algorithm.
## How was this patch tested?
New tests are added to CollectionExpressionsSuite.scala and DataFrameFunctionsSuite.scala.
Author: Takuya UESHIN <ueshin@databricks.com>
Author: pkuwm <ihuizhi.lu@gmail.com>
Closes#21802 from ueshin/issues/SPARK-23928/shuffle.
## What changes were proposed in this pull request?
Add a JDBC Option "pushDownPredicate" (default `true`) to allow/disallow predicate push-down in JDBC data source.
## How was this patch tested?
Add a test in `JDBCSuite`
Author: maryannxue <maryannxue@apache.org>
Closes#21875 from maryannxue/spark-24288.
## What changes were proposed in this pull request?
AnalysisBarrier was introduced in SPARK-20392 to improve analysis speed (don't re-analyze nodes that have already been analyzed).
Before AnalysisBarrier, we already had some infrastructure in place, with analysis specific functions (resolveOperators and resolveExpressions). These functions do not recursively traverse down subplans that are already analyzed (with a mutable boolean flag _analyzed). The issue with the old system was that developers started using transformDown, which does a top-down traversal of the plan tree, because there was not top-down resolution function, and as a result analyzer performance became pretty bad.
In order to fix the issue in SPARK-20392, AnalysisBarrier was introduced as a special node and for this special node, transform/transformUp/transformDown don't traverse down. However, the introduction of this special node caused a lot more troubles than it solves. This implicit node breaks assumptions and code in a few places, and it's hard to know when analysis barrier would exist, and when it wouldn't. Just a simple search of AnalysisBarrier in PR discussions demonstrates it is a source of bugs and additional complexity.
Instead, this pull request removes AnalysisBarrier and reverts back to the old approach. We added infrastructure in tests that fail explicitly if transform methods are used in the analyzer.
## How was this patch tested?
Added a test suite AnalysisHelperSuite for testing the resolve* methods and transform* methods.
Author: Reynold Xin <rxin@databricks.com>
Author: Xiao Li <gatorsmile@gmail.com>
Closes#21822 from rxin/SPARK-24865.
## What changes were proposed in this pull request?
In most cases, we should use `spark.sessionState.newHadoopConf()` instead of `sparkContext.hadoopConfiguration`, so that the hadoop configurations specified in Spark session
configuration will come into effect.
Add a rule matching `spark.sparkContext.hadoopConfiguration` or `spark.sqlContext.sparkContext.hadoopConfiguration` to prevent the usage.
## How was this patch tested?
Unit test
Author: Gengliang Wang <gengliang.wang@databricks.com>
Closes#21873 from gengliangwang/linterRule.
## What changes were proposed in this pull request?
This is an extension to the original PR, in which rule exclusion did not work for classes derived from Optimizer, e.g., SparkOptimizer.
To solve this issue, Optimizer and its derived classes will define/override `defaultBatches` and `nonExcludableRules` in order to define its default rule set as well as rules that cannot be excluded by the SQL config. In the meantime, Optimizer's `batches` method is dedicated to the rule exclusion logic and is defined "final".
## How was this patch tested?
Added UT.
Author: maryannxue <maryannxue@apache.org>
Closes#21876 from maryannxue/rule-exclusion.
## What changes were proposed in this pull request?
This PR aims to the followings.
1. Like `com.databricks.spark.csv` mapping, we had better map `com.databricks.spark.avro` to built-in Avro data source.
2. Remove incorrect error message, `Please find an Avro package at ...`.
## How was this patch tested?
Pass the newly added tests.
Author: Dongjoon Hyun <dongjoon@apache.org>
Closes#21878 from dongjoon-hyun/SPARK-24924.
## What changes were proposed in this pull request?
If we use `reverse` function for array type of primitive type containing `null` and the child array is `UnsafeArrayData`, the function returns a wrong result because `UnsafeArrayData` doesn't define the behavior of re-assignment, especially we can't set a valid value after we set `null`.
## How was this patch tested?
Added some tests.
Author: Takuya UESHIN <ueshin@databricks.com>
Closes#21830 from ueshin/issues/SPARK-24878/fix_reverse.
## What changes were proposed in this pull request?
```Scala
val udf1 = udf({(x: Int, y: Int) => x + y})
val df = spark.range(0, 3).toDF("a")
.withColumn("b", udf1($"a", udf1($"a", lit(10))))
df.cache()
df.write.saveAsTable("t")
```
Cache is not being used because the plans do not match with the cached plan. This is a regression caused by the changes we made in AnalysisBarrier, since not all the Analyzer rules are idempotent.
## How was this patch tested?
Added a test.
Also found a bug in the DSV1 write path. This is not a regression. Thus, opened a separate JIRA https://issues.apache.org/jira/browse/SPARK-24869
Author: Xiao Li <gatorsmile@gmail.com>
Closes#21821 from gatorsmile/testMaster22.
## What changes were proposed in this pull request?
Besides spark setting spark.sql.sources.partitionOverwriteMode also allow setting partitionOverWriteMode per write
## How was this patch tested?
Added unit test in InsertSuite
Please review http://spark.apache.org/contributing.html before opening a pull request.
Author: Koert Kuipers <koert@tresata.com>
Closes#21818 from koertkuipers/feat-partition-overwrite-mode-per-write.
## What changes were proposed in this pull request?
In the PR, I propose to extend the `StructType`/`StructField` classes by new method `toDDL` which converts a value of the `StructType`/`StructField` type to a string formatted in DDL style. The resulted string can be used in a table creation.
The `toDDL` method of `StructField` is reused in `SHOW CREATE TABLE`. In this way the PR fixes the bug of unquoted names of nested fields.
## How was this patch tested?
I add a test for checking the new method and 2 round trip tests: `fromDDL` -> `toDDL` and `toDDL` -> `fromDDL`
Author: Maxim Gekk <maxim.gekk@databricks.com>
Closes#21803 from MaxGekk/to-ddl.
## What changes were proposed in this pull request?
Improvement `IN` predicate type mismatched message:
```sql
Mismatched columns:
[(, t, 4, ., `, t, 4, a, `, :, d, o, u, b, l, e, ,, , t, 5, ., `, t, 5, a, `, :, d, e, c, i, m, a, l, (, 1, 8, ,, 0, ), ), (, t, 4, ., `, t, 4, c, `, :, s, t, r, i, n, g, ,, , t, 5, ., `, t, 5, c, `, :, b, i, g, i, n, t, )]
```
After this patch:
```sql
Mismatched columns:
[(t4.`t4a`:double, t5.`t5a`:decimal(18,0)), (t4.`t4c`:string, t5.`t5c`:bigint)]
```
## How was this patch tested?
unit tests
Author: Yuming Wang <yumwang@ebay.com>
Closes#21863 from wangyum/SPARK-18874.
## What changes were proposed in this pull request?
Add support for custom encoding on csv writer, see https://issues.apache.org/jira/browse/SPARK-19018
## How was this patch tested?
Added two unit tests in CSVSuite
Author: crafty-coder <carlospb86@gmail.com>
Author: Carlos <crafty-coder@users.noreply.github.com>
Closes#20949 from crafty-coder/master.
## What changes were proposed in this pull request?
Thanks to henryr for the original idea at https://github.com/apache/spark/pull/21049
Description from the original PR :
Subqueries (at least in SQL) have 'bag of tuples' semantics. Ordering
them is therefore redundant (unless combined with a limit).
This patch removes the top sort operators from the subquery plans.
This closes https://github.com/apache/spark/pull/21049.
## How was this patch tested?
Added test cases in SubquerySuite to cover in, exists and scalar subqueries.
Please review http://spark.apache.org/contributing.html before opening a pull request.
Author: Dilip Biswal <dbiswal@us.ibm.com>
Closes#21853 from dilipbiswal/SPARK-23957.
## What changes were proposed in this pull request?
When `trueValue` and `falseValue` are semantic equivalence, the condition expression in `if` can be removed to avoid extra computation in runtime.
## How was this patch tested?
Test added.
Author: DB Tsai <d_tsai@apple.com>
Closes#21848 from dbtsai/short-circuit-if.
## What changes were proposed in this pull request?
The HandleNullInputsForUDF would always add a new `If` node every time it is applied. That would cause a difference between the same plan being analyzed once and being analyzed twice (or more), thus raising issues like plan not matched in the cache manager. The solution is to mark the arguments as null-checked, which is to add a "KnownNotNull" node above those arguments, when adding the UDF under an `If` node, because clearly the UDF will not be called when any of those arguments is null.
## How was this patch tested?
Add new tests under sql/UDFSuite and AnalysisSuite.
Author: maryannxue <maryannxue@apache.org>
Closes#21851 from maryannxue/spark-24891.
## What changes were proposed in this pull request?
Last Access Time will always displayed wrong date Thu Jan 01 05:30:00 IST 1970 when user run DESC FORMATTED table command
In hive its displayed as "UNKNOWN" which makes more sense than displaying wrong date. seems to be a limitation as of now even from hive, better we can follow the hive behavior unless the limitation has been resolved from hive.
spark client output
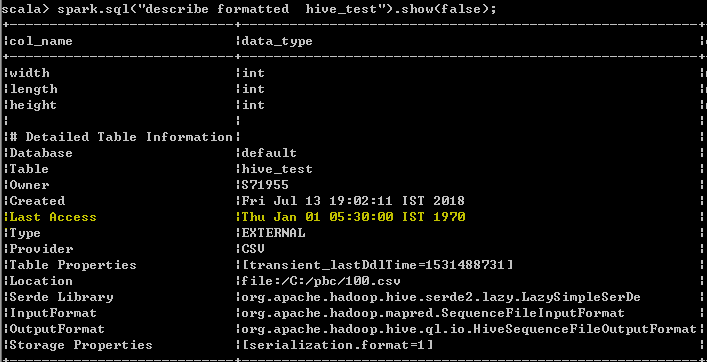
Hive client output
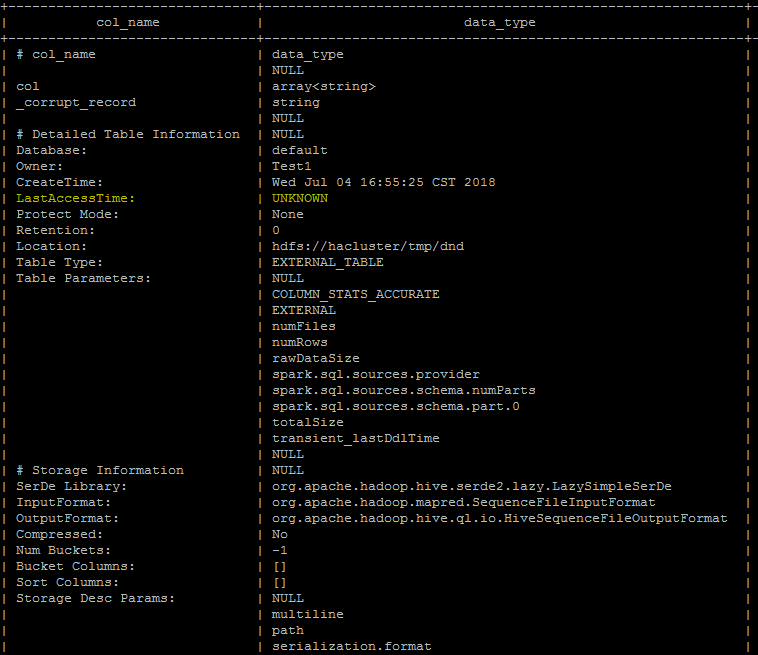
## How was this patch tested?
UT has been added which makes sure that the wrong date "Thu Jan 01 05:30:00 IST 1970 "
shall not be added as value for the Last Access property
Author: s71955 <sujithchacko.2010@gmail.com>
Closes#21775 from sujith71955/master_hive.
## What changes were proposed in this pull request?
This updates the DataSourceV2 API to use InternalRow instead of Row for the default case with no scan mix-ins.
Support for readers that produce Row is added through SupportsDeprecatedScanRow, which matches the previous API. Readers that used Row now implement this class and should be migrated to InternalRow.
Readers that previously implemented SupportsScanUnsafeRow have been migrated to use no SupportsScan mix-ins and produce InternalRow.
## How was this patch tested?
This uses existing tests.
Author: Ryan Blue <blue@apache.org>
Closes#21118 from rdblue/SPARK-23325-datasource-v2-internal-row.
## What changes were proposed in this pull request?
It's minor and trivial but looks 2000 input is good enough to reproduce and test in SPARK-22499.
## How was this patch tested?
Manually brought the change and tested.
Locally tested:
Before: 3m 21s 288ms
After: 1m 29s 134ms
Given the latest successful build took:
```
ArithmeticExpressionSuite:
- SPARK-22499: Least and greatest should not generate codes beyond 64KB (7 minutes, 49 seconds)
```
I expect it's going to save 4ish mins.
Author: hyukjinkwon <gurwls223@apache.org>
Closes#21855 from HyukjinKwon/minor-fix-suite.
## What changes were proposed in this pull request?
Modified the canonicalized to not case-insensitive.
Before the PR, cache can't work normally if there are case letters in SQL,
for example:
sql("CREATE TABLE IF NOT EXISTS src (key INT, value STRING) USING hive")
sql("select key, sum(case when Key > 0 then 1 else 0 end) as positiveNum " +
"from src group by key").cache().createOrReplaceTempView("src_cache")
sql(
s"""select a.key
from
(select key from src_cache where positiveNum = 1)a
left join
(select key from src_cache )b
on a.key=b.key
""").explain
The physical plan of the sql is:
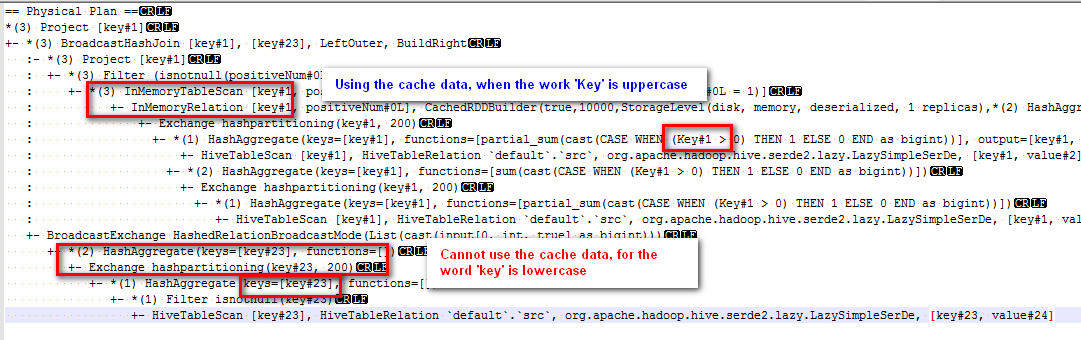
The subquery "select key from src_cache where positiveNum = 1" on the left of join can use the cache data, but the subquery "select key from src_cache" on the right of join cannot use the cache data.
## How was this patch tested?
new added test
Author: 10129659 <chen.yanshan@zte.com.cn>
Closes#21823 from eatoncys/canonicalized.
## What changes were proposed in this pull request?
Modify the strategy in ColumnPruning to add a Project between ScriptTransformation and its child, this strategy can reduce the scan time especially in the scenario of the table has many columns.
## How was this patch tested?
Add UT in ColumnPruningSuite and ScriptTransformationSuite.
Author: Yuanjian Li <xyliyuanjian@gmail.com>
Closes#21839 from xuanyuanking/SPARK-24339.
## What changes were proposed in this pull request?
Streaming queries with watermarks do not work with Trigger.Once because of the following.
- Watermark is updated in the driver memory after a batch completes, but it is persisted to checkpoint (in the offset log) only when the next batch is planned
- In trigger.once, the query terminated as soon as one batch has completed. Hence, the updated watermark is never persisted anywhere.
The simple solution is to persist the updated watermark value in the commit log when a batch is marked as completed. Then the next batch, in the next trigger.once run can pick it up from the commit log.
## How was this patch tested?
new unit tests
Co-authored-by: Tathagata Das <tathagata.das1565gmail.com>
Co-authored-by: c-horn <chorn4033gmail.com>
Author: Tathagata Das <tathagata.das1565@gmail.com>
Closes#21746 from tdas/SPARK-24699.
## What changes were proposed in this pull request?
Since Spark has provided fairly clear interfaces for adding user-defined optimization rules, it would be nice to have an easy-to-use interface for excluding an optimization rule from the Spark query optimizer as well.
This would make customizing Spark optimizer easier and sometimes could debugging issues too.
- Add a new config spark.sql.optimizer.excludedRules, with the value being a list of rule names separated by comma.
- Modify the current batches method to remove the excluded rules from the default batches. Log the rules that have been excluded.
- Split the existing default batches into "post-analysis batches" and "optimization batches" so that only rules in the "optimization batches" can be excluded.
## How was this patch tested?
Add a new test suite: OptimizerRuleExclusionSuite
Author: maryannxue <maryannxue@apache.org>
Closes#21764 from maryannxue/rule-exclusion.
## What changes were proposed in this pull request?
according to the context, "makeRDDForTablePartitions" in assert message should be "makeRDDForPartitionedTable", because "makeRDDForTablePartitions" does't exist in spark code.
## How was this patch tested?
unit tests
Please review http://spark.apache.org/contributing.html before opening a pull request.
Author: SongYadong <song.yadong1@zte.com.cn>
Closes#21836 from SongYadong/assert_info_modify.
## What changes were proposed in this pull request?
1. Add a new function from_avro for parsing a binary column of avro format and converting it into its corresponding catalyst value.
2. Add a new function to_avro for converting a column into binary of avro format with the specified schema.
I created #21774 for this, but it failed the build https://amplab.cs.berkeley.edu/jenkins/view/Spark%20QA%20Compile/job/spark-master-compile-maven-hadoop-2.6/7902/
Additional changes In this PR:
1. Add `scalacheck` dependency in pom.xml to resolve the failure.
2. Update the `log4j.properties` to make it consistent with other modules.
## How was this patch tested?
Unit test
Compile with different commands:
```
./build/mvn --force -DzincPort=3643 -DskipTests -Phadoop-2.6 -Phive-thriftserver -Pkinesis-asl -Pspark-ganglia-lgpl -Pmesos -Pyarn compile test-compile
./build/mvn --force -DzincPort=3643 -DskipTests -Phadoop-2.7 -Phive-thriftserver -Pkinesis-asl -Pspark-ganglia-lgpl -Pmesos -Pyarn compile test-compile
./build/mvn --force -DzincPort=3643 -DskipTests -Phadoop-3.1 -Phive-thriftserver -Pkinesis-asl -Pspark-ganglia-lgpl -Pmesos -Pyarn compile test-compile
```
Author: Gengliang Wang <gengliang.wang@databricks.com>
Closes#21838 from gengliangwang/from_and_to_avro.
## What changes were proposed in this pull request?
We get a NPE when we have a filter on a partition column of the form `col in (x, null)`. This is due to the filter converter in HiveShim not handling `null`s correctly. This patch fixes this bug while still pushing down as much of the partition pruning predicates as possible, by filtering out `null`s from any `in` predicate. Since Hive only supports very simple partition pruning filters, this change should preserve correctness.
## How was this patch tested?
Unit tests, manual tests
Author: William Sheu <william.sheu@databricks.com>
Closes#21832 from PenguinToast/partition-pruning-npe.
## What changes were proposed in this pull request?
Currently, the Analyzer throws an exception if your try to nest a generator. However, it special cases generators "nested" in an alias, and allows that. If you try to alias a generator twice, it is not caught by the special case, so an exception is thrown.
This PR trims the unnecessary, non-top-level aliases, so that the generator is allowed.
## How was this patch tested?
new tests in AnalysisSuite.
Author: Brandon Krieger <bkrieger@palantir.com>
Closes#21508 from bkrieger/bk/SPARK-24488.
This commit adds the `cascadeTruncate` option to the JDBC datasource
API, for databases that support this functionality (PostgreSQL and
Oracle at the moment). This allows for applying a cascading truncate
that affects tables that have foreign key constraints on the table
being truncated.
## What changes were proposed in this pull request?
Add `cascadeTruncate` option to JDBC datasource API. Allow this to affect the
`TRUNCATE` query for databases that support this option.
## How was this patch tested?
Existing tests for `truncateQuery` were updated. Also, an additional test was added
to ensure that the correct syntax was applied, and that enabling the config for databases
that do not support this option does not result in invalid queries.
Author: Daniel van der Ende <daniel.vanderende@gmail.com>
Closes#20057 from danielvdende/SPARK-22880.
## What changes were proposed in this pull request?
Add a new function from_avro for parsing a binary column of avro format and converting it into its corresponding catalyst value.
Add a new function to_avro for converting a column into binary of avro format with the specified schema.
This PR is in progress. Will add test cases.
## How was this patch tested?
Author: Gengliang Wang <gengliang.wang@databricks.com>
Closes#21774 from gengliangwang/from_and_to_avro.
## What changes were proposed in this pull request?
### What's problem?
In some cases, sub scalar query could throw a NPE, which is caused in execution side.
```
java.lang.NullPointerException
at org.apache.spark.sql.execution.FileSourceScanExec.<init>(DataSourceScanExec.scala:169)
at org.apache.spark.sql.execution.FileSourceScanExec.doCanonicalize(DataSourceScanExec.scala:526)
at org.apache.spark.sql.execution.FileSourceScanExec.doCanonicalize(DataSourceScanExec.scala:159)
at org.apache.spark.sql.catalyst.plans.QueryPlan.canonicalized$lzycompute(QueryPlan.scala:211)
at org.apache.spark.sql.catalyst.plans.QueryPlan.canonicalized(QueryPlan.scala:210)
at org.apache.spark.sql.catalyst.plans.QueryPlan$$anonfun$3.apply(QueryPlan.scala:225)
at org.apache.spark.sql.catalyst.plans.QueryPlan$$anonfun$3.apply(QueryPlan.scala:225)
at scala.collection.TraversableLike$$anonfun$map$1.apply(TraversableLike.scala:234)
at scala.collection.TraversableLike$$anonfun$map$1.apply(TraversableLike.scala:234)
at scala.collection.immutable.List.foreach(List.scala:392)
at scala.collection.TraversableLike$class.map(TraversableLike.scala:234)
at scala.collection.immutable.List.map(List.scala:296)
at org.apache.spark.sql.catalyst.plans.QueryPlan.doCanonicalize(QueryPlan.scala:225)
at org.apache.spark.sql.catalyst.plans.QueryPlan.canonicalized$lzycompute(QueryPlan.scala:211)
at org.apache.spark.sql.catalyst.plans.QueryPlan.canonicalized(QueryPlan.scala:210)
at org.apache.spark.sql.catalyst.plans.QueryPlan.sameResult(QueryPlan.scala:258)
at org.apache.spark.sql.execution.ScalarSubquery.semanticEquals(subquery.scala:58)
at org.apache.spark.sql.catalyst.expressions.EquivalentExpressions$Expr.equals(EquivalentExpressions.scala:36)
at scala.collection.mutable.HashTable$class.elemEquals(HashTable.scala:364)
at scala.collection.mutable.HashMap.elemEquals(HashMap.scala:40)
at scala.collection.mutable.HashTable$class.scala$collection$mutable$HashTable$$findEntry0(HashTable.scala:139)
at scala.collection.mutable.HashTable$class.findEntry(HashTable.scala:135)
at scala.collection.mutable.HashMap.findEntry(HashMap.scala:40)
at scala.collection.mutable.HashMap.get(HashMap.scala:70)
at org.apache.spark.sql.catalyst.expressions.EquivalentExpressions.addExpr(EquivalentExpressions.scala:56)
at org.apache.spark.sql.catalyst.expressions.EquivalentExpressions.addExprTree(EquivalentExpressions.scala:97)
at org.apache.spark.sql.catalyst.expressions.EquivalentExpressions$$anonfun$addExprTree$1.apply(EquivalentExpressions.scala:98)
at org.apache.spark.sql.catalyst.expressions.EquivalentExpressions$$anonfun$addExprTree$1.apply(EquivalentExpressions.scala:98)
at scala.collection.immutable.List.foreach(List.scala:392)
at org.apache.spark.sql.catalyst.expressions.EquivalentExpressions.addExprTree(EquivalentExpressions.scala:98)
at org.apache.spark.sql.catalyst.expressions.codegen.CodegenContext$$anonfun$subexpressionElimination$1.apply(CodeGenerator.scala:1102)
at org.apache.spark.sql.catalyst.expressions.codegen.CodegenContext$$anonfun$subexpressionElimination$1.apply(CodeGenerator.scala:1102)
at scala.collection.immutable.List.foreach(List.scala:392)
at org.apache.spark.sql.catalyst.expressions.codegen.CodegenContext.subexpressionElimination(CodeGenerator.scala:1102)
at org.apache.spark.sql.catalyst.expressions.codegen.CodegenContext.generateExpressions(CodeGenerator.scala:1154)
at org.apache.spark.sql.catalyst.expressions.codegen.GenerateUnsafeProjection$.createCode(GenerateUnsafeProjection.scala:270)
at org.apache.spark.sql.catalyst.expressions.codegen.GenerateUnsafeProjection$.create(GenerateUnsafeProjection.scala:319)
at org.apache.spark.sql.catalyst.expressions.codegen.GenerateUnsafeProjection$.generate(GenerateUnsafeProjection.scala:308)
at org.apache.spark.sql.catalyst.expressions.UnsafeProjection$.create(Projection.scala:181)
at org.apache.spark.sql.execution.ProjectExec$$anonfun$9.apply(basicPhysicalOperators.scala:71)
at org.apache.spark.sql.execution.ProjectExec$$anonfun$9.apply(basicPhysicalOperators.scala:70)
at org.apache.spark.rdd.RDD$$anonfun$mapPartitionsWithIndexInternal$1$$anonfun$apply$24.apply(RDD.scala:818)
at org.apache.spark.rdd.RDD$$anonfun$mapPartitionsWithIndexInternal$1$$anonfun$apply$24.apply(RDD.scala:818)
at org.apache.spark.rdd.MapPartitionsRDD.compute(MapPartitionsRDD.scala:38)
at org.apache.spark.rdd.RDD.computeOrReadCheckpoint(RDD.scala:324)
at org.apache.spark.rdd.RDD.iterator(RDD.scala:288)
at org.apache.spark.rdd.MapPartitionsRDD.compute(MapPartitionsRDD.scala:38)
at org.apache.spark.rdd.RDD.computeOrReadCheckpoint(RDD.scala:324)
at org.apache.spark.rdd.RDD.iterator(RDD.scala:288)
at org.apache.spark.scheduler.ResultTask.runTask(ResultTask.scala:87)
at org.apache.spark.scheduler.Task.run(Task.scala:109)
at org.apache.spark.executor.Executor$TaskRunner.run(Executor.scala:367)
at java.util.concurrent.ThreadPoolExecutor.runWorker(ThreadPoolExecutor.java:1149)
at java.util.concurrent.ThreadPoolExecutor$Worker.run(ThreadPoolExecutor.java:624)
at java.lang.Thread.run(Thread.java:748)
```
### How does this happen?
Here looks what happen now:
1. Sub scalar query was made (for instance `SELECT (SELECT id FROM foo)`).
2. Try to extract some common expressions (via `CodeGenerator.subexpressionElimination`) so that it can generates some common codes and can be reused.
3. During this, seems it extracts some expressions that can be reused (via `EquivalentExpressions.addExprTree`)
b2deef64f6/sql/catalyst/src/main/scala/org/apache/spark/sql/catalyst/expressions/codegen/CodeGenerator.scala (L1102)
4. During this, if the hash (`EquivalentExpressions.Expr.hashCode`) happened to be the same at `EquivalentExpressions.addExpr` anyhow, `EquivalentExpressions.Expr.equals` is called to identify object in the same hash, which eventually calls `semanticEquals` in `ScalarSubquery`
087879a77a/sql/catalyst/src/main/scala/org/apache/spark/sql/catalyst/expressions/EquivalentExpressions.scala (L54)087879a77a/sql/catalyst/src/main/scala/org/apache/spark/sql/catalyst/expressions/EquivalentExpressions.scala (L36)
5. `ScalarSubquery`'s `semanticEquals` needs `SubqueryExec`'s `sameResult`
77a2fc5b52/sql/core/src/main/scala/org/apache/spark/sql/execution/subquery.scala (L58)
6. `SubqueryExec`'s `sameResult` requires a canonicalized plan which calls `FileSourceScanExec`'s `doCanonicalize`
e008ad1752/sql/catalyst/src/main/scala/org/apache/spark/sql/catalyst/plans/QueryPlan.scala (L258)
7. In `FileSourceScanExec`'s `doCanonicalize`, `FileSourceScanExec`'s `relation` is required but seems `transient` so it becomes `null`.
e76b0124fb/sql/core/src/main/scala/org/apache/spark/sql/execution/DataSourceScanExec.scala (L527)e76b0124fb/sql/core/src/main/scala/org/apache/spark/sql/execution/DataSourceScanExec.scala (L160)
8. NPE is thrown.
\*1. driver side
\*2., 3., 4., 5., 6., 7., 8. executor side
Note that most of cases, it looks fine because we will usually call:
087879a77a/sql/catalyst/src/main/scala/org/apache/spark/sql/catalyst/expressions/EquivalentExpressions.scala (L40)
which make a canonicalized plan via:
b045315e5d/sql/catalyst/src/main/scala/org/apache/spark/sql/catalyst/expressions/Expression.scala (L192)77a2fc5b52/sql/core/src/main/scala/org/apache/spark/sql/execution/subquery.scala (L52)
### How to reproduce?
This looks what happened now. I can reproduce this by a bit of messy way:
```diff
diff --git a/sql/catalyst/src/main/scala/org/apache/spark/sql/catalyst/expressions/EquivalentExpressions.scala b/sql/catalyst/src/main/scala/org/apache/spark/sql/catalyst/expressions/EquivalentExpressions.scala
index 8d06804ce1e..d25fc9a7ba9 100644
--- a/sql/catalyst/src/main/scala/org/apache/spark/sql/catalyst/expressions/EquivalentExpressions.scala
+++ b/sql/catalyst/src/main/scala/org/apache/spark/sql/catalyst/expressions/EquivalentExpressions.scala
-37,7 +37,9 class EquivalentExpressions {
case _ => false
}
- override def hashCode: Int = e.semanticHash()
+ override def hashCode: Int = {
+ 1
+ }
}
```
```scala
spark.range(1).write.mode("overwrite").parquet("/tmp/foo")
spark.read.parquet("/tmp/foo").createOrReplaceTempView("foo")
spark.conf.set("spark.sql.codegen.wholeStage", false)
sql("SELECT (SELECT id FROM foo) == (SELECT id FROM foo)").collect()
```
### How does this PR fix?
- Make all variables that access to `FileSourceScanExec`'s `relation` as `lazy val` so that we avoid NPE. This is a temporary fix.
- Allow `makeCopy` in `SparkPlan` without Spark session too. This looks still able to be accessed within executor side. For instance:
```
at org.apache.spark.sql.execution.SparkPlan.makeCopy(SparkPlan.scala:70)
at org.apache.spark.sql.execution.SparkPlan.makeCopy(SparkPlan.scala:47)
at org.apache.spark.sql.catalyst.trees.TreeNode.withNewChildren(TreeNode.scala:233)
at org.apache.spark.sql.catalyst.plans.QueryPlan.doCanonicalize(QueryPlan.scala:243)
at org.apache.spark.sql.catalyst.plans.QueryPlan.canonicalized$lzycompute(QueryPlan.scala:211)
at org.apache.spark.sql.catalyst.plans.QueryPlan.canonicalized(QueryPlan.scala:210)
at org.apache.spark.sql.catalyst.plans.QueryPlan.sameResult(QueryPlan.scala:258)
at org.apache.spark.sql.execution.ScalarSubquery.semanticEquals(subquery.scala:58)
at org.apache.spark.sql.catalyst.expressions.EquivalentExpressions$Expr.equals(EquivalentExpressions.scala:36)
at scala.collection.mutable.HashTable$class.elemEquals(HashTable.scala:364)
at scala.collection.mutable.HashMap.elemEquals(HashMap.scala:40)
at scala.collection.mutable.HashTable$class.scala$collection$mutable$HashTable$$findEntry0(HashTable.scala:139)
at scala.collection.mutable.HashTable$class.findEntry(HashTable.scala:135)
at scala.collection.mutable.HashMap.findEntry(HashMap.scala:40)
at scala.collection.mutable.HashMap.get(HashMap.scala:70)
at org.apache.spark.sql.catalyst.expressions.EquivalentExpressions.addExpr(EquivalentExpressions.scala:54)
at org.apache.spark.sql.catalyst.expressions.EquivalentExpressions.addExprTree(EquivalentExpressions.scala:95)
at org.apache.spark.sql.catalyst.expressions.EquivalentExpressions$$anonfun$addExprTree$1.apply(EquivalentExpressions.scala:96)
at org.apache.spark.sql.catalyst.expressions.EquivalentExpressions$$anonfun$addExprTree$1.apply(EquivalentExpressions.scala:96)
at scala.collection.immutable.List.foreach(List.scala:392)
at org.apache.spark.sql.catalyst.expressions.EquivalentExpressions.addExprTree(EquivalentExpressions.scala:96)
at org.apache.spark.sql.catalyst.expressions.codegen.CodegenContext$$anonfun$subexpressionElimination$1.apply(CodeGenerator.scala:1102)
at org.apache.spark.sql.catalyst.expressions.codegen.CodegenContext$$anonfun$subexpressionElimination$1.apply(CodeGenerator.scala:1102)
at scala.collection.immutable.List.foreach(List.scala:392)
at org.apache.spark.sql.catalyst.expressions.codegen.CodegenContext.subexpressionElimination(CodeGenerator.scala:1102)
at org.apache.spark.sql.catalyst.expressions.codegen.CodegenContext.generateExpressions(CodeGenerator.scala:1154)
at org.apache.spark.sql.catalyst.expressions.codegen.GenerateUnsafeProjection$.createCode(GenerateUnsafeProjection.scala:270)
at org.apache.spark.sql.catalyst.expressions.codegen.GenerateUnsafeProjection$.create(GenerateUnsafeProjection.scala:319)
at org.apache.spark.sql.catalyst.expressions.codegen.GenerateUnsafeProjection$.generate(GenerateUnsafeProjection.scala:308)
at org.apache.spark.sql.catalyst.expressions.UnsafeProjection$.create(Projection.scala:181)
at org.apache.spark.sql.execution.ProjectExec$$anonfun$9.apply(basicPhysicalOperators.scala:71)
at org.apache.spark.sql.execution.ProjectExec$$anonfun$9.apply(basicPhysicalOperators.scala:70)
at org.apache.spark.rdd.RDD$$anonfun$mapPartitionsWithIndexInternal$1$$anonfun$apply$24.apply(RDD.scala:818)
at org.apache.spark.rdd.RDD$$anonfun$mapPartitionsWithIndexInternal$1$$anonfun$apply$24.apply(RDD.scala:818)
at org.apache.spark.rdd.MapPartitionsRDD.compute(MapPartitionsRDD.scala:38)
at org.apache.spark.rdd.RDD.computeOrReadCheckpoint(RDD.scala:324)
at org.apache.spark.rdd.RDD.iterator(RDD.scala:288)
at org.apache.spark.rdd.MapPartitionsRDD.compute(MapPartitionsRDD.scala:38)
at org.apache.spark.rdd.RDD.computeOrReadCheckpoint(RDD.scala:324)
at org.apache.spark.rdd.RDD.iterator(RDD.scala:288)
at org.apache.spark.scheduler.ResultTask.runTask(ResultTask.scala:87)
at org.apache.spark.scheduler.Task.run(Task.scala:109)
at org.apache.spark.executor.Executor$TaskRunner.run(Executor.scala:367)
at java.util.concurrent.ThreadPoolExecutor.runWorker(ThreadPoolExecutor.java:1149)
at java.util.concurrent.ThreadPoolExecutor$Worker.run(ThreadPoolExecutor.java:624)
at java.lang.Thread.run(Thread.java:748)
```
This PR takes over https://github.com/apache/spark/pull/20856.
## How was this patch tested?
Manually tested and unit test was added.
Closes#20856
Author: hyukjinkwon <gurwls223@apache.org>
Closes#21815 from HyukjinKwon/SPARK-23731.
## What changes were proposed in this pull request?
Refactor `Concat` and `MapConcat` to:
- avoid creating concatenator object for each row.
- make `Concat` handle `containsNull` properly.
- make `Concat` shortcut if `null` child is found.
## How was this patch tested?
Added some tests and existing tests.
Author: Takuya UESHIN <ueshin@databricks.com>
Closes#21824 from ueshin/issues/SPARK-24871/refactor_concat_mapconcat.
## What changes were proposed in this pull request?
Enhances the parser and analyzer to support ANSI compliant syntax for GROUPING SET. As part of this change we derive the grouping expressions from user supplied groupings in the grouping sets clause.
```SQL
SELECT c1, c2, max(c3)
FROM t1
GROUP BY GROUPING SETS ((c1), (c1, c2))
```
## How was this patch tested?
Added tests in SQLQueryTestSuite and ResolveGroupingAnalyticsSuite.
Please review http://spark.apache.org/contributing.html before opening a pull request.
Author: Dilip Biswal <dbiswal@us.ibm.com>
Closes#21813 from dilipbiswal/spark-24424.
## What changes were proposed in this pull request?
As stated in https://github.com/apache/spark/pull/21321, in the error messages we should use `catalogString`. This is not the case, as SPARK-22893 used `simpleString` in order to have the same representation everywhere and it missed some places.
The PR unifies the messages using alway the `catalogString` representation of the dataTypes in the messages.
## How was this patch tested?
existing/modified UTs
Author: Marco Gaido <marcogaido91@gmail.com>
Closes#21804 from mgaido91/SPARK-24268_catalog.
## What changes were proposed in this pull request?
`RateSourceSuite` may leave garbage files under `sql/core/dummy`, we should use a corrected temp directory
## How was this patch tested?
test only
Author: Wenchen Fan <wenchen@databricks.com>
Closes#21817 from cloud-fan/minor.
## What changes were proposed in this pull request?
Made ExprId hashCode independent of jvmId to make canonicalization independent of JVM, by overriding hashCode (and necessarily also equality) to depend on id only
## How was this patch tested?
Created a unit test ExprIdSuite
Ran all unit tests of sql/catalyst
Author: Ger van Rossum <gvr@users.noreply.github.com>
Closes#21806 from gvr/spark24846-canonicalization.
## What changes were proposed in this pull request?
Currently, the group state of user-defined-type is encoded as top-level columns in the UnsafeRows stores in the state store. The timeout timestamp is also saved as (when needed) as the last top-level column. Since the group state is serialized to top-level columns, you cannot save "null" as a value of state (setting null in all the top-level columns is not equivalent). So we don't let the user set the timeout without initializing the state for a key. Based on user experience, this leads to confusion.
This PR is to change the row format such that the state is saved as nested columns. This would allow the state to be set to null, and avoid these confusing corner cases. However, queries recovering from existing checkpoint will use the previous format to maintain compatibility with existing production queries.
## How was this patch tested?
Refactored existing end-to-end tests and added new tests for explicitly testing obj-to-row conversion for both state formats.
Author: Tathagata Das <tathagata.das1565@gmail.com>
Closes#21739 from tdas/SPARK-22187-1.
## What changes were proposed in this pull request?
Currently the same Parquet footer is read twice in the function `buildReaderWithPartitionValues` of ParquetFileFormat if filter push down is enabled.
Fix it with simple changes.
## How was this patch tested?
Unit test
Author: Gengliang Wang <gengliang.wang@databricks.com>
Closes#21814 from gengliangwang/parquetFooter.
## What changes were proposed in this pull request?
This patch proposes breaking down configuration of retaining batch size on state into two pieces: files and in memory (cache). While this patch reuses existing configuration for files, it introduces new configuration, "spark.sql.streaming.maxBatchesToRetainInMemory" to configure max count of batch to retain in memory.
## How was this patch tested?
Apply this patch on top of SPARK-24441 (https://github.com/apache/spark/pull/21469), and manually tested in various workloads to ensure overall size of states in memory is around 2x or less of the size of latest version of state, while it was 10x ~ 80x before applying the patch.
Author: Jungtaek Lim <kabhwan@gmail.com>
Closes#21700 from HeartSaVioR/SPARK-24717.
## What changes were proposed in this pull request?
It's a little tricky and fragile to use a dummy filter to switch codegen on/off. For now we should use local/cached relation to switch. In the future when we are able to use a config to turn off codegen, we shall use that.
## How was this patch tested?
test only PR.
Author: Wenchen Fan <wenchen@databricks.com>
Closes#21795 from cloud-fan/follow.
## What changes were proposed in this pull request?
Fix regexes in spark-sql command examples.
This takes over https://github.com/apache/spark/pull/18477
## How was this patch tested?
Existing tests. I verified the existing example doesn't work in spark-sql, but new ones does.
Author: Sean Owen <srowen@gmail.com>
Closes#21808 from srowen/SPARK-21261.
## What changes were proposed in this pull request?
1. Extend the Parser to enable parsing a column list as the pivot column.
2. Extend the Parser and the Pivot node to enable parsing complex expressions with aliases as the pivot value.
3. Add type check and constant check in Analyzer for Pivot node.
## How was this patch tested?
Add tests in pivot.sql
Author: maryannxue <maryannxue@apache.org>
Closes#21720 from maryannxue/spark-24164.
## What changes were proposed in this pull request?
In DatasetSuite.scala, in the 1299 line,
test("SPARK-19896: cannot have circular references in in case class") ,
there are duplicate words "in in". We can get rid of one.
## How was this patch tested?
(Please explain how this patch was tested. E.g. unit tests, integration tests, manual tests)
(If this patch involves UI changes, please attach a screenshot; otherwise, remove this)
Please review http://spark.apache.org/contributing.html before opening a pull request.
Author: 韩田田00222924 <han.tiantian@zte.com.cn>
Closes#21767 from httfighter/inin.
## What changes were proposed in this pull request?
This pr fixes lint-java and Scala 2.12 build.
lint-java:
```
[ERROR] src/test/resources/log4j.properties:[0] (misc) NewlineAtEndOfFile: File does not end with a newline.
```
Scala 2.12 build:
```
[error] /.../sql/core/src/main/scala/org/apache/spark/sql/execution/streaming/continuous/ContinuousCoalesceRDD.scala:121: overloaded method value addTaskCompletionListener with alternatives:
[error] (f: org.apache.spark.TaskContext => Unit)org.apache.spark.TaskContext <and>
[error] (listener: org.apache.spark.util.TaskCompletionListener)org.apache.spark.TaskContext
[error] cannot be applied to (org.apache.spark.TaskContext => java.util.List[Runnable])
[error] context.addTaskCompletionListener { ctx =>
[error] ^
```
## How was this patch tested?
Manually executed lint-java and Scala 2.12 build in my local environment.
Author: Takuya UESHIN <ueshin@databricks.com>
Closes#21801 from ueshin/issues/SPARK-24386_24768/fix_build.
## What changes were proposed in this pull request?
This issue aims to upgrade Apache ORC library from 1.4.4 to 1.5.2 in order to bring the following benefits into Apache Spark.
- [ORC-91](https://issues.apache.org/jira/browse/ORC-91) Support for variable length blocks in HDFS (The current space wasted in ORC to padding is known to be 5%.)
- [ORC-344](https://issues.apache.org/jira/browse/ORC-344) Support for using Decimal64ColumnVector
In addition to that, Apache Hive 3.1 and 3.2 will use ORC 1.5.1 ([HIVE-19669](https://issues.apache.org/jira/browse/HIVE-19465)) and 1.5.2 ([HIVE-19792](https://issues.apache.org/jira/browse/HIVE-19792)) respectively. This will improve the compatibility between Apache Spark and Apache Hive by sharing the common library.
## How was this patch tested?
Pass the Jenkins with all existing tests.
Author: Dongjoon Hyun <dongjoon@apache.org>
Closes#21582 from dongjoon-hyun/SPARK-24576.
## What changes were proposed in this pull request?
Two new rules in the logical plan optimizers are added.
1. When there is only one element in the **`Collection`**, the
physical plan will be optimized to **`EqualTo`**, so predicate
pushdown can be used.
```scala
profileDF.filter( $"profileID".isInCollection(Set(6))).explain(true)
"""
|== Physical Plan ==
|*(1) Project [profileID#0]
|+- *(1) Filter (isnotnull(profileID#0) && (profileID#0 = 6))
| +- *(1) FileScan parquet [profileID#0] Batched: true, Format: Parquet,
| PartitionFilters: [],
| PushedFilters: [IsNotNull(profileID), EqualTo(profileID,6)],
| ReadSchema: struct<profileID:int>
""".stripMargin
```
2. When the **`Collection`** is empty, and the input is nullable, the
logical plan will be simplified to
```scala
profileDF.filter( $"profileID".isInCollection(Set())).explain(true)
"""
|== Optimized Logical Plan ==
|Filter if (isnull(profileID#0)) null else false
|+- Relation[profileID#0] parquet
""".stripMargin
```
TODO:
1. For multiple conditions with numbers less than certain thresholds,
we should still allow predicate pushdown.
2. Optimize the **`In`** using **`tableswitch`** or **`lookupswitch`**
when the numbers of the categories are low, and they are **`Int`**,
**`Long`**.
3. The default immutable hash trees set is slow for query, and we
should do benchmark for using different set implementation for faster
query.
4. **`filter(if (condition) null else false)`** can be optimized to false.
## How was this patch tested?
Couple new tests are added.
Author: DB Tsai <d_tsai@apple.com>
Closes#21797 from dbtsai/optimize-in.
## What changes were proposed in this pull request?
Remove the non-negative checks of window start time to make window support negative start time, and add a check to guarantee the absolute value of start time is less than slide duration.
