### What changes were proposed in this pull request?
This PR reduces the execution time of `DeduplicateRelations` by:
1) use `Set` instead `Seq` to check duplicate relations
2) avoid plan output traverse and attribute rewrites when there are no changes in the children plan
### Why are the changes needed?
Rule `DeduplicateRelations` is slow.
### Does this PR introduce _any_ user-facing change?
No.
### How was this patch tested?
Run `TPCDSQuerySuite` and checked the run time of `DeduplicateRelations`. The time has been reduced by 77.9% after this PR.
Closes#32590 from Ngone51/improve-dedup.
Authored-by: yi.wu <yi.wu@databricks.com>
Signed-off-by: Gengliang Wang <ltnwgl@gmail.com>
### What changes were proposed in this pull request?
CTAS with location clause acts as an insert overwrite. This can cause problems when there are subdirectories within a location directory.
This causes some users to accidentally wipe out directories with very important data. We should not allow CTAS with location to a non-empty directory.
### Why are the changes needed?
Hive already handled this scenario: HIVE-11319
Steps to reproduce:
```scala
sql("""create external table `demo_CTAS`( `comment` string) PARTITIONED BY (`col1` string, `col2` string) STORED AS parquet location '/tmp/u1/demo_CTAS'""")
sql("""INSERT OVERWRITE TABLE demo_CTAS partition (col1='1',col2='1') VALUES ('abc')""")
sql("select* from demo_CTAS").show
sql("""create table ctas1 location '/tmp/u2/ctas1' as select * from demo_CTAS""")
sql("select* from ctas1").show
sql("""create table ctas2 location '/tmp/u2' as select * from demo_CTAS""")
```
Before the fix: Both create table operations will succeed. But values in table ctas1 will be replaced by ctas2 accidentally.
After the fix: `create table ctas2...` will throw `AnalysisException`:
```
org.apache.spark.sql.AnalysisException: CREATE-TABLE-AS-SELECT cannot create table with location to a non-empty directory /tmp/u2 . To allow overwriting the existing non-empty directory, set 'spark.sql.legacy.allowNonEmptyLocationInCTAS' to true.
```
### Does this PR introduce _any_ user-facing change?
Yes, if the location directory is not empty, CTAS with location will throw AnalysisException
```
sql("""create table ctas2 location '/tmp/u2' as select * from demo_CTAS""")
```
```
org.apache.spark.sql.AnalysisException: CREATE-TABLE-AS-SELECT cannot create table with location to a non-empty directory /tmp/u2 . To allow overwriting the existing non-empty directory, set 'spark.sql.legacy.allowNonEmptyLocationInCTAS' to true.
```
`CREATE TABLE AS SELECT` with non-empty `LOCATION` will throw `AnalysisException`. To restore the behavior before Spark 3.2, need to set `spark.sql.legacy.allowNonEmptyLocationInCTAS` to `true`. , default value is `false`.
Updated SQL migration guide.
### How was this patch tested?
Test case added in SQLQuerySuite.scala
Closes#32411 from vinodkc/br_fixCTAS_nonempty_dir.
Authored-by: Vinod KC <vinod.kc.in@gmail.com>
Signed-off-by: Wenchen Fan <wenchen@databricks.com>
### What changes were proposed in this pull request?
Updating column stats for Union operator stats estimation
### Why are the changes needed?
This is a followup PR to update the null count also in the Union stats operator estimation. https://github.com/apache/spark/pull/30334
### Does this PR introduce _any_ user-facing change?
No
### How was this patch tested?
Updated UTs, manual testing
Closes#32494 from shahidki31/shahid/updateNullCountForUnion.
Lead-authored-by: shahid <shahidki31@gmail.com>
Co-authored-by: Shahid <shahidki31@gmail.com>
Signed-off-by: Takeshi Yamamuro <yamamuro@apache.org>
### What changes were proposed in this pull request?
Update histogram statistics for RANGE operator stats estimation
### Why are the changes needed?
If histogram optimization is enabled, this statistics can be used in various cost based optimizations.
### Does this PR introduce _any_ user-facing change?
No
### How was this patch tested?
Added UTs. Manual test.
Closes#32498 from shahidki31/shahid/histogram.
Lead-authored-by: shahid <shahidki31@gmail.com>
Co-authored-by: Shahid <shahidki31@gmail.com>
Signed-off-by: Takeshi Yamamuro <yamamuro@apache.org>
### What changes were proposed in this pull request?
1. In HadoopMapReduceCommitProtocol, create parent directory before renaming custom partition path staging files
2. In InMemoryCatalog and HiveExternalCatalog, create new partition directory before renaming old partition path
3. Check return value of FileSystem#rename, if false, throw exception to avoid silent data loss cause by rename failure
4. Change DebugFilesystem#rename behavior to make it match HDFS's behavior (return false without rename when dst parent directory not exist)
### Why are the changes needed?
Depends on FileSystem#rename implementation, when destination directory does not exist, file system may
1. return false without renaming file nor throwing exception (e.g. HDFS), or
2. create destination directory, rename files, and return true (e.g. LocalFileSystem)
In the first case above, renames in HadoopMapReduceCommitProtocol for custom partition path will fail silently if the destination partition path does not exist. Failed renames can happen when
1. dynamicPartitionOverwrite == true, the custom partition path directories are deleted by the job before the rename; or
2. the custom partition path directories do not exist before the job; or
3. something else is wrong when file system handle `rename`
The renames in MemoryCatalog and HiveExternalCatalog for partition renaming also have similar issue.
### Does this PR introduce _any_ user-facing change?
No
### How was this patch tested?
Modified DebugFilesystem#rename, and added new unit tests.
Without the fix in src code, five InsertSuite tests and one AlterTableRenamePartitionSuite test failed:
InsertSuite.SPARK-20236: dynamic partition overwrite with custom partition path (existing test with modified FS)
```
== Results ==
!== Correct Answer - 1 == == Spark Answer - 0 ==
struct<> struct<>
![2,1,1]
```
InsertSuite.SPARK-35106: insert overwrite with custom partition path
```
== Results ==
!== Correct Answer - 1 == == Spark Answer - 0 ==
struct<> struct<>
![2,1,1]
```
InsertSuite.SPARK-35106: dynamic partition overwrite with custom partition path
```
== Results ==
!== Correct Answer - 2 == == Spark Answer - 1 ==
!struct<> struct<i:int,part1:int,part2:int>
[1,1,1] [1,1,1]
![1,1,2]
```
InsertSuite.SPARK-35106: Throw exception when rename custom partition paths returns false
```
Expected exception org.apache.spark.SparkException to be thrown, but no exception was thrown
```
InsertSuite.SPARK-35106: Throw exception when rename dynamic partition paths returns false
```
Expected exception org.apache.spark.SparkException to be thrown, but no exception was thrown
```
AlterTableRenamePartitionSuite.ALTER TABLE .. RENAME PARTITION V1: multi part partition (existing test with modified FS)
```
== Results ==
!== Correct Answer - 1 == == Spark Answer - 0 ==
struct<> struct<>
![3,123,3]
```
Closes#32530 from YuzhouSun/SPARK-35106.
Authored-by: Yuzhou Sun <yuzhosun@amazon.com>
Signed-off-by: Wenchen Fan <wenchen@databricks.com>
### What changes were proposed in this pull request?
SPARK-35253 upgraded janino from 3.0.16 to 3.1.4, `ClassBodyEvaluator` provides the `getBytecodes` method to get
the mapping from `ClassFile#getThisClassName` to `ClassFile#toByteArray` directly in this version and we don't need to get this variable by reflection api anymore.
So the main purpose of this pr is simplify the way to get `bytecodes` from `ClassBodyEvaluator` in `CodeGenerator#updateAndGetCompilationStats` method.
### Why are the changes needed?
Code simplification.
### Does this PR introduce _any_ user-facing change?
No
### How was this patch tested?
- Pass the Jenkins or GitHub Action
- Manual test:
1. Define a code fragment to be tested, for example:
```
val codeBody = s"""
public java.lang.Object generate(Object[] references) {
return new TestMetricCode(references);
}
class TestMetricCode {
public TestMetricCode(Object[] references) {
}
public long sumOfSquares(long left, long right) {
return left * left + right * right;
}
}
"""
```
2. Create a `ClassBodyEvaluator` and `cook` the `codeBody` as above, the process of creating `ClassBodyEvaluator` can extract from `CodeGenerator#doCompile` method.
3. Get `bytecodes` using `ClassBodyEvaluator#getBytecodes` api(after this pr) and reflection api(before this pr) respectively, then assert that they are the same. If the `bytecodes` not changed, we can be sure that metrics state will not change. The test code example as follows:
```
import scala.collection.JavaConverters._
val bytecodesFromApi = evaluator.getBytecodes.asScala
val bytecodesFromReflectionApi = {
val scField = classOf[ClassBodyEvaluator].getDeclaredField("sc")
scField.setAccessible(true)
val compiler = scField.get(evaluator).asInstanceOf[SimpleCompiler]
val loader = compiler.getClassLoader.asInstanceOf[ByteArrayClassLoader]
val classesField = loader.getClass.getDeclaredField("classes")
classesField.setAccessible(true)
classesField.get(loader).asInstanceOf[java.util.Map[String, Array[Byte]]].asScala
}
assert(bytecodesFromApi == bytecodesFromReflectionApi)
```
Closes#32536 from LuciferYang/SPARK-35253-FOLLOWUP.
Authored-by: yangjie01 <yangjie01@baidu.com>
Signed-off-by: Takeshi Yamamuro <yamamuro@apache.org>
### What changes were proposed in this pull request?
Write out Seq of product objects which contain TreeNode, to avoid the cases as described in https://issues.apache.org/jira/browse/SPARK-35411 that essential information will be ignored and just written out as null values. These information are necessary to understand the query plans.
### Why are the changes needed?
Information like cteRelations in With node, and branches in CaseWhen expression are necessary to understand the query plans, they should be written out to the result json string.
### Does this PR introduce _any_ user-facing change?
No
### How was this patch tested?
UT case added.
Closes#32557 from ivoson/plan-json-fix.
Authored-by: Tengfei Huang <tengfei.h@gmail.com>
Signed-off-by: Wenchen Fan <wenchen@databricks.com>
### What changes were proposed in this pull request?
To pass the TPCDS-related plan stability tests in scala-2.13, this PR proposes to fix two things below;
- (1) Sorts elements in the predicate `InSet` and the source filter `In` for printing their nodes.
- (2) Formats nested collection elements (`Seq`, `Array`, and `Set`) recursively in `TreeNode.argString`.
As for (1), it seems v2.12/v2.13 prints `Set` elements with a different order, so we need to sort them explicitly. As for (2), the `Seq` implementation is different between v2.12/v2.13, so we need to format nested `Seq` elements correctly to hide the name of its implementation (See an example below);
```
(74) Expand [codegen id : 20]
Input [5]: [sales#41, RETURNS#42, profit#43, channel#44, id#45]
-Arguments: [ArrayBuffer(sales#41, returns#42, ... <-- scala-2.12
+Arguments: [Vector(sales#41, returns#42, ... <-- scala-2.13
+Arguments: [[(sales#41, returns#42, ... <-- the proposed fix to hide the name of its implementation
```
### Why are the changes needed?
To pass the tests in Scala v2.13.
### Does this PR introduce _any_ user-facing change?
Yes, this fix changes query explain strings.
### How was this patch tested?
Manually checked.
Closes#32577 from maropu/FixTPCDSTestIssueInScala213.
Authored-by: Takeshi Yamamuro <yamamuro@apache.org>
Signed-off-by: Wenchen Fan <wenchen@databricks.com>
### What changes were proposed in this pull request?
When creating `Invoke` and `StaticInvoke` for `ScalarFunction`'s magic method, set `propagateNull` to false.
### Why are the changes needed?
When `propgagateNull` is true (which is the default value), `Invoke` and `StaticInvoke` will return null if any of the argument is null. For scalar function this is incorrect, as we should leave the logic to function implementation instead.
### Does this PR introduce _any_ user-facing change?
Yes. Now null arguments shall be properly handled with magic method.
### How was this patch tested?
Added new tests.
Closes#32553 from sunchao/SPARK-35389.
Authored-by: Chao Sun <sunchao@apple.com>
Signed-off-by: Wenchen Fan <wenchen@databricks.com>
### What changes were proposed in this pull request?
https://github.com/apache/spark/pull/30309 added a configuration (disabled by default) that simplifies the error messages from Python UDFS, which removed internal stacktrace from Python workers:
```python
from pyspark.sql.functions import udf; spark.range(10).select(udf(lambda x: x/0)("id")).collect()
```
**Before**
```
Traceback (most recent call last):
File "<stdin>", line 1, in <module>
File "/.../python/pyspark/sql/dataframe.py", line 427, in show
print(self._jdf.showString(n, 20, vertical))
File "/.../python/lib/py4j-0.10.9-src.zip/py4j/java_gateway.py", line 1305, in __call__
File "/.../python/pyspark/sql/utils.py", line 127, in deco
raise_from(converted)
File "<string>", line 3, in raise_from
pyspark.sql.utils.PythonException:
An exception was thrown from Python worker in the executor:
Traceback (most recent call last):
File "/.../python/lib/pyspark.zip/pyspark/worker.py", line 605, in main
process()
File "/.../python/lib/pyspark.zip/pyspark/worker.py", line 597, in process
serializer.dump_stream(out_iter, outfile)
File "/.../python/lib/pyspark.zip/pyspark/serializers.py", line 223, in dump_stream
self.serializer.dump_stream(self._batched(iterator), stream)
File "/.../python/lib/pyspark.zip/pyspark/serializers.py", line 141, in dump_stream
for obj in iterator:
File "/.../python/lib/pyspark.zip/pyspark/serializers.py", line 212, in _batched
for item in iterator:
File "/.../python/lib/pyspark.zip/pyspark/worker.py", line 450, in mapper
result = tuple(f(*[a[o] for o in arg_offsets]) for (arg_offsets, f) in udfs)
File "/.../python/lib/pyspark.zip/pyspark/worker.py", line 450, in <genexpr>
result = tuple(f(*[a[o] for o in arg_offsets]) for (arg_offsets, f) in udfs)
File "/.../python/lib/pyspark.zip/pyspark/worker.py", line 90, in <lambda>
return lambda *a: f(*a)
File "/.../python/lib/pyspark.zip/pyspark/util.py", line 107, in wrapper
return f(*args, **kwargs)
File "<stdin>", line 1, in <lambda>
ZeroDivisionError: division by zero
```
**After**
```
Traceback (most recent call last):
File "<stdin>", line 1, in <module>
File "/.../python/pyspark/sql/dataframe.py", line 427, in show
print(self._jdf.showString(n, 20, vertical))
File "/.../python/lib/py4j-0.10.9-src.zip/py4j/java_gateway.py", line 1305, in __call__
File "/.../python/pyspark/sql/utils.py", line 127, in deco
raise_from(converted)
File "<string>", line 3, in raise_from
pyspark.sql.utils.PythonException:
An exception was thrown from Python worker in the executor:
Traceback (most recent call last):
File "<stdin>", line 1, in <lambda>
ZeroDivisionError: division by zero
```
Note that the traceback (`return f(*args, **kwargs)`) is almost always same - I would say more than 99%. For 1% case, we can guide developers to enable this configuration for further debugging.
In Databricks, it has been enabled for around 6 months, and I have had zero negative feedback on it.
### Why are the changes needed?
To show simplified exception messages to end users.
### Does this PR introduce _any_ user-facing change?
Yes, it will hide the internal Python worker traceback.
### How was this patch tested?
Existing test cases should cover.
Closes#32569 from HyukjinKwon/SPARK-35419.
Authored-by: Hyukjin Kwon <gurwls223@apache.org>
Signed-off-by: Hyukjin Kwon <gurwls223@apache.org>
### What changes were proposed in this pull request?
This PR is used to fix this bug:
```
set spark.sql.legacy.charVarcharAsString=true;
create table chartb01(a char(3));
insert into chartb01 select 'aaaaa';
```
here we expect the data of table chartb01 is 'aaa', but it runs failed.
### Why are the changes needed?
Improve backward compatibility
```
spark-sql>
> create table tchar01(col char(2)) using parquet;
Time taken: 0.767 seconds
spark-sql>
> insert into tchar01 select 'aaa';
ERROR | Executor task launch worker for task 0.0 in stage 0.0 (TID 0) | Aborting task | org.apache.spark.util.Utils.logError(Logging.scala:94)
java.lang.RuntimeException: Exceeds char/varchar type length limitation: 2
at org.apache.spark.sql.catalyst.util.CharVarcharCodegenUtils.trimTrailingSpaces(CharVarcharCodegenUtils.java:31)
at org.apache.spark.sql.catalyst.util.CharVarcharCodegenUtils.charTypeWriteSideCheck(CharVarcharCodegenUtils.java:44)
at org.apache.spark.sql.catalyst.expressions.GeneratedClass$GeneratedIteratorForCodegenStage1.project_doConsume_0$(Unknown Source)
at org.apache.spark.sql.catalyst.expressions.GeneratedClass$GeneratedIteratorForCodegenStage1.processNext(Unknown Source)
at org.apache.spark.sql.execution.BufferedRowIterator.hasNext(BufferedRowIterator.java:43)
at org.apache.spark.sql.execution.WholeStageCodegenExec$$anon$1.hasNext(WholeStageCodegenExec.scala:755)
at org.apache.spark.sql.execution.datasources.FileFormatWriter$.$anonfun$executeTask$1(FileFormatWriter.scala:279)
at org.apache.spark.util.Utils$.tryWithSafeFinallyAndFailureCallbacks(Utils.scala:1500)
at org.apache.spark.sql.execution.datasources.FileFormatWriter$.executeTask(FileFormatWriter.scala:288)
at org.apache.spark.sql.execution.datasources.FileFormatWriter$.$anonfun$write$15(FileFormatWriter.scala:212)
at org.apache.spark.scheduler.ResultTask.runTask(ResultTask.scala:90)
at org.apache.spark.scheduler.Task.run(Task.scala:131)
at org.apache.spark.executor.Executor$TaskRunner.$anonfun$run$3(Executor.scala:497)
at org.apache.spark.util.Utils$.tryWithSafeFinally(Utils.scala:1466)
at org.apache.spark.executor.Executor$TaskRunner.run(Executor.scala:500)
at java.util.concurrent.ThreadPoolExecutor.runWorker(ThreadPoolExecutor.java:1149)
at java.util.concurrent.ThreadPoolExecutor$Worker.run(ThreadPoolExecutor.java:624)
at java.lang.Thread.run(Thread.java:748)
```
### Does this PR introduce _any_ user-facing change?
No (the legacy config is false by default).
### How was this patch tested?
Added unit tests.
Closes#32501 from fhygh/master.
Authored-by: fhygh <283452027@qq.com>
Signed-off-by: Wenchen Fan <wenchen@databricks.com>
### What changes were proposed in this pull request?
Spark doesn't support aggregate functions with mixed outer and local references. This PR applies this check earlier to fail with a clear error message instead of some weird ones, and simplifies the related code in `SubExprUtils.getOuterReferences`. This PR also refines the error message a bit.
### Why are the changes needed?
better error message
### Does this PR introduce _any_ user-facing change?
no
### How was this patch tested?
updated tests
Closes#32503 from cloud-fan/try.
Authored-by: Wenchen Fan <wenchen@databricks.com>
Signed-off-by: Wenchen Fan <wenchen@databricks.com>
Introduction: this PR is a part of SPARK-10816 (`EventTime based sessionization (session window)`). Please refer #31937 to see the overall view of the code change. (Note that code diff could be diverged a bit.)
### What changes were proposed in this pull request?
This PR introduces UpdatingSessionsIterator, which analyzes neighbor elements and adjust session information on elements.
UpdatingSessionsIterator calculates and updates the session window for each element in the given iterator, which makes elements in the same session window having same session spec. Downstream can apply aggregation to finally merge these elements bound to the same session window.
UpdatingSessionsIterator works on the precondition that given iterator is sorted by "group keys + start time of session window", and the iterator still retains the characteristic of the sort.
UpdatingSessionsIterator copies the elements to safely update on each element, as well as buffers elements which are bound to the same session window. Due to such overheads, MergingSessionsIterator which will be introduced via SPARK-34889 should be used whenever possible.
This PR also introduces UpdatingSessionsExec which is the physical node on leveraging UpdatingSessionsIterator to sort the input rows and updates session information on input rows.
### Why are the changes needed?
This part is a one of required on implementing SPARK-10816.
### Does this PR introduce _any_ user-facing change?
No.
### How was this patch tested?
New test suite added.
Closes#31986 from HeartSaVioR/SPARK-34888-SPARK-10816-PR-31570-part-1.
Lead-authored-by: Jungtaek Lim <kabhwan.opensource@gmail.com>
Co-authored-by: Jungtaek Lim (HeartSaVioR) <kabhwan.opensource@gmail.com>
Signed-off-by: Jungtaek Lim <kabhwan.opensource@gmail.com>
### What changes were proposed in this pull request?
Move hash map lookup operation out of `InvokeLike.invoke` since it doesn't depend on the input.
### Why are the changes needed?
We shouldn't need to look up the hash map for every input row evaluated by `InvokeLike.invoke` since it doesn't depend on input. This could speed up the performance a bit.
### Does this PR introduce _any_ user-facing change?
No
### How was this patch tested?
Existing tests.
Closes#32532 from sunchao/SPARK-35384-follow-up.
Authored-by: Chao Sun <sunchao@apple.com>
Signed-off-by: Dongjoon Hyun <dhyun@apple.com>
### What changes were proposed in this pull request?
Add a common functions `getWorkspaceFilePath` (which prefixed with spark home) to `SparkFunctionSuite`, and applies these the function to where they're extracted from.
### Why are the changes needed?
Spark sql has test suites to read resources when running tests. The way of getting the path of resources is commonly used in different suites. We can extract them into a function to ease the code maintenance.
### Does this PR introduce _any_ user-facing change?
No.
### How was this patch tested?
Pass existing tests.
Closes#32315 from Ngone51/extract-common-file-path.
Authored-by: yi.wu <yi.wu@databricks.com>
Signed-off-by: Wenchen Fan <wenchen@databricks.com>
### What changes were proposed in this pull request?
Generally, we would expect that x = y => hash( x ) = hash( y ). However +-0 hash to different values for floating point types.
```
scala> spark.sql("select hash(cast('0.0' as double)), hash(cast('-0.0' as double))").show
+-------------------------+--------------------------+
|hash(CAST(0.0 AS DOUBLE))|hash(CAST(-0.0 AS DOUBLE))|
+-------------------------+--------------------------+
| -1670924195| -853646085|
+-------------------------+--------------------------+
scala> spark.sql("select cast('0.0' as double) == cast('-0.0' as double)").show
+--------------------------------------------+
|(CAST(0.0 AS DOUBLE) = CAST(-0.0 AS DOUBLE))|
+--------------------------------------------+
| true|
+--------------------------------------------+
```
Here is an extract from IEEE 754:
> The two zeros are distinguishable arithmetically only by either division-byzero ( producing appropriately signed infinities ) or else by the CopySign function recommended by IEEE 754 /854. Infinities, SNaNs, NaNs and Subnormal numbers necessitate four more special cases
From this, I deduce that the hash function must produce the same result for 0 and -0.
### Why are the changes needed?
It is a correctness issue
### Does this PR introduce _any_ user-facing change?
This changes only affect to the hash function applied to -0 value in float and double types
### How was this patch tested?
Unit testing and manual testing
Closes#32496 from planga82/feature/spark35207_hashnegativezero.
Authored-by: Pablo Langa <soypab@gmail.com>
Signed-off-by: Wenchen Fan <wenchen@databricks.com>
### What changes were proposed in this pull request?
This patch replaces `sys.err` usages with explicit exception types.
### Why are the changes needed?
Motivated by the previous comment https://github.com/apache/spark/pull/32519#discussion_r630787080, it sounds better to replace `sys.err` usages with explicit exception type.
### Does this PR introduce _any_ user-facing change?
No.
### How was this patch tested?
Existing tests.
Closes#32535 from viirya/replace-sys-err.
Authored-by: Liang-Chi Hsieh <viirya@gmail.com>
Signed-off-by: Dongjoon Hyun <dhyun@apple.com>
### What changes were proposed in this pull request?
This PR group exception messages in `sql/core/src/main/scala/org/apache/spark/sql/streaming`.
### Why are the changes needed?
It will largely help with standardization of error messages and its maintenance.
### Does this PR introduce _any_ user-facing change?
No. Error messages remain unchanged.
### How was this patch tested?
No new tests - pass all original tests to make sure it doesn't break any existing behavior.
Closes#32464 from beliefer/SPARK-35062.
Lead-authored-by: gengjiaan <gengjiaan@360.cn>
Co-authored-by: Jiaan Geng <beliefer@163.com>
Signed-off-by: Wenchen Fan <wenchen@databricks.com>
### What changes were proposed in this pull request?
Add a new config to make cache plan disable configs configurable.
### Why are the changes needed?
The disable configs of cache plan if to avoid the perfermance regression, but not all the query will slow than before due to AQE or bucket scan enabled. It's useful to make a new config so that user can decide if some configs should be disabled during cache plan.
### Does this PR introduce _any_ user-facing change?
Yes, a new config.
### How was this patch tested?
Add test.
Closes#32482 from ulysses-you/SPARK-35332.
Authored-by: ulysses-you <ulyssesyou18@gmail.com>
Signed-off-by: Wenchen Fan <wenchen@databricks.com>
### What changes were proposed in this pull request?
Add New SQL functions:
* TRY_ADD
* TRY_DIVIDE
These expressions are identical to the following expression under ANSI mode except that it returns null if error occurs:
* ADD
* DIVIDE
Note: it is easy to add other expressions like `TRY_SUBTRACT`/`TRY_MULTIPLY` but let's control the number of these new expressions and just add `TRY_ADD` and `TRY_DIVIDE` for now.
### Why are the changes needed?
1. Users can manage to finish queries without interruptions in ANSI mode.
2. Users can get NULLs instead of unreasonable results if overflow occurs when ANSI mode is off.
For example, the behavior of the following SQL operations is unreasonable:
```
2147483647 + 2 => -2147483647
```
With the new safe version SQL functions:
```
TRY_ADD(2147483647, 2) => null
```
Note: **We should only add new expressions to important operators, instead of adding new safe expressions for all the expressions that can throw errors.**
### Does this PR introduce _any_ user-facing change?
Yes, new SQL functions: TRY_ADD/TRY_DIVIDE
### How was this patch tested?
Unit test
Closes#32292 from gengliangwang/try_add.
Authored-by: Gengliang Wang <ltnwgl@gmail.com>
Signed-off-by: Gengliang Wang <ltnwgl@gmail.com>
### What changes were proposed in this pull request?
In Spark, we have an extension in the MERGE syntax: INSERT/UPDATE *. This is not from ANSI standard or any other mainstream databases, so we need to define the behaviors by our own.
The behavior today is very weird: assume the source table has `n1` columns, target table has `n2` columns. We generate the assignments by taking the first `min(n1, n2)` columns from source & target tables and pairing them by ordinal.
This PR proposes a more reasonable behavior: take all the columns from target table as keys, and find the corresponding columns from source table by name as values.
### Why are the changes needed?
Fix the MEREG INSERT/UPDATE * to be more user-friendly and easy to do schema evolution.
### Does this PR introduce _any_ user-facing change?
Yes, but MERGE is only supported by very few data sources.
### How was this patch tested?
new tests
Closes#32192 from cloud-fan/merge.
Authored-by: Wenchen Fan <wenchen@databricks.com>
Signed-off-by: Wenchen Fan <wenchen@databricks.com>
### What changes were proposed in this pull request?
Change `map` in `InvokeLike.invoke` to a while loop to improve performance, following Spark [style guide](https://github.com/databricks/scala-style-guide#traversal-and-zipwithindex).
### Why are the changes needed?
`InvokeLike.invoke`, which is used in non-codegen path for `Invoke` and `StaticInvoke`, currently uses `map` to evaluate arguments:
```scala
val args = arguments.map(e => e.eval(input).asInstanceOf[Object])
if (needNullCheck && args.exists(_ == null)) {
// return null if one of arguments is null
null
} else {
...
```
which is pretty expensive if the method itself is trivial. We can change it to a plain while loop.
<img width="871" alt="Screen Shot 2021-05-12 at 12 19 59 AM" src="https://user-images.githubusercontent.com/506679/118055719-7f985a00-b33d-11eb-943b-cf85eab35f44.png">
Benchmark results show this can improve as much as 3x from `V2FunctionBenchmark`:
Before
```
OpenJDK 64-Bit Server VM 1.8.0_292-b10 on Linux 5.4.0-1046-azure
Intel(R) Xeon(R) CPU E5-2673 v3 2.40GHz
scalar function (long + long) -> long, result_nullable = false codegen = false: Best Time(ms) Avg Time(ms) Stdev(ms) Rate(M/s) Per Row(ns) Relative
--------------------------------------------------------------------------------------------------------------------------------------------------------------
native_long_add 36506 36656 251 13.7 73.0 1.0X
java_long_add_default 47151 47540 370 10.6 94.3 0.8X
java_long_add_magic 178691 182457 1327 2.8 357.4 0.2X
java_long_add_static_magic 177151 178258 1151 2.8 354.3 0.2X
```
After
```
OpenJDK 64-Bit Server VM 1.8.0_292-b10 on Linux 5.4.0-1046-azure
Intel(R) Xeon(R) CPU E5-2673 v3 2.40GHz
scalar function (long + long) -> long, result_nullable = false codegen = false: Best Time(ms) Avg Time(ms) Stdev(ms) Rate(M/s) Per Row(ns) Relative
--------------------------------------------------------------------------------------------------------------------------------------------------------------
native_long_add 29897 30342 568 16.7 59.8 1.0X
java_long_add_default 40628 41075 664 12.3 81.3 0.7X
java_long_add_magic 54553 54755 182 9.2 109.1 0.5X
java_long_add_static_magic 55410 55532 127 9.0 110.8 0.5X
```
### Does this PR introduce _any_ user-facing change?
No
### How was this patch tested?
Existing tests.
Closes#32527 from sunchao/SPARK-35384.
Authored-by: Chao Sun <sunchao@apple.com>
Signed-off-by: Dongjoon Hyun <dhyun@apple.com>
### What changes were proposed in this pull request?
Switch to plain `while` loop following Spark [style guide](https://github.com/databricks/scala-style-guide#traversal-and-zipwithindex).
### Why are the changes needed?
`while` loop may yield better performance comparing to `foreach`.
### Does this PR introduce _any_ user-facing change?
No
### How was this patch tested?
N/A
Closes#32522 from sunchao/SPARK-35361-follow-up.
Authored-by: Chao Sun <sunchao@apple.com>
Signed-off-by: Dongjoon Hyun <dongjoon@apache.org>
### What changes were proposed in this pull request?
A simple follow-up of #32474 to throw exception instead of sys.error.
### Why are the changes needed?
An exception only fails the query, instead of sys.error.
### Does this PR introduce _any_ user-facing change?
Yes, if `Invoke` or `StaticInvoke` cannot find the method, instead of original `sys.error` now we only throw an exception.
### How was this patch tested?
Existing tests.
Closes#32519 from viirya/SPARK-35347-followup.
Authored-by: Liang-Chi Hsieh <viirya@gmail.com>
Signed-off-by: Liang-Chi Hsieh <viirya@gmail.com>
### What changes were proposed in this pull request?
This PR proposes to bump up the janino version from 3.0.16 to v3.1.4.
The major changes of this upgrade are as follows:
- Fixed issue #131: Janino 3.1.2 is 10x slower than 3.0.11: The Compiler's IClassLoader was initialized way too eagerly, thus lots of classes were loaded from the class path, which is very slow.
- Improved the encoding of stack map frames according to JVMS11 4.7.4: Previously, only "full_frame"s were generated.
- Fixed issue #107: Janino requires "org.codehaus.commons.compiler.io", but commons-compiler does not export this package
- Fixed the promotion of the array access index expression (see JLS7 15.13 Array Access Expressions).
For all the changes, please see the change log: http://janino-compiler.github.io/janino/changelog.html
NOTE1: I've checked that there is no obvious performance regression. For all the data, see a link: https://docs.google.com/spreadsheets/d/1srxT9CioGQg1fLKM3Uo8z1sTzgCsMj4pg6JzpdcG6VU/edit?usp=sharing
NOTE2: We upgraded janino to 3.1.2 (#27860) once before, but the commit had been reverted in #29495 because of the correctness issue. Recently, #32374 had checked if Spark could land on v3.1.3 or not, but a new bug was found there. These known issues has been fixed in v3.1.4 by following PRs:
- janino-compiler/janino#145
- janino-compiler/janino#146
### Why are the changes needed?
janino v3.0.X is no longer maintained.
### Does this PR introduce _any_ user-facing change?
No.
### How was this patch tested?
GA passed.
Closes#32455 from maropu/janino_v3.1.4.
Authored-by: Takeshi Yamamuro <yamamuro@apache.org>
Signed-off-by: Sean Owen <srowen@gmail.com>
### What changes were proposed in this pull request?
According to discuss https://github.com/apache/spark/pull/25854#discussion_r629451135
### Why are the changes needed?
Clean code
### Does this PR introduce _any_ user-facing change?
No
### How was this patch tested?
Existed UT
Closes#32499 from AngersZhuuuu/SPARK-29145-fix.
Authored-by: Angerszhuuuu <angers.zhu@gmail.com>
Signed-off-by: Wenchen Fan <wenchen@databricks.com>
### What changes were proposed in this pull request?
In `ApplyFunctionExpression`, move `zipWithIndex` out of the loop for each input row.
### Why are the changes needed?
When the `ScalarFunction` is trivial, `zipWithIndex` could incur significant costs, as shown below:
<img width="899" alt="Screen Shot 2021-05-11 at 10 03 42 AM" src="https://user-images.githubusercontent.com/506679/117866421-fb19de80-b24b-11eb-8c94-d5e8c8b1eda9.png">
By removing it out of the loop, I'm seeing sometimes 2x speedup from `V2FunctionBenchmark`. For instance:
Before:
```
scalar function (long + long) -> long, result_nullable = false codegen = false: Best Time(ms) Avg Time(ms) Stdev(ms) Rate(M/s) Per Row(ns) Relative
native_long_add 32437 32896 434 15.4 64.9 1.0X
java_long_add_default 85675 97045 NaN 5.8 171.3 0.4X
```
After:
```
scalar function (long + long) -> long, result_nullable = false codegen = false: Best Time(ms) Avg Time(ms) Stdev(ms) Rate(M/s) Per Row(ns) Relative
native_long_add 30182 30387 279 16.6 60.4 1.0X
java_long_add_default 42862 43009 209 11.7 85.7 0.7X
```
### Does this PR introduce _any_ user-facing change?
No
### How was this patch tested?
Existing tests
Closes#32507 from sunchao/SPARK-35361.
Authored-by: Chao Sun <sunchao@apple.com>
Signed-off-by: Hyukjin Kwon <gurwls223@apache.org>
### What changes were proposed in this pull request?
Added the following TreePattern enums:
- BOOL_AGG
- COUNT_IF
- CURRENT_LIKE
- RUNTIME_REPLACEABLE
Added tree traversal pruning to the following rules:
- ReplaceExpressions
- RewriteNonCorrelatedExists
- ComputeCurrentTime
- GetCurrentDatabaseAndCatalog
### Why are the changes needed?
Reduce the number of tree traversals and hence improve the query compilation latency.
Performance improvement (org.apache.spark.sql.TPCDSQuerySuite):
Rule name | Total Time (baseline) | Total Time (experiment) | experiment/baseline
ReplaceExpressions | 27546369 | 19753804 | 0.72
RewriteNonCorrelatedExists | 17304883 | 2086194 | 0.12
ComputeCurrentTime | 35751301 | 19984477 | 0.56
GetCurrentDatabaseAndCatalog | 37230787 | 18874013 | 0.51
### How was this patch tested?
Existing tests.
Closes#32461 from sigmod/finish_analysis.
Authored-by: Yingyi Bu <yingyi.bu@databricks.com>
Signed-off-by: Gengliang Wang <ltnwgl@gmail.com>
### What changes were proposed in this pull request?
Sequence expression output a message looks confused.
This PR will fix the issue.
### Why are the changes needed?
Improve the error message for Sequence expression
### Does this PR introduce _any_ user-facing change?
Yes. this PR updates the error message of Sequence expression.
### How was this patch tested?
Tests updated.
Closes#32492 from beliefer/SPARK-35088-followup.
Authored-by: gengjiaan <gengjiaan@360.cn>
Signed-off-by: Hyukjin Kwon <gurwls223@apache.org>
### What changes were proposed in this pull request?
Change the definition of `findTightestCommonType` from
```
def findTightestCommonType(t1: DataType, t2: DataType): Option[DataType]
```
to
```
val findTightestCommonType: (DataType, DataType) => Option[DataType]
```
### Why are the changes needed?
For backward compatibility.
When running a MongoDB connector (built with Spark 3.1.1) with the latest master, there is such an error
```
java.lang.NoSuchMethodError: org.apache.spark.sql.catalyst.analysis.TypeCoercion$.findTightestCommonType()Lscala/Function2
```
from https://github.com/mongodb/mongo-spark/blob/master/src/main/scala/com/mongodb/spark/sql/MongoInferSchema.scala#L150
In the previous release, the function was
```
static public scala.Function2<org.apache.spark.sql.types.DataType, org.apache.spark.sql.types.DataType, scala.Option<org.apache.spark.sql.types.DataType>> findTightestCommonType ()
```
After https://github.com/apache/spark/pull/31349, the function becomes:
```
static public scala.Option<org.apache.spark.sql.types.DataType> findTightestCommonType (org.apache.spark.sql.types.DataType t1, org.apache.spark.sql.types.DataType t2)
```
This PR is to reduce the unnecessary API change.
### Does this PR introduce _any_ user-facing change?
Yes, the definition of `TypeCoercion.findTightestCommonType` is consistent with previous release again.
### How was this patch tested?
Existing unit tests
Closes#32493 from gengliangwang/typecoercion.
Authored-by: Gengliang Wang <ltnwgl@gmail.com>
Signed-off-by: Gengliang Wang <ltnwgl@gmail.com>
### What changes were proposed in this pull request?
RepairTableCommand respects `spark.sql.addPartitionInBatch.size` too
### Why are the changes needed?
Make RepairTableCommand add partition batch size configurable.
### Does this PR introduce _any_ user-facing change?
User can use `spark.sql.addPartitionInBatch.size` to change batch size when repair table.
### How was this patch tested?
Not need
Closes#32489 from AngersZhuuuu/SPARK-35360.
Authored-by: Angerszhuuuu <angers.zhu@gmail.com>
Signed-off-by: Max Gekk <max.gekk@gmail.com>
### What changes were proposed in this pull request?
Rename pattern strings and regexps of year-month and day-time intervals.
### Why are the changes needed?
To improve code maintainability.
### Does this PR introduce _any_ user-facing change?
No
### How was this patch tested?
By existing test suites.
Closes#32444 from AngersZhuuuu/SPARK-35111-followup.
Authored-by: Angerszhuuuu <angers.zhu@gmail.com>
Signed-off-by: Max Gekk <max.gekk@gmail.com>
### What changes were proposed in this pull request?
when `numSlices` is avaiable, `logical.Range` should compute a exact `maxRowsPerPartition`
### Why are the changes needed?
`maxRowsPerPartition` is used in optimizer, we should provide an exact value if possible
### Does this PR introduce _any_ user-facing change?
No
### How was this patch tested?
existing testsuites
Closes#32350 from zhengruifeng/range_maxRowsPerPartition.
Authored-by: Ruifeng Zheng <ruifengz@foxmail.com>
Signed-off-by: Takeshi Yamamuro <yamamuro@apache.org>
### What changes were proposed in this pull request?
This patch proposes to use `MethodUtils` for looking up methods `Invoke` and `StaticInvoke` expressions.
### Why are the changes needed?
Currently we wrote our logic in `Invoke` and `StaticInvoke` expressions for looking up methods. It is tricky to consider all the cases and there is already existing utility package for this purpose. We should reuse the utility package.
### Does this PR introduce _any_ user-facing change?
No, internal change only.
### How was this patch tested?
Existing tests.
Closes#32474 from viirya/invoke-util.
Authored-by: Liang-Chi Hsieh <viirya@gmail.com>
Signed-off-by: Dongjoon Hyun <dongjoon@apache.org>
### What changes were proposed in this pull request?
This PR makes the below case work well.
```sql
select a b from values(1) t(a) distribute by a;
```
```logtalk
== Parsed Logical Plan ==
'RepartitionByExpression ['a]
+- 'Project ['a AS b#42]
+- 'SubqueryAlias t
+- 'UnresolvedInlineTable [a], [List(1)]
== Analyzed Logical Plan ==
org.apache.spark.sql.AnalysisException: cannot resolve 'a' given input columns: [b]; line 1 pos 62;
'RepartitionByExpression ['a]
+- Project [a#48 AS b#42]
+- SubqueryAlias t
+- LocalRelation [a#48]
```
### Why are the changes needed?
bugfix
### Does this PR introduce _any_ user-facing change?
yes, the original attributes can be used in `distribute by` / `cluster by` and hints like `/*+ REPARTITION(3, c) */`
### How was this patch tested?
new tests
Closes#32465 from yaooqinn/SPARK-35331.
Authored-by: Kent Yao <yao@apache.org>
Signed-off-by: Dongjoon Hyun <dongjoon@apache.org>
### What changes were proposed in this pull request?
Retain column metadata during the process of nested column pruning, when constructing `StructField`.
To test the above change, this also added the logic of column projection in `InMemoryTable`. Without the fix `DSV2CharVarcharDDLTestSuite` will fail.
### Why are the changes needed?
The column metadata is used in a few places such as re-constructing CHAR/VARCHAR information such as in [SPARK-33901](https://issues.apache.org/jira/browse/SPARK-33901). Therefore, we should retain the info during nested column pruning.
### Does this PR introduce _any_ user-facing change?
No
### How was this patch tested?
Existing tests.
Closes#32354 from sunchao/SPARK-35232.
Authored-by: Chao Sun <sunchao@apache.org>
Signed-off-by: Liang-Chi Hsieh <viirya@gmail.com>
### What changes were proposed in this pull request?
This allows `ScalarFunction` implemented in Java to optionally specify the magic method `invoke` to be static, which can be used if the UDF is stateless. Comparing to the non-static method, it can potentially give better performance due to elimination of dynamic dispatch, etc.
Also added a benchmark to measure performance of: the default `produceResult`, non-static magic method and static magic method.
### Why are the changes needed?
For UDFs that are stateless (e.g., no need to maintain intermediate state between each function call), it's better to allow users to implement the UDF function as static method which could potentially give better performance.
### Does this PR introduce _any_ user-facing change?
Yes. Spark users can now have the choice to define static magic method for `ScalarFunction` when it is written in Java and when the UDF is stateless.
### How was this patch tested?
Added new UT.
Closes#32407 from sunchao/SPARK-35261.
Authored-by: Chao Sun <sunchao@apache.org>
Signed-off-by: Dongjoon Hyun <dhyun@apple.com>
### What changes were proposed in this pull request?
This patch proposes to make StaticInvoke able to find method with given method name even the parameter types do not exactly match to argument classes.
### Why are the changes needed?
Unlike `Invoke`, `StaticInvoke` only tries to get the method with exact argument classes. If the calling method's parameter types are not exactly matched with the argument classes, `StaticInvoke` cannot find the method.
`StaticInvoke` should be able to find the method under the cases too.
### Does this PR introduce _any_ user-facing change?
Yes. `StaticInvoke` can find a method even the argument classes are not exactly matched.
### How was this patch tested?
Unit test.
Closes#32413 from viirya/static-invoke.
Authored-by: Liang-Chi Hsieh <viirya@gmail.com>
Signed-off-by: Liang-Chi Hsieh <viirya@gmail.com>
### What changes were proposed in this pull request?
This PR group exception messages in `sql/catalyst/src/main/scala/org/apache/spark/sql/connector/catalog`.
### Why are the changes needed?
It will largely help with standardization of error messages and its maintenance.
### Does this PR introduce _any_ user-facing change?
No. Error messages remain unchanged.
### How was this patch tested?
No new tests - pass all original tests to make sure it doesn't break any existing behavior.
Closes#32377 from beliefer/SPARK-35021.
Lead-authored-by: beliefer <beliefer@163.com>
Co-authored-by: gengjiaan <gengjiaan@360.cn>
Co-authored-by: Jiaan Geng <beliefer@163.com>
Signed-off-by: Wenchen Fan <wenchen@databricks.com>
### What changes were proposed in this pull request?
Added the following TreePattern enums:
- APPEND_COLUMNS
- DESERIALIZE_TO_OBJECT
- LAMBDA_VARIABLE
- MAP_OBJECTS
- SERIALIZE_FROM_OBJECT
- PROJECT
- TYPED_FILTER
Added tree traversal pruning to the following rules dealing with objects:
- EliminateSerialization
- CombineTypedFilters
- EliminateMapObjects
- ObjectSerializerPruning
### Why are the changes needed?
Reduce the number of tree traversals and hence improve the query compilation latency.
### How was this patch tested?
Existing tests.
Closes#32451 from sigmod/object.
Authored-by: Yingyi Bu <yingyi.bu@databricks.com>
Signed-off-by: Gengliang Wang <ltnwgl@gmail.com>
### What changes were proposed in this pull request?
If `targetObject` is not nullable, we don't need the object null check in `Invoke`.
### Why are the changes needed?
small perf improvement
### Does this PR introduce _any_ user-facing change?
no
### How was this patch tested?
existing tests
Closes#32466 from cloud-fan/invoke.
Authored-by: Wenchen Fan <wenchen@databricks.com>
Signed-off-by: Wenchen Fan <wenchen@databricks.com>
### What changes were proposed in this pull request?
This PR group exception messages in `sql/catalyst/src/main/scala/org/apache/spark/sql/catalyst/util`.
### Why are the changes needed?
It will largely help with standardization of error messages and its maintenance.
### Does this PR introduce _any_ user-facing change?
No. Error messages remain unchanged.
### How was this patch tested?
No new tests - pass all original tests to make sure it doesn't break any existing behavior.
Closes#32367 from beliefer/SPARK-35020.
Lead-authored-by: gengjiaan <gengjiaan@360.cn>
Co-authored-by: beliefer <beliefer@163.com>
Signed-off-by: Wenchen Fan <wenchen@databricks.com>
### What changes were proposed in this pull request?
This is a follow up to https://github.com/apache/spark/pull/32032#discussion_r620928086. Basically, `children`/`innerChildren` should be mutually exclusive for `AlterViewAsCommand` and `CreateViewCommand`, which extend `AnalysisOnlyCommand`. Otherwise, there could be an issue in the `EXPLAIN` command. Currently, this is not an issue, because these commands will be analyzed (children will always be empty) when the `EXPLAIN` command is run.
### Why are the changes needed?
To be future-proof where these commands are directly used.
### Does this PR introduce _any_ user-facing change?
No.
### How was this patch tested?
Added new tsts
Closes#32447 from imback82/SPARK-34701-followup.
Authored-by: Terry Kim <yuminkim@gmail.com>
Signed-off-by: Wenchen Fan <wenchen@databricks.com>
### What changes were proposed in this pull request?
Hide internal view properties for describe table command, because those
properties are generated by spark and should be transparent to the end-user.
### Why are the changes needed?
Avoid internal properties confusing the users.
### Does this PR introduce _any_ user-facing change?
Yes
Before this change, the user will see below output for `describe formatted test_view`
```
....
Table Properties [view.catalogAndNamespace.numParts=2, view.catalogAndNamespace.part.0=spark_catalog, view.catalogAndNamespace.part.1=default, view.query.out.col.0=c, view.query.out.col.1=v, view.query.out.numCols=2, view.referredTempFunctionsNames=[], view.referredTempViewNames=[]]
...
```
After this change, the internal properties will be hidden for `describe formatted test_view`
```
...
Table Properties []
...
```
### How was this patch tested?
existing UT
Closes#32441 from linhongliu-db/hide-properties.
Authored-by: Linhong Liu <linhong.liu@databricks.com>
Signed-off-by: Wenchen Fan <wenchen@databricks.com>
### What changes were proposed in this pull request?
This pull request proposes a new API for streaming sources to signal that they can report metrics, and adds a use case to support Kafka micro batch stream to report the stats of # of offsets for the current offset falling behind the latest.
A public interface is added.
`metrics`: returns the metrics reported by the streaming source with given offset.
### Why are the changes needed?
The new API can expose any custom metrics for the "current" offset for streaming sources. Different from #31398, this PR makes metrics available to user through progress report, not through spark UI. A use case is that people want to know how the current offset falls behind the latest offset.
### Does this PR introduce _any_ user-facing change?
No.
### How was this patch tested?
Unit test for Kafka micro batch source v2 are added to test the Kafka use case.
Closes#31944 from yijiacui-db/SPARK-34297.
Authored-by: Yijia Cui <yijia.cui@databricks.com>
Signed-off-by: Jungtaek Lim <kabhwan.opensource@gmail.com>
### What changes were proposed in this pull request?
To fix lambda variable name issues in nested DataFrame functions, this PR modifies code to use a global counter for `LambdaVariables` names created by higher order functions.
This is the rework of #31887. Closes#31887.
### Why are the changes needed?
This moves away from the current hard-coded variable names which break on nested function calls. There is currently a bug where nested transforms in particular fail (the inner variable shadows the outer variable)
For this query:
```
val df = Seq(
(Seq(1,2,3), Seq("a", "b", "c"))
).toDF("numbers", "letters")
df.select(
f.flatten(
f.transform(
$"numbers",
(number: Column) => { f.transform(
$"letters",
(letter: Column) => { f.struct(
number.as("number"),
letter.as("letter")
) }
) }
)
).as("zipped")
).show(10, false)
```
This is the current (incorrect) output:
```
+------------------------------------------------------------------------+
|zipped |
+------------------------------------------------------------------------+
|[{a, a}, {b, b}, {c, c}, {a, a}, {b, b}, {c, c}, {a, a}, {b, b}, {c, c}]|
+------------------------------------------------------------------------+
```
And this is the correct output after fix:
```
+------------------------------------------------------------------------+
|zipped |
+------------------------------------------------------------------------+
|[{1, a}, {1, b}, {1, c}, {2, a}, {2, b}, {2, c}, {3, a}, {3, b}, {3, c}]|
+------------------------------------------------------------------------+
```
### Does this PR introduce _any_ user-facing change?
No
### How was this patch tested?
Added the new test in `DataFrameFunctionsSuite`.
Closes#32424 from maropu/pr31887.
Lead-authored-by: dsolow <dsolow@sayari.com>
Co-authored-by: Takeshi Yamamuro <yamamuro@apache.org>
Co-authored-by: dmsolow <dsolow@sayarianalytics.com>
Signed-off-by: Takeshi Yamamuro <yamamuro@apache.org>
### What changes were proposed in this pull request?
Added the following TreePattern enums:
- CREATE_NAMED_STRUCT
- EXTRACT_VALUE
- JSON_TO_STRUCT
- OUTER_REFERENCE
- AGGREGATE
- LOCAL_RELATION
- EXCEPT
- LIMIT
- WINDOW
Used them in the following rules:
- DecorrelateInnerQuery
- LimitPushDownThroughWindow
- OptimizeCsvJsonExprs
- PropagateEmptyRelation
- PullOutGroupingExpressions
- PushLeftSemiLeftAntiThroughJoin
- ReplaceExceptWithFilter
- RewriteDistinctAggregates
- SimplifyConditionalsInPredicate
- UnwrapCastInBinaryComparison
### Why are the changes needed?
Reduce the number of tree traversals and hence improve the query compilation latency.
### How was this patch tested?
Existing tests.
Closes#32421 from sigmod/opt.
Authored-by: Yingyi Bu <yingyi.bu@databricks.com>
Signed-off-by: Gengliang Wang <ltnwgl@gmail.com>
### What changes were proposed in this pull request?
In `StaticInvoke`, when result is nullable, don't box the return value if its type is primitive.
### Why are the changes needed?
It is unnecessary to apply boxing when the method return value is of primitive type, and it would hurt performance a lot if the method is simple. The check is done in `Invoke` but not in `StaticInvoke`.
### Does this PR introduce _any_ user-facing change?
No
### How was this patch tested?
Added a UT.
Closes#32416 from sunchao/SPARK-35281.
Authored-by: Chao Sun <sunchao@apache.org>
Signed-off-by: HyukjinKwon <gurwls223@apache.org>
### What changes were proposed in this pull request?
1. Extend Spark SQL parser to support parsing of:
- `INTERVAL YEAR TO MONTH` to `YearMonthIntervalType`
- `INTERVAL DAY TO SECOND` to `DayTimeIntervalType`
2. Assign new names to the ANSI interval types according to the SQL standard to be able to parse the names back by Spark SQL parser. Override the `typeName()` name of `YearMonthIntervalType`/`DayTimeIntervalType`.
### Why are the changes needed?
To be able to use new ANSI interval types in SQL. The SQL standard requires the types to be defined according to the rules:
```
<interval type> ::= INTERVAL <interval qualifier>
<interval qualifier> ::= <start field> TO <end field> | <single datetime field>
<start field> ::= <non-second primary datetime field> [ <left paren> <interval leading field precision> <right paren> ]
<end field> ::= <non-second primary datetime field> | SECOND [ <left paren> <interval fractional seconds precision> <right paren> ]
<primary datetime field> ::= <non-second primary datetime field | SECOND
<non-second primary datetime field> ::= YEAR | MONTH | DAY | HOUR | MINUTE
<interval fractional seconds precision> ::= <unsigned integer>
<interval leading field precision> ::= <unsigned integer>
```
Currently, Spark SQL supports only `YEAR TO MONTH` and `DAY TO SECOND` as `<interval qualifier>`.
### Does this PR introduce _any_ user-facing change?
Should not since the types has not been released yet.
### How was this patch tested?
By running the affected tests such as:
```
$ build/sbt "sql/testOnly *SQLQueryTestSuite -- -z interval.sql"
$ build/sbt "sql/testOnly *SQLQueryTestSuite -- -z datetime.sql"
$ build/sbt "test:testOnly *ExpressionTypeCheckingSuite"
$ build/sbt "sql/testOnly *SQLQueryTestSuite -- -z windowFrameCoercion.sql"
$ build/sbt "sql/testOnly *SQLQueryTestSuite -- -z literals.sql"
```
Closes#32409 from MaxGekk/parse-ansi-interval-types.
Authored-by: Max Gekk <max.gekk@gmail.com>
Signed-off-by: HyukjinKwon <gurwls223@apache.org>
### What changes were proposed in this pull request?
Support Cast string to day-seconds interval
### Why are the changes needed?
Users can cast day-second interval string to DayTimeIntervalType.
### Does this PR introduce _any_ user-facing change?
No
### How was this patch tested?
Added UT
Closes#32271 from AngersZhuuuu/SPARK-35112.
Lead-authored-by: Angerszhuuuu <angers.zhu@gmail.com>
Co-authored-by: AngersZhuuuu <angers.zhu@gmail.com>
Signed-off-by: Max Gekk <max.gekk@gmail.com>
### What changes were proposed in this pull request?
This PR adds a new rule `PullOutGroupingExpressions` to pull out complex grouping expressions to a `Project` node under an `Aggregate`. These expressions are then referenced in both grouping expressions and aggregate expressions without aggregate functions to ensure that optimization rules don't change the aggregate expressions to invalid ones that no longer refer to any grouping expressions.
### Why are the changes needed?
If aggregate expressions (without aggregate functions) in an `Aggregate` node are complex then the `Optimizer` can optimize out grouping expressions from them and so making aggregate expressions invalid.
Here is a simple example:
```
SELECT not(t.id IS NULL) , count(*)
FROM t
GROUP BY t.id IS NULL
```
In this case the `BooleanSimplification` rule does this:
```
=== Applying Rule org.apache.spark.sql.catalyst.optimizer.BooleanSimplification ===
!Aggregate [isnull(id#222)], [NOT isnull(id#222) AS (NOT (id IS NULL))#226, count(1) AS c#224L] Aggregate [isnull(id#222)], [isnotnull(id#222) AS (NOT (id IS NULL))#226, count(1) AS c#224L]
+- Project [value#219 AS id#222] +- Project [value#219 AS id#222]
+- LocalRelation [value#219] +- LocalRelation [value#219]
```
where `NOT isnull(id#222)` is optimized to `isnotnull(id#222)` and so it no longer refers to any grouping expression.
Before this PR:
```
== Optimized Logical Plan ==
Aggregate [isnull(id#222)], [isnotnull(id#222) AS (NOT (id IS NULL))#234, count(1) AS c#232L]
+- Project [value#219 AS id#222]
+- LocalRelation [value#219]
```
and running the query throws an error:
```
Couldn't find id#222 in [isnull(id#222)#230,count(1)#226L]
java.lang.IllegalStateException: Couldn't find id#222 in [isnull(id#222)#230,count(1)#226L]
```
After this PR:
```
== Optimized Logical Plan ==
Aggregate [_groupingexpression#233], [NOT _groupingexpression#233 AS (NOT (id IS NULL))#230, count(1) AS c#228L]
+- Project [isnull(value#219) AS _groupingexpression#233]
+- LocalRelation [value#219]
```
and the query works.
### Does this PR introduce _any_ user-facing change?
Yes, the query works.
### How was this patch tested?
Added new UT.
Closes#32396 from peter-toth/SPARK-34581-keep-grouping-expressions-2.
Authored-by: Peter Toth <peter.toth@gmail.com>
Signed-off-by: Wenchen Fan <wenchen@databricks.com>
### What changes were proposed in this pull request?
This patch fixes `Invoke` expression when the target object has more than one method with the given method name.
### Why are the changes needed?
`Invoke` will find out the method on the target object with given method name. If there are more than one method with the name, currently it is undeterministic which method will be used. We should add the condition of parameter number when finding the method.
### Does this PR introduce _any_ user-facing change?
Yes, fixed a bug when using `Invoke` on a object where more than one method with the given method name.
### How was this patch tested?
Unit test.
Closes#32404 from viirya/verify-invoke-param-len.
Authored-by: Liang-Chi Hsieh <viirya@gmail.com>
Signed-off-by: Liang-Chi Hsieh <viirya@gmail.com>
### What changes were proposed in this pull request?
This pr makes `CombineFilters` support non-deterministic expressions. For example:
```sql
spark.sql("CREATE TABLE t1(id INT, dt STRING) using parquet PARTITIONED BY (dt)")
spark.sql("CREATE VIEW v1 AS SELECT * FROM t1 WHERE dt NOT IN ('2020-01-01', '2021-01-01')")
spark.sql("SELECT * FROM v1 WHERE dt = '2021-05-01' AND rand() <= 0.01").explain()
```
Before this pr:
```
== Physical Plan ==
*(1) Filter (isnotnull(dt#1) AND ((dt#1 = 2021-05-01) AND (rand(-6723800298719475098) <= 0.01)))
+- *(1) ColumnarToRow
+- FileScan parquet default.t1[id#0,dt#1] Batched: true, DataFilters: [], Format: Parquet, Location: InMemoryFileIndex(0 paths)[], PartitionFilters: [NOT dt#1 IN (2020-01-01,2021-01-01)], PushedFilters: [], ReadSchema: struct<id:int>
```
After this pr:
```
== Physical Plan ==
*(1) Filter (rand(-2400509328955813273) <= 0.01)
+- *(1) ColumnarToRow
+- FileScan parquet default.t1[id#0,dt#1] Batched: true, DataFilters: [], Format: Parquet, Location: InMemoryFileIndex(0 paths)[], PartitionFilters: [isnotnull(dt#1), NOT dt#1 IN (2020-01-01,2021-01-01), (dt#1 = 2021-05-01)], PushedFilters: [], ReadSchema: struct<id:int>
```
### Why are the changes needed?
Improve query performance.
### Does this PR introduce _any_ user-facing change?
No.
### How was this patch tested?
Unit test.
Closes#32405 from wangyum/SPARK-35273.
Authored-by: Yuming Wang <yumwang@ebay.com>
Signed-off-by: Wenchen Fan <wenchen@databricks.com>
### What changes were proposed in this pull request?
~~This PR aims to add a new AQE optimizer rule `DynamicJoinSelection`. Like other AQE partition number configs, this rule add a new broadcast threshold config `spark.sql.adaptive.autoBroadcastJoinThreshold`.~~
This PR amis to add a flag in `Statistics` to distinguish AQE stats or normal stats, so that we can make some sql configs isolation between AQE and normal.
### Why are the changes needed?
The main idea here is that make join config isolation between normal planner and aqe planner which shared the same code path.
Actually we do not very trust using the static stats to consider if it can build broadcast hash join. In our experience it's very common that Spark throw broadcast timeout or driver side OOM exception when execute a bit large plan. And due to braodcast join is not reversed which means if we covert join to braodcast hash join at first time, we(AQE) can not optimize it again, so it should make sense to decide if we can do broadcast at aqe side using different sql config.
### Does this PR introduce _any_ user-facing change?
Yes, a new config `spark.sql.adaptive.autoBroadcastJoinThreshold` added.
### How was this patch tested?
Add new test.
Closes#32391 from ulysses-you/SPARK-35264.
Authored-by: ulysses-you <ulyssesyou18@gmail.com>
Signed-off-by: Wenchen Fan <wenchen@databricks.com>
### What changes were proposed in this pull request?
Support Cast string to year-month interval
Supported format as below
```
ANSI_STYLE, like
INTERVAL -'-10-1' YEAR TO MONTH
HIVE_STYLE like
10-1 or -10-1
Rules from the SQL standard about ANSI_STYLE:
<interval literal> ::=
INTERVAL [ <sign> ] <interval string> <interval qualifier>
<interval string> ::=
<quote> <unquoted interval string> <quote>
<unquoted interval string> ::=
[ <sign> ] { <year-month literal> | <day-time literal> }
<year-month literal> ::=
<years value> [ <minus sign> <months value> ]
| <months value>
<years value> ::=
<datetime value>
<months value> ::=
<datetime value>
<datetime value> ::=
<unsigned integer>
<unsigned integer> ::= <digit>...
```
### Why are the changes needed?
Support Cast string to year-month interval
### Does this PR introduce _any_ user-facing change?
User can cast year month interval string to YearMonthIntervalType
### How was this patch tested?
Added UT
Closes#32266 from AngersZhuuuu/SPARK-SPARK-35111.
Authored-by: Angerszhuuuu <angers.zhu@gmail.com>
Signed-off-by: Max Gekk <max.gekk@gmail.com>
### What changes were proposed in this pull request?
This PR proposes to enable the JSON datasources to write non-ascii characters as codepoints.
To enable/disable this feature, I introduce a new option `writeNonAsciiCharacterAsCodePoint` for JSON datasources.
### Why are the changes needed?
JSON specification allows codepoints as literal but Spark SQL's JSON datasources don't support the way to do it.
It's great if we can write non-ascii characters as codepoints, which is a platform neutral representation.
### Does this PR introduce _any_ user-facing change?
Yes. Users can write non-ascii characters as codepoints with JSON datasources.
### How was this patch tested?
New test.
Closes#32147 from sarutak/json-unicode-write.
Authored-by: Kousuke Saruta <sarutak@oss.nttdata.com>
Signed-off-by: Dongjoon Hyun <dhyun@apple.com>
### What changes were proposed in this pull request?
Make sure we re-throw an exception that is not null.
### Why are the changes needed?
to be super safe
### Does this PR introduce _any_ user-facing change?
no
### How was this patch tested?
N/A
Closes#32387 from cloud-fan/minor.
Authored-by: Wenchen Fan <wenchen@databricks.com>
Signed-off-by: Takeshi Yamamuro <yamamuro@apache.org>
Co-Authored-By: Chao Sun <sunchaoapple.com>
Co-Authored-By: Ryan Blue <rbluenetflix.com>
### What changes were proposed in this pull request?
This implements function resolution and evaluation for functions registered through V2 FunctionCatalog [SPARK-27658](https://issues.apache.org/jira/browse/SPARK-27658). In particular:
- Added documentation for how to define the "magic method" in `ScalarFunction`.
- Added a new expression `ApplyFunctionExpression` which evaluates input by delegating to `ScalarFunction.produceResult` method.
- added a new expression `V2Aggregator` which is a type of `TypedImperativeAggregate`. It's a wrapper of V2 `AggregateFunction` and mostly delegate methods to the implementation of the latter. It also uses plain Java serde for intermediate state.
- Added function resolution logic for `ScalarFunction` and `AggregateFunction` in `Analyzer`.
+ For `ScalarFunction` this checks if the magic method is implemented through Java reflection, and create a `Invoke` expression if so. Otherwise, it checks if the default `produceResult` is overridden. If so, it creates a `ApplyFunctionExpression` which evaluates through `InternalRow`. Otherwise an analysis exception is thrown.
+ For `AggregateFunction`, this checks if the `update` method is overridden. If so, it converts it to `V2Aggregator`. Otherwise an analysis exception is thrown similar to the case of `ScalarFunction`.
- Extended existing `InMemoryTableCatalog` to add the function catalog capability. Also renamed it to `InMemoryCatalog` since it no longer only covers tables.
**Note**: this currently can successfully detect whether a subclass overrides the default `produceResult` or `update` method from the parent interface **only for Java implementations**. It seems in Scala it's hard to differentiate whether a subclass overrides a default method from its parent interface. In this case, it will be a runtime error instead of analysis error.
A few TODOs:
- Extend `V2SessionCatalog` with function catalog. This seems a little tricky since API such V2 `FunctionCatalog`'s `loadFunction` is different from V1 `SessionCatalog`'s `lookupFunction`.
- Add magic method for `AggregateFunction`.
- Type coercion when looking up functions
### Why are the changes needed?
As V2 FunctionCatalog APIs are finalized, we should integrate it with function resolution and evaluation process so that they are actually useful.
### Does this PR introduce _any_ user-facing change?
Yes, now a function exposed through V2 FunctionCatalog can be analyzed and evaluated.
### How was this patch tested?
Added new unit tests.
Closes#32082 from sunchao/resolve-func-v2.
Lead-authored-by: Chao Sun <sunchao@apple.com>
Co-authored-by: Chao Sun <sunchao@apache.org>
Co-authored-by: Chao Sun <sunchao@uber.com>
Signed-off-by: Wenchen Fan <wenchen@databricks.com>
### What changes were proposed in this pull request?
Add `ShuffledHashJoin` pattern check in `OptimizeSkewedJoin` so that we can optimize it.
### Why are the changes needed?
Currently, we have already supported all type of join through hint that make it easy to choose the join implementation.
We would choose `ShuffledHashJoin` if one table is not big but over the broadcast threshold. It's better that we can support optimize it in `OptimizeSkewedJoin`.
### Does this PR introduce _any_ user-facing change?
Probably yes, the execute plan in AQE mode may be changed.
### How was this patch tested?
Improve exists test in `AdaptiveQueryExecSuite`
Closes#32328 from ulysses-you/SPARK-35214.
Authored-by: ulysses-you <ulyssesyou18@gmail.com>
Signed-off-by: Takeshi Yamamuro <yamamuro@apache.org>
### What changes were proposed in this pull request?
The UnsupportedOperationChecker shouldn't allow streaming-batch intersects. As described in the ticket, they can't actually be planned correctly, and even simple cases like the below will fail:
```
test("intersect") {
val input = MemoryStream[Long]
val df = input.toDS().intersect(spark.range(10).as[Long])
testStream(df) (
AddData(input, 1L),
CheckAnswer(1)
)
}
```
### Why are the changes needed?
Users will be confused by the cryptic errors produced from trying to run an invalid query plan.
### Does this PR introduce _any_ user-facing change?
Some queries which previously failed with a poor error will now fail with a better one.
### How was this patch tested?
modified unit test
Closes#32371 from jose-torres/ossthing.
Authored-by: Jose Torres <joseph.torres@databricks.com>
Signed-off-by: hyukjinkwon <gurwls223@apache.org>
### What changes were proposed in this pull request?
This PR updates the interpreted code path of invoke expressions, to unwrap the `InvocationTargetException`
### Why are the changes needed?
Make interpreted and codegen path consistent for invoke expressions.
### Does this PR introduce _any_ user-facing change?
no
### How was this patch tested?
new UT
Closes#32370 from cloud-fan/minor.
Authored-by: Wenchen Fan <wenchen@databricks.com>
Signed-off-by: hyukjinkwon <gurwls223@apache.org>
### What changes were proposed in this pull request?
In this PR, we add extract/date_part support for ANSI Intervals
The `extract` is an ANSI expression and `date_part` is NON-ANSI but exists as an equivalence for `extract`
#### expression
```
<extract expression> ::=
EXTRACT <left paren> <extract field> FROM <extract source> <right paren>
```
#### <extract field> for interval source
```
<primary datetime field> ::=
<non-second primary datetime field>
| SECOND
<non-second primary datetime field> ::=
YEAR
| MONTH
| DAY
| HOUR
| MINUTE
```
#### dataType
```
If <extract field> is a <primary datetime field> that does not specify SECOND or <extract field> is not a <primary datetime field>, then the declared type of the result is an implementation-defined exact numeric type with scale 0 (zero)
Otherwise, the declared type of the result is an implementation-defined exact numeric type with scale not less than the specified or implied <time fractional seconds precision> or <interval fractional seconds precision>, as appropriate, of the SECOND <primary datetime field> of the <extract source>.
```
### Why are the changes needed?
Subtask of ANSI Intervals Support
### Does this PR introduce _any_ user-facing change?
Yes
1. extract/date_part support ANSI intervals
2. for non-ansi intervals, the return type is changed from long to byte when extracting hours
### How was this patch tested?
new added tests
Closes#32351 from yaooqinn/SPARK-35091.
Authored-by: Kent Yao <yao@apache.org>
Signed-off-by: Wenchen Fan <wenchen@databricks.com>
### What changes were proposed in this pull request?
https://github.com/apache/spark/pull/32229 support ANSI SQL intervals by the aggregate function `avg`.
But have not treat that the input zero rows. so this will lead to:
```
Caused by: java.lang.ArithmeticException: / by zero
at com.google.common.math.LongMath.divide(LongMath.java:367)
at org.apache.spark.sql.catalyst.expressions.GeneratedClass$GeneratedIteratorForCodegenStage2.processNext(Unknown Source)
at org.apache.spark.sql.execution.BufferedRowIterator.hasNext(BufferedRowIterator.java:43)
at org.apache.spark.sql.execution.WholeStageCodegenExec$$anon$1.hasNext(WholeStageCodegenExec.scala:759)
at scala.collection.Iterator$$anon$10.hasNext(Iterator.scala:458)
at scala.collection.Iterator$$anon$10.hasNext(Iterator.scala:458)
at org.apache.spark.util.Utils$.getIteratorSize(Utils.scala:1864)
at org.apache.spark.rdd.RDD.$anonfun$count$1(RDD.scala:1253)
at org.apache.spark.rdd.RDD.$anonfun$count$1$adapted(RDD.scala:1253)
at org.apache.spark.SparkContext.$anonfun$runJob$5(SparkContext.scala:2248)
at org.apache.spark.scheduler.ResultTask.runTask(ResultTask.scala:90)
at org.apache.spark.scheduler.Task.run(Task.scala:131)
at org.apache.spark.executor.Executor$TaskRunner.$anonfun$run$3(Executor.scala:498)
at org.apache.spark.util.Utils$.tryWithSafeFinally(Utils.scala:1437)
at org.apache.spark.executor.Executor$TaskRunner.run(Executor.scala:501)
at java.util.concurrent.ThreadPoolExecutor.runWorker(ThreadPoolExecutor.java:1149)
at java.util.concurrent.ThreadPoolExecutor$Worker.run(ThreadPoolExecutor.java:624)
at java.lang.Thread.run(Thread.java:748)
```
### Why are the changes needed?
Fix a bug.
### Does this PR introduce _any_ user-facing change?
No. Just new feature.
### How was this patch tested?
new tests.
Closes#32358 from beliefer/SPARK-34837-followup.
Authored-by: gengjiaan <gengjiaan@360.cn>
Signed-off-by: Max Gekk <max.gekk@gmail.com>
### What changes were proposed in this pull request?
Before this patch
```
scala> Seq(java.time.Period.ofMonths(Int.MinValue)).toDF("i").select($"i" / -1).show(false)
+-------------------------------------+
|(i / -1) |
+-------------------------------------+
|INTERVAL '-178956970-8' YEAR TO MONTH|
+-------------------------------------+
scala> Seq(java.time.Duration.of(Long.MinValue, java.time.temporal.ChronoUnit.MICROS)).toDF("i").select($"i" / -1).show(false)
+---------------------------------------------------+
|(i / -1) |
+---------------------------------------------------+
|INTERVAL '-106751991 04:00:54.775808' DAY TO SECOND|
+---------------------------------------------------+
```
Wrong result of min ANSI interval division by -1, this pr fix this
### Why are the changes needed?
Fix bug
### Does this PR introduce _any_ user-facing change?
No
### How was this patch tested?
Added UT
Closes#32314 from AngersZhuuuu/SPARK-35169.
Authored-by: Angerszhuuuu <angers.zhu@gmail.com>
Signed-off-by: Wenchen Fan <wenchen@databricks.com>
### What changes were proposed in this pull request?
Support YearMonthIntervalType and DayTimeIntervalType to extend ArrowColumnVector
### Why are the changes needed?
https://issues.apache.org/jira/browse/SPARK-35139
### Does this PR introduce _any_ user-facing change?
No
### How was this patch tested?
1. By checking coding style via:
$ ./dev/scalastyle
$ ./dev/lint-java
2. Run the test "ArrowWriterSuite"
Closes#32340 from Peng-Lei/SPARK-35139.
Authored-by: PengLei <18066542445@189.cn>
Signed-off-by: Wenchen Fan <wenchen@databricks.com>
### What changes were proposed in this pull request?
This is a re-proposal of https://github.com/apache/spark/pull/23163. Currently spark always requires a [local sort](https://github.com/apache/spark/blob/master/sql/core/src/main/scala/org/apache/spark/sql/execution/datasources/FileFormatWriter.scala#L188) before writing to output table with dynamic partition/bucket columns. The sort can be unnecessary if cardinality of partition/bucket values is small, and can be avoided by keeping multiple output writers concurrently.
This PR introduces a config `spark.sql.maxConcurrentOutputFileWriters` (which disables this feature by default), where user can tune the maximal number of concurrent writers. The config is needed here as we cannot keep arbitrary number of writers in task memory which can cause OOM (especially for Parquet/ORC vectorization writer).
The feature is to first use concurrent writers to write rows. If the number of writers exceeds the above config specified limit. Sort rest of rows and write rows one by one (See `DynamicPartitionDataConcurrentWriter.writeWithIterator()`).
In addition, interface `WriteTaskStatsTracker` and its implementation `BasicWriteTaskStatsTracker` are also changed because previously they are relying on the assumption that only one writer is active for writing dynamic partitions and bucketed table.
### Why are the changes needed?
Avoid the sort before writing output for dynamic partitioned query and bucketed table.
Help improve CPU and IO performance for these queries.
### Does this PR introduce _any_ user-facing change?
No.
### How was this patch tested?
Added unit test in `DataFrameReaderWriterSuite.scala`.
Closes#32198 from c21/writer.
Authored-by: Cheng Su <chengsu@fb.com>
Signed-off-by: Wenchen Fan <wenchen@databricks.com>
### What changes were proposed in this pull request?
This PR group exception messages in `sql/catalyst/src/main/scala/org/apache/spark/sql/types`.
### Why are the changes needed?
It will largely help with standardization of error messages and its maintenance.
### Does this PR introduce _any_ user-facing change?
No. Error messages remain unchanged.
### How was this patch tested?
No new tests - pass all original tests to make sure it doesn't break any existing behavior.
Closes#32244 from beliefer/SPARK-35060.
Lead-authored-by: beliefer <beliefer@163.com>
Co-authored-by: gengjiaan <gengjiaan@360.cn>
Signed-off-by: Wenchen Fan <wenchen@databricks.com>
### What changes were proposed in this pull request?
This patch proposes an improvement on nested column pruning if the pruning target is generator's output. Previously we disallow such case. This patch allows to prune on it if there is only one single nested column is accessed after `Generate`.
E.g., `df.select(explode($"items").as('item)).select($"item.itemId")`. As we only need `itemId` from `item`, we can prune other fields out and only keep `itemId`.
In this patch, we only address explode-like generators. We will address other generators in followups.
### Why are the changes needed?
This helps to extend the availability of nested column pruning.
### Does this PR introduce _any_ user-facing change?
No
### How was this patch tested?
Unit test
Closes#31966 from viirya/SPARK-34638.
Authored-by: Liang-Chi Hsieh <viirya@gmail.com>
Signed-off-by: Liang-Chi Hsieh <viirya@gmail.com>
### What changes were proposed in this pull request?
This patch moves DS v2 custom metric classes to `org.apache.spark.sql.connector.metric` package. Moving `CustomAvgMetric` and `CustomSumMetric` to above package and make them as public java abstract class too.
### Why are the changes needed?
`CustomAvgMetric` and `CustomSumMetric` should be public APIs for developers to extend. As there are a few metric classes, we should put them together in one package.
### Does this PR introduce _any_ user-facing change?
No, dev only and they are not released yet.
### How was this patch tested?
Unit tests.
Closes#32348 from viirya/move-custom-metric-classes.
Authored-by: Liang-Chi Hsieh <viirya@gmail.com>
Signed-off-by: Dongjoon Hyun <dhyun@apple.com>
### What changes were proposed in this pull request?
This PR makes `Sequence` expression supports ANSI intervals as step expression.
If the start and stop expression is `TimestampType,` then the step expression could select year-month or day-time interval.
If the start and stop expression is `DateType,` then the step expression must be year-month.
### Why are the changes needed?
Extends the function of `Sequence` expression.
### Does this PR introduce _any_ user-facing change?
'Yes'. Users could use ANSI intervals as step expression for `Sequence` expression.
### How was this patch tested?
New tests.
Closes#32311 from beliefer/SPARK-35088.
Lead-authored-by: beliefer <beliefer@163.com>
Co-authored-by: gengjiaan <gengjiaan@360.cn>
Signed-off-by: Max Gekk <max.gekk@gmail.com>
### What changes were proposed in this pull request?
Modifies the UpdateFields optimizer to fix correctness issues with certain nested and chained withField operations. Examples for recreating the issue are in the new unit tests as well as the JIRA issue.
### Why are the changes needed?
Certain withField patterns can cause Exceptions or even incorrect results. It appears to be a result of the additional UpdateFields optimization added in https://github.com/apache/spark/pull/29812. It traverses fieldOps in reverse order to take the last one per field, but this can cause nested structs to change order which leads to mismatches between the schema and the actual data. This updates the optimization to maintain the initial ordering of nested structs to match the generated schema.
### Does this PR introduce _any_ user-facing change?
It fixes exceptions and incorrect results for valid uses in the latest Spark release.
### How was this patch tested?
Added new unit tests for these edge cases.
Closes#32338 from Kimahriman/bug/optimize-with-fields.
Authored-by: Adam Binford <adamq43@gmail.com>
Signed-off-by: Liang-Chi Hsieh <viirya@gmail.com>
### What changes were proposed in this pull request?
In the test `"unsafe buffer with NO_CODEGEN"` of `MutableProjectionSuite`, fix unsafe buffer size calculation to be able to place all input fields without buffer overflow + meta-data.
### Why are the changes needed?
To make the test suite `MutableProjectionSuite` more stable.
### Does this PR introduce _any_ user-facing change?
No
### How was this patch tested?
By running the affected test suite:
```
$ build/sbt "test:testOnly *MutableProjectionSuite"
```
Closes#32339 from MaxGekk/fix-buffer-overflow-MutableProjectionSuite.
Authored-by: Max Gekk <max.gekk@gmail.com>
Signed-off-by: Max Gekk <max.gekk@gmail.com>
### What changes were proposed in this pull request?
`CatalystTypeConverters` is useful when the type of the input data classes are not known statically (otherwise we can use `ExpressionEncoder`). However, the current `CatalystTypeConverters` requires you to know the datetime data class statically, which makes it hard to use.
This PR improves the `CatalystTypeConverters` for date/timestamp, to support the old and new Java time classes at the same time.
### Why are the changes needed?
Make `CatalystTypeConverters` easier to use.
### Does this PR introduce _any_ user-facing change?
No.
### How was this patch tested?
new test
Closes#32312 from cloud-fan/minor.
Authored-by: Wenchen Fan <wenchen@databricks.com>
Signed-off-by: Max Gekk <max.gekk@gmail.com>
### What changes were proposed in this pull request?
Format empty grouping set exception in CUBE/ROLLUP
### Why are the changes needed?
Format empty grouping set exception in CUBE/ROLLUP
### Does this PR introduce _any_ user-facing change?
No
### How was this patch tested?
Not need
Closes#32307 from AngersZhuuuu/SPARK-35201.
Authored-by: Angerszhuuuu <angers.zhu@gmail.com>
Signed-off-by: Takeshi Yamamuro <yamamuro@apache.org>
### What changes were proposed in this pull request?
Added the following TreePattern enums:
- AND_OR
- BINARY_ARITHMETIC
- BINARY_COMPARISON
- CASE_WHEN
- CAST
- CONCAT
- COUNT
- IF
- LIKE_FAMLIY
- NOT
- NULL_CHECK
- UNARY_POSITIVE
- UPPER_OR_LOWER
Used them in the following rules:
- ConstantPropagation
- ReorderAssociativeOperator
- BooleanSimplification
- SimplifyBinaryComparison
- SimplifyCaseConversionExpressions
- SimplifyConditionals
- PushFoldableIntoBranches
- LikeSimplification
- NullPropagation
- SimplifyCasts
- RemoveDispensableExpressions
- CombineConcats
### Why are the changes needed?
Reduce the number of tree traversals and hence improve the query compilation latency.
### How was this patch tested?
Existing tests.
Closes#32280 from sigmod/expression.
Authored-by: Yingyi Bu <yingyi.bu@databricks.com>
Signed-off-by: Gengliang Wang <ltnwgl@gmail.com>
### What changes were proposed in this pull request?
For partial hash aggregation (code-gen path), we have two level of hash map for aggregation. First level is from `RowBasedHashMapGenerator`, which is computation faster compared to the second level from `UnsafeFixedWidthAggregationMap`. The introducing of two level hash map can help improve CPU performance of query as the first level hash map normally fits in hardware cache and has cheaper hash function for key lookup.
For final hash aggregation, we can also support two level of hash map, to improve query performance further.
The original two level of hash map code works for final aggregation mostly out of box. The major change here is to support testing fall back of final aggregation (see change related to `bitMaxCapacity` and `checkFallbackForGeneratedHashMap`).
Example:
An aggregation query:
```
spark.sql(
"""
|SELECT key, avg(value)
|FROM agg1
|GROUP BY key
""".stripMargin)
```
The generated code for final aggregation is [here](https://gist.github.com/c21/20c10cc8e2c7e561aafbe9b8da055242).
An aggregation query with testing fallback:
```
withSQLConf("spark.sql.TungstenAggregate.testFallbackStartsAt" -> "2, 3") {
spark.sql(
"""
|SELECT key, avg(value)
|FROM agg1
|GROUP BY key
""".stripMargin)
}
```
The generated code for final aggregation is [here](https://gist.github.com/c21/dabf176cbc18a5e2138bc0a29e81c878). Note the no more counter condition for first level fast map.
### Why are the changes needed?
Improve the CPU performance of hash aggregation query in general.
For `AggregateBenchmark."Aggregate w multiple keys"`, seeing query performance improved by 10%.
`codegen = T` means whole stage code-gen is enabled.
`hashmap = T` means two level maps is enabled for partial aggregation.
`finalhashmap = T` means two level maps is enabled for final aggregation.
```
Running benchmark: Aggregate w multiple keys
Running case: codegen = F
Stopped after 2 iterations, 8284 ms
Running case: codegen = T hashmap = F
Stopped after 2 iterations, 5424 ms
Running case: codegen = T hashmap = T finalhashmap = F
Stopped after 2 iterations, 4753 ms
Running case: codegen = T hashmap = T finalhashmap = T
Stopped after 2 iterations, 4508 ms
Java HotSpot(TM) 64-Bit Server VM 1.8.0_181-b13 on Mac OS X 10.15.7
Intel(R) Core(TM) i9-9980HK CPU 2.40GHz
Aggregate w multiple keys: Best Time(ms) Avg Time(ms) Stdev(ms) Rate(M/s) Per Row(ns) Relative
------------------------------------------------------------------------------------------------------------------------
codegen = F 3881 4142 370 5.4 185.1 1.0X
codegen = T hashmap = F 2701 2712 16 7.8 128.8 1.4X
codegen = T hashmap = T finalhashmap = F 2363 2377 19 8.9 112.7 1.6X
codegen = T hashmap = T finalhashmap = T 2252 2254 3 9.3 107.4 1.7X
```
### Does this PR introduce _any_ user-facing change?
No.
### How was this patch tested?
Existing unit test in `HashAggregationQuerySuite` and `HashAggregationQueryWithControlledFallbackSuite` already cover the test.
Closes#32242 from c21/agg.
Authored-by: Cheng Su <chengsu@fb.com>
Signed-off-by: Wenchen Fan <wenchen@databricks.com>
### What changes were proposed in this pull request?
Move the following classes:
- `InMemoryAtomicPartitionTable`
- `InMemoryPartitionTable`
- `InMemoryPartitionTableCatalog`
- `InMemoryTable`
- `InMemoryTableCatalog`
- `StagingInMemoryTableCatalog`
from `org.apache.spark.sql.connector` to `org.apache.spark.sql.connector.catalog`.
### Why are the changes needed?
These classes implement catalog related interfaces but reside in `org.apache.spark.sql.connector`. A more suitable place should be `org.apache.spark.sql.connector.catalog`.
### Does this PR introduce _any_ user-facing change?
No
### How was this patch tested?
N/A
Closes#32302 from sunchao/SPARK-35195.
Authored-by: Chao Sun <sunchao@apple.com>
Signed-off-by: Liang-Chi Hsieh <viirya@gmail.com>
### What changes were proposed in this pull request?
Added the following TreePattern enums:
- DYNAMIC_PRUNING_SUBQUERY
- EXISTS_SUBQUERY
- IN_SUBQUERY
- LIST_SUBQUERY
- PLAN_EXPRESSION
- SCALAR_SUBQUERY
- FILTER
Used them in the following rules:
- ResolveSubquery
- UpdateOuterReferences
- OptimizeSubqueries
- RewritePredicateSubquery
- PullupCorrelatedPredicates
- RewriteCorrelatedScalarSubquery (not the rule itself but an internal transform call, the full support is in SPARK-35148)
- InsertAdaptiveSparkPlan
- PlanAdaptiveSubqueries
### Why are the changes needed?
Reduce the number of tree traversals and hence improve the query compilation latency.
### How was this patch tested?
Existing tests.
Closes#32247 from sigmod/subquery.
Authored-by: Yingyi Bu <yingyi.bu@databricks.com>
Signed-off-by: Gengliang Wang <ltnwgl@gmail.com>
### What changes were proposed in this pull request?
If the sign '-' inside of interval string, everything is fine after bb5459fb26:
```
spark-sql> SELECT INTERVAL '-178956970-8' YEAR TO MONTH;
-178956970-8
```
but the sign outside of interval string is not handled properly:
```
spark-sql> SELECT INTERVAL -'178956970-8' YEAR TO MONTH;
Error in query:
Error parsing interval year-month string: integer overflow(line 1, pos 16)
== SQL ==
SELECT INTERVAL -'178956970-8' YEAR TO MONTH
----------------^^^
```
This pr fix this issue
### Why are the changes needed?
Fix bug
### Does this PR introduce _any_ user-facing change?
No
### How was this patch tested?
Added UT
Closes#32296 from AngersZhuuuu/SPARK-35187.
Authored-by: Angerszhuuuu <angers.zhu@gmail.com>
Signed-off-by: Max Gekk <max.gekk@gmail.com>
### What changes were proposed in this pull request?
This PR makes window frame could support `YearMonthIntervalType` and `DayTimeIntervalType`.
### Why are the changes needed?
Extend the function of window frame
### Does this PR introduce _any_ user-facing change?
Yes. Users could use `YearMonthIntervalType` or `DayTimeIntervalType` as the sort expression for window frame.
### How was this patch tested?
New tests
Closes#32294 from beliefer/SPARK-35110.
Authored-by: beliefer <beliefer@163.com>
Signed-off-by: Max Gekk <max.gekk@gmail.com>
### What changes were proposed in this pull request?
Use transformAllExpressions instead of transformExpressionsDown in CombineConcats. The latter only transforms the root plan node.
### Why are the changes needed?
It allows CombineConcats to cover more cases where `concat` are not in the root plan node.
### How was this patch tested?
Unit test. The updated tests would fail without the code change.
Closes#32290 from sigmod/concat.
Authored-by: Yingyi Bu <yingyi.bu@databricks.com>
Signed-off-by: Wenchen Fan <wenchen@databricks.com>
### What changes were proposed in this pull request?
PG and Oracle both support use CUBE/ROLLUP/GROUPING SETS in GROUPING SETS's grouping set as a sugar syntax.
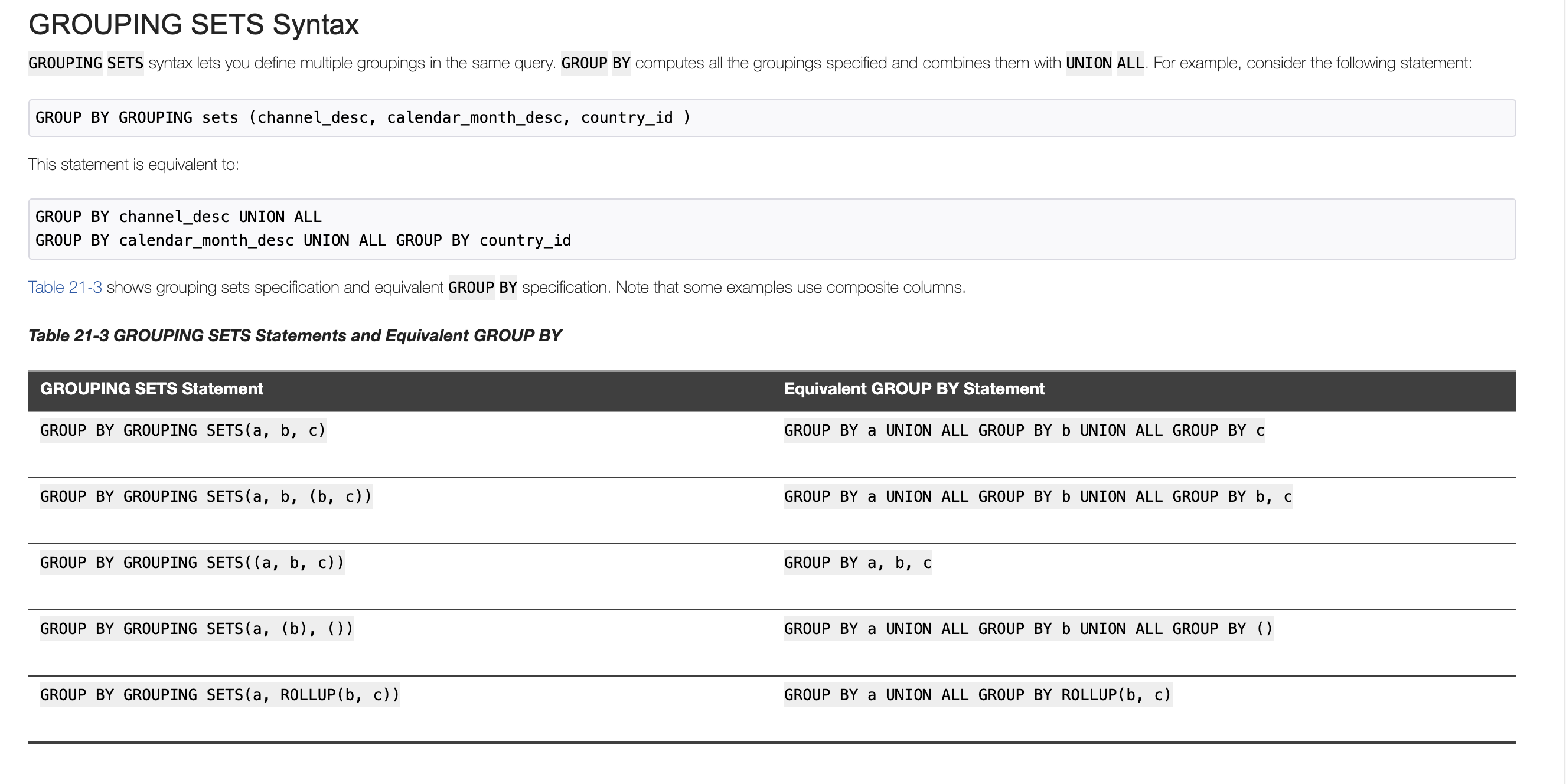
In this PR, we support it in Spark SQL too
### Why are the changes needed?
Keep consistent with PG and oracle
### Does this PR introduce _any_ user-facing change?
User can write grouping analytics like
```
SELECT a, b, count(1) FROM testData GROUP BY a, GROUPING SETS(ROLLUP(a, b));
SELECT a, b, count(1) FROM testData GROUP BY a, GROUPING SETS((a, b), (a), ());
SELECT a, b, count(1) FROM testData GROUP BY a, GROUPING SETS(GROUPING SETS((a, b), (a), ()));
```
### How was this patch tested?
Added Test
Closes#32201 from AngersZhuuuu/SPARK-35026.
Authored-by: Angerszhuuuu <angers.zhu@gmail.com>
Signed-off-by: Wenchen Fan <wenchen@databricks.com>
### What changes were proposed in this pull request?
IntervalUtils.fromYearMonthString should handle Int.MinValue months correctly.
In current logic, just use `Math.addExact(Math.multiplyExact(years, 12), months)` to calculate negative total months will overflow when actual total months is Int.MinValue, this pr fixes this bug.
### Why are the changes needed?
IntervalUtils.fromYearMonthString should handle Int.MinValue months correctly
### Does this PR introduce _any_ user-facing change?
No
### How was this patch tested?
Added UT
Closes#32281 from AngersZhuuuu/SPARK-35177.
Authored-by: Angerszhuuuu <angers.zhu@gmail.com>
Signed-off-by: Max Gekk <max.gekk@gmail.com>
### What changes were proposed in this pull request?
It will remove `StructField` when [pruning nested columns](0f2c0b53e8/sql/catalyst/src/main/scala/org/apache/spark/sql/catalyst/expressions/SchemaPruning.scala (L28-L42)). For example:
```scala
spark.sql(
"""
|CREATE TABLE t1 (
| _col0 INT,
| _col1 STRING,
| _col2 STRUCT<c1: STRING, c2: STRING, c3: STRING, c4: BIGINT>)
|USING ORC
|""".stripMargin)
spark.sql("INSERT INTO t1 values(1, '2', struct('a', 'b', 'c', 10L))")
spark.sql("SELECT _col0, _col2.c1 FROM t1").show
```
Before this pr. The returned schema is: ``` `_col0` INT,`_col2` STRUCT<`c1`: STRING> ``` add it will throw exception:
```
java.lang.AssertionError: assertion failed: The given data schema struct<_col0:int,_col2:struct<c1:string>> has less fields than the actual ORC physical schema, no idea which columns were dropped, fail to read.
at scala.Predef$.assert(Predef.scala:223)
at org.apache.spark.sql.execution.datasources.orc.OrcUtils$.requestedColumnIds(OrcUtils.scala:160)
```
After this pr. The returned schema is: ``` `_col0` INT,`_col1` STRING,`_col2` STRUCT<`c1`: STRING> ```.
The finally schema is ``` `_col0` INT,`_col2` STRUCT<`c1`: STRING> ``` after the complete column pruning:
7a5647a93a/sql/core/src/main/scala/org/apache/spark/sql/execution/datasources/FileSourceStrategy.scala (L208-L213)e64eb75aed/sql/core/src/main/scala/org/apache/spark/sql/execution/datasources/v2/PushDownUtils.scala (L96-L97)
### Why are the changes needed?
Fix bug.
### Does this PR introduce _any_ user-facing change?
No.
### How was this patch tested?
Unit test.
Closes#31993 from wangyum/SPARK-34897.
Authored-by: Yuming Wang <yumwang@ebay.com>
Signed-off-by: Liang-Chi Hsieh <viirya@gmail.com>
### What changes were proposed in this pull request?
* Add `Not(In)` and `Not(InSet)` check in `NullPropagation` rule.
* Add more test for `In` and `Not(In)` in `Project` level.
### Why are the changes needed?
The semantics of `Not(In)` could be seen like `And(a != b, a != c)` that match the `NullIntolerant`.
As we already simplify the `NullIntolerant` expression to null if it's children have null. E.g. `a != null` => `null`. It's safe to do this with `Not(In)`/`Not(InSet)`.
Note that, we can only do the simplify in predicate which `ReplaceNullWithFalseInPredicate` rule do.
Let's say we have two sqls:
```
select 1 not in (2, null);
select 1 where 1 not in (2, null);
```
The first sql we cannot optimize since it would return `NULL` instead of `false`. The second is postive.
### Does this PR introduce _any_ user-facing change?
No.
### How was this patch tested?
Add test.
Closes#31797 from ulysses-you/SPARK-34692.
Authored-by: ulysses-you <ulyssesyou18@gmail.com>
Signed-off-by: Wenchen Fan <wenchen@databricks.com>
### What changes were proposed in this pull request?
IntegralDivide should throw an exception on overflow in ANSI mode.
There is only one case that can cause that:
```
Long.MinValue div -1
```
### Why are the changes needed?
ANSI compliance
### Does this PR introduce _any_ user-facing change?
Yes, IntegralDivide throws an exception on overflow in ANSI mode
### How was this patch tested?
Unit test
Closes#32260 from gengliangwang/integralDiv.
Authored-by: Gengliang Wang <ltnwgl@gmail.com>
Signed-off-by: Gengliang Wang <ltnwgl@gmail.com>
### What changes were proposed in this pull request?
As a part of the SPARK-26837 pruning of nested fields from object serializers are supported. But it is missed to handle case insensitivity nature of spark
In this PR I have resolved the column names to be pruned based on `spark.sql.caseSensitive ` config
**Exception Before Fix**
```
Caused by: java.lang.ArrayIndexOutOfBoundsException: 0
at org.apache.spark.sql.types.StructType.apply(StructType.scala:414)
at org.apache.spark.sql.catalyst.optimizer.ObjectSerializerPruning$$anonfun$apply$4.$anonfun$applyOrElse$3(objects.scala:216)
at scala.collection.TraversableLike.$anonfun$map$1(TraversableLike.scala:238)
at scala.collection.immutable.List.foreach(List.scala:392)
at scala.collection.TraversableLike.map(TraversableLike.scala:238)
at scala.collection.TraversableLike.map$(TraversableLike.scala:231)
at scala.collection.immutable.List.map(List.scala:298)
at org.apache.spark.sql.catalyst.optimizer.ObjectSerializerPruning$$anonfun$apply$4.applyOrElse(objects.scala:215)
at org.apache.spark.sql.catalyst.optimizer.ObjectSerializerPruning$$anonfun$apply$4.applyOrElse(objects.scala:203)
at org.apache.spark.sql.catalyst.trees.TreeNode.$anonfun$transformDown$1(TreeNode.scala:309)
at org.apache.spark.sql.catalyst.trees.CurrentOrigin$.withOrigin(TreeNode.scala:72)
at org.apache.spark.sql.catalyst.trees.TreeNode.transformDown(TreeNode.scala:309)
at
```
### Why are the changes needed?
After Upgrade to Spark 3 `foreachBatch` API throws` java.lang.ArrayIndexOutOfBoundsException`. This issue will be fixed using this PR
### Does this PR introduce _any_ user-facing change?
No, Infact fixes the regression
### How was this patch tested?
Added tests and also tested verified manually
Closes#32194 from sandeep-katta/SPARK-35096.