## How was this patch tested?
New unit tests.
Author: HanShuliang <kevinzwx1992@gmail.com>
Closes#18903 from KevinZwx/dev.
## What changes were proposed in this pull request?
Test HiveExternalCatalogVersionsSuite vs only current Spark releases
## How was this patch tested?
`HiveExternalCatalogVersionsSuite`
Author: Sean Owen <srowen@gmail.com>
Closes#21793 from srowen/SPARK-24813.3.
## What changes were proposed in this pull request?
The PR tries to avoid serialization of private fields of already added collection functions and follows up on comments in [SPARK-23922](https://github.com/apache/spark/pull/21028) and [SPARK-23935](https://github.com/apache/spark/pull/21236)
## How was this patch tested?
Run tests from:
- CollectionExpressionSuite.scala
- DataFrameFunctionsSuite.scala
Author: Marek Novotny <mn.mikke@gmail.com>
Closes#21352 from mn-mikke/SPARK-24305.
## What changes were proposed in this pull request?
Three legacy statements are removed by this patch:
- in HiveExternalCatalog: The withClient wrapper is not necessary for the private method getRawTable.
- in HiveClientImpl: There are some redundant code in both the tableExists and getTableOption method.
This PR takes over https://github.com/apache/spark/pull/20425
## How was this patch tested?
Existing tests
Closes#20425
Author: hyukjinkwon <gurwls223@apache.org>
Closes#21780 from HyukjinKwon/SPARK-23259.
## What changes were proposed in this pull request?
Two new rules in the logical plan optimizers are added.
1. When there is only one element in the **`Collection`**, the
physical plan will be optimized to **`EqualTo`**, so predicate
pushdown can be used.
```scala
profileDF.filter( $"profileID".isInCollection(Set(6))).explain(true)
"""
|== Physical Plan ==
|*(1) Project [profileID#0]
|+- *(1) Filter (isnotnull(profileID#0) && (profileID#0 = 6))
| +- *(1) FileScan parquet [profileID#0] Batched: true, Format: Parquet,
| PartitionFilters: [],
| PushedFilters: [IsNotNull(profileID), EqualTo(profileID,6)],
| ReadSchema: struct<profileID:int>
""".stripMargin
```
2. When the **`Collection`** is empty, and the input is nullable, the
logical plan will be simplified to
```scala
profileDF.filter( $"profileID".isInCollection(Set())).explain(true)
"""
|== Optimized Logical Plan ==
|Filter if (isnull(profileID#0)) null else false
|+- Relation[profileID#0] parquet
""".stripMargin
```
TODO:
1. For multiple conditions with numbers less than certain thresholds,
we should still allow predicate pushdown.
2. Optimize the **`In`** using **`tableswitch`** or **`lookupswitch`**
when the numbers of the categories are low, and they are **`Int`**,
**`Long`**.
3. The default immutable hash trees set is slow for query, and we
should do benchmark for using different set implementation for faster
query.
4. **`filter(if (condition) null else false)`** can be optimized to false.
## How was this patch tested?
Couple new tests are added.
Author: DB Tsai <d_tsai@apple.com>
Closes#21442 from dbtsai/optimize-in.
## What changes were proposed in this pull request?
We have some functions which need to aware the nullabilities of all children, such as `CreateArray`, `CreateMap`, `Concat`, and so on. Currently we add casts to fix the nullabilities, but the casts might be removed during the optimization phase.
After the discussion, we decided to not add extra casts for just fixing the nullabilities of the nested types, but handle them by functions themselves.
## How was this patch tested?
Modified and added some tests.
Author: Takuya UESHIN <ueshin@databricks.com>
Closes#21704 from ueshin/issues/SPARK-24734/concat_containsnull.
## What changes were proposed in this pull request?
Support Decimal type push down to the parquet data sources.
The Decimal comparator used is: [`BINARY_AS_SIGNED_INTEGER_COMPARATOR`](c6764c4a08/parquet-column/src/main/java/org/apache/parquet/schema/PrimitiveComparator.java (L224-L292)).
## How was this patch tested?
unit tests and manual tests.
**manual tests**:
```scala
spark.range(10000000).selectExpr("id", "cast(id as decimal(9)) as d1", "cast(id as decimal(9, 2)) as d2", "cast(id as decimal(18)) as d3", "cast(id as decimal(18, 4)) as d4", "cast(id as decimal(38)) as d5", "cast(id as decimal(38, 18)) as d6").coalesce(1).write.option("parquet.block.size", 1048576).parquet("/tmp/spark/parquet/decimal")
val df = spark.read.parquet("/tmp/spark/parquet/decimal/")
spark.sql("set spark.sql.parquet.filterPushdown.decimal=true")
// Only read about 1 MB data
df.filter("d2 = 10000").show
// Only read about 1 MB data
df.filter("d4 = 10000").show
spark.sql("set spark.sql.parquet.filterPushdown.decimal=false")
// Read 174.3 MB data
df.filter("d2 = 10000").show
// Read 174.3 MB data
df.filter("d4 = 10000").show
```
Author: Yuming Wang <yumwang@ebay.com>
Closes#21556 from wangyum/SPARK-24549.
## What changes were proposed in this pull request?
In the PR, I propose to move `testFile()` to the common trait `SQLTestUtilsBase` and wrap test files in `AvroSuite` by the method `testFile()` which returns full paths to test files in the resource folder.
Author: Maxim Gekk <maxim.gekk@databricks.com>
Closes#21773 from MaxGekk/test-file.
## What changes were proposed in this pull request?
This pr modified code to project required data from CSV parsed data when column pruning disabled.
In the current master, an exception below happens if `spark.sql.csv.parser.columnPruning.enabled` is false. This is because required formats and CSV parsed formats are different from each other;
```
./bin/spark-shell --conf spark.sql.csv.parser.columnPruning.enabled=false
scala> val dir = "/tmp/spark-csv/csv"
scala> spark.range(10).selectExpr("id % 2 AS p", "id").write.mode("overwrite").partitionBy("p").csv(dir)
scala> spark.read.csv(dir).selectExpr("sum(p)").collect()
18/06/25 13:48:46 ERROR Executor: Exception in task 2.0 in stage 2.0 (TID 7)
java.lang.ClassCastException: org.apache.spark.unsafe.types.UTF8String cannot be cast to java.lang.Integer
at scala.runtime.BoxesRunTime.unboxToInt(BoxesRunTime.java:101)
at org.apache.spark.sql.catalyst.expressions.BaseGenericInternalRow$class.getInt(rows.scala:41)
...
```
## How was this patch tested?
Added tests in `CSVSuite`.
Author: Takeshi Yamamuro <yamamuro@apache.org>
Closes#21657 from maropu/SPARK-24676.
## What changes were proposed in this pull request?
Try only unique ASF mirrors to download Spark release; fall back to Apache archive if no mirrors available or release is not mirrored
## How was this patch tested?
Existing HiveExternalCatalogVersionsSuite
Author: Sean Owen <srowen@gmail.com>
Closes#21776 from srowen/SPARK-24813.
## What changes were proposed in this pull request?
`Timestamp` support pushdown to parquet data source.
Only `TIMESTAMP_MICROS` and `TIMESTAMP_MILLIS` support push down.
## How was this patch tested?
unit tests and benchmark tests
Author: Yuming Wang <yumwang@ebay.com>
Closes#21741 from wangyum/SPARK-24718.
## What changes were proposed in this pull request?
The original pr is: https://github.com/apache/spark/pull/18424
Add a new optimizer rule to convert an IN predicate to an equivalent Parquet filter and add `spark.sql.parquet.pushdown.inFilterThreshold` to control limit thresholds. Different data types have different limit thresholds, this is a copy of data for reference:
Type | limit threshold
-- | --
string | 370
int | 210
long | 285
double | 270
float | 220
decimal | Won't provide better performance before [SPARK-24549](https://issues.apache.org/jira/browse/SPARK-24549)
## How was this patch tested?
unit tests and manual tests
Author: Yuming Wang <yumwang@ebay.com>
Closes#21603 from wangyum/SPARK-17091.
## What changes were proposed in this pull request?
Add `org.apache.derby` to `IsolatedClientLoader`, otherwise it may throw an exception:
```scala
...
[info] Cause: java.sql.SQLException: Failed to start database 'metastore_db' with class loader org.apache.spark.sql.hive.client.IsolatedClientLoader$$anon$12439ab23, see the next exception for details.
[info] at org.apache.derby.impl.jdbc.SQLExceptionFactory.getSQLException(Unknown Source)
[info] at org.apache.derby.impl.jdbc.SQLExceptionFactory.getSQLException(Unknown Source)
[info] at org.apache.derby.impl.jdbc.Util.seeNextException(Unknown Source)
[info] at org.apache.derby.impl.jdbc.EmbedConnection.bootDatabase(Unknown Source)
[info] at org.apache.derby.impl.jdbc.EmbedConnection.<init>(Unknown Source)
[info] at org.apache.derby.jdbc.InternalDriver$1.run(Unknown Source)
...
```
## How was this patch tested?
unit tests and manual tests
Author: Yuming Wang <yumwang@ebay.com>
Closes#20944 from wangyum/SPARK-23831.
## What changes were proposed in this pull request?
When we use a reference from Dataset in filter or sort, which was not used in the prior select, an AnalysisException occurs, e.g.,
```scala
val df = Seq(("test1", 0), ("test2", 1)).toDF("name", "id")
df.select(df("name")).filter(df("id") === 0).show()
```
```scala
org.apache.spark.sql.AnalysisException: Resolved attribute(s) id#6 missing from name#5 in operator !Filter (id#6 = 0).;;
!Filter (id#6 = 0)
+- AnalysisBarrier
+- Project [name#5]
+- Project [_1#2 AS name#5, _2#3 AS id#6]
+- LocalRelation [_1#2, _2#3]
```
This change updates the rule `ResolveMissingReferences` so `Filter` and `Sort` with non-empty `missingInputs` will also be transformed.
## How was this patch tested?
Added tests.
Author: Liang-Chi Hsieh <viirya@gmail.com>
Closes#21745 from viirya/SPARK-24781.
## What changes were proposed in this pull request?
This PR will cache the function name from external catalog, it is used by lookupFunctions in the analyzer, and it is cached for each query plan. The original problem is reported in the [ spark-19737](https://issues.apache.org/jira/browse/SPARK-19737)
## How was this patch tested?
create new test file LookupFunctionsSuite and add test case in SessionCatalogSuite
Author: Kevin Yu <qyu@us.ibm.com>
Closes#20795 from kevinyu98/spark-23486.
## What changes were proposed in this pull request?
Relax the check to allow complex aggregate expressions, like `ceil(sum(col1))` or `sum(col1) + 1`, which roughly means any aggregate expression that could appear in an Aggregate plan except pandas UDF (due to the fact that it is not supported in pivot yet).
## How was this patch tested?
Added 2 tests in pivot.sql
Author: maryannxue <maryannxue@apache.org>
Closes#21753 from maryannxue/pivot-relax-syntax.
## What changes were proposed in this pull request?
The PR is a followup to move the test cases introduced by the original PR in their proper location.
## How was this patch tested?
moved UTs
Author: Marco Gaido <marcogaido91@gmail.com>
Closes#21751 from mgaido91/SPARK-24208_followup.
## What changes were proposed in this pull request?
The reader schema is said to be evolved (or projected) when it changed after the data is written. The followings are already supported in file-based data sources. Note that partition columns are not maintained in files. In this PR, `column` means `non-partition column`.
1. Add a column
2. Hide a column
3. Change a column position
4. Change a column type (upcast)
This issue aims to guarantee users a backward-compatible read-schema test coverage on file-based data sources and to prevent future regressions by *adding read schema tests explicitly*.
Here, we consider safe changes without data loss. For example, data type change should be from small types to larger types like `int`-to-`long`, not vice versa.
As of today, in the master branch, file-based data sources have the following coverage.
File Format | Coverage | Note
----------- | ---------- | ------------------------------------------------
TEXT | N/A | Schema consists of a single string column.
CSV | 1, 2, 4 |
JSON | 1, 2, 3, 4 |
ORC | 1, 2, 3, 4 | Native vectorized ORC reader has the widest coverage among ORC formats.
PARQUET | 1, 2, 3 |
## How was this patch tested?
Pass the Jenkins with newly added test suites.
Author: Dongjoon Hyun <dongjoon@apache.org>
Closes#20208 from dongjoon-hyun/SPARK-SCHEMA-EVOLUTION.
## What changes were proposed in this pull request?
With https://github.com/apache/spark/pull/21389, data source schema is validated on driver side before launching read/write tasks.
However,
1. Putting all the validations together in `DataSourceUtils` is tricky and hard to maintain. On second thought after review, I find that the `OrcFileFormat` in hive package is not matched, so that its validation wrong.
2. `DataSourceUtils.verifyWriteSchema` and `DataSourceUtils.verifyReadSchema` is not supposed to be called in every file format. We can move them to some upper entry.
So, I propose we can add a new method `validateDataType` in FileFormat. File format implementation can override the method to specify its supported/non-supported data types.
Although we should focus on data source V2 API, `FileFormat` should remain workable for some time. Adding this new method should be helpful.
## How was this patch tested?
Unit test
Author: Gengliang Wang <gengliang.wang@databricks.com>
Closes#21667 from gengliangwang/refactorSchemaValidate.
## What changes were proposed in this pull request?
The PR adds the SQL function `array_union`. The behavior of the function is based on Presto's one.
This function returns returns an array of the elements in the union of array1 and array2.
Note: The order of elements in the result is not defined.
## How was this patch tested?
Added UTs
Author: Kazuaki Ishizaki <ishizaki@jp.ibm.com>
Closes#21061 from kiszk/SPARK-23914.
## What changes were proposed in this pull request?
This PR enables a Java bytecode check tool [spotbugs](https://spotbugs.github.io/) to avoid possible integer overflow at multiplication. When an violation is detected, the build process is stopped.
Due to the tool limitation, some other checks will be enabled. In this PR, [these patterns](http://spotbugs-in-kengo-toda.readthedocs.io/en/lqc-list-detectors/detectors.html#findpuzzlers) in `FindPuzzlers` can be detected.
This check is enabled at `compile` phase. Thus, `mvn compile` or `mvn package` launches this check.
## How was this patch tested?
Existing UTs
Author: Kazuaki Ishizaki <ishizaki@jp.ibm.com>
Closes#21542 from kiszk/SPARK-24529.
## What changes were proposed in this pull request?
In the PR, I propose to extend `RuntimeConfig` by new method `isModifiable()` which returns `true` if a config parameter can be modified at runtime (for current session state). For static SQL and core parameters, the method returns `false`.
## How was this patch tested?
Added new test to `RuntimeConfigSuite` for checking Spark core and SQL parameters.
Author: Maxim Gekk <maxim.gekk@databricks.com>
Closes#21730 from MaxGekk/is-modifiable.
## What changes were proposed in this pull request?
The PR simplifies the retrieval of config in `size`, as we can access them from tasks too thanks to SPARK-24250.
## How was this patch tested?
existing UTs
Author: Marco Gaido <marcogaido91@gmail.com>
Closes#21736 from mgaido91/SPARK-24605_followup.
## What changes were proposed in this pull request?
In ProgressReporter for streams, we use the `committedOffsets` as the startOffset and `availableOffsets` as the end offset when reporting the status of a trigger in `finishTrigger`. This is a bad pattern that has existed since the beginning of ProgressReporter and it is bad because its super hard to reason about when `availableOffsets` and `committedOffsets` are updated, and when they are recorded. Case in point, this bug silently existed in ContinuousExecution, since before MicroBatchExecution was refactored.
The correct fix it to record the offsets explicitly. This PR adds a simple method which is explicitly called from MicroBatch/ContinuousExecition before updating the `committedOffsets`.
## How was this patch tested?
Added new tests
Author: Tathagata Das <tathagata.das1565@gmail.com>
Closes#21744 from tdas/SPARK-24697.
## What changes were proposed in this pull request?
A self-join on a dataset which contains a `FlatMapGroupsInPandas` fails because of duplicate attributes. This happens because we are not dealing with this specific case in our `dedupAttr` rules.
The PR fix the issue by adding the management of the specific case
## How was this patch tested?
added UT + manual tests
Author: Marco Gaido <marcogaido91@gmail.com>
Author: Marco Gaido <mgaido@hortonworks.com>
Closes#21737 from mgaido91/SPARK-24208.
## What changes were proposed in this pull request?
The PR proposes to add support for running the same SQL test input files against different configs leading to the same result.
## How was this patch tested?
Involved UTs
Author: Marco Gaido <marcogaido91@gmail.com>
Closes#21568 from mgaido91/SPARK-24562.
## What changes were proposed in this pull request?
This PR is proposing a fix for the output data type of ```If``` and ```CaseWhen``` expression. Upon till now, the implementation of exprassions has ignored nullability of nested types from different execution branches and returned the type of the first branch.
This could lead to an unwanted ```NullPointerException``` from other expressions depending on a ```If```/```CaseWhen``` expression.
Example:
```
val rows = new util.ArrayList[Row]()
rows.add(Row(true, ("a", 1)))
rows.add(Row(false, (null, 2)))
val schema = StructType(Seq(
StructField("cond", BooleanType, false),
StructField("s", StructType(Seq(
StructField("val1", StringType, true),
StructField("val2", IntegerType, false)
)), false)
))
val df = spark.createDataFrame(rows, schema)
df
.select(when('cond, struct(lit("x").as("val1"), lit(10).as("val2"))).otherwise('s) as "res")
.select('res.getField("val1"))
.show()
```
Exception:
```
Exception in thread "main" java.lang.NullPointerException
at org.apache.spark.sql.catalyst.expressions.codegen.UnsafeWriter.write(UnsafeWriter.java:109)
at org.apache.spark.sql.catalyst.expressions.GeneratedClass$SpecificUnsafeProjection.apply(Unknown Source)
at org.apache.spark.sql.execution.LocalTableScanExec$$anonfun$unsafeRows$1.apply(LocalTableScanExec.scala:44)
at org.apache.spark.sql.execution.LocalTableScanExec$$anonfun$unsafeRows$1.apply(LocalTableScanExec.scala:44)
...
```
Output schema:
```
root
|-- res.val1: string (nullable = false)
```
## How was this patch tested?
New test cases added into
- DataFrameSuite.scala
- conditionalExpressions.scala
Author: Marek Novotny <mn.mikke@gmail.com>
Closes#21687 from mn-mikke/SPARK-24165.
## What changes were proposed in this pull request?
Currently, when a streaming query has multiple watermark, the policy is to choose the min of them as the global watermark. This is safe to do as the global watermark moves with the slowest stream, and is therefore is safe as it does not unexpectedly drop some data as late, etc. While this is indeed the safe thing to do, in some cases, you may want the watermark to advance with the fastest stream, that is, take the max of multiple watermarks. This PR is to add that configuration. It makes the following changes.
- Adds a configuration to specify max as the policy.
- Saves the configuration in OffsetSeqMetadata because changing it in the middle can lead to unpredictable results.
- For old checkpoints without the configuration, it assumes the default policy as min (irrespective of the policy set at the session where the query is being restarted). This is to ensure that existing queries are affected in any way.
TODO
- [ ] Add a test for recovery from existing checkpoints.
## How was this patch tested?
New unit test
Author: Tathagata Das <tathagata.das1565@gmail.com>
Closes#21701 from tdas/SPARK-24730.
## What changes were proposed in this pull request?
Support the LIMIT operator in structured streaming.
For streams in append or complete output mode, a stream with a LIMIT operator will return no more than the specified number of rows. LIMIT is still unsupported for the update output mode.
This change reverts e4fee395ec as part of it because it is a better and more complete implementation.
## How was this patch tested?
New and existing unit tests.
Author: Mukul Murthy <mukul.murthy@gmail.com>
Closes#21662 from mukulmurthy/SPARK-24662.
## What changes were proposed in this pull request?
This is the first follow-up of https://github.com/apache/spark/pull/21573 , which was only merged to 2.3.
This PR fixes the memory leak in another way: free the `UnsafeExternalMap` when the task ends. All the data buffers in Spark SQL are using `UnsafeExternalMap` and `UnsafeExternalSorter` under the hood, e.g. sort, aggregate, window, SMJ, etc. `UnsafeExternalSorter` registers a task completion listener to free the resource, we should apply the same thing to `UnsafeExternalMap`.
TODO in the next PR:
do not consume all the inputs when having limit in whole stage codegen.
## How was this patch tested?
existing tests
Author: Wenchen Fan <wenchen@databricks.com>
Closes#21738 from cloud-fan/limit.
## What changes were proposed in this pull request?
As the implementation of the broadcast hash join is independent of the input hash partitioning, reordering keys is not necessary. Thus, we solve this issue by simply removing the broadcast hash join from the reordering rule in EnsureRequirements.
## How was this patch tested?
N/A
Author: Xiao Li <gatorsmile@gmail.com>
Closes#21728 from gatorsmile/cleanER.
## What changes were proposed in this pull request?
SPARK-22893 tried to unify error messages about dataTypes. Unfortunately, still many places were missing the `simpleString` method in other to have the same representation everywhere.
The PR unified the messages using alway the simpleString representation of the dataTypes in the messages.
## How was this patch tested?
existing/modified UTs
Author: Marco Gaido <marcogaido91@gmail.com>
Closes#21321 from mgaido91/SPARK-24268.
## What changes were proposed in this pull request?
Implement map_concat high order function.
This implementation does not pick a winner when the specified maps have overlapping keys. Therefore, this implementation preserves existing duplicate keys in the maps and potentially introduces new duplicates (After discussion with ueshin, we settled on option 1 from [here](https://issues.apache.org/jira/browse/SPARK-23936?focusedCommentId=16464245&page=com.atlassian.jira.plugin.system.issuetabpanels%3Acomment-tabpanel#comment-16464245)).
## How was this patch tested?
New tests
Manual tests
Run all sbt SQL tests
Run all pyspark sql tests
Author: Bruce Robbins <bersprockets@gmail.com>
Closes#21073 from bersprockets/SPARK-23936.
## What changes were proposed in this pull request?
In the PR, I propose to provide a tip to user how to resolve the issue of timeout expiration for broadcast joins. In particular, they can increase the timeout via **spark.sql.broadcastTimeout** or disable the broadcast at all by setting **spark.sql.autoBroadcastJoinThreshold** to `-1`.
## How was this patch tested?
It tested manually from `spark-shell`:
```
scala> spark.conf.set("spark.sql.broadcastTimeout", 1)
scala> val df = spark.range(100).join(spark.range(15).as[Long].map { x =>
Thread.sleep(5000)
x
}).where("id = value")
scala> df.count()
```
```
org.apache.spark.SparkException: Could not execute broadcast in 1 secs. You can increase the timeout for broadcasts via spark.sql.broadcastTimeout or disable broadcast join by setting spark.sql.autoBroadcastJoinThreshold to -1
at org.apache.spark.sql.execution.exchange.BroadcastExchangeExec.doExecuteBroadcast(BroadcastExchangeExec.scala:150)
```
Author: Maxim Gekk <maxim.gekk@databricks.com>
Closes#21727 from MaxGekk/broadcast-timeout-error.
## What changes were proposed in this pull request?
We should use `DataType.sameType` to compare element type in `ArrayContains`, otherwise nullability affects comparison result.
## How was this patch tested?
Added test.
Author: Liang-Chi Hsieh <viirya@gmail.com>
Closes#21724 from viirya/SPARK-24749.
## What changes were proposed in this pull request?
SQL `Aggregator` with output type `Option[Boolean]` creates column of type `StructType`. It's not in consistency with a Dataset of similar java class.
This changes the way `definedByConstructorParams` checks given type. For `Option[_]`, it goes to check its type argument.
## How was this patch tested?
Added test.
Author: Liang-Chi Hsieh <viirya@gmail.com>
Closes#21611 from viirya/SPARK-24569.
## What changes were proposed in this pull request?
Refer to the [`WideSchemaBenchmark`](https://github.com/apache/spark/blob/v2.3.1/sql/core/src/test/scala/org/apache/spark/sql/execution/benchmark/WideSchemaBenchmark.scala) update `FilterPushdownBenchmark`:
1. Write the result to `benchmarks/FilterPushdownBenchmark-results.txt` for easy maintenance.
2. Add more benchmark case: `StringStartsWith`, `Decimal`, `InSet -> InFilters` and `tinyint`.
## How was this patch tested?
manual tests
Author: Yuming Wang <yumwang@ebay.com>
Closes#21677 from wangyum/SPARK-24692.
## What changes were proposed in this pull request?
We can support type coercion between `StructType`s where all the internal types are compatible.
## How was this patch tested?
Added tests.
Author: Takuya UESHIN <ueshin@databricks.com>
Closes#21713 from ueshin/issues/SPARK-24737/structtypecoercion.
## What changes were proposed in this pull request?
If table is renamed to a existing new location, data won't show up.
```
scala> Seq("hello").toDF("a").write.format("parquet").saveAsTable("t")
scala> sql("select * from t").show()
+-----+
| a|
+-----+
|hello|
+-----+
scala> sql("alter table t rename to test")
res2: org.apache.spark.sql.DataFrame = []
scala> sql("select * from test").show()
+---+
| a|
+---+
+---+
```
The file layout is like
```
$ tree test
test
├── gabage
└── t
├── _SUCCESS
└── part-00000-856b0f10-08f1-42d6-9eb3-7719261f3d5e-c000.snappy.parquet
```
In Hive, if the new location exists, the renaming will fail even the location is empty.
We should have the same validation in Catalog, in case of unexpected bugs.
## How was this patch tested?
New unit test.
Author: Gengliang Wang <gengliang.wang@databricks.com>
Closes#21655 from gengliangwang/validate_rename_table.
## What changes were proposed in this pull request?
Current code block manipulation API is immature and hacky. We need a formal API to manipulate code blocks.
The basic idea is making `JavaCode` as `TreeNode`. So we can use familiar `transform` API to manipulate code blocks and expressions in code blocks.
For example, we can replace `SimpleExprValue` in a code block like this:
```scala
code.transformExprValues {
case SimpleExprValue("1 + 1", _) => aliasedParam
}
```
The example use case is splitting code to methods.
For example, we have an `ExprCode` containing generated code. But it is too long and we need to split it as method. Because statement-based expressions can't be directly passed into. We need to transform them as variables first:
```scala
def getExprValues(block: Block): Set[ExprValue] = block match {
case c: CodeBlock =>
c.blockInputs.collect {
case e: ExprValue => e
}.toSet
case _ => Set.empty
}
def currentCodegenInputs(ctx: CodegenContext): Set[ExprValue] = {
// Collects current variables in ctx.currentVars and ctx.INPUT_ROW.
// It looks roughly like...
ctx.currentVars.flatMap { v =>
getExprValues(v.code) ++ Set(v.value, v.isNull)
}.toSet + ctx.INPUT_ROW
}
// A code block of an expression contains too long code, making it as method
if (eval.code.length > 1024) {
val setIsNull = if (!eval.isNull.isInstanceOf[LiteralValue]) {
...
} else {
""
}
// Pick up variables and statements necessary to pass in.
val currentVars = currentCodegenInputs(ctx)
val varsPassIn = getExprValues(eval.code).intersect(currentVars)
val aliasedExprs = HashMap.empty[SimpleExprValue, VariableValue]
// Replace statement-based expressions which can't be directly passed in the method.
val newCode = eval.code.transform {
case block =>
block.transformExprValues {
case s: SimpleExprValue(_, javaType) if varsPassIn.contains(s) =>
if (aliasedExprs.contains(s)) {
aliasedExprs(s)
} else {
val aliasedVariable = JavaCode.variable(ctx.freshName("aliasedVar"), javaType)
aliasedExprs += s -> aliasedVariable
varsPassIn += aliasedVariable
aliasedVariable
}
}
}
val params = varsPassIn.filter(!_.isInstanceOf[SimpleExprValue])).map { variable =>
s"${variable.javaType.getName} ${variable.variableName}"
}.mkString(", ")
val funcName = ctx.freshName("nodeName")
val javaType = CodeGenerator.javaType(dataType)
val newValue = JavaCode.variable(ctx.freshName("value"), dataType)
val funcFullName = ctx.addNewFunction(funcName,
s"""
|private $javaType $funcName($params) {
| $newCode
| $setIsNull
| return ${eval.value};
|}
""".stripMargin))
eval.value = newValue
val args = varsPassIn.filter(!_.isInstanceOf[SimpleExprValue])).map { variable =>
s"${variable.variableName}"
}
// Create a code block to assign statements to aliased variables.
val createVariables = aliasedExprs.foldLeft(EmptyBlock) { (block, (statement, variable)) =>
block + code"${statement.javaType.getName} $variable = $statement;"
}
eval.code = createVariables + code"$javaType $newValue = $funcFullName($args);"
}
```
## How was this patch tested?
Added unite tests.
Author: Liang-Chi Hsieh <viirya@gmail.com>
Closes#21405 from viirya/codeblock-api.
## What changes were proposed in this pull request?
Add an overloaded version to `from_utc_timestamp` and `to_utc_timestamp` having second argument as a `Column` instead of `String`.
## How was this patch tested?
Unit testing, especially adding two tests to org.apache.spark.sql.DateFunctionsSuite.scala
Author: Antonio Murgia <antonio.murgia@agilelab.it>
Author: Antonio Murgia <antonio.murgia2@studio.unibo.it>
Closes#21693 from tmnd1991/feature/SPARK-24673.
## What changes were proposed in this pull request?
This is a minor improvement for the test of SPARK-17213
## How was this patch tested?
N/A
Author: Xiao Li <gatorsmile@gmail.com>
Closes#21716 from gatorsmile/testMaster23.
## What changes were proposed in this pull request?
As mentioned in https://github.com/apache/spark/pull/21586 , `Cast.mayTruncate` is not 100% safe, string to boolean is allowed. Since changing `Cast.mayTruncate` also changes the behavior of Dataset, here I propose to add a new `Cast.canSafeCast` for partition pruning.
## How was this patch tested?
new test cases
Author: Wenchen Fan <wenchen@databricks.com>
Closes#21712 from cloud-fan/safeCast.
## What changes were proposed in this pull request?
The `Blocks` class in `JavaCode` class hierarchy is not necessary. Its function can be taken by `CodeBlock`. We should remove it to make simpler class hierarchy.
## How was this patch tested?
Existing tests.
Author: Liang-Chi Hsieh <viirya@gmail.com>
Closes#21619 from viirya/SPARK-24635.
## What changes were proposed in this pull request?
Since SPARK-24250 has been resolved, executors correctly references user-defined configurations. So, this pr added a static config to control cache size for generated classes in `CodeGenerator`.
## How was this patch tested?
Added tests in `ExecutorSideSQLConfSuite`.
Author: Takeshi Yamamuro <yamamuro@apache.org>
Closes#21705 from maropu/SPARK-24727.
## What changes were proposed in this pull request?
Currently we don't allow type coercion between maps.
We can support type coercion between MapTypes where both the key types and the value types are compatible.
## How was this patch tested?
Added tests.
Author: Takuya UESHIN <ueshin@databricks.com>
Closes#21703 from ueshin/issues/SPARK-24732/maptypecoercion.
## What changes were proposed in this pull request?
In the PR, I propose to add new function - *schema_of_json()* which infers schema of JSON string literal. The result of the function is a string containing a schema in DDL format.
One of the use cases is using of *schema_of_json()* in the combination with *from_json()*. Currently, _from_json()_ requires a schema as a mandatory argument. The *schema_of_json()* function will allow to point out an JSON string as an example which has the same schema as the first argument of _from_json()_. For instance:
```sql
select from_json(json_column, schema_of_json('{"c1": [0], "c2": [{"c3":0}]}'))
from json_table;
```
## How was this patch tested?
Added new test to `JsonFunctionsSuite`, `JsonExpressionsSuite` and SQL tests to `json-functions.sql`
Author: Maxim Gekk <maxim.gekk@databricks.com>
Closes#21686 from MaxGekk/infer_schema_json.
## What changes were proposed in this pull request?
Upgrade ASM to 6.1 to support JDK9+
## How was this patch tested?
Existing tests.
Author: DB Tsai <d_tsai@apple.com>
Closes#21459 from dbtsai/asm.
## What changes were proposed in this pull request?
In Dataset.join we have a small hack for resolving ambiguity in the column name for self-joins. The current code supports only `EqualTo`.
The PR extends the fix to `EqualNullSafe`.
Credit for this PR should be given to daniel-shields.
## How was this patch tested?
added UT
Author: Marco Gaido <marcogaido91@gmail.com>
Closes#21605 from mgaido91/SPARK-24385_2.
## What changes were proposed in this pull request?
Use SQLConf for PySpark to manage all sql configs, drop all the hard code in config usage.
## How was this patch tested?
Existing UT.
Author: Yuanjian Li <xyliyuanjian@gmail.com>
Closes#21648 from xuanyuanking/SPARK-24665.
## What changes were proposed in this pull request?
The ColumnPruning rule tries adding an extra Project if an input node produces fields more than needed, but as a post-processing step, it needs to remove the lower Project in the form of "Project - Filter - Project" otherwise it would conflict with PushPredicatesThroughProject and would thus cause a infinite optimization loop. The current post-processing method is defined as:
```
private def removeProjectBeforeFilter(plan: LogicalPlan): LogicalPlan = plan transform {
case p1 Project(_, f Filter(_, p2 Project(_, child)))
if p2.outputSet.subsetOf(child.outputSet) =>
p1.copy(child = f.copy(child = child))
}
```
This method works well when there is only one Filter but would not if there's two or more Filters. In this case, there is a deterministic filter and a non-deterministic filter so they stay as separate filter nodes and cannot be combined together.
An simplified illustration of the optimization process that forms the infinite loop is shown below (F1 stands for the 1st filter, F2 for the 2nd filter, P for project, S for scan of relation, PredicatePushDown as abbrev. of PushPredicatesThroughProject):
```
F1 - F2 - P - S
PredicatePushDown => F1 - P - F2 - S
ColumnPruning => F1 - P - F2 - P - S
=> F1 - P - F2 - S (Project removed)
PredicatePushDown => P - F1 - F2 - S
ColumnPruning => P - F1 - P - F2 - S
=> P - F1 - P - F2 - P - S
=> P - F1 - F2 - P - S (only one Project removed)
RemoveRedundantProject => F1 - F2 - P - S (goes back to the loop start)
```
So the problem is the ColumnPruning rule adds a Project under a Filter (and fails to remove it in the end), and that new Project triggers PushPredicateThroughProject. Once the filters have been push through the Project, a new Project will be added by the ColumnPruning rule and this goes on and on.
The fix should be when adding Projects, the rule applies top-down, but later when removing extra Projects, the process should go bottom-up to ensure all extra Projects can be matched.
## How was this patch tested?
Added a optimization rule test in ColumnPruningSuite; and a end-to-end test in SQLQuerySuite.
Author: maryannxue <maryannxue@apache.org>
Closes#21674 from maryannxue/spark-24696.
## What changes were proposed in this pull request?
Provide a continuous processing implementation of coalesce(1), as well as allowing aggregates on top of it.
The changes in ContinuousQueuedDataReader and such are to use split.index (the ID of the partition within the RDD currently being compute()d) rather than context.partitionId() (the partition ID of the scheduled task within the Spark job - that is, the post coalesce writer). In the absence of a narrow dependency, these values were previously always the same, so there was no need to distinguish.
## How was this patch tested?
new unit test
Author: Jose Torres <torres.joseph.f+github@gmail.com>
Closes#21560 from jose-torres/coalesce.
## What changes were proposed in this pull request?
A few math functions (`abs` , `bitwiseNOT`, `isnan`, `nanvl`) are not in **math_funcs** group. They should really be.
## How was this patch tested?
Awaiting Jenkins
Author: Jacek Laskowski <jacek@japila.pl>
Closes#21448 from jaceklaskowski/SPARK-24408-math-funcs-doc.
## What changes were proposed in this pull request?
Add a new test suite to test RecordBinaryComparator.
## How was this patch tested?
New test suite.
Author: Xingbo Jiang <xingbo.jiang@databricks.com>
Closes#21570 from jiangxb1987/rbc-test.
findTightestCommonTypeOfTwo has been renamed to findTightestCommonType
## What changes were proposed in this pull request?
(Please fill in changes proposed in this fix)
## How was this patch tested?
(Please explain how this patch was tested. E.g. unit tests, integration tests, manual tests)
(If this patch involves UI changes, please attach a screenshot; otherwise, remove this)
Please review http://spark.apache.org/contributing.html before opening a pull request.
Author: Fokko Driesprong <fokkodriesprong@godatadriven.com>
Closes#21597 from Fokko/fd-typo.
## What changes were proposed in this pull request?
This pr corrected the default configuration (`spark.master=local[1]`) for benchmarks. Also, this updated performance results on the AWS `r3.xlarge`.
## How was this patch tested?
N/A
Author: Takeshi Yamamuro <yamamuro@apache.org>
Closes#21625 from maropu/FixDataSourceReadBenchmark.
## What changes were proposed in this pull request?
In the master, when `csvColumnPruning`(implemented in [this commit](64fad0b519 (diff-d19881aceddcaa5c60620fdcda99b4c4))) enabled and partitions scanned only, it throws an exception below;
```
scala> val dir = "/tmp/spark-csv/csv"
scala> spark.range(10).selectExpr("id % 2 AS p", "id").write.mode("overwrite").partitionBy("p").csv(dir)
scala> spark.read.csv(dir).selectExpr("sum(p)").collect()
18/06/25 13:12:51 ERROR Executor: Exception in task 0.0 in stage 2.0 (TID 5)
java.lang.NullPointerException
at org.apache.spark.sql.execution.datasources.csv.UnivocityParser.org$apache$spark$sql$execution$datasources$csv$UnivocityParser$$convert(UnivocityParser.scala:197)
at org.apache.spark.sql.execution.datasources.csv.UnivocityParser.parse(UnivocityParser.scala:190)
at org.apache.spark.sql.execution.datasources.csv.UnivocityParser$$anonfun$5.apply(UnivocityParser.scala:309)
at org.apache.spark.sql.execution.datasources.csv.UnivocityParser$$anonfun$5.apply(UnivocityParser.scala:309)
at org.apache.spark.sql.execution.datasources.FailureSafeParser.parse(FailureSafeParser.scala:61)
...
```
This pr modified code to skip CSV parsing in the case.
## How was this patch tested?
Added tests in `CSVSuite`.
Author: Takeshi Yamamuro <yamamuro@apache.org>
Closes#21631 from maropu/SPARK-24645.
## What changes were proposed in this pull request?
Updated URL/href links to include a '/' before '?id' to make links consistent and avoid http 302 redirect errors within UI port 4040 tabs.
## How was this patch tested?
Built a runnable distribution and executed jobs. Validated that http 302 redirects are no longer encountered when clicking on links within UI port 4040 tabs.
Author: Steven Kallman <SJKallmangmail.com>
Author: Kallman, Steven <Steven.Kallman@CapitalOne.com>
Closes#21600 from SJKallman/{Spark-24553}{WEB-UI}-redirect-href-fixes.
## What changes were proposed in this pull request?
This pr added code to verify a schema in Json/Orc/ParquetFileFormat along with CSVFileFormat.
## How was this patch tested?
Added verification tests in `FileBasedDataSourceSuite` and `HiveOrcSourceSuite`.
Author: Takeshi Yamamuro <yamamuro@apache.org>
Closes#21389 from maropu/SPARK-24204.
## What changes were proposed in this pull request?
Set createTime for every hive partition created in Spark SQL, which could be used to manage data lifecycle in Hive warehouse. We found that almost every partition modified by spark sql has not been set createTime.
```
mysql> select * from partitions where create_time=0 limit 1\G;
*************************** 1. row ***************************
PART_ID: 1028584
CREATE_TIME: 0
LAST_ACCESS_TIME: 1502203611
PART_NAME: date=20170130
SD_ID: 1543605
TBL_ID: 211605
LINK_TARGET_ID: NULL
1 row in set (0.27 sec)
```
## How was this patch tested?
N/A
Author: debugger87 <yangchaozhong.2009@gmail.com>
Author: Chaozhong Yang <yangchaozhong.2009@gmail.com>
Closes#18900 from debugger87/fix/set-create-time-for-hive-partition.
## What changes were proposed in this pull request?
Address comments in #21370 and add more test.
## How was this patch tested?
Enhance test in pyspark/sql/test.py and DataFrameSuite
Author: Yuanjian Li <xyliyuanjian@gmail.com>
Closes#21553 from xuanyuanking/SPARK-24215-follow.
## What changes were proposed in this pull request?
This pr is a follow-up pr of #21155.
The #21155 removed unnecessary import at that time, but the import became necessary in another pr.
## How was this patch tested?
Existing tests.
Author: Takuya UESHIN <ueshin@databricks.com>
Closes#21646 from ueshin/issues/SPARK-23927/fup1.
## What changes were proposed in this pull request?
The PR adds the SQL function ```sequence```.
https://issues.apache.org/jira/browse/SPARK-23927
The behavior of the function is based on Presto's one.
Ref: https://prestodb.io/docs/current/functions/array.html
- ```sequence(start, stop) → array<bigint>```
Generate a sequence of integers from ```start``` to ```stop```, incrementing by ```1``` if ```start``` is less than or equal to ```stop```, otherwise ```-1```.
- ```sequence(start, stop, step) → array<bigint>```
Generate a sequence of integers from ```start``` to ```stop```, incrementing by ```step```.
- ```sequence(start_date, stop_date) → array<date>```
Generate a sequence of dates from ```start_date``` to ```stop_date```, incrementing by ```interval 1 day``` if ```start_date``` is less than or equal to ```stop_date```, otherwise ```- interval 1 day```.
- ```sequence(start_date, stop_date, step_interval) → array<date>```
Generate a sequence of dates from ```start_date``` to ```stop_date```, incrementing by ```step_interval```. The type of ```step_interval``` is ```CalendarInterval```.
- ```sequence(start_timestemp, stop_timestemp) → array<timestamp>```
Generate a sequence of timestamps from ```start_timestamps``` to ```stop_timestamps```, incrementing by ```interval 1 day``` if ```start_date``` is less than or equal to ```stop_date```, otherwise ```- interval 1 day```.
- ```sequence(start_timestamp, stop_timestamp, step_interval) → array<timestamp>```
Generate a sequence of timestamps from ```start_timestamps``` to ```stop_timestamps```, incrementing by ```step_interval```. The type of ```step_interval``` is ```CalendarInterval```.
## How was this patch tested?
Added unit tests.
Author: Vayda, Oleksandr: IT (PRG) <Oleksandr.Vayda@barclayscapital.com>
Closes#21155 from wajda/feature/array-api-sequence.
## What changes were proposed in this pull request?
In PR, I propose new behavior of `size(null)` under the config flag `spark.sql.legacy.sizeOfNull`. If the former one is disabled, the `size()` function returns `null` for `null` input. By default the `spark.sql.legacy.sizeOfNull` is enabled to keep backward compatibility with previous versions. In that case, `size(null)` returns `-1`.
## How was this patch tested?
Modified existing tests for the `size()` function to check new behavior (`null`) and old one (`-1`).
Author: Maxim Gekk <maxim.gekk@databricks.com>
Closes#21598 from MaxGekk/legacy-size-of-null.
## What changes were proposed in this pull request?
Fix `GenericArrayData.equals`, so that it respects the actual types of the elements.
e.g. an instance that represents an `array<int>` and another instance that represents an `array<long>` should be considered incompatible, and thus should return false for `equals`.
`GenericArrayData` doesn't keep any schema information by itself, and rather relies on the Java objects referenced by its `array` field's elements to keep track of their own object types. So, the most straightforward way to respect their types is to call `equals` on the elements, instead of using Scala's `==` operator, which can have semantics that are not always desirable:
```
new java.lang.Integer(123) == new java.lang.Long(123L) // true in Scala
new java.lang.Integer(123).equals(new java.lang.Long(123L)) // false in Scala
```
## How was this patch tested?
Added unit test in `ComplexDataSuite`
Author: Kris Mok <kris.mok@databricks.com>
Closes#21643 from rednaxelafx/fix-genericarraydata-equals.
## What changes were proposed in this pull request?
Here is the description in the JIRA -
Currently, our JDBC connector provides the option `dbtable` for users to specify the to-be-loaded JDBC source table.
```SQL
val jdbcDf = spark.read
.format("jdbc")
.option("dbtable", "dbName.tableName")
.options(jdbcCredentials: Map)
.load()
```
Normally, users do not fetch the whole JDBC table due to the poor performance/throughput of JDBC. Thus, they normally just fetch a small set of tables. For advanced users, they can pass a subquery as the option.
```SQL
val query = """ (select * from tableName limit 10) as tmp """
val jdbcDf = spark.read
.format("jdbc")
.option("dbtable", query)
.options(jdbcCredentials: Map)
.load()
```
However, this is straightforward to end users. We should simply allow users to specify the query by a new option `query`. We will handle the complexity for them.
```SQL
val query = """select * from tableName limit 10"""
val jdbcDf = spark.read
.format("jdbc")
.option("query", query)
.options(jdbcCredentials: Map)
.load()
```
## How was this patch tested?
Added tests in JDBCSuite and JDBCWriterSuite.
Also tested against MySQL, Postgress, Oracle, DB2 (using docker infrastructure) to make sure there are no syntax issues.
Author: Dilip Biswal <dbiswal@us.ibm.com>
Closes#21590 from dilipbiswal/SPARK-24423.
## What changes were proposed in this pull request?
Issue antlr/antlr4#781 has already been fixed, so the workaround of extracting the pattern into a separate rule is no longer needed. The presto already removed it: https://github.com/prestodb/presto/pull/10744.
## How was this patch tested?
Existing tests
Author: Yuming Wang <yumwang@ebay.com>
Closes#21641 from wangyum/ANTLR-780.