Authored-by: sandeep.katta <sandeep.katta2007@gmail.com>
Signed-off-by: Wenchen Fan <wenchen@databricks.com>
### What changes were proposed in this pull request?
Support ANSI interval in HashExpression and add UT
### Why are the changes needed?
Support ANSI interval in HashExpression
### Does this PR introduce _any_ user-facing change?
User can pass ANSI interval in HashExpression function
### How was this patch tested?
Added UT
Closes#32259 from AngersZhuuuu/SPARK-35113.
Authored-by: Angerszhuuuu <angers.zhu@gmail.com>
Signed-off-by: Max Gekk <max.gekk@gmail.com>
### What changes were proposed in this pull request?
In the PR, I propose to override the `sql` and `toString` methods of the expressions that implement operators over ANSI intervals (`YearMonthIntervalType`/`DayTimeIntervalType`), and replace internal expression class names by operators like `*`, `/` and `-`.
### Why are the changes needed?
Proposed methods should make the textual representation of such operators more readable, and potentially parsable by Spark SQL parser.
### Does this PR introduce _any_ user-facing change?
Yes. This can influence on column names.
### How was this patch tested?
By running existing test suites for interval and datetime expressions, and re-generating the `*.sql` tests:
```
$ build/sbt "sql/testOnly *SQLQueryTestSuite -- -z interval.sql"
$ build/sbt "sql/testOnly *SQLQueryTestSuite -- -z datetime.sql"
```
Closes#32262 from MaxGekk/interval-operator-sql.
Authored-by: Max Gekk <max.gekk@gmail.com>
Signed-off-by: Max Gekk <max.gekk@gmail.com>
### What changes were proposed in this pull request?
`CurrentOrigin` is a thread-local variable to track the original SQL line position in plan/expression. Usually, we set `CurrentOrigin`, create `TreeNode` instances, and reset `CurrentOrigin`.
This PR updates the last step to set `CurrentOrigin` to its previous value, instead of resetting it. This is necessary when we invoke `CurrentOrigin` in a nested way, like with subqueries.
### Why are the changes needed?
To keep the original SQL line position in the error message in more cases.
### Does this PR introduce _any_ user-facing change?
No, only minor error message changes.
### How was this patch tested?
existing tests
Closes#32249 from cloud-fan/origin.
Authored-by: Wenchen Fan <wenchen@databricks.com>
Signed-off-by: Wenchen Fan <wenchen@databricks.com>
### What changes were proposed in this pull request?
This patch proposes to leverage `CustomMetric`, `CustomTaskMetric` API to report custom metrics from DS v2 scan to Spark.
### Why are the changes needed?
This is related to #31398. In SPARK-34297, we want to add a couple of metrics when reading from Kafka in SS. We need some public API change in DS v2 to make it possible. This extracts only DS v2 change and make it general for DS v2 instead of micro-batch DS v2 API.
### Does this PR introduce _any_ user-facing change?
No
### How was this patch tested?
Unit test.
Implement a simple test DS v2 class locally and run it:
```scala
scala> import org.apache.spark.sql.execution.datasources.v2._
import org.apache.spark.sql.execution.datasources.v2._
scala> classOf[CustomMetricDataSourceV2].getName
res0: String = org.apache.spark.sql.execution.datasources.v2.CustomMetricDataSourceV2
scala> val df = spark.read.format(res0).load()
df: org.apache.spark.sql.DataFrame = [i: int, j: int]
scala> df.collect
```
<img width="703" alt="Screen Shot 2021-03-30 at 11 07 13 PM" src="https://user-images.githubusercontent.com/68855/113098080-d8a49800-91ac-11eb-8681-be408a0f2e69.png">
Closes#31451 from viirya/dsv2-metrics.
Authored-by: Liang-Chi Hsieh <viirya@gmail.com>
Signed-off-by: Wenchen Fan <wenchen@databricks.com>
### What changes were proposed in this pull request?
Refactor ScriptTransformation to remove input parameter and replace it by child.output
### Why are the changes needed?
refactor code
### Does this PR introduce _any_ user-facing change?
No
### How was this patch tested?
Existed UT
Closes#32228 from AngersZhuuuu/SPARK-34035.
Lead-authored-by: Angerszhuuuu <angers.zhu@gmail.com>
Co-authored-by: AngersZhuuuu <angers.zhu@gmail.com>
Signed-off-by: Wenchen Fan <wenchen@databricks.com>
### What changes were proposed in this pull request?
This PR implements the decorrelation technique in the paper "Unnesting Arbitrary Queries" by T. Neumann; A. Kemper
(http://www.btw-2015.de/res/proceedings/Hauptband/Wiss/Neumann-Unnesting_Arbitrary_Querie.pdf). It currently supports Filter, Project, Aggregate, Join, and UnaryNode that passes CheckAnalysis.
This feature can be controlled by the config `spark.sql.optimizer.decorrelateInnerQuery.enabled` (default: true).
A few notes:
1. This PR does not relax any constraints in CheckAnalysis for correlated subqueries, even though some cases can be supported by this new framework, such as aggregate with correlated non-equality predicates. This PR focuses on adding the new framework and making sure all existing cases can be supported. Constraints can be relaxed gradually in the future via separate PRs.
2. The new framework is only enabled for correlated scalar subqueries, as the first step. EXISTS/IN subqueries can be supported in the future.
### Why are the changes needed?
Currently, Spark has limited support for correlated subqueries. It only allows `Filter` to reference outer query columns and does not support non-equality predicates when the subquery is aggregated. This new framework will allow more operators to host outer column references and support correlated non-equality predicates and more types of operators in correlated subqueries.
### Does this PR introduce _any_ user-facing change?
No
### How was this patch tested?
Existing unit and SQL query tests and new optimizer plan tests.
Closes#32072 from allisonwang-db/spark-34974-decorrelation.
Authored-by: allisonwang-db <66282705+allisonwang-db@users.noreply.github.com>
Signed-off-by: Wenchen Fan <wenchen@databricks.com>
### What changes were proposed in this pull request?
- Share a static ImmutableBitSet for `treePatternBits` in all object instances of AttributeReference.
- Share three static ImmutableBitSets for `treePatternBits` in three kinds of Literals.
- Add an ImmutableBitSet as a subclass of BitSet.
### Why are the changes needed?
Reduce the additional memory usage caused by `treePatternBits`.
### Does this PR introduce _any_ user-facing change?
No.
### How was this patch tested?
Existing tests.
Closes#32157 from sigmod/leaf.
Authored-by: Yingyi Bu <yingyi.bu@databricks.com>
Signed-off-by: Gengliang Wang <ltnwgl@gmail.com>
### What changes were proposed in this pull request?
This PR updated the `foundNonEqualCorrelatedPred` logic for correlated subqueries in `CheckAnalysis` to only allow correlated equality predicates that guarantee one-to-one mapping between inner and outer attributes, instead of all equality predicates.
### Why are the changes needed?
To fix correctness bugs. Before this fix Spark can give wrong results for certain correlated subqueries that pass CheckAnalysis:
Example 1:
```sql
create or replace view t1(c) as values ('a'), ('b')
create or replace view t2(c) as values ('ab'), ('abc'), ('bc')
select c, (select count(*) from t2 where t1.c = substring(t2.c, 1, 1)) from t1
```
Correct results: [(a, 2), (b, 1)]
Spark results:
```
+---+-----------------+
|c |scalarsubquery(c)|
+---+-----------------+
|a |1 |
|a |1 |
|b |1 |
+---+-----------------+
```
Example 2:
```sql
create or replace view t1(a, b) as values (0, 6), (1, 5), (2, 4), (3, 3);
create or replace view t2(c) as values (6);
select c, (select count(*) from t1 where a + b = c) from t2;
```
Correct results: [(6, 4)]
Spark results:
```
+---+-----------------+
|c |scalarsubquery(c)|
+---+-----------------+
|6 |1 |
|6 |1 |
|6 |1 |
|6 |1 |
+---+-----------------+
```
### Does this PR introduce _any_ user-facing change?
Yes. Users will not be able to run queries that contain unsupported correlated equality predicates.
### How was this patch tested?
Added unit tests.
Closes#32179 from allisonwang-db/spark-35080-subquery-bug.
Lead-authored-by: allisonwang-db <66282705+allisonwang-db@users.noreply.github.com>
Co-authored-by: Wenchen Fan <cloud0fan@gmail.com>
Signed-off-by: Wenchen Fan <wenchen@databricks.com>
## What changes were proposed in this pull request?
This PR fixes a couple of things in TypeCoercion rules:
- Only run the propagate types step if the children of a node have output attributes with changed dataTypes and/or nullability. This is implemented as custom tree transformation. The TypeCoercion rules now only implement a partial function.
- Combine multiple type coercion rules into a single rule. Multiple rules are applied in single tree traversal.
- Reduce calls to conf.get in DecimalPrecision. This now happens once per tree traversal, instead of once per matched expression.
- Reduce the use of withNewChildren.
This brings down the number of CPU cycles spend in analysis by ~28% (benchmark: 10 iterations of all TPC-DS queries on SF10).
## How was this patch tested?
Existing tests.
Closes#32208 from sigmod/coercion.
Authored-by: Yingyi Bu <yingyi.bu@databricks.com>
Signed-off-by: herman <herman@databricks.com>
### What changes were proposed in this pull request?
Remove Antlr 4.7 workaround.
### Why are the changes needed?
The https://github.com/antlr/antlr4/commit/ac9f7530 has been fixed in upstream, so remove the workaround to simplify code.
### Does this PR introduce _any_ user-facing change?
No
### How was this patch tested?
Existed UTs.
Closes#32238 from pan3793/antlr-minor.
Authored-by: Cheng Pan <379377944@qq.com>
Signed-off-by: Dongjoon Hyun <dhyun@apple.com>
### What changes were proposed in this pull request?
Similarly to the test from the PR https://github.com/apache/spark/pull/31799, add tests:
1. Months -> Period -> Months
2. Period -> Months -> Period
3. Duration -> micros -> Duration
### Why are the changes needed?
Add round trip tests for period <-> month and duration <-> micros
### Does this PR introduce _any_ user-facing change?
'No'. Just test cases.
### How was this patch tested?
Jenkins test
Closes#32234 from beliefer/SPARK-34715.
Authored-by: gengjiaan <gengjiaan@360.cn>
Signed-off-by: Max Gekk <max.gekk@gmail.com>
### What changes were proposed in this pull request?
Parse the year-month interval literals like `INTERVAL '1-1' YEAR TO MONTH` to values of `YearMonthIntervalType`, and day-time interval literals to `DayTimeIntervalType` values. Currently, Spark SQL supports:
- DAY TO HOUR
- DAY TO MINUTE
- DAY TO SECOND
- HOUR TO MINUTE
- HOUR TO SECOND
- MINUTE TO SECOND
All such interval literals are converted to `DayTimeIntervalType`, and `YEAR TO MONTH` to `YearMonthIntervalType` while loosing info about `from` and `to` units.
**Note**: new behavior is under the SQL config `spark.sql.legacy.interval.enabled` which is `false` by default. When the config is set to `true`, the interval literals are parsed to `CaledarIntervalType` values.
Closes#32176
### Why are the changes needed?
To conform the ANSI SQL standard which assumes conversions of interval literals to year-month or day-time interval but not to mixed interval type like Catalyst's `CalendarIntervalType`.
### Does this PR introduce _any_ user-facing change?
Yes.
Before:
```sql
spark-sql> SELECT INTERVAL '1 01:02:03.123' DAY TO SECOND;
1 days 1 hours 2 minutes 3.123 seconds
spark-sql> SELECT typeof(INTERVAL '1 01:02:03.123' DAY TO SECOND);
interval
```
After:
```sql
spark-sql> SELECT INTERVAL '1 01:02:03.123' DAY TO SECOND;
1 01:02:03.123000000
spark-sql> SELECT typeof(INTERVAL '1 01:02:03.123' DAY TO SECOND);
day-time interval
```
### How was this patch tested?
1. By running the affected test suites:
```
$ ./build/sbt "test:testOnly *.ExpressionParserSuite"
$ SPARK_GENERATE_GOLDEN_FILES=1 build/sbt "sql/testOnly *SQLQueryTestSuite -- -z interval.sql"
$ SPARK_GENERATE_GOLDEN_FILES=1 build/sbt "sql/testOnly *SQLQueryTestSuite -- -z create_view.sql"
$ SPARK_GENERATE_GOLDEN_FILES=1 build/sbt "sql/testOnly *SQLQueryTestSuite -- -z date.sql"
$ SPARK_GENERATE_GOLDEN_FILES=1 build/sbt "sql/testOnly *SQLQueryTestSuite -- -z timestamp.sql"
```
2. PostgresSQL tests are executed with `spark.sql.legacy.interval.enabled` is set to `true` to keep compatibility with PostgreSQL output:
```sql
> SELECT interval '999' second;
0 years 0 mons 0 days 0 hours 16 mins 39.00 secs
```
Closes#32209 from MaxGekk/parse-ansi-interval-literals.
Authored-by: Max Gekk <max.gekk@gmail.com>
Signed-off-by: Max Gekk <max.gekk@gmail.com>
### What changes were proposed in this pull request?
Extend the `Average` expression to support `DayTimeIntervalType` and `YearMonthIntervalType` added by #31614.
Note: the expressions can throw the overflow exception independently from the SQL config `spark.sql.ansi.enabled`. In this way, the modified expressions always behave in the ANSI mode for the intervals.
### Why are the changes needed?
Extend `org.apache.spark.sql.catalyst.expressions.aggregate.Average` to support `DayTimeIntervalType` and `YearMonthIntervalType`.
### Does this PR introduce _any_ user-facing change?
'No'.
Should not since new types have not been released yet.
### How was this patch tested?
Jenkins test
Closes#32229 from beliefer/SPARK-34837.
Authored-by: gengjiaan <gengjiaan@360.cn>
Signed-off-by: Max Gekk <max.gekk@gmail.com>
### What changes were proposed in this pull request?
This PR makes the input buffer configurable (as an internal configuration). This is mainly to work around the regression in uniVocity/univocity-parsers#449.
This is particularly useful for SQL workloads that requires to rewrite the `CREATE TABLE` with options.
### Why are the changes needed?
To work around uniVocity/univocity-parsers#449.
### Does this PR introduce _any_ user-facing change?
No, it's only internal option.
### How was this patch tested?
Manually tested by modifying the unittest added in https://github.com/apache/spark/pull/31858 as below:
```diff
diff --git a/sql/core/src/test/scala/org/apache/spark/sql/execution/datasources/csv/CSVSuite.scala b/sql/core/src/test/scala/org/apache/spark/sql/execution/datasources/csv/CSVSuite.scala
index fd25a79619d..705f38dbfbd 100644
--- a/sql/core/src/test/scala/org/apache/spark/sql/execution/datasources/csv/CSVSuite.scala
+++ b/sql/core/src/test/scala/org/apache/spark/sql/execution/datasources/csv/CSVSuite.scala
-2456,6 +2456,7 abstract class CSVSuite
test("SPARK-34768: counting a long record with ignoreTrailingWhiteSpace set to true") {
val bufSize = 128
val line = "X" * (bufSize - 1) + "| |"
+ spark.conf.set("spark.sql.csv.parser.inputBufferSize", 128)
withTempPath { path =>
Seq(line).toDF.write.text(path.getAbsolutePath)
assert(spark.read.format("csv")
```
Closes#32231 from HyukjinKwon/SPARK-35045-followup.
Authored-by: HyukjinKwon <gurwls223@apache.org>
Signed-off-by: HyukjinKwon <gurwls223@apache.org>
### What changes were proposed in this pull request?
Now that `AnalysisOnlyCommand` in introduced in #32032, `CacheTable` and `UncacheTable` can extend `AnalysisOnlyCommand` to simplify the code base. For example, the logic to handle these commands such that the tables are only analyzed is scattered across different places.
### Why are the changes needed?
To simplify the code base to handle these two commands.
### Does this PR introduce _any_ user-facing change?
No, just internal refactoring.
### How was this patch tested?
The existing tests (e.g., `CachedTableSuite`) cover the changes in this PR. For example, if I make `CacheTable`/`UncacheTable` extend `LeafCommand`, there are few failures in `CachedTableSuite`.
Closes#32220 from imback82/cache_cmd_analysis_only.
Authored-by: Terry Kim <yuminkim@gmail.com>
Signed-off-by: Wenchen Fan <wenchen@databricks.com>
### What changes were proposed in this pull request?
This PR:
- Adds a new expression `GroupingExprRef` that can be used in aggregate expressions of `Aggregate` nodes to refer grouping expressions by index. These expressions capture the data type and nullability of the referred grouping expression.
- Adds a new rule `EnforceGroupingReferencesInAggregates` that inserts the references in the beginning of the optimization phase.
- Adds a new rule `UpdateGroupingExprRefNullability` to update nullability of `GroupingExprRef` expressions as nullability of referred grouping expression can change during optimization.
### Why are the changes needed?
If aggregate expressions (without aggregate functions) in an `Aggregate` node are complex then the `Optimizer` can optimize out grouping expressions from them and so making aggregate expressions invalid.
Here is a simple example:
```
SELECT not(t.id IS NULL) , count(*)
FROM t
GROUP BY t.id IS NULL
```
In this case the `BooleanSimplification` rule does this:
```
=== Applying Rule org.apache.spark.sql.catalyst.optimizer.BooleanSimplification ===
!Aggregate [isnull(id#222)], [NOT isnull(id#222) AS (NOT (id IS NULL))#226, count(1) AS c#224L] Aggregate [isnull(id#222)], [isnotnull(id#222) AS (NOT (id IS NULL))#226, count(1) AS c#224L]
+- Project [value#219 AS id#222] +- Project [value#219 AS id#222]
+- LocalRelation [value#219] +- LocalRelation [value#219]
```
where `NOT isnull(id#222)` is optimized to `isnotnull(id#222)` and so it no longer refers to any grouping expression.
Before this PR:
```
== Optimized Logical Plan ==
Aggregate [isnull(id#222)], [isnotnull(id#222) AS (NOT (id IS NULL))#234, count(1) AS c#232L]
+- Project [value#219 AS id#222]
+- LocalRelation [value#219]
```
and running the query throws an error:
```
Couldn't find id#222 in [isnull(id#222)#230,count(1)#226L]
java.lang.IllegalStateException: Couldn't find id#222 in [isnull(id#222)#230,count(1)#226L]
```
After this PR:
```
== Optimized Logical Plan ==
Aggregate [isnull(id#222)], [NOT groupingexprref(0) AS (NOT (id IS NULL))#234, count(1) AS c#232L]
+- Project [value#219 AS id#222]
+- LocalRelation [value#219]
```
and the query works.
### Does this PR introduce _any_ user-facing change?
Yes, the query works.
### How was this patch tested?
Added new UT.
Closes#31913 from peter-toth/SPARK-34581-keep-grouping-expressions.
Authored-by: Peter Toth <peter.toth@gmail.com>
Signed-off-by: Wenchen Fan <wenchen@databricks.com>
### What changes were proposed in this pull request?
It seems that we miss classifying one `SparkOutOfMemoryError` in `HashedRelation`. Add the error classification for it. In addition, clean up two errors definition of `HashJoin` as they are not used.
### Why are the changes needed?
Better error classification.
### Does this PR introduce _any_ user-facing change?
No.
### How was this patch tested?
Existing tests.
Closes#32211 from c21/error-message.
Authored-by: Cheng Su <chengsu@fb.com>
Signed-off-by: Takeshi Yamamuro <yamamuro@apache.org>
### What changes were proposed in this pull request?
Add checks for `YearMonthIntervalType` and `DayTimeIntervalType` to `MutableProjectionSuite`.
### Why are the changes needed?
To improve test coverage, and the same checks as for `CalendarIntervalType`.
### Does this PR introduce _any_ user-facing change?
No
### How was this patch tested?
By running the modified test suite:
```
$ build/sbt "test:testOnly *MutableProjectionSuite"
```
Closes#32225 from MaxGekk/test-ansi-intervals-in-MutableProjectionSuite.
Authored-by: Max Gekk <max.gekk@gmail.com>
Signed-off-by: Takeshi Yamamuro <yamamuro@apache.org>
### What changes were proposed in this pull request?
Extend the `Sum` expression to to support `DayTimeIntervalType` and `YearMonthIntervalType` added by #31614.
Note: the expressions can throw the overflow exception independently from the SQL config `spark.sql.ansi.enabled`. In this way, the modified expressions always behave in the ANSI mode for the intervals.
### Why are the changes needed?
Extend `org.apache.spark.sql.catalyst.expressions.aggregate.Sum` to support `DayTimeIntervalType` and `YearMonthIntervalType`.
### Does this PR introduce _any_ user-facing change?
'No'.
Should not since new types have not been released yet.
### How was this patch tested?
Jenkins test
Closes#32107 from beliefer/SPARK-34716.
Lead-authored-by: gengjiaan <gengjiaan@360.cn>
Co-authored-by: beliefer <beliefer@163.com>
Co-authored-by: Hyukjin Kwon <gurwls223@gmail.com>
Signed-off-by: Max Gekk <max.gekk@gmail.com>
### What changes were proposed in this pull request?
In the PR, I propose to add additional checks for ANSI interval types `YearMonthIntervalType` and `DayTimeIntervalType` to `LiteralExpressionSuite`.
Also, I replaced some long literal values by `CalendarInterval` to check `CalendarIntervalType` that the tests were supposed to check.
### Why are the changes needed?
To improve test coverage and have the same checks for ANSI types as for `CalendarIntervalType`.
### Does this PR introduce _any_ user-facing change?
No
### How was this patch tested?
By running the modified test suite:
```
$ build/sbt "test:testOnly *LiteralExpressionSuite"
```
Closes#32213 from MaxGekk/interval-literal-tests.
Authored-by: Max Gekk <max.gekk@gmail.com>
Signed-off-by: Max Gekk <max.gekk@gmail.com>
### What changes were proposed in this pull request?
The precision of `java.time.Duration` is nanosecond, but when it is used as `DayTimeIntervalType` in Spark, it is microsecond.
At present, the `DayTimeIntervalType` data generated in the implementation of `RandomDataGenerator` is accurate to nanosecond, which will cause the `DayTimeIntervalType` to be converted to long, and then back to `DayTimeIntervalType` to lose the accuracy, which will cause the test to fail. For example: https://amplab.cs.berkeley.edu/jenkins/job/SparkPullRequestBuilder/137390/testReport/org.apache.spark.sql.hive.execution/HashAggregationQueryWithControlledFallbackSuite/udaf_with_all_data_types/
### Why are the changes needed?
Improve `RandomDataGenerator` so that the generated data fits the precision of DayTimeIntervalType in spark.
### Does this PR introduce _any_ user-facing change?
'No'. Just change the test class.
### How was this patch tested?
Jenkins test.
Closes#32212 from beliefer/SPARK-35116.
Authored-by: beliefer <beliefer@163.com>
Signed-off-by: Max Gekk <max.gekk@gmail.com>
### What changes were proposed in this pull request?
This issue fixes an issue that indentation of multiple output JSON records in a single split file are broken except for the first record in the split when `pretty` option is `true`.
```
// Run in the Spark Shell.
// Set spark.sql.leafNodeDefaultParallelism to 1 for the current master.
// Or set spark.default.parallelism for the previous releases.
spark.conf.set("spark.sql.leafNodeDefaultParallelism", 1)
val df = Seq("a", "b", "c").toDF
df.write.option("pretty", "true").json("/path/to/output")
# Run in a Shell
$ cat /path/to/output/*.json
{
"value" : "a"
}
{
"value" : "b"
}
{
"value" : "c"
}
```
### Why are the changes needed?
It's not pretty even though `pretty` option is true.
### Does this PR introduce _any_ user-facing change?
I think "No". Indentation style is changed but JSON format is not changed.
### How was this patch tested?
New test.
Closes#32203 from sarutak/fix-ugly-indentation.
Authored-by: Kousuke Saruta <sarutak@oss.nttdata.com>
Signed-off-by: Max Gekk <max.gekk@gmail.com>
### What changes were proposed in this pull request?
Handle `YearMonthIntervalType` and `DayTimeIntervalType` in the `sql()` and `toString()` method of `Literal`, and format the ANSI interval in the ANSI style.
### Why are the changes needed?
To improve readability and UX with Spark SQL. For example, a test output before the changes:
```
-- !query
select timestamp'2011-11-11 11:11:11' - interval '2' day
-- !query schema
struct<TIMESTAMP '2011-11-11 11:11:11' - 172800000000:timestamp>
-- !query output
2011-11-09 11:11:11
```
### Does this PR introduce _any_ user-facing change?
Should not since the new intervals haven't been released yet.
### How was this patch tested?
By running new tests:
```
$ ./build/sbt "test:testOnly *LiteralExpressionSuite"
```
Closes#32196 from MaxGekk/literal-ansi-interval-sql.
Authored-by: Max Gekk <max.gekk@gmail.com>
Signed-off-by: Max Gekk <max.gekk@gmail.com>
### What changes were proposed in this pull request?
Normal function parameters should not support alias, hive not support too
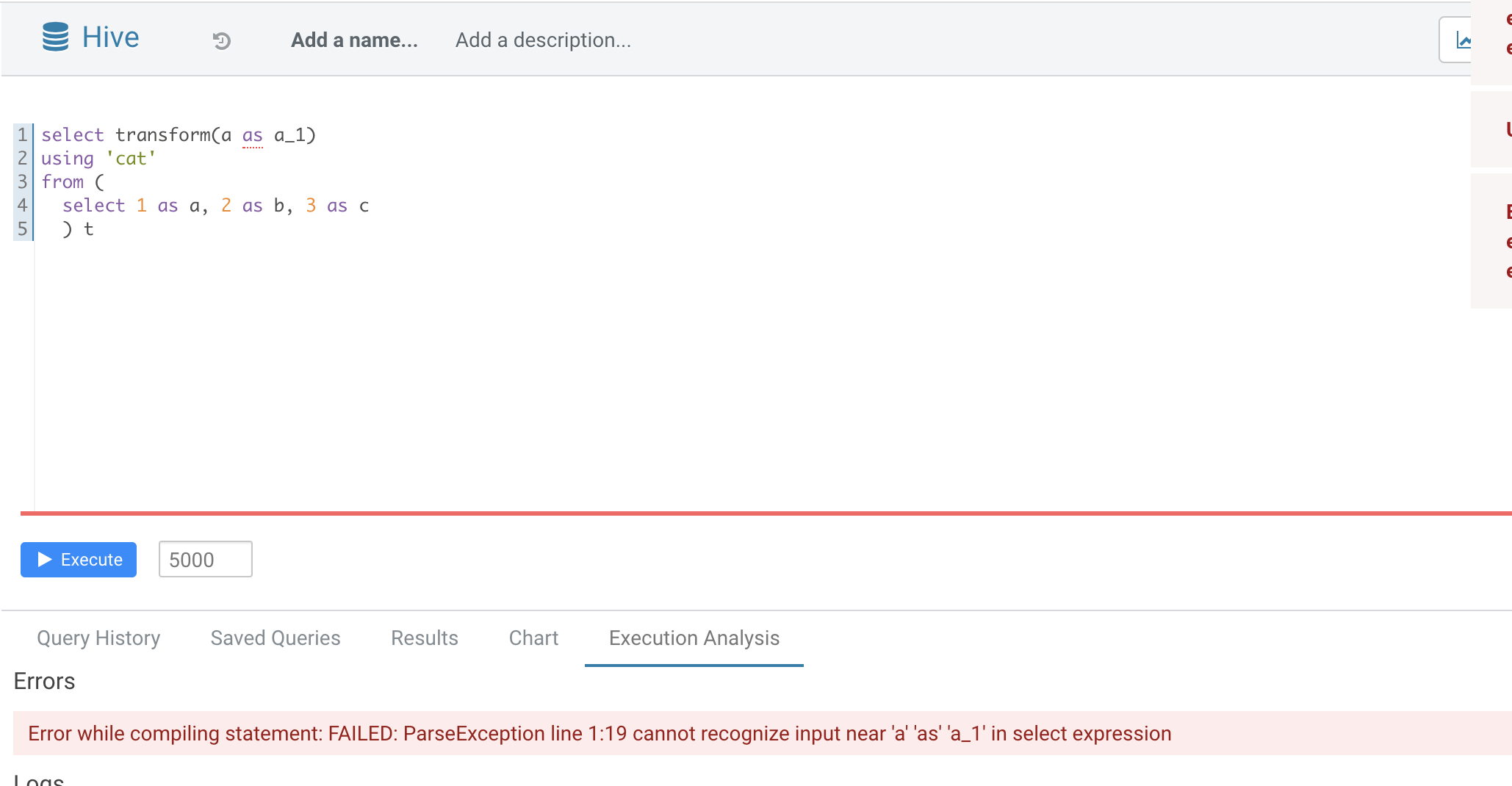
In this pr we forbid use alias in `TRANSFORM`'s inputs
### Why are the changes needed?
Fix bug
### Does this PR introduce _any_ user-facing change?
No
### How was this patch tested?
Added UT
Closes#32165 from AngersZhuuuu/SPARK-35070.
Authored-by: Angerszhuuuu <angers.zhu@gmail.com>
Signed-off-by: Wenchen Fan <wenchen@databricks.com>
### What changes were proposed in this pull request?
Support `date +/- day-time interval`. In the PR, I propose to update the binary arithmetic rules, and cast an input date to a timestamp at the session time zone, and then add a day-time interval to it.
### Why are the changes needed?
1. To conform the ANSI SQL standard which requires to support such operation over dates and intervals:
<img width="811" alt="Screenshot 2021-03-12 at 11 36 14" src="https://user-images.githubusercontent.com/1580697/111081674-865d4900-8515-11eb-86c8-3538ecaf4804.png">
2. To fix the regression comparing to the recent Spark release 3.1 with default settings.
Before the changes:
```sql
spark-sql> select date'now' + (timestamp'now' - timestamp'yesterday');
Error in query: cannot resolve 'DATE '2021-04-14' + subtracttimestamps(TIMESTAMP '2021-04-14 18:14:56.497', TIMESTAMP '2021-04-13 00:00:00')' due to data type mismatch: argument 1 requires timestamp type, however, 'DATE '2021-04-14'' is of date type.; line 1 pos 7;
'Project [unresolvedalias(cast(2021-04-14 + subtracttimestamps(2021-04-14 18:14:56.497, 2021-04-13 00:00:00, false, Some(Europe/Moscow)) as date), None)]
+- OneRowRelation
```
Spark 3.1:
```sql
spark-sql> select date'now' + (timestamp'now' - timestamp'yesterday');
2021-04-15
```
Hive:
```sql
0: jdbc:hive2://localhost:10000/default> select date'2021-04-14' + (timestamp'2020-04-14 18:15:30' - timestamp'2020-04-13 00:00:00');
+------------------------+
| _c0 |
+------------------------+
| 2021-04-15 18:15:30.0 |
+------------------------+
```
### Does this PR introduce _any_ user-facing change?
Should not since new intervals have not been released yet.
After the changes:
```sql
spark-sql> select date'now' + (timestamp'now' - timestamp'yesterday');
2021-04-15 18:13:16.555
```
### How was this patch tested?
By running new tests:
```
$ build/sbt "test:testOnly *ColumnExpressionSuite"
```
Closes#32170 from MaxGekk/date-add-day-time-interval.
Authored-by: Max Gekk <max.gekk@gmail.com>
Signed-off-by: Max Gekk <max.gekk@gmail.com>
### What changes were proposed in this pull request?
According to https://github.com/apache/spark/pull/29087#discussion_r612267050, add UT in `transform.sql`
It seems that distinct is not recognized as a reserved word here
```
-- !query
explain extended SELECT TRANSFORM(distinct b, a, c)
USING 'cat' AS (a, b, c)
FROM script_trans
WHERE a <= 4
-- !query schema
struct<plan:string>
-- !query output
== Parsed Logical Plan ==
'ScriptTransformation [*], cat, [a#x, b#x, c#x], ScriptInputOutputSchema(List(),List(),None,None,List(),List(),None,None,false)
+- 'Project ['distinct AS b#x, 'a, 'c]
+- 'Filter ('a <= 4)
+- 'UnresolvedRelation [script_trans], [], false
== Analyzed Logical Plan ==
org.apache.spark.sql.AnalysisException: cannot resolve 'distinct' given input columns: [script_trans.a, script_trans.b, script_trans.c]; line 1 pos 34;
'ScriptTransformation [*], cat, [a#x, b#x, c#x], ScriptInputOutputSchema(List(),List(),None,None,List(),List(),None,None,false)
+- 'Project ['distinct AS b#x, a#x, c#x]
+- Filter (a#x <= 4)
+- SubqueryAlias script_trans
+- View (`script_trans`, [a#x,b#x,c#x])
+- Project [cast(a#x as int) AS a#x, cast(b#x as int) AS b#x, cast(c#x as int) AS c#x]
+- Project [a#x, b#x, c#x]
+- SubqueryAlias script_trans
+- LocalRelation [a#x, b#x, c#x]
```
Hive's error
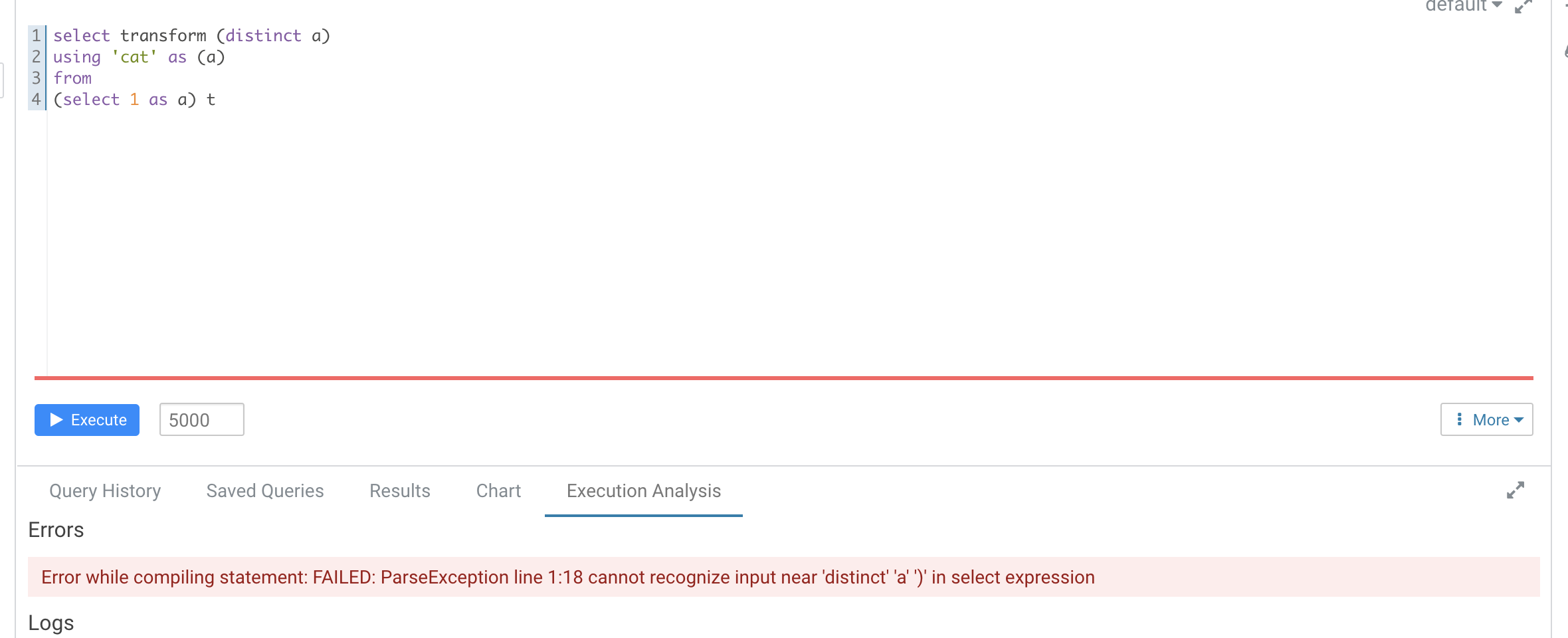
### Why are the changes needed?
### Does this PR introduce _any_ user-facing change?
No
### How was this patch tested?
Added Ut
Closes#32149 from AngersZhuuuu/SPARK-28227-new-followup.
Authored-by: Angerszhuuuu <angers.zhu@gmail.com>
Signed-off-by: Wenchen Fan <wenchen@databricks.com>
### What changes were proposed in this pull request?
This PR proposes to introduce the `AnalysisOnlyCommand` trait such that a command that extends this trait can have its children only analyzed, but not optimized. There is a corresponding analysis rule `HandleAnalysisOnlyCommand` that marks the command as analyzed after all other analysis rules are run.
This can be useful if a logical plan has children where they need to be only analyzed, but not optimized - e.g., `CREATE VIEW` or `CACHE TABLE AS`. This also addresses the issue found in #31933.
This PR also updates `CreateViewCommand`, `CacheTableAsSelect`, and `AlterViewAsCommand` to use the new trait / rule such that their children are only analyzed.
### Why are the changes needed?
To address the issue where the plan is unnecessarily re-analyzed in `CreateViewCommand`.
### Does this PR introduce _any_ user-facing change?
No.
### How was this patch tested?
Existing tests should cover the changes.
Closes#32032 from imback82/skip_transform.
Authored-by: Terry Kim <yuminkim@gmail.com>
Signed-off-by: Wenchen Fan <wenchen@databricks.com>
### What changes were proposed in this pull request?
Adds the duplicated common columns as hidden columns to the Projection used to rewrite NATURAL/USING JOINs.
### Why are the changes needed?
Allows users to resolve either side of the NATURAL/USING JOIN's common keys.
Previously, the user could only resolve the following columns:
| Join type | Left key columns | Right key columns |
| --- | --- | --- |
| Inner | Yes | No |
| Left | Yes | No |
| Right | No | Yes |
| Outer | No | No |
### Does this PR introduce _any_ user-facing change?
Yes. The user can now symmetrically resolve the common columns from a NATURAL/USING JOIN.
### How was this patch tested?
SQL-side tests. The behavior matches PostgreSQL and MySQL.
Closes#31666 from karenfeng/spark-34527.
Authored-by: Karen Feng <karen.feng@databricks.com>
Signed-off-by: Wenchen Fan <wenchen@databricks.com>
### What changes were proposed in this pull request?
This PR fixes an issue that `LIST FILES/JARS/ARCHIVES path1 path2 ...` cannot list all paths if at least one path is quoted.
An example here.
```
ADD FILE /tmp/test1;
ADD FILE /tmp/test2;
LIST FILES /tmp/test1 /tmp/test2;
file:/tmp/test1
file:/tmp/test2
LIST FILES /tmp/test1 "/tmp/test2";
file:/tmp/test2
```
In this example, the second `LIST FILES` doesn't show `file:/tmp/test1`.
To resolve this issue, I modified the syntax rule to be able to handle this case.
I also changed `SparkSQLParser` to be able to handle paths which contains white spaces.
### Why are the changes needed?
This is a bug.
I also have a plan which extends `ADD FILE/JAR/ARCHIVE` to take multiple paths like Hive and the syntax rule change is necessary for that.
### Does this PR introduce _any_ user-facing change?
Yes. Users can pass quoted paths when using `ADD FILE/JAR/ARCHIVE`.
### How was this patch tested?
New test.
Closes#32074 from sarutak/fix-list-files-bug.
Authored-by: Kousuke Saruta <sarutak@oss.nttdata.com>
Signed-off-by: Kousuke Saruta <sarutak@oss.nttdata.com>
### What changes were proposed in this pull request?
This PR group exception messages in `/core/src/main/scala/org/apache/spark/sql/execution`.
### Why are the changes needed?
It will largely help with standardization of error messages and its maintenance.
### Does this PR introduce _any_ user-facing change?
No. Error messages remain unchanged.
### How was this patch tested?
No new tests - pass all original tests to make sure it doesn't break any existing behavior.
Closes#31920 from beliefer/SPARK-33604.
Lead-authored-by: gengjiaan <gengjiaan@360.cn>
Co-authored-by: Jiaan Geng <beliefer@163.com>
Signed-off-by: Wenchen Fan <wenchen@databricks.com>
### What changes were proposed in this pull request?
Populate table catalog and identifier from `DataStreamWriter` to `WriteToMicroBatchDataSource` so that we can invalidate cache for tables that are updated by a streaming write.
This is somewhat related [SPARK-27484](https://issues.apache.org/jira/browse/SPARK-27484) and [SPARK-34183](https://issues.apache.org/jira/browse/SPARK-34183) (#31700), as ideally we may want to replace `WriteToMicroBatchDataSource` and `WriteToDataSourceV2` with logical write nodes and feed them to analyzer. That will potentially change the code path involved in this PR.
### Why are the changes needed?
Currently `WriteToDataSourceV2` doesn't have cache invalidation logic, and therefore, when the target table for a micro batch streaming job is cached, the cache entry won't be removed when the table is updated.
### Does this PR introduce _any_ user-facing change?
Yes now when a DSv2 table which supports streaming write is updated by a streaming job, its cache will also be invalidated.
### How was this patch tested?
Added a new UT.
Closes#32039 from sunchao/streaming-cache.
Authored-by: Chao Sun <sunchao@apple.com>
Signed-off-by: Wenchen Fan <wenchen@databricks.com>
### What changes were proposed in this pull request?
Remove duplicate code in `TreeNode.treePatternBits`
### Why are the changes needed?
Code clean up. Make it easier for maintainence.
### Does this PR introduce _any_ user-facing change?
No
### How was this patch tested?
Existing tests.
Closes#32143 from gengliangwang/getBits.
Authored-by: Gengliang Wang <ltnwgl@gmail.com>
Signed-off-by: Gengliang Wang <ltnwgl@gmail.com>
### What changes were proposed in this pull request?
This PR makes the input buffer configurable (as an internal option). This is mainly to work around uniVocity/univocity-parsers#449.
### Why are the changes needed?
To work around uniVocity/univocity-parsers#449.
### Does this PR introduce _any_ user-facing change?
No, it's only internal option.
### How was this patch tested?
Manually tested by modifying the unittest added in https://github.com/apache/spark/pull/31858 as below:
```diff
diff --git a/sql/core/src/test/scala/org/apache/spark/sql/execution/datasources/csv/CSVSuite.scala b/sql/core/src/test/scala/org/apache/spark/sql/execution/datasources/csv/CSVSuite.scala
index fd25a79619d..b58f0bd3661 100644
--- a/sql/core/src/test/scala/org/apache/spark/sql/execution/datasources/csv/CSVSuite.scala
+++ b/sql/core/src/test/scala/org/apache/spark/sql/execution/datasources/csv/CSVSuite.scala
-2460,6 +2460,7 abstract class CSVSuite
Seq(line).toDF.write.text(path.getAbsolutePath)
assert(spark.read.format("csv")
.option("delimiter", "|")
+ .option("inputBufferSize", "128")
.option("ignoreTrailingWhiteSpace", "true").load(path.getAbsolutePath).count() == 1)
}
}
```
Closes#32145 from HyukjinKwon/SPARK-35045.
Lead-authored-by: Hyukjin Kwon <gurwls223@apache.org>
Co-authored-by: HyukjinKwon <gurwls223@apache.org>
Signed-off-by: Max Gekk <max.gekk@gmail.com>
### What changes were proposed in this pull request?
Fix PhysicalAggregation to not transform a foldable expression.
### Why are the changes needed?
It can potentially break certain queries like the added unit test shows.
### Does this PR introduce _any_ user-facing change?
Yes, it fixes undesirable errors caused by a returned TypeCheckFailure from places like RegExpReplace.checkInputDataTypes.
Closes#32113 from sigmod/foldable.
Authored-by: Yingyi Bu <yingyi.bu@databricks.com>
Signed-off-by: Wenchen Fan <wenchen@databricks.com>
### What changes were proposed in this pull request?
This PR contains:
- AnalysisHelper changes to allow the resolve function family to stop earlier without traversing the entire tree;
- Example changes in a few rules to support such pruning, e.g., ResolveRandomSeed, ResolveWindowFrame, ResolveWindowOrder, and ResolveNaturalAndUsingJoin.
### Why are the changes needed?
It's a framework-level change for reducing the query compilation time.
In particular, if we update existing analysis rules' call sites as per the examples in this PR, the analysis time can be reduced as described in the [doc](https://docs.google.com/document/d/1SEUhkbo8X-0cYAJFYFDQhxUnKJBz4lLn3u4xR2qfWqk).
### Does this PR introduce any user-facing change?
No.
### How was this patch tested?
It is tested by existing tests.
Closes#32135 from sigmod/resolver.
Authored-by: Yingyi Bu <yingyi.bu@databricks.com>
Signed-off-by: Gengliang Wang <ltnwgl@gmail.com>
### What changes were proposed in this pull request?
This PR allows non-aggregated correlated scalar subquery if the max output row is less than 2. Correlated scalar subqueries need to be aggregated because they are going to be decorrelated and rewritten as LEFT OUTER joins. If the correlated scalar subquery produces more than one output row, the rewrite will yield wrong results.
But this constraint can be relaxed when the subquery plan's the max number of output rows is less than or equal to 1.
### Why are the changes needed?
To relax a constraint in CheckAnalysis for the correlated scalar subquery.
### Does this PR introduce _any_ user-facing change?
Yes
### How was this patch tested?
Unit tests
Closes#32111 from allisonwang-db/spark-28379-aggregated.
Authored-by: allisonwang-db <66282705+allisonwang-db@users.noreply.github.com>
Signed-off-by: Wenchen Fan <wenchen@databricks.com>
### What changes were proposed in this pull request?
Supports cardinality estimation of union, sort and range operator.
1. **Union**: number of rows in output will be the sum of number of rows in the output for each child of union, min and max for each column in the output will be the min and max of that particular column coming from its children.
Example:
Table 1
a b
1 6
2 3
Table 2
a b
1 3
4 1
stats for table1 union table2 would be number of rows = 4, columnStats = (a: {min: 1, max: 4}, b: {min: 1, max: 6})
2. **Sort**: row and columns stats would be same as its children.
3. **Range**: number of output rows and distinct count will be equal to number of elements, min and max is calculated from start, end and step param.
### Why are the changes needed?
The change will enhance the feature https://issues.apache.org/jira/browse/SPARK-16026 and will help in other stats based optimizations.
### Does this PR introduce _any_ user-facing change?
No
### How was this patch tested?
New unit tests added.
Closes#30334 from ayushi-agarwal/SPARK-33411.
Lead-authored-by: ayushi agarwal <ayaga@microsoft.com>
Co-authored-by: ayushi-agarwal <36420535+ayushi-agarwal@users.noreply.github.com>
Signed-off-by: Takeshi Yamamuro <yamamuro@apache.org>
### What changes were proposed in this pull request?
1. Extend SQL syntax rules to support a sign before the interval strings of ANSI year-month and day-time intervals.
2. Recognize `-` in `AstBuilder` and negate parsed intervals.
### Why are the changes needed?
To conform to the SQL standard which allows a sign before the string interval, see `"5.3 <literal>"`:
```
<interval literal> ::=
INTERVAL [ <sign> ] <interval string> <interval qualifier>
<interval string> ::=
<quote> <unquoted interval string> <quote>
<unquoted interval string> ::=
[ <sign> ] { <year-month literal> | <day-time literal> }
<sign> ::=
<plus sign>
| <minus sign>
```
### Does this PR introduce _any_ user-facing change?
Should not because it just extends supported intervals syntax.
### How was this patch tested?
By running new tests in `interval.sql`:
```
$ build/sbt "sql/testOnly *SQLQueryTestSuite -- -z interval.sql"
```
Closes#32134 from MaxGekk/negative-parsed-intervals.
Authored-by: Max Gekk <max.gekk@gmail.com>
Signed-off-by: Max Gekk <max.gekk@gmail.com>
### What changes were proposed in this pull request?
Disallow group by aliases under ANSI mode.
### Why are the changes needed?
As per the ANSI SQL standard secion 7.12 <group by clause>:
>Each `grouping column reference` shall unambiguously reference a column of the table resulting from the `from clause`. A column referenced in a `group by clause` is a grouping column.
By forbidding it, we can avoid ambiguous SQL queries like:
```
SELECT col + 1 as col FROM t GROUP BY col
```
### Does this PR introduce _any_ user-facing change?
Yes, group by aliases is not allowed under ANSI mode.
### How was this patch tested?
Unit tests
Closes#32129 from gengliangwang/disallowGroupByAlias.
Authored-by: Gengliang Wang <ltnwgl@gmail.com>
Signed-off-by: Gengliang Wang <ltnwgl@gmail.com>
### What changes were proposed in this pull request?
For Spark SQL, it can't support script transform SQL with aggregationClause/windowClause/LateralView.
This case we can't directly migration Hive SQL to Spark SQL.
In this PR, we treat all script transform statement's query part (exclude transform about part) as a separate query block and solve it as ScriptTransformation's child and pass a UnresolvedStart as ScriptTransform's input. Then in analyzer level, we pass child's output as ScriptTransform's input. Then we can support all kind of normal SELECT query combine with script transformation.
Such as transform with aggregation:
```
SELECT TRANSFORM ( d2, max(d1) as max_d1, sum(d3))
USING 'cat' AS (a,b,c)
FROM script_trans
WHERE d1 <= 100
GROUP BY d2
HAVING max_d1 > 0
```
When we build AST, we treat it as
```
SELECT TRANSFORM (*)
USING 'cat' AS (a,b,c)
FROM (
SELECT d2, max(d1) as max_d1, sum(d3)
FROM script_trans
WHERE d1 <= 100
GROUP BY d2
HAVING max_d1 > 0
) tmp
```
then in Analyzer's `ResolveReferences`, resolve `* (UnresolvedStar)`, then sql behavior like
```
SELECT TRANSFORM ( d2, max(d1) as max_d1, sum(d3))
USING 'cat' AS (a,b,c)
FROM script_trans
WHERE d1 <= 100
GROUP BY d2
HAVING max_d1 > 0
```
About UT, in this pr we add a lot of different SQL to check we can support all kind of such SQL and each kind of expressions can work well, such as alias, case when, binary compute etc...
### Why are the changes needed?
Support transform with aggregateClause/windowClause/LateralView etc , make sql migration more smoothly
### Does this PR introduce _any_ user-facing change?
User can write transform with aggregateClause/windowClause/LateralView.
### How was this patch tested?
Added UT
Closes#29087 from AngersZhuuuu/SPARK-28227-NEW.
Lead-authored-by: angerszhu <angers.zhu@gmail.com>
Co-authored-by: Angerszhuuuu <angers.zhu@gmail.com>
Co-authored-by: AngersZhuuuu <angers.zhu@gmail.com>
Signed-off-by: Takeshi Yamamuro <yamamuro@apache.org>
### What changes were proposed in this pull request?
Support GROUP BY use Separate columns and CUBE/ROLLUP
In postgres sql, it support
```
select a, b, c, count(1) from t group by a, b, cube (a, b, c);
select a, b, c, count(1) from t group by a, b, rollup(a, b, c);
select a, b, c, count(1) from t group by cube(a, b), rollup (a, b, c);
select a, b, c, count(1) from t group by a, b, grouping sets((a, b), (a), ());
```
In this pr, we have done two things as below:
1. Support partial grouping analytics such as `group by a, cube(a, b)`
2. Support mixed grouping analytics such as `group by cube(a, b), rollup(b,c)`
*Partial Groupings*
Partial Groupings means there are both `group_expression` and `CUBE|ROLLUP|GROUPING SETS`
in GROUP BY clause. For example:
`GROUP BY warehouse, CUBE(product, location)` is equivalent to
`GROUP BY GROUPING SETS((warehouse, product, location), (warehouse, product), (warehouse, location), (warehouse))`.
`GROUP BY warehouse, ROLLUP(product, location)` is equivalent to
`GROUP BY GROUPING SETS((warehouse, product, location), (warehouse, product), (warehouse))`.
`GROUP BY warehouse, GROUPING SETS((product, location), (producet), ())` is equivalent to
`GROUP BY GROUPING SETS((warehouse, product, location), (warehouse, location), (warehouse))`.
*Concatenated Groupings*
Concatenated groupings offer a concise way to generate useful combinations of groupings. Groupings specified
with concatenated groupings yield the cross-product of groupings from each grouping set. The cross-product
operation enables even a small number of concatenated groupings to generate a large number of final groups.
The concatenated groupings are specified simply by listing multiple `GROUPING SETS`, `CUBES`, and `ROLLUP`,
and separating them with commas. For example:
`GROUP BY GROUPING SETS((warehouse), (producet)), GROUPING SETS((location), (size))` is equivalent to
`GROUP BY GROUPING SETS((warehouse, location), (warehouse, size), (product, location), (product, size))`.
`GROUP BY CUBE((warehouse), (producet)), ROLLUP((location), (size))` is equivalent to
`GROUP BY GROUPING SETS((warehouse, product), (warehouse), (producet), ()), GROUPING SETS((location, size), (location), ())`
`GROUP BY GROUPING SETS(
(warehouse, product, location, size), (warehouse, product, location), (warehouse, product),
(warehouse, location, size), (warehouse, location), (warehouse),
(product, location, size), (product, location), (product),
(location, size), (location), ())`.
`GROUP BY order, CUBE((warehouse), (producet)), ROLLUP((location), (size))` is equivalent to
`GROUP BY order, GROUPING SETS((warehouse, product), (warehouse), (producet), ()), GROUPING SETS((location, size), (location), ())`
`GROUP BY GROUPING SETS(
(order, warehouse, product, location, size), (order, warehouse, product, location), (order, warehouse, product),
(order, warehouse, location, size), (order, warehouse, location), (order, warehouse),
(order, product, location, size), (order, product, location), (order, product),
(order, location, size), (order, location), (order))`.
### Why are the changes needed?
Support more flexible grouping analytics
### Does this PR introduce _any_ user-facing change?
User can use sql like
```
select a, b, c, agg_expr() from table group by a, cube(b, c)
```
### How was this patch tested?
Added UT
Closes#30144 from AngersZhuuuu/SPARK-33229.
Lead-authored-by: Angerszhuuuu <angers.zhu@gmail.com>
Co-authored-by: angerszhu <angers.zhu@gmail.com>
Co-authored-by: Wenchen Fan <cloud0fan@gmail.com>
Signed-off-by: Wenchen Fan <wenchen@databricks.com>
### What changes were proposed in this pull request?
This PR contains:
- TreeNode, QueryPlan, AnalysisHelper changes to allow the transform function family to stop earlier without traversing the entire tree;
- Example changes in a few rules to support such pruning, e.g., ReorderJoin and OptimizeIn.
Here is a [design doc](https://docs.google.com/document/d/1SEUhkbo8X-0cYAJFYFDQhxUnKJBz4lLn3u4xR2qfWqk) that elaborates the ideas and benchmark numbers.
### Why are the changes needed?
It's a framework-level change for reducing the query compilation time.
In particular, if we update existing rules and transform call sites as per the examples in this PR, the analysis time and query optimization time can be reduced as described in this [doc](https://docs.google.com/document/d/1SEUhkbo8X-0cYAJFYFDQhxUnKJBz4lLn3u4xR2qfWqk) .
### Does this PR introduce _any_ user-facing change?
No.
### How was this patch tested?
It is tested by existing tests.
Closes#32060 from sigmod/bits.
Authored-by: Yingyi Bu <yingyi.bu@databricks.com>
Signed-off-by: Gengliang Wang <ltnwgl@gmail.com>
### What changes were proposed in this pull request?
before when we use aggregate ordinal in group by expression and index position is a aggregate function, it will show error as
```
– !query
select a, b, sum(b) from data group by 3
– !query schema
struct<>
– !query output
org.apache.spark.sql.AnalysisException
aggregate functions are not allowed in GROUP BY, but found sum(data.b)
```
It't not clear enough refactor this error message in this pr
### Why are the changes needed?
refactor error message
### Does this PR introduce _any_ user-facing change?
No
### How was this patch tested?
Existed UT
Closes#32089 from AngersZhuuuu/SPARK-34986.
Lead-authored-by: Angerszhuuuu <angers.zhu@gmail.com>
Co-authored-by: AngersZhuuuu <angers.zhu@gmail.com>
Signed-off-by: HyukjinKwon <gurwls223@apache.org>
### What changes were proposed in this pull request?
1. Extend `IntervalUtils` methods: `toYearMonthIntervalString` and `toDayTimeIntervalString` to support formatting of year-month/day-time intervals in Hive style. The methods get new parameter style which can have to values; `HIVE_STYLE` and `ANSI_STYLE`.
2. Invoke `toYearMonthIntervalString` and `toDayTimeIntervalString` from the `Cast` expression with the `style` parameter is set to `ANSI_STYLE`.
3. Invoke `toYearMonthIntervalString` and `toDayTimeIntervalString` from `HiveResult` with `style` is set to `HIVE_STYLE`.
### Why are the changes needed?
The `spark-sql` shell formats its output in Hive style by using `HiveResult.hiveResultString()`. The changes are needed to match Hive behavior. For instance,
Hive:
```sql
0: jdbc:hive2://localhost:10000/default> select timestamp'2021-01-01 01:02:03.000001' - date'2020-12-31';
+-----------------------+
| _c0 |
+-----------------------+
| 1 01:02:03.000001000 |
+-----------------------+
```
Spark before the changes:
```sql
spark-sql> select timestamp'2021-01-01 01:02:03.000001' - date'2020-12-31';
INTERVAL '1 01:02:03.000001' DAY TO SECOND
```
Also this should unblock #32099 which enables *.sql tests in `SQLQueryTestSuite`.
### Does this PR introduce _any_ user-facing change?
Yes. After the changes:
```sql
spark-sql> select timestamp'2021-01-01 01:02:03.000001' - date'2020-12-31';
1 01:02:03.000001000
```
### How was this patch tested?
1. Added new tests to `IntervalUtilsSuite`:
```
$ build/sbt "test:testOnly *IntervalUtilsSuite"
```
2. Modified existing tests in `HiveResultSuite`:
```
$ build/sbt -Phive-2.3 -Phive-thriftserver "testOnly *HiveResultSuite"
```
3. By running cast tests:
```
$ build/sbt "testOnly *CastSuite*"
```
Closes#32120 from MaxGekk/ansi-intervals-hive-thrift-server.
Authored-by: Max Gekk <max.gekk@gmail.com>
Signed-off-by: Max Gekk <max.gekk@gmail.com>
### What changes were proposed in this pull request?
This patch proposes a fix of nested column pruning for extracting case-insensitive struct field from array of struct.
### Why are the changes needed?
Under case-insensitive mode, nested column pruning rule cannot correctly push down extractor of a struct field of an array of struct, e.g.,
```scala
val query = spark.table("contacts").select("friends.First", "friends.MiDDle")
```
Error stack:
```
[info] java.lang.IllegalArgumentException: Field "First" does not exist.
[info] Available fields:
[info] at org.apache.spark.sql.types.StructType$$anonfun$apply$1.apply(StructType.scala:274)
[info] at org.apache.spark.sql.types.StructType$$anonfun$apply$1.apply(StructType.scala:274)
[info] at scala.collection.MapLike$class.getOrElse(MapLike.scala:128)
[info] at scala.collection.AbstractMap.getOrElse(Map.scala:59)
[info] at org.apache.spark.sql.types.StructType.apply(StructType.scala:273)
[info] at org.apache.spark.sql.execution.ProjectionOverSchema$$anonfun$getProjection$3.apply(ProjectionOverSchema.scala:44)
[info] at org.apache.spark.sql.execution.ProjectionOverSchema$$anonfun$getProjection$3.apply(ProjectionOverSchema.scala:41)
```
### Does this PR introduce _any_ user-facing change?
No
### How was this patch tested?
Unit test
Closes#32059 from viirya/fix-array-nested-pruning.
Authored-by: Liang-Chi Hsieh <viirya@gmail.com>
Signed-off-by: Liang-Chi Hsieh <viirya@gmail.com>
### What changes were proposed in this pull request?
One of the main performance bottlenecks in query compilation is overly-generic tree transformation methods, namely `mapChildren` and `withNewChildren` (defined in `TreeNode`). These methods have an overly-generic implementation to iterate over the children and rely on reflection to create new instances. We have observed that, especially for queries with large query plans, a significant amount of CPU cycles are wasted in these methods. In this PR we make these methods more efficient, by delegating the iteration and instantiation to concrete node types. The benchmarks show that we can expect significant performance improvement in total query compilation time in queries with large query plans (from 30-80%) and about 20% on average.
#### Problem detail
The `mapChildren` method in `TreeNode` is overly generic and costly. To be more specific, this method:
- iterates over all the fields of a node using Scala’s product iterator. While the iteration is not reflection-based, thanks to the Scala compiler generating code for `Product`, we create many anonymous functions and visit many nested structures (recursive calls).
The anonymous functions (presumably compiled to Java anonymous inner classes) also show up quite high on the list in the object allocation profiles, so we are putting unnecessary pressure on GC here.
- does a lot of comparisons. Basically for each element returned from the product iterator, we check if it is a child (contained in the list of children) and then transform it. We can avoid that by just iterating over children, but in the current implementation, we need to gather all the fields (only transform the children) so that we can instantiate the object using the reflection.
- creates objects using reflection, by delegating to the `makeCopy` method, which is several orders of magnitude slower than using the constructor.
#### Solution
The proposed solution in this PR is rather straightforward: we rewrite the `mapChildren` method using the `children` and `withNewChildren` methods. The default `withNewChildren` method suffers from the same problems as `mapChildren` and we need to make it more efficient by specializing it in concrete classes. Similar to how each concrete query plan node already defines its children, it should also define how they can be constructed given a new list of children. Actually, the implementation is quite simple in most cases and is a one-liner thanks to the copy method present in Scala case classes. Note that we cannot abstract over the copy method, it’s generated by the compiler for case classes if no other type higher in the hierarchy defines it. For most concrete nodes, the implementation of `withNewChildren` looks like this:
```
override def withNewChildren(newChildren: Seq[LogicalPlan]): LogicalPlan = copy(children = newChildren)
```
The current `withNewChildren` method has two properties that we should preserve:
- It returns the same instance if the provided children are the same as its children, i.e., it preserves referential equality.
- It copies tags and maintains the origin links when a new copy is created.
These properties are hard to enforce in the concrete node type implementation. Therefore, we propose a template method `withNewChildrenInternal` that should be rewritten by the concrete classes and let the `withNewChildren` method take care of referential equality and copying:
```
override def withNewChildren(newChildren: Seq[LogicalPlan]): LogicalPlan = {
if (childrenFastEquals(children, newChildren)) {
this
} else {
CurrentOrigin.withOrigin(origin) {
val res = withNewChildrenInternal(newChildren)
res.copyTagsFrom(this)
res
}
}
}
```
With the refactoring done in a previous PR (https://github.com/apache/spark/pull/31932) most tree node types fall in one of the categories of `Leaf`, `Unary`, `Binary` or `Ternary`. These traits have a more efficient implementation for `mapChildren` and define a more specialized version of `withNewChildrenInternal` that avoids creating unnecessary lists. For example, the `mapChildren` method in `UnaryLike` is defined as follows:
```
override final def mapChildren(f: T => T): T = {
val newChild = f(child)
if (newChild fastEquals child) {
this.asInstanceOf[T]
} else {
CurrentOrigin.withOrigin(origin) {
val res = withNewChildInternal(newChild)
res.copyTagsFrom(this.asInstanceOf[T])
res
}
}
}
```
#### Results
With this PR, we have observed significant performance improvements in query compilation time, more specifically in the analysis and optimization phases. The table below shows the TPC-DS queries that had more than 25% speedup in compilation times. Biggest speedups are observed in queries with large query plans.
| Query | Speedup |
| ------------- | ------------- |
|q4 |29%|
|q9 |81%|
|q14a |31%|
|q14b |28%|
|q22 |33%|
|q33 |29%|
|q34 |25%|
|q39 |27%|
|q41 |27%|
|q44 |26%|
|q47 |28%|
|q48 |76%|
|q49 |46%|
|q56 |26%|
|q58 |43%|
|q59 |46%|
|q60 |50%|
|q65 |59%|
|q66 |46%|
|q67 |52%|
|q69 |31%|
|q70 |30%|
|q96 |26%|
|q98 |32%|
#### Binary incompatibility
Changing the `withNewChildren` in `TreeNode` breaks the binary compatibility of the code compiled against older versions of Spark because now it is expected that concrete `TreeNode` subclasses all implement the `withNewChildrenInternal` method. This is a problem, for example, when users write custom expressions. This change is the right choice, since it forces all newly added expressions to Catalyst implement it in an efficient manner and will prevent future regressions.
Please note that we have not completely removed the old implementation and renamed it to `legacyWithNewChildren`. This method will be removed in the future and for now helps the transition. There are expressions such as `UpdateFields` that have a complex way of defining children. Writing `withNewChildren` for them requires refactoring the expression. For now, these expressions use the old, slow method. In a future PR we address these expressions.
### Does this PR introduce _any_ user-facing change?
This PR does not introduce user facing changes but my break binary compatibility of the code compiled against older versions. See the binary compatibility section.
### How was this patch tested?
This PR is mainly a refactoring and passes existing tests.
Closes#32030 from dbaliafroozeh/ImprovedMapChildren.
Authored-by: Ali Afroozeh <ali.afroozeh@databricks.com>
Signed-off-by: herman <herman@databricks.com>
### What changes were proposed in this pull request?
Implement toString() and sql() methods for TRY_CAST
### Why are the changes needed?
The new expression should have a different name from `CAST` in SQL/String representation.
### Does this PR introduce _any_ user-facing change?
Yes, in the result of `explain()`, users can see try_cast if the new expression is used.
### How was this patch tested?
Unit tests.
Closes#32098 from gengliangwang/tryCastString.
Authored-by: Gengliang Wang <ltnwgl@gmail.com>
Signed-off-by: Gengliang Wang <ltnwgl@gmail.com>
### What changes were proposed in this pull request?
Change UpdateAction and InsertAction of MergeIntoTable to explicitly represent star,
### Why are the changes needed?
Currently, UpdateAction and InsertAction in the MergeIntoTable implicitly represent `update set *` and `insert *` with empty assignments. That means there is no way to differentiate between the representations of "update all columns" and "update no columns". For SQL MERGE queries, this inability does not matter because the SQL MERGE grammar that generated the MergeIntoTable plan does not allow "update no columns". However, other ways of generating the MergeIntoTable plan may not have that limitation, and may want to allow specifying "update no columns". For example, in the Delta Lake project we provide a type-safe Scala API for Merge, where it is perfectly valid to produce a Merge query with an update clause but no update assignments. Currently, we cannot use MergeIntoTable to represent this plan, thus complicating the generation, and resolution of merge query from scala API.
Side note: fixed another bug where a merge plan with star and no other expressions with unresolved attributes (e.g. all non-optional predicates are `literal(true)`), then resolution will be skipped and star wont expanded. added test for that.
### Does this PR introduce _any_ user-facing change?
No
### How was this patch tested?
Existing unit tests
Closes#32067 from tdas/SPARK-34962-2.
Authored-by: Tathagata Das <tathagata.das1565@gmail.com>
Signed-off-by: Wenchen Fan <wenchen@databricks.com>
### What changes were proposed in this pull request?
Currently, we can't support use ordinal in CUBE/ROLLUP/GROUPING SETS,
this pr make CUBE/ROLLUP/GROUPING SETS support GROUP BY ordinal
### Why are the changes needed?
Make CUBE/ROLLUP/GROUPING SETS support GROUP BY ordinal.
Postgres SQL and TeraData support this use case.
### Does this PR introduce _any_ user-facing change?
User can use ordinal in CUBE/ROLLUP/GROUPING SETS, such as
```
-- can use ordinal in CUBE
select a, b, count(1) from data group by cube(1, 2);
-- mixed cases: can use ordinal in CUBE
select a, b, count(1) from data group by cube(1, b);
-- can use ordinal with cube
select a, b, count(1) from data group by 1, 2 with cube;
-- can use ordinal in ROLLUP
select a, b, count(1) from data group by rollup(1, 2);
-- mixed cases: can use ordinal in ROLLUP
select a, b, count(1) from data group by rollup(1, b);
-- can use ordinal with rollup
select a, b, count(1) from data group by 1, 2 with rollup;
-- can use ordinal in GROUPING SETS
select a, b, count(1) from data group by grouping sets((1), (2), (1, 2));
-- mixed cases: can use ordinal in GROUPING SETS
select a, b, count(1) from data group by grouping sets((1), (b), (a, 2));
select a, b, count(1) from data group by a, 2 grouping sets((1), (b), (a, 2));
```
### How was this patch tested?
Added UT
Closes#30145 from AngersZhuuuu/SPARK-33233.
Lead-authored-by: Angerszhuuuu <angers.zhu@gmail.com>
Co-authored-by: angerszhu <angers.zhu@gmail.com>
Co-authored-by: AngersZhuuuu <angers.zhu@gmail.com>
Signed-off-by: Wenchen Fan <wenchen@databricks.com>
### What changes were proposed in this pull request?
This PR adds two additional checks in `CheckAnalysis` for correlated scalar subquery in Aggregate. It blocks the cases that Spark do not currently support based on the rewrite logic in `RewriteCorrelatedScalarSubquery`:
aff6c0febb/sql/catalyst/src/main/scala/org/apache/spark/sql/catalyst/optimizer/subquery.scala (L618-L624)
### Why are the changes needed?
It can be confusing to users when their queries pass the check analysis but cannot be executed. Also, the error messages are confusing:
#### Case 1: correlated scalar subquery in the grouping expressions but not in aggregate expressions
```sql
SELECT SUM(c2) FROM t t1 GROUP BY (SELECT SUM(c2) FROM t t2 WHERE t1.c1 = t2.c1)
```
We get this error:
```
java.lang.AssertionError: assertion failed: Expects 1 field, but got 2; something went wrong in analysis
```
because the correlated scalar subquery is not rewritten properly:
```scala
== Optimized Logical Plan ==
Aggregate [scalar-subquery#5 [(c1#6 = c1#6#93)]], [sum(c2#7) AS sum(c2)#11L]
: +- Aggregate [c1#6], [sum(c2#7) AS sum(c2)#15L, c1#6 AS c1#6#93]
: +- LocalRelation [c1#6, c2#7]
+- LocalRelation [c1#6, c2#7]
```
#### Case 2: correlated scalar subquery in the aggregate expressions but not in the grouping expressions
```sql
SELECT (SELECT SUM(c2) FROM t t2 WHERE t1.c1 = t2.c1), SUM(c2) FROM t t1 GROUP BY c1
```
We get this error:
```
java.lang.IllegalStateException: Couldn't find sum(c2)#69L in [c1#60,sum(c2#61)#64L]
```
because the transformed correlated scalar subquery output is not present in the grouping expression of the Aggregate:
```scala
== Optimized Logical Plan ==
Aggregate [c1#60], [sum(c2)#69L AS scalarsubquery(c1)#70L, sum(c2#61) AS sum(c2)#65L]
+- Project [c1#60, c2#61, sum(c2)#69L]
+- Join LeftOuter, (c1#60 = c1#60#95)
:- LocalRelation [c1#60, c2#61]
+- Aggregate [c1#60], [sum(c2#61) AS sum(c2)#69L, c1#60 AS c1#60#95]
+- LocalRelation [c1#60, c2#61]
```
### Does this PR introduce _any_ user-facing change?
Yes
### How was this patch tested?
New unit tests
Closes#32054 from allisonwang-db/spark-34946-scalar-subquery-agg.
Authored-by: allisonwang-db <66282705+allisonwang-db@users.noreply.github.com>
Signed-off-by: Wenchen Fan <wenchen@databricks.com>
### What changes were proposed in this pull request?
1. Added new method `toDayTimeIntervalString()` to `IntervalUtils` which converts a day-time interval as a number of microseconds to a string in the form **"INTERVAL '[sign]days hours:minutes:secondsWithFraction' DAY TO SECOND"**.
2. Extended the `Cast` expression to support casting of `DayTimeIntervalType` to `StringType`.
### Why are the changes needed?
To conform the ANSI SQL standard which requires to support such casting.
### Does this PR introduce _any_ user-facing change?
Should not because new day-time interval has not been released yet.
### How was this patch tested?
Added new tests for casting:
```
$ build/sbt "testOnly *CastSuite*"
```
Closes#32070 from MaxGekk/cast-dt-interval-to-string.
Authored-by: Max Gekk <max.gekk@gmail.com>
Signed-off-by: Wenchen Fan <wenchen@databricks.com>
### What changes were proposed in this pull request?
Current trait `GroupingSet` is ambiguous, since `grouping set` in parser level means one set of a group.
Rename this to `BaseGroupingSets` since cube/rollup is syntax sugar for grouping sets.`
### Why are the changes needed?
Refactor class name
### Does this PR introduce _any_ user-facing change?
No
### How was this patch tested?
Not need
Closes#32073 from AngersZhuuuu/SPARK-34976.
Authored-by: Angerszhuuuu <angers.zhu@gmail.com>
Signed-off-by: Wenchen Fan <wenchen@databricks.com>
### What changes were proposed in this pull request?
The `explain()` method prints the arguments of tree nodes in logical/physical plans. The arguments could contain a map-type option that contains sensitive data.
We should map-type options in the output of `explain()`. Otherwise, we will see sensitive data in explain output or Spark UI.
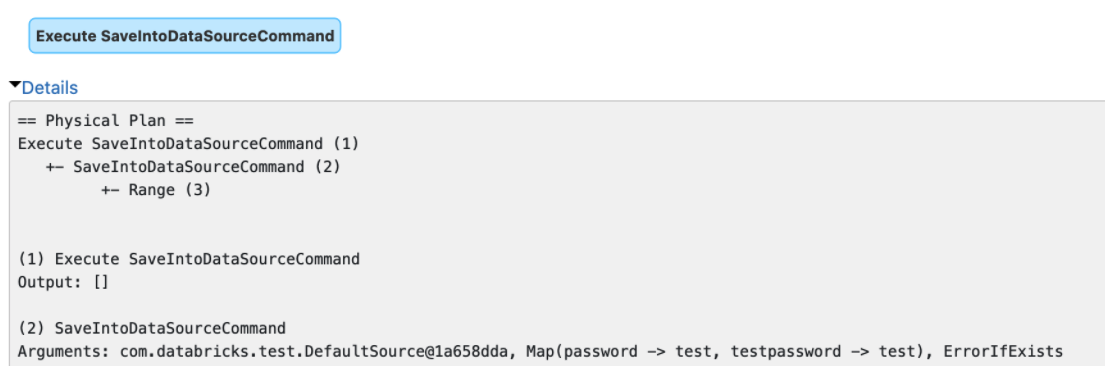
### Why are the changes needed?
Data security.
### Does this PR introduce _any_ user-facing change?
Yes, redact the map-type options in the output of `explain()`
### How was this patch tested?
Unit tests
Closes#32066 from gengliangwang/redactOptions.
Authored-by: Gengliang Wang <ltnwgl@gmail.com>
Signed-off-by: Gengliang Wang <ltnwgl@gmail.com>
## What changes were proposed in this pull request?
This adds a new API for catalog plugins that exposes functions to Spark. The API can list and load functions. This does not include create, delete, or alter operations.
- [Design Document](https://docs.google.com/document/d/1PLBieHIlxZjmoUB0ERF-VozCRJ0xw2j3qKvUNWpWA2U/edit?usp=sharing)
There are 3 types of functions defined:
* A `ScalarFunction` that produces a value for every call
* An `AggregateFunction` that produces a value after updates for a group of rows
Functions loaded from the catalog by name as `UnboundFunction`. Once input arguments are determined `bind` is called on the unbound function to get a `BoundFunction` implementation that is one of the 3 types above. Binding can fail if the function doesn't support the input type. `BoundFunction` returns the result type produced by the function.
## How was this patch tested?
This includes a test that demonstrates the new API.
Closes#24559 from rdblue/SPARK-27658-add-function-catalog-api.
Authored-by: Ryan Blue <blue@apache.org>
Signed-off-by: Wenchen Fan <wenchen@databricks.com>
### What changes were proposed in this pull request?
This is a followup for https://github.com/apache/spark/pull/31932.
In this PR we:
- Introduce the `QuaternaryLike` trait for node types with 4 children.
- Specialize more node types
- Fix a number of style errors that were introduced in the original PR.
### Why are the changes needed?
### Does this PR introduce _any_ user-facing change?
### How was this patch tested?
This is a refactoring, passes existing tests.
Closes#32065 from dbaliafroozeh/FollowupSPARK-34906.
Authored-by: Ali Afroozeh <ali.afroozeh@databricks.com>
Signed-off-by: herman <herman@databricks.com>
### What changes were proposed in this pull request?
This PR extends the current function registry and catalog to support table-valued functions by adding a table function registry. It also refactors `range` to be a built-in function in the table function registry.
### Why are the changes needed?
Currently, Spark resolves table-valued functions very differently from the other functions. This change is to make the behavior for table and non-table functions consistent. It also allows Spark to display information about built-in table-valued functions:
Before:
```scala
scala> sql("describe function range").show(false)
+--------------------------+
|function_desc |
+--------------------------+
|Function: range not found.|
+--------------------------+
```
After:
```scala
Function: range
Class: org.apache.spark.sql.catalyst.plans.logical.Range
Usage:
range(start: Long, end: Long, step: Long, numPartitions: Int)
range(start: Long, end: Long, step: Long)
range(start: Long, end: Long)
range(end: Long)
// Extended
Function: range
Class: org.apache.spark.sql.catalyst.plans.logical.Range
Usage:
range(start: Long, end: Long, step: Long, numPartitions: Int)
range(start: Long, end: Long, step: Long)
range(start: Long, end: Long)
range(end: Long)
Extended Usage:
Examples:
> SELECT * FROM range(1);
+---+
| id|
+---+
| 0|
+---+
> SELECT * FROM range(0, 2);
+---+
|id |
+---+
|0 |
|1 |
+---+
> SELECT range(0, 4, 2);
+---+
|id |
+---+
|0 |
|2 |
+---+
Since: 2.0.0
```
### Does this PR introduce _any_ user-facing change?
Yes. User will not be able to create a function with name `range` in the default database:
Before:
```scala
scala> sql("create function range as 'range'")
res3: org.apache.spark.sql.DataFrame = []
```
After:
```
scala> sql("create function range as 'range'")
org.apache.spark.sql.catalyst.analysis.FunctionAlreadyExistsException: Function 'default.range' already exists in database 'default'
```
### How was this patch tested?
Unit test
Closes#31791 from allisonwang-db/spark-34678-table-func-registry.
Authored-by: allisonwang-db <66282705+allisonwang-db@users.noreply.github.com>
Signed-off-by: Wenchen Fan <wenchen@databricks.com>
### What changes were proposed in this pull request?
Changed the cost comparison function of the CBO to use the ratios of row counts and sizes in bytes.
### Why are the changes needed?
In #30965 we changed to CBO cost comparison function so it would be "symetric": `A.betterThan(B)` now implies, that `!B.betterThan(A)`.
With that we caused a performance regressions in some queries - TPCDS q19 for example.
The original cost comparison function used the ratios `relativeRows = A.rowCount / B.rowCount` and `relativeSize = A.size / B.size`. The changed function compared "absolute" cost values `costA = w*A.rowCount + (1-w)*A.size` and `costB = w*B.rowCount + (1-w)*B.size`.
Given the input from wzhfy we decided to go back to the relative values, because otherwise one (size) may overwhelm the other (rowCount). But this time we avoid adding up the ratios.
Originally `A.betterThan(B) => w*relativeRows + (1-w)*relativeSize < 1` was used. Besides being "non-symteric", this also can exhibit one overwhelming other.
For `w=0.5` If `A` size (bytes) is at least 2x larger than `B`, then no matter how many times more rows does the `B` plan have, `B` will allways be considered to be better - `0.5*2 + 0.5*0.00000000000001 > 1`.
When working with ratios, then it would be better to multiply them.
The proposed cost comparison function is: `A.betterThan(B) => relativeRows^w * relativeSize^(1-w) < 1`.
### Does this PR introduce _any_ user-facing change?
Comparison of the changed TPCDS v1.4 query execution times at sf=10:
| absolute | multiplicative | | additive |
-- | -- | -- | -- | -- | --
q12 | 145 | 137 | -5.52% | 141 | -2.76%
q13 | 264 | 271 | 2.65% | 271 | 2.65%
q17 | 4521 | 4243 | -6.15% | 4348 | -3.83%
q18 | 758 | 466 | -38.52% | 480 | -36.68%
q19 | 38503 | 2167 | -94.37% | 2176 | -94.35%
q20 | 119 | 120 | 0.84% | 126 | 5.88%
q24a | 16429 | 16838 | 2.49% | 17103 | 4.10%
q24b | 16592 | 16999 | 2.45% | 17268 | 4.07%
q25 | 3558 | 3556 | -0.06% | 3675 | 3.29%
q33 | 362 | 361 | -0.28% | 380 | 4.97%
q52 | 1020 | 1032 | 1.18% | 1052 | 3.14%
q55 | 927 | 938 | 1.19% | 961 | 3.67%
q72 | 24169 | 13377 | -44.65% | 24306 | 0.57%
q81 | 1285 | 1185 | -7.78% | 1168 | -9.11%
q91 | 324 | 336 | 3.70% | 337 | 4.01%
q98 | 126 | 129 | 2.38% | 131 | 3.97%
All times are in ms, the change is compared to the situation in the master branch (absolute).
The proposed cost function (multiplicative) significantlly improves the performance on q18, q19 and q72. The original cost function (additive) has similar improvements at q18 and q19. All other chagnes are within the error bars and I would ignore them - perhaps q81 has also improved.
### How was this patch tested?
PlanStabilitySuite
Closes#32014 from tanelk/SPARK-34922_cbo_better_cost_function.
Lead-authored-by: Tanel Kiis <tanel.kiis@gmail.com>
Co-authored-by: tanel.kiis@gmail.com <tanel.kiis@gmail.com>
Signed-off-by: Takeshi Yamamuro <yamamuro@apache.org>
### What changes were proposed in this pull request?
1. Added new method `toYearMonthIntervalString()` to `IntervalUtils` which converts an year-month interval as a number of month to a string in the form **"INTERVAL '[sign]yearField-monthField' YEAR TO MONTH"**.
2. Extended the `Cast` expression to support casting of `YearMonthIntervalType` to `StringType`.
### Why are the changes needed?
To conform the ANSI SQL standard which requires to support such casting.
### Does this PR introduce _any_ user-facing change?
Should not because new year-month interval has not been released yet.
### How was this patch tested?
Added new tests for casting:
```
$ build/sbt "testOnly *CastSuite*"
```
Closes#32056 from MaxGekk/cast-ym-interval-to-string.
Authored-by: Max Gekk <max.gekk@gmail.com>
Signed-off-by: Max Gekk <max.gekk@gmail.com>
### What changes were proposed in this pull request?
Changes the metadata propagation framework.
Previously, most `LogicalPlan`'s propagated their `children`'s `metadataOutput`. This did not make sense in cases where the `LogicalPlan` did not even propagate their `children`'s `output`.
I set the metadata output for plans that do not propagate their `children`'s `output` to be `Nil`. Notably, `Project` and `View` no longer have metadata output.
### Why are the changes needed?
Previously, `SELECT m from (SELECT a from tb)` would output `m` if it were metadata. This did not make sense.
### Does this PR introduce _any_ user-facing change?
Yes. Now, `SELECT m from (SELECT a from tb)` will encounter an `AnalysisException`.
### How was this patch tested?
Added unit tests. I did not cover all cases, as they are fairly extensive. However, the new tests cover major cases (and an existing test already covers Join).
Closes#32017 from karenfeng/spark-34923.
Authored-by: Karen Feng <karen.feng@databricks.com>
Signed-off-by: Wenchen Fan <wenchen@databricks.com>
### What changes were proposed in this pull request?
GROUP BY ... GROUPING SETS (...) is a weird SQL syntax we copied from Hive. It's not in the SQL standard or any other mainstream databases. This syntax requires users to repeat the expressions inside `GROUPING SETS (...)` after `GROUP BY`, and has a weird null semantic if `GROUP BY` contains extra expressions than `GROUPING SETS (...)`.
This PR deprecates this syntax:
1. Do not promote it in the document and only mention it as a Hive compatible sytax.
2. Simplify the code to only keep it for Hive compatibility.
### Why are the changes needed?
Deprecate a weird grammar.
### Does this PR introduce _any_ user-facing change?
No breaking change, but it removes a check to simplify the code: `GROUP BY a GROUPING SETS(a, b)` fails before and forces users to also put `b` after `GROUP BY`. Now this works just as `GROUP BY GROUPING SETS(a, b)`.
### How was this patch tested?
existing tests
Closes#32022 from cloud-fan/followup.
Authored-by: Wenchen Fan <wenchen@databricks.com>
Signed-off-by: Takeshi Yamamuro <yamamuro@apache.org>
### What changes were proposed in this pull request?
https://github.com/apache/spark/pull/32015 added a way to run benchmarks much more easily in the same GitHub Actions build. This PR updates the benchmark results by using the way.
**NOTE** that looks like GitHub Actions use four types of CPU given my observations:
- Intel(R) Xeon(R) Platinum 8171M CPU 2.60GHz
- Intel(R) Xeon(R) CPU E5-2673 v4 2.30GHz
- Intel(R) Xeon(R) CPU E5-2673 v3 2.40GHz
- Intel(R) Xeon(R) Platinum 8272CL CPU 2.60GHz
Given my quick research, seems like they perform roughly similarly:
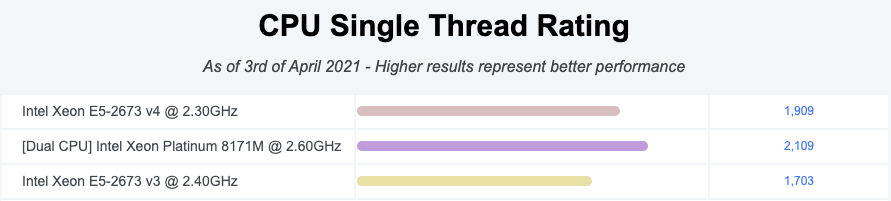
I couldn't find enough information about Intel(R) Xeon(R) Platinum 8272CL CPU 2.60GHz but the performance seems roughly similar given the numbers.
So shouldn't be a big deal especially given that this way is much easier, encourages contributors to run more and guarantee the same number of cores and same memory with the same softwares.
### Why are the changes needed?
To have a base line of the benchmarks accordingly.
### Does this PR introduce _any_ user-facing change?
No, dev-only.
### How was this patch tested?
It was generated from:
- [Run benchmarks: * (JDK 11)](https://github.com/HyukjinKwon/spark/actions/runs/713575465)
- [Run benchmarks: * (JDK 8)](https://github.com/HyukjinKwon/spark/actions/runs/713154337)
Closes#32044 from HyukjinKwon/SPARK-34950.
Authored-by: HyukjinKwon <gurwls223@apache.org>
Signed-off-by: Max Gekk <max.gekk@gmail.com>
### What changes were proposed in this pull request?
Use proper Java doc format for Java classes within `catalyst` module
### Why are the changes needed?
Many Java classes in `catalyst`, especially those for DataSource V2, do not have proper Java doc format. By fixing the format it helps to improve the doc's readability.
### Does this PR introduce _any_ user-facing change?
No
### How was this patch tested?
N/A
Closes#32038 from sunchao/javadoc.
Authored-by: Chao Sun <sunchao@apple.com>
Signed-off-by: Dongjoon Hyun <dhyun@apple.com>
### What changes were proposed in this pull request?
When we insert data into a partition table partition with empty DataFrame. We will call `PartitioningUtils.getPathFragment()`
then to update this partition's metadata too.
When we insert to a partition when partition value is `null`, it will throw exception like
```
[info] java.lang.NullPointerException:
[info] at scala.collection.immutable.StringOps$.length$extension(StringOps.scala:51)
[info] at scala.collection.immutable.StringOps.length(StringOps.scala:51)
[info] at scala.collection.IndexedSeqOptimized.foreach(IndexedSeqOptimized.scala:35)
[info] at scala.collection.IndexedSeqOptimized.foreach$(IndexedSeqOptimized.scala:33)
[info] at scala.collection.immutable.StringOps.foreach(StringOps.scala:33)
[info] at org.apache.spark.sql.catalyst.catalog.ExternalCatalogUtils$.escapePathName(ExternalCatalogUtils.scala:69)
[info] at org.apache.spark.sql.catalyst.catalog.ExternalCatalogUtils$.getPartitionValueString(ExternalCatalogUtils.scala:126)
[info] at org.apache.spark.sql.execution.datasources.PartitioningUtils$.$anonfun$getPathFragment$1(PartitioningUtils.scala:354)
[info] at scala.collection.TraversableLike.$anonfun$map$1(TraversableLike.scala:238)
[info] at scala.collection.Iterator.foreach(Iterator.scala:941)
[info] at scala.collection.Iterator.foreach$(Iterator.scala:941)
[info] at scala.collection.AbstractIterator.foreach(Iterator.scala:1429)
[info] at scala.collection.IterableLike.foreach(IterableLike.scala:74)
[info] at scala.collection.IterableLike.foreach$(IterableLike.scala:73)
```
`PartitioningUtils.getPathFragment()` should support `null` value too
### Why are the changes needed?
Fix bug
### Does this PR introduce _any_ user-facing change?
No
### How was this patch tested?
Added UT
Closes#32018 from AngersZhuuuu/SPARK-34926.
Authored-by: Angerszhuuuu <angers.zhu@gmail.com>
Signed-off-by: Max Gekk <max.gekk@gmail.com>
### What changes were proposed in this pull request?
This PR introduces a new analysis rule `DeduplicateRelations`, which deduplicates any duplicate relations in a plan first and then deduplicates conflicting attributes(which resued the `dedupRight` of `ResolveReferences`).
### Why are the changes needed?
`CostBasedJoinReorder` could fail when applying on self-join, e.g.,
```scala
// test in JoinReorderSuite
test("join reorder with self-join") {
val plan = t2.join(t1, Inner, Some(nameToAttr("t1.k-1-2") === nameToAttr("t2.k-1-5")))
.select(nameToAttr("t1.v-1-10"))
.join(t2, Inner, Some(nameToAttr("t1.v-1-10") === nameToAttr("t2.k-1-5")))
// this can fail
Optimize.execute(plan.analyze)
}
```
Besides, with the new rule `DeduplicateRelations`, we'd be able to enable some optimizations, e.g., LeftSemiAnti pushdown, redundant project removal, as reflects in updated unit tests.
### Does this PR introduce _any_ user-facing change?
### How was this patch tested?
Added and updated unit tests.
Closes#32027 from Ngone51/join-reorder-3.
Lead-authored-by: yi.wu <yi.wu@databricks.com>
Co-authored-by: Wenchen Fan <wenchen@databricks.com>
Signed-off-by: Wenchen Fan <wenchen@databricks.com>
### What changes were proposed in this pull request?
This PR is to support nested column type in Spark ORC vectorized reader. Currently ORC vectorized reader [does not support nested column type (struct, array and map)](https://github.com/apache/spark/blob/master/sql/core/src/main/scala/org/apache/spark/sql/execution/datasources/orc/OrcFileFormat.scala#L138). We implemented nested column vectorized reader for FB-ORC in our internal fork of Spark. We are seeing performance improvement compared to non-vectorized reader when reading nested columns. In addition, this can also help improve the non-nested column performance when reading non-nested and nested columns together in one query.
Before this PR:
* `OrcColumnVector` is the implementation class for Spark's `ColumnVector` to wrap Hive's/ORC's `ColumnVector` to read `AtomicType` data.
After this PR:
* `OrcColumnVector` is an abstract class to keep interface being shared between multiple implementation class of orc column vectors, namely `OrcAtomicColumnVector` (for `AtomicType`), `OrcArrayColumnVector` (for `ArrayType`), `OrcMapColumnVector` (for `MapType`), `OrcStructColumnVector` (for `StructType`). So the original logic to read `AtomicType` data is moved from `OrcColumnVector` to `OrcAtomicColumnVector`. The abstract class of `OrcColumnVector` is needed here because of supporting nested column (i.e. nested column vectors).
* A utility method `OrcColumnVectorUtils.toOrcColumnVector` is added to create Spark's `OrcColumnVector` from Hive's/ORC's `ColumnVector`.
* A new user-facing config `spark.sql.orc.enableNestedColumnVectorizedReader` is added to control enabling/disabling vectorized reader for nested columns. The default value is false (i.e. disabling by default). For certain tables having deep nested columns, vectorized reader might take too much memory for each sub-column vectors, compared to non-vectorized reader. So providing a config here to work around OOM for query reading wide and deep nested columns if any. We plan to enable it by default on 3.3. Leave it disable in 3.2 in case for any unknown bugs.
### Why are the changes needed?
Improve query performance when reading nested columns from ORC file format.
Tested with locally adding a small benchmark in `OrcReadBenchmark.scala`. Seeing more than 1x run time improvement.
```
Running benchmark: SQL Nested Column Scan
Running case: Native ORC MR
Stopped after 2 iterations, 37850 ms
Running case: Native ORC Vectorized (Enabled Nested Column)
Stopped after 2 iterations, 15892 ms
Running case: Native ORC Vectorized (Disabled Nested Column)
Stopped after 2 iterations, 37954 ms
Running case: Hive built-in ORC
Stopped after 2 iterations, 35118 ms
Java HotSpot(TM) 64-Bit Server VM 1.8.0_181-b13 on Mac OS X 10.15.7
Intel(R) Core(TM) i9-9980HK CPU 2.40GHz
SQL Nested Column Scan: Best Time(ms) Avg Time(ms) Stdev(ms) Rate(M/s) Per Row(ns) Relative
------------------------------------------------------------------------------------------------------------------------------
Native ORC MR 18706 18925 310 0.1 17839.6 1.0X
Native ORC Vectorized (Enabled Nested Column) 7625 7946 455 0.1 7271.6 2.5X
Native ORC Vectorized (Disabled Nested Column) 18415 18977 796 0.1 17561.5 1.0X
Hive built-in ORC 17469 17559 127 0.1 16660.1 1.1X
```
Benchmark:
```
nestedColumnScanBenchmark(1024 * 1024)
def nestedColumnScanBenchmark(values: Int): Unit = {
val benchmark = new Benchmark(s"SQL Nested Column Scan", values, output = output)
withTempPath { dir =>
withTempTable("t1", "nativeOrcTable", "hiveOrcTable") {
import spark.implicits._
spark.range(values).map(_ => Random.nextLong).map { x =>
val arrayOfStructColumn = (0 until 5).map(i => (x + i, s"$x" * 5))
val mapOfStructColumn = Map(
s"$x" -> (x * 0.1, (x, s"$x" * 100)),
(s"$x" * 2) -> (x * 0.2, (x, s"$x" * 200)),
(s"$x" * 3) -> (x * 0.3, (x, s"$x" * 300)))
(arrayOfStructColumn, mapOfStructColumn)
}.toDF("col1", "col2")
.createOrReplaceTempView("t1")
prepareTable(dir, spark.sql(s"SELECT * FROM t1"))
benchmark.addCase("Native ORC MR") { _ =>
withSQLConf(SQLConf.ORC_VECTORIZED_READER_ENABLED.key -> "false") {
spark.sql("SELECT SUM(SIZE(col1)), SUM(SIZE(col2)) FROM nativeOrcTable").noop()
}
}
benchmark.addCase("Native ORC Vectorized (Enabled Nested Column)") { _ =>
spark.sql("SELECT SUM(SIZE(col1)), SUM(SIZE(col2)) FROM nativeOrcTable").noop()
}
benchmark.addCase("Native ORC Vectorized (Disabled Nested Column)") { _ =>
withSQLConf(SQLConf.ORC_VECTORIZED_READER_NESTED_COLUMN_ENABLED.key -> "false") {
spark.sql("SELECT SUM(SIZE(col1)), SUM(SIZE(col2)) FROM nativeOrcTable").noop()
}
}
benchmark.addCase("Hive built-in ORC") { _ =>
spark.sql("SELECT SUM(SIZE(col1)), SUM(SIZE(col2)) FROM hiveOrcTable").noop()
}
benchmark.run()
}
}
}
```
### Does this PR introduce _any_ user-facing change?
No.
### How was this patch tested?
Added one simple test in `OrcSourceSuite.scala` to verify correctness.
Definitely need more unit tests and add benchmark here, but I want to first collect feedback before crafting more tests.
Closes#31958 from c21/orc-vector.
Authored-by: Cheng Su <chengsu@fb.com>
Signed-off-by: Liang-Chi Hsieh <viirya@gmail.com>
### What changes were proposed in this pull request?
Modify the `SubtractTimestamps` expression to return values of `DayTimeIntervalType` when `spark.sql.legacy.interval.enabled` is set to `false` (which is the default).
### Why are the changes needed?
To conform to the ANSI SQL standard which requires ANSI intervals as the result of timestamps subtraction, see
<img width="656" alt="Screenshot 2021-03-29 at 19 09 34" src="https://user-images.githubusercontent.com/1580697/112866455-7e2f0d00-90c2-11eb-96e6-3feb7eea7e09.png">
### Does this PR introduce _any_ user-facing change?
Yes.
### How was this patch tested?
By running new tests:
```
$ build/sbt "test:testOnly *DateTimeUtilsSuite"
$ build/sbt "test:testOnly *DateExpressionsSuite"
$ build/sbt "test:testOnly *ColumnExpressionSuite"
```
and some tests from `SQLQueryTestSuite`:
```
$ build/sbt "sql/testOnly *SQLQueryTestSuite -- -z timestamp.sql"
$ build/sbt "sql/testOnly *SQLQueryTestSuite -- -z datetime.sql"
$ build/sbt "sql/testOnly *SQLQueryTestSuite -- -z interval.sql"
```
Closes#32016 from MaxGekk/subtract-timestamps-to-intervals.
Authored-by: Max Gekk <max.gekk@gmail.com>
Signed-off-by: Max Gekk <max.gekk@gmail.com>
### What changes were proposed in this pull request?
Change partitioning to `SinglePartition`.
### Why are the changes needed?
For node `Repartition` and `RepartitionByExpression`, if partition number is 1 we can use `SinglePartition` instead of other `Partitioning`.
### Does this PR introduce _any_ user-facing change?
No
### How was this patch tested?
Add test
Closes#32012 from ulysses-you/SPARK-34919.
Authored-by: ulysses-you <ulyssesyou18@gmail.com>
Signed-off-by: Wenchen Fan <wenchen@databricks.com>
### What changes were proposed in this pull request?
This PR fixes JDK 11 compilation failed:
```
/home/runner/work/spark/spark/sql/catalyst/src/main/scala/org/apache/spark/sql/catalyst/expressions/TryCast.scala:35: error: annotation argument needs to be a constant; found: "_FUNC_(expr AS type) - Casts the value `expr` to the target data type `type`. ".+("This expression is identical to CAST with configuration `spark.sql.ansi.enabled` as ").+("true, except it returns NULL instead of raising an error. Note that the behavior of this ").+("expression doesn\'t depend on configuration `spark.sql.ansi.enabled`.")
"true, except it returns NULL instead of raising an error. Note that the behavior of this " +
```
For whatever reason, it doesn't know that the string is actually a constant. This PR simply switches it to multi-line style (which is actually more correct).
Reference:
bd0990e3e8/sql/catalyst/src/main/scala/org/apache/spark/sql/catalyst/expressions/aggregate/ApproximatePercentile.scala (L53-L57)
### Why are the changes needed?
To recover the build.
### Does this PR introduce _any_ user-facing change?
No, dev-only.
### How was this patch tested?
CI in this PR
Closes#32019 from HyukjinKwon/SPARK-34881.
Lead-authored-by: Hyukjin Kwon <gurwls223@apache.org>
Co-authored-by: HyukjinKwon <gurwls223@apache.org>
Signed-off-by: Gengliang Wang <ltnwgl@gmail.com>
### What changes were proposed in this pull request?
Replaced the `agg(if (('gid = 1)) 'cat1 else null)` pattern in `RewriteDistinctAggregates` with `agg('cat1) FILTER (WHERE 'gid = 1)`
### Why are the changes needed?
For aggregate functions, that do not ignore NULL values (`First`, `Last` or `UDAF`s) the current approach can return wrong results.
In the added UT there are no nulls in the input `testData`. The query returned `Row(0, 1, 0, 51, 100)` before this PR.
### Does this PR introduce _any_ user-facing change?
Bugfix
### How was this patch tested?
UT
Closes#31983 from tanelk/SPARK-34882_distinct_agg_filter.