## What changes were proposed in this pull request?
Presto's implementation accepts arbitrary arrays of primitive types as an input:
```
presto> SELECT array_join(ARRAY [1, 2, 3], ', ');
_col0
---------
1, 2, 3
(1 row)
```
This PR proposes to implement a type coercion rule for ```array_join``` function that converts arrays of primitive as well as non-primitive types to arrays of string.
## How was this patch tested?
New test cases add into:
- sql-tests/inputs/typeCoercion/native/arrayJoin.sql
- DataFrameFunctionsSuite.scala
Author: Marek Novotny <mn.mikke@gmail.com>
Closes#21620 from mn-mikke/SPARK-24636.
## What changes were proposed in this pull request?
Followup to the discussion of the added conf in SPARK-24324 which allows assignment by column position only. This conf is to preserve old behavior and will be removed in future releases, so it should have a note to indicate that.
## How was this patch tested?
NA
Author: Bryan Cutler <cutlerb@gmail.com>
Closes#21637 from BryanCutler/arrow-groupedMap-conf-deprecate-followup-SPARK-24324.
This passes the unique task attempt id instead of attempt number to v2 data sources because attempt number is reused when stages are retried. When attempt numbers are reused, sources that track data by partition id and attempt number may incorrectly clean up data because the same attempt number can be both committed and aborted.
For v1 / Hadoop writes, generate a unique ID based on available attempt numbers to avoid a similar problem.
Closes#21558
Author: Marcelo Vanzin <vanzin@cloudera.com>
Author: Ryan Blue <blue@apache.org>
Closes#21606 from vanzin/SPARK-24552.2.
Use LongAdder to make SQLMetrics thread safe.
## What changes were proposed in this pull request?
Replace += with LongAdder.add() for concurrent counting
## How was this patch tested?
Unit tests with local threads
Author: Stacy Kerkela <stacy.kerkela@databricks.com>
Closes#21634 from dbkerkela/sqlmetrics-concurrency-stacy.
## What changes were proposed in this pull request?
In function array_zip, when split is required by the high number of arguments, a codegen error can happen.
The PR fixes codegen for cases when splitting the code is required.
## How was this patch tested?
added UT
Author: Marco Gaido <marcogaido91@gmail.com>
Closes#21621 from mgaido91/SPARK-24633.
## What changes were proposed in this pull request?
1. Add parameter 'cascade' in CacheManager.uncacheQuery(). Under 'cascade=false' mode, only invalidate the current cache, and for other dependent caches, rebuild execution plan and reuse cached buffer.
2. Pass true/false from callers in different uncache scenarios:
- Drop tables and regular (persistent) views: regular mode
- Drop temporary views: non-cascading mode
- Modify table contents (INSERT/UPDATE/MERGE/DELETE): regular mode
- Call `DataSet.unpersist()`: non-cascading mode
- Call `Catalog.uncacheTable()`: follow the same convention as drop tables/view, which is, use non-cascading mode for temporary views and regular mode for the rest
Note that a regular (persistent) view is a database object just like a table, so after dropping a regular view (whether cached or not cached), any query referring to that view should no long be valid. Hence if a cached persistent view is dropped, we need to invalidate the all dependent caches so that exceptions will be thrown for any later reference. On the other hand, a temporary view is in fact equivalent to an unnamed DataSet, and dropping a temporary view should have no impact on queries referencing that view. Thus we should do non-cascading uncaching for temporary views, which also guarantees a consistent uncaching behavior between temporary views and unnamed DataSets.
## How was this patch tested?
New tests in CachedTableSuite and DatasetCacheSuite.
Author: Maryann Xue <maryannxue@apache.org>
Closes#21594 from maryannxue/noncascading-cache.
## What changes were proposed in this pull request?
This is a follow-up pr of #21045 which added `arrays_zip`.
The `arrays_zip` in functions.scala should've been `scala.annotation.varargs`.
This pr makes it `scala.annotation.varargs`.
## How was this patch tested?
Existing tests.
Author: Takuya UESHIN <ueshin@databricks.com>
Closes#21630 from ueshin/issues/SPARK-23931/fup1.
## What changes were proposed in this pull request?
This pr modified JDBC datasource code to verify and normalize a partition column based on the JDBC resolved schema before building `JDBCRelation`.
Closes#20370
## How was this patch tested?
Added tests in `JDBCSuite`.
Author: Takeshi Yamamuro <yamamuro@apache.org>
Closes#21379 from maropu/SPARK-24327.
## What changes were proposed in this pull request?
Currently, a `pandas_udf` of type `PandasUDFType.GROUPED_MAP` will assign the resulting columns based on index of the return pandas.DataFrame. If a new DataFrame is returned and constructed using a dict, then the order of the columns could be arbitrary and be different than the defined schema for the UDF. If the schema types still match, then no error will be raised and the user will see column names and column data mixed up.
This change will first try to assign columns using the return type field names. If a KeyError occurs, then the column index is checked if it is string based. If so, then the error is raised as it is most likely a naming mistake, else it will fallback to assign columns by position and raise a TypeError if the field types do not match.
## How was this patch tested?
Added a test that returns a new DataFrame with column order different than the schema.
Author: Bryan Cutler <cutlerb@gmail.com>
Closes#21427 from BryanCutler/arrow-grouped-map-mixesup-cols-SPARK-24324.
## What changes were proposed in this pull request?
This pr added benchmark code `FilterPushdownBenchmark` for string pushdown and updated performance results on the AWS `r3.xlarge`.
## How was this patch tested?
N/A
Author: Takeshi Yamamuro <yamamuro@apache.org>
Closes#21288 from maropu/UpdateParquetBenchmark.
## What changes were proposed in this pull request?
Currently, restrictions in JSONOptions for `encoding` and `lineSep` are the same for read and for write. For example, a requirement for `lineSep` in the code:
```
df.write.option("encoding", "UTF-32BE").json(file)
```
doesn't allow to skip `lineSep` and use its default value `\n` because it throws the exception:
```
equirement failed: The lineSep option must be specified for the UTF-32BE encoding
java.lang.IllegalArgumentException: requirement failed: The lineSep option must be specified for the UTF-32BE encoding
```
In the PR, I propose to separate JSONOptions in read and write, and make JSONOptions in write less restrictive.
## How was this patch tested?
Added new test for blacklisted encodings in read. And the `lineSep` option was removed in write for some tests.
Author: Maxim Gekk <maxim.gekk@databricks.com>
Author: Maxim Gekk <max.gekk@gmail.com>
Closes#21247 from MaxGekk/json-options-in-write.
## What changes were proposed in this pull request?
In https://github.com/apache/spark/pull/19080 we simplified the distribution/partitioning framework, and make all the join-like operators require `HashClusteredDistribution` from children. Unfortunately streaming join operator was missed.
This can cause wrong result. Think about
```
val input1 = MemoryStream[Int]
val input2 = MemoryStream[Int]
val df1 = input1.toDF.select('value as 'a, 'value * 2 as 'b)
val df2 = input2.toDF.select('value as 'a, 'value * 2 as 'b).repartition('b)
val joined = df1.join(df2, Seq("a", "b")).select('a)
```
The physical plan is
```
*(3) Project [a#5]
+- StreamingSymmetricHashJoin [a#5, b#6], [a#10, b#11], Inner, condition = [ leftOnly = null, rightOnly = null, both = null, full = null ], state info [ checkpoint = <unknown>, runId = 54e31fce-f055-4686-b75d-fcd2b076f8d8, opId = 0, ver = 0, numPartitions = 5], 0, state cleanup [ left = null, right = null ]
:- Exchange hashpartitioning(a#5, b#6, 5)
: +- *(1) Project [value#1 AS a#5, (value#1 * 2) AS b#6]
: +- StreamingRelation MemoryStream[value#1], [value#1]
+- Exchange hashpartitioning(b#11, 5)
+- *(2) Project [value#3 AS a#10, (value#3 * 2) AS b#11]
+- StreamingRelation MemoryStream[value#3], [value#3]
```
The left table is hash partitioned by `a, b`, while the right table is hash partitioned by `b`. This means, we may have a matching record that is in different partitions, which should be in the output but not.
## How was this patch tested?
N/A
Author: Wenchen Fan <wenchen@databricks.com>
Closes#21587 from cloud-fan/join.
## What changes were proposed in this pull request?
Wrap the logical plan with a `AnalysisBarrier` for execution plan compilation in CacheManager, in order to avoid the plan being analyzed again.
## How was this patch tested?
Add one test in `DatasetCacheSuite`
Author: Maryann Xue <maryannxue@apache.org>
Closes#21602 from maryannxue/cache-mismatch.
When an output stage is retried, it's possible that tasks from the previous
attempt are still running. In that case, there would be a new task for the
same partition in the new attempt, and the coordinator would allow both
tasks to commit their output since it did not keep track of stage attempts.
The change adds more information to the stage state tracked by the coordinator,
so that only one task is allowed to commit the output in the above case.
The stage state in the coordinator is also maintained across stage retries,
so that a stray speculative task from a previous stage attempt is not allowed
to commit.
This also removes some code added in SPARK-18113 that allowed for duplicate
commit requests; with the RPC code used in Spark 2, that situation cannot
happen, so there is no need to handle it.
Author: Marcelo Vanzin <vanzin@cloudera.com>
Closes#21577 from vanzin/SPARK-24552.
## What changes were proposed in this pull request?
For the function ```def array_contains(column: Column, value: Any): Column ``` , if we pass the `value` parameter as a Column type, it will yield a runtime exception.
This PR proposes a pattern matching to detect if `value` is of type Column. If yes, it will use the .expr of the column, otherwise it will work as it used to.
Same thing for ```array_position, array_remove and element_at``` functions
## How was this patch tested?
Unit test modified to cover this code change.
Ping ueshin
Author: Chongguang LIU <chong@Chongguangs-MacBook-Pro.local>
Closes#21581 from chongguang/SPARK-24574.
## What changes were proposed in this pull request?
In the PR, I propose to automatically convert a `Literal` with `Char` type to a `Literal` of `String` type. Currently, the following code:
```scala
val df = Seq("Amsterdam", "San Francisco", "London").toDF("city")
df.where($"city".contains('o')).show(false)
```
fails with the exception:
```
Unsupported literal type class java.lang.Character o
java.lang.RuntimeException: Unsupported literal type class java.lang.Character o
at org.apache.spark.sql.catalyst.expressions.Literal$.apply(literals.scala:78)
```
The PR fixes this issue by converting `char` to `string` of length `1`. I believe it makes sense to does not differentiate `char` and `string(1)` in _a unified, multi-language data platform_ like Spark which supports languages like Python/R.
Author: Maxim Gekk <maxim.gekk@databricks.com>
Author: Maxim Gekk <max.gekk@gmail.com>
Closes#21578 from MaxGekk/support-char-literals.
## What changes were proposed in this pull request?
Add array_distinct to remove duplicate value from the array.
## How was this patch tested?
Add unit tests
Author: Huaxin Gao <huaxing@us.ibm.com>
Closes#21050 from huaxingao/spark-23912.
## What changes were proposed in this pull request?
As discussed [before](https://github.com/apache/spark/pull/19193#issuecomment-393726964), this PR prohibits window expressions inside WHERE and HAVING clauses.
## How was this patch tested?
This PR comes with a dedicated unit test.
Author: aokolnychyi <anton.okolnychyi@sap.com>
Closes#21580 from aokolnychyi/spark-24575.
## What changes were proposed in this pull request?
This patch is removing invalid comment from SparkStrategies, given that TODO-like comment is no longer preferred one as the comment: https://github.com/apache/spark/pull/21388#issuecomment-396856235
Removing invalid comment will prevent contributors to spend their times which is not going to be merged.
## How was this patch tested?
N/A
Author: Jungtaek Lim <kabhwan@gmail.com>
Closes#21595 from HeartSaVioR/MINOR-remove-invalid-comment-on-spark-strategies.
## What changes were proposed in this pull request?
Change insert input schema type: "insertRelationType" -> "insertRelationType.asNullable", in order to avoid nullable being overridden.
## How was this patch tested?
Added one test in InsertSuite.
Author: Maryann Xue <maryannxue@apache.org>
Closes#21585 from maryannxue/spark-24583.
## What changes were proposed in this pull request?
Currently, the micro-batches in the MicroBatchExecution is not exposed to the user through any public API. This was because we did not want to expose the micro-batches, so that all the APIs we expose, we can eventually support them in the Continuous engine. But now that we have better sense of buiding a ContinuousExecution, I am considering adding APIs which will run only the MicroBatchExecution. I have quite a few use cases where exposing the microbatch output as a dataframe is useful.
- Pass the output rows of each batch to a library that is designed only the batch jobs (example, uses many ML libraries need to collect() while learning).
- Reuse batch data sources for output whose streaming version does not exists (e.g. redshift data source).
- Writer the output rows to multiple places by writing twice for each batch. This is not the most elegant thing to do for multiple-output streaming queries but is likely to be better than running two streaming queries processing the same data twice.
The proposal is to add a method `foreachBatch(f: Dataset[T] => Unit)` to Scala/Java/Python `DataStreamWriter`.
## How was this patch tested?
New unit tests.
Author: Tathagata Das <tathagata.das1565@gmail.com>
Closes#21571 from tdas/foreachBatch.
## What changes were proposed in this pull request?
Currently, ReusedExchange and InMemoryTableScanExec only rewrite output partitioning if child's partitioning is HashPartitioning and do nothing for other partitioning, e.g., RangePartitioning. We should always rewrite it, otherwise, unnecessary shuffle could be introduced like https://issues.apache.org/jira/browse/SPARK-24556.
## How was this patch tested?
Add new tests.
Author: yucai <yyu1@ebay.com>
Closes#21564 from yucai/SPARK-24556.
## What changes were proposed in this pull request?
test("withColumn doesn't invalidate cached dataframe") in CachedTableSuite doesn't not work because:
The UDF is executed and test count incremented when "df.cache()" is called and the subsequent "df.collect()" has no effect on the test result.
This PR fixed this test and add another test for caching UDF.
## How was this patch tested?
Add new tests.
Author: Li Jin <ice.xelloss@gmail.com>
Closes#21531 from icexelloss/fix-cache-test.
## What changes were proposed in this pull request?
UDF series UDFXPathXXXX allow users to pass carefully crafted XML to access arbitrary files. Spark does not have built-in access control. When users use the external access control library, users might bypass them and access the file contents.
This PR basically patches the Hive fix to Apache Spark. https://issues.apache.org/jira/browse/HIVE-18879
## How was this patch tested?
A unit test case
Author: Xiao Li <gatorsmile@gmail.com>
Closes#21549 from gatorsmile/xpathSecurity.
## What changes were proposed in this pull request?
This is a followup of https://github.com/apache/spark/pull/21503, to completely move operator pushdown to the planner rule.
The code are mostly from https://github.com/apache/spark/pull/21319
## How was this patch tested?
existing tests
Author: Wenchen Fan <wenchen@databricks.com>
Closes#21574 from cloud-fan/followup.
## What changes were proposed in this pull request?
When creating tuple expression encoders, we should give the serializer expressions of tuple items correct names, so we can have correct output schema when we use such tuple encoders.
## How was this patch tested?
Added test.
Author: Liang-Chi Hsieh <viirya@gmail.com>
Closes#21576 from viirya/SPARK-24548.
## What changes were proposed in this pull request?
This pr added a new JSON option `dropFieldIfAllNull ` to ignore column of all null values or empty array/struct during JSON schema inference.
## How was this patch tested?
Added tests in `JsonSuite`.
Author: Takeshi Yamamuro <yamamuro@apache.org>
Author: Xiangrui Meng <meng@databricks.com>
Closes#20929 from maropu/SPARK-23772.
## What changes were proposed in this pull request?
The supported java.math.BigInteger type is not mentioned in the javadoc of Encoders.bean()
## How was this patch tested?
only Javadoc fix
Please review http://spark.apache.org/contributing.html before opening a pull request.
Author: James Yu <james@ispot.tv>
Closes#21544 from yuj/master.
## What changes were proposed in this pull request?
Provide an option to limit number of rows in a MemorySink. Currently, MemorySink and MemorySinkV2 have unbounded size, meaning that if they're used on big data, they can OOM the stream. This change adds a maxMemorySinkRows option to limit how many rows MemorySink and MemorySinkV2 can hold. By default, they are still unbounded.
## How was this patch tested?
Added new unit tests.
Author: Mukul Murthy <mukul.murthy@databricks.com>
Closes#21559 from mukulmurthy/SPARK-24525.
## What changes were proposed in this pull request?
This PR fixes possible overflow in int add or multiply. In particular, their overflows in multiply are detected by [Spotbugs](https://spotbugs.github.io/)
The following assignments may cause overflow in right hand side. As a result, the result may be negative.
```
long = int * int
long = int + int
```
To avoid this problem, this PR performs cast from int to long in right hand side.
## How was this patch tested?
Existing UTs.
Author: Kazuaki Ishizaki <ishizaki@jp.ibm.com>
Closes#21481 from kiszk/SPARK-24452.
## What changes were proposed in this pull request?
This PR adds `foreach` for streaming queries in Python. Users will be able to specify their processing logic in two different ways.
- As a function that takes a row as input.
- As an object that has methods `open`, `process`, and `close` methods.
See the python docs in this PR for more details.
## How was this patch tested?
Added java and python unit tests
Author: Tathagata Das <tathagata.das1565@gmail.com>
Closes#21477 from tdas/SPARK-24396.
## What changes were proposed in this pull request?
This removes the v2 optimizer rule for push-down and instead pushes filters and required columns when converting to a physical plan, as suggested by marmbrus. This makes the v2 relation cleaner because the output and filters do not change in the logical plan.
A side-effect of this change is that the stats from the logical (optimized) plan no longer reflect pushed filters and projection. This is a temporary state, until the planner gathers stats from the physical plan instead. An alternative to this approach is 9d3a11e68b.
The first commit was proposed in #21262. This PR replaces #21262.
## How was this patch tested?
Existing tests.
Author: Ryan Blue <blue@apache.org>
Closes#21503 from rdblue/SPARK-24478-move-push-down-to-physical-conversion.
## What changes were proposed in this pull request?
In the PR, I propose to support any DataType represented as DDL string for the from_json function. After the changes, it will be possible to specify `MapType` in SQL like:
```sql
select from_json('{"a":1, "b":2}', 'map<string, int>')
```
and in Scala (similar in other languages)
```scala
val in = Seq("""{"a": {"b": 1}}""").toDS()
val schema = "map<string, map<string, int>>"
val out = in.select(from_json($"value", schema, Map.empty[String, String]))
```
## How was this patch tested?
Added a couple sql tests and modified existing tests for Python and Scala. The former tests were modified because it is not imported for them in which format schema for `from_json` is provided.
Author: Maxim Gekk <maxim.gekk@databricks.com>
Closes#21550 from MaxGekk/from_json-ddl-schema.
## What changes were proposed in this pull request?
`EnsureRequirement` in its `reorder` method currently assumes that the same key appears only once in the join condition. This of course might not be the case, and when it is not satisfied, it returns a wrong plan which produces a wrong result of the query.
## How was this patch tested?
added UT
Author: Marco Gaido <marcogaido91@gmail.com>
Closes#21529 from mgaido91/SPARK-24495.
## What changes were proposed in this pull request?
The PR updates the 2.3 version tested to the new release 2.3.1.
## How was this patch tested?
existing UTs
Author: Marco Gaido <marcogaido91@gmail.com>
Closes#21543 from mgaido91/patch-1.
## What changes were proposed in this pull request?
https://docs.google.com/document/d/1IL4kJoKrZWeyIhklKUJqsW-yEN7V7aL05MmM65AYOfE/edit
Implement continuous shuffle write RDD for a single reader partition. (I don't believe any implementation changes are actually required for multiple reader partitions, but this PR is already very large, so I want to exclude those for now to keep the size down.)
## How was this patch tested?
new unit tests
Author: Jose Torres <torres.joseph.f+github@gmail.com>
Closes#21428 from jose-torres/writerTask.
## What changes were proposed in this pull request?
If you construct catalyst trees using `scala.collection.immutable.Stream` you can run into situations where valid transformations do not seem to have any effect. There are two causes for this behavior:
- `Stream` is evaluated lazily. Note that default implementation will generally only evaluate a function for the first element (this makes testing a bit tricky).
- `TreeNode` and `QueryPlan` use side effects to detect if a tree has changed. Mapping over a stream is lazy and does not need to trigger this side effect. If this happens the node will invalidly assume that it did not change and return itself instead if the newly created node (this is for GC reasons).
This PR fixes this issue by forcing materialization on streams in `TreeNode` and `QueryPlan`.
## How was this patch tested?
Unit tests were added to `TreeNodeSuite` and `LogicalPlanSuite`. An integration test was added to the `PlannerSuite`
Author: Herman van Hovell <hvanhovell@databricks.com>
Closes#21539 from hvanhovell/SPARK-24500.
## What changes were proposed in this pull request?
Currently a "StreamingQueryListener" can only be registered programatically. We could have a new config "spark.sql.streamingQueryListeners" similar to "spark.sql.queryExecutionListeners" and "spark.extraListeners" for users to register custom streaming listeners.
## How was this patch tested?
New unit test and running example programs.
Please review http://spark.apache.org/contributing.html before opening a pull request.
Author: Arun Mahadevan <arunm@apache.org>
Closes#21504 from arunmahadevan/SPARK-24480.
## What changes were proposed in this pull request?
This patch measures and logs elapsed time for each operation which communicate with file system (mostly remote HDFS in production) in HDFSBackedStateStoreProvider to help investigating any latency issue.
## How was this patch tested?
Manually tested.
Author: Jungtaek Lim <kabhwan@gmail.com>
Closes#21506 from HeartSaVioR/SPARK-24485.
## What changes were proposed in this pull request?
TextSocketMicroBatchReader was no longer be compatible with netcat due to launching temporary reader for reading schema, and closing reader, and re-opening reader. While reliable socket server should be able to handle this without any issue, nc command normally can't handle multiple connections and simply exits when closing temporary reader.
This patch fixes TextSocketMicroBatchReader to be compatible with netcat again, via deferring opening socket to the first call of planInputPartitions() instead of constructor.
## How was this patch tested?
Added unit test which fails on current and succeeds with the patch. And also manually tested.
Author: Jungtaek Lim <kabhwan@gmail.com>
Closes#21497 from HeartSaVioR/SPARK-24466.
## What changes were proposed in this pull request?
This PR enables using a grouped aggregate pandas UDFs as window functions. The semantics is the same as using SQL aggregation function as window functions.
```
>>> from pyspark.sql.functions import pandas_udf, PandasUDFType
>>> from pyspark.sql import Window
>>> df = spark.createDataFrame(
... [(1, 1.0), (1, 2.0), (2, 3.0), (2, 5.0), (2, 10.0)],
... ("id", "v"))
>>> pandas_udf("double", PandasUDFType.GROUPED_AGG)
... def mean_udf(v):
... return v.mean()
>>> w = Window.partitionBy('id')
>>> df.withColumn('mean_v', mean_udf(df['v']).over(w)).show()
+---+----+------+
| id| v|mean_v|
+---+----+------+
| 1| 1.0| 1.5|
| 1| 2.0| 1.5|
| 2| 3.0| 6.0|
| 2| 5.0| 6.0|
| 2|10.0| 6.0|
+---+----+------+
```
The scope of this PR is somewhat limited in terms of:
(1) Only supports unbounded window, which acts essentially as group by.
(2) Only supports aggregation functions, not "transform" like window functions (n -> n mapping)
Both of these are left as future work. Especially, (1) needs careful thinking w.r.t. how to pass rolling window data to python efficiently. (2) is a bit easier but does require more changes therefore I think it's better to leave it as a separate PR.
## How was this patch tested?
WindowPandasUDFTests
Author: Li Jin <ice.xelloss@gmail.com>
Closes#21082 from icexelloss/SPARK-22239-window-udf.
## What changes were proposed in this pull request?
The PR adds the SQL function `map_from_arrays`. The behavior of the function is based on Presto's `map`. Since SparkSQL already had a `map` function, we prepared the different name for this behavior.
This function returns returns a map from a pair of arrays for keys and values.
## How was this patch tested?
Added UTs
Author: Kazuaki Ishizaki <ishizaki@jp.ibm.com>
Closes#21258 from kiszk/SPARK-23933.
## What changes were proposed in this pull request?
When user create a aggregator object in scala and pass the aggregator to Spark Dataset's agg() method, Spark's will initialize TypedAggregateExpression with the nodeName field as aggregator.getClass.getSimpleName. However, getSimpleName is not safe in scala environment, depending on how user creates the aggregator object. For example, if the aggregator class full qualified name is "com.my.company.MyUtils$myAgg$2$", the getSimpleName will throw java.lang.InternalError "Malformed class name". This has been reported in scalatest https://github.com/scalatest/scalatest/pull/1044 and discussed in many scala upstream jiras such as SI-8110, SI-5425.
To fix this issue, we follow the solution in https://github.com/scalatest/scalatest/pull/1044 to add safer version of getSimpleName as a util method, and TypedAggregateExpression will invoke this util method rather than getClass.getSimpleName.
## How was this patch tested?
added unit test
Author: Fangshi Li <fli@linkedin.com>
Closes#21276 from fangshil/SPARK-24216.
Signed-off-by: DylanGuedes <djmgguedesgmail.com>
## What changes were proposed in this pull request?
Addition of arrays_zip function to spark sql functions.
## How was this patch tested?
(Please explain how this patch was tested. E.g. unit tests, integration tests, manual tests)
Unit tests that checks if the results are correct.
Author: DylanGuedes <djmgguedes@gmail.com>
Closes#21045 from DylanGuedes/SPARK-23931.
## What changes were proposed in this pull request?
Removing version 2.2.0 from testing versions in HiveExternalCatalogVersionsSuite as it is not present anymore in the mirrors and this is blocking all the open PRs.
## How was this patch tested?
running UTs
Author: Marco Gaido <marcogaido91@gmail.com>
Closes#21540 from mgaido91/SPARK-24531.
no => no[t]
## What changes were proposed in this pull request?
Fixing a typo.
## How was this patch tested?
Visual check of the docs.
Please review http://spark.apache.org/contributing.html before opening a pull request.
Author: Tom Saleeba <tom.saleeba@gmail.com>
Closes#21496 from tomsaleeba/patch-1.
## What changes were proposed in this pull request?
`UnsafeRowSerializerSuite` calls `UnsafeProjection.create` which accesses `SQLConf.get`, while the current active SparkSession may already be stopped, and we may hit exception like this
```
sbt.ForkMain$ForkError: java.lang.IllegalStateException: LiveListenerBus is stopped.
at org.apache.spark.scheduler.LiveListenerBus.addToQueue(LiveListenerBus.scala:97)
at org.apache.spark.scheduler.LiveListenerBus.addToStatusQueue(LiveListenerBus.scala:80)
at org.apache.spark.sql.internal.SharedState.<init>(SharedState.scala:93)
at org.apache.spark.sql.SparkSession$$anonfun$sharedState$1.apply(SparkSession.scala:120)
at org.apache.spark.sql.SparkSession$$anonfun$sharedState$1.apply(SparkSession.scala:120)
at scala.Option.getOrElse(Option.scala:121)
at org.apache.spark.sql.SparkSession.sharedState$lzycompute(SparkSession.scala:120)
at org.apache.spark.sql.SparkSession.sharedState(SparkSession.scala:119)
at org.apache.spark.sql.internal.BaseSessionStateBuilder.build(BaseSessionStateBuilder.scala:286)
at org.apache.spark.sql.test.TestSparkSession.sessionState$lzycompute(TestSQLContext.scala:42)
at org.apache.spark.sql.test.TestSparkSession.sessionState(TestSQLContext.scala:41)
at org.apache.spark.sql.SparkSession$$anonfun$1$$anonfun$apply$1.apply(SparkSession.scala:95)
at org.apache.spark.sql.SparkSession$$anonfun$1$$anonfun$apply$1.apply(SparkSession.scala:95)
at scala.Option.map(Option.scala:146)
at org.apache.spark.sql.SparkSession$$anonfun$1.apply(SparkSession.scala:95)
at org.apache.spark.sql.SparkSession$$anonfun$1.apply(SparkSession.scala:94)
at org.apache.spark.sql.internal.SQLConf$.get(SQLConf.scala:126)
at org.apache.spark.sql.catalyst.expressions.CodeGeneratorWithInterpretedFallback.createObject(CodeGeneratorWithInterpretedFallback.scala:54)
at org.apache.spark.sql.catalyst.expressions.UnsafeProjection$.create(Projection.scala:157)
at org.apache.spark.sql.catalyst.expressions.UnsafeProjection$.create(Projection.scala:150)
at org.apache.spark.sql.execution.UnsafeRowSerializerSuite.org$apache$spark$sql$execution$UnsafeRowSerializerSuite$$unsafeRowConverter(UnsafeRowSerializerSuite.scala:54)
at org.apache.spark.sql.execution.UnsafeRowSerializerSuite.org$apache$spark$sql$execution$UnsafeRowSerializerSuite$$toUnsafeRow(UnsafeRowSerializerSuite.scala:49)
at org.apache.spark.sql.execution.UnsafeRowSerializerSuite$$anonfun$2.apply(UnsafeRowSerializerSuite.scala:63)
at org.apache.spark.sql.execution.UnsafeRowSerializerSuite$$anonfun$2.apply(UnsafeRowSerializerSuite.scala:60)
...
```
## How was this patch tested?
N/A
Author: Wenchen Fan <wenchen@databricks.com>
Closes#21518 from cloud-fan/test.
## What changes were proposed in this pull request?
when the length of pre-shuffle's partitions is 0, the length of post-shuffle's partitions should be 0 instead of spark.sql.shuffle.partitions.
## How was this patch tested?
ExchangeCoordinator converted a pre-shuffle that partitions is 0 to a post-shuffle that partitions is 0 instead of one that partitions is spark.sql.shuffle.partitions.
Author: liutang123 <liutang123@yeah.net>
Closes#19364 from liutang123/SPARK-22144.
## What changes were proposed in this pull request?
In SPARK-22036 we introduced the possibility to allow precision loss in arithmetic operations (according to the SQL standard). The implementation was drawn from Hive's one, where Decimals with a negative scale are not allowed in the operations.
The PR handles the case when the scale is negative, removing the assertion that it is not.
## How was this patch tested?
added UTs
Author: Marco Gaido <marcogaido91@gmail.com>
Closes#21499 from mgaido91/SPARK-24468.
## What changes were proposed in this pull request?
Update documentation for `isInCollection` API to clealy explain the "auto-casting" of elements if their types are different.
## How was this patch tested?
No-Op
Author: Thiruvasakan Paramasivan <thiru@apple.com>
Closes#21519 from trvskn/sql-doc-update.
## What changes were proposed in this pull request?
Implemented eval in SortPrefix expression.
## How was this patch tested?
- ran existing sbt SQL tests
- added unit test
- ran existing Python SQL tests
- manual tests: disabling codegen -- patching code to disable beyond what spark.sql.codegen.wholeStage=false can do -- and running sbt SQL tests
Author: Bruce Robbins <bersprockets@gmail.com>
Closes#21231 from bersprockets/sortprefixeval.
## What changes were proposed in this pull request?
support bucket pruning when filtering on a single bucketed column on the following predicates -
EqualTo, EqualNullSafe, In, And/Or predicates
## How was this patch tested?
refactored unit tests to test the above.
based on gatorsmile work in e3c75c6398
Author: Asher Saban <asaban@palantir.com>
Author: asaban <asaban@palantir.com>
Closes#20915 from sabanas/filter-prune-buckets.
## What changes were proposed in this pull request?
Sql below will get all partitions from metastore, which put much burden on metastore;
```
CREATE TABLE `partition_test`(`col` int) PARTITIONED BY (`pt` byte)
SELECT * FROM partition_test WHERE CAST(pt AS INT)=1
```
The reason is that the the analyzed attribute `dt` is wrapped in `Cast` and `HiveShim` fails to generate a proper partition filter.
This pr proposes to take `Cast` into consideration when generate partition filter.
## How was this patch tested?
Test added.
This pr proposes to use analyzed expressions in `HiveClientSuite`
Author: jinxing <jinxing6042@126.com>
Closes#19602 from jinxing64/SPARK-22384.
## What changes were proposed in this pull request?
The error occurs when we are recovering from a failure in a no-data batch (say X) that has been planned (i.e. written to offset log) but not executed (i.e. not written to commit log). Upon recovery the following sequence of events happen.
1. `MicroBatchExecution.populateStartOffsets` sets `currentBatchId` to X. Since there was no data in the batch, the `availableOffsets` is same as `committedOffsets`, so `isNewDataAvailable` is `false`.
2. When `MicroBatchExecution.constructNextBatch` is called, ideally it should immediately return true because the next batch has already been constructed. However, the check of whether the batch has been constructed was `if (isNewDataAvailable) return true`. Since the planned batch is a no-data batch, it escaped this check and proceeded to plan the same batch X *once again*.
The solution is to have an explicit flag that signifies whether a batch has already been constructed or not. `populateStartOffsets` is going to set the flag appropriately.
## How was this patch tested?
new unit test
Author: Tathagata Das <tathagata.das1565@gmail.com>
Closes#21491 from tdas/SPARK-24453.
## What changes were proposed in this pull request?
This PR explicitly prohibits window functions inside aggregates. Currently, this will cause StackOverflow during analysis. See PR #19193 for previous discussion.
## How was this patch tested?
This PR comes with a dedicated unit test.
Author: aokolnychyi <anton.okolnychyi@sap.com>
Closes#21473 from aokolnychyi/fix-stackoverflow-window-funcs.
## What changes were proposed in this pull request?
Add support for date `extract` function:
```sql
spark-sql> SELECT EXTRACT(YEAR FROM TIMESTAMP '2000-12-16 12:21:13');
2000
```
Supported field same as [Hive](https://github.com/apache/hive/blob/rel/release-2.3.3/ql/src/java/org/apache/hadoop/hive/ql/parse/IdentifiersParser.g#L308-L316): `YEAR`, `QUARTER`, `MONTH`, `WEEK`, `DAY`, `DAYOFWEEK`, `HOUR`, `MINUTE`, `SECOND`.
## How was this patch tested?
unit tests
Author: Yuming Wang <yumwang@ebay.com>
Closes#21479 from wangyum/SPARK-23903.
## What changes were proposed in this pull request?
Currently column names of headers in CSV files are not checked against provided schema of CSV data. It could cause errors like showed in the [SPARK-23786](https://issues.apache.org/jira/browse/SPARK-23786) and https://github.com/apache/spark/pull/20894#issuecomment-375957777. I introduced new CSV option - `enforceSchema`. If it is enabled (by default `true`), Spark forcibly applies provided or inferred schema to CSV files. In that case, CSV headers are ignored and not checked against the schema. If `enforceSchema` is set to `false`, additional checks can be performed. For example, if column in CSV header and in the schema have different ordering, the following exception is thrown:
```
java.lang.IllegalArgumentException: CSV file header does not contain the expected fields
Header: depth, temperature
Schema: temperature, depth
CSV file: marina.csv
```
## How was this patch tested?
The changes were tested by existing tests of CSVSuite and by 2 new tests.
Author: Maxim Gekk <maxim.gekk@databricks.com>
Author: Maxim Gekk <max.gekk@gmail.com>
Closes#20894 from MaxGekk/check-column-names.
## What changes were proposed in this pull request?
bring back https://github.com/apache/spark/pull/21443
This is a different approach: just change the check to count distinct columns with `toSet`
## How was this patch tested?
a new test to verify the planner behavior.
Author: Wenchen Fan <wenchen@databricks.com>
Author: Takeshi Yamamuro <yamamuro@apache.org>
Closes#21487 from cloud-fan/back.
## What changes were proposed in this pull request?
Compute the thresholdBatchId to purge metadata based on current committed epoch instead of currentBatchId in CP mode to avoid cleaning all the committed metadata in some case as described in the jira [SPARK-24351](https://issues.apache.org/jira/browse/SPARK-24351).
## How was this patch tested?
Add new unit test.
Author: Huang Tengfei <tengfei.h@gmail.com>
Closes#21400 from ivoson/branch-cp-meta.
## What changes were proposed in this pull request?
add array_remove to remove all elements that equal element from array
## How was this patch tested?
add unit tests
Author: Huaxin Gao <huaxing@us.ibm.com>
Closes#21069 from huaxingao/spark-23920.
## What changes were proposed in this pull request?
1. Refactor ExecuteWriteTask in FileFormatWriter to reduce common logic and improve readability.
After the change, callers only need to call `commit()` or `abort` at the end of task.
Also there is less code in `SingleDirectoryWriteTask` and `DynamicPartitionWriteTask`.
Definitions of related classes are moved to a new file, and `ExecuteWriteTask` is renamed to `FileFormatDataWriter`.
2. As per code style guide: https://github.com/databricks/scala-style-guide#traversal-and-zipwithindex , we avoid using `for` for looping in [FileFormatWriter](https://github.com/apache/spark/pull/21381/files#diff-3b69eb0963b68c65cfe8075f8a42e850L536) , or `foreach` in [WriteToDataSourceV2Exec](https://github.com/apache/spark/pull/21381/files#diff-6fbe10db766049a395bae2e785e9d56eL119).
In such critical code path, using `while` is good for performance.
## How was this patch tested?
Existing unit test.
I tried the microbenchmark in https://github.com/apache/spark/pull/21409
| Workload | Before changes(Best/Avg Time(ms)) | After changes(Best/Avg Time(ms)) |
| --- | --- | -- |
|Output Single Int Column| 2018 / 2043 | 2096 / 2236 |
|Output Single Double Column| 1978 / 2043 | 2013 / 2018 |
|Output Int and String Column| 6332 / 6706 | 6162 / 6298 |
|Output Partitions| 4458 / 5094 | 3792 / 4008 |
|Output Buckets| 5695 / 6102 | 5120 / 5154 |
Also a microbenchmark on my laptop for general comparison among while/foreach/for :
```
class Writer {
var sum = 0L
def write(l: Long): Unit = sum += l
}
def testWhile(iterator: Iterator[Long]): Long = {
val w = new Writer
while (iterator.hasNext) {
w.write(iterator.next())
}
w.sum
}
def testForeach(iterator: Iterator[Long]): Long = {
val w = new Writer
iterator.foreach(w.write)
w.sum
}
def testFor(iterator: Iterator[Long]): Long = {
val w = new Writer
for (x <- iterator) {
w.write(x)
}
w.sum
}
val data = 0L to 100000000L
val start = System.nanoTime
(0 to 10).foreach(_ => testWhile(data.iterator))
println("benchmark while: " + (System.nanoTime - start)/1000000)
val start2 = System.nanoTime
(0 to 10).foreach(_ => testForeach(data.iterator))
println("benchmark foreach: " + (System.nanoTime - start2)/1000000)
val start3 = System.nanoTime
(0 to 10).foreach(_ => testForeach(data.iterator))
println("benchmark for: " + (System.nanoTime - start3)/1000000)
```
Benchmark result:
`while`: 15401 ms
`foreach`: 43034 ms
`for`: 41279 ms
Author: Gengliang Wang <gengliang.wang@databricks.com>
Closes#21381 from gengliangwang/refactorExecuteWriteTask.
## What changes were proposed in this pull request?
`format_number` support user specifed format as argument. For example:
```sql
spark-sql> SELECT format_number(12332.123456, '##################.###');
12332.123
```
## How was this patch tested?
unit test
Author: Yuming Wang <yumwang@ebay.com>
Closes#21010 from wangyum/SPARK-23900.
## What changes were proposed in this pull request?
When two `In` operators are created with the same list of values, but different order, we are considering them as semantically different. This is wrong, since they have the same semantic meaning.
The PR adds a canonicalization rule which orders the literals in the `In` operator so the semantic equality works properly.
## How was this patch tested?
added UT
Author: Marco Gaido <marcogaido91@gmail.com>
Closes#21331 from mgaido91/SPARK-24276.
## What changes were proposed in this pull request?
The PR adds the masking function as they are described in Hive's documentation: https://cwiki.apache.org/confluence/display/Hive/LanguageManual+UDF#LanguageManualUDF-DataMaskingFunctions.
This means that only `string`s are accepted as parameter for the masking functions.
## How was this patch tested?
added UTs
Author: Marco Gaido <marcogaido91@gmail.com>
Closes#21246 from mgaido91/SPARK-23901.
## What changes were proposed in this pull request?
This pr fixed an issue when having multiple distinct aggregations having the same argument set, e.g.,
```
scala>: paste
val df = sql(
s"""SELECT corr(DISTINCT x, y), corr(DISTINCT y, x), count(*)
| FROM (VALUES (1, 1), (2, 2), (2, 2)) t(x, y)
""".stripMargin)
java.lang.RuntimeException
You hit a query analyzer bug. Please report your query to Spark user mailing list.
```
The root cause is that `RewriteDistinctAggregates` can't detect multiple distinct aggregations if they have the same argument set. This pr modified code so that `RewriteDistinctAggregates` could count the number of aggregate expressions with `isDistinct=true`.
## How was this patch tested?
Added tests in `DataFrameAggregateSuite`.
Author: Takeshi Yamamuro <yamamuro@apache.org>
Closes#21443 from maropu/SPARK-24369.
## What changes were proposed in this pull request?
Add Data Source write benchmark. So that it would be easier to measure the writer performance.
Author: Gengliang Wang <gengliang.wang@databricks.com>
Closes#21409 from gengliangwang/parquetWriteBenchmark.
## What changes were proposed in this pull request?
Implemented **`isInCollection `** in DataFrame API for both Scala and Java, so users can do
```scala
val profileDF = Seq(
Some(1), Some(2), Some(3), Some(4),
Some(5), Some(6), Some(7), None
).toDF("profileID")
val validUsers: Seq[Any] = Seq(6, 7.toShort, 8L, "3")
val result = profileDF.withColumn("isValid", $"profileID". isInCollection(validUsers))
result.show(10)
"""
+---------+-------+
|profileID|isValid|
+---------+-------+
| 1| false|
| 2| false|
| 3| true|
| 4| false|
| 5| false|
| 6| true|
| 7| true|
| null| null|
+---------+-------+
""".stripMargin
```
## How was this patch tested?
Several unit tests are added.
Author: DB Tsai <d_tsai@apple.com>
Closes#21416 from dbtsai/optimize-set.
## What changes were proposed in this pull request?
We should not stop users from calling `getActiveSession` and `getDefaultSession` in executors. To not break the existing behaviors, we should simply return None.
## How was this patch tested?
N/A
Author: Xiao Li <gatorsmile@gmail.com>
Closes#21436 from gatorsmile/followUpSPARK-24250.
## What changes were proposed in this pull request?
`Random.nextString` is good for generating random string data, but it's not proper for directory name prefix in `Utils.createDirectory(tempDir, Random.nextString(10))`. This PR uses more safe directory namePrefix.
```scala
scala> scala.util.Random.nextString(10)
res0: String = 馨쭔ᎰႻ穚䃈兩㻞藑並
```
```scala
StateStoreRDDSuite:
- versioning and immutability
- recovering from files
- usage with iterators - only gets and only puts
- preferred locations using StateStoreCoordinator *** FAILED ***
java.io.IOException: Failed to create a temp directory (under /.../spark/sql/core/target/tmp/StateStoreRDDSuite8712796397908632676) after 10 attempts!
at org.apache.spark.util.Utils$.createDirectory(Utils.scala:295)
at org.apache.spark.sql.execution.streaming.state.StateStoreRDDSuite$$anonfun$13$$anonfun$apply$6.apply(StateStoreRDDSuite.scala:152)
at org.apache.spark.sql.execution.streaming.state.StateStoreRDDSuite$$anonfun$13$$anonfun$apply$6.apply(StateStoreRDDSuite.scala:149)
at org.apache.spark.sql.catalyst.util.package$.quietly(package.scala:42)
at org.apache.spark.sql.execution.streaming.state.StateStoreRDDSuite$$anonfun$13.apply(StateStoreRDDSuite.scala:149)
at org.apache.spark.sql.execution.streaming.state.StateStoreRDDSuite$$anonfun$13.apply(StateStoreRDDSuite.scala:149)
...
- distributed test *** FAILED ***
java.io.IOException: Failed to create a temp directory (under /.../spark/sql/core/target/tmp/StateStoreRDDSuite8712796397908632676) after 10 attempts!
at org.apache.spark.util.Utils$.createDirectory(Utils.scala:295)
```
## How was this patch tested?
Pass the existing tests.StateStoreRDDSuite:
Author: Dongjoon Hyun <dongjoon@apache.org>
Closes#21446 from dongjoon-hyun/SPARK-19613.
## What changes were proposed in this pull request?
When we create a `RelationalGroupedDataset` or a `KeyValueGroupedDataset` we set its child to the `logicalPlan` of the `DataFrame` we need to aggregate. Since the `logicalPlan` is already analyzed, we should not analyze it again. But this happens when the new plan of the aggregate is analyzed.
The current behavior in most of the cases is likely to produce no harm, but in other cases re-analyzing an analyzed plan can change it, since the analysis is not idempotent. This can cause issues like the one described in the JIRA (missing to find a cached plan).
The PR adds an `AnalysisBarrier` to the `logicalPlan` which is used as child of `RelationalGroupedDataset` or a `KeyValueGroupedDataset`.
## How was this patch tested?
added UT
Author: Marco Gaido <marcogaido91@gmail.com>
Closes#21432 from mgaido91/SPARK-24373.
## What changes were proposed in this pull request?
There is a race condition of closing Arrow VectorSchemaRoot and Allocator in the writer thread of ArrowPythonRunner.
The race results in memory leak exception when closing the allocator. This patch removes the closing routine from the TaskCompletionListener and make the writer thread responsible for cleaning up the Arrow memory.