Lead-authored-by: Tanel Kiis <tanel.kiis@gmail.com>
Co-authored-by: tanel.kiis@gmail.com <tanel.kiis@gmail.com>
Signed-off-by: Takeshi Yamamuro <yamamuro@apache.org>
### What changes were proposed in this pull request?
Add a new SQL function `try_cast`.
`try_cast` is identical to `AnsiCast` (or `Cast` when `spark.sql.ansi.enabled` is true), except it returns NULL instead of raising an error.
This expression has one major difference from `cast` with `spark.sql.ansi.enabled` as true: when the source value can't be stored in the target integral(Byte/Short/Int/Long) type, `try_cast` returns null instead of returning the low order bytes of the source value.
Note that the result of `try_cast` is not affected by the configuration `spark.sql.ansi.enabled`.
This is learned from Google BigQuery and Snowflake:
https://docs.snowflake.com/en/sql-reference/functions/try_cast.htmlhttps://cloud.google.com/bigquery/docs/reference/standard-sql/functions-and-operators#safe_casting
### Why are the changes needed?
This is an useful for the following scenarios:
1. When ANSI mode is on, users can choose `try_cast` an alternative way to run SQL without errors for certain operations.
2. When ANSI mode is off, users can use `try_cast` to get a more reasonable result for casting a value to an integral type: when an overflow error happens, `try_cast` returns null while `cast` returns the low order bytes of the source value.
### Does this PR introduce _any_ user-facing change?
Yes, adding a new function `try_cast`
### How was this patch tested?
Unit tests.
Closes#31982 from gengliangwang/tryCast.
Authored-by: Gengliang Wang <ltnwgl@gmail.com>
Signed-off-by: Gengliang Wang <ltnwgl@gmail.com>
### What changes were proposed in this pull request?
This PR introduces a new analysis rule `DeduplicateRelations`, which deduplicates any duplicate relations in a plan first and then deduplicates conflicting attributes(which resued the `dedupRight` of `ResolveReferences`).
### Why are the changes needed?
`CostBasedJoinReorder` could fail when applying on self-join, e.g.,
```scala
// test in JoinReorderSuite
test("join reorder with self-join") {
val plan = t2.join(t1, Inner, Some(nameToAttr("t1.k-1-2") === nameToAttr("t2.k-1-5")))
.select(nameToAttr("t1.v-1-10"))
.join(t2, Inner, Some(nameToAttr("t1.v-1-10") === nameToAttr("t2.k-1-5")))
// this can fail
Optimize.execute(plan.analyze)
}
```
Besides, with the new rule `DeduplicateRelations`, we'd be able to enable some optimizations, e.g., LeftSemiAnti pushdown, redundant project removal, as reflects in updated unit tests.
### Does this PR introduce _any_ user-facing change?
### How was this patch tested?
Added and updated unit tests.
Closes#31470 from Ngone51/join-reorder.
Lead-authored-by: yi.wu <yi.wu@databricks.com>
Co-authored-by: Wenchen Fan <wenchen@databricks.com>
Signed-off-by: Wenchen Fan <wenchen@databricks.com>
### What changes were proposed in this pull request?
1. Add the SQL config `spark.sql.legacy.interval.enabled` which will control when Spark SQL should use `CalendarIntervalType` instead of ANSI intervals.
2. Modify the `SubtractDates` expression to return values of `DayTimeIntervalType` when `spark.sql.legacy.interval.enabled` is set to `false` (which is the default).
### Why are the changes needed?
To conform to the ANSI SQL standard which requires ANSI intervals as the result of dates subtraction, see
<img width="656" alt="Screenshot 2021-03-29 at 19 09 34" src="https://user-images.githubusercontent.com/1580697/112866455-7e2f0d00-90c2-11eb-96e6-3feb7eea7e09.png">
### Does this PR introduce _any_ user-facing change?
Yes.
### How was this patch tested?
By running new tests:
```
$ build/sbt "test:testOnly *DateExpressionsSuite"
$ build/sbt "test:testOnly *ColumnExpressionSuite"
```
and some tests from `SQLQueryTestSuite`:
```
$ build/sbt "sql/testOnly *SQLQueryTestSuite -- -z date.sql"
$ build/sbt "sql/testOnly *SQLQueryTestSuite -- -z datetime.sql"
$ build/sbt "sql/testOnly *SQLQueryTestSuite -- -z interval.sql"
```
Closes#31996 from MaxGekk/subtract-dates-to-intervals.
Authored-by: Max Gekk <max.gekk@gmail.com>
Signed-off-by: Max Gekk <max.gekk@gmail.com>
### What changes were proposed in this pull request?
Use `java.lang.Long.divideUnsigned()` to do integer division in `NumberConverter` to avoid a bug in `unsignedLongDiv` that produced invalid results.
### Why are the changes needed?
The previous results are incorrect, the result of the below query should be 45012021522523134134555
```
scala> spark.sql("select conv('-10', 11, 7)").show(20, 150)
+-----------------------+
| conv(-10, 11, 7)|
+-----------------------+
|4501202152252313413456|
+-----------------------+
scala> spark.sql("select hex(conv('-10', 11, 7))").show(20, 150)
+----------------------------------------------+
| hex(conv(-10, 11, 7))|
+----------------------------------------------+
|3435303132303231353232353233313334313334353600|
+----------------------------------------------+
```
### Does this PR introduce _any_ user-facing change?
`conv()` will produce different results because the bug is fixed.
### How was this patch tested?
Added a simple unit test.
Closes#32006 from timarmstrong/conv-unsigned.
Authored-by: Tim Armstrong <tim.armstrong@databricks.com>
Signed-off-by: Wenchen Fan <wenchen@databricks.com>
### What changes were proposed in this pull request?
After https://github.com/apache/spark/pull/31954/, Array type is allowed to be cast as String type. So the customized conversion failure message branch from AnsiCast.typeCheckFailureMessage won't be reached anymore.
This PR is to remove the dead code.
### Why are the changes needed?
Code clean up.
### Does this PR introduce _any_ user-facing change?
No
### How was this patch tested?
Just removing dead code.
Closes#32004 from gengliangwang/SPARK-34856-followup.
Authored-by: Gengliang Wang <ltnwgl@gmail.com>
Signed-off-by: Sean Owen <srowen@gmail.com>
### What changes were proposed in this pull request?
Spark query plan node hierarchy has specialized traits (or abstract classes) for handling nodes with fixed number of children, for example `UnaryExpression`, `UnaryNode` and `UnaryExec` for representing an expression, a logical plan and a physical plan with only one child, respectively. This PR refactors the `TreeNode` hierarchy by extracting the children handling functionality into the following traits. `UnaryExpression` and other similar classes now extend the corresponding new trait:
```
trait LeafLike[T <: TreeNode[T]] { self: TreeNode[T] =>
override final def children: Seq[T] = Nil
}
trait UnaryLike[T <: TreeNode[T]] { self: TreeNode[T] =>
def child: T
transient override final lazy val children: Seq[T] = child :: Nil
}
trait BinaryLike[T <: TreeNode[T]] { self: TreeNode[T] =>
def left: T
def right: T
transient override final lazy val children: Seq[T] = left :: right :: Nil
}
trait TernaryLike[T <: TreeNode[T]] { self: TreeNode[T] =>
def first: T
def second: T
def third: T
transient override final lazy val children: Seq[T] = first :: second :: third :: Nil
}
```
This refactoring, which is part of a bigger effort to make tree transformations in Spark more efficient, has two benefits:
- It moves the children handling methods to a single place, instead of being spread in specific subclasses, which will help the future optimizations for tree traversals.
- It allows to mix in these traits with some concrete node types that could not extend the previous classes. For example, expressions with one child that extend `AggregateFunction` cannot extend `UnaryExpression` as `AggregateFunction` defines the `foldable` method final while `UnaryExpression` defines it as non final. With the new traits, we can directly extend the concrete class from `UnaryLike` in these cases. Classes with more specific child handling will make tree traversal methods faster.
In this PR we have also updated many concrete node types to extend these traits to benefit from more specific child handling.
### Why are the changes needed?
### Does this PR introduce _any_ user-facing change?
### How was this patch tested?
This is a refactoring, passes existing tests.
Closes#31932 from dbaliafroozeh/FactorOutChildHandlnigIntoSeparateTraits.
Authored-by: Ali Afroozeh <ali.afroozeh@databricks.com>
Signed-off-by: herman <herman@databricks.com>
### What changes were proposed in this pull request?
As discussed in
https://github.com/apache/spark/pull/30145#discussion_r514728642https://github.com/apache/spark/pull/30145#discussion_r514734648
We need to rewrite current Grouping Analytics grammar to support as flexible as Postgres SQL to support subsequent development.
In postgres sql, it support
```
select a, b, c, count(1) from t group by cube (a, b, c);
select a, b, c, count(1) from t group by cube(a, b, c);
select a, b, c, count(1) from t group by cube (a, b, c, (a, b), (a, b, c));
select a, b, c, count(1) from t group by rollup(a, b, c);
select a, b, c, count(1) from t group by rollup (a, b, c);
select a, b, c, count(1) from t group by rollup (a, b, c, (a, b), (a, b, c));
```
In this pr, we have done three things as below, and we will split it to different pr:
- Refactor CUBE/ROLLUP (regarding them as ANTLR tokens in a parser)
- Refactor GROUPING SETS (the logical node -> a new expr)
- Support new syntax for CUBE/ROLLUP (e.g., GROUP BY CUBE ((a, b), (a, c)))
### Why are the changes needed?
Rewrite current Grouping Analytics grammar to support as flexible as Postgres SQL to support subsequent development.
### Does this PR introduce _any_ user-facing change?
User can write Grouping Analytics grammar as flexible as Postgres SQL to support subsequent development.
### How was this patch tested?
Added UT
Closes#30212 from AngersZhuuuu/refact-grouping-analytics.
Lead-authored-by: angerszhu <angers.zhu@gmail.com>
Co-authored-by: Angerszhuuuu <angers.zhu@gmail.com>
Co-authored-by: AngersZhuuuu <angers.zhu@gmail.com>
Co-authored-by: Takeshi Yamamuro <yamamuro@apache.org>
Signed-off-by: Wenchen Fan <wenchen@databricks.com>
### What changes were proposed in this pull request?
As a followup for #29818, document caveats of using the Arrow selfDestruct option in toPandas, which include:
- toPandas() may be slower;
- the resulting dataframe may not support some Pandas operations due to immutable backing arrays.
### Why are the changes needed?
This will hopefully reduce user confusion as with SPARK-34463.
### Does this PR introduce _any_ user-facing change?
Yes - documentation is updated and a config setting description is updated to clearly indicate the config is experimental.
### How was this patch tested?
This is a documentation-only change.
Closes#31738 from lidavidm/spark-34463.
Authored-by: David Li <li.davidm96@gmail.com>
Signed-off-by: HyukjinKwon <gurwls223@apache.org>
### What changes were proposed in this pull request?
Some `spark-submit` commands used to run benchmarks in the user's guide is wrong, we can't use these commands to run benchmarks successful.
So the major changes of this pr is correct these wrong commands, for example, run a benchmark which inherits from `SqlBasedBenchmark`, we must specify `--jars <spark core test jar>,<spark catalyst test jar>` because `SqlBasedBenchmark` based benchmark extends `BenchmarkBase(defined in spark core test jar)` and `SQLHelper(defined in spark catalyst test jar)`.
Another change of this pr is removed the `scalatest Assertions` dependency of Benchmarks because `scalatest-*.jar` are not in the distribution package, it will be troublesome to use.
### Why are the changes needed?
Make sure benchmarks can run using spark-submit cmd described in the guide
### Does this PR introduce _any_ user-facing change?
No.
### How was this patch tested?
Use the corrected `spark-submit` commands to run benchmarks successfully.
Closes#31995 from LuciferYang/fix-benchmark-guide.
Authored-by: yangjie01 <yangjie01@baidu.com>
Signed-off-by: HyukjinKwon <gurwls223@apache.org>
### What changes were proposed in this pull request?
There is a `Project` between `LocalLimit` and `Join` if `Join`'s output do not match the `LocalLimit`'s output. This pr add support push down limit through this case. For example:
```scala
spark.sql("create table t1(a int, b int, c int) using parquet")
spark.sql("create table t2(x int, y int, z int) using parquet")
spark.sql("select a from t1 left join t2 on a = x and b = y limit 5").explain("extended")
```
```
== Optimized Logical Plan ==
GlobalLimit 5
+- LocalLimit 5
+- Project [a#0]
+- Join LeftOuter, ((a#0 = x#3) AND (b#1 = y#4))
:- Project [a#0, b#1]
: +- Relation default.t1[a#0,b#1,c#2] parquet
+- Project [x#3, y#4]
+- Filter (isnotnull(x#3) AND isnotnull(y#4))
+- Relation default.t2[x#3,y#4,z#5] parquet
```
After this pr:
```
== Optimized Logical Plan ==
GlobalLimit 5
+- LocalLimit 5
+- Project [a#0]
+- Join LeftOuter, ((a#0 = x#3) AND (b#1 = y#4))
:- LocalLimit 5
: +- Project [a#0, b#1]
: +- Relation default.t1[a#0,b#1,c#2] parquet
+- Project [x#3, y#4]
+- Filter (isnotnull(x#3) AND isnotnull(y#4))
+- Relation default.t2[x#3,y#4,z#5] parquet
```
### Why are the changes needed?
Improve limit push down to improve query performance.
### Does this PR introduce _any_ user-facing change?
No.
### How was this patch tested?
Unit test.
Closes#31739 from wangyum/SPARK-34622.
Authored-by: Yuming Wang <yumwang@ebay.com>
Signed-off-by: Takeshi Yamamuro <yamamuro@apache.org>
### What changes were proposed in this pull request?
This PR proposes to extend the functionality of requirement for distribution and ordering on V2 write to specify the number of partitioning on repartition, so that data source is able to control the parallelism and determine the data distribution per partition in prior.
The partitioning with static number is optional, and by default disabled via default method, so only implementations required to restrict the number of partition statically need to override the method and provide the number.
Note that we don't support static number of partitions with unspecified distribution for this PR, as we haven't found the real use cases, and for hypothetical case the static number isn't good enough. Javadoc clearly describes the limitation.
### Why are the changes needed?
The use case comes from feature parity with DSv1.
I have state data source which enables the state in SS to be rewritten, which enables repartitioning, schema evolution, etc via batch query. The writer requires hash partitioning against group key, with the "desired number of partitions", which is same as what Spark does read and write against state.
This is now implemented as DSv1, and the requirement is simply done by calling repartition with the "desired number".
```
val fullPathsForKeyColumns = keySchema.map(key => new Column(s"key.${key.name}"))
data
.repartition(newPartitions, fullPathsForKeyColumns: _*)
.queryExecution
.toRdd
.foreachPartition(
writeFn(resolvedCpLocation, version, operatorId, storeName, keySchema, valueSchema,
storeConf, hadoopConfBroadcast, queryId))
```
Thanks to SPARK-34026, it's now possible to require the hash partitioning, but still not able to require the number of partitions. This PR will enable to let data source require the number of partitions.
### Does this PR introduce _any_ user-facing change?
Yes, but only for data source implementors. Even for them, this is no breaking change as default method is added.
### How was this patch tested?
Added UTs.
Closes#31355 from HeartSaVioR/SPARK-34255.
Lead-authored-by: Jungtaek Lim <kabhwan.opensource@gmail.com>
Co-authored-by: Jungtaek Lim (HeartSaVioR) <kabhwan.opensource@gmail.com>
Signed-off-by: Wenchen Fan <wenchen@databricks.com>
### What changes were proposed in this pull request?
This PR fixes an issue that `quoteIfNeeded` quotes a name only if it contains `.` or ``` ` ```.
This method should quote it if it contains non-word characters.
### Why are the changes needed?
It's a potential bug.
### Does this PR introduce _any_ user-facing change?
No.
### How was this patch tested?
New test.
Closes#31964 from sarutak/fix-quoteIfNeeded.
Authored-by: Kousuke Saruta <sarutak@oss.nttdata.com>
Signed-off-by: Wenchen Fan <wenchen@databricks.com>
### What changes were proposed in this pull request?
LikeSimplification should handle NULL.
UT will failed before this pr
```
test("SPARK-34814: LikeSimplification should handle NULL") {
withSQLConf(SQLConf.OPTIMIZER_EXCLUDED_RULES.key ->
ConstantFolding.getClass.getName.stripSuffix("$")) {
checkEvaluation(Literal.create("foo", StringType)
.likeAll("%foo%", Literal.create(null, StringType)), null)
}
}
[info] - test *** FAILED *** (2 seconds, 443 milliseconds)
[info] java.lang.NullPointerException:
[info] at org.apache.spark.sql.catalyst.optimizer.LikeSimplification$.$anonfun$simplifyMultiLike$1(expressions.scala:697)
[info] at scala.collection.TraversableLike.$anonfun$map$1(TraversableLike.scala:238)
[info] at scala.collection.mutable.ResizableArray.foreach(ResizableArray.scala:62)
[info] at scala.collection.mutable.ResizableArray.foreach$(ResizableArray.scala:55)
[info] at scala.collection.mutable.ArrayBuffer.foreach(ArrayBuffer.scala:49)
[info] at scala.collection.TraversableLike.map(TraversableLike.scala:238)
[info] at scala.collection.TraversableLike.map$(TraversableLike.scala:231)
[info] at scala.collection.AbstractTraversable.map(Traversable.scala:108)
[info] at org.apache.spark.sql.catalyst.optimizer.LikeSimplification$.org$apache$spark$sql$catalyst$optimizer$LikeSimplification$$simplifyMultiLike(expressions.scala:697)
[info] at org.apache.spark.sql.catalyst.optimizer.LikeSimplification$$anonfun$apply$9.applyOrElse(expressions.scala:722)
[info] at org.apache.spark.sql.catalyst.optimizer.LikeSimplification$$anonfun$apply$9.applyOrElse(expressions.scala:714)
[info] at org.apache.spark.sql.catalyst.trees.TreeNode.$anonfun$transformDown$1(TreeNode.scala:316)
[info] at org.apache.spark.sql.catalyst.trees.CurrentOrigin$.withOrigin(TreeNode.scala:72)
[info] at org.apache.spark.sql.catalyst.trees.TreeNode.transformDown(TreeNode.scala:316)
[info] at org.apache.spark.sql.catalyst.trees.TreeNode.$anonfun$transformDown$3(TreeNode.scala:321)
[info] at org.apache.spark.sql.catalyst.trees.TreeNode.$anonfun$mapChildren$1(TreeNode.scala:406)
[info] at org.apache.spark.sql.catalyst.trees.TreeNode.mapProductIterator(TreeNode.scala:242)
[info] at org.apache.spark.sql.catalyst.trees.TreeNode.mapChildren(TreeNode.scala:404)
[info] at org.apache.spark.sql.catalyst.trees.TreeNode.mapChildren(TreeNode.scala:357)
[info] at org.apache.spark.sql.catalyst.trees.TreeNode.transformDown(TreeNode.scala:321)
[info] at org.apache.spark.sql.catalyst.plans.QueryPlan.$anonfun$transformExpressionsDown$1(QueryPlan.scala:94)
[info] at org.apache.spark.sql.catalyst.plans.QueryPlan.$anonfun$mapExpressions$1(QueryPlan.scala:116)
[info] at org.apache.spark.sql.catalyst.trees.CurrentOrigin$.withOrigin(TreeNode.scala:72)
```
### Why are the changes needed?
Fix bug
### Does this PR introduce _any_ user-facing change?
No
### How was this patch tested?
Added UT
Closes#31976 from AngersZhuuuu/SPARK-34814.
Authored-by: Angerszhuuuu <angers.zhu@gmail.com>
Signed-off-by: HyukjinKwon <gurwls223@apache.org>
### What changes were proposed in this pull request?
Filled the `defaultResult` field on non-nullable aggregates
### Why are the changes needed?
The `defaultResult` defaults to `None` and in some situations (like correlated scalar subqueries) it is used for the value of the aggregation.
The UT result before the fix:
```
-- !query
SELECT t1a,
(SELECT count(t2d) FROM t2 WHERE t2a = t1a) count_t2,
(SELECT count_if(t2d > 0) FROM t2 WHERE t2a = t1a) count_if_t2,
(SELECT approx_count_distinct(t2d) FROM t2 WHERE t2a = t1a) approx_count_distinct_t2,
(SELECT collect_list(t2d) FROM t2 WHERE t2a = t1a) collect_list_t2,
(SELECT collect_set(t2d) FROM t2 WHERE t2a = t1a) collect_set_t2,
(SELECT hex(count_min_sketch(t2d, 0.5d, 0.5d, 1)) FROM t2 WHERE t2a = t1a) collect_set_t2
FROM t1
-- !query schema
struct<t1a:string,count_t2:bigint,count_if_t2:bigint,approx_count_distinct_t2:bigint,collect_list_t2:array<bigint>,collect_set_t2:array<bigint>,collect_set_t2:string>
-- !query output
val1a 0 0 NULL NULL NULL NULL
val1a 0 0 NULL NULL NULL NULL
val1a 0 0 NULL NULL NULL NULL
val1a 0 0 NULL NULL NULL NULL
val1b 6 6 3 [19,119,319,19,19,19] [19,119,319] 0000000100000000000000060000000100000004000000005D8D6AB90000000000000000000000000000000400000000000000010000000000000001
val1c 2 2 2 [219,19] [219,19] 0000000100000000000000020000000100000004000000005D8D6AB90000000000000000000000000000000100000000000000000000000000000001
val1d 0 0 NULL NULL NULL NULL
val1d 0 0 NULL NULL NULL NULL
val1d 0 0 NULL NULL NULL NULL
val1e 1 1 1 [19] [19] 0000000100000000000000010000000100000004000000005D8D6AB90000000000000000000000000000000100000000000000000000000000000000
val1e 1 1 1 [19] [19] 0000000100000000000000010000000100000004000000005D8D6AB90000000000000000000000000000000100000000000000000000000000000000
val1e 1 1 1 [19] [19] 0000000100000000000000010000000100000004000000005D8D6AB90000000000000000000000000000000100000000000000000000000000000000
```
### Does this PR introduce _any_ user-facing change?
Bugfix
### How was this patch tested?
UT
Closes#31973 from tanelk/SPARK-34876_non_nullable_agg_subquery.
Authored-by: Tanel Kiis <tanel.kiis@gmail.com>
Signed-off-by: HyukjinKwon <gurwls223@apache.org>
### What changes were proposed in this pull request?
This PR fixes a correctness issue with higher order functions. The results of function expressions needs to be copied in some higher order functions as such an expression can return with internal buffers and higher order functions can call multiple times the expression.
The issue was discovered with typed `ScalaUDF`s after https://github.com/apache/spark/pull/28979.
### Why are the changes needed?
To fix a bug.
### Does this PR introduce _any_ user-facing change?
Yes, some queries return the right results again.
### How was this patch tested?
Added new UT.
Closes#31955 from peter-toth/SPARK-34829-fix-scalaudf-resultconversion.
Authored-by: Peter Toth <peter.toth@gmail.com>
Signed-off-by: Dongjoon Hyun <dhyun@apple.com>
### What changes were proposed in this pull request?
Fix code format in `SQLConf` and comment in `PartitionPruning`.
### Why are the changes needed?
Make code more readable.
### Does this PR introduce _any_ user-facing change?
No.
### How was this patch tested?
N/A
Closes#31969 from wangyum/SPARK-32855-2.
Authored-by: Yuming Wang <yumwang@ebay.com>
Signed-off-by: Dongjoon Hyun <dhyun@apple.com>
### What changes were proposed in this pull request?
Push ANSI interval binary expressions into into (if / case) branches
### Why are the changes needed?
Support more binary expression to push into if/else and casewhen
### Does this PR introduce _any_ user-facing change?
No
### How was this patch tested?
Added UT
Closes#31978 from AngersZhuuuu/SPARK-34841.
Authored-by: Angerszhuuuu <angers.zhu@gmail.com>
Signed-off-by: Max Gekk <max.gekk@gmail.com>
### What changes were proposed in this pull request?
Improve error message for casting cause overflow error. We should use DataType's catalogString.
### Why are the changes needed?
Improve error message
### Does this PR introduce _any_ user-facing change?
For example:
```
set spark.sql.ansi.enabled=true;
select tinyint(128) * tinyint(2);
```
Error message before this pr:
```
Casting 128 to scala.Byte$ causes overflow
```
After this pr:
```
Casting 128 to tinyint causes overflow
```
### How was this patch tested?
Added UT
Closes#31971 from AngersZhuuuu/SPARK-34744.
Authored-by: Angerszhuuuu <angers.zhu@gmail.com>
Signed-off-by: Kent Yao <yao@apache.org>
### What changes were proposed in this pull request?
1. Add new expression `DivideDTInterval` which multiplies a `DayTimeIntervalType` expression by a `NumericType` expression including ByteType, ShortType, IntegerType, LongType, FloatType, DoubleType, DecimalType.
2. Extend binary arithmetic rules to support `day-time interval / numeric`.
### Why are the changes needed?
To conform the ANSI SQL standard which requires such operation over day-time intervals:
<img width="656" alt="Screenshot 2021-03-25 at 18 44 58" src="https://user-images.githubusercontent.com/1580697/112501559-68f07080-8d9a-11eb-8781-66e6631bb7ef.png">
### Does this PR introduce _any_ user-facing change?
No
### How was this patch tested?
By running new tests:
```
$ build/sbt "test:testOnly *IntervalExpressionsSuite"
$ build/sbt "test:testOnly *ColumnExpressionSuite"
```
Closes#31972 from MaxGekk/div-dt-interval-by-num.
Authored-by: Max Gekk <max.gekk@gmail.com>
Signed-off-by: Wenchen Fan <wenchen@databricks.com>
### What changes were proposed in this pull request?
1. Add new expression `DivideYMInterval` which multiplies a `YearMonthIntervalType` expression by a `NumericType` expression including ByteType, ShortType, IntegerType, LongType, FloatType, DoubleType, DecimalType.
2. Extend binary arithmetic rules to support `year-month interval / numeric`.
### Why are the changes needed?
To conform the ANSI SQL standard which requires such operation over year-month intervals:
<img width="656" alt="Screenshot 2021-03-25 at 18 44 58" src="https://user-images.githubusercontent.com/1580697/112501559-68f07080-8d9a-11eb-8781-66e6631bb7ef.png">
### Does this PR introduce _any_ user-facing change?
No
### How was this patch tested?
By running new tests:
```
$ build/sbt "test:testOnly *IntervalExpressionsSuite"
$ build/sbt "test:testOnly *ColumnExpressionSuite"
```
Closes#31961 from MaxGekk/div-ym-interval-by-num.
Authored-by: Max Gekk <max.gekk@gmail.com>
Signed-off-by: Wenchen Fan <wenchen@databricks.com>
### What changes were proposed in this pull request?
This pr improve the cost model in `pruningHasBenefit` for filtering side can not build broadcast by join type:
1. The filtering side must be small enough to build broadcast by size.
2. The estimated size of the pruning side must be big enough: `estimatePruningSideSize * spark.sql.optimizer.dynamicPartitionPruning.pruningSideExtraFilterRatio > overhead`.
### Why are the changes needed?
Improve query performance for these cases.
This a real case from cluster. Left join and left size very small and right side can build DPP:
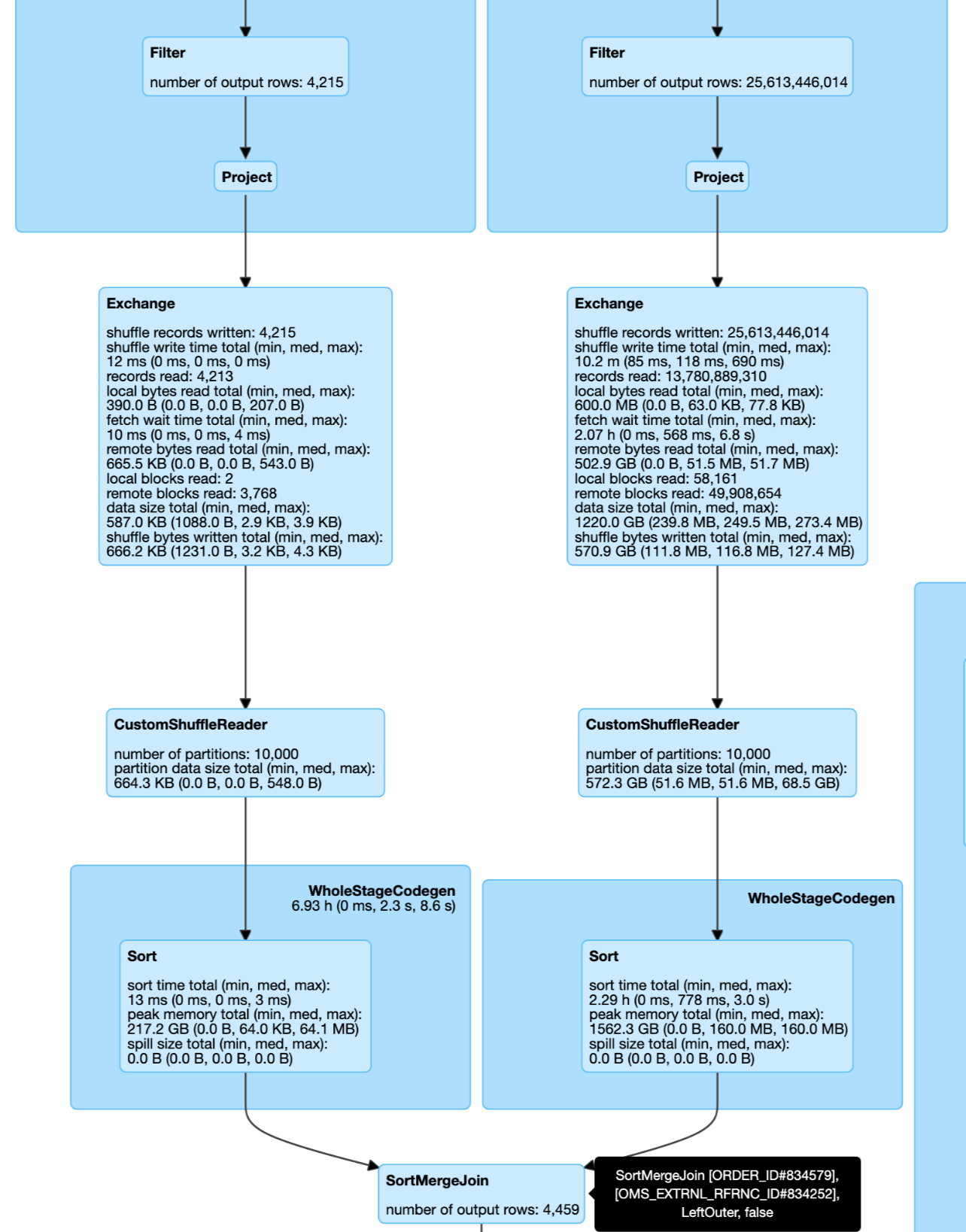
### Does this PR introduce _any_ user-facing change?
No.
### How was this patch tested?
Unit test.
Closes#29726 from wangyum/SPARK-32855.
Authored-by: Yuming Wang <yumwang@ebay.com>
Signed-off-by: Wenchen Fan <wenchen@databricks.com>
### What changes were proposed in this pull request?
A companion PR for SPARK-34817, when we handle the unsigned int(<=32) logical types. In this PR, we map the unsigned int64 to decimal(20, 0) for better compatibility.
### Why are the changes needed?
Spark won't have unsigned types, but spark should be able to read existing parquet files written by other systems that support unsigned types for better compatibility.
### Does this PR introduce _any_ user-facing change?
yes, we can read parquet uint64 now
### How was this patch tested?
new unit tests
Closes#31960 from yaooqinn/SPARK-34786-2.
Authored-by: Kent Yao <yao@apache.org>
Signed-off-by: Kent Yao <yao@apache.org>
### What changes were proposed in this pull request?
Move the checkpoint location resolving into the rule ResolveWriteToStream, which is added in SPARK-34748.
### Why are the changes needed?
After SPARK-34748, we have a rule ResolveWriteToStream for the analysis logic for the resolving logic of stream write plans. Based on it, we can further move the checkpoint location resolving work in the rule. Then, all the checkpoint resolving logic was done in the analyzer.
### Does this PR introduce _any_ user-facing change?
No.
### How was this patch tested?
Existing UT.
Closes#31963 from xuanyuanking/SPARK-34871.
Authored-by: Yuanjian Li <yuanjian.li@databricks.com>
Signed-off-by: HyukjinKwon <gurwls223@apache.org>
### What changes were proposed in this pull request?
This is a follow-up of https://github.com/apache/spark/pull/31940 . This PR generalizes the matching of attributes and outer references, so that outer references are handled everywhere.
Note that, currently correlated subquery has a lot of limitations in Spark, and the newly covered cases are not possible to happen. So this PR is a code refactor.
### Why are the changes needed?
code cleanup
### Does this PR introduce _any_ user-facing change?
no
### How was this patch tested?
existing tests
Closes#31959 from cloud-fan/follow.
Authored-by: Wenchen Fan <wenchen@databricks.com>
Signed-off-by: Takeshi Yamamuro <yamamuro@apache.org>
### What changes were proposed in this pull request?
Allow casting complex types as string type in ANSI mode.
### Why are the changes needed?
Currently, complex types are not allowed to cast as string type. This breaks the DataFrame.show() API. E.g
```
scala> sql(“select array(1, 2, 2)“).show(false)
org.apache.spark.sql.AnalysisException: cannot resolve ‘CAST(`array(1, 2, 2)` AS STRING)’ due to data type mismatch:
cannot cast array<int> to string with ANSI mode on.
```
We should allow the conversion as the extension of the ANSI SQL standard, so that the DataFrame.show() still work in ANSI mode.
### Does this PR introduce _any_ user-facing change?
Yes, casting complex types as string type is now allowed in ANSI mode.
### How was this patch tested?
Unit tests.
Closes#31954 from gengliangwang/fixExplicitCast.
Authored-by: Gengliang Wang <ltnwgl@gmail.com>
Signed-off-by: Gengliang Wang <ltnwgl@gmail.com>
### What changes were proposed in this pull request?
This PR groups exception messages in `execution/datasources/v2`.
### Why are the changes needed?
It will largely help with standardization of error messages and its maintenance.
### Does this PR introduce _any_ user-facing change?
No. Error messages remain unchanged.
### How was this patch tested?
No new tests - pass all original tests to make sure it doesn't break any existing behavior.
Closes#31619 from karenfeng/spark-33600.
Authored-by: Karen Feng <karen.feng@databricks.com>
Signed-off-by: Wenchen Fan <wenchen@databricks.com>
### What changes were proposed in this pull request?
Removed the custom toString implementation of AtLeastNNoneNulls.
### Why are the changes needed?
It shows up wrong in the explain plan. The name of the function is wrong and the actual value of the first argument is not shown. Both of these would make it easier to understand the plan.
```
(12) Filter
Input [3]: [c1#2410L, c2#2419, c3#2422]
Condition : AtLeastNNulls(n, c1#2410L)
```
### Does this PR introduce _any_ user-facing change?
Only the explain plan changes if this function is used.
### How was this patch tested?
Added a simple unit test to make sure that the toString output is correct.
Closes#31956 from timarmstrong/atleastnnonnulls.
Authored-by: Tim Armstrong <tim.armstrong@databricks.com>
Signed-off-by: HyukjinKwon <gurwls223@apache.org>
### What changes were proposed in this pull request?
1. Add new expression `MultiplyDTInterval` which multiplies a `DayTimeIntervalType` expression by a `NumericType` expression including ByteType, ShortType, IntegerType, LongType, FloatType, DoubleType, DecimalType.
2. Extend binary arithmetic rules to support `numeric * day-time interval` and `day-time interval * numeric`.
3. Invoke `DoubleMath.roundToInt` in `double/float * year-month interval`.
### Why are the changes needed?
To conform the ANSI SQL standard which requires such operation over day-time intervals:
<img width="667" alt="Screenshot 2021-03-22 at 16 33 16" src="https://user-images.githubusercontent.com/1580697/111997810-77d1eb80-8b2c-11eb-951d-e43911d9c5db.png">
### Does this PR introduce _any_ user-facing change?
No
### How was this patch tested?
By running new tests:
```
$ build/sbt "test:testOnly *IntervalExpressionsSuite"
$ build/sbt "test:testOnly *ColumnExpressionSuite"
```
Closes#31951 from MaxGekk/mul-day-time-interval.
Authored-by: Max Gekk <max.gekk@gmail.com>
Signed-off-by: Max Gekk <max.gekk@gmail.com>
### What changes were proposed in this pull request?
This PR proposes to remove re-analyzing the already analyzed plan for `CreateViewCommand` as discussed https://github.com/apache/spark/pull/31273/files#r581592786.
### Why are the changes needed?
No need to analyze the plan if it's already analyzed.
### Does this PR introduce _any_ user-facing change?
No.
### How was this patch tested?
Existing tests should cover this.
Closes#31933 from imback82/remove_analyzed_from_create_temp_view.
Authored-by: Terry Kim <yuminkim@gmail.com>
Signed-off-by: Wenchen Fan <wenchen@databricks.com>
### What changes were proposed in this pull request?
This PR intends to fix the bug that does not apply right-padding for char types inside correlated subquries.
For example, a query below returns nothing in master, but a correct result is `c`.
```
scala> sql(s"CREATE TABLE t1(v VARCHAR(3), c CHAR(5)) USING parquet")
scala> sql(s"CREATE TABLE t2(v VARCHAR(5), c CHAR(7)) USING parquet")
scala> sql("INSERT INTO t1 VALUES ('c', 'b')")
scala> sql("INSERT INTO t2 VALUES ('a', 'b')")
scala> val df = sql("""
|SELECT v FROM t1
|WHERE 'a' IN (SELECT v FROM t2 WHERE t2.c = t1.c )""".stripMargin)
scala> df.show()
+---+
| v|
+---+
+---+
```
This is because `ApplyCharTypePadding` does not handle the case above to apply right-padding into `'abc'`. This PR modifies the code in `ApplyCharTypePadding` for handling it correctly.
```
// Before this PR:
scala> df.explain(true)
== Analyzed Logical Plan ==
v: string
Project [v#13]
+- Filter a IN (list#12 [c#14])
: +- Project [v#15]
: +- Filter (c#16 = outer(c#14))
: +- SubqueryAlias spark_catalog.default.t2
: +- Relation default.t2[v#15,c#16] parquet
+- SubqueryAlias spark_catalog.default.t1
+- Relation default.t1[v#13,c#14] parquet
scala> df.show()
+---+
| v|
+---+
+---+
// After this PR:
scala> df.explain(true)
== Analyzed Logical Plan ==
v: string
Project [v#43]
+- Filter a IN (list#42 [c#44])
: +- Project [v#45]
: +- Filter (c#46 = rpad(outer(c#44), 7, ))
: +- SubqueryAlias spark_catalog.default.t2
: +- Relation default.t2[v#45,c#46] parquet
+- SubqueryAlias spark_catalog.default.t1
+- Relation default.t1[v#43,c#44] parquet
scala> df.show()
+---+
| v|
+---+
| c|
+---+
```
This fix is lated to TPCDS q17; the query returns nothing because of this bug: https://github.com/apache/spark/pull/31886/files#r599333799
### Why are the changes needed?
Bugfix.
### Does this PR introduce _any_ user-facing change?
No.
### How was this patch tested?
Unit tests added.
Closes#31940 from maropu/FixCharPadding.
Authored-by: Takeshi Yamamuro <yamamuro@apache.org>
Signed-off-by: Takeshi Yamamuro <yamamuro@apache.org>
### What changes were proposed in this pull request?
Currently, when implicit casting a data type to a `TypeCollection`, Spark returns the first convertible data type among `TypeCollection`.
In ANSI mode, we can make the behavior more reasonable by returning the closet convertible data type in `TypeCollection`.
In details, we first try to find the all the expected types we can implicitly cast:
1. if there is no convertible data types, return None;
2. if there is only one convertible data type, cast input as it;
3. otherwise if there are multiple convertible data types, find the closet data
type among them. If there is no such closet data type, return None.
Note that if the closet type is Float type and the convertible types contains Double type, simply return Double type as the closet type to avoid potential
precision loss on converting the Integral type as Float type.
### Why are the changes needed?
Make the type coercion rule for TypeCollection more reasonable and ANSI compatible.
E.g. returning Long instead of Double for`implicast(int, TypeCollect(Double, Long))`.
From ANSI SQL Spec section 4.33 "SQL-invoked routines"
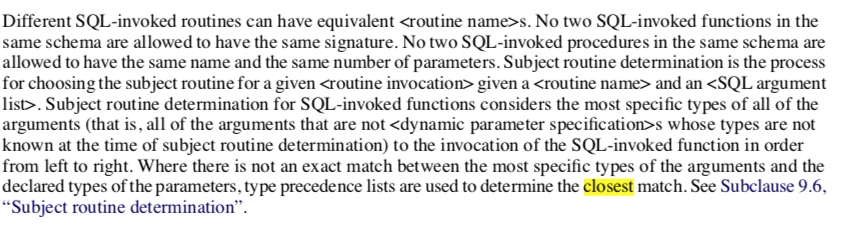
Section 9.6 "Subject routine determination"
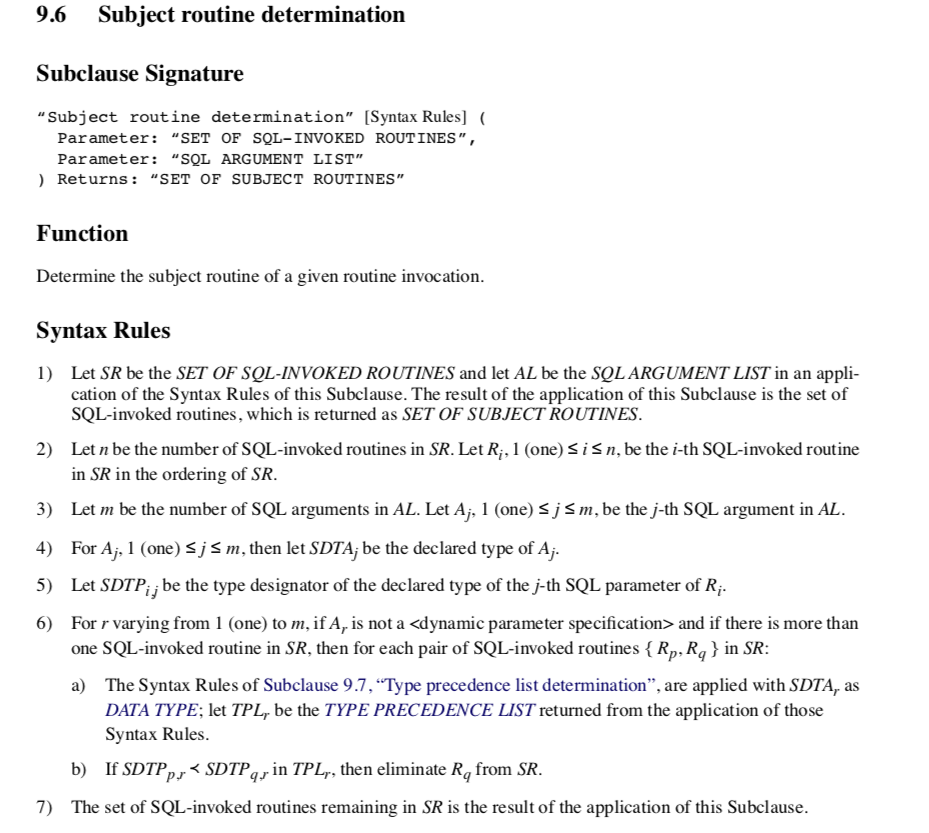
Section 10.4 "routine invocation"
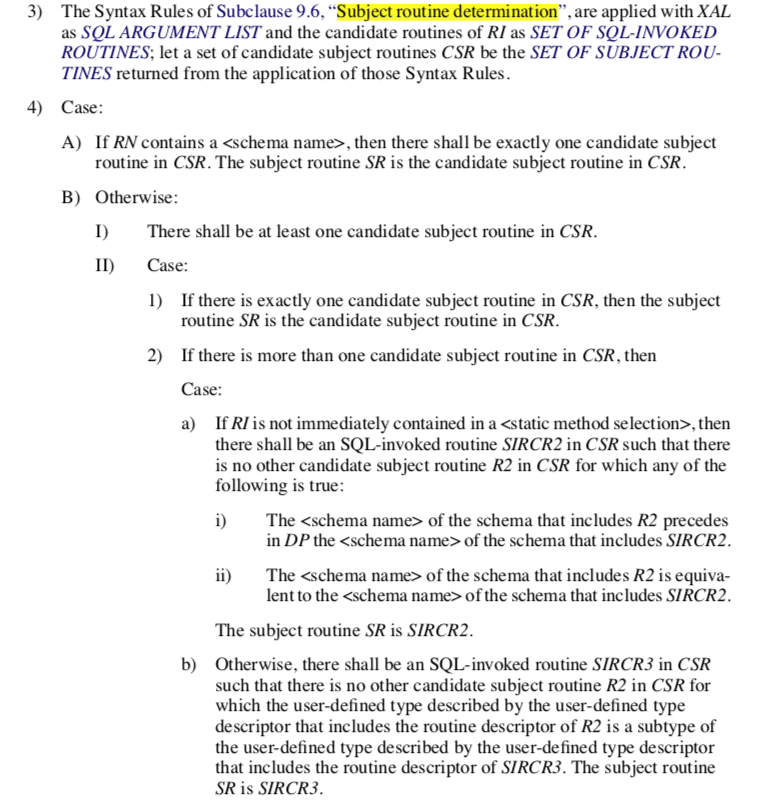
### Does this PR introduce _any_ user-facing change?
Yes, in ANSI mode, implicit casting to a `TypeCollection` returns the narrowest convertible data type instead of the first convertible one.
### How was this patch tested?
Unit tests.
Closes#31859 from gengliangwang/implicitCastTypeCollection.
Lead-authored-by: Gengliang Wang <gengliang.wang@databricks.com>
Co-authored-by: Gengliang Wang <ltnwgl@gmail.com>
Signed-off-by: Wenchen Fan <wenchen@databricks.com>
### What changes were proposed in this pull request?
This PR fixes an issue that `col()`, `$"<name>"` and `df("name")` don't handle quoted column names like ``` `a``b.c` ```properly.
For example, if we have a following DataFrame.
```
val df1 = spark.sql("SELECT 'col1' AS `a``b.c`")
```
For the DataFrame, this query is successfully executed.
```
scala> df1.selectExpr("`a``b.c`").show
+-----+
|a`b.c|
+-----+
| col1|
+-----+
```
But the following query will fail because ``` df1("`a``b.c`") ``` throws an exception.
```
scala> df1.select(df1("`a``b.c`")).show
org.apache.spark.sql.AnalysisException: syntax error in attribute name: `a``b.c`;
at org.apache.spark.sql.catalyst.analysis.UnresolvedAttribute$.e$1(unresolved.scala:152)
at org.apache.spark.sql.catalyst.analysis.UnresolvedAttribute$.parseAttributeName(unresolved.scala:162)
at org.apache.spark.sql.catalyst.plans.logical.LogicalPlan.resolveQuoted(LogicalPlan.scala:121)
at org.apache.spark.sql.Dataset.resolve(Dataset.scala:221)
at org.apache.spark.sql.Dataset.col(Dataset.scala:1274)
at org.apache.spark.sql.Dataset.apply(Dataset.scala:1241)
... 49 elided
```
### Why are the changes needed?
It's a bug.
### Does this PR introduce _any_ user-facing change?
No.
### How was this patch tested?
New tests.
Closes#31854 from sarutak/fix-parseAttributeName.
Authored-by: Kousuke Saruta <sarutak@oss.nttdata.com>
Signed-off-by: Wenchen Fan <wenchen@databricks.com>
### What changes were proposed in this pull request?
1. Add new expression `MultiplyYMInterval` which multiplies a `YearMonthIntervalType` expression by a `NumericType` expression including ByteType, ShortType, IntegerType, LongType, FloatType, DoubleType, DecimalType.
2. Extend binary arithmetic rules to support `numeric * year-month interval` and `year-month interval * numeric`.
### Why are the changes needed?
To conform the ANSI SQL standard which requires such operation over year-month intervals:
<img width="667" alt="Screenshot 2021-03-22 at 16 33 16" src="https://user-images.githubusercontent.com/1580697/111997810-77d1eb80-8b2c-11eb-951d-e43911d9c5db.png">
### Does this PR introduce _any_ user-facing change?
No
### How was this patch tested?
By running new tests:
```
$ build/sbt "test:testOnly *IntervalExpressionsSuite"
$ build/sbt "test:testOnly *ColumnExpressionSuite"
```
Closes#31929 from MaxGekk/interval-mul-div.
Authored-by: Max Gekk <max.gekk@gmail.com>
Signed-off-by: Max Gekk <max.gekk@gmail.com>
forward-port https://github.com/apache/spark/pull/31811 to master
### What changes were proposed in this pull request?
For permanent views (and the new SQL temp view in Spark 3.1), we store the view SQL text and re-parse/analyze the view SQL text when reading the view. In the case of `SELECT * FROM ...`, we want to avoid view schema change (e.g. the referenced table changes its schema) and will record the view query output column names when creating the view, so that when reading the view we can add a `SELECT recorded_column_names FROM ...` to retain the original view query schema.
In Spark 3.1 and before, the final SELECT is added after the analysis phase: https://github.com/apache/spark/blob/branch-3.1/sql/catalyst/src/main/scala/org/apache/spark/sql/catalyst/analysis/view.scala#L67
If the view query has duplicated output column names, we always pick the first column when reading a view. A simple repro:
```
scala> sql("create view c(x, y) as select 1 a, 2 a")
res0: org.apache.spark.sql.DataFrame = []
scala> sql("select * from c").show
+---+---+
| x| y|
+---+---+
| 1| 1|
+---+---+
```
In the master branch, we will fail at the view reading time due to b891862fb6 , which adds the final SELECT during analysis, so that the query fails with `Reference 'a' is ambiguous`
This PR proposes to resolve the view query output column names from the matching attributes by ordinal.
For example, `create view c(x, y) as select 1 a, 2 a`, the view query output column names are `[a, a]`. When we reading the view, there are 2 matching attributes (e.g.`[a#1, a#2]`) and we can simply match them by ordinal.
A negative example is
```
create table t(a int)
create view v as select *, 1 as col from t
replace table t(a int, col int)
```
When reading the view, the view query output column names are `[a, col]`, and there are two matching attributes of `col`, and we should fail the query. See the tests for details.
### Why are the changes needed?
bug fix
### Does this PR introduce _any_ user-facing change?
yes
### How was this patch tested?
new test
Closes#31930 from cloud-fan/view2.
Authored-by: Wenchen Fan <wenchen@databricks.com>
Signed-off-by: Wenchen Fan <wenchen@databricks.com>
### What changes were proposed in this pull request?
This patch proposes to add a few public API change to DS v2, to make DS v2 scan can report metrics to Spark.
Two public interfaces are added.
* `CustomMetric`: metric interface at the driver side. It basically defines how Spark aggregates task metrics with the same metric name.
* `CustomTaskMetric`: task metric reported at executors. It includes a name and long value. Spark will collect these metric values and update internal metrics.
There are two public methods added to existing public interfaces. They are optional to DS v2 implementations.
* `PartitionReader.currentMetricsValues()`: returns an array of CustomTaskMetric. Here is where the actual metrics values are collected. Empty array by default.
* `Scan.supportedCustomMetrics()`: returns an array of supported custom metrics `CustomMetric`. Empty array by default.
### Why are the changes needed?
In order to report custom metrics, we need some public API change in DS v2 to make it possible.
### Does this PR introduce _any_ user-facing change?
No
### How was this patch tested?
This only adds interfaces. In follow-up PRs where adding implementation there will be tests added. See #31451 and #31398 for some details and manual test there.
Closes#31476 from viirya/SPARK-34366.
Authored-by: Liang-Chi Hsieh <viirya@gmail.com>
Signed-off-by: Wenchen Fan <wenchen@databricks.com>
### What changes were proposed in this pull request?
This patch proposes to disable fetching shuffle blocks in batch when io encryption is enabled. Adaptive Query Execution fetch contiguous shuffle blocks for the same map task in batch to reduce IO and improve performance. However, we found that batch fetching is incompatible with io encryption.
### Why are the changes needed?
Before this patch, we set `spark.io.encryption.enabled` to true, then run some queries which coalesced partitions by AEQ, may got following error message:
```14:05:52.638 WARN org.apache.spark.scheduler.TaskSetManager: Lost task 1.0 in stage 2.0 (TID 3) (11.240.37.88 executor driver): FetchFailed(BlockManagerId(driver, 11.240.37.88, 63574, None), shuffleId=0, mapIndex=0, mapId=0, reduceId=2, message=
org.apache.spark.shuffle.FetchFailedException: Stream is corrupted
at org.apache.spark.storage.ShuffleBlockFetcherIterator.throwFetchFailedException(ShuffleBlockFetcherIterator.scala:772)
at org.apache.spark.storage.BufferReleasingInputStream.read(ShuffleBlockFetcherIterator.scala:845)
at java.io.BufferedInputStream.fill(BufferedInputStream.java:246)
at java.io.BufferedInputStream.read(BufferedInputStream.java:265)
at java.io.DataInputStream.readInt(DataInputStream.java:387)
at org.apache.spark.sql.execution.UnsafeRowSerializerInstance$$anon$2$$anon$3.readSize(UnsafeRowSerializer.scala:113)
at org.apache.spark.sql.execution.UnsafeRowSerializerInstance$$anon$2$$anon$3.next(UnsafeRowSerializer.scala:129)
at org.apache.spark.sql.execution.UnsafeRowSerializerInstance$$anon$2$$anon$3.next(UnsafeRowSerializer.scala:110)
at scala.collection.Iterator$$anon$11.next(Iterator.scala:494)
at scala.collection.Iterator$$anon$10.next(Iterator.scala:459)
at org.apache.spark.util.CompletionIterator.next(CompletionIterator.scala:29)
at org.apache.spark.InterruptibleIterator.next(InterruptibleIterator.scala:40)
at scala.collection.Iterator$$anon$10.next(Iterator.scala:459)
at org.apache.spark.sql.execution.SparkPlan.$anonfun$getByteArrayRdd$1(SparkPlan.scala:345)
at org.apache.spark.rdd.RDD.$anonfun$mapPartitionsInternal$2(RDD.scala:898)
at org.apache.spark.rdd.RDD.$anonfun$mapPartitionsInternal$2$adapted(RDD.scala:898)
at org.apache.spark.rdd.MapPartitionsRDD.compute(MapPartitionsRDD.scala:52)
at org.apache.spark.rdd.RDD.computeOrReadCheckpoint(RDD.scala:373)
at org.apache.spark.rdd.RDD.iterator(RDD.scala:337)
at org.apache.spark.scheduler.ResultTask.runTask(ResultTask.scala:90)
at org.apache.spark.scheduler.Task.run(Task.scala:131)
at org.apache.spark.executor.Executor$TaskRunner.$anonfun$run$3(Executor.scala:498)
at org.apache.spark.util.Utils$.tryWithSafeFinally(Utils.scala:1437)
at org.apache.spark.executor.Executor$TaskRunner.run(Executor.scala:501)
at java.util.concurrent.ThreadPoolExecutor.runWorker(ThreadPoolExecutor.java:1149)
at java.util.concurrent.ThreadPoolExecutor$Worker.run(ThreadPoolExecutor.java:624)
at java.lang.Thread.run(Thread.java:748)
Caused by: java.io.IOException: Stream is corrupted
at net.jpountz.lz4.LZ4BlockInputStream.refill(LZ4BlockInputStream.java:200)
at net.jpountz.lz4.LZ4BlockInputStream.refill(LZ4BlockInputStream.java:226)
at net.jpountz.lz4.LZ4BlockInputStream.read(LZ4BlockInputStream.java:157)
at org.apache.spark.storage.BufferReleasingInputStream.read(ShuffleBlockFetcherIterator.scala:841)
... 25 more
)
```
### Does this PR introduce any user-facing change?
No
### How was this patch tested?
New tests.
Closes#31898 from hezuojiao/fetch_shuffle_in_batch.
Authored-by: hezuojiao <hezuojiao@gmail.com>
Signed-off-by: Dongjoon Hyun <dhyun@apple.com>
### What changes were proposed in this pull request?
Marked `RowNumberLike` and `RankLike` as not-nullable.
### Why are the changes needed?
`RowNumberLike` and `RankLike` SQL expressions never return null value. Marking them as non-nullable can have some performance benefits, because some optimizer rules apply only to non-nullable expressions
### Does this PR introduce _any_ user-facing change?
No
### How was this patch tested?
Did not find any existing tests on the nullability of aggregate functions.
Plan stability suite partially covers this.
Closes#31924 from tanelk/SPARK-34812_nullability.
Authored-by: tanel.kiis@gmail.com <tanel.kiis@gmail.com>
Signed-off-by: Wenchen Fan <wenchen@databricks.com>
### What changes were proposed in this pull request?
Use fine-grained lock in SessionCatalog.tableExists, in order to lock currentDB variable rather than lock `tableExists` method which will block inner external catalog's behaviour.
### Why are the changes needed?
We have modified the underlying hive meta store which a different hive database is placed in its own shard for performance. However, we found that the synchronized lock limits the concurrency.
### How was this patch tested?
Existing tests.
Closes#31891 from woyumen4597/SPARK-34800.
Authored-by: woyumen4597 <woyumen4597@gmail.com>
Signed-off-by: Wenchen Fan <wenchen@databricks.com>
### What changes were proposed in this pull request?
Now that all the temporary views are wrapped with `TemporaryViewRelation`(#31273, #31652, and #31825), this PR proposes to update `SessionCatalog`'s APIs for temporary views to take or return more concrete types.
APIs that will take `TemporaryViewRelation` instead of `LogicalPlan`:
```
createTempView, createGlobalTempView, alterTempViewDefinition
```
APIs that will return `TemporaryViewRelation` instead of `LogicalPlan`:
```
getRawTempView, getRawGlobalTempView
```
APIs that will return `View` instead of `LogicalPlan`:
```
getTempView, getGlobalTempView, lookupTempView
```
### Why are the changes needed?
Internal refactoring to work with more concrete types.
### Does this PR introduce _any_ user-facing change?
No, this is internal refactoring.
### How was this patch tested?
Updated existing tests affected by the refactoring.
Closes#31906 from imback82/use_temporary_view_relation.
Authored-by: Terry Kim <yuminkim@gmail.com>
Signed-off-by: Wenchen Fan <wenchen@databricks.com>
### What changes were proposed in this pull request?
This PR fixes the `HybridRowQueue ` to respect the configured memory mode.
Besides, this PR also refactored the constructor of `MemoryConsumer` to accept the memory mode explicitly.
### Why are the changes needed?
`HybridRowQueue` supports both onHeap and offHeap manipulation. But it inherited the wrong `MemoryConsumer` constructor, which hard-coded the memory mode to `onHeap`.
### Does this PR introduce _any_ user-facing change?
No. (Maybe yes in some cases where users can't complete the job before could complete successfully after the fix because of `HybridRowQueue` is able to spill under offHeap mode now. )
### How was this patch tested?
Updated the existing test to make it test both offHeap and onHeap modes.
Closes#31152 from Ngone51/fix-MemoryConsumer-memorymode.
Authored-by: yi.wu <yi.wu@databricks.com>
Signed-off-by: Wenchen Fan <wenchen@databricks.com>
### What changes were proposed in this pull request?
- Create a new rule `ResolveStreamWrite` for all analysis logic for streaming write.
- Add corresponding logical plans `WriteToStreamStatement` and `WriteToStream`.
### Why are the changes needed?
Currently, the analysis logic for streaming write is mixed in StreamingQueryManager. If we create a specific analyzer rule and separated logical plans, it should be helpful for further extension.
### Does this PR introduce _any_ user-facing change?
No.
### How was this patch tested?
Existing tests.
Closes#31842 from xuanyuanking/SPARK-34748.
Authored-by: Yuanjian Li <yuanjian.li@databricks.com>
Signed-off-by: Wenchen Fan <wenchen@databricks.com>
### What changes were proposed in this pull request?
This PR fixes an incompatible behavior introduced by #31754.
The problem is that quoted name parts represented as a string are given to the constructor of `UnresolvedAttribute` which takes single string parameter, `sql` method invocation against the `UnresolvedAttrribute` returns different result than before.
One example is ``` UnresolvedAttribute("`a.b`").sql ```. This returned `a.b` before but it doesn't now.
See [this duscussion](https://github.com/apache/spark/pull/31754/files#r597181927) for more details.
### Why are the changes needed?
For compatibility.
### Does this PR introduce _any_ user-facing change?
No.
### How was this patch tested?
New assertion.
Closes#31885 from sarutak/followup-SPARK-34636.
Authored-by: Kousuke Saruta <sarutak@oss.nttdata.com>
Signed-off-by: Dongjoon Hyun <dhyun@apple.com>
### What changes were proposed in this pull request?
Improve `PropagateEmptyRelation` to support join with false condition. For example:
```sql
SELECT * FROM t1 LEFT JOIN t2 ON false
```
Before this pr:
```
== Physical Plan ==
AdaptiveSparkPlan isFinalPlan=false
+- BroadcastNestedLoopJoin BuildRight, LeftOuter, false
:- FileScan parquet default.t1[a#4L]
+- BroadcastExchange IdentityBroadcastMode, [id=#40]
+- FileScan parquet default.t2[b#5L]
```
After this pr:
```
== Physical Plan ==
*(1) Project [a#4L, null AS b#5L]
+- *(1) ColumnarToRow
+- FileScan parquet default.t1[a#4L]
```
### Why are the changes needed?
Avoid `BroadcastNestedLoopJoin` to improve query performance.
### Does this PR introduce _any_ user-facing change?
No.
### How was this patch tested?
Unit test.
Closes#31857 from wangyum/SPARK-28220.
Authored-by: Yuming Wang <yumwang@ebay.com>
Signed-off-by: Yuming Wang <yumwang@ebay.com>
### What changes were proposed in this pull request?
This patch proposes to fix a bug related to `NestedColumnAliasing`. The root cause is `Window` doesn't override `producedAttributes` so `NestedColumnAliasing` rule wrongly prune attributes produced by `Window`.
The master and branch-3.1 both have this issue.
### Why are the changes needed?
It is needed to fix a bug of nested column pruning.
### Does this PR introduce _any_ user-facing change?
No
### How was this patch tested?
Unit test.
Closes#31897 from viirya/SPARK-34776.
Authored-by: Liang-Chi Hsieh <viirya@gmail.com>
Signed-off-by: Dongjoon Hyun <dhyun@apple.com>
### What changes were proposed in this pull request?
For all built-in datasources, prohibit saving of year-month and day-time intervals that were introduced by SPARK-27793. We plan to support saving of such types at the milestone 2, see SPARK-27790.
### Why are the changes needed?
To improve user experience with Spark SQL, and print nicer error message. Current error message might confuse users:
```
scala> Seq(java.time.Period.ofMonths(1)).toDF.write.mode("overwrite").json("/Users/maximgekk/tmp/123")
21/03/18 22:44:35 ERROR FileFormatWriter: Aborting job 8de402d7-ab69-4dc0-aa8e-14ef06bd2d6b.
org.apache.spark.SparkException: Job aborted due to stage failure: Task 0 in stage 1.0 failed 1 times, most recent failure: Lost task 0.0 in stage 1.0 (TID 1) (192.168.1.66 executor driver): org.apache.spark.SparkException: Task failed while writing rows.
at org.apache.spark.sql.errors.QueryExecutionErrors$.taskFailedWhileWritingRowsError(QueryExecutionErrors.scala:418)
at org.apache.spark.sql.execution.datasources.FileFormatWriter$.executeTask(FileFormatWriter.scala:298)
at org.apache.spark.sql.execution.datasources.FileFormatWriter$.$anonfun$write$15(FileFormatWriter.scala:211)
at org.apache.spark.scheduler.ResultTask.runTask(ResultTask.scala:90)
at org.apache.spark.scheduler.Task.run(Task.scala:131)
at org.apache.spark.executor.Executor$TaskRunner.$anonfun$run$3(Executor.scala:498)
at org.apache.spark.util.Utils$.tryWithSafeFinally(Utils.scala:1437)
at org.apache.spark.executor.Executor$TaskRunner.run(Executor.scala:501)
at java.util.concurrent.ThreadPoolExecutor.runWorker(ThreadPoolExecutor.java:1149)
at java.util.concurrent.ThreadPoolExecutor$Worker.run(ThreadPoolExecutor.java:624)
at java.lang.Thread.run(Thread.java:748)
Caused by: java.lang.RuntimeException: Failed to convert value 1 (class of class java.lang.Integer}) with the type of YearMonthIntervalType to JSON.
at scala.sys.package$.error(package.scala:30)
at org.apache.spark.sql.catalyst.json.JacksonGenerator.$anonfun$makeWriter$23(JacksonGenerator.scala:179)
at org.apache.spark.sql.catalyst.json.JacksonGenerator.$anonfun$makeWriter$23$adapted(JacksonGenerator.scala:176)
```
### Does this PR introduce _any_ user-facing change?
Yes. After the changes, the example above:
```
scala> Seq(java.time.Period.ofMonths(1)).toDF.write.mode("overwrite").json("/Users/maximgekk/tmp/123")
org.apache.spark.sql.AnalysisException: Cannot save interval data type into external storage.
```
### How was this patch tested?
1. Checked nested intervals:
```
scala> spark.range(1).selectExpr("""struct(timestamp'2021-01-02 00:01:02' - timestamp'2021-01-01 00:00:00')""").write.mode("overwrite").parquet("/Users/maximgekk/tmp/123")
org.apache.spark.sql.AnalysisException: Cannot save interval data type into external storage.
scala> Seq(Seq(java.time.Period.ofMonths(1))).toDF.write.mode("overwrite").json("/Users/maximgekk/tmp/123")
org.apache.spark.sql.AnalysisException: Cannot save interval data type into external storage.
```
2. By running existing test suites:
```
$ build/sbt -Phive-2.3 -Phive-thriftserver "test:testOnly *DataSourceV2DataFrameSuite"
$ build/sbt -Phive-2.3 -Phive-thriftserver "test:testOnly *DataSourceV2SQLSuite"
```
Closes#31884 from MaxGekk/ban-save-intervals.
Authored-by: Max Gekk <max.gekk@gmail.com>
Signed-off-by: Max Gekk <max.gekk@gmail.com>
### What changes were proposed in this pull request?
join condition 'a.attr == 'c.attr check the reference of these 2 objects which will always returns false. we need to use === instead
### Why are the changes needed?
Although this join condition always false doesn't break the test but it is not what we expected. We should fix it to avoid future confusing
### Does this PR introduce _any_ user-facing change?
No
### How was this patch tested?
UT
Closes#31890 from opensky142857/SPARK-34798.
Authored-by: Hongyi Zhang <hongyzhang@ebay.com>
Signed-off-by: Yuming Wang <yumwang@ebay.com>
### What changes were proposed in this pull request?
Change context classloader to Spark classloader at `RebaseDateTime.loadRebaseRecords`
### Why are the changes needed?
With custom `spark.sql.hive.metastore.version` and `spark.sql.hive.metastore.jars`.
Spark would use date formatter in `HiveShim` that convert `date` to `string`, if we set `spark.sql.legacy.timeParserPolicy=LEGACY` and the partition type is `date` the `RebaseDateTime` code will be invoked. At that moment, if `RebaseDateTime` is initialized the first time then context class loader is `IsolatedClientLoader`. Such error msg would throw:
```
java.lang.IllegalArgumentException: argument "src" is null
at com.fasterxml.jackson.databind.ObjectMapper._assertNotNull(ObjectMapper.java:4413)
at com.fasterxml.jackson.databind.ObjectMapper.readValue(ObjectMapper.java:3157)
at com.fasterxml.jackson.module.scala.ScalaObjectMapper.readValue(ScalaObjectMapper.scala:187)
at com.fasterxml.jackson.module.scala.ScalaObjectMapper.readValue$(ScalaObjectMapper.scala:186)
at org.apache.spark.sql.catalyst.util.RebaseDateTime$$anon$1.readValue(RebaseDateTime.scala:267)
at org.apache.spark.sql.catalyst.util.RebaseDateTime$.loadRebaseRecords(RebaseDateTime.scala:269)
at org.apache.spark.sql.catalyst.util.RebaseDateTime$.<init>(RebaseDateTime.scala:291)
at org.apache.spark.sql.catalyst.util.RebaseDateTime$.<clinit>(RebaseDateTime.scala)
at org.apache.spark.sql.catalyst.util.DateTimeUtils$.toJavaDate(DateTimeUtils.scala:109)
at org.apache.spark.sql.catalyst.util.LegacyDateFormatter.format(DateFormatter.scala:95)
at org.apache.spark.sql.catalyst.util.LegacyDateFormatter.format$(DateFormatter.scala:94)
at org.apache.spark.sql.catalyst.util.LegacySimpleDateFormatter.format(DateFormatter.scala:138)
at org.apache.spark.sql.hive.client.Shim_v0_13$ExtractableLiteral$1$.unapply(HiveShim.scala:661)
at org.apache.spark.sql.hive.client.Shim_v0_13.convert$1(HiveShim.scala:785)
at org.apache.spark.sql.hive.client.Shim_v0_13.$anonfun$convertFilters$4(HiveShim.scala:826)
```
```
java.lang.NoClassDefFoundError: Could not initialize class org.apache.spark.sql.catalyst.util.RebaseDateTime$
at org.apache.spark.sql.catalyst.util.DateTimeUtils$.toJavaDate(DateTimeUtils.scala:109)
at org.apache.spark.sql.catalyst.util.LegacyDateFormatter.format(DateFormatter.scala:95)
at org.apache.spark.sql.catalyst.util.LegacyDateFormatter.format$(DateFormatter.scala:94)
at org.apache.spark.sql.catalyst.util.LegacySimpleDateFormatter.format(DateFormatter.scala:138)
at org.apache.spark.sql.hive.client.Shim_v0_13$ExtractableLiteral$1$.unapply(HiveShim.scala:661)
at org.apache.spark.sql.hive.client.Shim_v0_13.convert$1(HiveShim.scala:785)
at org.apache.spark.sql.hive.client.Shim_v0_13.$anonfun$convertFilters$4(HiveShim.scala:826)
at scala.collection.immutable.Stream.flatMap(Stream.scala:493)
at org.apache.spark.sql.hive.client.Shim_v0_13.convertFilters(HiveShim.scala:826)
at org.apache.spark.sql.hive.client.Shim_v0_13.getPartitionsByFilter(HiveShim.scala:848)
at org.apache.spark.sql.hive.client.HiveClientImpl.$anonfun$getPartitionsByFilter$1(HiveClientImpl.scala:749)
at org.apache.spark.sql.hive.client.HiveClientImpl.$anonfun$withHiveState$1(HiveClientImpl.scala:291)
at org.apache.spark.sql.hive.client.HiveClientImpl.liftedTree1$1(HiveClientImpl.scala:224)
at org.apache.spark.sql.hive.client.HiveClientImpl.retryLocked(HiveClientImpl.scala:223)
at org.apache.spark.sql.hive.client.HiveClientImpl.withHiveState(HiveClientImpl.scala:273)
at org.apache.spark.sql.hive.client.HiveClientImpl.getPartitionsByFilter(HiveClientImpl.scala:747)
at org.apache.spark.sql.hive.HiveExternalCatalog.$anonfun$listPartitionsByFilter$1(HiveExternalCatalog.scala:1273)
```
The reproduce steps:
1. `spark.sql.hive.metastore.version` and `spark.sql.hive.metastore.jars`.
2. `CREATE TABLE t (c int) PARTITIONED BY (p date)`
3. `SET spark.sql.legacy.timeParserPolicy=LEGACY`
4. `SELECT * FROM t WHERE p='2021-01-01'`
### Does this PR introduce _any_ user-facing change?
Yes, bug fix.
### How was this patch tested?
pass `org.apache.spark.sql.catalyst.util.RebaseDateTimeSuite` and add new unit test to `HiveSparkSubmitSuite.scala`.
Closes#31864 from ulysses-you/SPARK-34772.
Authored-by: ulysses-you <ulyssesyou18@gmail.com>
Signed-off-by: Yuming Wang <yumwang@ebay.com>
### What changes were proposed in this pull request?
Support `timestamp +/- day-time interval`. In the PR, I propose to extend the `TimeAdd` expression and support `DayTimeIntervalType` as the `interval` parameter. The expression invokes the new method `DateTimeUtils.timestampAddDayTime()` which splits the input day-time interval to `days` and `microsecond adjustment` of a day, and adds `days` (and the microseconds) to a local timestamp derived from the given timestamp at the given time zone. The resulted local timestamp is converted back to the offset in microseconds since the epoch.
Also I updated the rules that handle `CalendarIntervalType` and produce `TimeAdd` to take into account new type `DateTimeIntervalType` for the `interval` parameter of `TimeAdd`.
### Why are the changes needed?
To conform the ANSI SQL standard which requires to support such operation over timestamps and intervals:
<img width="811" alt="Screenshot 2021-03-12 at 11 36 14" src="https://user-images.githubusercontent.com/1580697/111081674-865d4900-8515-11eb-86c8-3538ecaf4804.png">
### Does this PR introduce _any_ user-facing change?
Should not since new intervals have not been released yet.
### How was this patch tested?
By running new tests:
```
$ build/sbt "test:testOnly *DateTimeUtilsSuite"
$ build/sbt "test:testOnly *DateExpressionsSuite"
$ build/sbt "test:testOnly *ColumnExpressionSuite"
```
Closes#31855 from MaxGekk/timestamp-add-day-time-interval.
Authored-by: Max Gekk <max.gekk@gmail.com>
Signed-off-by: Wenchen Fan <wenchen@databricks.com>
### What changes were proposed in this pull request?
This PR proposes to deduplicate the source table when there're conflicting attributes between the target table and the source table.
### Why are the changes needed?
When resolving the `UpdateAction`, which could reference attributes from both target and source tables, Spark should know clearly where the attribute comes from when there're conflicting attributes instead of picking up a random one.
### Does this PR introduce _any_ user-facing change?
No.
### How was this patch tested?
Added a unit test and updated existing tests.
Closes#31835 from Ngone51/dedup-MergeIntoTable.
Authored-by: yi.wu <yi.wu@databricks.com>
Signed-off-by: Wenchen Fan <wenchen@databricks.com>
### What changes were proposed in this pull request?
Remove all SQLConf.get to conf if extends from SQLConfHelper
### Why are the changes needed?
Clean up code.
### Does this PR introduce _any_ user-facing change?
No
### How was this patch tested?
Existing unit tests.
Closes#31822 from leoluan2009/SPARK-34728.
Authored-by: Luan <luanxuedong2009@gmail.com>
Signed-off-by: HyukjinKwon <gurwls223@apache.org>
### What changes were proposed in this pull request?
This PR group exception messages in `/core/src/main/scala/org/apache/spark/sql/execution/datasources`.
### Why are the changes needed?
It will largely help with standardization of error messages and its maintenance.
### Does this PR introduce _any_ user-facing change?
No. Error messages remain unchanged.
### How was this patch tested?
No new tests - pass all original tests to make sure it doesn't break any existing behavior.
Closes#31757 from beliefer/SPARK-33602.
Authored-by: gengjiaan <gengjiaan@360.cn>
Signed-off-by: Wenchen Fan <wenchen@databricks.com>
### What changes were proposed in this pull request?
This PR simplifies `Analyzer.resolveLiteralFunction` to always create the `Alias`. The caller side will remove the `Alias` if it's not necessary.
### Why are the changes needed?
code simplification.
### Does this PR introduce _any_ user-facing change?
no
### How was this patch tested?
existing tests
Closes#31844 from cloud-fan/minor.
Authored-by: Wenchen Fan <wenchen@databricks.com>
Signed-off-by: Takeshi Yamamuro <yamamuro@apache.org>
### What changes were proposed in this pull request?
This is a follow-up of https://github.com/apache/spark/pull/31808 and simplifies its fix to one line (excluding comments).
### Why are the changes needed?
code simplification
### Does this PR introduce _any_ user-facing change?
no
### How was this patch tested?
N/A
Closes#31843 from cloud-fan/simplify.
Authored-by: Wenchen Fan <wenchen@databricks.com>
Signed-off-by: Takeshi Yamamuro <yamamuro@apache.org>
### What changes were proposed in this pull request?
This PR proposes to follow Univocity's input buffer.
### Why are the changes needed?
- Firstly, it's best to trust their judgement on the default values. Also 128 is too low.
- Default values arguably have more test coverage in Univocity.
- It will also fix https://github.com/uniVocity/univocity-parsers/issues/449
- ^ is a regression compared to Spark 2.4
### Does this PR introduce _any_ user-facing change?
No. In addition, It fixes a regression.
### How was this patch tested?
Manually tested, and added a unit test.
Closes#31858 from HyukjinKwon/SPARK-34768.
Authored-by: HyukjinKwon <gurwls223@apache.org>
Signed-off-by: HyukjinKwon <gurwls223@apache.org>
### What changes were proposed in this pull request?
This PR updates `InMemoryCatalog.tableExists` to return false if database doesn't exist, instead of failing. The new behavior is consistent with `HiveExternalCatalog` which is used in production, so this bug mostly only affects tests.
### Why are the changes needed?
bug fix
### Does this PR introduce _any_ user-facing change?
no
### How was this patch tested?
a new test
Closes#31860 from cloud-fan/catalog.
Authored-by: Wenchen Fan <wenchen@databricks.com>
Signed-off-by: Wenchen Fan <wenchen@databricks.com>
### What changes were proposed in this pull request?
Push down limit through `Window` when the partitionSpec of all window functions is empty and the same order is used. This is a real case from production:

This pr support 2 cases:
1. All window functions have same orderSpec:
```sql
SELECT *, ROW_NUMBER() OVER(ORDER BY a) AS rn, RANK() OVER(ORDER BY a) AS rk FROM t1 LIMIT 5;
== Optimized Logical Plan ==
Window [row_number() windowspecdefinition(a#9L ASC NULLS FIRST, specifiedwindowframe(RowFrame, unboundedpreceding$(), currentrow$())) AS rn#4, rank(a#9L) windowspecdefinition(a#9L ASC NULLS FIRST, specifiedwindowframe(RowFrame, unboundedpreceding$(), currentrow$())) AS rk#5], [a#9L ASC NULLS FIRST]
+- GlobalLimit 5
+- LocalLimit 5
+- Sort [a#9L ASC NULLS FIRST], true
+- Relation default.t1[A#9L,B#10L,C#11L] parquet
```
2. There is a window function with a different orderSpec:
```sql
SELECT a, ROW_NUMBER() OVER(ORDER BY a) AS rn, RANK() OVER(ORDER BY b DESC) AS rk FROM t1 LIMIT 5;
== Optimized Logical Plan ==
Project [a#9L, rn#4, rk#5]
+- Window [rank(b#10L) windowspecdefinition(b#10L DESC NULLS LAST, specifiedwindowframe(RowFrame, unboundedpreceding$(), currentrow$())) AS rk#5], [b#10L DESC NULLS LAST]
+- GlobalLimit 5
+- LocalLimit 5
+- Sort [b#10L DESC NULLS LAST], true
+- Window [row_number() windowspecdefinition(a#9L ASC NULLS FIRST, specifiedwindowframe(RowFrame, unboundedpreceding$(), currentrow$())) AS rn#4], [a#9L ASC NULLS FIRST]
+- Project [a#9L, b#10L]
+- Relation default.t1[A#9L,B#10L,C#11L] parquet
```
### Why are the changes needed?
Improve query performance.
```scala
spark.range(500000000L).selectExpr("id AS a", "id AS b").write.saveAsTable("t1")
spark.sql("SELECT *, ROW_NUMBER() OVER(ORDER BY a) AS rowId FROM t1 LIMIT 5").show
```
Before this pr | After this pr
-- | --
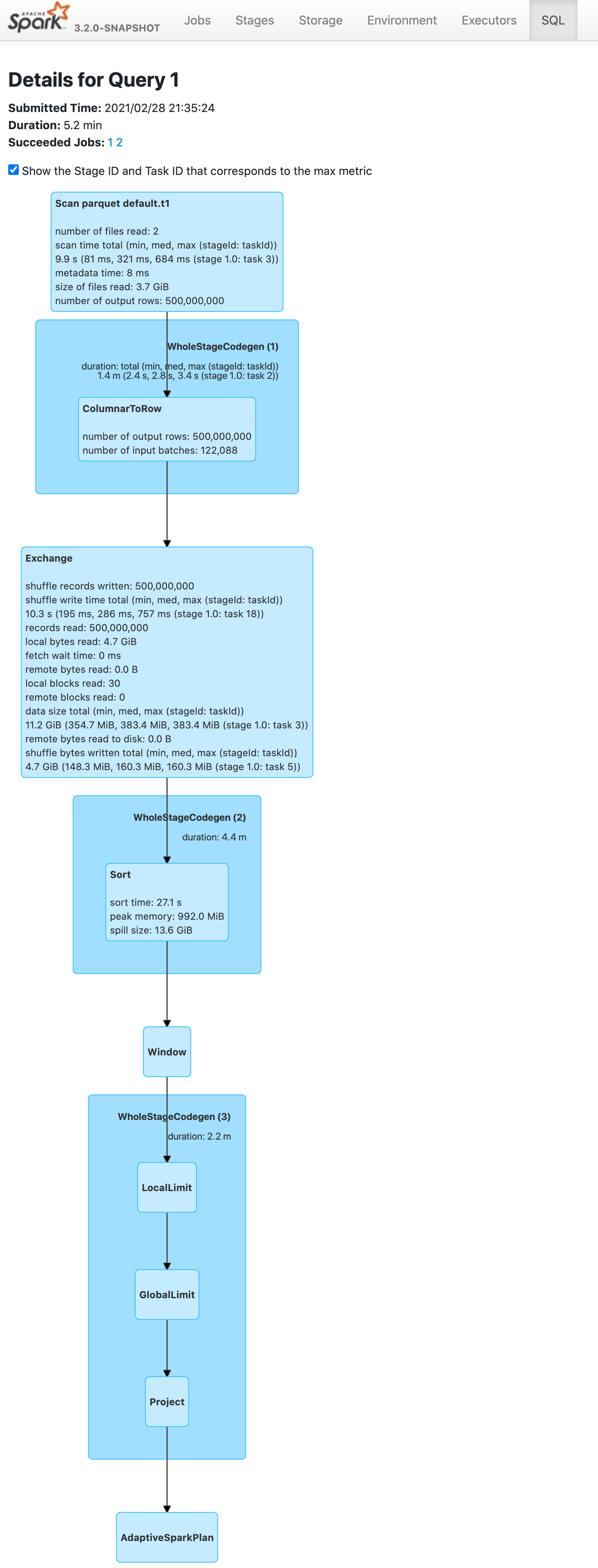 | 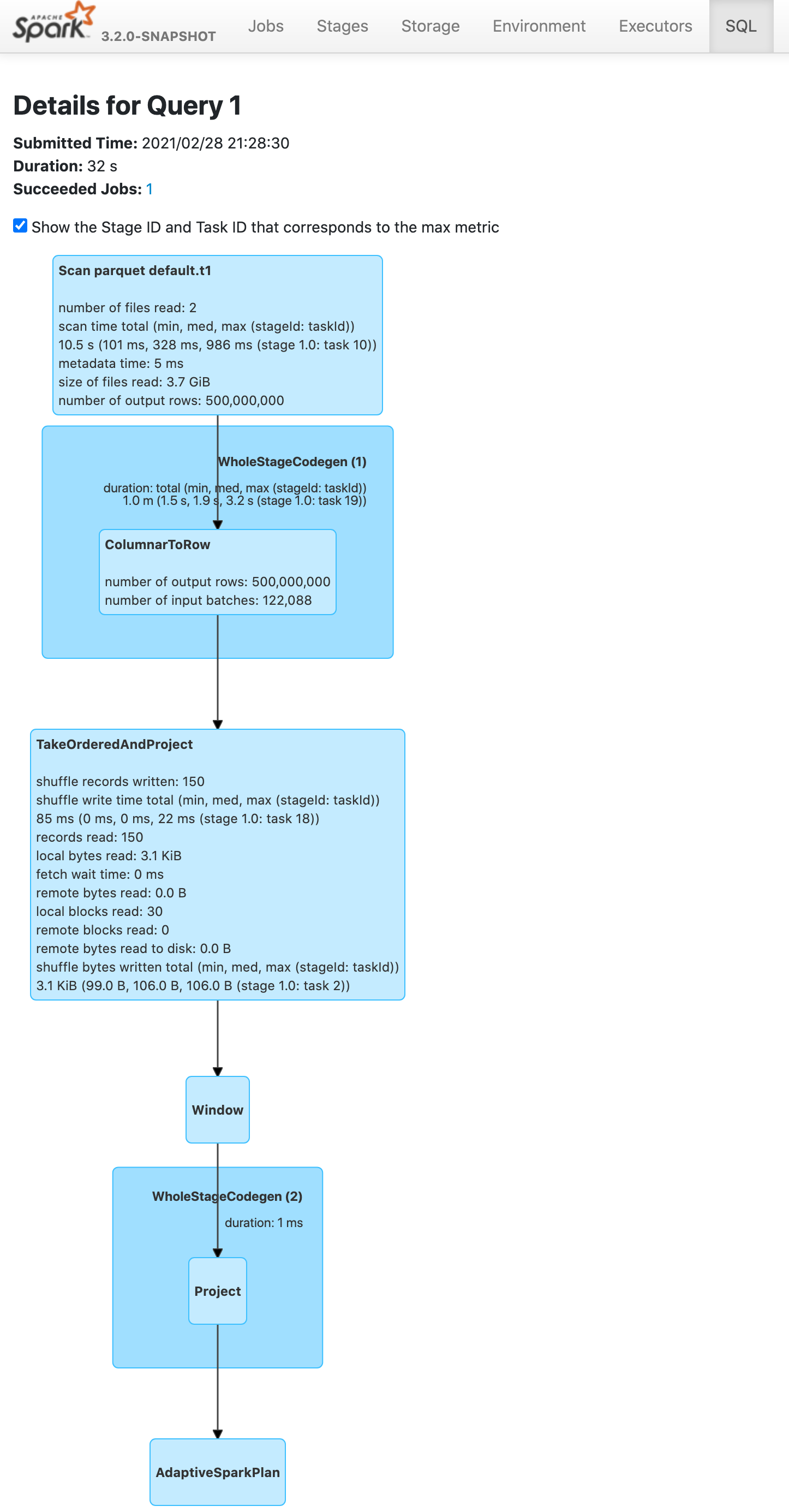
### Does this PR introduce _any_ user-facing change?
No.
### How was this patch tested?
Unit test.
Closes#31691 from wangyum/SPARK-34575.
Authored-by: Yuming Wang <yumwang@ebay.com>
Signed-off-by: Wenchen Fan <wenchen@databricks.com>
### What changes were proposed in this pull request?
For the following cases, ABS should throw exceptions since the results are out of the range of the result data types in ANSI mode.
```
SELECT abs(${Int.MinValue});
SELECT abs(${Long.MinValue});
```
### Why are the changes needed?
Better ANSI compliance
### Does this PR introduce _any_ user-facing change?
Yes, Abs throws an exception if input is out of range in ANSI mode
### How was this patch tested?
Unit test
Closes#31836 from gengliangwang/ansiAbs.
Authored-by: Gengliang Wang <gengliang.wang@databricks.com>
Signed-off-by: Wenchen Fan <wenchen@databricks.com>
### What changes were proposed in this pull request?
For DDL commands like DROP VIEW, they don't really need to resolve the view (parse and analyze the view SQL text), they just need to get the view metadata.
This PR fixes the rule `ResolveTempViews` to only resolve the temp view for `UnresolvedRelation`. This also fixes a bug for DROP VIEW, as previously it tried to resolve the view and failed to drop invalid views.
### Why are the changes needed?
bug fix
### Does this PR introduce _any_ user-facing change?
no
### How was this patch tested?
new test
Closes#31853 from cloud-fan/view-resolve.
Authored-by: Wenchen Fan <wenchen@databricks.com>
Signed-off-by: Wenchen Fan <wenchen@databricks.com>
### What changes were proposed in this pull request?
This PR removes one unused variable in `NewInstance.constructor`.
### Why are the changes needed?
This looks like a variable for debugging at the initial commit of SPARK-23584 .
- 1b08c4393c (diff-2a36e31684505fd22e2d12a864ce89fd350656d716a3f2d7789d2cdbe38e15fbR461)
### Does this PR introduce _any_ user-facing change?
No.
### How was this patch tested?
Pass the CIs.
Closes#31838 from dongjoon-hyun/minor-object.
Authored-by: Dongjoon Hyun <dhyun@apple.com>
Signed-off-by: Dongjoon Hyun <dhyun@apple.com>
### What changes were proposed in this pull request?
Support `timestamp +/- year-month interval`. In the PR, I propose to introduce new binary expression `TimestampAddYMInterval` similarly to `DateAddYMInterval`. It invokes new method `timestampAddMonths` from `DateTimeUtils` by passing a timestamp as an offset in microseconds since the epoch, amount of months from the giveb year-month interval, and the time zone ID in which the operation is performed. The `timestampAddMonths()` method converts the input microseconds to a local timestamp, adds months to it, and converts the results back to an instant in microseconds at the given time zone.
### Why are the changes needed?
To conform the ANSI SQL standard which requires to support such operation over timestamps and intervals:
<img width="811" alt="Screenshot 2021-03-12 at 11 36 14" src="https://user-images.githubusercontent.com/1580697/111081674-865d4900-8515-11eb-86c8-3538ecaf4804.png">
### Does this PR introduce _any_ user-facing change?
Should not since new intervals have not been released yet.
### How was this patch tested?
By running new tests:
```
$ build/sbt "test:testOnly *DateTimeUtilsSuite"
$ build/sbt "test:testOnly *DateExpressionsSuite"
$ build/sbt "test:testOnly *ColumnExpressionSuite"
```
Closes#31832 from MaxGekk/timestamp-add-year-month-interval.
Authored-by: Max Gekk <max.gekk@gmail.com>
Signed-off-by: Max Gekk <max.gekk@gmail.com>
### What changes were proposed in this pull request?
This PR aims to make `ExpressionEncoderSuite` to use `deepEquals` instead of `equals` when `input` is `array of array`.
This comparison code itself was added by SPARK-11727 at Apache Spark 1.6.0.
### Why are the changes needed?
Currently, the interpreted mode fails for `array of array` because the following line is used.
```
Arrays.equals(b1.asInstanceOf[Array[AnyRef]], b2.asInstanceOf[Array[AnyRef]])
```
### Does this PR introduce _any_ user-facing change?
No. This is a test-only PR.
### How was this patch tested?
Pass the existing CIs.
Closes#31837 from dongjoon-hyun/SPARK-34743.
Authored-by: Dongjoon Hyun <dhyun@apple.com>
Signed-off-by: Dongjoon Hyun <dhyun@apple.com>
### What changes were proposed in this pull request?
In `Analyzer.resolveExpression`, we have a parameter to decide if we should remove unnecessary `Alias` or not. This is over complicated and we can always remove unnecessary `Alias`.
This PR simplifies this part and removes the parameter.
### Why are the changes needed?
code cleanup
### Does this PR introduce _any_ user-facing change?
no
### How was this patch tested?
existing tests
Closes#31758 from cloud-fan/resolve.
Authored-by: Wenchen Fan <wenchen@databricks.com>
Signed-off-by: Wenchen Fan <wenchen@databricks.com>
### What changes were proposed in this pull request?
In the PR, I propose to cast the input float to double in the `SecondsToTimestamp` expression in the same way as in the `Cast` expression.
### Why are the changes needed?
To have the same results from `CAST(<float> AS TIMESTAMP)` and from `TIMESTAMP_SECONDS`:
```sql
spark-sql> SELECT CAST(16777215.0f AS TIMESTAMP);
1970-07-14 07:20:15
spark-sql> SELECT TIMESTAMP_SECONDS(16777215.0f);
1970-07-14 07:20:14.951424
```
### Does this PR introduce _any_ user-facing change?
Yes. After the changes:
```sql
spark-sql> SELECT TIMESTAMP_SECONDS(16777215.0f);
1970-07-14 07:20:15
```
### How was this patch tested?
By running new test:
```
$ build/sbt "test:testOnly *DateExpressionsSuite"
```
Closes#31831 from MaxGekk/adjust-SecondsToTimestamp.
Authored-by: Max Gekk <max.gekk@gmail.com>
Signed-off-by: HyukjinKwon <gurwls223@apache.org>
### What changes were proposed in this pull request?
In non-ANSI mode, casting float to timestamp has different implementation for codegen on and off.
Codegen on:
1. Multiply float input by MICROS_PER_SECOND
2. Cast resulting float value to long
Codegen off:
1. CAST float input to double input
2. Multiply double input by MICROS_PER_SECOND
3. Cast resulting double value to long
In the PR, I propose to align to non-codegen code, and cast input float to double in codegen.
### Why are the changes needed?
This fixes the issue which is demonstrated by the code:
```sql
spark-sql> CREATE TEMP VIEW v1 AS SELECT 16777215.0f AS f;
spark-sql> SELECT * FROM v1;
1.6777215E7
spark-sql> SELECT CAST(f AS TIMESTAMP) FROM v1;
1970-07-14 07:20:15
spark-sql> CACHE TABLE v1;
spark-sql> SELECT * FROM v1;
1.6777215E7
spark-sql> SELECT CAST(f AS TIMESTAMP) FROM v1;
1970-07-14 07:20:14.951424
```
The result from the cached view **1970-07-14 07:20:14.951424** is different from un-cached view **1970-07-14 07:20:15**.
### Does this PR introduce _any_ user-facing change?
Yes. After the changes, the example above outputs the same timestamp for the cached view:
```sql
spark-sql> CACHE TABLE v1;
spark-sql> SELECT * FROM v1;
1.6777215E7
spark-sql> SELECT CAST(f AS TIMESTAMP) FROM v1;
1970-07-14 07:20:15
```
### How was this patch tested?
By running new test:
```
$ build/sbt "test:testOnly *CastSuite"
```
Closes#31819 from MaxGekk/fix-float-to-timestamp.
Authored-by: Max Gekk <max.gekk@gmail.com>
Signed-off-by: HyukjinKwon <gurwls223@apache.org>
### What changes were proposed in this pull request?
This patch proposes to fix incorrect parameter type for subexpression elimination under whole-stage.
### Why are the changes needed?
If the parameter is a byte array, the subexpression elimination under wholestage codegen will use incorrect parameter type and cause compile error. Although Spark can automatically fallback to interpreted mode, we should fix it.
### Does this PR introduce _any_ user-facing change?
No
### How was this patch tested?
Manually test with customer application. Unit test.
Closes#31814 from viirya/SPARK-34723.
Authored-by: Liang-Chi Hsieh <viirya@gmail.com>
Signed-off-by: Liang-Chi Hsieh <viirya@gmail.com>
### What changes were proposed in this pull request?
Support `date +/- year-month interval`. In the PR, I propose to re-use existing code from the `AddMonths` expression, and extract it to the common base class `AddMonthsBase`. That base class is used in new expression `DateAddYMInterval` and in the existing one `AddMonths` (the `add_months` function).
### Why are the changes needed?
To conform the ANSI SQL standard which requires to support such operation over dates and intervals:
<img width="811" alt="Screenshot 2021-03-12 at 11 36 14" src="https://user-images.githubusercontent.com/1580697/110914390-5f412480-8327-11eb-9f8b-e92e73c0b9cd.png">
### Does this PR introduce _any_ user-facing change?
Should not since new intervals have not been released yet.
### How was this patch tested?
By running new tests:
```
$ build/sbt "test:testOnly *ColumnExpressionSuite"
$ build/sbt "test:testOnly *DateExpressionsSuite"
```
Closes#31812 from MaxGekk/date-add-year-month-interval.
Authored-by: Max Gekk <max.gekk@gmail.com>
Signed-off-by: Wenchen Fan <wenchen@databricks.com>
### What changes were proposed in this pull request?
This bug was introduced by SPARK-23583 at Apache Spark 2.4.0.
This PR aims to use `getMethod` instead of `getDeclaredMethod`.
```scala
- obj.getClass.getDeclaredMethod(functionName, argClasses: _*)
+ obj.getClass.getMethod(functionName, argClasses: _*)
```
### Why are the changes needed?
`getDeclaredMethod` does not search the super class's method. To invoke `GenericArrayData.toIntArray`, we need to use `getMethod` because it's declared at the super class `ArrayData`.
```
[info] - encode/decode for array of int: [I74655d03 (interpreted path) *** FAILED *** (14 milliseconds)
[info] Exception thrown while decoding
[info] Converted: [0,1000000020,3,0,ffffff850000001f,4]
[info] Schema: value#680
[info] root
[info] -- value: array (nullable = true)
[info] |-- element: integer (containsNull = false)
[info]
[info]
[info] Encoder:
[info] class[value[0]: array<int>] (ExpressionEncoderSuite.scala:578)
[info] org.scalatest.exceptions.TestFailedException:
[info] at org.scalatest.Assertions.newAssertionFailedException(Assertions.scala:472)
[info] at org.scalatest.Assertions.newAssertionFailedException$(Assertions.scala:471)
[info] at org.scalatest.funsuite.AnyFunSuite.newAssertionFailedException(AnyFunSuite.scala:1563)
[info] at org.scalatest.Assertions.fail(Assertions.scala:949)
[info] at org.scalatest.Assertions.fail$(Assertions.scala:945)
[info] at org.scalatest.funsuite.AnyFunSuite.fail(AnyFunSuite.scala:1563)
[info] at org.apache.spark.sql.catalyst.encoders.ExpressionEncoderSuite.$anonfun$encodeDecodeTest$1(ExpressionEncoderSuite.scala:578)
[info] at org.apache.spark.sql.catalyst.encoders.ExpressionEncoderSuite.verifyNotLeakingReflectionObjects(ExpressionEncoderSuite.scala:656)
[info] at org.apache.spark.sql.catalyst.encoders.ExpressionEncoderSuite.$anonfun$testAndVerifyNotLeakingReflectionObjects$2(ExpressionEncoderSuite.scala:669)
[info] at org.apache.spark.sql.catalyst.plans.CodegenInterpretedPlanTest.$anonfun$test$4(PlanTest.scala:50)
[info] at org.apache.spark.sql.catalyst.plans.SQLHelper.withSQLConf(SQLHelper.scala:54)
[info] at org.apache.spark.sql.catalyst.plans.SQLHelper.withSQLConf$(SQLHelper.scala:38)
[info] at org.apache.spark.sql.catalyst.encoders.ExpressionEncoderSuite.withSQLConf(ExpressionEncoderSuite.scala:118)
[info] at org.apache.spark.sql.catalyst.plans.CodegenInterpretedPlanTest.$anonfun$test$3(PlanTest.scala:50)
...
[info] Cause: java.lang.RuntimeException: Error while decoding: java.lang.NoSuchMethodException: org.apache.spark.sql.catalyst.util.GenericArrayData.toIntArray()
[info] mapobjects(lambdavariable(MapObject, IntegerType, false, -1), assertnotnull(lambdavariable(MapObject, IntegerType, false, -1)), input[0, array<int>, true], None).toIntArray
[info] at org.apache.spark.sql.catalyst.encoders.ExpressionEncoder$Deserializer.apply(ExpressionEncoder.scala:186)
[info] at org.apache.spark.sql.catalyst.encoders.ExpressionEncoderSuite.$anonfun$encodeDecodeTest$1(ExpressionEncoderSuite.scala:576)
[info] at org.apache.spark.sql.catalyst.encoders.ExpressionEncoderSuite.verifyNotLeakingReflectionObjects(ExpressionEncoderSuite.scala:656)
[info] at org.apache.spark.sql.catalyst.encoders.ExpressionEncoderSuite.$anonfun$testAndVerifyNotLeakingReflectionObjects$2(ExpressionEncoderSuite.scala:669)
```
### Does this PR introduce _any_ user-facing change?
This causes a runtime exception when we use the interpreted mode.
### How was this patch tested?
Pass the modified unit test case.