This issue be reproduced by this test:
```
def test_memory_leak(self):
from pyspark.sql.functions import pandas_udf, col, PandasUDFType, array, lit, explode
# Have all data in a single executor thread so it can trigger the race condition easier
with self.sql_conf({'spark.sql.shuffle.partitions': 1}):
df = self.spark.range(0, 1000)
df = df.withColumn('id', array([lit(i) for i in range(0, 300)])) \
.withColumn('id', explode(col('id'))) \
.withColumn('v', array([lit(i) for i in range(0, 1000)]))
pandas_udf(df.schema, PandasUDFType.GROUPED_MAP)
def foo(pdf):
xxx
return pdf
result = df.groupby('id').apply(foo)
with QuietTest(self.sc):
with self.assertRaises(py4j.protocol.Py4JJavaError) as context:
result.count()
self.assertTrue('Memory leaked' not in str(context.exception))
```
Note: Because of the race condition, the test case cannot reproduce the issue reliably so it's not added to test cases.
## How was this patch tested?
Because of the race condition, the bug cannot be unit test easily. So far it has only happens on large amount of data. This is currently tested manually.
Author: Li Jin <ice.xelloss@gmail.com>
Closes#21397 from icexelloss/SPARK-24334-arrow-memory-leak.
## What changes were proposed in this pull request?
This PR adds several unit tests along the `cols NOT IN (subquery)` pathway. There are a scattering of tests here and there which cover this codepath, but there doesn't seem to be a unified unit test of the correctness of null-aware anti joins anywhere. I have also added a brief explanation of how this expression behaves in SubquerySuite. Lastly, I made some clarifying changes in the NOT IN pathway in RewritePredicateSubquery.
## How was this patch tested?
Added unit tests! There should be no behavioral change in this PR.
Author: Miles Yucht <miles@databricks.com>
Closes#21425 from mgyucht/spark-24381.
## What changes were proposed in this pull request?
Currently, users are getting the following error messages on type conversions:
```
scala.MatchError: test (of class java.lang.String)
```
The message doesn't give any clues to the users where in the schema the error happened. In this PR, I would like to improve the error message like:
```
The value (test) of the type (java.lang.String) cannot be converted to struct<f1:int>
```
## How was this patch tested?
Added tests for converting of wrong values to `struct`, `map`, `array`, `string` and `decimal`.
Author: Maxim Gekk <maxim.gekk@databricks.com>
Closes#21410 from MaxGekk/type-conv-error.
## What changes were proposed in this pull request?
uniVocity parser allows to specify only required column names or indexes for [parsing](https://www.univocity.com/pages/parsers-tutorial) like:
```
// Here we select only the columns by their indexes.
// The parser just skips the values in other columns
parserSettings.selectIndexes(4, 0, 1);
CsvParser parser = new CsvParser(parserSettings);
```
In this PR, I propose to extract indexes from required schema and pass them into the CSV parser. Benchmarks show the following improvements in parsing of 1000 columns:
```
Select 100 columns out of 1000: x1.76
Select 1 column out of 1000: x2
```
**Note**: Comparing to current implementation, the changes can return different result for malformed rows in the `DROPMALFORMED` and `FAILFAST` modes if only subset of all columns is requested. To have previous behavior, set `spark.sql.csv.parser.columnPruning.enabled` to `false`.
## How was this patch tested?
It was tested by new test which selects 3 columns out of 15, by existing tests and by new benchmarks.
Author: Maxim Gekk <maxim.gekk@databricks.com>
Author: Maxim Gekk <max.gekk@gmail.com>
Closes#21415 from MaxGekk/csv-column-pruning2.
## What changes were proposed in this pull request?
In current parquet version,the conf ENABLE_JOB_SUMMARY is deprecated.
When writing to Parquet files, the warning message
```WARN org.apache.parquet.hadoop.ParquetOutputFormat: Setting parquet.enable.summary-metadata is deprecated, please use parquet.summary.metadata.level```
keeps showing up.
From https://github.com/apache/parquet-mr/blame/master/parquet-hadoop/src/main/java/org/apache/parquet/hadoop/ParquetOutputFormat.java#L164 we can see that we should use JOB_SUMMARY_LEVEL.
## How was this patch tested?
Unit test
Author: Gengliang Wang <gengliang.wang@databricks.com>
Closes#21411 from gengliangwang/summaryLevel.
## What changes were proposed in this pull request?
https://docs.google.com/document/d/1IL4kJoKrZWeyIhklKUJqsW-yEN7V7aL05MmM65AYOfE/edit#heading=h.8t3ci57f7uii
Support multiple different row writers in continuous processing shuffle reader.
Note that having multiple read-side buffers ended up being the natural way to do this. Otherwise it's hard to express the constraint of sending an epoch marker only when all writers have sent one.
## How was this patch tested?
new unit tests
Author: Jose Torres <torres.joseph.f+github@gmail.com>
Closes#21385 from jose-torres/multipleWrite.
## What changes were proposed in this pull request?
Fix `date_trunc` function incorrect examples.
## How was this patch tested?
N/A
Author: Yuming Wang <yumwang@ebay.com>
Closes#21423 from wangyum/SPARK-24378.
## What changes were proposed in this pull request?
I missed this commit when preparing #21070.
When Parquet is able to filter blocks with dictionary filtering, the expected total value count to be too high in Spark, leading to an error when there were fewer than expected row groups to process. Spark should get the row groups from Parquet to pick up new filter schemes in Parquet like dictionary filtering.
## How was this patch tested?
Using in production at Netflix. Added test case for dictionary-filtered blocks.
Author: Ryan Blue <blue@apache.org>
Closes#21295 from rdblue/SPARK-24230-fix-parquet-block-tracking.
## What changes were proposed in this pull request?
This PR proposes to follow up https://github.com/apache/spark/pull/15153 and complete SPARK-17599.
`FileSystem` operation (`fs.getFileBlockLocations`) can still fail if the file path does not exist. For example see the exception message below:
```
Error occurred while processing: File does not exist: /rel/00171151/input/PJ/part-00136-b6403bac-a240-44f8-a792-fc2e174682b7-c000.csv
...
java.io.FileNotFoundException: File does not exist: /rel/00171151/input/PJ/part-00136-b6403bac-a240-44f8-a792-fc2e174682b7-c000.csv
...
org.apache.hadoop.hdfs.DistributedFileSystem.getFileBlockLocations(DistributedFileSystem.java:249)
at org.apache.hadoop.hdfs.DistributedFileSystem.getFileBlockLocations(DistributedFileSystem.java:229)
at org.apache.spark.sql.execution.datasources.InMemoryFileIndex$$anonfun$org$apache$spark$sql$execution$datasources$InMemoryFileIndex$$listLeafFiles$3.apply(InMemoryFileIndex.scala:314)
at org.apache.spark.sql.execution.datasources.InMemoryFileIndex$$anonfun$org$apache$spark$sql$execution$datasources$InMemoryFileIndex$$listLeafFiles$3.apply(InMemoryFileIndex.scala:297)
at scala.collection.TraversableLike$$anonfun$map$1.apply(TraversableLike.scala:234)
at scala.collection.TraversableLike$$anonfun$map$1.apply(TraversableLike.scala:234)
at scala.collection.IndexedSeqOptimized$class.foreach(IndexedSeqOptimized.scala:33)
at scala.collection.mutable.ArrayOps$ofRef.foreach(ArrayOps.scala:186)
at scala.collection.TraversableLike$class.map(TraversableLike.scala:234)
at scala.collection.mutable.ArrayOps$ofRef.map(ArrayOps.scala:186)
at org.apache.spark.sql.execution.datasources.InMemoryFileIndex$.org$apache$spark$sql$execution$datasources$InMemoryFileIndex$$listLeafFiles(InMemoryFileIndex.scala:297)
at org.apache.spark.sql.execution.datasources.InMemoryFileIndex$$anonfun$org$apache$spark$sql$execution$datasources$InMemoryFileIndex$$bulkListLeafFiles$1.apply(InMemoryFileIndex.scala:174)
at org.apache.spark.sql.execution.datasources.InMemoryFileIndex$$anonfun$org$apache$spark$sql$execution$datasources$InMemoryFileIndex$$bulkListLeafFiles$1.apply(InMemoryFileIndex.scala:173)
at scala.collection.TraversableLike$$anonfun$map$1.apply(TraversableLike.scala:234)
at scala.collection.TraversableLike$$anonfun$map$1.apply(TraversableLike.scala:234)
at scala.collection.mutable.ResizableArray$class.foreach(ResizableArray.scala:59)
at scala.collection.mutable.ArrayBuffer.foreach(ArrayBuffer.scala:48)
at scala.collection.TraversableLike$class.map(TraversableLike.scala:234)
at scala.collection.AbstractTraversable.map(Traversable.scala:104)
at org.apache.spark.sql.execution.datasources.InMemoryFileIndex$.org$apache$spark$sql$execution$datasources$InMemoryFileIndex$$bulkListLeafFiles(InMemoryFileIndex.scala:173)
at org.apache.spark.sql.execution.datasources.InMemoryFileIndex.listLeafFiles(InMemoryFileIndex.scala:126)
at org.apache.spark.sql.execution.datasources.InMemoryFileIndex.refresh0(InMemoryFileIndex.scala:91)
at org.apache.spark.sql.execution.datasources.InMemoryFileIndex.<init>(InMemoryFileIndex.scala:67)
at org.apache.spark.sql.execution.datasources.DataSource.tempFileIndex$lzycompute$1(DataSource.scala:161)
at org.apache.spark.sql.execution.datasources.DataSource.org$apache$spark$sql$execution$datasources$DataSource$$tempFileIndex$1(DataSource.scala:152)
at org.apache.spark.sql.execution.datasources.DataSource.getOrInferFileFormatSchema(DataSource.scala:166)
at org.apache.spark.sql.execution.datasources.DataSource.sourceSchema(DataSource.scala:261)
at org.apache.spark.sql.execution.datasources.DataSource.sourceInfo$lzycompute(DataSource.scala:94)
at org.apache.spark.sql.execution.datasources.DataSource.sourceInfo(DataSource.scala:94)
at org.apache.spark.sql.execution.streaming.StreamingRelation$.apply(StreamingRelation.scala:33)
at org.apache.spark.sql.streaming.DataStreamReader.load(DataStreamReader.scala:196)
at org.apache.spark.sql.streaming.DataStreamReader.load(DataStreamReader.scala:206)
at com.hwx.StreamTest$.main(StreamTest.scala:97)
at com.hwx.StreamTest.main(StreamTest.scala)
at sun.reflect.NativeMethodAccessorImpl.invoke0(Native Method)
at sun.reflect.NativeMethodAccessorImpl.invoke(NativeMethodAccessorImpl.java:62)
at sun.reflect.DelegatingMethodAccessorImpl.invoke(DelegatingMethodAccessorImpl.java:43)
at java.lang.reflect.Method.invoke(Method.java:498)
at org.apache.spark.deploy.JavaMainApplication.start(SparkApplication.scala:52)
at org.apache.spark.deploy.SparkSubmit$.org$apache$spark$deploy$SparkSubmit$$runMain(SparkSubmit.scala:906)
at org.apache.spark.deploy.SparkSubmit$.doRunMain$1(SparkSubmit.scala:197)
at org.apache.spark.deploy.SparkSubmit$.submit(SparkSubmit.scala:227)
at org.apache.spark.deploy.SparkSubmit$.main(SparkSubmit.scala:136)
at org.apache.spark.deploy.SparkSubmit.main(SparkSubmit.scala)
Caused by: org.apache.hadoop.ipc.RemoteException(java.io.FileNotFoundException): File does not exist: /rel/00171151/input/PJ/part-00136-b6403bac-a240-44f8-a792-fc2e174682b7-c000.csv
...
```
So, it fixes it to make a warning instead.
## How was this patch tested?
It's hard to write a test. Manually tested multiple times.
Author: hyukjinkwon <gurwls223@apache.org>
Closes#21408 from HyukjinKwon/missing-files.
## What changes were proposed in this pull request?
ORC 1.4.4 includes [nine fixes](https://issues.apache.org/jira/issues/?filter=12342568&jql=project%20%3D%20ORC%20AND%20resolution%20%3D%20Fixed%20AND%20fixVersion%20%3D%201.4.4). One of the issues is about `Timestamp` bug (ORC-306) which occurs when `native` ORC vectorized reader reads ORC column vector's sub-vector `times` and `nanos`. ORC-306 fixes this according to the [original definition](https://github.com/apache/hive/blob/master/storage-api/src/java/org/apache/hadoop/hive/ql/exec/vector/TimestampColumnVector.java#L45-L46) and this PR includes the updated interpretation on ORC column vectors. Note that `hive` ORC reader and ORC MR reader is not affected.
```scala
scala> spark.version
res0: String = 2.3.0
scala> spark.sql("set spark.sql.orc.impl=native")
scala> Seq(java.sql.Timestamp.valueOf("1900-05-05 12:34:56.000789")).toDF().write.orc("/tmp/orc")
scala> spark.read.orc("/tmp/orc").show(false)
+--------------------------+
|value |
+--------------------------+
|1900-05-05 12:34:55.000789|
+--------------------------+
```
This PR aims to update Apache Spark to use it.
**FULL LIST**
ID | TITLE
-- | --
ORC-281 | Fix compiler warnings from clang 5.0
ORC-301 | `extractFileTail` should open a file in `try` statement
ORC-304 | Fix TestRecordReaderImpl to not fail with new storage-api
ORC-306 | Fix incorrect workaround for bug in java.sql.Timestamp
ORC-324 | Add support for ARM and PPC arch
ORC-330 | Remove unnecessary Hive artifacts from root pom
ORC-332 | Add syntax version to orc_proto.proto
ORC-336 | Remove avro and parquet dependency management entries
ORC-360 | Implement error checking on subtype fields in Java
## How was this patch tested?
Pass the Jenkins.
Author: Dongjoon Hyun <dongjoon@apache.org>
Closes#21372 from dongjoon-hyun/SPARK_ORC144.
LongToUnsafeRowMap has a mistake when growing its page array: it blindly grows to `oldSize * 2`, while the new record may be larger than `oldSize * 2`. Then we may have a malformed UnsafeRow when querying this map, whose actual data is smaller than its declared size, and the data is corrupted.
Author: sychen <sychen@ctrip.com>
Closes#21311 from cxzl25/fix_LongToUnsafeRowMap_page_size.
## What changes were proposed in this pull request?
### Fixes `ClassCastException` in the `array_position` function - [SPARK-24350](https://issues.apache.org/jira/browse/SPARK-24350)
When calling `array_position` function with a wrong type of the 1st argument an `AnalysisException` should be thrown instead of `ClassCastException`
Example:
```sql
select array_position('foo', 'bar')
```
```
java.lang.ClassCastException: org.apache.spark.sql.types.StringType$ cannot be cast to org.apache.spark.sql.types.ArrayType
at org.apache.spark.sql.catalyst.expressions.ArrayPosition.inputTypes(collectionOperations.scala:1398)
at org.apache.spark.sql.catalyst.expressions.ExpectsInputTypes$class.checkInputDataTypes(ExpectsInputTypes.scala:44)
at org.apache.spark.sql.catalyst.expressions.ArrayPosition.checkInputDataTypes(collectionOperations.scala:1401)
at org.apache.spark.sql.catalyst.expressions.Expression.resolved$lzycompute(Expression.scala:168)
at org.apache.spark.sql.catalyst.expressions.Expression.resolved(Expression.scala:168)
at org.apache.spark.sql.catalyst.analysis.Analyzer$ResolveAliases$$anonfun$org$apache$spark$sql$catalyst$analysis$Analyzer$ResolveAliases$$assignAliases$1$$anonfun$apply$3.applyOrElse(Analyzer.scala:256)
at org.apache.spark.sql.catalyst.analysis.Analyzer$ResolveAliases$$anonfun$org$apache$spark$sql$catalyst$analysis$Analyzer$ResolveAliases$$assignAliases$1$$anonfun$apply$3.applyOrElse(Analyzer.scala:252)
at org.apache.spark.sql.catalyst.trees.TreeNode$$anonfun$transformUp$1.apply(TreeNode.scala:289)
at org.apache.spark.sql.catalyst.trees.TreeNode$$anonfun$transformUp$1.apply(TreeNode.scala:289)
at org.apache.spark.sql.catalyst.trees.CurrentOrigin$.withOrigin(TreeNode.scala:70)
at org.apache.spark.sql.catalyst.trees.TreeNode.transformUp(TreeNode.scala:288)
```
## How was this patch tested?
unit test
Author: Vayda, Oleksandr: IT (PRG) <Oleksandr.Vayda@barclayscapital.com>
Closes#21401 from wajda/SPARK-24350-array_position-error-fix.
## What changes were proposed in this pull request?
Add a specific stop method for ContinuousExecution. The previous StreamExecution.stop() method had a race condition as applied to continuous processing: if the cancellation was round-tripped to the driver too quickly, the generic SparkException it caused would be reported as the query death cause. We earlier decided that SparkException should not be added to the StreamExecution.isInterruptionException() whitelist, so we need to ensure this never happens instead.
## How was this patch tested?
Existing tests. I could consistently reproduce the previous flakiness by putting Thread.sleep(1000) between the first job cancellation and thread interruption in StreamExecution.stop().
Author: Jose Torres <torres.joseph.f+github@gmail.com>
Closes#21384 from jose-torres/fixKafka.
## What changes were proposed in this pull request?
When OutOfMemoryError thrown from BroadcastExchangeExec, scala.concurrent.Future will hit scala bug – https://github.com/scala/bug/issues/9554, and hang until future timeout:
We could wrap the OOM inside SparkException to resolve this issue.
## How was this patch tested?
Manually tested.
Author: jinxing <jinxing6042@126.com>
Closes#21342 from jinxing64/SPARK-24294.
## What changes were proposed in this pull request?
This pr added benchmark code `DataSourceReadBenchmark` for `orc`, `paruqet`, `csv`, and `json` based on the existing `ParquetReadBenchmark` and `OrcReadBenchmark`.
## How was this patch tested?
N/A
Author: Takeshi Yamamuro <yamamuro@apache.org>
Closes#21266 from maropu/DataSourceReadBenchmark.
## What changes were proposed in this pull request?
Add fallback logic for `UnsafeProjection`. In production we can try to create unsafe projection using codegen implementation. Once any compile error happens, it fallbacks to interpreted implementation.
## How was this patch tested?
Added test.
Author: Liang-Chi Hsieh <viirya@gmail.com>
Closes#21106 from viirya/SPARK-23711.
This is a documentation-only correction; `org.apache.spark.sql.sources.v2.reader.Offset` is actually `org.apache.spark.sql.sources.v2.reader.streaming.Offset`.
Author: Seth Fitzsimmons <seth@mojodna.net>
Closes#21387 from mojodna/patch-1.
## What changes were proposed in this pull request?
### Fixes a `scala.MatchError` in the `element_at` operation - [SPARK-24348](https://issues.apache.org/jira/browse/SPARK-24348)
When calling `element_at` with a wrong first operand type an `AnalysisException` should be thrown instead of `scala.MatchError`
*Example:*
```sql
select element_at('foo', 1)
```
results in:
```
scala.MatchError: StringType (of class org.apache.spark.sql.types.StringType$)
at org.apache.spark.sql.catalyst.expressions.ElementAt.inputTypes(collectionOperations.scala:1469)
at org.apache.spark.sql.catalyst.expressions.ExpectsInputTypes$class.checkInputDataTypes(ExpectsInputTypes.scala:44)
at org.apache.spark.sql.catalyst.expressions.ElementAt.checkInputDataTypes(collectionOperations.scala:1478)
at org.apache.spark.sql.catalyst.expressions.Expression.resolved$lzycompute(Expression.scala:168)
at org.apache.spark.sql.catalyst.expressions.Expression.resolved(Expression.scala:168)
at org.apache.spark.sql.catalyst.analysis.Analyzer$ResolveAliases$$anonfun$org$apache$spark$sql$catalyst$analysis$Analyzer$ResolveAliases$$assignAliases$1$$anonfun$apply$3.applyOrElse(Analyzer.scala:256)
at org.apache.spark.sql.catalyst.analysis.Analyzer$ResolveAliases$$anonfun$org$apache$spark$sql$catalyst$analysis$Analyzer$ResolveAliases$$assignAliases$1$$anonfun$apply$3.applyOrElse(Analyzer.scala:252)
at org.apache.spark.sql.catalyst.trees.TreeNode$$anonfun$transformUp$1.apply(TreeNode.scala:289)
at org.apache.spark.sql.catalyst.trees.TreeNode$$anonfun$transformUp$1.apply(TreeNode.scala:289)
at org.apache.spark.sql.catalyst.trees.CurrentOrigin$.withOrigin(TreeNode.scala:70)
at org.apache.spark.sql.catalyst.trees.TreeNode.transformUp(TreeNode.scala:288)
```
## How was this patch tested?
unit tests
Author: Vayda, Oleksandr: IT (PRG) <Oleksandr.Vayda@barclayscapital.com>
Closes#21395 from wajda/SPARK-24348-element_at-error-fix.
## What changes were proposed in this pull request?
This patch tries to implement this [proposal](https://github.com/apache/spark/pull/19813#issuecomment-354045400) to add an API for handling expression code generation. It should allow us to manipulate how to generate codes for expressions.
In details, this adds an new abstraction `CodeBlock` to `JavaCode`. `CodeBlock` holds the code snippet and inputs for generating actual java code.
For example, in following java code:
```java
int ${variable} = 1;
boolean ${isNull} = ${CodeGenerator.defaultValue(BooleanType)};
```
`variable`, `isNull` are two `VariableValue` and `CodeGenerator.defaultValue(BooleanType)` is a string. They are all inputs to this code block and held by `CodeBlock` representing this code.
For codegen, we provide a specified string interpolator `code`, so you can define a code like this:
```scala
val codeBlock =
code"""
|int ${variable} = 1;
|boolean ${isNull} = ${CodeGenerator.defaultValue(BooleanType)};
""".stripMargin
// Generates actual java code.
codeBlock.toString
```
Because those inputs are held separately in `CodeBlock` before generating code, we can safely manipulate them, e.g., replacing statements to aliased variables, etc..
## How was this patch tested?
Added tests.
Author: Liang-Chi Hsieh <viirya@gmail.com>
Closes#21193 from viirya/SPARK-24121.
## What changes were proposed in this pull request?
uniVocity parser allows to specify only required column names or indexes for [parsing](https://www.univocity.com/pages/parsers-tutorial) like:
```
// Here we select only the columns by their indexes.
// The parser just skips the values in other columns
parserSettings.selectIndexes(4, 0, 1);
CsvParser parser = new CsvParser(parserSettings);
```
In this PR, I propose to extract indexes from required schema and pass them into the CSV parser. Benchmarks show the following improvements in parsing of 1000 columns:
```
Select 100 columns out of 1000: x1.76
Select 1 column out of 1000: x2
```
**Note**: Comparing to current implementation, the changes can return different result for malformed rows in the `DROPMALFORMED` and `FAILFAST` modes if only subset of all columns is requested. To have previous behavior, set `spark.sql.csv.parser.columnPruning.enabled` to `false`.
## How was this patch tested?
It was tested by new test which selects 3 columns out of 15, by existing tests and by new benchmarks.
Author: Maxim Gekk <maxim.gekk@databricks.com>
Closes#21296 from MaxGekk/csv-column-pruning.
## What changes were proposed in this pull request?
`spark-sql` silent mode will broken if`SPARK_HOME/jars` missing `kubernetes-model-2.0.0.jar`.
This pr use `sc.setLogLevel (<logLevel>)` to implement silent mode.
## How was this patch tested?
manual tests
```
build/sbt -Phive -Phive-thriftserver package
export SPARK_PREPEND_CLASSES=true
./bin/spark-sql -S
```
Author: Yuming Wang <yumwang@ebay.com>
Closes#20274 from wangyum/SPARK-20120-FOLLOW-UP.
## What changes were proposed in this pull request?
The interpreted evaluation of several collection operations works only for simple datatypes. For complex data types, for instance, `array_contains` it returns always `false`. The list of the affected functions is `array_contains`, `array_position`, `element_at` and `GetMapValue`.
The PR fixes the behavior for all the datatypes.
## How was this patch tested?
added UT
Author: Marco Gaido <marcogaido91@gmail.com>
Closes#21361 from mgaido91/SPARK-24313.
## What changes were proposed in this pull request?
Extract common code from `Divide`/`Remainder` to a new base trait, `DivModLike`.
Further refactoring to make `Pmod` work with `DivModLike` is to be done as a separate task.
## How was this patch tested?
Existing tests in `ArithmeticExpressionSuite` covers the functionality.
Author: Kris Mok <kris.mok@databricks.com>
Closes#21367 from rednaxelafx/catalyst-divmod.
## What changes were proposed in this pull request?
The PR retrieves the proxyBase automatically from the header `X-Forwarded-Context` (if available). This is the header used by Knox to inform the proxied service about the base path.
This provides 0-configuration support for Knox gateway (instead of having to properly set `spark.ui.proxyBase`) and it allows to access directly SHS when it is proxied by Knox. In the previous scenario, indeed, after setting `spark.ui.proxyBase`, direct access to SHS was not working fine (due to bad link generated).
## How was this patch tested?
added UT + manual tests
Author: Marco Gaido <marcogaido91@gmail.com>
Closes#21268 from mgaido91/SPARK-24209.
## What changes were proposed in this pull request?
The tests cover basic functionality of [Hadoop LinesReader](8d79113b81/sql/core/src/main/scala/org/apache/spark/sql/execution/datasources/HadoopFileLinesReader.scala (L42)). In particular, the added tests check:
- A split slices a line or delimiter
- A split slices two consecutive lines and cover a delimiter between the lines
- Two splits slice a line and there are no duplicates
- Internal buffer size (`io.file.buffer.size`) is less than line length
- Constrain of maximum line length - `mapreduce.input.linerecordreader.line.maxlength`
Author: Maxim Gekk <maxim.gekk@databricks.com>
Closes#21377 from MaxGekk/line-reader-tests.
re-submit https://github.com/apache/spark/pull/21299 which broke build.
A few new commits are added to fix the SQLConf problem in `JsonSchemaInference.infer`, and prevent us to access `SQLConf` in DAGScheduler event loop thread.
## What changes were proposed in this pull request?
Previously in #20136 we decided to forbid tasks to access `SQLConf`, because it doesn't work and always give you the default conf value. In #21190 we fixed the check and all the places that violate it.
Currently the pattern of accessing configs at the executor side is: read the configs at the driver side, then access the variables holding the config values in the RDD closure, so that they will be serialized to the executor side. Something like
```
val someConf = conf.getXXX
child.execute().mapPartitions {
if (someConf == ...) ...
...
}
```
However, this pattern is hard to apply if the config needs to be propagated via a long call stack. An example is `DataType.sameType`, and see how many changes were made in #21190 .
When it comes to code generation, it's even worse. I tried it locally and we need to change a ton of files to propagate configs to code generators.
This PR proposes to allow tasks to access `SQLConf`. The idea is, we can save all the SQL configs to job properties when an SQL execution is triggered. At executor side we rebuild the `SQLConf` from job properties.
## How was this patch tested?
a new test suite
Author: Wenchen Fan <wenchen@databricks.com>
Closes#21376 from cloud-fan/config.
## What changes were proposed in this pull request?
This PR fixes the following errors reported by `lint-java`
```
% dev/lint-java
Using `mvn` from path: /usr/bin/mvn
Checkstyle checks failed at following occurrences:
[ERROR] src/main/java/org/apache/spark/sql/sources/v2/reader/InputPartition.java:[39] (sizes) LineLength: Line is longer than 100 characters (found 104).
[ERROR] src/main/java/org/apache/spark/sql/sources/v2/reader/InputPartitionReader.java:[26] (sizes) LineLength: Line is longer than 100 characters (found 110).
[ERROR] src/main/java/org/apache/spark/sql/sources/v2/reader/InputPartitionReader.java:[30] (sizes) LineLength: Line is longer than 100 characters (found 104).
```
## How was this patch tested?
Run `lint-java` manually.
Author: Kazuaki Ishizaki <ishizaki@jp.ibm.com>
Closes#21374 from kiszk/SPARK-24323.
## What changes were proposed in this pull request?
Logical `Range` node has been added with `outputOrdering` recently. It's used to eliminate redundant `Sort` during optimization. However, this `outputOrdering` doesn't not propagate to physical `RangeExec` node.
We also add correct `outputPartitioning` to `RangeExec` node.
## How was this patch tested?
Added test.
Author: Liang-Chi Hsieh <viirya@gmail.com>
Closes#21291 from viirya/SPARK-24242.
## What changes were proposed in this pull request?
Previously in #20136 we decided to forbid tasks to access `SQLConf`, because it doesn't work and always give you the default conf value. In #21190 we fixed the check and all the places that violate it.
Currently the pattern of accessing configs at the executor side is: read the configs at the driver side, then access the variables holding the config values in the RDD closure, so that they will be serialized to the executor side. Something like
```
val someConf = conf.getXXX
child.execute().mapPartitions {
if (someConf == ...) ...
...
}
```
However, this pattern is hard to apply if the config needs to be propagated via a long call stack. An example is `DataType.sameType`, and see how many changes were made in #21190 .
When it comes to code generation, it's even worse. I tried it locally and we need to change a ton of files to propagate configs to code generators.
This PR proposes to allow tasks to access `SQLConf`. The idea is, we can save all the SQL configs to job properties when an SQL execution is triggered. At executor side we rebuild the `SQLConf` from job properties.
## How was this patch tested?
a new test suite
Author: Wenchen Fan <wenchen@databricks.com>
Closes#21299 from cloud-fan/config.
## What changes were proposed in this pull request?
Made changes to EpochCoordinator so that it enforces a commit order. In case a message for epoch n is lost and epoch (n + 1) is ready for commit before epoch n is, epoch (n + 1) will wait for epoch n to be committed first.
## How was this patch tested?
Existing tests in ContinuousSuite and EpochCoordinatorSuite.
Author: Efim Poberezkin <efim@poberezkin.ru>
Closes#20936 from efimpoberezkin/pr/sequence-commited-epochs.
## What changes were proposed in this pull request?
SPARK-24073 renames DataReaderFactory -> InputPartition and DataReader -> InputPartitionReader. Some classes still reflects the old name and causes confusion. This patch renames the left over classes to reflect the new interface and fixes a few comments.
## How was this patch tested?
Existing unit tests.
Please review http://spark.apache.org/contributing.html before opening a pull request.
Author: Arun Mahadevan <arunm@apache.org>
Closes#21355 from arunmahadevan/SPARK-24308.
## What changes were proposed in this pull request?
This pr added an option `queryTimeout` for the number of seconds the the driver will wait for a Statement object to execute.
## How was this patch tested?
Added tests in `JDBCSuite`.
Author: Takeshi Yamamuro <yamamuro@apache.org>
Closes#21173 from maropu/SPARK-23856.
The old code was relying on a core configuration and extended its
default value to include things that redact desired things in the
app's environment. Instead, add a SQL-specific option for which
options to redact, and apply both the core and SQL-specific rules
when redacting the options in the save command.
This is a little sub-optimal since it adds another config, but it
retains the current default behavior.
While there I also fixed a typo and a couple of minor config API
usage issues in the related redaction option that SQL already had.
Tested with existing unit tests, plus checking the env page on
a shell UI.
Author: Marcelo Vanzin <vanzin@cloudera.com>
Closes#21158 from vanzin/SPARK-23850.
## What changes were proposed in this pull request?
Enabled no-data batches in flatMapGroupsWithState in following two cases.
- When ProcessingTime timeout is used, then we always run a batch every trigger interval.
- When event-time watermark is defined, then the user may be doing arbitrary logic against the watermark value even if timeouts are not set. In such cases, it's best to run batches whenever the watermark has changed, irrespective of whether timeouts (i.e. event-time timeout) have been explicitly enabled.
## How was this patch tested?
updated tests
Author: Tathagata Das <tathagata.das1565@gmail.com>
Closes#21345 from tdas/SPARK-24159.
## What changes were proposed in this pull request?
Wrap Dataset.reduce with `withNewExecutionId`.
Author: Soham Aurangabadkar <sohama4@gmail.com>
Closes#21316 from sohama4/dataset_reduce_withexecutionid.
## What changes were proposed in this pull request?
In HadoopMapReduceCommitProtocol and FileFormatWriter, there are unnecessary settings in hadoop configuration.
Also clean up some code in SQL module.
## How was this patch tested?
Unit test
Author: Gengliang Wang <gengliang.wang@databricks.com>
Closes#21329 from gengliangwang/codeCleanWrite.
## What changes were proposed in this pull request?
Physical plan of `select colA from t order by colB limit M` is `TakeOrderedAndProject`;
Currently `TakeOrderedAndProject` sorts data in memory, see https://github.com/apache/spark/blob/master/sql/core/src/main/scala/org/apache/spark/sql/execution/limit.scala#L158
We can add a config – if the number of limit (M) is too big, we can sort by disk. Thus memory issue can be resolved.
## How was this patch tested?
Test added
Author: jinxing <jinxing6042@126.com>
Closes#21252 from jinxing64/SPARK-24193.
## What changes were proposed in this pull request?
The PR adds the function `arrays_overlap`. This function returns `true` if the input arrays contain a non-null common element; if not, it returns `null` if any of the arrays contains a `null` element, `false` otherwise.
## How was this patch tested?
added UTs
Author: Marco Gaido <marcogaido91@gmail.com>
Closes#21028 from mgaido91/SPARK-23922.
## What changes were proposed in this pull request?
The PR adds a new collection function, array_repeat. As there already was a function repeat with the same signature, with the only difference being the expected return type (String instead of Array), the new function is called array_repeat to distinguish.
The behaviour of the function is based on Presto's one.
The function creates an array containing a given element repeated the requested number of times.
## How was this patch tested?
New unit tests added into:
- CollectionExpressionsSuite
- DataFrameFunctionsSuite
Author: Florent Pépin <florentpepin.92@gmail.com>
Author: Florent Pépin <florent.pepin14@imperial.ac.uk>
Closes#21208 from pepinoflo/SPARK-23925.
## What changes were proposed in this pull request?
This is a continuation of the larger task of enabling zero-data batches for more eager state cleanup. This PR enables it for stream-stream joins.
## How was this patch tested?
- Updated join tests. Additionally, updated them to not use `CheckLastBatch` anywhere to set good precedence for future.
Author: Tathagata Das <tathagata.das1565@gmail.com>
Closes#21253 from tdas/SPARK-24158.
## What changes were proposed in this pull request?
In #21145, DataReaderFactory is renamed to InputPartition.
This PR is to revise wording in the comments to make it more clear.
## How was this patch tested?
None
Author: Gengliang Wang <gengliang.wang@databricks.com>
Closes#21326 from gengliangwang/revise_reader_comments.
## What changes were proposed in this pull request?
Support aggregates with exactly 1 partition in continuous processing.
A few small tweaks are needed to make this work:
* Replace currentEpoch tracking with an ThreadLocal. This means that current epoch is scoped to a task rather than a node, but I think that's sustainable even once we add shuffle.
* Add a new testing-only flag to disable the UnsupportedOperationChecker whitelist of allowed continuous processing nodes. I think this is preferable to writing a pile of custom logic to enforce that there is in fact only 1 partition; we plan to support multi-partition aggregates before the next Spark release, so we'd just have to tear that logic back out.
* Restart continuous processing queries from the first available uncommitted epoch, rather than one that's guaranteed to be unused. This is required for stateful operators to overwrite partial state from the previous attempt at the epoch, and there was no specific motivation for the original strategy. In another PR before stabilizing the StreamWriter API, we'll need to narrow down and document more precise semantic guarantees for the epoch IDs.
* We need a single-partition ContinuousMemoryStream. The way MemoryStream is constructed means it can't be a text option like it is for rate source, unfortunately.
## How was this patch tested?
new unit tests
Author: Jose Torres <torres.joseph.f+github@gmail.com>
Closes#21239 from jose-torres/withAggr.
## What changes were proposed in this pull request?
Right now `ArrayWriter` used to output Arrow data for array type, doesn't do `clear` or `reset` after each batch. It produces wrong output.
## How was this patch tested?
Added test.
Author: Liang-Chi Hsieh <viirya@gmail.com>
Closes#21312 from viirya/SPARK-24259.
## What changes were proposed in this pull request?
1. Change antlr rule to fix the warning.
2. Add PIVOT/LATERAL check in AstBuilder with a more meaningful error message.
## How was this patch tested?
1. Add a counter case in `PlanParserSuite.test("lateral view")`
Author: maryannxue <maryann.xue@gmail.com>
Closes#21324 from maryannxue/spark-24035-fix.
## What changes were proposed in this pull request?
This PR adds isEmpty() in DataSet
## How was this patch tested?
Unit tests added
Please review http://spark.apache.org/contributing.html before opening a pull request.
Author: Goun Na <gounna@gmail.com>
Author: goungoun <gounna@gmail.com>
Closes#20800 from goungoun/SPARK-23627.
## What changes were proposed in this pull request?
Add a `withSQLConf(...)` wrapper to force Parquet filter pushdown for a test that relies on it.
## How was this patch tested?
Test passes
Author: Henry Robinson <henry@apache.org>
Closes#21323 from henryr/spark-23582.
## What changes were proposed in this pull request?
Currently, the from_json function support StructType or ArrayType as the root type. The PR allows to specify MapType(StringType, DataType) as the root type additionally to mentioned types. For example:
```scala
import org.apache.spark.sql.types._
val schema = MapType(StringType, IntegerType)
val in = Seq("""{"a": 1, "b": 2, "c": 3}""").toDS()
in.select(from_json($"value", schema, Map[String, String]())).collect()
```
```
res1: Array[org.apache.spark.sql.Row] = Array([Map(a -> 1, b -> 2, c -> 3)])
```
## How was this patch tested?
It was checked by new tests for the map type with integer type and struct type as value types. Also roundtrip tests like from_json(to_json) and to_json(from_json) for MapType are added.
Author: Maxim Gekk <maxim.gekk@databricks.com>
Author: Maxim Gekk <max.gekk@gmail.com>
Closes#21108 from MaxGekk/from_json-map-type.
## What changes were proposed in this pull request?
If there is an exception, it's better to set it as the cause of AnalysisException since the exception may contain useful debug information.
## How was this patch tested?
Jenkins
Author: Shixiong Zhu <zsxwing@gmail.com>
Closes#21297 from zsxwing/SPARK-24246.
## What changes were proposed in this pull request?
This PR fixes the following Java lint errors due to importing unimport classes
```
$ dev/lint-java
Using `mvn` from path: /usr/bin/mvn
Checkstyle checks failed at following occurrences:
[ERROR] src/main/java/org/apache/spark/sql/sources/v2/reader/partitioning/Distribution.java:[25] (sizes) LineLength: Line is longer than 100 characters (found 109).
[ERROR] src/main/java/org/apache/spark/sql/sources/v2/reader/streaming/ContinuousReader.java:[38] (sizes) LineLength: Line is longer than 100 characters (found 102).
[ERROR] src/main/java/org/apache/spark/sql/execution/datasources/parquet/SpecificParquetRecordReaderBase.java:[21,8] (imports) UnusedImports: Unused import - java.io.ByteArrayInputStream.
[ERROR] src/main/java/org/apache/spark/sql/execution/datasources/parquet/VectorizedPlainValuesReader.java:[29,8] (imports) UnusedImports: Unused import - org.apache.spark.unsafe.Platform.
[ERROR] src/test/java/test/org/apache/spark/sql/sources/v2/JavaAdvancedDataSourceV2.java:[110] (sizes) LineLength: Line is longer than 100 characters (found 101).
```
With this PR
```
$ dev/lint-java
Using `mvn` from path: /usr/bin/mvn
Checkstyle checks passed.
```
## How was this patch tested?
Existing UTs. Also manually run checkstyles against these two files.
Author: Kazuaki Ishizaki <ishizaki@jp.ibm.com>
Closes#21301 from kiszk/SPARK-24228.
The `implicitNotFound` message for `Encoder` doesn't mention the name of
the type for which it can't find an encoder. Furthermore, it covers up
the fact that `Encoder` is the name of the relevant type class.
Hopefully this new message provides a little more specific type detail
while still giving the general message about which types are supported.
## What changes were proposed in this pull request?
Augment the existing message to mention that it's looking for an `Encoder` and what the type of the encoder is.
For example instead of:
```
Unable to find encoder for type stored in a Dataset. Primitive types (Int, String, etc) and Product types (case classes) are supported by importing spark.implicits._ Support for serializing other types will be added in future releases.
```
return this message:
```
Unable to find encoder for type Exception. An implicit Encoder[Exception] is needed to store Exception instances in a Dataset. Primitive types (Int, String, etc) and Product types (ca
se classes) are supported by importing spark.implicits._ Support for serializing other types will be added in future releases.
```
## How was this patch tested?
It was tested manually in the Scala REPL, since triggering this in a test would cause a compilation error.
```
scala> implicitly[Encoder[Exception]]
<console>:51: error: Unable to find encoder for type Exception. An implicit Encoder[Exception] is needed to store Exception instances in a Dataset. Primitive types (Int, String, etc) and Product types (ca
se classes) are supported by importing spark.implicits._ Support for serializing other types will be added in future releases.
implicitly[Encoder[Exception]]
^
```
Author: Cody Allen <ceedubs@gmail.com>
Closes#20869 from ceedubs/encoder-implicit-msg.
## What changes were proposed in this pull request?
This patch removes the various regr_* functions in functions.scala. They are so uncommon that I don't think they deserve real estate in functions.scala. We can consider adding them later if more users need them.
## How was this patch tested?
Removed the associated test case as well.
Author: Reynold Xin <rxin@databricks.com>
Closes#21309 from rxin/SPARK-23907.
## What changes were proposed in this pull request?
It's useful to know what relationship between date1 and date2 results in a positive number.
Author: aditkumar <aditkumar@gmail.com>
Author: Adit Kumar <aditkumar@gmail.com>
Closes#20787 from aditkumar/master.
## What changes were proposed in this pull request?
In `PushDownOperatorsToDataSource`, we use `transformUp` to match `PhysicalOperation` and apply pushdown. This is problematic if we have multiple `Filter` and `Project` above the data source v2 relation.
e.g. for a query
```
Project
Filter
DataSourceV2Relation
```
The pattern match will be triggered twice and we will do operator pushdown twice. This is unnecessary, we can use `mapChildren` to only apply pushdown once.
## How was this patch tested?
existing test
Author: Wenchen Fan <wenchen@databricks.com>
Closes#21230 from cloud-fan/step2.
## What changes were proposed in this pull request?
This is a followup of https://github.com/apache/spark/pull/20136 . #20136 didn't really work because in the test, we are using local backend, which shares the driver side `SparkEnv`, so `SparkEnv.get.executorId == SparkContext.DRIVER_IDENTIFIER` doesn't work.
This PR changes the check to `TaskContext.get != null`, and move the check to `SQLConf.get`, and fix all the places that violate this check:
* `InMemoryTableScanExec#createAndDecompressColumn` is executed inside `rdd.map`, we can't access `conf.offHeapColumnVectorEnabled` there. https://github.com/apache/spark/pull/21223 merged
* `DataType#sameType` may be executed in executor side, for things like json schema inference, so we can't call `conf.caseSensitiveAnalysis` there. This contributes to most of the code changes, as we need to add `caseSensitive` parameter to a lot of methods.
* `ParquetFilters` is used in the file scan function, which is executed in executor side, so we can't can't call `conf.parquetFilterPushDownDate` there. https://github.com/apache/spark/pull/21224 merged
* `WindowExec#createBoundOrdering` is called on executor side, so we can't use `conf.sessionLocalTimezone` there. https://github.com/apache/spark/pull/21225 merged
* `JsonToStructs` can be serialized to executors and evaluate, we should not call `SQLConf.get.getConf(SQLConf.FROM_JSON_FORCE_NULLABLE_SCHEMA)` in the body. https://github.com/apache/spark/pull/21226 merged
## How was this patch tested?
existing test
Author: Wenchen Fan <wenchen@databricks.com>
Closes#21190 from cloud-fan/minor.
## What changes were proposed in this pull request?
I propose to add a clear statement for functions like `collect_list()` about non-deterministic behavior of such functions. The behavior must be taken into account by user while creating and running queries.
Author: Maxim Gekk <maxim.gekk@databricks.com>
Closes#21228 from MaxGekk/deterministic-comments.
## What changes were proposed in this pull request?
The PR introduces regr_slope, regr_intercept, regr_r2, regr_sxx, regr_syy, regr_sxy, regr_avgx, regr_avgy, regr_count.
The implementation of this functions mirrors Hive's one in HIVE-15978.
## How was this patch tested?
added UT (values compared with Hive)
Author: Marco Gaido <marcogaido91@gmail.com>
Closes#21054 from mgaido91/SPARK-23907.
## What changes were proposed in this pull request?
We reverted `spark.sql.hive.convertMetastoreOrc` at https://github.com/apache/spark/pull/20536 because we should not ignore the table-specific compression conf. Now, it's resolved via [SPARK-23355](8aa1d7b0ed).
## How was this patch tested?
Pass the Jenkins.
Author: Dongjoon Hyun <dongjoon@apache.org>
Closes#21186 from dongjoon-hyun/SPARK-24112.
## What changes were proposed in this pull request?
Renames:
* `DataReaderFactory` to `InputPartition`
* `DataReader` to `InputPartitionReader`
* `createDataReaderFactories` to `planInputPartitions`
* `createUnsafeDataReaderFactories` to `planUnsafeInputPartitions`
* `createBatchDataReaderFactories` to `planBatchInputPartitions`
This fixes the changes in SPARK-23219, which renamed ReadTask to
DataReaderFactory. The intent of that change was to make the read and
write API match (write side uses DataWriterFactory), but the underlying
problem is that the two classes are not equivalent.