Closes#31816 from dongjoon-hyun/SPARK-34724.
Authored-by: Dongjoon Hyun <dhyun@apple.com>
Signed-off-by: HyukjinKwon <gurwls223@apache.org>
### What changes were proposed in this pull request?
This PR fixes an issue that `sql` method in the following classes which take qualified names don't quote the qualified names properly.
* UnresolvedAttribute
* AttributeReference
* Alias
One instance caused by this issue is reported in SPARK-34626.
```
UnresolvedAttribute("a" :: "b" :: Nil).sql
`a.b` // expected: `a`.`b`
```
And other instances are like as follows.
```
UnresolvedAttribute("a`b"::"c.d"::Nil).sql
a`b.`c.d` // expected: `a``b`.`c.d`
AttributeReference("a.b", IntegerType)(qualifier = "c.d"::Nil).sql
c.d.`a.b` // expected: `c.d`.`a.b`
Alias(AttributeReference("a", IntegerType)(), "b.c")(qualifier = "d.e"::Nil).sql
`a` AS d.e.`b.c` // expected: `a` AS `d.e`.`b.c`
```
### Why are the changes needed?
This is a bug.
### Does this PR introduce _any_ user-facing change?
No.
### How was this patch tested?
New test.
Closes#31754 from sarutak/fix-qualified-names.
Authored-by: Kousuke Saruta <sarutak@oss.nttdata.com>
Signed-off-by: Wenchen Fan <wenchen@databricks.com>
### What changes were proposed in this pull request?
In the PR, I propose to override the `typeName()` method in `YearMonthIntervalType` and `DayTimeIntervalType`, and assign them names according to the ANSI SQL standard:
<img width="836" alt="Screenshot 2021-03-11 at 17 29 04" src="https://user-images.githubusercontent.com/1580697/110802854-a54aa980-828f-11eb-956d-dd4fbf14aa72.png">
but keep the type name as singular according existing naming convention for other types.
### Why are the changes needed?
To improve Spark SQL user experience, and have readable types in error messages.
### Does this PR introduce _any_ user-facing change?
Should not since the types has not been released yet.
### How was this patch tested?
By running the modified tests:
```
$ build/sbt "test:testOnly *ExpressionTypeCheckingSuite"
$ build/sbt "sql/testOnly *SQLQueryTestSuite -- -z windowFrameCoercion.sql"
$ build/sbt "sql/testOnly *SQLQueryTestSuite -- -z literals.sql"
```
Closes#31810 from MaxGekk/interval-types-name.
Authored-by: Max Gekk <max.gekk@gmail.com>
Signed-off-by: Dongjoon Hyun <dhyun@apple.com>
### What changes were proposed in this pull request?
This is a bug caused by https://issues.apache.org/jira/browse/SPARK-31670 . We remove the `Alias` when resolving column references in grouping expressions, which breaks `ResolveCreateNamedStruct`
### Why are the changes needed?
bug fix
### Does this PR introduce _any_ user-facing change?
no
### How was this patch tested?
new tests
Closes#31808 from cloud-fan/bug.
Authored-by: Wenchen Fan <wenchen@databricks.com>
Signed-off-by: Dongjoon Hyun <dhyun@apple.com>
### What changes were proposed in this pull request?
In the PR, I propose to especially handle the amount of seconds `-9223372036855` in `IntervalUtils. durationToMicros()`. Starting from the amount (any durations with the second field < `-9223372036855`), input durations cannot fit to `Long` in the conversion to microseconds. For example, the amount of microseconds = `Long.MinValue = -9223372036854775808` can be represented in two forms:
1. seconds = -9223372036854, nanoAdjustment = -775808, or
2. seconds = -9223372036855, nanoAdjustment = +224192
And the method `Duration.ofSeconds()` produces the last form but such form causes overflow while converting `-9223372036855` seconds to microseconds.
In the PR, I propose to convert the second form to the first one if the second field of input duration is equal to `-9223372036855`.
### Why are the changes needed?
The changes fix the issue demonstrated by the code:
```scala
scala> durationToMicros(microsToDuration(Long.MinValue))
java.lang.ArithmeticException: long overflow
at java.lang.Math.multiplyExact(Math.java:892)
at org.apache.spark.sql.catalyst.util.IntervalUtils$.durationToMicros(IntervalUtils.scala:782)
... 49 elided
```
The `durationToMicros()` method cannot handle valid output of `microsToDuration()`.
### Does this PR introduce _any_ user-facing change?
Should not since new interval types has not been released yet.
### How was this patch tested?
By running new UT from `IntervalUtilsSuite`.
Closes#31799 from MaxGekk/fix-min-duration.
Authored-by: Max Gekk <max.gekk@gmail.com>
Signed-off-by: Wenchen Fan <wenchen@databricks.com>
### What changes were proposed in this pull request?
Add `Not(In)` and `Not(InSet)` pattern when convert filter to metastore.
### Why are the changes needed?
`NOT IN` is a useful condition to prune partition, it would be better to support it.
Technically, we can convert `c not in(x,y)` to `c != x and c != y`, then push it to metastore.
Avoid metastore overflow and respect the config `spark.sql.hive.metastorePartitionPruningInSetThreshold`, `Not(InSet)` won't push to metastore if it's value exceeds the threshold.
### Does this PR introduce _any_ user-facing change?
No.
### How was this patch tested?
Add test.
Closes#31646 from ulysses-you/SPARK-34538.
Authored-by: ulysses-you <ulyssesyou18@gmail.com>
Signed-off-by: Wenchen Fan <wenchen@databricks.com>
### What changes were proposed in this pull request?
Extend the `Add`, `Subtract` and `UnaryMinus` expression to support `DayTimeIntervalType` and `YearMonthIntervalType` added by #31614.
Note: the expressions can throw the `overflow` exception independently from the SQL config `spark.sql.ansi.enabled`. In this way, the modified expressions always behave in the ANSI mode for the intervals.
### Why are the changes needed?
To conform to the ANSI SQL standard which defines `-/+` over intervals:
<img width="822" alt="Screenshot 2021-03-09 at 21 59 22" src="https://user-images.githubusercontent.com/1580697/110523128-bd50ea80-8122-11eb-9982-782da0088d27.png">
### Does this PR introduce _any_ user-facing change?
Should not since new types have not been released yet.
### How was this patch tested?
By running new tests in the test suites:
```
$ build/sbt "test:testOnly *ArithmeticExpressionSuite"
$ build/sbt "test:testOnly *ColumnExpressionSuite"
```
Closes#31789 from MaxGekk/add-subtruct-intervals.
Authored-by: Max Gekk <max.gekk@gmail.com>
Signed-off-by: Wenchen Fan <wenchen@databricks.com>
### What changes were proposed in this pull request?
SPARK-23596 added `CodegenInterpretedPlanTest` at Apache Spark 2.4.0 in a wrong way because `withSQLConf` depends on the execution time `SQLConf.get` instead of `test` function declaration time. So, the following code executes the test twice without controlling the `CodegenObjectFactoryMode`. This PR aims to fix it correct and introduce a new function `testFallback`.
```scala
trait CodegenInterpretedPlanTest extends PlanTest {
override protected def test(
testName: String,
testTags: Tag*)(testFun: => Any)(implicit pos: source.Position): Unit = {
val codegenMode = CodegenObjectFactoryMode.CODEGEN_ONLY.toString
val interpretedMode = CodegenObjectFactoryMode.NO_CODEGEN.toString
withSQLConf(SQLConf.CODEGEN_FACTORY_MODE.key -> codegenMode) {
super.test(testName + " (codegen path)", testTags: _*)(testFun)(pos)
}
withSQLConf(SQLConf.CODEGEN_FACTORY_MODE.key -> interpretedMode) {
super.test(testName + " (interpreted path)", testTags: _*)(testFun)(pos)
}
}
}
```
### Why are the changes needed?
1. We need to use like the following.
```scala
super.test(testName + " (codegen path)", testTags: _*)(
withSQLConf(SQLConf.CODEGEN_FACTORY_MODE.key -> codegenMode) { testFun })(pos)
super.test(testName + " (interpreted path)", testTags: _*)(
withSQLConf(SQLConf.CODEGEN_FACTORY_MODE.key -> interpretedMode) { testFun })(pos)
```
2. After we fix this behavior with the above code, several test cases including SPARK-34596 and SPARK-34607 fail because they didn't work at both `CODEGEN` and `INTERPRETED` mode. Those test cases only work at `FALLBACK` mode. So, inevitably, we need to introduce `testFallback`.
### Does this PR introduce _any_ user-facing change?
No.
### How was this patch tested?
Pass the CIs.
Closes#31766 from dongjoon-hyun/SPARK-34596-SPARK-34607.
Lead-authored-by: Dongjoon Hyun <dhyun@apple.com>
Co-authored-by: Dongjoon Hyun <dongjoon@apache.org>
Signed-off-by: Dongjoon Hyun <dhyun@apple.com>
### What changes were proposed in this pull request?
This PR adds a default null ordering to public `SortDirection` to match the Catalyst behavior.
### Why are the changes needed?
The SQL standard does not define the default null ordering for a sort direction. That's why it is up to a query engine to assign one. We need to standardize this in our public connector expressions to avoid ambiguity. That's why I propose to match the behavior in our Catalyst expressions.
### Does this PR introduce _any_ user-facing change?
Yes, it affects unreleased connector expression API.
### How was this patch tested?
Existing tests.
Closes#31580 from aokolnychyi/spark-34457.
Authored-by: Anton Okolnychyi <aokolnychyi@apple.com>
Signed-off-by: Wenchen Fan <wenchen@databricks.com>
### What changes were proposed in this pull request?
This PR proposes the following:
* `AlterViewAs.query` is currently analyzed in the physical operator `AlterViewAsCommand`, but it should be analyzed during the analysis phase.
* When `spark.sql.legacy.storeAnalyzedPlanForView` is set to true, store `TermporaryViewRelation` which wraps the analyzed plan, similar to #31273.
* Try to uncache the view you are altering.
### Why are the changes needed?
Analyzing a plan should be done in the analysis phase if possible.
Not uncaching the view (existing behavior) seems like a bug since the cache may not be used again.
### Does this PR introduce _any_ user-facing change?
Yes, now the view can be uncached if it's already cached.
### How was this patch tested?
Added new tests around uncaching.
The existing tests such as `SQLViewSuite` should cover the analysis changes.
Closes#31652 from imback82/alter_view_child.
Authored-by: Terry Kim <yuminkim@gmail.com>
Signed-off-by: Wenchen Fan <wenchen@databricks.com>
### What changes were proposed in this pull request?
This PR intends to remove unnecessary `SQLConf.withExistingConf` in `CastSuite`; since we've remove `ParVector ` in #31775, we no longer need to copy SQL configs into each thread env.
### Why are the changes needed?
Clean up the code.
### Does this PR introduce _any_ user-facing change?
No.
### How was this patch tested?
Run the existing tests.
Closes#31785 from maropu/UpdateCastSuite.
Authored-by: Takeshi Yamamuro <yamamuro@apache.org>
Signed-off-by: Dongjoon Hyun <dhyun@apple.com>
### What changes were proposed in this pull request?
Add `DayTimeIntervalType` and `YearMonthIntervalType` to `DataTypeTestUtils.ordered`/`atomicTypes`, and implement values generation of those types in `LiteralGenerator`/`RandomDataGenerator`. In this way, the types will be tested automatically in:
1. ArithmeticExpressionSuite:
- "function least"
- "function greatest"
2. PredicateSuite
- "BinaryComparison consistency check"
- "AND, OR, EqualTo, EqualNullSafe consistency check"
3. ConditionalExpressionSuite
- "if"
4. RandomDataGeneratorSuite
- "Basic types"
5. CastSuite
- "null cast"
- "up-cast"
- "SPARK-27671: cast from nested null type in struct"
6. OrderingSuite
- "GenerateOrdering with DayTimeIntervalType"
- "GenerateOrdering with YearMonthIntervalType"
7. PredicateSuite
- "IN with different types"
8. UnsafeRowSuite
- "calling get(ordinal, datatype) on null columns"
9. SortSuite
- "sorting on YearMonthIntervalType ..."
- "sorting on DayTimeIntervalType ..."
### Why are the changes needed?
To improve test coverage.
### Does this PR introduce _any_ user-facing change?
No
### How was this patch tested?
By running the affected test suites.
Closes#31782 from MaxGekk/test-interval-as-atomic.
Authored-by: Max Gekk <max.gekk@gmail.com>
Signed-off-by: Wenchen Fan <wenchen@databricks.com>
### What changes were proposed in this pull request?
This PR updates UnresolvedTableValuedFunction's name to be a FunctionIdentifier instead of a string.
### Why are the changes needed?
To make UnresolvedTableValuedFunction consistent with UnresolvedFunction that uses FunctionIdentifier as the function name.
### Does this PR introduce _any_ user-facing change?
No
### How was this patch tested?
Unit test.
Closes#31749 from allisonwang-db/spark-34627.
Authored-by: allisonwang-db <66282705+allisonwang-db@users.noreply.github.com>
Signed-off-by: Wenchen Fan <wenchen@databricks.com>
### What changes were proposed in this pull request?
RewritePredicateSubquery Optimizer Rule must not update Filters without subqueries.
Following is one such example.
```
=== Applying Rule org.apache.spark.sql.catalyst.optimizer.RewritePredicateSubquery ===
Project [a#0] Project [a#0]
!+- Filter (((a#0 > 1) OR (b#1 > 2)) AND ((c#2 > 1) AND (d#3 > 2))) +- Filter ((((a#0 > 1) OR (b#1 > 2)) AND (c#2 > 1)) AND (d#3 > 2))
+- LocalRelation <empty>, [a#0, b#1, c#2, d#3] +- LocalRelation <empty>, [a#0, b#1, c#2, d#3]
```
### Why are the changes needed?
minor change.
### Does this PR introduce _any_ user-facing change?
No
### How was this patch tested?
Existing UTs pass.
Closes#31712 from Swinky/rewritePredicateFix.
Authored-by: Swinky <mannswinky@gmail.com>
Signed-off-by: Wenchen Fan <wenchen@databricks.com>
### What changes were proposed in this pull request?
In the PR, I propose to extend Spark SQL API to accept [`java.time.Period`](https://docs.oracle.com/javase/8/docs/api/java/time/Period.html) as an external type of recently added new Catalyst type - `YearMonthIntervalType` (see #31614). The Java class `java.time.Period` has similar semantic to ANSI SQL year-month interval type, and it is the most suitable to be an external type for `YearMonthIntervalType`. In more details:
1. Added `PeriodConverter` which converts `java.time.Period` instances to/from internal representation of the Catalyst type `YearMonthIntervalType` (to `Int` type). The `PeriodConverter` object uses new methods of `IntervalUtils`:
- `periodToMonths()` converts the input period to the total length in months. If this period is too large to fit `Int`, the method throws the exception `ArithmeticException`. **Note:** _the input period has "days" precision, the method just ignores the days unit._
- `monthToPeriod()` obtains a `java.time.Period` representing a number of months.
2. Support new type `YearMonthIntervalType` in `RowEncoder` via the methods `createDeserializerForPeriod()` and `createSerializerForJavaPeriod()`.
3. Extended the Literal API to construct literals from `java.time.Period` instances.
### Why are the changes needed?
1. To allow users parallelization of `java.time.Period` collections, and construct year-month interval columns. Also to collect such columns back to the driver side.
2. This will allow to write tests in other sub-tasks of SPARK-27790.
### Does this PR introduce _any_ user-facing change?
The PR extends existing functionality. So, users can parallelize instances of the `java.time.Duration` class and collect them back:
```scala
scala> val ds = Seq(java.time.Period.ofYears(10).withMonths(2)).toDS
ds: org.apache.spark.sql.Dataset[java.time.Period] = [value: yearmonthinterval]
scala> ds.collect
res0: Array[java.time.Period] = Array(P10Y2M)
```
### How was this patch tested?
- Added a few tests to `CatalystTypeConvertersSuite` to check conversion from/to `java.time.Period`.
- Checking row encoding by new tests in `RowEncoderSuite`.
- Making literals of `YearMonthIntervalType` are tested in `LiteralExpressionSuite`.
- Check collecting by `DatasetSuite` and `JavaDatasetSuite`.
- New tests in `IntervalUtilsSuites` to check conversions `java.time.Period` <-> months.
Closes#31765 from MaxGekk/java-time-period.
Authored-by: Max Gekk <max.gekk@gmail.com>
Signed-off-by: Wenchen Fan <wenchen@databricks.com>
### What changes were proposed in this pull request?
When we do self join with transform in a CTE, spark will throw AnalysisException.
A simple way to reproduce is
```
create temporary view t as select * from values 0, 1, 2 as t(a);
WITH temp AS (
SELECT TRANSFORM(a) USING 'cat' AS (b string) FROM t
)
SELECT t1.b FROM temp t1 JOIN temp t2 ON t1.b = t2.b
```
before this patch, it throws
```
org.apache.spark.sql.AnalysisException: cannot resolve '`t1.b`' given input columns: [t1.b]; line 6 pos 41;
'Project ['t1.b]
+- 'Join Inner, ('t1.b = 't2.b)
:- SubqueryAlias t1
: +- SubqueryAlias temp
: +- ScriptTransformation [a#1], cat, [b#2], ScriptInputOutputSchema(List(),List(),Some(org.apache.hadoop.hive.serde2.DelimitedJSONSerDe),Some(org.apache.hadoop.hive.serde2.lazy.LazySimpleSerDe),List((field.delim, )),List((field.delim, )),Some(org.apache.hadoop.hive.ql.exec.TextRecordReader),Some(org.apache.hadoop.hive.ql.exec.TextRecordWriter),false)
: +- SubqueryAlias t
: +- Project [a#1]
: +- SubqueryAlias t
: +- LocalRelation [a#1]
+- SubqueryAlias t2
+- SubqueryAlias temp
+- ScriptTransformation [a#1], cat, [b#2], ScriptInputOutputSchema(List(),List(),Some(org.apache.hadoop.hive.serde2.DelimitedJSONSerDe),Some(org.apache.hadoop.hive.serde2.lazy.LazySimpleSerDe),List((field.delim, )),List((field.delim, )),Some(org.apache.hadoop.hive.ql.exec.TextRecordReader),Some(org.apache.hadoop.hive.ql.exec.TextRecordWriter),false)
+- SubqueryAlias t
+- Project [a#1]
+- SubqueryAlias t
+- LocalRelation [a#1]
```
### Does this PR introduce _any_ user-facing change?
NO
### How was this patch tested?
Add a UT
Closes#31752 from WangGuangxin/selfjoin-with-transform.
Authored-by: wangguangxin.cn <wangguangxin.cn@bytedance.com>
Signed-off-by: HyukjinKwon <gurwls223@apache.org>
### What changes were proposed in this pull request?
This pr remove `GlobalLimit` operator if its child max rows not larger than limit number. For example:
```
val testRelation = LocalRelation.fromExternalRows(Seq("a".attr.int, "b".attr.int, "c".attr.int), 1.to(10).map(_ => Row(1, 2, 3)) )
val query = GlobalLimit(100, testRelation)
```
We can remove this `GlobalLimit`.
### Why are the changes needed?
Further optimize the query.
### Does this PR introduce _any_ user-facing change?
No.
### How was this patch tested?
Unit test.
Closes#31750 from wangyum/SPARK-34628.
Authored-by: Yuming Wang <yumwang@ebay.com>
Signed-off-by: Dongjoon Hyun <dhyun@apple.com>
### What changes were proposed in this pull request?
Unify output of ShowCreateTableAsSerdeCommand and ShowCreateTableCommand
### Why are the changes needed?
Unify output of ShowCreateTableAsSerdeCommand and ShowCreateTableCommand
### Does this PR introduce _any_ user-facing change?
No
### How was this patch tested?
Closes#31737 from AngersZhuuuu/SPARK-34621.
Authored-by: Angerszhuuuu <angers.zhu@gmail.com>
Signed-off-by: Wenchen Fan <wenchen@databricks.com>
### What changes were proposed in this pull request?
This PR intends to refactor the logic to resolve `__grouping_id` in the `Analyzer`; it moves the logic from `ResolveFunctions` to `ResolveReferences` (`resolveLiteralFunction`).
The original author of this PR is sqlwindspeaker (#30781).
Closes#30781.
### Why are the changes needed?
Code refactoring.
### Does this PR introduce _any_ user-facing change?
No.
### How was this patch tested?
Added tests in `AnalysisSuite`.
Closes#31751 from maropu/SPARK-22748.
Authored-by: suqilong <suqilong@qiyi.com>
Signed-off-by: Wenchen Fan <wenchen@databricks.com>
### What changes were proposed in this pull request?
It's a bit confusing to see `resolveExpressionBottomUp` and `resolveExpressionTopDown`, which provide similar functionalities but with different tree traverse order. It turns out that the real difference between these 2 methods is: which attributes should the columns be resolved to? `resolveExpressionTopDown` resolves columns using output attributes of the plan children, `resolveExpressionBottomUp` resolves columns using output attributes of the plan itself.
This PR unifies `resolveExpressionBottomUp` and `resolveExpressionTopDown` and put the common logic in a new method, and let `resolveExpressionBottomUp` and `resolveExpressionTopDown` just call the new method. This PR also renames `resolveExpressionBottomUp` and `resolveExpressionTopDown` to make the difference clear.
### Why are the changes needed?
code cleanup
### Does this PR introduce _any_ user-facing change?
no
### How was this patch tested?
existing tests
Closes#31728 from cloud-fan/resolve.
Authored-by: Wenchen Fan <wenchen@databricks.com>
Signed-off-by: Wenchen Fan <wenchen@databricks.com>
### What changes were proposed in this pull request?
We have equality in `SqlBase.g4` for `RLIKE: 'RLIKE' | 'REGEXP';`
We seemed to miss adding` REGEXP` as a SQL function just like` RLIKE`
### Why are the changes needed?
symmetry and beauty
This is also a builtin function in Hive, we can reduce the migration pain for those users
### Does this PR introduce _any_ user-facing change?
yes new regexp function as an alias as rlike
### How was this patch tested?
new tests
Closes#31488 from yaooqinn/SPARK-34376.
Authored-by: Kent Yao <yao@apache.org>
Signed-off-by: HyukjinKwon <gurwls223@apache.org>
### What changes were proposed in this pull request?
This PR intends to fix a bug of `objects.NewInstance` if a user runs Spark on jdk8u and a given `cls` in `NewInstance` is a deeply-nested inner class, e.g.,.
```
object OuterLevelWithVeryVeryVeryLongClassName1 {
object OuterLevelWithVeryVeryVeryLongClassName2 {
object OuterLevelWithVeryVeryVeryLongClassName3 {
object OuterLevelWithVeryVeryVeryLongClassName4 {
object OuterLevelWithVeryVeryVeryLongClassName5 {
object OuterLevelWithVeryVeryVeryLongClassName6 {
object OuterLevelWithVeryVeryVeryLongClassName7 {
object OuterLevelWithVeryVeryVeryLongClassName8 {
object OuterLevelWithVeryVeryVeryLongClassName9 {
object OuterLevelWithVeryVeryVeryLongClassName10 {
object OuterLevelWithVeryVeryVeryLongClassName11 {
object OuterLevelWithVeryVeryVeryLongClassName12 {
object OuterLevelWithVeryVeryVeryLongClassName13 {
object OuterLevelWithVeryVeryVeryLongClassName14 {
object OuterLevelWithVeryVeryVeryLongClassName15 {
object OuterLevelWithVeryVeryVeryLongClassName16 {
object OuterLevelWithVeryVeryVeryLongClassName17 {
object OuterLevelWithVeryVeryVeryLongClassName18 {
object OuterLevelWithVeryVeryVeryLongClassName19 {
object OuterLevelWithVeryVeryVeryLongClassName20 {
case class MalformedNameExample2(x: Int)
}}}}}}}}}}}}}}}}}}}}
```
The root cause that Kris (rednaxelafx) investigated is as follows (Kudos to Kris);
The reason why the test case above is so convoluted is in the way Scala generates the class name for nested classes. In general, Scala generates a class name for a nested class by inserting the dollar-sign ( `$` ) in between each level of class nesting. The problem is that this format can concatenate into a very long string that goes beyond certain limits, so Scala will change the class name format beyond certain length threshold.
For the example above, we can see that the first two levels of class nesting have class names that look like this:
```
org.apache.spark.sql.catalyst.encoders.ExpressionEncoderSuite$OuterLevelWithVeryVeryVeryLongClassName1$
org.apache.spark.sql.catalyst.encoders.ExpressionEncoderSuite$OuterLevelWithVeryVeryVeryLongClassName1$OuterLevelWithVeryVeryVeryLongClassName2$
```
If we leave out the fact that Scala uses a dollar-sign ( `$` ) suffix for the class name of the companion object, `OuterLevelWithVeryVeryVeryLongClassName1`'s full name is a prefix (substring) of `OuterLevelWithVeryVeryVeryLongClassName2`.
But if we keep going deeper into the levels of nesting, you'll find names that look like:
```
org.apache.spark.sql.catalyst.encoders.ExpressionEncoderSuite$OuterLevelWithVeryVeryVeryLongClassNam$$$$2a1321b953c615695d7442b2adb1$$$$ryVeryLongClassName8$OuterLevelWithVeryVeryVeryLongClassName9$OuterLevelWithVeryVeryVeryLongClassName10$
org.apache.spark.sql.catalyst.encoders.ExpressionEncoderSuite$OuterLevelWithVeryVeryVeryLongClassNam$$$$2a1321b953c615695d7442b2adb1$$$$ryVeryLongClassName8$OuterLevelWithVeryVeryVeryLongClassName9$OuterLevelWithVeryVeryVeryLongClassName10$OuterLevelWithVeryVeryVeryLongClassName11$
org.apache.spark.sql.catalyst.encoders.ExpressionEncoderSuite$OuterLevelWithVeryVeryVeryLongClassNam$$$$85f068777e7ecf112afcbe997d461b$$$$VeryLongClassName11$OuterLevelWithVeryVeryVeryLongClassName12$
org.apache.spark.sql.catalyst.encoders.ExpressionEncoderSuite$OuterLevelWithVeryVeryVeryLongClassNam$$$$85f068777e7ecf112afcbe997d461b$$$$VeryLongClassName11$OuterLevelWithVeryVeryVeryLongClassName12$OuterLevelWithVeryVeryVeryLongClassName13$
org.apache.spark.sql.catalyst.encoders.ExpressionEncoderSuite$OuterLevelWithVeryVeryVeryLongClassNam$$$$85f068777e7ecf112afcbe997d461b$$$$VeryLongClassName11$OuterLevelWithVeryVeryVeryLongClassName12$OuterLevelWithVeryVeryVeryLongClassName13$OuterLevelWithVeryVeryVeryLongClassName14$
org.apache.spark.sql.catalyst.encoders.ExpressionEncoderSuite$OuterLevelWithVeryVeryVeryLongClassNam$$$$5f7ad51804cb1be53938ea804699fa$$$$VeryLongClassName14$OuterLevelWithVeryVeryVeryLongClassName15$
org.apache.spark.sql.catalyst.encoders.ExpressionEncoderSuite$OuterLevelWithVeryVeryVeryLongClassNam$$$$5f7ad51804cb1be53938ea804699fa$$$$VeryLongClassName14$OuterLevelWithVeryVeryVeryLongClassName15$OuterLevelWithVeryVeryVeryLongClassName16$
org.apache.spark.sql.catalyst.encoders.ExpressionEncoderSuite$OuterLevelWithVeryVeryVeryLongClassNam$$$$5f7ad51804cb1be53938ea804699fa$$$$VeryLongClassName14$OuterLevelWithVeryVeryVeryLongClassName15$OuterLevelWithVeryVeryVeryLongClassName16$OuterLevelWithVeryVeryVeryLongClassName17$
org.apache.spark.sql.catalyst.encoders.ExpressionEncoderSuite$OuterLevelWithVeryVeryVeryLongClassNam$$$$69b54f16b1965a31e88968df1a58d8$$$$VeryLongClassName17$OuterLevelWithVeryVeryVeryLongClassName18$
org.apache.spark.sql.catalyst.encoders.ExpressionEncoderSuite$OuterLevelWithVeryVeryVeryLongClassNam$$$$69b54f16b1965a31e88968df1a58d8$$$$VeryLongClassName17$OuterLevelWithVeryVeryVeryLongClassName18$OuterLevelWithVeryVeryVeryLongClassName19$
org.apache.spark.sql.catalyst.encoders.ExpressionEncoderSuite$OuterLevelWithVeryVeryVeryLongClassNam$$$$69b54f16b1965a31e88968df1a58d8$$$$VeryLongClassName17$OuterLevelWithVeryVeryVeryLongClassName18$OuterLevelWithVeryVeryVeryLongClassName19$OuterLevelWithVeryVeryVeryLongClassName20$
```
with a hash code in the middle and various levels of nesting omitted.
The `java.lang.Class.isMemberClass` method is implemented in JDK8u as:
http://hg.openjdk.java.net/jdk8u/jdk8u/jdk/file/tip/src/share/classes/java/lang/Class.java#l1425
```
/**
* Returns {code true} if and only if the underlying class
* is a member class.
*
* return {code true} if and only if this class is a member class.
* since 1.5
*/
public boolean isMemberClass() {
return getSimpleBinaryName() != null && !isLocalOrAnonymousClass();
}
/**
* Returns the "simple binary name" of the underlying class, i.e.,
* the binary name without the leading enclosing class name.
* Returns {code null} if the underlying class is a top level
* class.
*/
private String getSimpleBinaryName() {
Class<?> enclosingClass = getEnclosingClass();
if (enclosingClass == null) // top level class
return null;
// Otherwise, strip the enclosing class' name
try {
return getName().substring(enclosingClass.getName().length());
} catch (IndexOutOfBoundsException ex) {
throw new InternalError("Malformed class name", ex);
}
}
```
and the problematic code is `getName().substring(enclosingClass.getName().length())` -- if a class's enclosing class's full name is *longer* than the nested class's full name, this logic would end up going out of bounds.
The bug has been fixed in JDK9 by https://bugs.java.com/bugdatabase/view_bug.do?bug_id=8057919 , but still exists in the latest JDK8u release. So from the Spark side we'd need to do something to avoid hitting this problem.
### Why are the changes needed?
Bugfix on jdk8u.
### Does this PR introduce _any_ user-facing change?
No.
### How was this patch tested?
Added tests.
Closes#31733 from maropu/SPARK-34607.
Authored-by: Takeshi Yamamuro <yamamuro@apache.org>
Signed-off-by: Takeshi Yamamuro <yamamuro@apache.org>
### What changes were proposed in this pull request?
In the PR, I propose to extend Spark SQL API to accept [`java.time.Duration`](https://docs.oracle.com/javase/8/docs/api/java/time/Duration.html) as an external type of recently added new Catalyst type - `DayTimeIntervalType` (see #31614). The Java class `java.time.Duration` has similar semantic to ANSI SQL day-time interval type, and it is the most suitable to be an external type for `DayTimeIntervalType`. In more details:
1. Added `DurationConverter` which converts `java.time.Duration` instances to/from internal representation of the Catalyst type `DayTimeIntervalType` (to `Long` type). The `DurationConverter` object uses new methods of `IntervalUtils`:
- `durationToMicros()` converts the input duration to the total length in microseconds. If this duration is too large to fit `Long`, the method throws the exception `ArithmeticException`. **Note:** _the input duration has nanosecond precision, the method casts the nanos part to microseconds by dividing by 1000._
- `microsToDuration()` obtains a `java.time.Duration` representing a number of microseconds.
2. Support new type `DayTimeIntervalType` in `RowEncoder` via the methods `createDeserializerForDuration()` and `createSerializerForJavaDuration()`.
3. Extended the Literal API to construct literals from `java.time.Duration` instances.
### Why are the changes needed?
1. To allow users parallelization of `java.time.Duration` collections, and construct day-time interval columns. Also to collect such columns back to the driver side.
2. This will allow to write tests in other sub-tasks of SPARK-27790.
### Does this PR introduce _any_ user-facing change?
The PR extends existing functionality. So, users can parallelize instances of the `java.time.Duration` class and collect them back:
```Scala
scala> val ds = Seq(java.time.Duration.ofDays(10)).toDS
ds: org.apache.spark.sql.Dataset[java.time.Duration] = [value: daytimeinterval]
scala> ds.collect
res0: Array[java.time.Duration] = Array(PT240H)
```
### How was this patch tested?
- Added a few tests to `CatalystTypeConvertersSuite` to check conversion from/to `java.time.Duration`.
- Checking row encoding by new tests in `RowEncoderSuite`.
- Making literals of `DayTimeIntervalType` are tested in `LiteralExpressionSuite`
- Check collecting by `DatasetSuite` and `JavaDatasetSuite`.
Closes#31729 from MaxGekk/java-time-duration.
Authored-by: Max Gekk <max.gekk@gmail.com>
Signed-off-by: Wenchen Fan <wenchen@databricks.com>
### What changes were proposed in this pull request?
In ANSI mode, casting String to Boolean should throw an exception on parse error, instead of returning null
### Why are the changes needed?
For better ANSI compliance
### Does this PR introduce _any_ user-facing change?
Yes, in ANSI mode there will be an exception on parse failure of casting String value to Boolean type.
### How was this patch tested?
Unit tests.
Closes#31734 from gengliangwang/ansiCastToBoolean.
Authored-by: Gengliang Wang <gengliang.wang@databricks.com>
Signed-off-by: Gengliang Wang <gengliang.wang@databricks.com>
### What changes were proposed in this pull request?
`ResolveInsertInto.staticDeleteExpression` should use `UnresolvedAttribute.quoted` to create the delete expression so that we will treat the entire `attr.name` as a column name.
### Why are the changes needed?
When users use `dot` in a partition column name, queries like ```INSERT OVERWRITE $t1 PARTITION (`a.b` = 'a') (`c.d`) VALUES('b')``` is not working.
### Does this PR introduce _any_ user-facing change?
Without this test, the above query will throw
```
[info] org.apache.spark.sql.AnalysisException: cannot resolve '`a.b`' given input columns: [a.b, c.d];
[info] 'OverwriteByExpression RelationV2[a.b#17, c.d#18] default.tbl, ('a.b <=> cast(a as string)), false
[info] +- Project [a.b#19, ansi_cast(col1#16 as string) AS c.d#20]
[info] +- Project [cast(a as string) AS a.b#19, col1#16]
[info] +- LocalRelation [col1#16]
```
With the fix, the query will run correctly.
### How was this patch tested?
The new added test.
Closes#31713 from zsxwing/SPARK-34599.
Authored-by: Shixiong Zhu <zsxwing@gmail.com>
Signed-off-by: Wenchen Fan <wenchen@databricks.com>
### What changes were proposed in this pull request?
In the PR, I propose to generate "stable" output attributes per the logical node of the DESCRIBE NAMESPACE command.
### Why are the changes needed?
This fixes the issue demonstrated by the example:
```
sql(s"CREATE NAMESPACE ns")
val description = sql(s"DESCRIBE NAMESPACE ns")
description.drop("name")
```
```
[info] org.apache.spark.sql.AnalysisException: Resolved attribute(s) name#74 missing from name#25,value#26 in operator !Project [name#74]. Attribute(s) with the same name appear in the operation: name. Please check if the right attribute(s) are used.;
[info] !Project [name#74]
[info] +- LocalRelation [name#25, value#26]
```
### Does this PR introduce _any_ user-facing change?
After this change user `drop()/add()` works well.
### How was this patch tested?
Added UT
Closes#31705 from AngersZhuuuu/SPARK-34577.
Authored-by: Angerszhuuuu <angers.zhu@gmail.com>
Signed-off-by: Wenchen Fan <wenchen@databricks.com>
### What changes were proposed in this pull request?
This is a followup of https://github.com/apache/spark/pull/27597 and simply apply the fix in the v2 table insertion code path.
### Why are the changes needed?
bug fix
### Does this PR introduce _any_ user-facing change?
yes, now v2 table insertion with static partitions also follow StoreAssignmentPolicy.
### How was this patch tested?
moved the test from https://github.com/apache/spark/pull/27597 to the general test suite `SQLInsertTestSuite`, which covers DS v2, file source, and hive tables.
Closes#31726 from cloud-fan/insert.
Authored-by: Wenchen Fan <wenchen@databricks.com>
Signed-off-by: HyukjinKwon <gurwls223@apache.org>
### What changes were proposed in this pull request?
Use a non-recursive implementation for the function buildBalancedPredicate
### Why are the changes needed?
For better performance.
### Does this PR introduce _any_ user-facing change?
No
### How was this patch tested?
Existing unit tests.
Also, a quick benchmark:
```
test("buildBalancedPredicate") {
val expressions = (1 to 1000).map(_ => Literal(true))
val start = System.currentTimeMillis()
buildBalancedPredicate(expressions, And)
println(System.currentTimeMillis() - start)
}
```
Before: 47ms
After: 4ms
Closes#31724 from gengliangwang/nonrecursive.
Authored-by: Gengliang Wang <gengliang.wang@databricks.com>
Signed-off-by: Gengliang Wang <gengliang.wang@databricks.com>
### What changes were proposed in this pull request?
Add metadataOutput as a fallback to resolution.
Builds off https://github.com/apache/spark/pull/31654.
### Why are the changes needed?
The metadata columns could not be resolved via `df.col("metadataColName")` from the DataFrame API.
### Does this PR introduce _any_ user-facing change?
Yes, the metadata columns can now be resolved as described above.
### How was this patch tested?
Scala unit test.
Closes#31668 from karenfeng/spark-34555.
Authored-by: Karen Feng <karen.feng@databricks.com>
Signed-off-by: Wenchen Fan <wenchen@databricks.com>
### What changes were proposed in this pull request?
Hive support type constructed value as partition spec value, spark should support too.
### Why are the changes needed?
Support TypeConstructed partition spec value keep same with hive
### Does this PR introduce _any_ user-facing change?
Yes, user can use TypeConstruct value as partition spec value such as
```
CREATE TABLE t1(name STRING) PARTITIONED BY (part DATE)
INSERT INTO t1 PARTITION(part = date'2019-01-02') VALUES('a')
CREATE TABLE t2(name STRING) PARTITIONED BY (part TIMESTAMP)
INSERT INTO t2 PARTITION(part = timestamp'2019-01-02 11:11:11') VALUES('a')
CREATE TABLE t4(name STRING) PARTITIONED BY (part BINARY)
INSERT INTO t4 PARTITION(part = X'537061726B2053514C') VALUES('a')
```
### How was this patch tested?
Added UT
Closes#30421 from AngersZhuuuu/SPARK-33474.
Lead-authored-by: angerszhu <angers.zhu@gmail.com>
Co-authored-by: Angerszhuuuu <angers.zhu@gmail.com>
Co-authored-by: AngersZhuuuu <angers.zhu@gmail.com>
Signed-off-by: Takeshi Yamamuro <yamamuro@apache.org>
### What changes were proposed in this pull request?
spark.sql.adaptive.coalescePartitions.initialPartitionNum 200 -> (none)
spark.sql.adaptive.skewJoin.skewedPartitionFactor is 10 -> 5
### Why are the changes needed?
the wrong doc misguide people
### Does this PR introduce _any_ user-facing change?
no
### How was this patch tested?
passing doc
Closes#31717 from yaooqinn/minordoc0.
Authored-by: Kent Yao <yao@apache.org>
Signed-off-by: HyukjinKwon <gurwls223@apache.org>
### What changes were proposed in this pull request?
Enhance boolean simplification rule by handling following scenarios:
(((a && b) && a && (a && c))) => a && b && c)
(((a || b) || a || (a || c))) => a || b || c
### Why are the changes needed?
Minor improvement
### Does this PR introduce _any_ user-facing change?
No
### How was this patch tested?
Added UTs
Closes#31318 from Swinky/booleansimplification.
Authored-by: Swinky <mannswinky@gmail.com>
Signed-off-by: Wenchen Fan <wenchen@databricks.com>
### What changes were proposed in this pull request?
In the PR, I propose to generate "stable" output attributes per the logical node of the DESCRIBE COLUMN command.
### Why are the changes needed?
This fixes the issue demonstrated by the example:
```
val tbl = "testcat.ns1.ns2.tbl"
sql(s"CREATE TABLE $tbl (c0 INT) USING _")
val description = sql(s"DESCRIBE TABLE $tbl c0")
description.drop("info_name")
```
```
[info] org.apache.spark.sql.AnalysisException: Resolved attribute(s) info_name#74 missing from info_name#25,info_value#26 in operator !Project [info_name#74]. Attribute(s) with the same name appear in the operation: info_name. Please check if the right attribute(s) are used.;
[info] !Project [info_name#74]
[info] +- LocalRelation [info_name#25, info_value#26]
```
### Does this PR introduce _any_ user-facing change?
After this change user `drop()/add()` works well.
### How was this patch tested?
Added UT
Closes#31696 from AngersZhuuuu/SPARK-34576.
Authored-by: Angerszhuuuu <angers.zhu@gmail.com>
Signed-off-by: Wenchen Fan <wenchen@databricks.com>
### What changes were proposed in this pull request?
In the PR, I propose to extend Catalyst's type system by two new types that conform to the SQL standard (see SQL:2016, section 4.6.3):
- `DayTimeIntervalType` represents the day-time interval type,
- `YearMonthIntervalType` for SQL year-month interval type.
This PR only adds the two new DataType implementations, and there will be more PRs as sub-tasks of SPARK-27790 to completely support the new ANSI interval types.
### Why are the changes needed?
Spark as it is today supports an INTERVAL datatype. However this type is of very limited use. Existing interval values cannot be compared with any other interval values, or persisted to storage. Spark users request to either implement new or expand existing built-in functions which produce some sort of measures for elapsed time, such as `DATEDIFF()`. Rather than work around the edges to fill the potholes of the existing INTERVAL data type, I would like to propose to deliver a proper ANSI compliant INTERVAL type that can be introduced with minimal incompatibility, is comparable and thus sortable, and can be persisted in tables.
### Does this PR introduce _any_ user-facing change?
No
### How was this patch tested?
1. By checking coding style via:
```
$ ./dev/scalastyle
$ ./dev/lint-java
```
2. Run the test for the default sizes:
```
$ build/sbt "test:testOnly *DataTypeSuite"
```
Closes#31614 from MaxGekk/day-time-interval-type.
Authored-by: Max Gekk <max.gekk@gmail.com>
Signed-off-by: Wenchen Fan <wenchen@databricks.com>
### What changes were proposed in this pull request?
Use `Utils.getSimpleName` to avoid hitting `Malformed class name` error in `NewInstance.doGenCode`.
### Why are the changes needed?
On older JDK versions (e.g. JDK8u), nested Scala classes may trigger `java.lang.Class.getSimpleName` to throw an `java.lang.InternalError: Malformed class name` error.
In this particular case, creating an `ExpressionEncoder` on such a nested Scala class would create a `NewInstance` expression under the hood, which will trigger the problem during codegen.
Similar to https://github.com/apache/spark/pull/29050, we should use Spark's `Utils.getSimpleName` utility function in place of `Class.getSimpleName` to avoid hitting the issue.
There are two other occurrences of `java.lang.Class.getSimpleName` in the same file, but they're safe because they're only guaranteed to be only used on Java classes, which don't have this problem, e.g.:
```scala
// Make a copy of the data if it's unsafe-backed
def makeCopyIfInstanceOf(clazz: Class[_ <: Any], value: String) =
s"$value instanceof ${clazz.getSimpleName}? ${value}.copy() : $value"
val genFunctionValue: String = lambdaFunction.dataType match {
case StructType(_) => makeCopyIfInstanceOf(classOf[UnsafeRow], genFunction.value)
case ArrayType(_, _) => makeCopyIfInstanceOf(classOf[UnsafeArrayData], genFunction.value)
case MapType(_, _, _) => makeCopyIfInstanceOf(classOf[UnsafeMapData], genFunction.value)
case _ => genFunction.value
}
```
The Unsafe-* family of types are all Java types, so they're okay.
### Does this PR introduce _any_ user-facing change?
Fixes a bug that throws an error when using `ExpressionEncoder` on some nested Scala types, otherwise no changes.
### How was this patch tested?
Added a test case to `org.apache.spark.sql.catalyst.encoders.ExpressionEncoderSuite`. It'll fail on JDK8u before the fix, and pass after the fix.
Closes#31709 from rednaxelafx/spark-34596-master.
Authored-by: Kris Mok <kris.mok@databricks.com>
Signed-off-by: HyukjinKwon <gurwls223@apache.org>
### What changes were proposed in this pull request?
Call `toSeq` to fix Scala 2.13 build error.
### Why are the changes needed?
It is needed to fix 2.13 build error.
### Does this PR introduce _any_ user-facing change?
No.
### How was this patch tested?
Existing tests.
Closes#31716 from viirya/SPARK-34548-followup.
Authored-by: Liang-Chi Hsieh <viirya@gmail.com>
Signed-off-by: HyukjinKwon <gurwls223@apache.org>
### What changes were proposed in this pull request?
This patch proposes to remove unnecessary children from Union under Distince and Deduplicate
### Why are the changes needed?
If there are any duplicate child of `Union` under `Distinct` and `Deduplicate`, it can be removed to simplify query plan.
### Does this PR introduce _any_ user-facing change?
No
### How was this patch tested?
Unit test
Closes#31656 from viirya/SPARK-34548.
Authored-by: Liang-Chi Hsieh <viirya@gmail.com>
Signed-off-by: Liang-Chi Hsieh <viirya@gmail.com>
### What changes were proposed in this pull request?
Today, child expressions may be resolved based on "real" or metadata output attributes. We should prefer the real attribute during resolution if one exists.
### Why are the changes needed?
Today, attempting to resolve an expression when there is a "real" output attribute and a metadata attribute with the same name results in resolution failure. This is likely unexpected, as the user may not know about the metadata attribute.
### Does this PR introduce _any_ user-facing change?
Yes. Previously, the user would see an error message when resolving a column with the same name as a "real" output attribute and a metadata attribute as below:
```
org.apache.spark.sql.AnalysisException: Reference 'index' is ambiguous, could be: testcat.ns1.ns2.tableTwo.index, testcat.ns1.ns2.tableOne.index.; line 1 pos 71
at org.apache.spark.sql.catalyst.expressions.package$AttributeSeq.resolve(package.scala:363)
at org.apache.spark.sql.catalyst.plans.logical.LogicalPlan.resolveChildren(LogicalPlan.scala:107)
```
Now, resolution succeeds and provides the "real" output attribute.
### How was this patch tested?
Added a unit test.
Closes#31654 from karenfeng/fallback-resolve-metadata.
Authored-by: Karen Feng <karen.feng@databricks.com>
Signed-off-by: Wenchen Fan <wenchen@databricks.com>
### Why is this change being proposed?
This patch adds support for a new "product" aggregation function in `sql.functions` which multiplies-together all values in an aggregation group.
This is likely to be useful in statistical applications which involve combining probabilities, or financial applications that involve combining cumulative interest rates, but is also a versatile mathematical operation of similar status to `sum` or `stddev`. Other users [have noted](https://stackoverflow.com/questions/52991640/cumulative-product-in-spark) the absence of such a function in current releases of Spark.
This function is both much more concise than an expression of the form `exp(sum(log(...)))`, and avoids awkward edge-cases associated with some values being zero or negative, as well as being less computationally costly.
### Does this PR introduce _any_ user-facing change?
No - only adds new function.
### How was this patch tested?
Built-in tests have been added for the new `catalyst.expressions.aggregate.Product` class and its invocation via the (scala) `sql.functions.product` function. The latter, and the PySpark wrapper have also been manually tested in spark-shell and pyspark sessions. The SparkR wrapper is currently untested, and may need separate validation (I'm not an "R" user myself).
An illustration of the new functionality, within PySpark is as follows:
```
import pyspark.sql.functions as pf, pyspark.sql.window as pw
df = sqlContext.range(1, 17).toDF("x")
win = pw.Window.partitionBy(pf.lit(1)).orderBy(pf.col("x"))
df.withColumn("factorial", pf.product("x").over(win)).show(20, False)
+---+---------------+
|x |factorial |
+---+---------------+
|1 |1.0 |
|2 |2.0 |
|3 |6.0 |
|4 |24.0 |
|5 |120.0 |
|6 |720.0 |
|7 |5040.0 |
|8 |40320.0 |
|9 |362880.0 |
|10 |3628800.0 |
|11 |3.99168E7 |
|12 |4.790016E8 |
|13 |6.2270208E9 |
|14 |8.71782912E10 |
|15 |1.307674368E12 |
|16 |2.0922789888E13|
+---+---------------+
```
Closes#30745 from rwpenney/feature/agg-product.
Lead-authored-by: Richard Penney <rwp@rwpenney.uk>
Co-authored-by: Richard Penney <rwpenney@users.noreply.github.com>
Signed-off-by: HyukjinKwon <gurwls223@apache.org>
### What changes were proposed in this pull request?
In the `SQLConf` object, the `sqlConfEntries` map is globally synchronized (it is a Java `Collections.synchronizedMap`): any operation, including a get, will need to acquire the lock.
An example of this is calling the `DatatType.sameType` method. This will trigger a check on `SQLConf.get.caseSensitiveAnalysis`. So every time we compare two datatypes with sameType, we hit a lock.
To avoid having multiple tasks locking on this, a better approach would be to use a map that does not lock on read (like a `ConcurrentHashMap`). This map implementation does not lock on read, and on write it only locks the map partially. The only lock that happens is on write on the same map key.
### Why are the changes needed?
Multiple tasks performing any operation that directly or indirectly trigger a query to the `SQLConf.sqlConfEntries` map, will require acquiring a global lock on that map. Something as easy as calling `DataType.sameType(...)` would be locking on the global `sqlConfEntries` lock of the `Collections.synchronizedMap`.
### Does this PR introduce _any_ user-facing change?
No
### How was this patch tested?
No functionality change. Existing unit tests run normally.
Closes#31689 from gabrielenizzoli/SPARK-34573.
Authored-by: Gabriele Nizzoli <1545350+gabrielenizzoli@users.noreply.github.com>
Signed-off-by: Takeshi Yamamuro <yamamuro@apache.org>
### What changes were proposed in this pull request?
In the PR, I propose to generate unique attributes in the logical nodes of the `SHOW TABLES` command.