ReadTask/DataReader function as Iterable/Iterator. One InputPartition is
a specific partition of the data to be read, in contrast to
DataWriterFactory where the same factory instance is used in all write
tasks. InputPartition's purpose is to manage the lifecycle of the
associated reader, which is now called InputPartitionReader, with an
explicit create operation to mirror the close operation. This was no
longer clear from the API because DataReaderFactory appeared to be more
generic than it is and it isn't clear why a set of them is produced for
a read.
## How was this patch tested?
Existing tests, which have been updated to use the new name.
Author: Ryan Blue <blue@apache.org>
Closes#21145 from rdblue/SPARK-24073-revert-data-reader-factory-rename.
## What changes were proposed in this pull request?
Add a new test that triggers if PARQUET-1217 - a predicate pushdown bug - is not fixed in Spark's Parquet dependency.
## How was this patch tested?
New unit test passes.
Author: Henry Robinson <henry@apache.org>
Closes#21284 from henryr/spark-23852.
## What changes were proposed in this pull request?
We should overwrite "otherCopyArgs" to provide the SparkSession parameter otherwise TreeNode.toJSON cannot get the full constructor parameter list.
## How was this patch tested?
The new unit test.
Author: Shixiong Zhu <zsxwing@gmail.com>
Closes#21275 from zsxwing/SPARK-24214.
## What changes were proposed in this pull request?
The exception message should clearly distinguish sorting and bucketing in `save` and `jdbc` write.
When a user tries to write a sorted data using save or insertInto, it will throw an exception with message that `s"'$operation' does not support bucketing right now""`.
We should throw `s"'$operation' does not support sortBy right now""` instead.
## How was this patch tested?
More tests in `DataFrameReaderWriterSuite.scala`
Author: DB Tsai <d_tsai@apple.com>
Closes#21235 from dbtsai/fixException.
## What changes were proposed in this pull request?
This updates Parquet to 1.10.0 and updates the vectorized path for buffer management changes. Parquet 1.10.0 uses ByteBufferInputStream instead of byte arrays in encoders. This allows Parquet to break allocations into smaller chunks that are better for garbage collection.
## How was this patch tested?
Existing Parquet tests. Running in production at Netflix for about 3 months.
Author: Ryan Blue <blue@apache.org>
Closes#21070 from rdblue/SPARK-23972-update-parquet-to-1.10.0.
## What changes were proposed in this pull request?
While reading CSV or JSON files, DataFrameReader's options are converted to Hadoop's parameters, for example there:
https://github.com/apache/spark/blob/branch-2.3/sql/core/src/main/scala/org/apache/spark/sql/execution/DataSourceScanExec.scala#L302
but the options are not propagated to Text datasource on schema inferring, for instance:
https://github.com/apache/spark/blob/branch-2.3/sql/core/src/main/scala/org/apache/spark/sql/execution/datasources/csv/CSVDataSource.scala#L184-L188
The PR proposes propagation of user's options to Text datasource on scheme inferring in similar way as user's options are converted to Hadoop parameters if schema is specified.
## How was this patch tested?
The changes were tested manually by using https://github.com/twitter/hadoop-lzo:
```
hadoop-lzo> mvn clean package
hadoop-lzo> ln -s ./target/hadoop-lzo-0.4.21-SNAPSHOT.jar ./hadoop-lzo.jar
```
Create 2 test files in JSON and CSV format and compress them:
```shell
$ cat test.csv
col1|col2
a|1
$ lzop test.csv
$ cat test.json
{"col1":"a","col2":1}
$ lzop test.json
```
Run `spark-shell` with hadoop-lzo:
```
bin/spark-shell --jars ~/hadoop-lzo/hadoop-lzo.jar
```
reading compressed CSV and JSON without schema:
```scala
spark.read.option("io.compression.codecs", "com.hadoop.compression.lzo.LzopCodec").option("inferSchema",true).option("header",true).option("sep","|").csv("test.csv.lzo").show()
+----+----+
|col1|col2|
+----+----+
| a| 1|
+----+----+
```
```scala
spark.read.option("io.compression.codecs", "com.hadoop.compression.lzo.LzopCodec").option("multiLine", true).json("test.json.lzo").printSchema
root
|-- col1: string (nullable = true)
|-- col2: long (nullable = true)
```
Author: Maxim Gekk <maxim.gekk@databricks.com>
Author: Maxim Gekk <max.gekk@gmail.com>
Closes#21182 from MaxGekk/text-options.
## What changes were proposed in this pull request?
This is to add a test case to check the behaviors when users write json in the specified UTF-16/UTF-32 encoding with multiline off.
## How was this patch tested?
N/A
Author: gatorsmile <gatorsmile@gmail.com>
Closes#21254 from gatorsmile/followupSPARK-23094.
## What changes were proposed in this pull request?
HashAggregate uses the same hash algorithm and seed as ShuffleExchange, it may lead to bad hash conflict when shuffle.partitions=8192*n.
Considering below example:
```
SET spark.sql.shuffle.partitions=8192;
INSERT OVERWRITE TABLE target_xxx
SELECT
item_id,
auct_end_dt
FROM
from source_xxx
GROUP BY
item_id,
auct_end_dt;
```
In the shuffle stage, if user sets the shuffle.partition = 8192, all tuples in the same partition will meet the following relationship:
```
hash(tuple x) = hash(tuple y) + n * 8192
```
Then in the next HashAggregate stage, all tuples from the same partition need be put into a 16K BytesToBytesMap (unsafeRowAggBuffer).
Here, the HashAggregate uses the same hash algorithm on the same expression as shuffle, and uses the same seed, and 16K = 8192 * 2, so actually, all tuples in the same parititon will only be hashed to 2 different places in the BytesToBytesMap. It is bad hash conflict. With BytesToBytesMap growing, the conflict will always exist.
Before change:
<img width="334" alt="hash_conflict" src="https://user-images.githubusercontent.com/2989575/39250210-ed032d46-48d2-11e8-855a-c1afc2a0ceb5.png">
After change:
<img width="334" alt="no_hash_conflict" src="https://user-images.githubusercontent.com/2989575/39250218-f1cb89e0-48d2-11e8-9244-5a93c1e8b60d.png">
## How was this patch tested?
Unit tests and production cases.
Author: yucai <yyu1@ebay.com>
Closes#21149 from yucai/SPARK-24076.
## What changes were proposed in this pull request?
Mention `spark.sql.crossJoin.enabled` in error message when an implicit `CROSS JOIN` is detected.
## How was this patch tested?
`CartesianProductSuite` and `JoinSuite`.
Author: Henry Robinson <henry@apache.org>
Closes#21201 from henryr/spark-24128.
## What changes were proposed in this pull request?
When creating an InterpretedPredicate instance, initialize any Nondeterministic expressions in the expression tree to avoid java.lang.IllegalArgumentException on later call to eval().
## How was this patch tested?
- sbt SQL tests
- python SQL tests
- new unit test
Author: Bruce Robbins <bersprockets@gmail.com>
Closes#21144 from bersprockets/interpretedpredicate.
## What changes were proposed in this pull request?
`LogicalPlan.resolve(...)` uses linear searches to find an attribute matching a name. This is fine in normal cases, but gets problematic when you try to resolve a large number of columns on a plan with a large number of attributes.
This PR adds an indexing structure to `resolve(...)` in order to find potential matches quicker. This PR improves the reference resolution time for the following code by 4x (11.8s -> 2.4s):
``` scala
val n = 4000
val values = (1 to n).map(_.toString).mkString(", ")
val columns = (1 to n).map("column" + _).mkString(", ")
val query =
s"""
|SELECT $columns
|FROM VALUES ($values) T($columns)
|WHERE 1=2 AND 1 IN ($columns)
|GROUP BY $columns
|ORDER BY $columns
|""".stripMargin
spark.time(sql(query))
```
## How was this patch tested?
Existing tests.
Author: Herman van Hovell <hvanhovell@databricks.com>
Closes#14083 from hvanhovell/SPARK-16406.
## What changes were proposed in this pull request?
The PR add the `slice` function. The behavior of the function is based on Presto's one.
The function slices an array according to the requested start index and length.
## How was this patch tested?
added UTs
Author: Marco Gaido <marcogaido91@gmail.com>
Closes#21040 from mgaido91/SPARK-23930.
## What changes were proposed in this pull request?
DataFrameRangeSuite.test("Cancelling stage in a query with Range.") stays sometimes in an infinite loop and times out the build.
There were multiple issues with the test:
1. The first valid stageId is zero when the test started alone and not in a suite and the following code waits until timeout:
```
eventually(timeout(10.seconds), interval(1.millis)) {
assert(DataFrameRangeSuite.stageToKill > 0)
}
```
2. The `DataFrameRangeSuite.stageToKill` was overwritten by the task's thread after the reset which ended up in canceling the same stage 2 times. This caused the infinite wait.
This PR solves this mentioned flakyness by removing the shared `DataFrameRangeSuite.stageToKill` and using `onTaskStart` where stage ID is provided. In order to make sure cancelStage called for all stages `waitUntilEmpty` is called on `ListenerBus`.
In [PR20888](https://github.com/apache/spark/pull/20888) this tried to get solved by:
* Stopping the executor thread with `wait`
* Wait for all `cancelStage` called
* Kill the executor thread by setting `SparkContext.SPARK_JOB_INTERRUPT_ON_CANCEL`
but the thread killing left the shared `SparkContext` sometimes in a state where further jobs can't be submitted. As a result DataFrameRangeSuite.test("Cancelling stage in a query with Range.") test passed properly but the next test inside the suite was hanging.
## How was this patch tested?
Existing unit test executed 10k times.
Author: Gabor Somogyi <gabor.g.somogyi@gmail.com>
Closes#21214 from gaborgsomogyi/SPARK-23775_1.
## What changes were proposed in this pull request?
The PR adds the SQL function `array_sort`. The behavior of the function is based on Presto's one.
The function sorts the input array in ascending order. The elements of the input array must be orderable. Null elements will be placed at the end of the returned array.
## How was this patch tested?
Added UTs
Author: Kazuaki Ishizaki <ishizaki@jp.ibm.com>
Closes#21021 from kiszk/SPARK-23921.
## What changes were proposed in this pull request?
This refactors the external catalog to be an interface. It can be easier for the future work in the catalog federation. After the refactoring, `ExternalCatalog` is much cleaner without mixing the listener event generation logic.
## How was this patch tested?
The existing tests
Author: gatorsmile <gatorsmile@gmail.com>
Closes#21122 from gatorsmile/refactorExternalCatalog.
## What changes were proposed in this pull request?
This PR enables the MicroBatchExecution to run no-data batches if some SparkPlan requires running another batch to output results based on updated watermark / processing time. In this PR, I have enabled streaming aggregations and streaming deduplicates to automatically run addition batch even if new data is available. See https://issues.apache.org/jira/browse/SPARK-24156 for more context.
Major changes/refactoring done in this PR.
- Refactoring MicroBatchExecution - A major point of confusion in MicroBatchExecution control flow was always (at least to me) was that `populateStartOffsets` internally called `constructNextBatch` which was not obvious from just the name "populateStartOffsets" and made the control flow from the main trigger execution loop very confusing (main loop in `runActivatedStream` called `constructNextBatch` but only if `populateStartOffsets` hadn't already called it). Instead, the refactoring makes it cleaner.
- `populateStartOffsets` only the updates `availableOffsets` and `committedOffsets`. Does not call `constructNextBatch`.
- Main loop in `runActivatedStream` calls `constructNextBatch` which returns true or false reflecting whether the next batch is ready for executing. This method is now idempotent; if a batch has already been constructed, then it will always return true until the batch has been executed.
- If next batch is ready then we call `runBatch` or sleep.
- That's it.
- Refactoring watermark management logic - This has been refactored out from `MicroBatchExecution` in a separate class to simplify `MicroBatchExecution`.
- New method `shouldRunAnotherBatch` in `IncrementalExecution` - This returns true if there is any stateful operation in the last execution plan that requires another batch for state cleanup, etc. This is used to decide whether to construct a batch or not in `constructNextBatch`.
- Changes to stream testing framework - Many tests used CheckLastBatch to validate answers. This assumed that there will be no more batches after the last set of input has been processed, so the last batch is the one that has output corresponding to the last input. This is not true anymore. To account for that, I made two changes.
- `CheckNewAnswer` is a new test action that verifies the new rows generated since the last time the answer was checked by `CheckAnswer`, `CheckNewAnswer` or `CheckLastBatch`. This is agnostic to how many batches occurred between the last check and now. To do make this easier, I added a common trait between MemorySink and MemorySinkV2 to abstract out some common methods.
- `assertNumStateRows` has been updated in the same way to be agnostic to batches while checking what the total rows and how many state rows were updated (sums up updates since the last check).
## How was this patch tested?
- Changes made to existing tests - Tests have been changed in one of the following patterns.
- Tests where the last input was given again to force another batch to be executed and state cleaned up / output generated, they were simplified by removing the extra input.
- Tests using aggregation+watermark where CheckLastBatch were replaced with CheckNewAnswer to make them batch agnostic.
- New tests added to check whether the flag works for streaming aggregation and deduplication
Author: Tathagata Das <tathagata.das1565@gmail.com>
Closes#21220 from tdas/SPARK-24157.
## What changes were proposed in this pull request?
Do continuous processing writes with multiple compute() calls.
The current strategy (before this PR) is hacky; we just call next() on an iterator which has already returned hasNext = false, knowing that all the nodes we whitelist handle this properly. This will have to be changed before we can support more complex query plans. (In particular, I have a WIP https://github.com/jose-torres/spark/pull/13 which should be able to support aggregates in a single partition with minimal additional work.)
Most of the changes here are just refactoring to accommodate the new model. The behavioral changes are:
* The writer now calls prev.compute(split, context) once per epoch within the epoch loop.
* ContinuousDataSourceRDD now spawns a ContinuousQueuedDataReader which is shared across multiple calls to compute() for the same partition.
## How was this patch tested?
existing unit tests
Author: Jose Torres <torres.joseph.f+github@gmail.com>
Closes#21200 from jose-torres/noAggr.
## What changes were proposed in this pull request?
Avoid unnecessary sleep (10 ms) in each invocation of MemoryStreamDataReader.next.
## How was this patch tested?
Ran ContinuousSuite from IDE.
Please review http://spark.apache.org/contributing.html before opening a pull request.
Author: Arun Mahadevan <arunm@apache.org>
Closes#21207 from arunmahadevan/memorystream.
## What changes were proposed in this pull request?
This PR is extracted from #21190 , to make it easier to backport.
`ParquetFilters` is used in the file scan function, which is executed in executor side, so we can't call `conf.parquetFilterPushDownDate` there.
## How was this patch tested?
it's tested in #21190
Author: Wenchen Fan <wenchen@databricks.com>
Closes#21224 from cloud-fan/minor2.
## What changes were proposed in this pull request?
This PR is extracted from #21190 , to make it easier to backport.
`WindowExec#createBoundOrdering` is called on executor side, so we can't use `conf.sessionLocalTimezone` there.
## How was this patch tested?
tested in #21190
Author: Wenchen Fan <wenchen@databricks.com>
Closes#21225 from cloud-fan/minor3.
## What changes were proposed in this pull request?
Add SQL support for Pivot according to Pivot grammar defined by Oracle (https://docs.oracle.com/database/121/SQLRF/img_text/pivot_clause.htm) with some simplifications, based on our existing functionality and limitations for Pivot at the backend:
1. For pivot_for_clause (https://docs.oracle.com/database/121/SQLRF/img_text/pivot_for_clause.htm), the column list form is not supported, which means the pivot column can only be one single column.
2. For pivot_in_clause (https://docs.oracle.com/database/121/SQLRF/img_text/pivot_in_clause.htm), the sub-query form and "ANY" is not supported (this is only supported by Oracle for XML anyway).
3. For pivot_in_clause, aliases for the constant values are not supported.
The code changes are:
1. Add parser support for Pivot. Note that according to https://docs.oracle.com/database/121/SQLRF/statements_10002.htm#i2076542, Pivot cannot be used together with lateral views in the from clause. This restriction has been implemented in the Parser rule.
2. Infer group-by expressions: group-by expressions are not explicitly specified in SQL Pivot clause and need to be deduced based on this rule: https://docs.oracle.com/database/121/SQLRF/statements_10002.htm#CHDFAFIE, so we have to post-fix it at query analysis stage.
3. Override Pivot.resolved as "false": for the reason mentioned in [2] and the fact that output attributes change after Pivot being replaced by Project or Aggregate, we avoid resolving parent references until after Pivot has been resolved and replaced.
4. Verify aggregate expressions: only aggregate expressions with or without aliases can appear in the first part of the Pivot clause, and this check is performed as analysis stage.
## How was this patch tested?
A new test suite PivotSuite is added.
Author: maryannxue <maryann.xue@gmail.com>
Closes#21187 from maryannxue/spark-24035.
## What changes were proposed in this pull request?
This PR is extracted from #21190 , to make it easier to backport.
`JsonToStructs` can be serialized to executors and evaluate, we should not call `SQLConf.get.getConf(SQLConf.FROM_JSON_FORCE_NULLABLE_SCHEMA)` in the body.
## How was this patch tested?
tested in #21190
Author: Wenchen Fan <wenchen@databricks.com>
Closes#21226 from cloud-fan/minor4.
## What changes were proposed in this pull request?
This PR is extracted from https://github.com/apache/spark/pull/21190 , to make it easier to backport.
`InMemoryTableScanExec#createAndDecompressColumn` is executed inside `rdd.map`, we can't access `conf.offHeapColumnVectorEnabled` there.
## How was this patch tested?
it's tested in #21190
Author: Wenchen Fan <wenchen@databricks.com>
Closes#21223 from cloud-fan/minor1.
## What changes were proposed in this pull request?
`from_utc_timestamp` assumes its input is in UTC timezone and shifts it to the specified timezone. When the timestamp contains timezone(e.g. `2018-03-13T06:18:23+00:00`), Spark breaks the semantic and respect the timezone in the string. This is not what user expects and the result is different from Hive/Impala. `to_utc_timestamp` has the same problem.
More details please refer to the JIRA ticket.
This PR fixes this by returning null if the input timestamp contains timezone.
## How was this patch tested?
new tests
Author: Wenchen Fan <wenchen@databricks.com>
Closes#21169 from cloud-fan/from_utc_timezone.
## What changes were proposed in this pull request?
Although [SPARK-22654](https://issues.apache.org/jira/browse/SPARK-22654) made `HiveExternalCatalogVersionsSuite` download from Apache mirrors three times, it has been flaky because it didn't verify the downloaded file. Some Apache mirrors terminate the downloading abnormally, the *corrupted* file shows the following errors.
```
gzip: stdin: not in gzip format
tar: Child returned status 1
tar: Error is not recoverable: exiting now
22:46:32.700 WARN org.apache.spark.sql.hive.HiveExternalCatalogVersionsSuite:
===== POSSIBLE THREAD LEAK IN SUITE o.a.s.sql.hive.HiveExternalCatalogVersionsSuite, thread names: Keep-Alive-Timer =====
*** RUN ABORTED ***
java.io.IOException: Cannot run program "./bin/spark-submit" (in directory "/tmp/test-spark/spark-2.2.0"): error=2, No such file or directory
```
This has been reported weirdly in two ways. For example, the above case is reported as Case 2 `no failures`.
- Case 1. [Test Result (1 failure / +1)](https://amplab.cs.berkeley.edu/jenkins/view/Spark%20QA%20Test%20(Dashboard)/job/spark-master-test-sbt-hadoop-2.7/4389/)
- Case 2. [Test Result (no failures)](https://amplab.cs.berkeley.edu/jenkins/view/Spark%20QA%20Test%20(Dashboard)/job/spark-master-test-maven-hadoop-2.6/4811/)
This PR aims to make `HiveExternalCatalogVersionsSuite` more robust by verifying the downloaded `tgz` file by extracting and checking the existence of `bin/spark-submit`. If it turns out that the file is empty or corrupted, `HiveExternalCatalogVersionsSuite` will do retry logic like the download failure.
## How was this patch tested?
Pass the Jenkins.
Author: Dongjoon Hyun <dongjoon@apache.org>
Closes#21210 from dongjoon-hyun/SPARK-23489.
## What changes were proposed in this pull request?
Spark ThriftServer will call UGI.loginUserFromKeytab twice in initialization. This is unnecessary and will cause various potential problems, like Hadoop IPC failure after 7 days, or RM failover issue and so on.
So here we need to remove all the unnecessary login logics and make sure UGI in the context never be created again.
Note this is actually a HS2 issue, If later on we upgrade supported Hive version, the issue may already be fixed in Hive side.
## How was this patch tested?
Local verification in secure cluster.
Author: jerryshao <sshao@hortonworks.com>
Closes#21178 from jerryshao/SPARK-24110.
## What changes were proposed in this pull request?
This pr added the TPCDS v2.7 (latest) queries in `TPCDSQueryBenchmark`.
These query files have been added in `SPARK-23167`.
## How was this patch tested?
Manually checked.
Author: Takeshi Yamamuro <yamamuro@apache.org>
Closes#21177 from maropu/AddTpcdsV2_7InBenchmark.
## What changes were proposed in this pull request?
The PR adds the SQL function `cardinality`. The behavior of the function is based on Presto's one.
The function returns the length of the array or map stored in the column as `int` while the Presto version returns the value as `BigInt` (`long` in Spark). The discussions regarding the difference of return type are [here](https://github.com/apache/spark/pull/21031#issuecomment-381284638) and [there](https://github.com/apache/spark/pull/21031#discussion_r181622107).
## How was this patch tested?
Added UTs
Author: Kazuaki Ishizaki <ishizaki@jp.ibm.com>
Closes#21031 from kiszk/SPARK-23923.
## What changes were proposed in this pull request?
SPARK-23902 introduced the ability to retrieve more than 8 digits in `monthsBetween`. Unfortunately, current implementation can cause precision loss in such a case. This was causing also a flaky UT.
This PR mirrors Hive's implementation in order to avoid precision loss also when more than 8 digits are returned.
## How was this patch tested?
running 10000000 times the flaky UT
Author: Marco Gaido <marcogaido91@gmail.com>
Closes#21196 from mgaido91/SPARK-24123.
## What changes were proposed in this pull request?
`ColumnVector`s store string data in one big byte array. Since the array size is capped at just under Integer.MAX_VALUE, a single `ColumnVector` cannot store more than 2GB of string data.
But since the Parquet files commonly contain large blobs stored as strings, and `ColumnVector`s by default carry 4096 values, it's entirely possible to go past that limit. In such cases a negative capacity is requested from `WritableColumnVector.reserve()`. The call succeeds (requested capacity is smaller than already allocated capacity), and consequently `java.lang.ArrayIndexOutOfBoundsException` is thrown when the reader actually attempts to put the data into the array.
This change introduces a simple check for integer overflow to `WritableColumnVector.reserve()` which should help catch the error earlier and provide more informative exception. Additionally, the error message in `WritableColumnVector.throwUnsupportedException()` was corrected, as it previously encouraged users to increase rather than reduce the batch size.
## How was this patch tested?
New units tests were added.
Author: Ala Luszczak <ala@databricks.com>
Closes#21206 from ala/overflow-reserve.
## What changes were proposed in this pull request?
`ApproximatePercentile` contains a workaround logic to compress the samples since at the beginning `QuantileSummaries` was ignoring the compression threshold. This problem was fixed in SPARK-17439, but the workaround logic was not removed. So we are compressing the samples many more times than needed: this could lead to critical performance degradation.
This can create serious performance issues in queries like:
```
select approx_percentile(id, array(0.1)) from range(10000000)
```
## How was this patch tested?
added UT
Author: Marco Gaido <marcogaido91@gmail.com>
Closes#21133 from mgaido91/SPARK-24013.
## What changes were proposed in this pull request?
Add TypedFilter support for continuous processing application.
## How was this patch tested?
unit tests
Author: wangyanlin01 <wangyanlin01@baidu.com>
Closes#21136 from yanlin-Lynn/SPARK-24061.
## What changes were proposed in this pull request?
filters like parquet row group filter, which is actually pushed to the data source but still to be evaluated by Spark, should also count as `pushedFilters`.
## How was this patch tested?
existing tests
Author: Wenchen Fan <wenchen@databricks.com>
Closes#21143 from cloud-fan/step1.
## What changes were proposed in this pull request?
I propose to support the `samplingRatio` option for schema inferring of CSV datasource similar to the same option of JSON datasource:
b14993e1fc/sql/catalyst/src/main/scala/org/apache/spark/sql/catalyst/json/JSONOptions.scala (L49-L50)
## How was this patch tested?
Added 2 tests for json and 2 tests for csv datasources. The tests checks that only subset of input dataset is used for schema inferring.
Author: Maxim Gekk <maxim.gekk@databricks.com>
Author: Maxim Gekk <max.gekk@gmail.com>
Closes#20959 from MaxGekk/csv-sampling.
## What changes were proposed in this pull request?
I propose new option for JSON datasource which allows to specify encoding (charset) of input and output files. Here is an example of using of the option:
```
spark.read.schema(schema)
.option("multiline", "true")
.option("encoding", "UTF-16LE")
.json(fileName)
```
If the option is not specified, charset auto-detection mechanism is used by default.
The option can be used for saving datasets to jsons. Currently Spark is able to save datasets into json files in `UTF-8` charset only. The changes allow to save data in any supported charset. Here is the approximate list of supported charsets by Oracle Java SE: https://docs.oracle.com/javase/8/docs/technotes/guides/intl/encoding.doc.html . An user can specify the charset of output jsons via the charset option like `.option("charset", "UTF-16BE")`. By default the output charset is still `UTF-8` to keep backward compatibility.
The solution has the following restrictions for per-line mode (`multiline = false`):
- If charset is different from UTF-8, the lineSep option must be specified. The option required because Hadoop LineReader cannot detect the line separator correctly. Here is the ticket for solving the issue: https://issues.apache.org/jira/browse/SPARK-23725
- Encoding with [BOM](https://en.wikipedia.org/wiki/Byte_order_mark) are not supported. For example, the `UTF-16` and `UTF-32` encodings are blacklisted. The problem can be solved by https://github.com/MaxGekk/spark-1/pull/2
## How was this patch tested?
I added the following tests:
- reads an json file in `UTF-16LE` encoding with BOM in `multiline` mode
- read json file by using charset auto detection (`UTF-32BE` with BOM)
- read json file using of user's charset (`UTF-16LE`)
- saving in `UTF-32BE` and read the result by standard library (not by Spark)
- checking that default charset is `UTF-8`
- handling wrong (unsupported) charset
Author: Maxim Gekk <maxim.gekk@databricks.com>
Author: Maxim Gekk <max.gekk@gmail.com>
Closes#20937 from MaxGekk/json-encoding-line-sep.
## What changes were proposed in this pull request?
Fix `MemorySinkV2` toString() error
## How was this patch tested?
N/A
Author: Yuming Wang <yumwang@ebay.com>
Closes#21170 from wangyum/SPARK-22732.
## What changes were proposed in this pull request?
In the error messages we should return the SQL types (like `string` rather than the internal types like `StringType`).
## How was this patch tested?
added UT
Author: Marco Gaido <marcogaido91@gmail.com>
Closes#21181 from mgaido91/SPARK-23736_followup.
## What changes were proposed in this pull request?
Replace rate source with memory source in continuous mode test suite. Keep using "rate" source if the tests intend to put data periodically in background, or need to put short source name to load, since "memory" doesn't have provider for source.
## How was this patch tested?
Ran relevant test suite from IDE.
Author: Jungtaek Lim <kabhwan@gmail.com>
Closes#21152 from HeartSaVioR/SPARK-23688.
## What changes were proposed in this pull request?
Event `SparkListenerDriverAccumUpdates` may happen multiple times in a query - e.g. every `FileSourceScanExec` and `BroadcastExchangeExec` call `postDriverMetricUpdates`.
In Spark 2.2 `SQLListener` updated the map with new values. `SQLAppStatusListener` overwrites it.
Unless `update` preserved it in the KV store (dependant on `exec.lastWriteTime`), only the metrics from the last operator that does `postDriverMetricUpdates` are preserved.
## How was this patch tested?
Unit test added.
Author: Juliusz Sompolski <julek@databricks.com>
Closes#21171 from juliuszsompolski/SPARK-24104.
## What changes were proposed in this pull request?
In this case, the partition pruning happens before the planning phase of scalar subquery expressions.
For scalar subquery expressions, the planning occurs late in the cycle (after the physical planning) in "PlanSubqueries" just before execution. Currently we try to execute the scalar subquery expression as part of partition pruning and fail as it implements Unevaluable.
The fix attempts to ignore the Subquery expressions from partition pruning computation. Another option can be to somehow plan the subqueries before the partition pruning. Since this may not be a commonly occuring expression, i am opting for a simpler fix.
Repro
``` SQL
CREATE TABLE test_prc_bug (
id_value string
)
partitioned by (id_type string)
location '/tmp/test_prc_bug'
stored as parquet;
insert into test_prc_bug values ('1','a');
insert into test_prc_bug values ('2','a');
insert into test_prc_bug values ('3','b');
insert into test_prc_bug values ('4','b');
select * from test_prc_bug
where id_type = (select 'b');
```
## How was this patch tested?
Added test in SubquerySuite and hive/SQLQuerySuite
Author: Dilip Biswal <dbiswal@us.ibm.com>
Closes#21174 from dilipbiswal/spark-24085.
## What changes were proposed in this pull request?
A more informative message to tell you why a structured streaming query cannot continue if you have added more sources, than there are in the existing checkpoint offsets.
## How was this patch tested?
I added a Unit Test.
Author: Patrick McGloin <mcgloin.patrick@gmail.com>
Closes#20946 from patrickmcgloin/master.
## What changes were proposed in this pull request?
Previously, SPARK-22158 fixed for `USING hive` syntax. This PR aims to fix for `STORED AS` syntax. Although the test case covers ORC part, the patch considers both `convertMetastoreOrc` and `convertMetastoreParquet`.
## How was this patch tested?
Pass newly added test cases.
Author: Dongjoon Hyun <dongjoon@apache.org>
Closes#20522 from dongjoon-hyun/SPARK-22158-2.
## What changes were proposed in this pull request?
`colStat.min` AND `colStat.max` are empty for string type. Thus, `evaluateInSet` should not return zero when either `colStat.min` or `colStat.max`.
## How was this patch tested?
Added a test case.
Author: gatorsmile <gatorsmile@gmail.com>
Closes#21147 from gatorsmile/cached.
## What changes were proposed in this pull request?
This makes it easy to understand at runtime which version is running. Great for debugging production issues.
## How was this patch tested?
Not necessary.
Author: Tathagata Das <tathagata.das1565@gmail.com>
Closes#21160 from tdas/SPARK-24094.
## What changes were proposed in this pull request?
The PR adds the SQL function `array_join`. The behavior of the function is based on Presto's one.
The function accepts an `array` of `string` which is to be joined, a `string` which is the delimiter to use between the items of the first argument and optionally a `string` which is used to replace `null` values.
## How was this patch tested?
added UTs
Author: Marco Gaido <marcogaido91@gmail.com>
Closes#21011 from mgaido91/SPARK-23916.
## What changes were proposed in this pull request?
HIVE-15511 introduced the `roundOff` flag in order to disable the rounding to 8 digits which is performed in `months_between`. Since this can be a computational intensive operation, skipping it may improve performances when the rounding is not needed.
## How was this patch tested?
modified existing UT
Author: Marco Gaido <marcogaido91@gmail.com>
Closes#21008 from mgaido91/SPARK-23902.
## What changes were proposed in this pull request?
Added the `samplingRatio` option to the `json()` method of PySpark DataFrame Reader. Improving existing tests for Scala API according to review of the PR: https://github.com/apache/spark/pull/20959
## How was this patch tested?
Added new test for PySpark, updated 2 existing tests according to reviews of https://github.com/apache/spark/pull/20959 and added new negative test
Author: Maxim Gekk <maxim.gekk@databricks.com>
Closes#21056 from MaxGekk/json-sampling.
## What changes were proposed in this pull request?
a followup of https://github.com/apache/spark/pull/21100
## How was this patch tested?
N/A
Author: Wenchen Fan <wenchen@databricks.com>
Closes#21154 from cloud-fan/test.
## What changes were proposed in this pull request?
In some streaming queries, the input and processing rates are not calculated at all (shows up as zero) because MicroBatchExecution fails to associated metrics from the executed plan of a trigger with the sources in the logical plan of the trigger. The way this executed-plan-leaf-to-logical-source attribution works is as follows. With V1 sources, there was no way to identify which execution plan leaves were generated by a streaming source. So did a best-effort attempt to match logical and execution plan leaves when the number of leaves were same. In cases where the number of leaves is different, we just give up and report zero rates. An example where this may happen is as follows.
```
val cachedStaticDF = someStaticDF.union(anotherStaticDF).cache()
val streamingInputDF = ...
val query = streamingInputDF.join(cachedStaticDF).writeStream....
```
In this case, the `cachedStaticDF` has multiple logical leaves, but in the trigger's execution plan it only has leaf because a cached subplan is represented as a single InMemoryTableScanExec leaf. This leads to a mismatch in the number of leaves causing the input rates to be computed as zero.
With DataSourceV2, all inputs are represented in the executed plan using `DataSourceV2ScanExec`, each of which has a reference to the associated logical `DataSource` and `DataSourceReader`. So its easy to associate the metrics to the original streaming sources.
In this PR, the solution is as follows. If all the streaming sources in a streaming query as v2 sources, then use a new code path where the execution-metrics-to-source mapping is done directly. Otherwise we fall back to existing mapping logic.
## How was this patch tested?
- New unit tests using V2 memory source
- Existing unit tests using V1 source
Author: Tathagata Das <tathagata.das1565@gmail.com>
Closes#21126 from tdas/SPARK-24050.
## What changes were proposed in this pull request?
This pr fixed code so that `cache` could prevent any jobs from being triggered.
For example, in the current master, an operation below triggers a actual job;
```
val df = spark.range(10000000000L)
.filter('id > 1000)
.orderBy('id.desc)
.cache()
```
This triggers a job while the cache should be lazy. The problem is that, when creating `InMemoryRelation`, we build the RDD, which calls `SparkPlan.execute` and may trigger jobs, like sampling job for range partitioner, or broadcast job.
This pr removed the code to build a cached `RDD` in the constructor of `InMemoryRelation` and added `CachedRDDBuilder` to lazily build the `RDD` in `InMemoryRelation`. Then, the first call of `CachedRDDBuilder.cachedColumnBuffers` triggers a job to materialize the cache in `InMemoryTableScanExec` .
## How was this patch tested?
Added tests in `CachedTableSuite`.
Author: Takeshi Yamamuro <yamamuro@apache.org>
Closes#21018 from maropu/SPARK-23880.
## What changes were proposed in this pull request?
Union of map and other compatible column result in unresolved operator 'Union; exception
Reproduction
`spark-sql>select map(1,2), 'str' union all select map(1,2,3,null), 1`
Output:
```
Error in query: unresolved operator 'Union;;
'Union
:- Project [map(1, 2) AS map(1, 2)#106, str AS str#107]
: +- OneRowRelation$
+- Project [map(1, cast(2 as int), 3, cast(null as int)) AS map(1, CAST(2 AS INT), 3, CAST(NULL AS INT))#109, 1 AS 1#108]
+- OneRowRelation$
```
So, we should cast part of columns to be compatible when appropriate.
## How was this patch tested?
Added a test (query union of map and other columns) to SQLQueryTestSuite's union.sql.
Author: liutang123 <liutang123@yeah.net>
Closes#21100 from liutang123/SPARK-24012.
## What changes were proposed in this pull request?
Refactor continuous writing to its own class.
See WIP https://github.com/jose-torres/spark/pull/13 for the overall direction this is going, but I think this PR is very isolated and necessary anyway.
## How was this patch tested?
existing unit tests - refactoring only
Author: Jose Torres <torres.joseph.f+github@gmail.com>
Closes#21116 from jose-torres/SPARK-24038.
## What changes were proposed in this pull request?
This pr is a follow-up of #20980 and fixes code to reuse `InternalRow` for converting input keys/values in `ExternalMapToCatalyst` eval.
## How was this patch tested?
Existing tests.
Author: Takeshi Yamamuro <yamamuro@apache.org>
Closes#21137 from maropu/SPARK-23589-FOLLOWUP.
## What changes were proposed in this pull request?
In SPARK-23375 we introduced the ability of removing `Sort` operation during query optimization if the data is already sorted. In this follow-up we remove also a `Sort` which is followed by another `Sort`: in this case the first sort is not needed and can be safely removed.
The PR starts from henryr's comment: https://github.com/apache/spark/pull/20560#discussion_r180601594. So credit should be given to him.
## How was this patch tested?
added UT
Author: Marco Gaido <marcogaido91@gmail.com>
Closes#21072 from mgaido91/SPARK-23973.
## What changes were proposed in this pull request?
A structured streaming query with a streaming aggregation can throw the following error in rare cases.
```
java.lang.IllegalStateException: Cannot commit after already committed or aborted
at org.apache.spark.sql.execution.streaming.state.HDFSBackedStateStoreProvider.org$apache$spark$sql$execution$streaming$state$HDFSBackedStateStoreProvider$$verify(HDFSBackedStateStoreProvider.scala:643)
at org.apache.spark.sql.execution.streaming.state.HDFSBackedStateStoreProvider$HDFSBackedStateStore.commit(HDFSBackedStateStoreProvider.scala:135)
at org.apache.spark.sql.execution.streaming.StateStoreSaveExec$$anonfun$doExecute$3$$anon$2$$anonfun$hasNext$2.apply$mcV$sp(statefulOperators.scala:359)
at org.apache.spark.sql.execution.streaming.StateStoreWriter$class.timeTakenMs(statefulOperators.scala:102)
at org.apache.spark.sql.execution.streaming.StateStoreSaveExec.timeTakenMs(statefulOperators.scala:251)
at org.apache.spark.sql.execution.streaming.StateStoreSaveExec$$anonfun$doExecute$3$$anon$2.hasNext(statefulOperators.scala:359)
at org.apache.spark.sql.execution.aggregate.ObjectAggregationIterator.processInputs(ObjectAggregationIterator.scala:188)
at org.apache.spark.sql.execution.aggregate.ObjectAggregationIterator.<init>(ObjectAggregationIterator.scala:78)
at org.apache.spark.sql.execution.aggregate.ObjectHashAggregateExec$$anonfun$doExecute$1$$anonfun$2.apply(ObjectHashAggregateExec.scala:114)
at org.apache.spark.sql.execution.aggregate.ObjectHashAggregateExec$$anonfun$doExecute$1$$anonfun$2.apply(ObjectHashAggregateExec.scala:105)
at org.apache.spark.rdd.RDD$$anonfun$mapPartitionsWithIndexInternal$1$$anonfun$apply$24.apply(RDD.scala:830)
at org.apache.spark.rdd.RDD$$anonfun$mapPartitionsWithIndexInternal$1$$anonfun$apply$24.apply(RDD.scala:830)
at org.apache.spark.rdd.MapPartitionsRDD.compute(MapPartitionsRDD.scala:42)
at org.apache.spark.rdd.RDD.computeOrReadCheckpoint(RDD.scala:336)
```
This can happen when the following conditions are accidentally hit.
- Streaming aggregation with aggregation function that is a subset of [`TypedImperativeAggregation`](76b8b840dd/sql/catalyst/src/main/scala/org/apache/spark/sql/catalyst/expressions/aggregate/interfaces.scala (L473)) (for example, `collect_set`, `collect_list`, `percentile`, etc.).
- Query running in `update}` mode
- After the shuffle, a partition has exactly 128 records.
This causes StateStore.commit to be called twice. See the [JIRA](https://issues.apache.org/jira/browse/SPARK-23004) for a more detailed explanation. The solution is to use `NextIterator` or `CompletionIterator`, each of which has a flag to prevent the "onCompletion" task from being called more than once. In this PR, I chose to implement using `NextIterator`.
## How was this patch tested?
Added unit test that I have confirm will fail without the fix.
Author: Tathagata Das <tathagata.das1565@gmail.com>
Closes#21124 from tdas/SPARK-23004.
## What changes were proposed in this pull request?
This pr supported interpreted mode for `ExternalMapToCatalyst`.
## How was this patch tested?
Added tests in `ObjectExpressionsSuite`.
Author: Takeshi Yamamuro <yamamuro@apache.org>
Closes#20980 from maropu/SPARK-23589.
## What changes were proposed in this pull request?
The existing query constraints framework has 2 steps:
1. propagate constraints bottom up.
2. use constraints to infer additional filters for better data pruning.
For step 2, it mostly helps with Join, because we can connect the constraints from children to the join condition and infer powerful filters to prune the data of the join sides. e.g., the left side has constraints `a = 1`, the join condition is `left.a = right.a`, then we can infer `right.a = 1` to the right side and prune the right side a lot.
However, the current logic of inferring filters from constraints for Join is pretty weak. It infers the filters from Join's constraints. Some joins like left semi/anti exclude output from right side and the right side constraints will be lost here.
This PR propose to check the left and right constraints individually, expand the constraints with join condition and add filters to children of join directly, instead of adding to the join condition.
This reverts https://github.com/apache/spark/pull/20670 , covers https://github.com/apache/spark/pull/20717 and https://github.com/apache/spark/pull/20816
This is inspired by the original PRs and the tests are all from these PRs. Thanks to the authors mgaido91 maryannxue KaiXinXiaoLei !
## How was this patch tested?
new tests
Author: Wenchen Fan <wenchen@databricks.com>
Closes#21083 from cloud-fan/join.
## What changes were proposed in this pull request?
A followup of https://github.com/apache/spark/pull/20988
`PhysicalOperation` can collect Project and Filters over a certain plan and substitute the alias with the original attributes in the bottom plan. We can use it in `OptimizeMetadataOnlyQuery` rule to handle the Project and Filter over partitioned relation.
## How was this patch tested?
existing test
Author: Wenchen Fan <wenchen@databricks.com>
Closes#21111 from cloud-fan/refactor.
>What changes were proposed in this pull request?
During evaluation of IN conditions, if the source data frame, is represented by a plan, that uses hive table with columns, which were previously analysed, and the plan has conditions for these fields, that cannot be satisfied (which leads us to an empty data frame), FilterEstimation.evaluateInSet method produces NumberFormatException and ClassCastException.
In order to fix this bug, method FilterEstimation.evaluateInSet at first checks, if distinct count is not zero, and also checks if colStat.min and colStat.max are defined, and only in this case proceeds with the calculation. If at least one of the conditions is not satisfied, zero is returned.
>How was this patch tested?
In order to test the PR two tests were implemented: one in FilterEstimationSuite, that tests the plan with the statistics that violates the conditions mentioned above, and another one in StatisticsCollectionSuite, that test the whole process of analysis/optimisation of the query, that leads to the problems, mentioned in the first section.
Author: Mykhailo Shtelma <mykhailo.shtelma@bearingpoint.com>
Author: smikesh <mshtelma@gmail.com>
Closes#21052 from mshtelma/filter_estimation_evaluateInSet_Bugs.
## What changes were proposed in this pull request?
When the OffsetWindowFunction's frame is `UnaryMinus(Literal(1))` but the specified window frame has been simplified to `Literal(-1)` by some optimizer rules e.g., `ConstantFolding`. Thus, they do not match and cause the following error:
```
org.apache.spark.sql.AnalysisException: Window Frame specifiedwindowframe(RowFrame, -1, -1) must match the required frame specifiedwindowframe(RowFrame, -1, -1);
at org.apache.spark.sql.catalyst.analysis.CheckAnalysis$class.failAnalysis(CheckAnalysis.scala:41)
at org.apache.spark.sql.catalyst.analysis.Analyzer.failAnalysis(Analyzer.scala:91)
at
```
## How was this patch tested?
Added a test
Author: gatorsmile <gatorsmile@gmail.com>
Closes#21115 from gatorsmile/fixLag.
## What changes were proposed in this pull request?
This pr supported interpreted mode for `ValidateExternalType`.
## How was this patch tested?
Added tests in `ObjectExpressionsSuite`.
Author: Takeshi Yamamuro <yamamuro@apache.org>
Closes#20757 from maropu/SPARK-23595.
## What changes were proposed in this pull request?
This pr is a follow-up pr of #20979 and fixes code to resolve a map builder method per execution instead of per row in `CatalystToExternalMap`.
## How was this patch tested?
Existing tests.
Author: Takeshi Yamamuro <yamamuro@apache.org>
Closes#21112 from maropu/SPARK-23588-FOLLOWUP.
## What changes were proposed in this pull request?
This updates the OptimizeMetadataOnlyQuery rule to use filter expressions when listing partitions, if there are filter nodes in the logical plan. This avoids listing all partitions for large tables on the driver.
This also fixes a minor bug where the partitions returned from fsRelation cannot be serialized without hitting a stack level too deep error. This is caused by serializing a stream to executors, where the stream is a recursive structure. If the stream is too long, the serialization stack reaches the maximum level of depth. The fix is to create a LocalRelation using an Array instead of the incoming Seq.
## How was this patch tested?
Existing tests for metadata-only queries.
Author: Ryan Blue <blue@apache.org>
Closes#20988 from rdblue/SPARK-23877-metadata-only-push-filters.
## What changes were proposed in this pull request?
Improving the test coverage of window functions focusing on missing test for window aggregate functions. No new UDAF test is added as it has been tested already.
## How was this patch tested?
Only new tests were added, automated tests were executed.
Author: “attilapiros” <piros.attila.zsolt@gmail.com>
Author: Attila Zsolt Piros <2017933+attilapiros@users.noreply.github.com>
Closes#20046 from attilapiros/SPARK-22362.
## What changes were proposed in this pull request?
In Spark SQL, we usually reuse the `UnsafeRow` instance and need to copy the data when a place buffers non-serialized objects.
Shuffle may buffer objects if we don't make it to the bypass merge shuffle or unsafe shuffle.
`ShuffleExchangeExec.needToCopyObjectsBeforeShuffle` misses the case that, if `spark.sql.shuffle.partitions` is large enough, we could fail to run unsafe shuffle and go with the non-serialized shuffle.
This bug is very hard to hit since users wouldn't set such a large number of partitions(16 million) for Spark SQL exchange.
TODO: test
## How was this patch tested?
todo.
Author: Wenchen Fan <wenchen@databricks.com>
Closes#21101 from cloud-fan/shuffle.
## What changes were proposed in this pull request?
Currently we find the wider common type by comparing the two types from left to right, this can be a problem when you have two data types which don't have a common type but each can be promoted to StringType.
For instance, if you have a table with the schema:
[c1: date, c2: string, c3: int]
The following succeeds:
SELECT coalesce(c1, c2, c3) FROM table
While the following produces an exception:
SELECT coalesce(c1, c3, c2) FROM table
This is only a issue when the seq of dataTypes contains `StringType` and all the types can do string promotion.
close#19033
## How was this patch tested?
Add test in `TypeCoercionSuite`
Author: Xingbo Jiang <xingbo.jiang@databricks.com>
Closes#21074 from jiangxb1987/typeCoercion.
## What changes were proposed in this pull request?
This pr address comments in https://github.com/apache/spark/pull/19868 ;
Fix the code style for `org.apache.spark.sql.hive.QueryPartitionSuite` by using:
`withTempView`, `withTempDir`, `withTable`...
Author: jinxing <jinxing6042@126.com>
Closes#21091 from jinxing64/SPARK-22676-FOLLOW-UP.
## What changes were proposed in this pull request?
This pr supported interpreted mode for `CatalystToExternalMap`.
## How was this patch tested?
Added tests in `ObjectExpressionsSuite`.
Author: Takeshi Yamamuro <yamamuro@apache.org>
Closes#20979 from maropu/SPARK-23588.
## What changes were proposed in this pull request?
This pr supported interpreted mode for `NewInstance`.
## How was this patch tested?
Added tests in `ObjectExpressionsSuite`.
Author: Takeshi Yamamuro <yamamuro@apache.org>
Closes#20778 from maropu/SPARK-23584.
## What changes were proposed in this pull request?
The PR adds the SQL function `element_at`. The behavior of the function is based on Presto's one.
This function returns element of array at given index in value if column is array, or returns value for the given key in value if column is map.
## How was this patch tested?
Added UTs
Author: Kazuaki Ishizaki <ishizaki@jp.ibm.com>
Closes#21053 from kiszk/SPARK-23924.
## What changes were proposed in this pull request?
The PR adds the SQL function `array_position`. The behavior of the function is based on Presto's one.
The function returns the position of the first occurrence of the element in array x (or 0 if not found) using 1-based index as BigInt.
## How was this patch tested?
Added UTs
Author: Kazuaki Ishizaki <ishizaki@jp.ibm.com>
Closes#21037 from kiszk/SPARK-23919.
## What changes were proposed in this pull request?
DataFrameRangeSuite.test("Cancelling stage in a query with Range.") stays sometimes in an infinite loop and times out the build.
There were multiple issues with the test:
1. The first valid stageId is zero when the test started alone and not in a suite and the following code waits until timeout:
```
eventually(timeout(10.seconds), interval(1.millis)) {
assert(DataFrameRangeSuite.stageToKill > 0)
}
```
2. The `DataFrameRangeSuite.stageToKill` was overwritten by the task's thread after the reset which ended up in canceling the same stage 2 times. This caused the infinite wait.
This PR solves this mentioned flakyness by removing the shared `DataFrameRangeSuite.stageToKill` and using `wait` and `CountDownLatch` for synhronization.
## How was this patch tested?
Existing unit test.
Author: Gabor Somogyi <gabor.g.somogyi@gmail.com>
Closes#20888 from gaborgsomogyi/SPARK-23775.
## What changes were proposed in this pull request?
Use specified accessor in `ArrayData.foreach` and `toArray`.
## How was this patch tested?
Existing tests.
Author: Liang-Chi Hsieh <viirya@gmail.com>
Closes#21099 from viirya/SPARK-23875-followup.
## What changes were proposed in this pull request?
`EqualNullSafe` for `FloatType` and `DoubleType` might generate a wrong result by codegen.
```scala
scala> val df = Seq((Some(-1.0d), None), (None, Some(-1.0d))).toDF()
df: org.apache.spark.sql.DataFrame = [_1: double, _2: double]
scala> df.show()
+----+----+
| _1| _2|
+----+----+
|-1.0|null|
|null|-1.0|
+----+----+
scala> df.filter("_1 <=> _2").show()
+----+----+
| _1| _2|
+----+----+
|-1.0|null|
|null|-1.0|
+----+----+
```
The result should be empty but the result remains two rows.
## How was this patch tested?
Added a test.
Author: Takuya UESHIN <ueshin@databricks.com>
Closes#21094 from ueshin/issues/SPARK-24007/equalnullsafe.
## What changes were proposed in this pull request?
```
Py4JJavaError: An error occurred while calling o153.sql.
: org.apache.spark.SparkException: Job aborted.
at org.apache.spark.sql.execution.datasources.FileFormatWriter$.write(FileFormatWriter.scala:223)
at org.apache.spark.sql.execution.datasources.InsertIntoHadoopFsRelationCommand.run(InsertIntoHadoopFsRelationCommand.scala:189)
at org.apache.spark.sql.execution.command.ExecutedCommandExec.sideEffectResult$lzycompute(commands.scala:70)
at org.apache.spark.sql.execution.command.ExecutedCommandExec.sideEffectResult(commands.scala:68)
at org.apache.spark.sql.execution.command.ExecutedCommandExec.executeCollect(commands.scala:79)
at org.apache.spark.sql.Dataset$$anonfun$6.apply(Dataset.scala:190)
at org.apache.spark.sql.Dataset$$anonfun$6.apply(Dataset.scala:190)
at org.apache.spark.sql.Dataset$$anonfun$59.apply(Dataset.scala:3021)
at org.apache.spark.sql.execution.SQLExecution$.withCustomExecutionEnv(SQLExecution.scala:89)
at org.apache.spark.sql.execution.SQLExecution$.withNewExecutionId(SQLExecution.scala:127)
at org.apache.spark.sql.Dataset.withAction(Dataset.scala:3020)
at org.apache.spark.sql.Dataset.<init>(Dataset.scala:190)
at org.apache.spark.sql.Dataset$.ofRows(Dataset.scala:74)
at org.apache.spark.sql.SparkSession.sql(SparkSession.scala:646)
at sun.reflect.GeneratedMethodAccessor153.invoke(Unknown Source)
at sun.reflect.DelegatingMethodAccessorImpl.invoke(DelegatingMethodAccessorImpl.java:43)
at java.lang.reflect.Method.invoke(Method.java:498)
at py4j.reflection.MethodInvoker.invoke(MethodInvoker.java:244)
at py4j.reflection.ReflectionEngine.invoke(ReflectionEngine.java:380)
at py4j.Gateway.invoke(Gateway.java:293)
at py4j.commands.AbstractCommand.invokeMethod(AbstractCommand.java:132)
at py4j.commands.CallCommand.execute(CallCommand.java:79)
at py4j.GatewayConnection.run(GatewayConnection.java:226)
at java.lang.Thread.run(Thread.java:748)
Caused by: org.apache.spark.SparkException: Exception thrown in Future.get:
at org.apache.spark.sql.execution.exchange.BroadcastExchangeExec.doExecuteBroadcast(BroadcastExchangeExec.scala:190)
at org.apache.spark.sql.execution.InputAdapter.doExecuteBroadcast(WholeStageCodegenExec.scala:267)
at org.apache.spark.sql.execution.joins.BroadcastNestedLoopJoinExec.doConsume(BroadcastNestedLoopJoinExec.scala:530)
at org.apache.spark.sql.execution.CodegenSupport$class.consume(WholeStageCodegenExec.scala:155)
at org.apache.spark.sql.execution.ProjectExec.consume(basicPhysicalOperators.scala:37)
at org.apache.spark.sql.execution.ProjectExec.doConsume(basicPhysicalOperators.scala:69)
at org.apache.spark.sql.execution.CodegenSupport$class.consume(WholeStageCodegenExec.scala:155)
at org.apache.spark.sql.execution.FilterExec.consume(basicPhysicalOperators.scala:144)
...
at org.apache.spark.sql.execution.datasources.FileFormatWriter$.write(FileFormatWriter.scala:190)
... 23 more
Caused by: java.util.concurrent.ExecutionException: org.apache.spark.SparkException: Task not serializable
at java.util.concurrent.FutureTask.report(FutureTask.java:122)
at java.util.concurrent.FutureTask.get(FutureTask.java:206)
at org.apache.spark.sql.execution.exchange.BroadcastExchangeExec.doExecuteBroadcast(BroadcastExchangeExec.scala:179)
... 276 more
Caused by: org.apache.spark.SparkException: Task not serializable
at org.apache.spark.util.ClosureCleaner$.ensureSerializable(ClosureCleaner.scala:340)
at org.apache.spark.util.ClosureCleaner$.org$apache$spark$util$ClosureCleaner$$clean(ClosureCleaner.scala:330)
at org.apache.spark.util.ClosureCleaner$.clean(ClosureCleaner.scala:156)
at org.apache.spark.SparkContext.clean(SparkContext.scala:2380)
at org.apache.spark.rdd.RDD$$anonfun$mapPartitionsWithIndex$1.apply(RDD.scala:850)
at org.apache.spark.rdd.RDD$$anonfun$mapPartitionsWithIndex$1.apply(RDD.scala:849)
at org.apache.spark.rdd.RDDOperationScope$.withScope(RDDOperationScope.scala:151)
at org.apache.spark.rdd.RDDOperationScope$.withScope(RDDOperationScope.scala:112)
at org.apache.spark.rdd.RDD.withScope(RDD.scala:371)
at org.apache.spark.rdd.RDD.mapPartitionsWithIndex(RDD.scala:849)
at org.apache.spark.sql.execution.WholeStageCodegenExec.doExecute(WholeStageCodegenExec.scala:417)
at org.apache.spark.sql.execution.SparkPlan$$anonfun$execute$1.apply(SparkPlan.scala:123)
at org.apache.spark.sql.execution.SparkPlan$$anonfun$execute$1.apply(SparkPlan.scala:118)
at org.apache.spark.sql.execution.SparkPlan$$anonfun$executeQuery$3.apply(SparkPlan.scala:152)
at org.apache.spark.rdd.RDDOperationScope$.withScope(RDDOperationScope.scala:151)
at org.apache.spark.sql.execution.SparkPlan.executeQuery(SparkPlan.scala:149)
at org.apache.spark.sql.execution.SparkPlan.execute(SparkPlan.scala:118)
at org.apache.spark.sql.execution.exchange.ShuffleExchangeExec.prepareShuffleDependency(ShuffleExchangeExec.scala:89)
at org.apache.spark.sql.execution.exchange.ShuffleExchangeExec$$anonfun$doExecute$1.apply(ShuffleExchangeExec.scala:125)
at org.apache.spark.sql.execution.exchange.ShuffleExchangeExec$$anonfun$doExecute$1.apply(ShuffleExchangeExec.scala:116)
at org.apache.spark.sql.catalyst.errors.package$.attachTree(package.scala:52)
at org.apache.spark.sql.execution.exchange.ShuffleExchangeExec.doExecute(ShuffleExchangeExec.scala:116)
at org.apache.spark.sql.execution.SparkPlan$$anonfun$execute$1.apply(SparkPlan.scala:123)
at org.apache.spark.sql.execution.SparkPlan$$anonfun$execute$1.apply(SparkPlan.scala:118)
at org.apache.spark.sql.execution.SparkPlan$$anonfun$executeQuery$3.apply(SparkPlan.scala:152)
at org.apache.spark.rdd.RDDOperationScope$.withScope(RDDOperationScope.scala:151)
at org.apache.spark.sql.execution.SparkPlan.executeQuery(SparkPlan.scala:149)
at org.apache.spark.sql.execution.SparkPlan.execute(SparkPlan.scala:118)
at org.apache.spark.sql.execution.InputAdapter.inputRDDs(WholeStageCodegenExec.scala:271)
at org.apache.spark.sql.execution.aggregate.HashAggregateExec.inputRDDs(HashAggregateExec.scala:181)
at org.apache.spark.sql.execution.WholeStageCodegenExec.doExecute(WholeStageCodegenExec.scala:414)
at org.apache.spark.sql.execution.SparkPlan$$anonfun$execute$1.apply(SparkPlan.scala:123)
at org.apache.spark.sql.execution.SparkPlan$$anonfun$execute$1.apply(SparkPlan.scala:118)
at org.apache.spark.sql.execution.SparkPlan$$anonfun$executeQuery$3.apply(SparkPlan.scala:152)
at org.apache.spark.rdd.RDDOperationScope$.withScope(RDDOperationScope.scala:151)
at org.apache.spark.sql.execution.SparkPlan.executeQuery(SparkPlan.scala:149)
at org.apache.spark.sql.execution.SparkPlan.execute(SparkPlan.scala:118)
at org.apache.spark.sql.execution.collect.Collector$.collect(Collector.scala:61)
at org.apache.spark.sql.execution.collect.Collector$.collect(Collector.scala:70)
at org.apache.spark.sql.execution.SparkPlan.executeCollectResult(SparkPlan.scala:264)
at org.apache.spark.sql.execution.exchange.BroadcastExchangeExec$$anon$1$$anonfun$call$1.apply(BroadcastExchangeExec.scala:93)
at org.apache.spark.sql.execution.exchange.BroadcastExchangeExec$$anon$1$$anonfun$call$1.apply(BroadcastExchangeExec.scala:81)
at org.apache.spark.sql.execution.SQLExecution$.withExecutionId(SQLExecution.scala:150)
at org.apache.spark.sql.execution.exchange.BroadcastExchangeExec$$anon$1.call(BroadcastExchangeExec.scala:80)
at org.apache.spark.sql.execution.exchange.BroadcastExchangeExec$$anon$1.call(BroadcastExchangeExec.scala:76)
at java.util.concurrent.FutureTask.run(FutureTask.java:266)
at java.util.concurrent.ThreadPoolExecutor.runWorker(ThreadPoolExecutor.java:1149)
at java.util.concurrent.ThreadPoolExecutor$Worker.run(ThreadPoolExecutor.java:624)
... 1 more
Caused by: java.nio.BufferUnderflowException
at java.nio.HeapByteBuffer.get(HeapByteBuffer.java:151)
at java.nio.ByteBuffer.get(ByteBuffer.java:715)
at org.apache.parquet.io.api.Binary$ByteBufferBackedBinary.getBytes(Binary.java:405)
at org.apache.parquet.io.api.Binary$ByteBufferBackedBinary.getBytesUnsafe(Binary.java:414)
at org.apache.parquet.io.api.Binary$ByteBufferBackedBinary.writeObject(Binary.java:484)
at sun.reflect.NativeMethodAccessorImpl.invoke0(Native Method)
at sun.reflect.NativeMethodAccessorImpl.invoke(NativeMethodAccessorImpl.java:62)
at sun.reflect.DelegatingMethodAccessorImpl.invoke(DelegatingMethodAccessorImpl.java:43)
at java.lang.reflect.Method.invoke(Method.java:498)
at java.io.ObjectStreamClass.invokeWriteObject(ObjectStreamClass.java:1128)
at java.io.ObjectOutputStream.writeSerialData(ObjectOutputStream.java:1496)
```
The Parquet filters are serializable but not thread safe. SparkPlan.prepare() could be called in different threads (BroadcastExchange will call it in a thread pool). Thus, we could serialize the same Parquet filter at the same time. This is not easily reproduced. The fix is to avoid serializing these Parquet filters in the driver. This PR is to avoid serializing these Parquet filters by moving the parquet filter generation from the driver to executors.
## How was this patch tested?
Having two queries one is a 1000-line SQL query and a 3000-line SQL query. Need to run at least one hour with a heavy write workload to reproduce once.
Author: gatorsmile <gatorsmile@gmail.com>
Closes#21086 from gatorsmile/taskNotSerializable.
## What changes were proposed in this pull request?
Each data source implementation can define its own options and teach its users how to set them. Spark doesn't have any restrictions about what options a data source should or should not have. It's possible that some options are very common and many data sources use them. However different data sources may define the common options(key and meaning) differently, which is quite confusing to end users.
This PR defines some standard options that data sources can optionally adopt: path, table and database.
## How was this patch tested?
a new test case.
Author: Wenchen Fan <wenchen@databricks.com>
Closes#20535 from cloud-fan/options.
## What changes were proposed in this pull request?
Added `TransitPredicateInOuterJoin` optimization rule that transits constraints from the preserved side of an outer join to the null-supplying side. The constraints of the join operator will remain unchanged.
## How was this patch tested?
Added 3 tests in `InferFiltersFromConstraintsSuite`.
Author: maryannxue <maryann.xue@gmail.com>
Closes#20816 from maryannxue/spark-21479.
## What changes were proposed in this pull request?
We are using `CodegenContext.freshName` to get a unique name for any new variable we are adding. Unfortunately, this method currently fails to create a unique name when we request more than one instance of variables with starting name `name1` and an instance with starting name `name11`.
The PR changes the way a new name is generated by `CodegenContext.freshName` so that we generate unique names in this scenario too.
## How was this patch tested?
added UT
Author: Marco Gaido <marcogaido91@gmail.com>
Closes#21080 from mgaido91/SPARK-23986.
## What changes were proposed in this pull request?
In current code, it will scanning all partition paths when spark.sql.hive.verifyPartitionPath=true.
e.g. table like below:
```
CREATE TABLE `test`(
`id` int,
`age` int,
`name` string)
PARTITIONED BY (
`A` string,
`B` string)
load data local inpath '/tmp/data0' into table test partition(A='00', B='00')
load data local inpath '/tmp/data1' into table test partition(A='01', B='01')
load data local inpath '/tmp/data2' into table test partition(A='10', B='10')
load data local inpath '/tmp/data3' into table test partition(A='11', B='11')
```
If I query with SQL – "select * from test where A='00' and B='01' ", current code will scan all partition paths including '/data/A=00/B=00', '/data/A=00/B=00', '/data/A=01/B=01', '/data/A=10/B=10', '/data/A=11/B=11'. It costs much time and memory cost.
This pr proposes to avoid iterating all partition paths. Add a config `spark.files.ignoreMissingFiles` and ignore the `file not found` when `getPartitions/compute`(for hive table scan). This is much like the logic brought by
`spark.sql.files.ignoreMissingFiles`(which is for datasource scan).
## How was this patch tested?
UT
Author: jinxing <jinxing6042@126.com>
Closes#19868 from jinxing64/SPARK-22676.
## What changes were proposed in this pull request?
There was no check on nullability for arguments of `Tuple`s. This could lead to have weird behavior when a null value had to be deserialized into a non-nullable Scala object: in those cases, the `null` got silently transformed in a valid value (like `-1` for `Int`), corresponding to the default value we are using in the SQL codebase. This situation was very likely to happen when deserializing to a Tuple of primitive Scala types (like Double, Int, ...).
The PR adds the `AssertNotNull` to arguments of tuples which have been asked to be converted to non-nullable types.
## How was this patch tested?
added UT
Author: Marco Gaido <marcogaido91@gmail.com>
Closes#20976 from mgaido91/SPARK-23835.
## What changes were proposed in this pull request?
We don't have a good way to sequentially access `UnsafeArrayData` with a common interface such as `Seq`. An example is `MapObject` where we need to access several sequence collection types together. But `UnsafeArrayData` doesn't implement `ArrayData.array`. Calling `toArray` will copy the entire array. We can provide an `IndexedSeq` wrapper for `ArrayData`, so we can avoid copying the entire array.
## How was this patch tested?
Added test.
Author: Liang-Chi Hsieh <viirya@gmail.com>
Closes#20984 from viirya/SPARK-23875.
## What changes were proposed in this pull request?
Unit tests for EpochCoordinator that test correct sequencing of committed epochs. Several tests are ignored since they test functionality implemented in SPARK-23503 which is not yet merged, otherwise they fail.
Author: Efim Poberezkin <efim@poberezkin.ru>
Closes#20983 from efimpoberezkin/pr/EpochCoordinator-tests.
## What changes were proposed in this pull request?
Add a memory source for continuous processing.
Note that only one of the ContinuousSuite tests is migrated to minimize the diff here. I'll submit a second PR for SPARK-23688 to change the rest and get rid of waitForRateSourceTriggers.
## How was this patch tested?
unit test
Author: Jose Torres <torres.joseph.f+github@gmail.com>
Closes#20828 from jose-torres/continuousMemory.
## What changes were proposed in this pull request?
The PR adds the SQL function `array_min`. It takes an array as argument and returns the minimum value in it.
## How was this patch tested?
added UTs
Author: Marco Gaido <marcogaido91@gmail.com>
Closes#21025 from mgaido91/SPARK-23918.
## What changes were proposed in this pull request?
Currently, interpreted execution of `LambdaVariable` just uses `InternalRow.get` to access element. We should use specified accessors if possible.
## How was this patch tested?
Added test.
Author: Liang-Chi Hsieh <viirya@gmail.com>
Closes#20981 from viirya/SPARK-23873.
## What changes were proposed in this pull request?
The PR adds the SQL function `array_max`. It takes an array as argument and returns the maximum value in it.
## How was this patch tested?
added UTs
Author: Marco Gaido <marcogaido91@gmail.com>
Closes#21024 from mgaido91/SPARK-23917.
## What changes were proposed in this pull request?
Just found `MultiAlias` is a `CodegenFallback`. It should not be as looks like `MultiAlias` won't be evaluated.
## How was this patch tested?
Existing tests.
Author: Liang-Chi Hsieh <viirya@gmail.com>
Closes#21065 from viirya/multialias-without-codegenfallback.
## What changes were proposed in this pull request?
Checkpoint files (offset log files, state store files) in Structured Streaming must be written atomically such that no partial files are generated (would break fault-tolerance guarantees). Currently, there are 3 locations which try to do this individually, and in some cases, incorrectly.
1. HDFSOffsetMetadataLog - This uses a FileManager interface to use any implementation of `FileSystem` or `FileContext` APIs. It preferably loads `FileContext` implementation as FileContext of HDFS has atomic renames.
1. HDFSBackedStateStore (aka in-memory state store)
- Writing a version.delta file - This uses FileSystem APIs only to perform a rename. This is incorrect as rename is not atomic in HDFS FileSystem implementation.
- Writing a snapshot file - Same as above.
#### Current problems:
1. State Store behavior is incorrect - HDFS FileSystem implementation does not have atomic rename.
1. Inflexible - Some file systems provide mechanisms other than write-to-temp-file-and-rename for writing atomically and more efficiently. For example, with S3 you can write directly to the final file and it will be made visible only when the entire file is written and closed correctly. Any failure can be made to terminate the writing without making any partial files visible in S3. The current code does not abstract out this mechanism enough that it can be customized.
#### Solution:
1. Introduce a common interface that all 3 cases above can use to write checkpoint files atomically.
2. This interface must provide the necessary interfaces that allow customization of the write-and-rename mechanism.
This PR does that by introducing the interface `CheckpointFileManager` and modifying `HDFSMetadataLog` and `HDFSBackedStateStore` to use the interface. Similar to earlier `FileManager`, there are implementations based on `FileSystem` and `FileContext` APIs, and the latter implementation is preferred to make it work correctly with HDFS.
The key method this interface has is `createAtomic(path, overwrite)` which returns a `CancellableFSDataOutputStream` that has the method `cancel()`. All users of this method need to either call `close()` to successfully write the file, or `cancel()` in case of an error.
## How was this patch tested?
New tests in `CheckpointFileManagerSuite` and slightly modified existing tests.
Author: Tathagata Das <tathagata.das1565@gmail.com>
Closes#21048 from tdas/SPARK-23966.
## What changes were proposed in this pull request?
TableReader would get disproportionately slower as the number of columns in the query increased.
I fixed the way TableReader was looking up metadata for each column in the row. Previously, it had been looking up this data in linked lists, accessing each linked list by an index (column number). Now it looks up this data in arrays, where indexing by column number works better.
## How was this patch tested?
Manual testing
All sbt unit tests
python sql tests
Author: Bruce Robbins <bersprockets@gmail.com>
Closes#21043 from bersprockets/tabreadfix.
## What changes were proposed in this pull request?
Currently `PartitioningAwareFileIndex` accepts an optional parameter `userPartitionSchema`. If provided, it will combine the inferred partition schema with the parameter.
However,
1. to get `userPartitionSchema`, we need to combine inferred partition schema with `userSpecifiedSchema`
2. to get the inferred partition schema, we have to create a temporary file index.
Only after that, a final version of `PartitioningAwareFileIndex` can be created.
This can be improved by passing `userSpecifiedSchema` to `PartitioningAwareFileIndex`.
With the improvement, we can reduce redundant code and avoid parsing the file partition twice.
## How was this patch tested?
Unit test
Author: Gengliang Wang <gengliang.wang@databricks.com>
Closes#21004 from gengliangwang/PartitioningAwareFileIndex.
## What changes were proposed in this pull request?
Add UDF weekday
## How was this patch tested?
A new test
Author: yucai <yyu1@ebay.com>
Closes#21009 from yucai/SPARK-23905.
## What changes were proposed in this pull request?
Many suites currently leak Spark sessions (sometimes with stopped SparkContexts) via the thread-local active Spark session and default Spark session. We should attempt to clean these up and detect when this happens to improve the reproducibility of tests.
## How was this patch tested?
Existing tests
Author: Eric Liang <ekl@databricks.com>
Closes#21058 from ericl/clear-session.
## What changes were proposed in this pull request?
This PR proposes to add `collect` to a query executor as an action.
Seems `collect` / `collect` with Arrow are not recognised via `QueryExecutionListener` as an action. For example, if we have a custom listener as below:
```scala
package org.apache.spark.sql
import org.apache.spark.internal.Logging
import org.apache.spark.sql.execution.QueryExecution
import org.apache.spark.sql.util.QueryExecutionListener
class TestQueryExecutionListener extends QueryExecutionListener with Logging {
override def onSuccess(funcName: String, qe: QueryExecution, durationNs: Long): Unit = {
logError("Look at me! I'm 'onSuccess'")
}
override def onFailure(funcName: String, qe: QueryExecution, exception: Exception): Unit = { }
}
```
and set `spark.sql.queryExecutionListeners` to `org.apache.spark.sql.TestQueryExecutionListener`
Other operations in PySpark or Scala side seems fine:
```python
>>> sql("SELECT * FROM range(1)").show()
```
```
18/04/09 17:02:04 ERROR TestQueryExecutionListener: Look at me! I'm 'onSuccess'
+---+
| id|
+---+
| 0|
+---+
```
```scala
scala> sql("SELECT * FROM range(1)").collect()
```
```
18/04/09 16:58:41 ERROR TestQueryExecutionListener: Look at me! I'm 'onSuccess'
res1: Array[org.apache.spark.sql.Row] = Array([0])
```
but ..
**Before**
```python
>>> sql("SELECT * FROM range(1)").collect()
```
```
[Row(id=0)]
```
```python
>>> spark.conf.set("spark.sql.execution.arrow.enabled", "true")
>>> sql("SELECT * FROM range(1)").toPandas()
```
```
id
0 0
```
**After**
```python
>>> sql("SELECT * FROM range(1)").collect()
```
```
18/04/09 16:57:58 ERROR TestQueryExecutionListener: Look at me! I'm 'onSuccess'
[Row(id=0)]
```
```python
>>> spark.conf.set("spark.sql.execution.arrow.enabled", "true")
>>> sql("SELECT * FROM range(1)").toPandas()
```
```
18/04/09 17:53:26 ERROR TestQueryExecutionListener: Look at me! I'm 'onSuccess'
id
0 0
```
## How was this patch tested?
I have manually tested as described above and unit test was added.
Author: hyukjinkwon <gurwls223@apache.org>
Closes#21007 from HyukjinKwon/SPARK-23942.
## What changes were proposed in this pull request?
Current SS continuous doesn't support processing on temp table or `df.as("xxx")`, SS will throw an exception as LogicalPlan not supported, details described in [here](https://issues.apache.org/jira/browse/SPARK-23748).
So here propose to add this support.
## How was this patch tested?
new UT.
Author: jerryshao <sshao@hortonworks.com>
Closes#21017 from jerryshao/SPARK-23748.
## What changes were proposed in this pull request?
This PR tries to use `MemoryBlock` in `UTF8StringBuffer`. In general, there are two advantages to use `MemoryBlock`.
1. Has clean API calls rather than using a Java array or `PlatformMemory`
2. Improve runtime performance of memory access instead of using `Object`.
## How was this patch tested?
Added `UTF8StringBufferSuite`
Author: Kazuaki Ishizaki <ishizaki@jp.ibm.com>
Closes#20871 from kiszk/SPARK-23762.
SQLMetricsTestUtils.currentExecutionIds() was racing with the listener
bus, which lead to some flaky tests. We should wait till the listener bus is
empty.
I tested by adding some Thread.sleep()s in SQLAppStatusListener, which
reproduced the exceptions I saw on Jenkins. With this change, they went
away.
Author: Imran Rashid <irashid@cloudera.com>
Closes#21041 from squito/SPARK-23962.
## What changes were proposed in this pull request?
Mark `HashAggregateExec.bufVars` as transient to avoid it from being serialized.
Also manually null out this field at the end of `doProduceWithoutKeys()` to shorten its lifecycle, because it'll no longer be used after that.
## How was this patch tested?
Existing tests.
Author: Kris Mok <kris.mok@databricks.com>
Closes#21039 from rednaxelafx/codegen-improve.
## What changes were proposed in this pull request?
This PR slightly refactors the newly added `ExprValue` API by quite a bit. The following changes are introduced:
1. `ExprValue` now uses the actual class instead of the class name as its type. This should give some more flexibility with generating code in the future.
2. Renamed `StatementValue` to `SimpleExprValue`. The statement concept is broader then an expression (untyped and it cannot be on the right hand side of an assignment), and this was not really what we were using it for. I have added a top level `JavaCode` trait that can be used in the future to reinstate (no pun intended) a statement a-like code fragment.
3. Added factory methods to the `JavaCode` companion object to make it slightly less verbose to create `JavaCode`/`ExprValue` objects. This is also what makes the diff quite large.
4. Added one more factory method to `ExprCode` to make it easier to create code-less expressions.
## How was this patch tested?
Existing tests.
Author: Herman van Hovell <hvanhovell@databricks.com>
Closes#21026 from hvanhovell/SPARK-23951.
## What changes were proposed in this pull request?
In the PR #20886, I mistakenly check the table location only when `ignoreIfExists` is false, which was following the original deprecated PR.
That was wrong. When `ignoreIfExists` is true and the target table doesn't exist, we should also check the table location. In other word, **`ignoreIfExists` has nothing to do with table location validation**.
This is a follow-up PR to fix the mistake.
## How was this patch tested?
Add one unit test.
Author: Gengliang Wang <gengliang.wang@databricks.com>
Closes#21001 from gengliangwang/SPARK-19724-followup.
## What changes were proposed in this pull request?
This PR moves writing of `UnsafeRow`, `UnsafeArrayData` & `UnsafeMapData` out of the `GenerateUnsafeProjection`/`InterpretedUnsafeProjection` classes into the `UnsafeWriter` interface. This cleans up the code a little bit, and it should also result in less byte code for the code generated path.
## How was this patch tested?
Existing tests
Author: Herman van Hovell <hvanhovell@databricks.com>
Closes#20986 from hvanhovell/SPARK-23864.
## What changes were proposed in this pull request?
Code generation for the `Add` and `Subtract` expressions was not done using the `BinaryArithmetic.doCodeGen` method because these expressions also support `CalendarInterval`. This leads to a bit of duplication.
This PR gets rid of that duplication by adding `calendarIntervalMethod` to `BinaryArithmetic` and doing the code generation for `CalendarInterval` in `BinaryArithmetic` instead.
## How was this patch tested?
Existing tests.
Author: Herman van Hovell <hvanhovell@databricks.com>
Closes#21005 from hvanhovell/SPARK-23898.
## What changes were proposed in this pull request?
Add `hashUTF8String()` to the hasher classes to allow Spark SQL codegen to generate cleaner code for hashing `UTF8String`s. No change in behavior otherwise.
Although with the introduction of SPARK-10399, the code size for hashing `UTF8String` is already smaller, it's still good to extract a separate function in the hasher classes so that the generated code can stay clean.
## How was this patch tested?
Existing tests.
Author: Kris Mok <kris.mok@databricks.com>
Closes#21016 from rednaxelafx/hashutf8.
## What changes were proposed in this pull request?
The codegen output of `Expression`, aka `ExprCode`, now encapsulates only strings of output value (`value`) and nullability (`isNull`). It makes difficulty for us to know what the output really is. I think it is better if we can add wrappers for the value and nullability that let us to easily know that.
## How was this patch tested?
Existing tests.
Author: Liang-Chi Hsieh <viirya@gmail.com>
Closes#20043 from viirya/SPARK-22856.
## What changes were proposed in this pull request?
This PR avoids possible overflow at an operation `long = (long)(int * int)`. The multiplication of large positive integer values may set one to MSB. This leads to a negative value in long while we expected a positive value (e.g. `0111_0000_0000_0000 * 0000_0000_0000_0010`).
This PR performs long cast before the multiplication to avoid this situation.
## How was this patch tested?
Existing UTs
Author: Kazuaki Ishizaki <ishizaki@jp.ibm.com>
Closes#21002 from kiszk/SPARK-23893.
## What changes were proposed in this pull request?
Proposed tests checks that only subset of input dataset is touched during schema inferring.
Author: Maxim Gekk <maxim.gekk@databricks.com>
Closes#20963 from MaxGekk/json-sampling-tests.
## What changes were proposed in this pull request?
Column.scala and Functions.scala have asc_nulls_first, asc_nulls_last, desc_nulls_first and desc_nulls_last. Add the corresponding python APIs in column.py and functions.py
## How was this patch tested?
Add doctest
Author: Huaxin Gao <huaxing@us.ibm.com>
Closes#20962 from huaxingao/spark-23847.
## What changes were proposed in this pull request?
Add docstring to clarify default window frame boundaries with and without orderBy clause
## How was this patch tested?
Manually generate doc and check.
Author: Li Jin <ice.xelloss@gmail.com>
Closes#20978 from icexelloss/SPARK-23861-window-doc.
## What changes were proposed in this pull request?
This pull request tries to improve the error message for spark while reading parquet files with different schemas, e.g. One with a STRING column and the other with a INT column. A new ParquetSchemaColumnConvertNotSupportedException is added to replace the old UnsupportedOperationException. The Exception is again wrapped in FileScanRdd.scala to throw a more a general QueryExecutionException with the actual parquet file name which trigger the exception.
## How was this patch tested?
Unit tests added to check the new exception and verify the error messages.
Also manually tested with two parquet with different schema to check the error message.
<img width="1125" alt="screen shot 2018-03-30 at 4 03 04 pm" src="https://user-images.githubusercontent.com/37087310/38156580-dd58a140-3433-11e8-973a-b816d859fbe1.png">
Author: Yuchen Huo <yuchen.huo@databricks.com>
Closes#20953 from yuchenhuo/SPARK-23822.
## What changes were proposed in this pull request?
Easy fix in the documentation.
## How was this patch tested?
N/A
Closes#20948
Author: Daniel Sakuma <dsakuma@gmail.com>
Closes#20928 from dsakuma/fix_typo_configuration_docs.
## What changes were proposed in this pull request?
This PR is to finish https://github.com/apache/spark/pull/17272
This JIRA is a follow up work after SPARK-19583
As we discussed in that PR
The following DDL for a managed table with an existed default location should throw an exception:
CREATE TABLE ... (PARTITIONED BY ...) AS SELECT ...
CREATE TABLE ... (PARTITIONED BY ...)
Currently there are some situations which are not consist with above logic:
CREATE TABLE ... (PARTITIONED BY ...) succeed with an existed default location
situation: for both hive/datasource(with HiveExternalCatalog/InMemoryCatalog)
CREATE TABLE ... (PARTITIONED BY ...) AS SELECT ...
situation: hive table succeed with an existed default location
This PR is going to make above two situations consist with the logic that it should throw an exception
with an existed default location.
## How was this patch tested?
unit test added
Author: Gengliang Wang <gengliang.wang@databricks.com>
Closes#20886 from gengliangwang/pr-17272.
Fixes https://issues.apache.org/jira/browse/SPARK-23823
Keep origin for all the methods using transformExpression
## What changes were proposed in this pull request?
Keep origin in transformExpression
## How was this patch tested?
Manually tested that this fixes https://issues.apache.org/jira/browse/SPARK-23823 and columns have correct origins after Analyzer.analyze
Author: JiahuiJiang <jjiang@palantir.com>
Author: Jiahui Jiang <jjiang@palantir.com>
Closes#20961 from JiahuiJiang/jj/keep-origin.
## What changes were proposed in this pull request?
This PR allows us to use one of several types of `MemoryBlock`, such as byte array, int array, long array, or `java.nio.DirectByteBuffer`. To use `java.nio.DirectByteBuffer` allows to have off heap memory which is automatically deallocated by JVM. `MemoryBlock` class has primitive accessors like `Platform.getInt()`, `Platform.putint()`, or `Platform.copyMemory()`.
This PR uses `MemoryBlock` for `OffHeapColumnVector`, `UTF8String`, and other places. This PR can improve performance of operations involving memory accesses (e.g. `UTF8String.trim`) by 1.8x.
For now, this PR does not use `MemoryBlock` for `BufferHolder` based on cloud-fan's [suggestion](https://github.com/apache/spark/pull/11494#issuecomment-309694290).
Since this PR is a successor of #11494, close#11494. Many codes were ported from #11494. Many efforts were put here. **I think this PR should credit to yzotov.**
This PR can achieve **1.1-1.4x performance improvements** for operations in `UTF8String` or `Murmur3_x86_32`. Other operations are almost comparable performances.
Without this PR
```
OpenJDK 64-Bit Server VM 1.8.0_121-8u121-b13-0ubuntu1.16.04.2-b13 on Linux 4.4.0-22-generic
Intel(R) Xeon(R) CPU E5-2667 v3 3.20GHz
OpenJDK 64-Bit Server VM 1.8.0_121-8u121-b13-0ubuntu1.16.04.2-b13 on Linux 4.4.0-22-generic
Intel(R) Xeon(R) CPU E5-2667 v3 3.20GHz
Hash byte arrays with length 268435487: Best/Avg Time(ms) Rate(M/s) Per Row(ns) Relative
------------------------------------------------------------------------------------------------
Murmur3_x86_32 526 / 536 0.0 131399881.5 1.0X
UTF8String benchmark: Best/Avg Time(ms) Rate(M/s) Per Row(ns) Relative
------------------------------------------------------------------------------------------------
hashCode 525 / 552 1022.6 1.0 1.0X
substring 414 / 423 1298.0 0.8 1.3X
```
With this PR
```
OpenJDK 64-Bit Server VM 1.8.0_121-8u121-b13-0ubuntu1.16.04.2-b13 on Linux 4.4.0-22-generic
Intel(R) Xeon(R) CPU E5-2667 v3 3.20GHz
Hash byte arrays with length 268435487: Best/Avg Time(ms) Rate(M/s) Per Row(ns) Relative
------------------------------------------------------------------------------------------------
Murmur3_x86_32 474 / 488 0.0 118552232.0 1.0X
UTF8String benchmark: Best/Avg Time(ms) Rate(M/s) Per Row(ns) Relative
------------------------------------------------------------------------------------------------
hashCode 476 / 480 1127.3 0.9 1.0X
substring 287 / 291 1869.9 0.5 1.7X
```
Benchmark program
```
test("benchmark Murmur3_x86_32") {
val length = 8192 * 32768 + 31
val seed = 42L
val iters = 1 << 2
val random = new Random(seed)
val arrays = Array.fill[MemoryBlock](numArrays) {
val bytes = new Array[Byte](length)
random.nextBytes(bytes)
new ByteArrayMemoryBlock(bytes, Platform.BYTE_ARRAY_OFFSET, length)
}
val benchmark = new Benchmark("Hash byte arrays with length " + length,
iters * numArrays, minNumIters = 20)
benchmark.addCase("HiveHasher") { _: Int =>
var sum = 0L
for (_ <- 0L until iters) {
sum += HiveHasher.hashUnsafeBytesBlock(
arrays(i), Platform.BYTE_ARRAY_OFFSET, length)
}
}
benchmark.run()
}
test("benchmark UTF8String") {
val N = 512 * 1024 * 1024
val iters = 2
val benchmark = new Benchmark("UTF8String benchmark", N, minNumIters = 20)
val str0 = new java.io.StringWriter() { { for (i <- 0 until N) { write(" ") } } }.toString
val s0 = UTF8String.fromString(str0)
benchmark.addCase("hashCode") { _: Int =>
var h: Int = 0
for (_ <- 0L until iters) { h += s0.hashCode }
}
benchmark.addCase("substring") { _: Int =>
var s: UTF8String = null
for (_ <- 0L until iters) { s = s0.substring(N / 2 - 5, N / 2 + 5) }
}
benchmark.run()
}
```
I run [this benchmark program](https://gist.github.com/kiszk/94f75b506c93a663bbbc372ffe8f05de) using [the commit](ee5a79861c). I got the following results:
```
OpenJDK 64-Bit Server VM 1.8.0_151-8u151-b12-0ubuntu0.16.04.2-b12 on Linux 4.4.0-66-generic
Intel(R) Xeon(R) CPU E5-2667 v3 3.20GHz
Memory access benchmarks: Best/Avg Time(ms) Rate(M/s) Per Row(ns) Relative
------------------------------------------------------------------------------------------------
ByteArrayMemoryBlock get/putInt() 220 / 221 609.3 1.6 1.0X
Platform get/putInt(byte[]) 220 / 236 610.9 1.6 1.0X
Platform get/putInt(Object) 492 / 494 272.8 3.7 0.4X
OnHeapMemoryBlock get/putLong() 322 / 323 416.5 2.4 0.7X
long[] 221 / 221 608.0 1.6 1.0X
Platform get/putLong(long[]) 321 / 321 418.7 2.4 0.7X
Platform get/putLong(Object) 561 / 563 239.2 4.2 0.4X
```
I also run [this benchmark program](https://gist.github.com/kiszk/5fdb4e03733a5d110421177e289d1fb5) for comparing performance of `Platform.copyMemory()`.
```
OpenJDK 64-Bit Server VM 1.8.0_151-8u151-b12-0ubuntu0.16.04.2-b12 on Linux 4.4.0-66-generic
Intel(R) Xeon(R) CPU E5-2667 v3 3.20GHz
Platform copyMemory: Best/Avg Time(ms) Rate(M/s) Per Row(ns) Relative
------------------------------------------------------------------------------------------------
Object to Object 1961 / 1967 8.6 116.9 1.0X
System.arraycopy Object to Object 1917 / 1921 8.8 114.3 1.0X
byte array to byte array 1961 / 1968 8.6 116.9 1.0X
System.arraycopy byte array to byte array 1909 / 1937 8.8 113.8 1.0X
int array to int array 1921 / 1990 8.7 114.5 1.0X
double array to double array 1918 / 1923 8.7 114.3 1.0X
Object to byte array 1961 / 1967 8.6 116.9 1.0X
Object to short array 1965 / 1972 8.5 117.1 1.0X
Object to int array 1910 / 1915 8.8 113.9 1.0X
Object to float array 1971 / 1978 8.5 117.5 1.0X
Object to double array 1919 / 1944 8.7 114.4 1.0X
byte array to Object 1959 / 1967 8.6 116.8 1.0X
int array to Object 1961 / 1970 8.6 116.9 1.0X
double array to Object 1917 / 1924 8.8 114.3 1.0X
```
These results show three facts:
1. According to the second/third or sixth/seventh results in the first experiment, if we use `Platform.get/putInt(Object)`, we achieve more than 2x worse performance than `Platform.get/putInt(byte[])` with concrete type (i.e. `byte[]`).
2. According to the second/third or fourth/fifth/sixth results in the first experiment, the fastest way to access an array element on Java heap is `array[]`. **Cons of `array[]` is that it is not possible to support unaligned-8byte access.**
3. According to the first/second/third or fourth/sixth/seventh results in the first experiment, `getInt()/putInt() or getLong()/putLong()` in subclasses of `MemoryBlock` can achieve comparable performance to `Platform.get/putInt()` or `Platform.get/putLong()` with concrete type (second or sixth result). There is no overhead regarding virtual call.
4. According to results in the second experiment, for `Platform.copy()`, to pass `Object` can achieve the same performance as to pass any type of primitive array as source or destination.
5. According to second/fourth results in the second experiment, `Platform.copy()` can achieve the same performance as `System.arrayCopy`. **It would be good to use `Platform.copy()` since `Platform.copy()` can take any types for src and dst.**
We are incrementally replace `Platform.get/putXXX` with `MemoryBlock.get/putXXX`. This is because we have two advantages.
1) Achieve better performance due to having a concrete type for an array.
2) Use simple OO design instead of passing `Object`
It is easy to use `MemoryBlock` in `InternalRow`, `BufferHolder`, `TaskMemoryManager`, and others that are already abstracted. It is not easy to use `MemoryBlock` in utility classes related to hashing or others.
Other candidates are
- UnsafeRow, UnsafeArrayData, UnsafeMapData, SpecificUnsafeRowJoiner
- UTF8StringBuffer
- BufferHolder
- TaskMemoryManager
- OnHeapColumnVector
- BytesToBytesMap
- CachedBatch
- classes for hash
- others.
## How was this patch tested?
Added `UnsafeMemoryAllocator`
Author: Kazuaki Ishizaki <ishizaki@jp.ibm.com>
Closes#19222 from kiszk/SPARK-10399.
## What changes were proposed in this pull request?
Add interpreted execution for `InitializeJavaBean` expression.
## How was this patch tested?
Added unit test.
Author: Liang-Chi Hsieh <viirya@gmail.com>
Closes#20985 from viirya/SPARK-23593-2.
## What changes were proposed in this pull request?
This pr added interpreted execution for `StaticInvoke`.
## How was this patch tested?
Added tests in `ObjectExpressionsSuite`.
Author: Kazuaki Ishizaki <ishizaki@jp.ibm.com>
Closes#20753 from kiszk/SPARK-23582.
## What changes were proposed in this pull request?
Add interpreted execution for `InitializeJavaBean` expression.
## How was this patch tested?
Added unit test.
Author: Liang-Chi Hsieh <viirya@gmail.com>
Closes#20756 from viirya/SPARK-23593.
## What changes were proposed in this pull request?
This pr added interpreted execution for `Invoke`.
## How was this patch tested?
Added tests in `ObjectExpressionsSuite`.
Author: Kazuaki Ishizaki <ishizaki@jp.ibm.com>
Closes#20797 from kiszk/SPARK-28583.
## What changes were proposed in this pull request?
In TestHive, the base spark session does this in getOrCreate(), we emulate that behavior for tests.
## How was this patch tested?
N/A
Author: gatorsmile <gatorsmile@gmail.com>
Closes#20969 from gatorsmile/setDefault.
## What changes were proposed in this pull request?
Add cast to nulls introduced by PropagateEmptyRelation so in cases they're part of coalesce they will not break its type checking rules
## How was this patch tested?
Added unit test
Author: Robert Kruszewski <robertk@palantir.com>
Closes#20914 from robert3005/rk/propagate-empty-fix.
## What changes were proposed in this pull request?
Currently, the active spark session is set inconsistently (e.g., in createDataFrame, prior to query execution). Many places in spark also incorrectly query active session when they should be calling activeSession.getOrElse(defaultSession) and so might get None even if a Spark session exists.
The semantics here can be cleaned up if we also set the active session when the default session is set.
Related: https://github.com/apache/spark/pull/20926/files
## How was this patch tested?
Unit test, existing test. Note that if https://github.com/apache/spark/pull/20926 merges first we should also update the tests there.
Author: Eric Liang <ekl@databricks.com>
Closes#20927 from ericl/active-session-cleanup.
## What changes were proposed in this pull request?
Add interpreted execution for `MapObjects` expression.
## How was this patch tested?
Added unit test.
Author: Liang-Chi Hsieh <viirya@gmail.com>
Closes#20771 from viirya/SPARK-23587.
## What changes were proposed in this pull request?
Migrate foreach sink to DataSourceV2.
Since the previous attempt at this PR #20552, we've changed and strictly defined the lifecycle of writer components. This means we no longer need the complicated lifecycle shim from that PR; it just naturally works.
## How was this patch tested?
existing tests
Author: Jose Torres <torres.joseph.f+github@gmail.com>
Closes#20951 from jose-torres/foreach.
## What changes were proposed in this pull request?
This PR implemented the following cleanups related to `UnsafeWriter` class:
- Remove code duplication between `UnsafeRowWriter` and `UnsafeArrayWriter`
- Make `BufferHolder` class internal by delegating its accessor methods to `UnsafeWriter`
- Replace `UnsafeRow.setTotalSize(...)` with `UnsafeRowWriter.setTotalSize()`
## How was this patch tested?
Tested by existing UTs
Author: Kazuaki Ishizaki <ishizaki@jp.ibm.com>
Closes#20850 from kiszk/SPARK-23713.
## What changes were proposed in this pull request?
Currently, the requiredChildDistribution does not specify the partitions. This can cause the weird corner cases where the child's distribution is `SinglePartition` which satisfies the required distribution of `ClusterDistribution(no-num-partition-requirement)`, thus eliminating the shuffle needed to repartition input data into the required number of partitions (i.e. same as state stores). That can lead to "file not found" errors on the state store delta files as the micro-batch-with-no-shuffle will not run certain tasks and therefore not generate the expected state store delta files.
This PR adds the required constraint on the number of partitions.
## How was this patch tested?
Modified test harness to always check that ANY stateful operator should have a constraint on the number of partitions. As part of that, the existing opt-in checks on child output partitioning were removed, as they are redundant.
Author: Tathagata Das <tathagata.das1565@gmail.com>
Closes#20941 from tdas/SPARK-23827.
## What changes were proposed in this pull request?
This PR is to improve the test coverage of the original PR https://github.com/apache/spark/pull/20687
## How was this patch tested?
N/A
Author: gatorsmile <gatorsmile@gmail.com>
Closes#20911 from gatorsmile/addTests.
## What changes were proposed in this pull request?
Roll forward c68ec4e (#20688).
There are two minor test changes required:
* An error which used to be TreeNodeException[ArithmeticException] is no longer wrapped and is now just ArithmeticException.
* The test framework simply does not set the active Spark session. (Or rather, it doesn't do so early enough - I think it only happens when a query is analyzed.) I've added the required logic to SQLTestUtils.
## How was this patch tested?
existing tests
Author: Jose Torres <torres.joseph.f+github@gmail.com>
Author: jerryshao <sshao@hortonworks.com>
Closes#20922 from jose-torres/ratefix.
## What changes were proposed in this pull request?
This PR supports for pushing down filters for DateType in parquet
## How was this patch tested?
Added UT and tested in local.
Author: yucai <yyu1@ebay.com>
Closes#20851 from yucai/SPARK-23727.
## What changes were proposed in this pull request?
isSharedClass returns if some classes can/should be shared or not. It checks if the classes names have some keywords or start with some names. Following the logic, it can occur unintended behaviors when a custom package has `slf4j` inside the package or class name. As I guess, the first intention seems to figure out the class containing `org.slf4j`. It would be better to change the comparison logic to `name.startsWith("org.slf4j")`
## How was this patch tested?
This patch should pass all of the current tests and keep all of the current behaviors. In my case, I'm using ProtobufDeserializer to get a table schema from hive tables. Thus some Protobuf packages and names have `slf4j` inside. Without this patch, it cannot be resolved because of ClassCastException from different classloaders.
Author: Jongyoul Lee <jongyoul@gmail.com>
Closes#20860 from jongyoul/SPARK-23743.
## What changes were proposed in this pull request?
Set default Spark session in the TestSparkSession and TestHiveSparkSession constructors.
## How was this patch tested?
new unit tests
Author: Jose Torres <torres.joseph.f+github@gmail.com>
Closes#20926 from jose-torres/test3.
## What changes were proposed in this pull request?
In SparkSQLCLI, SessionState generates before SparkContext instantiating. When we use --proxy-user to impersonate, it's unable to initializing a metastore client to talk to the secured metastore for no kerberos ticket.
This PR use real user ugi to obtain token for owner before talking to kerberized metastore.
## How was this patch tested?
Manually verified with kerberized hive metasotre / hdfs.
Author: Kent Yao <yaooqinn@hotmail.com>
Closes#20784 from yaooqinn/SPARK-23639.
## What changes were proposed in this pull request?
This PR proposes to add lineSep option for a configurable line separator in text datasource.
It supports this option by using `LineRecordReader`'s functionality with passing it to the constructor.
The approach is similar with https://github.com/apache/spark/pull/20727; however, one main difference is, it uses text datasource's `lineSep` option to parse line by line in JSON's schema inference.
## How was this patch tested?
Manually tested and unit tests were added.
Author: hyukjinkwon <gurwls223@apache.org>
Author: hyukjinkwon <gurwls223@gmail.com>
Closes#20877 from HyukjinKwon/linesep-json.
## What changes were proposed in this pull request?
This PR migrate micro batch rate source to V2 API and rewrite UTs to suite V2 test.
## How was this patch tested?
UTs.
Author: jerryshao <sshao@hortonworks.com>
Closes#20688 from jerryshao/SPARK-23096.
## What changes were proposed in this pull request?
The UUID() expression is stateful and should implement the `Stateful` trait instead of the `Nondeterministic` trait.
## How was this patch tested?
Added test.
Author: Liang-Chi Hsieh <viirya@gmail.com>
Closes#20912 from viirya/SPARK-23794.
## What changes were proposed in this pull request?
This PR fixes an incorrect comparison in SQL between timestamp and date. This is because both of them are casted to `string` and then are compared lexicographically. This implementation shows `false` regarding this query `spark.sql("select cast('2017-03-01 00:00:00' as timestamp) between cast('2017-02-28' as date) and cast('2017-03-01' as date)").show`.
This PR shows `true` for this query by casting `date("2017-03-01")` to `timestamp("2017-03-01 00:00:00")`.
(Please fill in changes proposed in this fix)
## How was this patch tested?
Added new UTs to `TypeCoercionSuite`.
Author: Kazuaki Ishizaki <ishizaki@jp.ibm.com>
Closes#20774 from kiszk/SPARK-23549.
## What changes were proposed in this pull request?
This pr added TPCDS v2.7 (latest) queries in `TPCDSQuerySuite` because the current `TPCDSQuerySuite` tests older one (v1.4) and some queries are different from v1.4 and v2.7. Since the original v2.7 queries have the syntaxes that Spark cannot parse, I changed these queries in a following way:
- [date] + 14 days -> date + `INTERVAL` 14 days
- [column name] as "30 days" -> [column name] as \`30 days\`
- Fix some syntax errors, e.g., missing brackets
## How was this patch tested?
Added tests in `TPCDSQuerySuite`.
Author: Takeshi Yamamuro <yamamuro@apache.org>
Closes#20343 from maropu/TPCDSV2_7.
## What changes were proposed in this pull request?
The serializability test uses the same MemoryStream instance for 3 different queries. If any of those queries ask it to commit before the others have run, the rest will see empty dataframes. This can fail the test if q3 is affected.
We should use one instance per query instead.
## How was this patch tested?
Existing unit test. If I move q2.processAllAvailable() before starting q3, the test always fails without the fix.
Author: Jose Torres <torres.joseph.f+github@gmail.com>
Closes#20896 from jose-torres/fixrace.
## What changes were proposed in this pull request?
We should provide customized canonicalize plan for `InMemoryRelation` and `InMemoryTableScanExec`. Otherwise, we can wrongly treat two different cached plans as same result. It causes wrongly reused exchange then.
For a test query like this:
```scala
val cached = spark.createDataset(Seq(TestDataUnion(1, 2, 3), TestDataUnion(4, 5, 6))).cache()
val group1 = cached.groupBy("x").agg(min(col("y")) as "value")
val group2 = cached.groupBy("x").agg(min(col("z")) as "value")
group1.union(group2)
```
Canonicalized plans before:
First exchange:
```
Exchange hashpartitioning(none#0, 5)
+- *(1) HashAggregate(keys=[none#0], functions=[partial_min(none#1)], output=[none#0, none#4])
+- *(1) InMemoryTableScan [none#0, none#1]
+- InMemoryRelation [x#4253, y#4254, z#4255], true, 10000, StorageLevel(disk, memory, deserialized, 1 replicas)
+- LocalTableScan [x#4253, y#4254, z#4255]
```
Second exchange:
```
Exchange hashpartitioning(none#0, 5)
+- *(3) HashAggregate(keys=[none#0], functions=[partial_min(none#1)], output=[none#0, none#4])
+- *(3) InMemoryTableScan [none#0, none#1]
+- InMemoryRelation [x#4253, y#4254, z#4255], true, 10000, StorageLevel(disk, memory, deserialized, 1 replicas)
+- LocalTableScan [x#4253, y#4254, z#4255]
```
You can find that they have the canonicalized plans are the same, although we use different columns in two `InMemoryTableScan`s.
Canonicalized plan after:
First exchange:
```
Exchange hashpartitioning(none#0, 5)
+- *(1) HashAggregate(keys=[none#0], functions=[partial_min(none#1)], output=[none#0, none#4])
+- *(1) InMemoryTableScan [none#0, none#1]
+- InMemoryRelation [none#0, none#1, none#2], true, 10000, StorageLevel(memory, 1 replicas)
+- LocalTableScan [none#0, none#1, none#2]
```
Second exchange:
```
Exchange hashpartitioning(none#0, 5)
+- *(3) HashAggregate(keys=[none#0], functions=[partial_min(none#1)], output=[none#0, none#4])
+- *(3) InMemoryTableScan [none#0, none#2]
+- InMemoryRelation [none#0, none#1, none#2], true, 10000, StorageLevel(memory, 1 replicas)
+- LocalTableScan [none#0, none#1, none#2]
```
## How was this patch tested?
Added unit test.
Author: Liang-Chi Hsieh <viirya@gmail.com>
Closes#20831 from viirya/SPARK-23614.
## What changes were proposed in this pull request?
As stated in Jira, there are problems with current `Uuid` expression which uses `java.util.UUID.randomUUID` for UUID generation.
This patch uses the newly added `RandomUUIDGenerator` for UUID generation. So we can make `Uuid` deterministic between retries.
## How was this patch tested?
Added unit tests.
Author: Liang-Chi Hsieh <viirya@gmail.com>
Closes#20861 from viirya/SPARK-23599-2.
## What changes were proposed in this pull request?
Currently we allow writing data frames with empty schema into a file based datasource for certain file formats such as JSON, ORC etc. For formats such as Parquet and Text, we raise error at different times of execution. For text format, we return error from the driver early on in processing where as for format such as parquet, the error is raised from executor.
**Example**
spark.emptyDataFrame.write.format("parquet").mode("overwrite").save(path)
**Results in**
``` SQL
org.apache.parquet.schema.InvalidSchemaException: Cannot write a schema with an empty group: message spark_schema {
}
at org.apache.parquet.schema.TypeUtil$1.visit(TypeUtil.java:27)
at org.apache.parquet.schema.TypeUtil$1.visit(TypeUtil.java:37)
at org.apache.parquet.schema.MessageType.accept(MessageType.java:58)
at org.apache.parquet.schema.TypeUtil.checkValidWriteSchema(TypeUtil.java:23)
at org.apache.parquet.hadoop.ParquetFileWriter.<init>(ParquetFileWriter.java:225)
at org.apache.parquet.hadoop.ParquetOutputFormat.getRecordWriter(ParquetOutputFormat.java:342)
at org.apache.parquet.hadoop.ParquetOutputFormat.getRecordWriter(ParquetOutputFormat.java:302)
at org.apache.spark.sql.execution.datasources.parquet.ParquetOutputWriter.<init>(ParquetOutputWriter.scala:37)
at org.apache.spark.sql.execution.datasources.parquet.ParquetFileFormat$$anon$1.newInstance(ParquetFileFormat.scala:151)
at org.apache.spark.sql.execution.datasources.FileFormatWriter$SingleDirectoryWriteTask.newOutputWriter(FileFormatWriter.scala:376)
at org.apache.spark.sql.execution.datasources.FileFormatWriter$SingleDirectoryWriteTask.execute(FileFormatWriter.scala:387)
at org.apache.spark.sql.execution.datasources.FileFormatWriter$$anonfun$org$apache$spark$sql$execution$datasources$FileFormatWriter$$executeTask$3.apply(FileFormatWriter.scala:278)
at org.apache.spark.sql.execution.datasources.FileFormatWriter$$anonfun$org$apache$spark$sql$execution$datasources$FileFormatWriter$$executeTask$3.apply(FileFormatWriter.scala:276)
at org.apache.spark.util.Utils$.tryWithSafeFinallyAndFailureCallbacks(Utils.scala:1411)
at org.apache.spark.sql.execution.datasources.FileFormatWriter$.org$apache$spark$sql$execution$datasources$FileFormatWriter$$executeTask(FileFormatWriter.scala:281)
at org.apache.spark.sql.execution.datasources.FileFormatWriter$$anonfun$write$1.apply(FileFormatWriter.scala:206)
at org.apache.spark.sql.execution.datasources.FileFormatWriter$$anonfun$write$1.apply(FileFormatWriter.scala:205)
at org.apache.spark.scheduler.ResultTask.runTask(ResultTask.scala:87)
at org.apache.spark.scheduler.Task.run(Task.scala:109)
at org.apache.spark.executor.Executor$TaskRunner.run(Executor.scala:345)
at java.util.concurrent.ThreadPoolExecutor.runWorker(ThreadPoolExecutor.java:1142)
at java.util.concurrent.ThreadPoolExecutor$Worker.run(ThreadPoolExecutor.java:617)
at java.lang.Thread.run(Thread.
```
In this PR, we unify the error processing and raise error on attempt to write empty schema based dataframes into file based datasource (orc, parquet, text , csv, json etc) early on in the processing.
## How was this patch tested?
Unit tests added in FileBasedDatasourceSuite.
Author: Dilip Biswal <dbiswal@us.ibm.com>
Closes#20579 from dilipbiswal/spark-23372.
## What changes were proposed in this pull request?
Fixed `CodegenContext.withSubExprEliminationExprs()` so that it saves/restores CSE state correctly.
## How was this patch tested?
Added new unit test to verify that the old CSE state is indeed saved and restored around the `withSubExprEliminationExprs()` call. Manually verified that this test fails without this patch.
Author: Kris Mok <kris.mok@databricks.com>
Closes#20870 from rednaxelafx/codegen-subexpr-fix.
## What changes were proposed in this pull request?
Output metrics were not filled when parquet sink used.
This PR fixes this problem by passing a `BasicWriteJobStatsTracker` in `FileStreamSink`.
## How was this patch tested?
Additional unit test added.
Author: Gabor Somogyi <gabor.g.somogyi@gmail.com>
Closes#20745 from gaborgsomogyi/SPARK-23288.
## What changes were proposed in this pull request?
To fix `scala.MatchError` in `literals.sql.out`, this pr added an entry for `CalendarIntervalType` in `QueryExecution.toHiveStructString`.
## How was this patch tested?
Existing tests and added tests in `literals.sql`
Author: Takeshi Yamamuro <yamamuro@apache.org>
Closes#20872 from maropu/FixIntervalTests.
## What changes were proposed in this pull request?
This PR proposes to add `lineSep` option for a configurable line separator in text datasource.
It supports this option by using `LineRecordReader`'s functionality with passing it to the constructor.
## How was this patch tested?
Manual tests and unit tests were added.
Author: hyukjinkwon <gurwls223@gmail.com>
Closes#20727 from HyukjinKwon/linesep-text.
## What changes were proposed in this pull request?
To drop `exprId`s for `Alias` in user-facing info., this pr added an entry for `Alias` in `NonSQLExpression.sql`
## How was this patch tested?
Added tests in `UDFSuite`.
Author: Takeshi Yamamuro <yamamuro@apache.org>
Closes#20827 from maropu/SPARK-23666.
## What changes were proposed in this pull request?
Complex type simplification optimizer rules were not applied to the
entire plan, just the expressions reachable from the root node. This
patch fixes the rules to transform the entire plan.
## How was this patch tested?
New unit test + ran sql / core tests.
Author: Henry Robinson <henry@apache.org>
Author: Henry Robinson <henry@cloudera.com>
Closes#20687 from henryr/spark-25000.
## What changes were proposed in this pull request?
Report SinglePartition in DataSourceV2ScanExec when there's exactly 1 data reader factory.
Note that this means reader factories end up being constructed as partitioning is checked; let me know if you think that could be a problem.
## How was this patch tested?
existing unit tests
Author: Jose Torres <jose@databricks.com>
Author: Jose Torres <torres.joseph.f+github@gmail.com>
Closes#20726 from jose-torres/SPARK-23574.
## What changes were proposed in this pull request?
This patch adds a UUID generator from Pseudo-Random Numbers. We can use it later to have deterministic `UUID()` expression.
## How was this patch tested?
Added unit tests.
Author: Liang-Chi Hsieh <viirya@gmail.com>
Closes#20817 from viirya/SPARK-23599.
## What changes were proposed in this pull request?
We currently can only create unsafe rows using code generation. This is a problem for situations in which code generation fails. There is no fallback, and as a result we cannot execute the query.
This PR adds an interpreted version of `UnsafeProjection`. The implementation is modeled after `InterpretedMutableProjection`. It stores the expression results in a `GenericInternalRow`, and it then uses a conversion function to convert the `GenericInternalRow` into an `UnsafeRow`.
This PR does not implement the actual code generated to interpreted fallback logic. This will be done in a follow-up.
## How was this patch tested?
I am piggybacking on exiting `UnsafeProjection` tests, and I have added an interpreted version for each of these.
Author: Herman van Hovell <hvanhovell@databricks.com>
Closes#20750 from hvanhovell/SPARK-23581.
## What changes were proposed in this pull request?
Currently, some tests have an assumption that `spark.sql.sources.default=parquet`. In fact, that is a correct assumption, but that assumption makes it difficult to test new data source format.
This PR aims to
- Improve test suites more robust and makes it easy to test new data sources in the future.
- Test new native ORC data source with the full existing Apache Spark test coverage.
As an example, the PR uses `spark.sql.sources.default=orc` during reviews. The value should be `parquet` when this PR is accepted.
## How was this patch tested?
Pass the Jenkins with updated tests.
Author: Dongjoon Hyun <dongjoon@apache.org>
Closes#20705 from dongjoon-hyun/SPARK-23553.
Clean up SparkPlanGraphWrapper objects from InMemoryStore together with cleaning up SQLExecutionUIData
existing unit test was extended to check also SparkPlanGraphWrapper object count
vanzin
Author: myroslavlisniak <acnipin@gmail.com>
Closes#20813 from myroslavlisniak/master.
## What changes were proposed in this pull request?
As discussion in #20675, we need add a new interface `ContinuousDataReaderFactory` to support the requirements of setting start offset in Continuous Processing.
## How was this patch tested?
Existing UT.
Author: Yuanjian Li <xyliyuanjian@gmail.com>
Closes#20689 from xuanyuanking/SPARK-23533.
## What changes were proposed in this pull request?
This PR fixes a minor issue in `HadoopFsRelationTest`, that you should create table using `dataSourceName` instead of `parquet`. The issue won't affect the correctness, but it will generate wrong error message in case the test fails.
## How was this patch tested?
Exsiting tests.
Author: Xingbo Jiang <xingbo.jiang@databricks.com>
Closes#20780 from jiangxb1987/dataSourceName.
## What changes were proposed in this pull request?
This PR enables assertions in `XXH64Suite.testKnownByteArrayInputs()` on big endian platform, too. The current implementation performs them only on little endian platform. This PR increase test coverage of big endian platform.
## How was this patch tested?
Updated `XXH64Suite`
Tested on big endian platform using JIT compiler or interpreter `-Xint`.
Author: Kazuaki Ishizaki <ishizaki@jp.ibm.com>
Closes#20804 from kiszk/SPARK-23656.
## What changes were proposed in this pull request?
The error message ```s"""Field "$name" does not exist."""``` is thrown when looking up an unknown field in StructType. In the error message, we should also contain the information about which columns/fields exist in this struct.
## How was this patch tested?
Added new unit tests.
Note: I created a new `StructTypeSuite.scala` as I couldn't find an existing suite that's suitable to place these tests. I may be missing something so feel free to propose new locations.
Please review http://spark.apache.org/contributing.html before opening a pull request.
Author: Xiayun Sun <xiayunsun@gmail.com>
Closes#20649 from xysun/SPARK-23462.
## What changes were proposed in this pull request?
Revise doc of method pushFilters in SupportsPushDownFilters/SupportsPushDownCatalystFilters
In `FileSourceStrategy`, except `partitionKeyFilters`(the references of which is subset of partition keys), all filters needs to be evaluated after scanning. Otherwise, Spark will get wrong result from data sources like Orc/Parquet.
This PR is to improve the doc.
Author: Wang Gengliang <gengliang.wang@databricks.com>
Closes#20769 from gengliangwang/revise_pushdown_doc.
## What changes were proposed in this pull request?
The from_json() function accepts an additional parameter, where the user might specify the schema. The issue is that the specified schema might not be compatible with data. In particular, the JSON data might be missing data for fields declared as non-nullable in the schema. The from_json() function does not verify the data against such errors. When data with missing fields is sent to the parquet encoder, there is no verification either. The end results is a corrupt parquet file.
To avoid corruptions, make sure that all fields in the user-specified schema are set to be nullable.
Since this changes the behavior of a public function, we need to include it in release notes.
The behavior can be reverted by setting `spark.sql.fromJsonForceNullableSchema=false`
## How was this patch tested?
Added two new tests.
Author: Michał Świtakowski <michal.switakowski@databricks.com>
Closes#20694 from mswit-databricks/SPARK-23173.
## What changes were proposed in this pull request?
Below are the two cases.
``` SQL
case 1
scala> List.empty[String].toDF().rdd.partitions.length
res18: Int = 1
```
When we write the above data frame as parquet, we create a parquet file containing
just the schema of the data frame.
Case 2
``` SQL
scala> val anySchema = StructType(StructField("anyName", StringType, nullable = false) :: Nil)
anySchema: org.apache.spark.sql.types.StructType = StructType(StructField(anyName,StringType,false))
scala> spark.read.schema(anySchema).csv("/tmp/empty_folder").rdd.partitions.length
res22: Int = 0
```
For the 2nd case, since number of partitions = 0, we don't call the write task (the task has logic to create the empty metadata only parquet file)
The fix is to create a dummy single partition RDD and set up the write task based on it to ensure
the metadata-only file.
## How was this patch tested?
A new test is added to DataframeReaderWriterSuite.
Author: Dilip Biswal <dbiswal@us.ibm.com>
Closes#20525 from dilipbiswal/spark-23271.
## What changes were proposed in this pull request?
`PrintToStderr` was doing what is it supposed to only when code generation is enabled.
The PR adds the same behavior in interpreted mode too.
## How was this patch tested?
added UT
Author: Marco Gaido <marcogaido91@gmail.com>
Closes#20773 from mgaido91/SPARK-23602.
## What changes were proposed in this pull request?
There was a bug in `calculateParamLength` which caused it to return always 1 + the number of expressions. This could lead to Exceptions especially with expressions of type long.
## How was this patch tested?
added UT + fixed previous UT
Author: Marco Gaido <marcogaido91@gmail.com>
Closes#20772 from mgaido91/SPARK-23628.
## What changes were proposed in this pull request?
The PR adds interpreted execution to DecodeUsingSerializer.
## How was this patch tested?
added UT
Please review http://spark.apache.org/contributing.html before opening a pull request.
Author: Marco Gaido <marcogaido91@gmail.com>
Closes#20760 from mgaido91/SPARK-23592.
## What changes were proposed in this pull request?
This PR proposes to support an alternative function from with group aggregate pandas UDF.
The current form:
```
def foo(pdf):
return ...
```
Takes a single arg that is a pandas DataFrame.
With this PR, an alternative form is supported:
```
def foo(key, pdf):
return ...
```
The alternative form takes two argument - a tuple that presents the grouping key, and a pandas DataFrame represents the data.
## How was this patch tested?
GroupbyApplyTests
Author: Li Jin <ice.xelloss@gmail.com>
Closes#20295 from icexelloss/SPARK-23011-groupby-apply-key.
## What changes were proposed in this pull request?
This PR adds a configuration to control the fallback of Arrow optimization for `toPandas` and `createDataFrame` with Pandas DataFrame.
## How was this patch tested?
Manually tested and unit tests added.
You can test this by:
**`createDataFrame`**
```python
spark.conf.set("spark.sql.execution.arrow.enabled", False)
pdf = spark.createDataFrame([[{'a': 1}]]).toPandas()
spark.conf.set("spark.sql.execution.arrow.enabled", True)
spark.conf.set("spark.sql.execution.arrow.fallback.enabled", True)
spark.createDataFrame(pdf, "a: map<string, int>")
```
```python
spark.conf.set("spark.sql.execution.arrow.enabled", False)
pdf = spark.createDataFrame([[{'a': 1}]]).toPandas()
spark.conf.set("spark.sql.execution.arrow.enabled", True)
spark.conf.set("spark.sql.execution.arrow.fallback.enabled", False)
spark.createDataFrame(pdf, "a: map<string, int>")
```
**`toPandas`**
```python
spark.conf.set("spark.sql.execution.arrow.enabled", True)
spark.conf.set("spark.sql.execution.arrow.fallback.enabled", True)
spark.createDataFrame([[{'a': 1}]]).toPandas()
```
```python
spark.conf.set("spark.sql.execution.arrow.enabled", True)
spark.conf.set("spark.sql.execution.arrow.fallback.enabled", False)
spark.createDataFrame([[{'a': 1}]]).toPandas()
```
Author: hyukjinkwon <gurwls223@gmail.com>
Closes#20678 from HyukjinKwon/SPARK-23380-conf.
## What changes were proposed in this pull request?
The following query doesn't work as expected:
```
CREATE EXTERNAL TABLE ext_table(a STRING, b INT, c STRING) PARTITIONED BY (d STRING)
LOCATION 'sql/core/spark-warehouse/ext_table';
ALTER TABLE ext_table CHANGE a a STRING COMMENT "new comment";
DESC ext_table;
```
The comment of column `a` is not updated, that's because `HiveExternalCatalog.doAlterTable` ignores table schema changes. To fix the issue, we should call `doAlterTableDataSchema` instead of `doAlterTable`.
## How was this patch tested?
Updated `DDLSuite.testChangeColumn`.
Author: Xingbo Jiang <xingbo.jiang@databricks.com>
Closes#20696 from jiangxb1987/alterColumnComment.
A few different things going on:
- Remove unused methods.
- Move JSON methods to the only class that uses them.
- Move test-only methods to TestUtils.
- Make getMaxResultSize() a config constant.
- Reuse functionality from existing libraries (JRE or JavaUtils) where possible.
The change also includes changes to a few tests to call `Utils.createTempFile` correctly,
so that temp dirs are created under the designated top-level temp dir instead of
potentially polluting git index.
Author: Marcelo Vanzin <vanzin@cloudera.com>
Closes#20706 from vanzin/SPARK-23550.
## What changes were proposed in this pull request?
The PR adds interpreted execution to EncodeUsingSerializer.
## How was this patch tested?
added UT
Author: Marco Gaido <marcogaido91@gmail.com>
Closes#20751 from mgaido91/SPARK-23591.
## What changes were proposed in this pull request?
This pr added a helper function in `ExpressionEvalHelper` to check exceptions in all the path of expression evaluation.
## How was this patch tested?
Modified the existing tests.
Author: Takeshi Yamamuro <yamamuro@apache.org>
Closes#20748 from maropu/SPARK-23611.
## What changes were proposed in this pull request?
The PR adds interpreted execution to CreateExternalRow
## How was this patch tested?
added UT
Author: Marco Gaido <marcogaido91@gmail.com>
Closes#20749 from mgaido91/SPARK-23590.
## What changes were proposed in this pull request?
This pr added interpreted execution for `GetExternalRowField`.
## How was this patch tested?
Added tests in `ObjectExpressionsSuite`.
Author: Takeshi Yamamuro <yamamuro@apache.org>
Closes#20746 from maropu/SPARK-23594.
…lValue
## What changes were proposed in this pull request?
Parquet 1.9 will change the semantics of Statistics.isEmpty slightly
to reflect if the null value count has been set. That breaks a
timestamp interoperability test that cares only about whether there
are column values present in the statistics of a written file for an
INT96 column. Fix by using Statistics.hasNonNullValue instead.
## How was this patch tested?
Unit tests continue to pass against Parquet 1.8, and also pass against
a Parquet build including PARQUET-1217.
Author: Henry Robinson <henry@cloudera.com>
Closes#20740 from henryr/spark-23604.
## What changes were proposed in this pull request?
The PR adds interpreted execution to WrapOption.
## How was this patch tested?
added UT
Author: Marco Gaido <marcogaido91@gmail.com>
Closes#20741 from mgaido91/SPARK-23586_2.
## What changes were proposed in this pull request?
Add an epoch ID argument to DataWriterFactory for use in streaming. As a side effect of passing in this value, DataWriter will now have a consistent lifecycle; commit() or abort() ends the lifecycle of a DataWriter instance in any execution mode.
I considered making a separate streaming interface and adding the epoch ID only to that one, but I think it requires a lot of extra work for no real gain. I think it makes sense to define epoch 0 as the one and only epoch of a non-streaming query.
## How was this patch tested?
existing unit tests
Author: Jose Torres <jose@databricks.com>
Closes#20710 from jose-torres/api2.
## What changes were proposed in this pull request?
The PR adds interpreted execution to UnwrapOption.
## How was this patch tested?
added UT
Author: Marco Gaido <marcogaido91@gmail.com>
Closes#20736 from mgaido91/SPARK-23586.
## What changes were proposed in this pull request?
Provide more details in trigonometric function documentations. Referenced `java.lang.Math` for further details in the descriptions.
## How was this patch tested?
Ran full build, checked generated documentation manually
Author: Mihaly Toth <misutoth@gmail.com>
Closes#20618 from misutoth/trigonometric-doc.
## What changes were proposed in this pull request?
A current `CodegenContext` class has immutable value or method without mutable state, too.
This refactoring moves them to `CodeGenerator` object class which can be accessed from anywhere without an instantiated `CodegenContext` in the program.
## How was this patch tested?
Existing tests
Author: Kazuaki Ishizaki <ishizaki@jp.ibm.com>
Closes#20700 from kiszk/SPARK-23546.
## What changes were proposed in this pull request?
It looks like this was incorrectly copied from `XPathFloat` in the class above.
## How was this patch tested?
(Please explain how this patch was tested. E.g. unit tests, integration tests, manual tests)
(If this patch involves UI changes, please attach a screenshot; otherwise, remove this)
Please review http://spark.apache.org/contributing.html before opening a pull request.
Author: Eric Liang <ekhliang@gmail.com>
Closes#20730 from ericl/fix-typo-xpath.
## What changes were proposed in this pull request?
In https://github.com/apache/spark/pull/20679 I missed a few places in SQL tests.
For hygiene, they should also use the sessionState interface where possible.
## How was this patch tested?
Modified existing tests.
Author: Juliusz Sompolski <julek@databricks.com>
Closes#20718 from juliuszsompolski/SPARK-23514-followup.
## What changes were proposed in this pull request?
Add Spark 2.3.0 in HiveExternalCatalogVersionsSuite since Spark 2.3.0 is released for ensuring backward compatibility.
## How was this patch tested?
N/A
Author: gatorsmile <gatorsmile@gmail.com>
Closes#20720 from gatorsmile/add2.3.
## What changes were proposed in this pull request?
This PR moves structured streaming text socket source to V2.
Questions: do we need to remove old "socket" source?
## How was this patch tested?
Unit test and manual verification.
Author: jerryshao <sshao@hortonworks.com>
Closes#20382 from jerryshao/SPARK-23097.
## What changes were proposed in this pull request?
https://github.com/apache/spark/pull/18944 added one patch, which allowed a spark session to be created when the hive metastore server is down. However, it did not allow running any commands with the spark session. This brings troubles to the user who only wants to read / write data frames without metastore setup.
## How was this patch tested?
Added some unit tests to read and write data frames based on the original HiveMetastoreLazyInitializationSuite.
Please review http://spark.apache.org/contributing.html before opening a pull request.
Author: Feng Liu <fengliu@databricks.com>
Closes#20681 from liufengdb/completely-lazy.
## What changes were proposed in this pull request?
(Please fill in changes proposed in this fix)
I run a sql: `select ls.cs_order_number from ls left semi join catalog_sales cs on ls.cs_order_number = cs.cs_order_number`, The `ls` table is a small table ,and the number is one. The `catalog_sales` table is a big table, and the number is 10 billion. The task will be hang up. And i find the many null values of `cs_order_number` in the `catalog_sales` table. I think the null value should be removed in the logical plan.
>== Optimized Logical Plan ==
>Join LeftSemi, (cs_order_number#1 = cs_order_number#22)
>:- Project cs_order_number#1
> : +- Filter isnotnull(cs_order_number#1)
> : +- MetastoreRelation 100t, ls
>+- Project cs_order_number#22
> +- MetastoreRelation 100t, catalog_sales
Now, use this patch, the plan will be:
>== Optimized Logical Plan ==
>Join LeftSemi, (cs_order_number#1 = cs_order_number#22)
>:- Project cs_order_number#1
> : +- Filter isnotnull(cs_order_number#1)
> : +- MetastoreRelation 100t, ls
>+- Project cs_order_number#22
> : **+- Filter isnotnull(cs_order_number#22)**
> :+- MetastoreRelation 100t, catalog_sales
## How was this patch tested?
(Please explain how this patch was tested. E.g. unit tests, integration tests, manual tests)
(If this patch involves UI changes, please attach a screenshot; otherwise, remove this)
Please review http://spark.apache.org/contributing.html before opening a pull request.
Author: KaiXinXiaoLei <584620569@qq.com>
Author: hanghang <584620569@qq.com>
Closes#20670 from KaiXinXiaoLei/Spark-23405.
## What changes were proposed in this pull request?
This is based on https://github.com/apache/spark/pull/20668 for supporting Hive 2.2 and Hive 2.3 metastore.
When we merge the PR, we should give the major credit to wangyum
## How was this patch tested?
Added the test cases
Author: Yuming Wang <yumwang@ebay.com>
Author: gatorsmile <gatorsmile@gmail.com>
Closes#20671 from gatorsmile/pr-20668.
## What changes were proposed in this pull request?
Inside `OptimizeMetadataOnlyQuery.getPartitionAttrs`, avoid using `zip` to generate attribute map.
Also include other minor update of comments and format.
## How was this patch tested?
Existing test cases.
Author: Xingbo Jiang <xingbo.jiang@databricks.com>
Closes#20693 from jiangxb1987/SPARK-23523.
## What changes were proposed in this pull request?
A few places in `spark-sql` were using `sc.hadoopConfiguration` directly. They should be using `sessionState.newHadoopConf()` to blend in configs that were set through `SQLConf`.
Also, for better UX, for these configs blended in from `SQLConf`, we should consider removing the `spark.hadoop` prefix, so that the settings are recognized whether or not they were specified by the user.
## How was this patch tested?
Tested that AlterTableRecoverPartitions now correctly recognizes settings that are passed in to the FileSystem through SQLConf.
Author: Juliusz Sompolski <julek@databricks.com>
Closes#20679 from juliuszsompolski/SPARK-23514.
## What changes were proposed in this pull request?
Clarify JSON and CSV reader behavior in document.
JSON doesn't support partial results for corrupted records.
CSV only supports partial results for the records with more or less tokens.
## How was this patch tested?
Pass existing tests.
Author: Liang-Chi Hsieh <viirya@gmail.com>
Closes#20666 from viirya/SPARK-23448-2.
## What changes were proposed in this pull request?
```Scala
val tablePath = new File(s"${path.getCanonicalPath}/cOl3=c/cOl1=a/cOl5=e")
Seq(("a", "b", "c", "d", "e")).toDF("cOl1", "cOl2", "cOl3", "cOl4", "cOl5")
.write.json(tablePath.getCanonicalPath)
val df = spark.read.json(path.getCanonicalPath).select("CoL1", "CoL5", "CoL3").distinct()
df.show()
```
It generates a wrong result.
```
[c,e,a]
```
We have a bug in the rule `OptimizeMetadataOnlyQuery `. We should respect the attribute order in the original leaf node. This PR is to fix it.
## How was this patch tested?
Added a test case
Author: gatorsmile <gatorsmile@gmail.com>
Closes#20684 from gatorsmile/optimizeMetadataOnly.
## What changes were proposed in this pull request?
Refactor ColumnStat to be more flexible.
* Split `ColumnStat` and `CatalogColumnStat` just like `CatalogStatistics` is split from `Statistics`. This detaches how the statistics are stored from how they are processed in the query plan. `CatalogColumnStat` keeps `min` and `max` as `String`, making it not depend on dataType information.
* For `CatalogColumnStat`, parse column names from property names in the metastore (`KEY_VERSION` property), not from metastore schema. This means that `CatalogColumnStat`s can be created for columns even if the schema itself is not stored in the metastore.
* Make all fields optional. `min`, `max` and `histogram` for columns were optional already. Having them all optional is more consistent, and gives flexibility to e.g. drop some of the fields through transformations if they are difficult / impossible to calculate.
The added flexibility will make it possible to have alternative implementations for stats, and separates stats collection from stats and estimation processing in plans.
## How was this patch tested?
Refactored existing tests to work with refactored `ColumnStat` and `CatalogColumnStat`.
New tests added in `StatisticsSuite` checking that backwards / forwards compatibility is not broken.
Author: Juliusz Sompolski <julek@databricks.com>
Closes#20624 from juliuszsompolski/SPARK-23445.
## What changes were proposed in this pull request?
Remove queryExecutionThread.interrupt() from ContinuousExecution. As detailed in the JIRA, interrupting the thread is only relevant in the microbatch case; for continuous processing the query execution can quickly clean itself up without.
## How was this patch tested?
existing tests
Author: Jose Torres <jose@databricks.com>
Closes#20622 from jose-torres/SPARK-23441.
## What changes were proposed in this pull request?
This PR avoids to print schema internal information when unknown column is specified in partition columns. This PR prints column names in the schema with more readable format.
The following is an example.
Source code
```
test("save with an unknown partition column") {
withTempDir { dir =>
val path = dir.getCanonicalPath
Seq(1L -> "a").toDF("i", "j").write
.format("parquet")
.partitionBy("unknownColumn")
.save(path)
}
```
Output without this PR
```
Partition column unknownColumn not found in schema StructType(StructField(i,LongType,false), StructField(j,StringType,true));
```
Output with this PR
```
Partition column unknownColumn not found in schema struct<i:bigint,j:string>;
```
## How was this patch tested?
Manually tested
Author: Kazuaki Ishizaki <ishizaki@jp.ibm.com>
Closes#20653 from kiszk/SPARK-23459.
**The best way to review this PR is to ignore whitespace/indent changes. Use this link - https://github.com/apache/spark/pull/20650/files?w=1**
## What changes were proposed in this pull request?
The stream-stream join tests add data to multiple sources and expect it all to show up in the next batch. But there's a race condition; the new batch might trigger when only one of the AddData actions has been reached.
Prior attempt to solve this issue by jose-torres in #20646 attempted to simultaneously synchronize on all memory sources together when consecutive AddData was found in the actions. However, this carries the risk of deadlock as well as unintended modification of stress tests (see the above PR for a detailed explanation). Instead, this PR attempts the following.
- A new action called `StreamProgressBlockedActions` that allows multiple actions to be executed while the streaming query is blocked from making progress. This allows data to be added to multiple sources that are made visible simultaneously in the next batch.
- An alias of `StreamProgressBlockedActions` called `MultiAddData` is explicitly used in the `Streaming*JoinSuites` to add data to two memory sources simultaneously.
This should avoid unintentional modification of the stress tests (or any other test for that matter) while making sure that the flaky tests are deterministic.
## How was this patch tested?
Modified test cases in `Streaming*JoinSuites` where there are consecutive `AddData` actions.
Author: Tathagata Das <tathagata.das1565@gmail.com>
Closes#20650 from tdas/SPARK-23408.
## What changes were proposed in this pull request?
For CreateTable with Append mode, we should check if `storage.locationUri` is the same with existing table in `PreprocessTableCreation`
In the current code, there is only a simple exception if the `storage.locationUri` is different with existing table:
`org.apache.spark.sql.AnalysisException: Table or view not found:`
which can be improved.
## How was this patch tested?
Unit test
Author: Wang Gengliang <gengliang.wang@databricks.com>
Closes#20660 from gengliangwang/locationUri.
## What changes were proposed in this pull request?
DataSourceV2 initially allowed user-supplied schemas when a source doesn't implement `ReadSupportWithSchema`, as long as the schema was identical to the source's schema. This is confusing behavior because changes to an underlying table can cause a previously working job to fail with an exception that user-supplied schemas are not allowed.
This reverts commit adcb25a0624, which was added to #20387 so that it could be removed in a separate JIRA issue and PR.
## How was this patch tested?
Existing tests.
Author: Ryan Blue <blue@apache.org>
Closes#20603 from rdblue/SPARK-23418-revert-adcb25a0624.
## What changes were proposed in this pull request?
This PR always adds `codegenStageId` in comment of the generated class. This is a replication of #20419 for post-Spark 2.3.
Closes#20419
```
/* 001 */ public Object generate(Object[] references) {
/* 002 */ return new GeneratedIteratorForCodegenStage1(references);
/* 003 */ }
/* 004 */
/* 005 */ // codegenStageId=1
/* 006 */ final class GeneratedIteratorForCodegenStage1 extends org.apache.spark.sql.execution.BufferedRowIterator {
/* 007 */ private Object[] references;
...
```
## How was this patch tested?
Existing tests
Author: Kazuaki Ishizaki <ishizaki@jp.ibm.com>
Closes#20612 from kiszk/SPARK-23424.
## What changes were proposed in this pull request?
In a kerberized cluster, when Spark reads a file path (e.g. `people.json`), it warns with a wrong warning message during looking up `people.json/_spark_metadata`. The root cause of this situation is the difference between `LocalFileSystem` and `DistributedFileSystem`. `LocalFileSystem.exists()` returns `false`, but `DistributedFileSystem.exists` raises `org.apache.hadoop.security.AccessControlException`.
```scala
scala> spark.version
res0: String = 2.4.0-SNAPSHOT
scala> spark.read.json("file:///usr/hdp/current/spark-client/examples/src/main/resources/people.json").show
+----+-------+
| age| name|
+----+-------+
|null|Michael|
| 30| Andy|
| 19| Justin|
+----+-------+
scala> spark.read.json("hdfs:///tmp/people.json")
18/02/15 05:00:48 WARN streaming.FileStreamSink: Error while looking for metadata directory.
18/02/15 05:00:48 WARN streaming.FileStreamSink: Error while looking for metadata directory.
```
After this PR,
```scala
scala> spark.read.json("hdfs:///tmp/people.json").show
+----+-------+
| age| name|
+----+-------+
|null|Michael|
| 30| Andy|
| 19| Justin|
+----+-------+
```
## How was this patch tested?
Manual.
Author: Dongjoon Hyun <dongjoon@apache.org>
Closes#20616 from dongjoon-hyun/SPARK-23434.
## What changes were proposed in this pull request?
Apache Spark 2.3 introduced `native` ORC supports with vectorization and many fixes. However, it's shipped as a not-default option. This PR enables `native` ORC implementation and predicate-pushdown by default for Apache Spark 2.4. We will improve and stabilize ORC data source before Apache Spark 2.4. And, eventually, Apache Spark will drop old Hive-based ORC code.
## How was this patch tested?
Pass the Jenkins with existing tests.
Author: Dongjoon Hyun <dongjoon@apache.org>
Closes#20634 from dongjoon-hyun/SPARK-23456.
## What changes were proposed in this pull request?
SPARK-23203: DataSourceV2 should use immutable catalyst trees instead of wrapping a mutable DataSourceV2Reader. This commit updates DataSourceV2Relation and consolidates much of the DataSourceV2 API requirements for the read path in it. Instead of wrapping a reader that changes, the relation lazily produces a reader from its configuration.
This commit also updates the predicate and projection push-down. Instead of the implementation from SPARK-22197, this reuses the rule matching from the Hive and DataSource read paths (using `PhysicalOperation`) and copies most of the implementation of `SparkPlanner.pruneFilterProject`, with updates for DataSourceV2. By reusing the implementation from other read paths, this should have fewer regressions from other read paths and is less code to maintain.
The new push-down rules also supports the following edge cases:
* The output of DataSourceV2Relation should be what is returned by the reader, in case the reader can only partially satisfy the requested schema projection
* The requested projection passed to the DataSourceV2Reader should include filter columns
* The push-down rule may be run more than once if filters are not pushed through projections
## How was this patch tested?
Existing push-down and read tests.
Author: Ryan Blue <blue@apache.org>
Closes#20387 from rdblue/SPARK-22386-push-down-immutable-trees.
## What changes were proposed in this pull request?
Before the patch, Spark could infer as Date a partition value which cannot be casted to Date (this can happen when there are extra characters after a valid date, like `2018-02-15AAA`).
When this happens and the input format has metadata which define the schema of the table, then `null` is returned as a value for the partition column, because the `cast` operator used in (`PartitioningAwareFileIndex.inferPartitioning`) is unable to convert the value.
The PR checks in the partition inference that values can be casted to Date and Timestamp, in order to infer that datatype to them.
## How was this patch tested?
added UT
Author: Marco Gaido <marcogaido91@gmail.com>
Closes#20621 from mgaido91/SPARK-23436.
## What changes were proposed in this pull request?
ParquetFileFormat leaks opened files in some cases. This PR prevents that by registering task completion listers first before initialization.
- [spark-branch-2.3-test-sbt-hadoop-2.7](https://amplab.cs.berkeley.edu/jenkins/view/Spark%20QA%20Test%20(Dashboard)/job/spark-branch-2.3-test-sbt-hadoop-2.7/205/testReport/org.apache.spark.sql/FileBasedDataSourceSuite/_It_is_not_a_test_it_is_a_sbt_testing_SuiteSelector_/)
- [spark-master-test-sbt-hadoop-2.6](https://amplab.cs.berkeley.edu/jenkins/view/Spark%20QA%20Test%20(Dashboard)/job/spark-master-test-sbt-hadoop-2.6/4228/testReport/junit/org.apache.spark.sql.execution.datasources.parquet/ParquetQuerySuite/_It_is_not_a_test_it_is_a_sbt_testing_SuiteSelector_/)
```
Caused by: sbt.ForkMain$ForkError: java.lang.Throwable: null
at org.apache.spark.DebugFilesystem$.addOpenStream(DebugFilesystem.scala:36)
at org.apache.spark.DebugFilesystem.open(DebugFilesystem.scala:70)
at org.apache.hadoop.fs.FileSystem.open(FileSystem.java:769)
at org.apache.parquet.hadoop.ParquetFileReader.<init>(ParquetFileReader.java:538)
at org.apache.spark.sql.execution.datasources.parquet.SpecificParquetRecordReaderBase.initialize(SpecificParquetRecordReaderBase.java:149)
at org.apache.spark.sql.execution.datasources.parquet.VectorizedParquetRecordReader.initialize(VectorizedParquetRecordReader.java:133)
at org.apache.spark.sql.execution.datasources.parquet.ParquetFileFormat$$anonfun$buildReaderWithPartitionValues$1.apply(ParquetFileFormat.scala:400)
at
```
## How was this patch tested?
Manual. The following test case generates the same leakage.
```scala
test("SPARK-23457 Register task completion listeners first in ParquetFileFormat") {
withSQLConf(SQLConf.PARQUET_VECTORIZED_READER_BATCH_SIZE.key -> s"${Int.MaxValue}") {
withTempDir { dir =>
val basePath = dir.getCanonicalPath
Seq(0).toDF("a").write.format("parquet").save(new Path(basePath, "first").toString)
Seq(1).toDF("a").write.format("parquet").save(new Path(basePath, "second").toString)
val df = spark.read.parquet(
new Path(basePath, "first").toString,
new Path(basePath, "second").toString)
val e = intercept[SparkException] {
df.collect()
}
assert(e.getCause.isInstanceOf[OutOfMemoryError])
}
}
}
```
Author: Dongjoon Hyun <dongjoon@apache.org>
Closes#20619 from dongjoon-hyun/SPARK-23390.
## What changes were proposed in this pull request?
This PR updates Apache ORC dependencies to 1.4.3 released on February 9th. Apache ORC 1.4.2 release removes unnecessary dependencies and 1.4.3 has 5 more patches (https://s.apache.org/Fll8).
Especially, the following ORC-285 is fixed at 1.4.3.
```scala
scala> val df = Seq(Array.empty[Float]).toDF()
scala> df.write.format("orc").save("/tmp/floatarray")
scala> spark.read.orc("/tmp/floatarray")
res1: org.apache.spark.sql.DataFrame = [value: array<float>]
scala> spark.read.orc("/tmp/floatarray").show()
18/02/12 22:09:10 ERROR Executor: Exception in task 0.0 in stage 1.0 (TID 1)
java.io.IOException: Error reading file: file:/tmp/floatarray/part-00000-9c0b461b-4df1-4c23-aac1-3e4f349ac7d6-c000.snappy.orc
at org.apache.orc.impl.RecordReaderImpl.nextBatch(RecordReaderImpl.java:1191)
at org.apache.orc.mapreduce.OrcMapreduceRecordReader.ensureBatch(OrcMapreduceRecordReader.java:78)
...
Caused by: java.io.EOFException: Read past EOF for compressed stream Stream for column 2 kind DATA position: 0 length: 0 range: 0 offset: 0 limit: 0
```
## How was this patch tested?
Pass the Jenkins test.
Author: Dongjoon Hyun <dongjoon@apache.org>
Closes#20511 from dongjoon-hyun/SPARK-23340.
## What changes were proposed in this pull request?
Cleaned up the codegen templates for `Literal`s, to make sure that the `ExprCode` returned from `Literal.doGenCode()` has:
1. an empty `code` field;
2. an `isNull` field of either literal `true` or `false`;
3. a `value` field that is just a simple literal/constant.
Before this PR, there are a couple of paths that would return a non-trivial `code` and all of them are actually unnecessary. The `NaN` and `Infinity` constants for `double` and `float` can be accessed through constants directly available so there's no need to add a reference for them.
Also took the opportunity to add a new util method for ease of creating `ExprCode` for inline-able non-null values.
## How was this patch tested?
Existing tests.
Author: Kris Mok <kris.mok@databricks.com>
Closes#20626 from rednaxelafx/codegen-literal.
## What changes were proposed in this pull request?
Migrating KafkaSource (with data source v1) to KafkaMicroBatchReader (with data source v2).
Performance comparison:
In a unit test with in-process Kafka broker, I tested the read throughput of V1 and V2 using 20M records in a single partition. They were comparable.
## How was this patch tested?
Existing tests, few modified to be better tests than the existing ones.
Author: Tathagata Das <tathagata.das1565@gmail.com>
Closes#20554 from tdas/SPARK-23362.
## What changes were proposed in this pull request?
This replaces `Sparkcurrently` to `Spark currently` in the following error message.
```scala
scala> sql("insert into t2 select * from v1")
org.apache.spark.sql.AnalysisException: Output Hive table `default`.`t2`
is bucketed but Sparkcurrently does NOT populate bucketed ...
```
## How was this patch tested?
Manual.
Author: Dongjoon Hyun <dongjoon@apache.org>
Closes#20617 from dongjoon-hyun/SPARK-ERROR-MSG.
## What changes were proposed in this pull request?
To prevent any regressions, this PR changes ORC implementation to `hive` by default like Spark 2.2.X.
Users can enable `native` ORC. Also, ORC PPD is also restored to `false` like Spark 2.2.X.
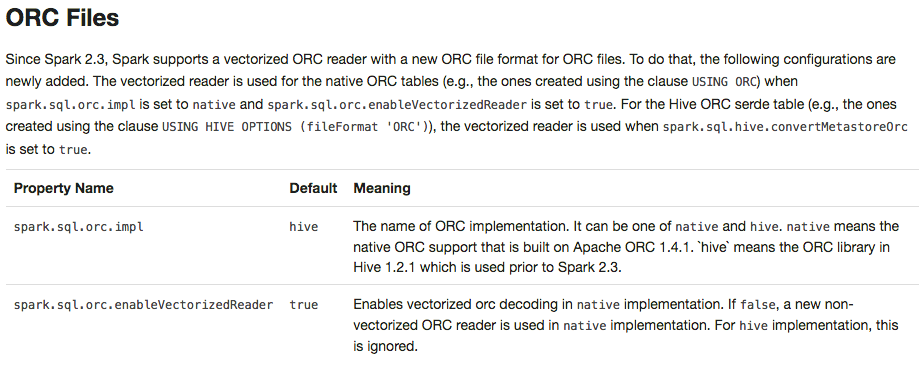
## How was this patch tested?
Pass all test cases.
Author: Dongjoon Hyun <dongjoon@apache.org>
Closes#20610 from dongjoon-hyun/SPARK-ORC-DISABLE.
## What changes were proposed in this pull request?
This PR proposes to add an alias 'names' of 'fieldNames' in Scala. Please see the discussion in [SPARK-20090](https://issues.apache.org/jira/browse/SPARK-20090).
## How was this patch tested?
Unit tests added in `DataTypeSuite.scala`.
Author: hyukjinkwon <gurwls223@gmail.com>
Closes#20545 from HyukjinKwon/SPARK-23359.
## What changes were proposed in this pull request?
Streaming execution has a list of exceptions that means interruption, and handle them specially. `WriteToDataSourceV2Exec` should also respect this list and not wrap them with `SparkException`.
## How was this patch tested?
existing test.
Author: Wenchen Fan <wenchen@databricks.com>
Closes#20605 from cloud-fan/write.
## What changes were proposed in this pull request?
This PR is to revert the PR https://github.com/apache/spark/pull/20302, because it causes a regression.
## How was this patch tested?
N/A
Author: gatorsmile <gatorsmile@gmail.com>
Closes#20614 from gatorsmile/revertJsonFix.
## What changes were proposed in this pull request?
https://github.com/apache/spark/pull/19579 introduces a behavior change. We need to document it in the migration guide.
## How was this patch tested?
Also update the HiveExternalCatalogVersionsSuite to verify it.
Author: gatorsmile <gatorsmile@gmail.com>
Closes#20606 from gatorsmile/addMigrationGuide.
## What changes were proposed in this pull request?
Solved two bugs to enable stream-stream self joins.
### Incorrect analysis due to missing MultiInstanceRelation trait
Streaming leaf nodes did not extend MultiInstanceRelation, which is necessary for the catalyst analyzer to convert the self-join logical plan DAG into a tree (by creating new instances of the leaf relations). This was causing the error `Failure when resolving conflicting references in Join:` (see JIRA for details).
### Incorrect attribute rewrite when splicing batch plans in MicroBatchExecution
When splicing the source's batch plan into the streaming plan (by replacing the StreamingExecutionPlan), we were rewriting the attribute reference in the streaming plan with the new attribute references from the batch plan. This was incorrectly handling the scenario when multiple StreamingExecutionRelation point to the same source, and therefore eventually point to the same batch plan returned by the source. Here is an example query, and its corresponding plan transformations.
```
val df = input.toDF
val join =
df.select('value % 5 as "key", 'value).join(
df.select('value % 5 as "key", 'value), "key")
```
Streaming logical plan before splicing the batch plan
```
Project [key#6, value#1, value#12]
+- Join Inner, (key#6 = key#9)
:- Project [(value#1 % 5) AS key#6, value#1]
: +- StreamingExecutionRelation Memory[#1], value#1
+- Project [(value#12 % 5) AS key#9, value#12]
+- StreamingExecutionRelation Memory[#1], value#12 // two different leaves pointing to same source
```
Batch logical plan after splicing the batch plan and before rewriting
```
Project [key#6, value#1, value#12]
+- Join Inner, (key#6 = key#9)
:- Project [(value#1 % 5) AS key#6, value#1]
: +- LocalRelation [value#66] // replaces StreamingExecutionRelation Memory[#1], value#1
+- Project [(value#12 % 5) AS key#9, value#12]
+- LocalRelation [value#66] // replaces StreamingExecutionRelation Memory[#1], value#12
```
Batch logical plan after rewriting the attributes. Specifically, for spliced, the new output attributes (value#66) replace the earlier output attributes (value#12, and value#1, one for each StreamingExecutionRelation).
```
Project [key#6, value#66, value#66] // both value#1 and value#12 replaces by value#66
+- Join Inner, (key#6 = key#9)
:- Project [(value#66 % 5) AS key#6, value#66]
: +- LocalRelation [value#66]
+- Project [(value#66 % 5) AS key#9, value#66]
+- LocalRelation [value#66]
```
This causes the optimizer to eliminate value#66 from one side of the join.
```
Project [key#6, value#66, value#66]
+- Join Inner, (key#6 = key#9)
:- Project [(value#66 % 5) AS key#6, value#66]
: +- LocalRelation [value#66]
+- Project [(value#66 % 5) AS key#9] // this does not generate value, incorrect join results
+- LocalRelation [value#66]
```
**Solution**: Instead of rewriting attributes, use a Project to introduce aliases between the output attribute references and the new reference generated by the spliced plans. The analyzer and optimizer will take care of the rest.
```
Project [key#6, value#1, value#12]
+- Join Inner, (key#6 = key#9)
:- Project [(value#1 % 5) AS key#6, value#1]
: +- Project [value#66 AS value#1] // solution: project with aliases
: +- LocalRelation [value#66]
+- Project [(value#12 % 5) AS key#9, value#12]
+- Project [value#66 AS value#12] // solution: project with aliases
+- LocalRelation [value#66]
```
## How was this patch tested?
New unit test
Author: Tathagata Das <tathagata.das1565@gmail.com>
Closes#20598 from tdas/SPARK-23406.
## What changes were proposed in this pull request?
This PR aims to resolve an open file leakage issue reported at [SPARK-23390](https://issues.apache.org/jira/browse/SPARK-23390) by moving the listener registration position. Currently, the sequence is like the following.
1. Create `batchReader`
2. `batchReader.initialize` opens a ORC file.
3. `batchReader.initBatch` may take a long time to alloc memory in some environment and cause errors.
4. `Option(TaskContext.get()).foreach(_.addTaskCompletionListener(_ => iter.close()))`
This PR moves 4 before 2 and 3. To sum up, the new sequence is 1 -> 4 -> 2 -> 3.
## How was this patch tested?
Manual. The following test case makes OOM intentionally to cause leaked filesystem connection in the current code base. With this patch, leakage doesn't occurs.
```scala
// This should be tested manually because it raises OOM intentionally
// in order to cause `Leaked filesystem connection`.
test("SPARK-23399 Register a task completion listener first for OrcColumnarBatchReader") {
withSQLConf(SQLConf.ORC_VECTORIZED_READER_BATCH_SIZE.key -> s"${Int.MaxValue}") {
withTempDir { dir =>
val basePath = dir.getCanonicalPath
Seq(0).toDF("a").write.format("orc").save(new Path(basePath, "first").toString)
Seq(1).toDF("a").write.format("orc").save(new Path(basePath, "second").toString)
val df = spark.read.orc(
new Path(basePath, "first").toString,
new Path(basePath, "second").toString)
val e = intercept[SparkException] {
df.collect()
}
assert(e.getCause.isInstanceOf[OutOfMemoryError])
}
}
}
```
Author: Dongjoon Hyun <dongjoon@apache.org>
Closes#20590 from dongjoon-hyun/SPARK-23399.
## What changes were proposed in this pull request?
In this upcoming 2.3 release, we changed the interface of `ScalaUDF`. Unfortunately, some Spark packages (e.g., spark-deep-learning) are using our internal class `ScalaUDF`. In the release 2.3, we added new parameters into this class. The users hit the binary compatibility issues and got the exception:
```
> java.lang.NoSuchMethodError: org.apache.spark.sql.catalyst.expressions.ScalaUDF.<init>(Ljava/lang/Object;Lorg/apache/spark/sql/types/DataType;Lscala/collection/Seq;Lscala/collection/Seq;Lscala/Option;)V
```
This PR is to improve the backward compatibility. However, we definitely should not encourage the external packages to use our internal classes. This might make us hard to maintain/develop the codes in Spark.
## How was this patch tested?
N/A
Author: gatorsmile <gatorsmile@gmail.com>
Closes#20591 from gatorsmile/scalaUDF.
## What changes were proposed in this pull request?
Added flag ignoreNullability to DataType.equalsStructurally.
The previous semantic is for ignoreNullability=false.
When ignoreNullability=true equalsStructurally ignores nullability of contained types (map key types, value types, array element types, structure field types).
In.checkInputTypes calls equalsStructurally to check if the children types match. They should match regardless of nullability (which is just a hint), so it is now called with ignoreNullability=true.
## How was this patch tested?
New test in SubquerySuite
Author: Bogdan Raducanu <bogdan@databricks.com>
Closes#20548 from bogdanrdc/SPARK-23316.
## What changes were proposed in this pull request?
If the target database name is as same as the current database, we should be able to skip one metastore access.
## How was this patch tested?
(Please explain how this patch was tested. E.g. unit tests, integration tests, manual tests)
(If this patch involves UI changes, please attach a screenshot; otherwise, remove this)
Please review http://spark.apache.org/contributing.html before opening a pull request.
Author: Feng Liu <fengliu@databricks.com>
Closes#20565 from liufengdb/remove-redundant.
## What changes were proposed in this pull request?
DataSourceV2 batch writes should use the output commit coordinator if it is required by the data source. This adds a new method, `DataWriterFactory#useCommitCoordinator`, that determines whether the coordinator will be used. If the write factory returns true, `WriteToDataSourceV2` will use the coordinator for batch writes.
## How was this patch tested?
This relies on existing write tests, which now use the commit coordinator.
Author: Ryan Blue <blue@apache.org>
Closes#20490 from rdblue/SPARK-23323-add-commit-coordinator.
When hive.default.fileformat is other kinds of file types, create textfile table cause a serde error.
We should take the default type of textfile and sequencefile both as org.apache.hadoop.hive.serde2.lazy.LazySimpleSerDe.
```
set hive.default.fileformat=orc;
create table tbl( i string ) stored as textfile;
desc formatted tbl;
Serde Library org.apache.hadoop.hive.ql.io.orc.OrcSerde
InputFormat org.apache.hadoop.mapred.TextInputFormat
OutputFormat org.apache.hadoop.hive.ql.io.HiveIgnoreKeyTextOutputFormat
```
Author: sychen <sychen@ctrip.com>
Closes#20406 from cxzl25/default_serde.
## What changes were proposed in this pull request?
This removes the special case that `alterPartitions` call from `HiveExternalCatalog` can reset the current database in the hive client as a side effect.
## How was this patch tested?
(Please explain how this patch was tested. E.g. unit tests, integration tests, manual tests)
(If this patch involves UI changes, please attach a screenshot; otherwise, remove this)
Please review http://spark.apache.org/contributing.html before opening a pull request.
Author: Feng Liu <fengliu@databricks.com>
Closes#20564 from liufengdb/move.
## What changes were proposed in this pull request?
This is a follow-up pr of #19231 which modified the behavior to remove metadata from JDBC table schema.
This pr adds a test to check if the schema doesn't have metadata.
## How was this patch tested?
Added a test and existing tests.
Author: Takuya UESHIN <ueshin@databricks.com>
Closes#20585 from ueshin/issues/SPARK-22002/fup1.
## What changes were proposed in this pull request?
Re-add support for parquet binary DecimalType in VectorizedColumnReader
## How was this patch tested?
Existing test suite
Author: James Thompson <jamesthomp@users.noreply.github.com>
Closes#20580 from jamesthomp/jt/add-back-binary-decimal.
## What changes were proposed in this pull request?
In the `getBlockData`,`blockId.reduceId` is the `Int` type, when it is greater than 2^28, `blockId.reduceId*8` will overflow
In the `decompress0`, `len` and `unitSize` are Int type, so `len * unitSize` may lead to overflow
## How was this patch tested?
N/A
Author: liuxian <liu.xian3@zte.com.cn>
Closes#20581 from 10110346/overflow2.
## What changes were proposed in this pull request?
This is a regression in Spark 2.3.
In Spark 2.2, we have a fragile UI support for SQL data writing commands. We only track the input query plan of `FileFormatWriter` and display its metrics. This is not ideal because we don't know who triggered the writing(can be table insertion, CTAS, etc.), but it's still useful to see the metrics of the input query.
In Spark 2.3, we introduced a new mechanism: `DataWritigCommand`, to fix the UI issue entirely. Now these writing commands have real children, and we don't need to hack into the `FileFormatWriter` for the UI. This also helps with `explain`, now `explain` can show the physical plan of the input query, while in 2.2 the physical writing plan is simply `ExecutedCommandExec` and it has no child.
However there is a regression in CTAS. CTAS commands don't extend `DataWritigCommand`, and we don't have the UI hack in `FileFormatWriter` anymore, so the UI for CTAS is just an empty node. See https://issues.apache.org/jira/browse/SPARK-22977 for more information about this UI issue.
To fix it, we should apply the `DataWritigCommand` mechanism to CTAS commands.
TODO: In the future, we should refactor this part and create some physical layer code pieces for data writing, and reuse them in different writing commands. We should have different logical nodes for different operators, even some of them share some same logic, e.g. CTAS, CREATE TABLE, INSERT TABLE. Internally we can share the same physical logic.
## How was this patch tested?
manually tested.
For data source table
<img width="644" alt="1" src="https://user-images.githubusercontent.com/3182036/35874155-bdffab28-0ba6-11e8-94a8-e32e106ba069.png">
For hive table
<img width="666" alt="2" src="https://user-images.githubusercontent.com/3182036/35874161-c437e2a8-0ba6-11e8-98ed-7930f01432c5.png">
Author: Wenchen Fan <wenchen@databricks.com>
Closes#20521 from cloud-fan/UI.
## What changes were proposed in this pull request?
Currently, we use SBT and MAVN to spark unit test, are affected by the parameters of `spark.testing`. However, when using the IDE test tool, `spark.testing` support is not very good, sometimes need to be manually added to the beforeEach. example: HiveSparkSubmitSuite RPackageUtilsSuite SparkSubmitSuite. The PR unified `spark.testing` parameter extraction to SparkFunSuite, support IDE test tool, and the test code is more compact.
## How was this patch tested?
the existed test cases.
Author: caoxuewen <cao.xuewen@zte.com.cn>
Closes#20582 from heary-cao/sparktesting.
## What changes were proposed in this pull request?
This PR targets to explicitly specify supported types in Pandas UDFs.
The main change here is to add a deduplicated and explicit type checking in `returnType` ahead with documenting this; however, it happened to fix multiple things.
1. Currently, we don't support `BinaryType` in Pandas UDFs, for example, see:
```python
from pyspark.sql.functions import pandas_udf
pudf = pandas_udf(lambda x: x, "binary")
df = spark.createDataFrame([[bytearray(1)]])
df.select(pudf("_1")).show()
```
```
...
TypeError: Unsupported type in conversion to Arrow: BinaryType
```
We can document this behaviour for its guide.
2. Also, the grouped aggregate Pandas UDF fails fast on `ArrayType` but seems we can support this case.
```python
from pyspark.sql.functions import pandas_udf, PandasUDFType
foo = pandas_udf(lambda v: v.mean(), 'array<double>', PandasUDFType.GROUPED_AGG)
df = spark.range(100).selectExpr("id", "array(id) as value")
df.groupBy("id").agg(foo("value")).show()
```
```
...
NotImplementedError: ArrayType, StructType and MapType are not supported with PandasUDFType.GROUPED_AGG
```
3. Since we can check the return type ahead, we can fail fast before actual execution.
```python
# we can fail fast at this stage because we know the schema ahead
pandas_udf(lambda x: x, BinaryType())
```
## How was this patch tested?
Manually tested and unit tests for `BinaryType` and `ArrayType(...)` were added.
Author: hyukjinkwon <gurwls223@gmail.com>
Closes#20531 from HyukjinKwon/pudf-cleanup.
## What changes were proposed in this pull request?
This test only fails with sbt on Hadoop 2.7, I can't reproduce it locally, but here is my speculation by looking at the code:
1. FileSystem.delete doesn't delete the directory entirely, somehow we can still open the file as a 0-length empty file.(just speculation)
2. ORC intentionally allow empty files, and the reader fails during reading without closing the file stream.
This PR improves the test to make sure all files are deleted and can't be opened.
## How was this patch tested?
N/A
Author: Wenchen Fan <wenchen@databricks.com>
Closes#20584 from cloud-fan/flaky-test.
## What changes were proposed in this pull request?
This is a long-standing bug in `UnsafeKVExternalSorter` and was reported in the dev list multiple times.
When creating `UnsafeKVExternalSorter` with `BytesToBytesMap`, we need to create a `UnsafeInMemorySorter` to sort the data in `BytesToBytesMap`. The data format of the sorter and the map is same, so no data movement is required. However, both the sorter and the map need a point array for some bookkeeping work.
There is an optimization in `UnsafeKVExternalSorter`: reuse the point array between the sorter and the map, to avoid an extra memory allocation. This sounds like a reasonable optimization, the length of the `BytesToBytesMap` point array is at least 4 times larger than the number of keys(to avoid hash collision, the hash table size should be at least 2 times larger than the number of keys, and each key occupies 2 slots). `UnsafeInMemorySorter` needs the pointer array size to be 4 times of the number of entries, so we are safe to reuse the point array.
However, the number of keys of the map doesn't equal to the number of entries in the map, because `BytesToBytesMap` supports duplicated keys. This breaks the assumption of the above optimization and we may run out of space when inserting data into the sorter, and hit error
```
java.lang.IllegalStateException: There is no space for new record
at org.apache.spark.util.collection.unsafe.sort.UnsafeInMemorySorter.insertRecord(UnsafeInMemorySorter.java:239)
at org.apache.spark.sql.execution.UnsafeKVExternalSorter.<init>(UnsafeKVExternalSorter.java:149)
...
```
This PR fixes this bug by creating a new point array if the existing one is not big enough.
## How was this patch tested?
a new test
Author: Wenchen Fan <wenchen@databricks.com>
Closes#20561 from cloud-fan/bug.
## What changes were proposed in this pull request?
This is a follow up of https://github.com/apache/spark/pull/20441.
The two lines actually can trigger the hive metastore bug: https://issues.apache.org/jira/browse/HIVE-16844
The two configs are not in the default `ObjectStore` properties, so any run hive commands after these two lines will set the `propsChanged` flag in the `ObjectStore.setConf` and then cause thread leaks.
I don't think the two lines are very useful. They can be removed safely.
## How was this patch tested?
(Please explain how this patch was tested. E.g. unit tests, integration tests, manual tests)
(If this patch involves UI changes, please attach a screenshot; otherwise, remove this)
Please review http://spark.apache.org/contributing.html before opening a pull request.
Author: Feng Liu <fengliu@databricks.com>
Closes#20562 from liufengdb/fix-omm.
## What changes were proposed in this pull request?
Typo fixes (with expanding a Hive property)
## How was this patch tested?
local build. Awaiting Jenkins
Author: Jacek Laskowski <jacek@japila.pl>
Closes#20550 from jaceklaskowski/hiveutils-typos.
## What changes were proposed in this pull request?
This is a followup of https://github.com/apache/spark/pull/20435.
While reorganizing the packages for streaming data source v2, the top level stream read/write support interfaces should not be in the reader/writer package, but should be in the `sources.v2` package, to follow the `ReadSupport`, `WriteSupport`, etc.
## How was this patch tested?
N/A
Author: Wenchen Fan <wenchen@databricks.com>
Closes#20509 from cloud-fan/followup.
## What changes were proposed in this pull request?
For inserting/appending data to an existing table, Spark should adjust the data types of the input query according to the table schema, or fail fast if it's uncastable.
There are several ways to insert/append data: SQL API, `DataFrameWriter.insertInto`, `DataFrameWriter.saveAsTable`. The first 2 ways create `InsertIntoTable` plan, and the last way creates `CreateTable` plan. However, we only adjust input query data types for `InsertIntoTable`, and users may hit weird errors when appending data using `saveAsTable`. See the JIRA for the error case.
This PR fixes this bug by adjusting data types for `CreateTable` too.
## How was this patch tested?
new test.
Author: Wenchen Fan <wenchen@databricks.com>
Closes#20527 from cloud-fan/saveAsTable.
## What changes were proposed in this pull request?
This is to revert the changes made in https://github.com/apache/spark/pull/19499 , because this causes a regression. We should not ignore the table-specific compression conf when the Hive serde tables are converted to the data source tables.
## How was this patch tested?
The existing tests.
Author: gatorsmile <gatorsmile@gmail.com>
Closes#20536 from gatorsmile/revert22279.
## What changes were proposed in this pull request?
This PR migrates the MemoryStream to DataSourceV2 APIs.
One additional change is in the reported keys in StreamingQueryProgress.durationMs. "getOffset" and "getBatch" replaced with "setOffsetRange" and "getEndOffset" as tracking these make more sense. Unit tests changed accordingly.
## How was this patch tested?
Existing unit tests, few updated unit tests.
Author: Tathagata Das <tathagata.das1565@gmail.com>
Author: Burak Yavuz <brkyvz@gmail.com>
Closes#20445 from tdas/SPARK-23092.
## What changes were proposed in this pull request?
When `DebugFilesystem` closes opened stream, if any exception occurs, we still need to remove the open stream record from `DebugFilesystem`. Otherwise, it goes to report leaked filesystem connection.
## How was this patch tested?
Existing tests.
Author: Liang-Chi Hsieh <viirya@gmail.com>
Closes#20524 from viirya/SPARK-23345.
## What changes were proposed in this pull request?
Replace `registerTempTable` by `createOrReplaceTempView`.
## How was this patch tested?
N/A
Author: gatorsmile <gatorsmile@gmail.com>
Closes#20523 from gatorsmile/updateExamples.
## What changes were proposed in this pull request?
Update the description and tests of three external API or functions `createFunction `, `length` and `repartitionByRange `
## How was this patch tested?
N/A
Author: gatorsmile <gatorsmile@gmail.com>
Closes#20495 from gatorsmile/updateFunc.
## What changes were proposed in this pull request?
`DataSourceV2Relation` keeps a `fullOutput` and resolves the real output on demand by column name lookup. i.e.
```
lazy val output: Seq[Attribute] = reader.readSchema().map(_.name).map { name =>
fullOutput.find(_.name == name).get
}
```
This will be broken after we canonicalize the plan, because all attribute names become "None", see https://github.com/apache/spark/blob/v2.3.0-rc1/sql/catalyst/src/main/scala/org/apache/spark/sql/catalyst/expressions/Canonicalize.scala#L42
To fix this, `DataSourceV2Relation` should just keep `output`, and update the `output` when doing column pruning.
## How was this patch tested?
a new test case
Author: Wenchen Fan <wenchen@databricks.com>
Closes#20485 from cloud-fan/canonicalize.
## What changes were proposed in this pull request?
https://github.com/apache/spark/pull/20483 tried to provide a way to turn off the new columnar cache reader, to restore the behavior in 2.2. However even we turn off that config, the behavior is still different than 2.2.
If the output data are rows, we still enable whole stage codegen for the scan node, which is different with 2.2, we should also fix it.
## How was this patch tested?
existing tests.
Author: Wenchen Fan <wenchen@databricks.com>
Closes#20513 from cloud-fan/cache.
## What changes were proposed in this pull request?
Spark SQL executions page throws the following error and the page crashes:
```
HTTP ERROR 500
Problem accessing /SQL/. Reason:
Server Error
Caused by:
java.lang.NullPointerException
at scala.collection.immutable.StringOps$.length$extension(StringOps.scala:47)
at scala.collection.immutable.StringOps.length(StringOps.scala:47)
at scala.collection.IndexedSeqOptimized$class.isEmpty(IndexedSeqOptimized.scala:27)
at scala.collection.immutable.StringOps.isEmpty(StringOps.scala:29)
at scala.collection.TraversableOnce$class.nonEmpty(TraversableOnce.scala:111)
at scala.collection.immutable.StringOps.nonEmpty(StringOps.scala:29)
at org.apache.spark.sql.execution.ui.ExecutionTable.descriptionCell(AllExecutionsPage.scala:182)
at org.apache.spark.sql.execution.ui.ExecutionTable.row(AllExecutionsPage.scala:155)
at org.apache.spark.sql.execution.ui.ExecutionTable$$anonfun$8.apply(AllExecutionsPage.scala:204)
at org.apache.spark.sql.execution.ui.ExecutionTable$$anonfun$8.apply(AllExecutionsPage.scala:204)
at org.apache.spark.ui.UIUtils$$anonfun$listingTable$2.apply(UIUtils.scala:339)
at org.apache.spark.ui.UIUtils$$anonfun$listingTable$2.apply(UIUtils.scala:339)
at scala.collection.TraversableLike$$anonfun$map$1.apply(TraversableLike.scala:234)
at scala.collection.TraversableLike$$anonfun$map$1.apply(TraversableLike.scala:234)
at scala.collection.mutable.ResizableArray$class.foreach(ResizableArray.scala:59)
at scala.collection.mutable.ArrayBuffer.foreach(ArrayBuffer.scala:48)
at scala.collection.TraversableLike$class.map(TraversableLike.scala:234)
at scala.collection.AbstractTraversable.map(Traversable.scala:104)
at org.apache.spark.ui.UIUtils$.listingTable(UIUtils.scala:339)
at org.apache.spark.sql.execution.ui.ExecutionTable.toNodeSeq(AllExecutionsPage.scala:203)
at org.apache.spark.sql.execution.ui.AllExecutionsPage.render(AllExecutionsPage.scala:67)
at org.apache.spark.ui.WebUI$$anonfun$2.apply(WebUI.scala:82)
at org.apache.spark.ui.WebUI$$anonfun$2.apply(WebUI.scala:82)
at org.apache.spark.ui.JettyUtils$$anon$3.doGet(JettyUtils.scala:90)
at javax.servlet.http.HttpServlet.service(HttpServlet.java:687)
at javax.servlet.http.HttpServlet.service(HttpServlet.java:790)
at org.eclipse.jetty.servlet.ServletHolder.handle(ServletHolder.java:848)
at org.eclipse.jetty.servlet.ServletHandler.doHandle(ServletHandler.java:584)
at org.eclipse.jetty.server.handler.ContextHandler.doHandle(ContextHandler.java:1180)
at org.eclipse.jetty.servlet.ServletHandler.doScope(ServletHandler.java:512)
at org.eclipse.jetty.server.handler.ContextHandler.doScope(ContextHandler.java:1112)
at org.eclipse.jetty.server.handler.ScopedHandler.handle(ScopedHandler.java:141)
at org.eclipse.jetty.server.handler.ContextHandlerCollection.handle(ContextHandlerCollection.java:213)
at org.eclipse.jetty.server.handler.HandlerWrapper.handle(HandlerWrapper.java:134)
at org.eclipse.jetty.server.Server.handle(Server.java:534)
at org.eclipse.jetty.server.HttpChannel.handle(HttpChannel.java:320)
at org.eclipse.jetty.server.HttpConnection.onFillable(HttpConnection.java:251)
at org.eclipse.jetty.io.AbstractConnection$ReadCallback.succeeded(AbstractConnection.java:283)
at org.eclipse.jetty.io.FillInterest.fillable(FillInterest.java:108)
at org.eclipse.jetty.io.SelectChannelEndPoint$2.run(SelectChannelEndPoint.java:93)
at org.eclipse.jetty.util.thread.strategy.ExecuteProduceConsume.executeProduceConsume(ExecuteProduceConsume.java:303)
at org.eclipse.jetty.util.thread.strategy.ExecuteProduceConsume.produceConsume(ExecuteProduceConsume.java:148)
at org.eclipse.jetty.util.thread.strategy.ExecuteProduceConsume.run(ExecuteProduceConsume.java:136)
at org.eclipse.jetty.util.thread.QueuedThreadPool.runJob(QueuedThreadPool.java:671)
at org.eclipse.jetty.util.thread.QueuedThreadPool$2.run(QueuedThreadPool.java:589)
at java.lang.Thread.run(Thread.java:748)
```
One of the possible reason that this page fails may be the `SparkListenerSQLExecutionStart` event get dropped before processed, so the execution description and details don't get updated.
This was not a issue in 2.2 because it would ignore any job start event that arrives before the corresponding execution start event, which doesn't sound like a good decision.
We shall try to handle the null values in the front page side, that is, try to give a default value when `execution.details` or `execution.description` is null.
Another possible approach is not to spill the `LiveExecutionData` in `SQLAppStatusListener.update(exec: LiveExecutionData)` if `exec.details` is null. This is not ideal because this way you will not see the execution if `SparkListenerSQLExecutionStart` event is lost, because `AllExecutionsPage` only read executions from KVStore.
## How was this patch tested?
After the change, the page shows the following:
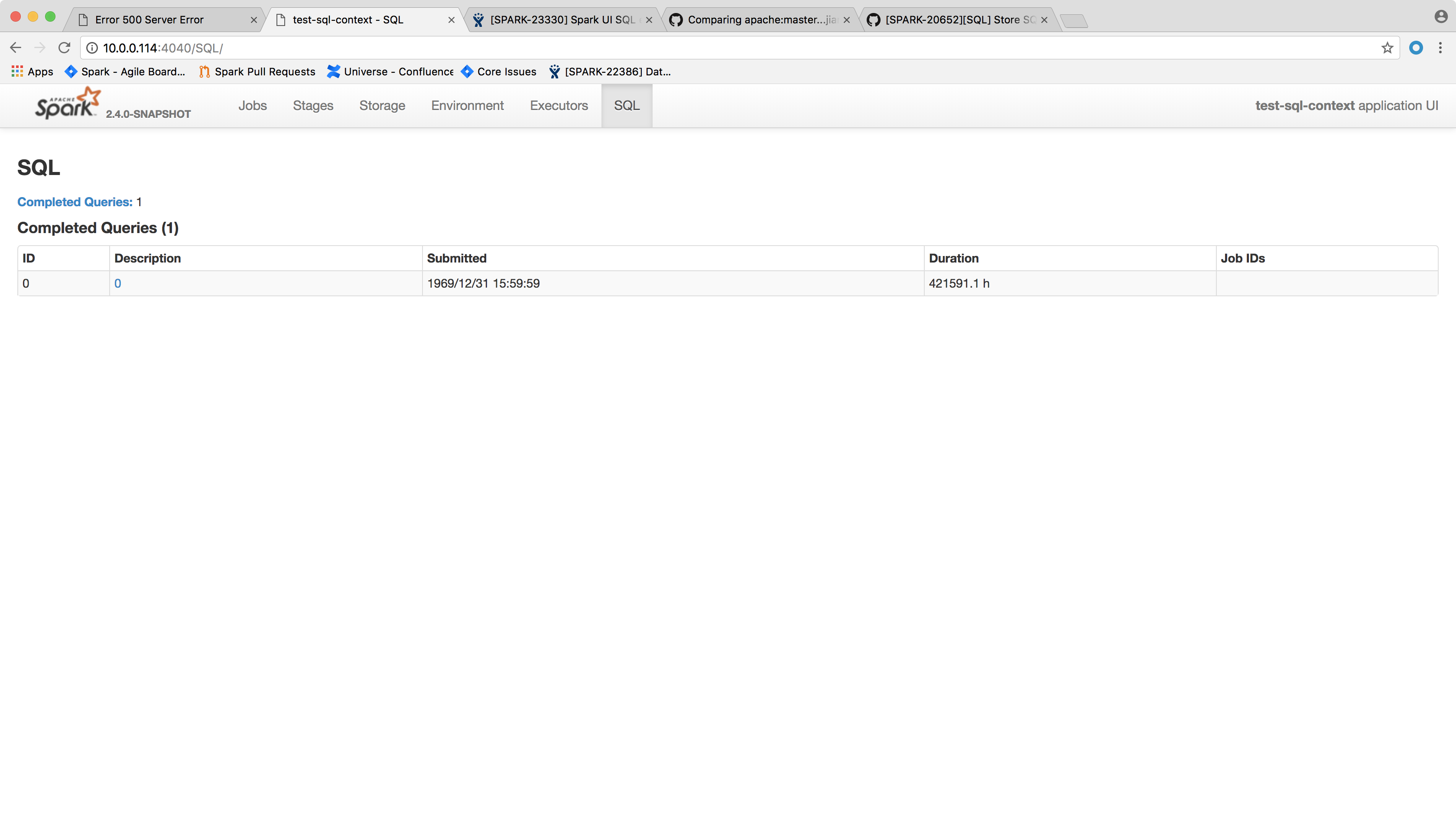
Author: Xingbo Jiang <xingbo.jiang@databricks.com>
Closes#20502 from jiangxb1987/executionPage.
## What changes were proposed in this pull request?
Sort jobs/stages/tasks/queries with the completed timestamp before cleaning up them to make the behavior consistent with 2.2.
## How was this patch tested?
- Jenkins.
- Manually ran the following codes and checked the UI for jobs/stages/tasks/queries.
```
spark.ui.retainedJobs 10
spark.ui.retainedStages 10
spark.sql.ui.retainedExecutions 10
spark.ui.retainedTasks 10
```
```
new Thread() {
override def run() {
spark.range(1, 2).foreach { i =>
Thread.sleep(10000)
}
}
}.start()
Thread.sleep(5000)
for (_ <- 1 to 20) {
new Thread() {
override def run() {
spark.range(1, 2).foreach { i =>
}
}
}.start()
}
Thread.sleep(15000)
spark.range(1, 2).foreach { i =>
}
sc.makeRDD(1 to 100, 100).foreach { i =>
}
```
Author: Shixiong Zhu <zsxwing@gmail.com>
Closes#20481 from zsxwing/SPARK-23307.
## What changes were proposed in this pull request?
Fix decimalArithmeticOperations.sql test
## How was this patch tested?
N/A
Author: Yuming Wang <wgyumg@gmail.com>
Author: wangyum <wgyumg@gmail.com>
Author: Yuming Wang <yumwang@ebay.com>
Closes#20498 from wangyum/SPARK-22036.
## What changes were proposed in this pull request?
Like Parquet, all file-based data source handles `spark.sql.files.ignoreMissingFiles` correctly. We had better have a test coverage for feature parity and in order to prevent future accidental regression for all data sources.
## How was this patch tested?
Pass Jenkins with a newly added test case.
Author: Dongjoon Hyun <dongjoon@apache.org>
Closes#20479 from dongjoon-hyun/SPARK-23305.
## What changes were proposed in this pull request?
In the current test case for CombineTypedFilters, we lack the test of FilterFunction, so let's add it.
In addition, in TypedFilterOptimizationSuite's existing test cases, Let's extract a common LocalRelation.
## How was this patch tested?
add new test cases.
Author: caoxuewen <cao.xuewen@zte.com.cn>
Closes#20482 from heary-cao/TypedFilterOptimizationSuite.
## What changes were proposed in this pull request?
In the document of `ContinuousReader.setOffset`, we say this method is used to specify the start offset. We also have a `ContinuousReader.getStartOffset` to get the value back. I think it makes more sense to rename `ContinuousReader.setOffset` to `setStartOffset`.
## How was this patch tested?
N/A
Author: Wenchen Fan <wenchen@databricks.com>
Closes#20486 from cloud-fan/rename.
## What changes were proposed in this pull request?
This patch adds a small example to the schema string definition of schema function. It isn't obvious how to use it, so an example would be useful.
## How was this patch tested?
N/A - doc only.
Author: Reynold Xin <rxin@databricks.com>
Closes#20491 from rxin/schema-doc.
## What changes were proposed in this pull request?
https://issues.apache.org/jira/browse/SPARK-23309 reported a performance regression about cached table in Spark 2.3. While the investigating is still going on, this PR adds a conf to turn off the vectorized cache reader, to unblock the 2.3 release.
## How was this patch tested?
a new test
Author: Wenchen Fan <wenchen@databricks.com>
Closes#20483 from cloud-fan/cache.
## What changes were proposed in this pull request?
This PR fixes a mistake in the `PushDownOperatorsToDataSource` rule, the column pruning logic is incorrect about `Project`.
## How was this patch tested?
a new test case for column pruning with arbitrary expressions, and improve the existing tests to make sure the `PushDownOperatorsToDataSource` really works.
Author: Wenchen Fan <wenchen@databricks.com>
Closes#20476 from cloud-fan/push-down.