Also, this PR fixes similar issues in other logical nodes:
- ShowTableExtended
- ShowViews
- ShowTableProperties
- ShowFunctions
- ShowColumns
- ShowPartitions
- ShowNamespaces
### Why are the changes needed?
This fixes the issue which is demonstrated by the example below:
```scala
scala> val show1 = sql("SHOW TABLES IN ns1")
show1: org.apache.spark.sql.DataFrame = [namespace: string, tableName: string ... 1 more field]
scala> val show2 = sql("SHOW TABLES IN ns2")
show2: org.apache.spark.sql.DataFrame = [namespace: string, tableName: string ... 1 more field]
scala> show1.show
+---------+---------+-----------+
|namespace|tableName|isTemporary|
+---------+---------+-----------+
| ns1| tbl1| false|
+---------+---------+-----------+
scala> show2.show
+---------+---------+-----------+
|namespace|tableName|isTemporary|
+---------+---------+-----------+
| ns2| tbl2| false|
+---------+---------+-----------+
scala> show1.join(show2).where(show1("tableName") =!= show2("tableName")).show
org.apache.spark.sql.AnalysisException: Column tableName#17 are ambiguous. It's probably because you joined several Datasets together, and some of these Datasets are the same. This column points to one of the Datasets but Spark is unable to figure out which one. Please alias the Datasets with different names via `Dataset.as` before joining them, and specify the column using qualified name, e.g. `df.as("a").join(df.as("b"), $"a.id" > $"b.id")`. You can also set spark.sql.analyzer.failAmbiguousSelfJoin to false to disable this check.
at org.apache.spark.sql.execution.analysis.DetectAmbiguousSelfJoin$.apply(DetectAmbiguousSelfJoin.scala:157)
```
### Does this PR introduce _any_ user-facing change?
Yes. After the changes, the example above works as expected:
```scala
scala> show1.join(show2).where(show1("tableName") =!= show2("tableName")).show
+---------+---------+-----------+---------+---------+-----------+
|namespace|tableName|isTemporary|namespace|tableName|isTemporary|
+---------+---------+-----------+---------+---------+-----------+
| ns1| tbl1| false| ns2| tbl2| false|
+---------+---------+-----------+---------+---------+-----------+
```
### How was this patch tested?
By running the new test:
```
$ build/sbt -Phive-2.3 -Phive-thriftserver "test:testOnly *ShowTablesSuite"
```
Closes#31675 from MaxGekk/fix-output-attrs.
Authored-by: Max Gekk <max.gekk@gmail.com>
Signed-off-by: Wenchen Fan <wenchen@databricks.com>
### What changes were proposed in this pull request?
In the PR, I propose to generate "stable" output attributes per the logical node of the `DESCRIBE TABLE` command.
### Why are the changes needed?
This fixes the issue demonstrated by the example:
```scala
val tbl = "testcat.ns1.ns2.tbl"
sql(s"CREATE TABLE $tbl (c0 INT) USING _")
val description = sql(s"DESCRIBE TABLE $tbl")
description.drop("comment")
```
The `drop()` method fails with the error:
```
org.apache.spark.sql.AnalysisException: Resolved attribute(s) col_name#102,data_type#103 missing from col_name#29,data_type#30,comment#31 in operator !Project [col_name#102, data_type#103]. Attribute(s) with the same name appear in the operation: col_name,data_type. Please check if the right attribute(s) are used.;
!Project [col_name#102, data_type#103]
+- LocalRelation [col_name#29, data_type#30, comment#31]
at org.apache.spark.sql.catalyst.analysis.CheckAnalysis.failAnalysis(CheckAnalysis.scala:51)
at org.apache.spark.sql.catalyst.analysis.CheckAnalysis.failAnalysis$(CheckAnalysis.scala:50)
```
### Does this PR introduce _any_ user-facing change?
Yes. After the changes, `drop()`/`add()` works as expected:
```scala
description.drop("comment").show()
+---------------+---------+
| col_name|data_type|
+---------------+---------+
| c0| int|
| | |
| # Partitioning| |
|Not partitioned| |
+---------------+---------+
```
### How was this patch tested?
1. Run new test:
```
$ build/sbt -Phive-2.3 -Phive-thriftserver "test:testOnly *DataSourceV2SQLSuite"
```
2. Run existing test suite:
```
$ build/sbt -Phive-2.3 -Phive-thriftserver "test:testOnly *CatalogedDDLSuite"
```
Closes#31676 from MaxGekk/describe-table-drop-column.
Authored-by: Max Gekk <max.gekk@gmail.com>
Signed-off-by: Wenchen Fan <wenchen@databricks.com>
### What changes were proposed in this pull request?
This PR makes partition spec parsing respect case sensitive conf.
### Why are the changes needed?
When parsing the partition spec, Spark will call `org.apache.spark.sql.catalyst.parser.ParserUtils.checkDuplicateKeys` to check if there are duplicate partition column names in the list. But this method is always case sensitive and doesn't detect duplicate partition column names when using different cases.
### Does this PR introduce _any_ user-facing change?
Yep. This prevents users from writing incorrect queries such as `INSERT OVERWRITE t PARTITION (c='2', C='3') VALUES (1)` when they don't enable case sensitive conf.
### How was this patch tested?
The new added test will fail without this change.
Closes#31669 from zsxwing/SPARK-34556.
Authored-by: Shixiong Zhu <zsxwing@gmail.com>
Signed-off-by: HyukjinKwon <gurwls223@apache.org>
### What changes were proposed in this pull request?
Avro add zstandard codec since AVRO-2195. This pr add zstandard codec to Avro compression codec list.
### Why are the changes needed?
To make Avro support zstandard codec.
### Does this PR introduce _any_ user-facing change?
No.
### How was this patch tested?
Unit test.
Closes#31673 from wangyum/SPARK-34479.
Authored-by: Yuming Wang <yumwang@ebay.com>
Signed-off-by: Dongjoon Hyun <dongjoon@apache.org>
### What changes were proposed in this pull request?
To support +8:00 in Spark3 when execute sql
`select to_utc_timestamp("2020-02-07 16:00:00", "GMT+8:00")`
### Why are the changes needed?
+8:00 this format is supported in PostgreSQL,hive, presto, but not supported in Spark3
https://issues.apache.org/jira/browse/SPARK-34392
### Does this PR introduce _any_ user-facing change?
no
### How was this patch tested?
unit test
Closes#31624 from Karl-WangSK/zone.
Lead-authored-by: ShiKai Wang <wskqing@gmail.com>
Co-authored-by: Karl-WangSK <shikai.wang@linkflowtech.com>
Signed-off-by: Sean Owen <srowen@gmail.com>
### What changes were proposed in this pull request?
Add more aggregate expressions to `EliminateDistinct` rule.
### Why are the changes needed?
Distinct aggregation can add a significant overhead. It's better to remove distinct whenever possible.
### Does this PR introduce _any_ user-facing change?
No
### How was this patch tested?
UT
Closes#30999 from tanelk/SPARK-33971_eliminate_distinct.
Authored-by: tanel.kiis@gmail.com <tanel.kiis@gmail.com>
Signed-off-by: Takeshi Yamamuro <yamamuro@apache.org>
### What changes were proposed in this pull request?
Implement `ColumnarMap.copy()` by using the `copy()` method of `ColumnarArray`.
### Why are the changes needed?
To eliminate `java.lang.UnsupportedOperationException` while using `ColumnarMap`.
### Does this PR introduce _any_ user-facing change?
Yes
### How was this patch tested?
By running new tests in `ColumnarBatchSuite`.
Closes#31663 from MaxGekk/columnar-map-copy.
Authored-by: Max Gekk <max.gekk@gmail.com>
Signed-off-by: HyukjinKwon <gurwls223@apache.org>
### What changes were proposed in this pull request?
This PR simplifies the resolution of v2 partition commands:
1. Add a common trait for v2 partition commands, so that we don't need to match them one by one in the rules.
2. Make partition spec an expression, so that it's easier to resolve them via tree node transformation.
3. Add `TruncatePartition` so that `TruncateTable` doesn't need to be a v2 partition command.
4. Simplify `CheckAnalysis` to only check if the table is partitioned. For partitioned tables, partition spec is always resolved, so we don't need to check it. The `SupportsAtomicPartitionManagement` check is also done in the runtime. Since Spark eagerly executes commands, exception in runtime will also be thrown at analysis time.
### Why are the changes needed?
code cleanup
### Does this PR introduce _any_ user-facing change?
no
### How was this patch tested?
existing tests
Closes#31637 from cloud-fan/simplify.
Authored-by: Wenchen Fan <wenchen@databricks.com>
Signed-off-by: Wenchen Fan <wenchen@databricks.com>
### What changes were proposed in this pull request?
This patch proposes to let optimizer to remove unnecessary `Union` under `Distinct`/`Deduplicate`.
### Why are the changes needed?
For an `Union` under `Distinct`/`Deduplicate`, if its children are all the same, we can just keep one among them and remove the `Union`.
### Does this PR introduce _any_ user-facing change?
No
### How was this patch tested?
Unit tests.
Closes#31595 from viirya/remove-union.
Authored-by: Liang-Chi Hsieh <viirya@gmail.com>
Signed-off-by: Liang-Chi Hsieh <viirya@gmail.com>
### What changes were proposed in this pull request?
I found out during code review of https://github.com/apache/spark/pull/31567#discussion_r577379572, where we can push down limit to the left side of LEFT SEMI and LEFT ANTI join, if the join condition is empty.
Why it's safe to push down limit:
The semantics of LEFT SEMI join without condition:
(1). if right side is non-empty, output all rows from left side.
(2). if right side is empty, output nothing.
The semantics of LEFT ANTI join without condition:
(1). if right side is non-empty, output nothing.
(2). if right side is empty, output all rows from left side.
With the semantics of output all rows from left side or nothing (all or nothing), it's safe to push down limit to left side.
NOTE: LEFT SEMI / LEFT ANTI join with non-empty condition is not safe for limit push down, because output can be a portion of left side rows.
Reference: physical operator implementation for LEFT SEMI / LEFT ANTI join without condition - https://github.com/apache/spark/blob/master/sql/core/src/main/scala/org/apache/spark/sql/execution/joins/BroadcastNestedLoopJoinExec.scala#L200-L204 .
### Why are the changes needed?
Better performance. Save CPU and IO for these joins, as limit being pushed down before join.
### Does this PR introduce _any_ user-facing change?
No.
### How was this patch tested?
Added unit test in `LimitPushdownSuite.scala` and `SQLQuerySuite.scala`.
Closes#31630 from c21/limit-pushdown.
Authored-by: Cheng Su <chengsu@fb.com>
Signed-off-by: Wenchen Fan <wenchen@databricks.com>
### What changes were proposed in this pull request?
This PR follows up https://github.com/apache/spark/pull/30717
Maybe some contributors don't know the job and added some exception by the old way.
### Why are the changes needed?
It will largely help with standardization of error messages and its maintenance.
### Does this PR introduce _any_ user-facing change?
No. Error messages remain unchanged.
### How was this patch tested?
No new tests - pass all original tests to make sure it doesn't break any existing behavior.
Closes#31316 from beliefer/SPARK-33599-followup.
Lead-authored-by: beliefer <beliefer@163.com>
Co-authored-by: gengjiaan <gengjiaan@360.cn>
Co-authored-by: Jiaan Geng <beliefer@163.com>
Signed-off-by: Wenchen Fan <wenchen@databricks.com>
### What changes were proposed in this pull request?
This PR proposes to make `CreateViewStatement.child` to be `LogicalPlan`'s `children` so that it's resolved in the analyze phase.
### Why are the changes needed?
Currently, the `CreateViewStatement.child` is resolved when the create view command runs, which is inconsistent with other plan resolutions. For example, you may see the following in the physical plan:
```
== Physical Plan ==
Execute CreateViewCommand (1)
+- CreateViewCommand (2)
+- Project (4)
+- UnresolvedRelation (3)
```
### Does this PR introduce _any_ user-facing change?
Yes. For the example, you will now see the resolved plan:
```
== Physical Plan ==
Execute CreateViewCommand (1)
+- CreateViewCommand (2)
+- Project (5)
+- SubqueryAlias (4)
+- LogicalRelation (3)
```
### How was this patch tested?
Updated existing tests.
Closes#31273 from imback82/spark-34152.
Authored-by: Terry Kim <yuminkim@gmail.com>
Signed-off-by: Wenchen Fan <wenchen@databricks.com>
### What changes were proposed in this pull request?
In Spark ANSI mode, the type coercion rules are based on the type precedence lists of the input data types.
As per the section "Type precedence list determination" of "ISO/IEC 9075-2:2011
Information technology — Database languages - SQL — Part 2: Foundation (SQL/Foundation)", the type precedence lists of primitive data types are as following:
- Byte: Byte, Short, Int, Long, Decimal, Float, Double
- Short: Short, Int, Long, Decimal, Float, Double
- Int: Int, Long, Decimal, Float, Double
- Long: Long, Decimal, Float, Double
- Decimal: Any wider Numeric type
- Float: Float, Double
- Double: Double
- String: String
- Date: Date, Timestamp
- Timestamp: Timestamp
- Binary: Binary
- Boolean: Boolean
- Interval: Interval
As for complex data types, Spark will determine the precedent list recursively based on their sub-types.
With the definition of type precedent list, the general type coercion rules are as following:
- Data type S is allowed to be implicitly cast as type T iff T is in the precedence list of S
- Comparison is allowed iff the data type precedence list of both sides has at least one common element. When evaluating the comparison, Spark casts both sides as the tightest common data type of their precedent lists.
- There should be at least one common data type among all the children's precedence lists for the following operators. The data type of the operator is the tightest common precedent data type.
```
In, Except(odd), Intersect, Greatest, Least, Union, If, CaseWhen, CreateArray, Array Concat,Sequence, MapConcat, CreateMap
```
- For complex types (struct, array, map), Spark recursively looks into the element type and applies the rules above. If the element nullability is converted from true to false, add runtime null check to the elements.
Note: this new type coercion system will allow implicit converting String type literals as other primitive types, in case of breaking too many existing Spark SQL queries. This is a special rule and it is not from the ANSI SQL standard.
### Why are the changes needed?
The current type coercion rules are complex. Also, they are very hard to describe and understand. For details please refer the attached documentation "Default Type coercion rules of Spark"
[Default Type coercion rules of Spark.pdf](https://github.com/apache/spark/files/5874362/Default.Type.coercion.rules.of.Spark.pdf)
This PR is to create a new and strict type coercion system under ANSI mode. The rules are simple and clean, so that users can follow them easily
### Does this PR introduce _any_ user-facing change?
Yes, new implicit cast syntax rules in ANSI mode. All the details are in the first section of this description.
### How was this patch tested?
Unit tests
Closes#31349 from gengliangwang/ansiImplicitConversion.
Authored-by: Gengliang Wang <gengliang.wang@databricks.com>
Signed-off-by: Gengliang Wang <gengliang.wang@databricks.com>
### What changes were proposed in this pull request?
Implement the v2 execution node for the `TRUNCATE TABLE` command.
### Why are the changes needed?
To have feature parity with DS v1, and support truncation of v2 tables.
### Does this PR introduce _any_ user-facing change?
Yes
### How was this patch tested?
By running the unified tests for v1 and v2 tables:
```
$ build/sbt -Phive -Phive-thriftserver "test:testOnly *TruncateTableSuite"
```
Closes#31605 from MaxGekk/truncate-table-v2.
Authored-by: Max Gekk <max.gekk@gmail.com>
Signed-off-by: Wenchen Fan <wenchen@databricks.com>
### What changes were proposed in this pull request?
In the PR, I propose to extend the `MSCK REPAIR TABLE` command, and support new options `{ADD|DROP|SYNC} PARTITIONS`. In particular:
1. Extend the logical node `RepairTable`, and add two new flags `enableAddPartitions` and `enableDropPartitions`.
2. Add similar flags to the v1 execution node `AlterTableRecoverPartitionsCommand`
3. Add new method `dropPartitions()` to `AlterTableRecoverPartitionsCommand` which drops partitions from the catalog if their locations in the file system don't exist.
4. Updated public docs about the `MSCK REPAIR TABLE` command:
<img width="1037" alt="Screenshot 2021-02-16 at 13 46 39" src="https://user-images.githubusercontent.com/1580697/108052607-7446d280-705d-11eb-8e25-7398254787a4.png">
Closes#31097
### Why are the changes needed?
- The changes allow to recover tables with removed partitions. The example below portraits the problem:
```sql
spark-sql> create table tbl2 (col int, part int) partitioned by (part);
spark-sql> insert into tbl2 partition (part=1) select 1;
spark-sql> insert into tbl2 partition (part=0) select 0;
spark-sql> show table extended like 'tbl2' partition (part = 0);
default tbl2 false Partition Values: [part=0]
Location: file:/Users/maximgekk/proj/apache-spark/spark-warehouse/tbl2/part=0
...
```
Remove the partition (part = 0) from the filesystem:
```
$ rm -rf /Users/maximgekk/proj/apache-spark/spark-warehouse/tbl2/part=0
```
Even after recovering, we cannot query the table:
```sql
spark-sql> msck repair table tbl2;
spark-sql> select * from tbl2;
21/01/08 22:49:13 ERROR SparkSQLDriver: Failed in [select * from tbl2]
org.apache.hadoop.mapred.InvalidInputException: Input path does not exist: file:/Users/maximgekk/proj/apache-spark/spark-warehouse/tbl2/part=0
```
- To have feature parity with Hive: https://cwiki.apache.org/confluence/display/Hive/LanguageManual+DDL#LanguageManualDDL-RecoverPartitions(MSCKREPAIRTABLE)
### Does this PR introduce _any_ user-facing change?
Yes. After the changes, we can query recovered table:
```sql
spark-sql> msck repair table tbl2 sync partitions;
spark-sql> select * from tbl2;
1 1
spark-sql> show partitions tbl2;
part=1
```
### How was this patch tested?
- By running the modified test suite:
```
$ build/sbt -Phive-2.3 -Phive-thriftserver "test:testOnly *MsckRepairTableParserSuite"
$ build/sbt -Phive-2.3 -Phive-thriftserver "test:testOnly *PlanResolutionSuite"
$ build/sbt -Phive-2.3 -Phive-thriftserver "test:testOnly *AlterTableRecoverPartitionsSuite"
$ build/sbt -Phive-2.3 -Phive-thriftserver "test:testOnly *AlterTableRecoverPartitionsParallelSuite"
```
- Added unified v1 and v2 tests for `MSCK REPAIR TABLE`:
```
$ build/sbt -Phive-2.3 -Phive-thriftserver "test:testOnly *MsckRepairTableSuite"
```
Closes#31499 from MaxGekk/repair-table-drop-partitions.
Authored-by: Max Gekk <max.gekk@gmail.com>
Signed-off-by: Dongjoon Hyun <dhyun@apple.com>
### What changes were proposed in this pull request?
In the PR, I propose to rename logical nodes of v2 commands in the form: `<verb> + <object>` like:
- AlterTableAddPartition -> AddPartition
- AlterTableSetLocation -> SetTableLocation
### Why are the changes needed?
1. For simplicity and readability of logical plans
2. For consistency with other logical nodes. For example, the logical node `RenameTable` for `ALTER TABLE .. RENAME TO` was added before `AlterTableRenamePartition`.
### Does this PR introduce _any_ user-facing change?
Should not since this is non-public APIs.
### How was this patch tested?
1. Check scala style: `./dev/scalastyle`
2. Affected test suites:
```
$ build/sbt -Phive-2.3 -Phive-thriftserver "test:testOnly *AlterTableRenamePartitionSuite"
```
Closes#31596 from MaxGekk/rename-alter-table-logic-nodes.
Authored-by: Max Gekk <max.gekk@gmail.com>
Signed-off-by: Wenchen Fan <wenchen@databricks.com>
### What changes were proposed in this pull request?
When resolving a view, we use the captured view name in `AnalysisContext` to
distinguish whether a relation name is a view or a table. But if the resolution failed,
other rules (e.g. `ResolveTables`) will try to resolve the relation again but without
`AnalysisContext`. So, in this case, the resolution may be incorrect. For example,
if the view refers to a dropped table while a view with the same name exists, the
dropped table will be resolved as a view rather than an unresolved exception.
### Why are the changes needed?
bugfix
### Does this PR introduce _any_ user-facing change?
no
### How was this patch tested?
newly added test cases
Closes#31606 from linhongliu-db/fix-temp-view-master.
Lead-authored-by: Linhong Liu <linhong.liu@databricks.com>
Co-authored-by: Linhong Liu <67896261+linhongliu-db@users.noreply.github.com>
Signed-off-by: Wenchen Fan <wenchen@databricks.com>
### What changes were proposed in this pull request?
In the PR, I propose to revert https://github.com/apache/spark/pull/26659 partially regarding to comparability of interval values. The comment became incorrect after https://github.com/apache/spark/pull/27262.
### Why are the changes needed?
The comment is incorrect, and it might confuse Spark's devs/users.
### Does this PR introduce _any_ user-facing change?
No
### How was this patch tested?
By checking scala coding style `./dev/scalastyle`.
Closes#31610 from MaxGekk/doc-interval-not-comparable.
Authored-by: Max Gekk <max.gekk@gmail.com>
Signed-off-by: Wenchen Fan <wenchen@databricks.com>
### What changes were proposed in this pull request?
1. Move parser tests from `DDLParserSuite` to `AlterTableRenameParserSuite`.
2. Port DS v1 tests from `DDLSuite` and other test suites to `v1.AlterTableRenameBase` and to `v1.AlterTableRenameSuite`.
3. Add a test for DSv2 `ALTER TABLE .. RENAME` to `v2.AlterTableRenameSuite`.
### Why are the changes needed?
To improve test coverage.
### Does this PR introduce _any_ user-facing change?
No
### How was this patch tested?
By running new test suites:
```
$ build/sbt -Phive-2.3 -Phive-thriftserver "test:testOnly *AlterTableRenameSuite"
$ build/sbt -Phive-2.3 -Phive-thriftserver "test:testOnly *AlterTableRenameParserSuite"
```
Closes#31575 from MaxGekk/unify-rename-table-tests.
Authored-by: Max Gekk <max.gekk@gmail.com>
Signed-off-by: Wenchen Fan <wenchen@databricks.com>
### What changes were proposed in this pull request?
1. Add new interface `TruncatableTable` which represents tables that allow atomic truncation.
2. Implement new method in `InMemoryTable` and in `InMemoryPartitionTable`.
### Why are the changes needed?
To support `TRUNCATE TABLE` for v2 tables.
### Does this PR introduce _any_ user-facing change?
Should not.
### How was this patch tested?
Added new tests to `TableCatalogSuite` that check truncation of non-partitioned and partitioned tables:
```
$ build/sbt "test:testOnly *TableCatalogSuite"
```
Closes#31475 from MaxGekk/dsv2-truncate-table.
Authored-by: Max Gekk <max.gekk@gmail.com>
Signed-off-by: HyukjinKwon <gurwls223@apache.org>
### What changes were proposed in this pull request?
UserDefinedType and UDTRegistration become public Developer APIs, not package-private to Spark.
### Why are the changes needed?
This proposes to simply open up the UserDefinedType class as a developer API. It was public in 1.x, but closed in 2.x for some possible redesign that does not seem to have happened.
Other libraries have managed to define UDTs anyway by inserting shims into the Spark namespace, and this evidently has worked OK. But package isolation in Java 9+ breaks this.
The logic here is mostly: this is de facto a stable API, so can at least be open to developers with the usual caveats about developer APIs.
Open questions:
- Is there in fact some important redesign that's needed before opening it? The comment to this effect is from 2016
- Is this all that needs to be opened up? Like PythonUserDefinedType?
- Should any of this be kept package-private?
This was first proposed in https://github.com/apache/spark/pull/16478 though it was a larger change, but, the other API issues it was fixing seem to have been addressed already (e.g. no need to return internal Spark types). It was never really reviewed.
My hunch is that there isn't much downside, and some upside, to just opening this as-is now.
### Does this PR introduce _any_ user-facing change?
UserDefinedType becomes visible to developers to subclass.
### How was this patch tested?
Existing tests; there is no change to the existing logic.
Closes#31461 from srowen/SPARK-7768.
Authored-by: Sean Owen <srowen@gmail.com>
Signed-off-by: Sean Owen <srowen@gmail.com>
### What changes were proposed in this pull request?
Handled 'Deduplicate(Keys, Union)' operation in rule 'CombineUnions' to combine adjacent 'Union' operators into a single 'Union' if necessary when using 'Dataset.union.distinct.union.distinct'.
Currently only handle distinct-like 'Deduplicate', where the keys == output, for example:
```
val df1 = Seq((1, 2, 3)).toDF("a", "b", "c")
val df2 = Seq((6, 2, 5)).toDF("a", "b", "c")
val df3 = Seq((2, 4, 3)).toDF("c", "a", "b")
val df4 = Seq((1, 4, 5)).toDF("b", "a", "c")
val unionDF1 = df1.unionByName(df2).dropDuplicates(Seq("b", "a", "c"))
.unionByName(df3).dropDuplicates().unionByName(df4)
.dropDuplicates("a")
```
In this case, **all Union operators will be combined**.
but,
```
val df1 = Seq((1, 2, 3)).toDF("a", "b", "c")
val df2 = Seq((6, 2, 5)).toDF("a", "b", "c")
val df3 = Seq((2, 4, 3)).toDF("c", "a", "b")
val df4 = Seq((1, 4, 5)).toDF("b", "a", "c")
val unionDF = df1.unionByName(df2).dropDuplicates(Seq("a"))
.unionByName(df3).dropDuplicates("c").unionByName(df4)
.dropDuplicates("b")
```
In this case, **all unions will not be combined, because the Deduplicate.keys doesn't equal to Union.output**.
### Why are the changes needed?
When using 'Dataset.union.distinct.union.distinct', the operator is 'Deduplicate(Keys, Union)', but AstBuilder transform sql-style 'Union' to operator 'Distinct(Union)', the rule 'CombineUnions' in Optimizer only handle 'Distinct(Union)' operator but not Deduplicate(Keys, Union).
Please see the detailed description in [SPARK-34283](https://issues.apache.org/jira/browse/SPARK-34283).
### Does this PR introduce _any_ user-facing change?
No
### How was this patch tested?
Unit tests.
Closes#31404 from zzcclp/SPARK-34283.
Authored-by: Zhichao Zhang <441586683@qq.com>
Signed-off-by: Wenchen Fan <wenchen@databricks.com>
### What changes were proposed in this pull request?
1. Make the following SQL configs as internal:
- spark.sql.legacy.allowHashOnMapType
- spark.sql.legacy.sessionInitWithConfigDefaults
2. Add a test to check that all SQL configs from the `legacy` namespace are marked as internal configs.
### Why are the changes needed?
Assuming that legacy SQL configs shouldn't be set by users in common cases. The purpose of such configs is to allow switching to old behavior in corner cases. So, the configs should be marked as internals.
### Does this PR introduce _any_ user-facing change?
Should not.
### How was this patch tested?
By running new test:
```
$ build/sbt "test:testOnly *SQLConfSuite"
```
Closes#31577 from MaxGekk/mark-legacy-configs-as-internal.
Authored-by: Max Gekk <max.gekk@gmail.com>
Signed-off-by: Dongjoon Hyun <dhyun@apple.com>
### What changes were proposed in this pull request?
The current implement of some DDL not unify the output and not pass the output properly to physical command.
Such as: The output attributes of `ShowFunctions` does't pass to `ShowFunctionsCommand` properly.
As the query plan, this PR pass the output attributes from `ShowFunctions` to `ShowFunctionsCommand`.
### Why are the changes needed?
This PR pass the output attributes could keep the expr ID unchanged, so that avoid bugs when we apply more operators above the command output dataframe.
### Does this PR introduce _any_ user-facing change?
'No'.
### How was this patch tested?
Jenkins test.
Closes#31519 from beliefer/SPARK-34394.
Authored-by: gengjiaan <gengjiaan@360.cn>
Signed-off-by: Wenchen Fan <wenchen@databricks.com>
### What changes were proposed in this pull request?
The current implement of some DDL not unify the output and not pass the output properly to physical command.
Such as: The output attributes of `ShowViews` does't pass to `ShowViewsCommand` properly.
As the query plan, this PR pass the output attributes from `ShowViews` to `ShowViewsCommand`.
### Why are the changes needed?
This PR pass the output attributes could keep the expr ID unchanged, so that avoid bugs when we apply more operators above the command output dataframe.
### Does this PR introduce _any_ user-facing change?
'No'.
### How was this patch tested?
Jenkins test.
Closes#31508 from beliefer/SPARK-34393.
Authored-by: gengjiaan <gengjiaan@360.cn>
Signed-off-by: Wenchen Fan <wenchen@databricks.com>
### What changes were proposed in this pull request?
1. Put the SQL config `spark.sql.legacy.replaceDatabricksSparkAvro.enabled` to the list of deprecated configs `deprecatedSQLConfigs`
2. Update docs for the Avro datasource
<img width="982" alt="Screenshot 2021-02-17 at 21 04 26" src="https://user-images.githubusercontent.com/1580697/108249890-abed7180-7166-11eb-8cb7-0c246d2a34fc.png">
### Why are the changes needed?
The config exists for enough time. We can deprecate it, and recommend users to use `.format("avro")` instead.
### Does this PR introduce _any_ user-facing change?
Should not except of the warning with the recommendation to use the `avro` format.
### How was this patch tested?
1. By generating docs via:
```
$ SKIP_API=1 SKIP_SCALADOC=1 SKIP_PYTHONDOC=1 SKIP_RDOC=1 jekyll serve --watch
```
2. Manually checking the warning:
```
scala> spark.conf.set("spark.sql.legacy.replaceDatabricksSparkAvro.enabled", false)
21/02/17 21:20:18 WARN SQLConf: The SQL config 'spark.sql.legacy.replaceDatabricksSparkAvro.enabled' has been deprecated in Spark v3.2 and may be removed in the future. Use `.format("avro")` in `DataFrameWriter` or `DataFrameReader` instead.
```
Closes#31578 from MaxGekk/deprecate-replaceDatabricksSparkAvro.
Authored-by: Max Gekk <max.gekk@gmail.com>
Signed-off-by: Dongjoon Hyun <dhyun@apple.com>
### What changes were proposed in this pull request?
This PR handles merge operations in `ReplaceNullWithFalseInPredicate`.
### Why are the changes needed?
These changes are needed to match what we already do for delete and update operations.
### Does this PR introduce _any_ user-facing change?
No.
### How was this patch tested?
This PR extends existing tests to cover merge operations.
Closes#31579 from aokolnychyi/spark-33736.
Authored-by: Anton Okolnychyi <aokolnychyi@apple.com>
Signed-off-by: Dongjoon Hyun <dhyun@apple.com>
### What changes were proposed in this pull request?
Move the datetime rebase SQL configs from the `legacy` namespace by:
1. Renaming of the existing rebase configs like `spark.sql.legacy.parquet.datetimeRebaseModeInRead` -> `spark.sql.parquet.datetimeRebaseModeInRead`.
2. Add the legacy configs as alternatives
3. Deprecate the legacy rebase configs.
### Why are the changes needed?
The rebasing SQL configs like `spark.sql.legacy.parquet.datetimeRebaseModeInRead` can be used not only for migration from previous Spark versions but also to read/write datatime columns saved by other systems/frameworks/libs. So, the configs shouldn't be considered as legacy configs.
### Does this PR introduce _any_ user-facing change?
Should not. Users will see a warning if they still use one of the legacy configs.
### How was this patch tested?
1. Manually checking new configs:
```scala
scala> spark.conf.get("spark.sql.parquet.datetimeRebaseModeInRead")
res0: String = EXCEPTION
scala> spark.conf.set("spark.sql.legacy.parquet.datetimeRebaseModeInRead", "LEGACY")
21/02/17 14:57:10 WARN SQLConf: The SQL config 'spark.sql.legacy.parquet.datetimeRebaseModeInRead' has been deprecated in Spark v3.2 and may be removed in the future. Use 'spark.sql.parquet.datetimeRebaseModeInRead' instead.
scala> spark.conf.get("spark.sql.parquet.datetimeRebaseModeInRead")
res2: String = LEGACY
```
2. By running a datetime rebasing test suite:
```
$ build/sbt "test:testOnly *ParquetRebaseDatetimeV1Suite"
```
Closes#31576 from MaxGekk/rebase-confs-alternatives.
Authored-by: Max Gekk <max.gekk@gmail.com>
Signed-off-by: Wenchen Fan <wenchen@databricks.com>
### What changes were proposed in this pull request?
Fix descriptions of the SQL configs `spark.sql.legacy.parquet.int96RebaseModeInRead` and `spark.sql.legacy.parquet.int96RebaseModeInWrite`, and mention `EXCEPTION` as the default value.
### Why are the changes needed?
This fixes incorrect descriptions that can mislead users.
### Does this PR introduce _any_ user-facing change?
No
### How was this patch tested?
By running `./dev/scalastyle`.
Closes#31557 from MaxGekk/int96-exception-by-default-followup.
Authored-by: Max Gekk <max.gekk@gmail.com>
Signed-off-by: HyukjinKwon <gurwls223@apache.org>
### What changes were proposed in this pull request?
Modify `RandomDataGenerator.forType()` to allow generation of dates/timestamps that are valid in both Julian and Proleptic Gregorian calendars. Currently, the function can produce a date (for example `1582-10-06`) which is valid in the Proleptic Gregorian calendar. Though it cannot be saved to ORC files AS IS since ORC format (ORC libs in fact) assumes Julian calendar. So, Spark shifts `1582-10-06` to the next valid date `1582-10-15` while saving it to ORC files. And as a consequence of that, the test fails because it compares original date `1582-10-06` and the date `1582-10-15` loaded back from the ORC files.
In this PR, I propose to generate valid dates/timestamps in both calendars for ORC datasource till SPARK-34440 is resolved.
### Why are the changes needed?
The changes fix failures of `HiveOrcHadoopFsRelationSuite`. For instance, the test "test all data types" fails with the seed **610710213676**:
```
== Results ==
!== Correct Answer - 20 == == Spark Answer - 20 ==
struct<index:int,col:date> struct<index:int,col:date>
...
![9,1582-10-06] [9,1582-10-15]
```
### Does this PR introduce _any_ user-facing change?
No
### How was this patch tested?
By running the modified test suite:
```
$ build/sbt -Phive -Phive-thriftserver "test:testOnly *HiveOrcHadoopFsRelationSuite"
```
Closes#31552 from MaxGekk/fix-HiveOrcHadoopFsRelationSuite.
Authored-by: Max Gekk <max.gekk@gmail.com>
Signed-off-by: HyukjinKwon <gurwls223@apache.org>
### What changes were proposed in this pull request?
This PR proposes to support `ifExists` flag for v2 `ALTER TABLE ... UNSET TBLPROPERTIES` command. Currently, the flag is not respected and the command behaves as `ifExists = true` where the command always succeeds when the properties do not exist.
### Why are the changes needed?
To support `ifExists` flag and align with v1 command behavior.
### Does this PR introduce _any_ user-facing change?
Yes, now if the property does not exist and `IF EXISTS` is not specified, the command will fail:
```
ALTER TABLE t UNSET TBLPROPERTIES ('unknown') // Fails with "Attempted to unset non-existent property 'unknown'"
ALTER TABLE t UNSET TBLPROPERTIES IF EXISTS ('unknown') // OK
```
### How was this patch tested?
Added new test
Closes#31494 from imback82/AlterTableUnsetPropertiesIfExists.
Authored-by: Terry Kim <yuminkim@gmail.com>
Signed-off-by: Dongjoon Hyun <dhyun@apple.com>
### What changes were proposed in this pull request?
Move `PartitionTransforms.scala` from `sql/catalyst/src/main/java/org/apache/spark/sql/catalyst/expressions` to `sql/catalyst/src/main/scala/org/apache/spark/sql/catalyst/expressions`.
### Why are the changes needed?
We should put java/scala files to their corresponding directories.
### Does this PR introduce _any_ user-facing change?
No.
### How was this patch tested?
N/A
Closes#31546 from sunchao/SPARK-34419.
Authored-by: Chao Sun <sunchao@apple.com>
Signed-off-by: Liang-Chi Hsieh <viirya@gmail.com>
### What changes were proposed in this pull request?
Creating a Pandas dataframe via Apache Arrow currently can use twice as much memory as the final result, because during the conversion, both Pandas and Arrow retain a copy of the data. Arrow has a "self-destruct" mode now (Arrow >= 0.16) to avoid this, by freeing each column after conversion. This PR integrates support for this in toPandas, handling a couple of edge cases:
self_destruct has no effect unless the memory is allocated appropriately, which is handled in the Arrow serializer here. Essentially, the issue is that self_destruct frees memory column-wise, but Arrow record batches are oriented row-wise:
```
Record batch 0: allocation 0: column 0 chunk 0, column 1 chunk 0, ...
Record batch 1: allocation 1: column 0 chunk 1, column 1 chunk 1, ...
```
In this scenario, Arrow will drop references to all of column 0's chunks, but no memory will actually be freed, as the chunks were just slices of an underlying allocation. The PR copies each column into its own allocation so that memory is instead arranged as so:
```
Record batch 0: allocation 0 column 0 chunk 0, allocation 1 column 1 chunk 0, ...
Record batch 1: allocation 2 column 0 chunk 1, allocation 3 column 1 chunk 1, ...
```
The optimization is disabled by default, and can be enabled with the Spark SQL conf "spark.sql.execution.arrow.pyspark.selfDestruct.enabled" set to "true". We can't always apply this optimization because it's more likely to generate a dataframe with immutable buffers, which Pandas doesn't always handle well, and because it is slower overall (since it only converts one column at a time instead of in parallel).
### Why are the changes needed?
This lets us load larger datasets - in particular, with N bytes of memory, before we could never load a dataset bigger than N/2 bytes; now the overhead is more like N/1.25 or so.
### Does this PR introduce _any_ user-facing change?
Yes - it adds a new SQL conf "spark.sql.execution.arrow.pyspark.selfDestruct.enabled"
### How was this patch tested?
See the [mailing list](http://apache-spark-developers-list.1001551.n3.nabble.com/DISCUSS-Reducing-memory-usage-of-toPandas-with-Arrow-quot-self-destruct-quot-option-td30149.html) - it was tested with Python memory_profiler. Unit tests added to check memory within certain bounds and correctness with the option enabled.
Closes#29818 from lidavidm/spark-32953.
Authored-by: David Li <li.davidm96@gmail.com>
Signed-off-by: Bryan Cutler <cutlerb@gmail.com>
### What changes were proposed in this pull request?
`TreeNodeException` causes the error msg not clear and it didn't work well.
Because the `TreeNodeException` looks redundancy, we could remove it.
There are show a case:
```
val df = Seq(("1", 1), ("1", 2), ("2", 3), ("2", 4)).toDF("x", "y")
val hashAggDF = df.groupBy("x").agg(c, sum("y"))
```
The above code will use `HashAggregateExec`. In order to ensure that an exception will be thrown when executing `HashAggregateExec`, I added `throw new RuntimeException("calculate error")` into 72b7f8abfb/sql/core/src/main/scala/org/apache/spark/sql/execution/aggregate/HashAggregateExec.scala (L85)
So, if the above code is executed, `RuntimeException("calculate error")` will be thrown.
Before this PR, the error is:
```
execute, tree:
ShuffleQueryStage 0
+- Exchange hashpartitioning(x#105, 5), ENSURE_REQUIREMENTS, [id=#168]
+- HashAggregate(keys=[x#105], functions=[partial_sum(y#106)], output=[x#105, sum#118L])
+- Project [_1#100 AS x#105, _2#101 AS y#106]
+- LocalTableScan [_1#100, _2#101]
org.apache.spark.sql.catalyst.errors.package$TreeNodeException: execute, tree:
ShuffleQueryStage 0
+- Exchange hashpartitioning(x#105, 5), ENSURE_REQUIREMENTS, [id=#168]
+- HashAggregate(keys=[x#105], functions=[partial_sum(y#106)], output=[x#105, sum#118L])
+- Project [_1#100 AS x#105, _2#101 AS y#106]
+- LocalTableScan [_1#100, _2#101]
at org.apache.spark.sql.catalyst.errors.package$.attachTree(package.scala:56)
at org.apache.spark.sql.execution.adaptive.ShuffleQueryStageExec.doMaterialize(QueryStageExec.scala:163)
at org.apache.spark.sql.execution.adaptive.QueryStageExec.$anonfun$materialize$1(QueryStageExec.scala:81)
at org.apache.spark.sql.execution.SparkPlan.$anonfun$executeQuery$1(SparkPlan.scala:218)
at org.apache.spark.rdd.RDDOperationScope$.withScope(RDDOperationScope.scala:151)
at org.apache.spark.sql.execution.SparkPlan.executeQuery(SparkPlan.scala:215)
at org.apache.spark.sql.execution.adaptive.QueryStageExec.materialize(QueryStageExec.scala:79)
at org.apache.spark.sql.execution.adaptive.AdaptiveSparkPlanExec.$anonfun$getFinalPhysicalPlan$5(AdaptiveSparkPlanExec.scala:207)
at org.apache.spark.sql.execution.adaptive.AdaptiveSparkPlanExec.$anonfun$getFinalPhysicalPlan$5$adapted(AdaptiveSparkPlanExec.scala:205)
at scala.collection.immutable.List.foreach(List.scala:392)
at org.apache.spark.sql.execution.adaptive.AdaptiveSparkPlanExec.$anonfun$getFinalPhysicalPlan$1(AdaptiveSparkPlanExec.scala:205)
at org.apache.spark.sql.SparkSession.withActive(SparkSession.scala:772)
at org.apache.spark.sql.execution.adaptive.AdaptiveSparkPlanExec.getFinalPhysicalPlan(AdaptiveSparkPlanExec.scala:179)
at org.apache.spark.sql.execution.adaptive.AdaptiveSparkPlanExec.executeCollect(AdaptiveSparkPlanExec.scala:289)
at org.apache.spark.sql.Dataset.collectFromPlan(Dataset.scala:3708)
at org.apache.spark.sql.Dataset.$anonfun$collect$1(Dataset.scala:2977)
at org.apache.spark.sql.Dataset.$anonfun$withAction$1(Dataset.scala:3699)
at org.apache.spark.sql.execution.SQLExecution$.$anonfun$withNewExecutionId$5(SQLExecution.scala:103)
at org.apache.spark.sql.execution.SQLExecution$.withSQLConfPropagated(SQLExecution.scala:163)
at org.apache.spark.sql.execution.SQLExecution$.$anonfun$withNewExecutionId$1(SQLExecution.scala:90)
at org.apache.spark.sql.SparkSession.withActive(SparkSession.scala:772)
at org.apache.spark.sql.execution.SQLExecution$.withNewExecutionId(SQLExecution.scala:64)
at org.apache.spark.sql.Dataset.withAction(Dataset.scala:3697)
at org.apache.spark.sql.Dataset.collect(Dataset.scala:2977)
at org.apache.spark.sql.DataFrameAggregateSuite.$anonfun$assertNoExceptions$3(DataFrameAggregateSuite.scala:665)
at org.apache.spark.sql.catalyst.plans.SQLHelper.withSQLConf(SQLHelper.scala:54)
at org.apache.spark.sql.catalyst.plans.SQLHelper.withSQLConf$(SQLHelper.scala:38)
at org.apache.spark.sql.DataFrameAggregateSuite.org$apache$spark$sql$test$SQLTestUtilsBase$$super$withSQLConf(DataFrameAggregateSuite.scala:37)
at org.apache.spark.sql.test.SQLTestUtilsBase.withSQLConf(SQLTestUtils.scala:246)
at org.apache.spark.sql.test.SQLTestUtilsBase.withSQLConf$(SQLTestUtils.scala:244)
at org.apache.spark.sql.DataFrameAggregateSuite.withSQLConf(DataFrameAggregateSuite.scala:37)
at org.apache.spark.sql.DataFrameAggregateSuite.$anonfun$assertNoExceptions$2(DataFrameAggregateSuite.scala:659)
at org.apache.spark.sql.DataFrameAggregateSuite.$anonfun$assertNoExceptions$2$adapted(DataFrameAggregateSuite.scala:655)
at scala.collection.TraversableLike$WithFilter.$anonfun$foreach$1(TraversableLike.scala:877)
at scala.collection.immutable.List.foreach(List.scala:392)
at scala.collection.TraversableLike$WithFilter.foreach(TraversableLike.scala:876)
at org.apache.spark.sql.DataFrameAggregateSuite.assertNoExceptions(DataFrameAggregateSuite.scala:655)
at org.apache.spark.sql.DataFrameAggregateSuite.$anonfun$new$126(DataFrameAggregateSuite.scala:695)
at org.apache.spark.sql.DataFrameAggregateSuite.$anonfun$new$126$adapted(DataFrameAggregateSuite.scala:695)
at scala.collection.immutable.List.foreach(List.scala:392)
at org.apache.spark.sql.DataFrameAggregateSuite.$anonfun$new$125(DataFrameAggregateSuite.scala:695)
at scala.runtime.java8.JFunction0$mcV$sp.apply(JFunction0$mcV$sp.java:23)
at org.scalatest.OutcomeOf.outcomeOf(OutcomeOf.scala:85)
at org.scalatest.OutcomeOf.outcomeOf$(OutcomeOf.scala:83)
at org.scalatest.OutcomeOf$.outcomeOf(OutcomeOf.scala:104)
at org.scalatest.Transformer.apply(Transformer.scala:22)
at org.scalatest.Transformer.apply(Transformer.scala:20)
at org.scalatest.funsuite.AnyFunSuiteLike$$anon$1.apply(AnyFunSuiteLike.scala:190)
at org.apache.spark.SparkFunSuite.withFixture(SparkFunSuite.scala:176)
at org.scalatest.funsuite.AnyFunSuiteLike.invokeWithFixture$1(AnyFunSuiteLike.scala:188)
at org.scalatest.funsuite.AnyFunSuiteLike.$anonfun$runTest$1(AnyFunSuiteLike.scala:200)
at org.scalatest.SuperEngine.runTestImpl(Engine.scala:306)
at org.scalatest.funsuite.AnyFunSuiteLike.runTest(AnyFunSuiteLike.scala:200)
at org.scalatest.funsuite.AnyFunSuiteLike.runTest$(AnyFunSuiteLike.scala:182)
at org.apache.spark.SparkFunSuite.org$scalatest$BeforeAndAfterEach$$super$runTest(SparkFunSuite.scala:61)
at org.scalatest.BeforeAndAfterEach.runTest(BeforeAndAfterEach.scala:234)
at org.scalatest.BeforeAndAfterEach.runTest$(BeforeAndAfterEach.scala:227)
at org.apache.spark.SparkFunSuite.runTest(SparkFunSuite.scala:61)
at org.scalatest.funsuite.AnyFunSuiteLike.$anonfun$runTests$1(AnyFunSuiteLike.scala:233)
at org.scalatest.SuperEngine.$anonfun$runTestsInBranch$1(Engine.scala:413)
at scala.collection.immutable.List.foreach(List.scala:392)
at org.scalatest.SuperEngine.traverseSubNodes$1(Engine.scala:401)
at org.scalatest.SuperEngine.runTestsInBranch(Engine.scala:396)
at org.scalatest.SuperEngine.runTestsImpl(Engine.scala:475)
at org.scalatest.funsuite.AnyFunSuiteLike.runTests(AnyFunSuiteLike.scala:233)
at org.scalatest.funsuite.AnyFunSuiteLike.runTests$(AnyFunSuiteLike.scala:232)
at org.scalatest.funsuite.AnyFunSuite.runTests(AnyFunSuite.scala:1563)
at org.scalatest.Suite.run(Suite.scala:1112)
at org.scalatest.Suite.run$(Suite.scala:1094)
at org.scalatest.funsuite.AnyFunSuite.org$scalatest$funsuite$AnyFunSuiteLike$$super$run(AnyFunSuite.scala:1563)
at org.scalatest.funsuite.AnyFunSuiteLike.$anonfun$run$1(AnyFunSuiteLike.scala:237)
at org.scalatest.SuperEngine.runImpl(Engine.scala:535)
at org.scalatest.funsuite.AnyFunSuiteLike.run(AnyFunSuiteLike.scala:237)
at org.scalatest.funsuite.AnyFunSuiteLike.run$(AnyFunSuiteLike.scala:236)
at org.apache.spark.SparkFunSuite.org$scalatest$BeforeAndAfterAll$$super$run(SparkFunSuite.scala:61)
at org.scalatest.BeforeAndAfterAll.liftedTree1$1(BeforeAndAfterAll.scala:213)
at org.scalatest.BeforeAndAfterAll.run(BeforeAndAfterAll.scala:210)
at org.scalatest.BeforeAndAfterAll.run$(BeforeAndAfterAll.scala:208)
at org.apache.spark.SparkFunSuite.run(SparkFunSuite.scala:61)
at org.scalatest.tools.SuiteRunner.run(SuiteRunner.scala:45)
at org.scalatest.tools.Runner$.$anonfun$doRunRunRunDaDoRunRun$13(Runner.scala:1320)
at org.scalatest.tools.Runner$.$anonfun$doRunRunRunDaDoRunRun$13$adapted(Runner.scala:1314)
at scala.collection.immutable.List.foreach(List.scala:392)
at org.scalatest.tools.Runner$.doRunRunRunDaDoRunRun(Runner.scala:1314)
at org.scalatest.tools.Runner$.$anonfun$runOptionallyWithPassFailReporter$24(Runner.scala:993)
at org.scalatest.tools.Runner$.$anonfun$runOptionallyWithPassFailReporter$24$adapted(Runner.scala:971)
at org.scalatest.tools.Runner$.withClassLoaderAndDispatchReporter(Runner.scala:1480)
at org.scalatest.tools.Runner$.runOptionallyWithPassFailReporter(Runner.scala:971)
at org.scalatest.tools.Runner$.run(Runner.scala:798)
at org.scalatest.tools.Runner.run(Runner.scala)
at org.jetbrains.plugins.scala.testingSupport.scalaTest.ScalaTestRunner.runScalaTest2(ScalaTestRunner.java:131)
at org.jetbrains.plugins.scala.testingSupport.scalaTest.ScalaTestRunner.main(ScalaTestRunner.java:28)
Caused by: org.apache.spark.sql.catalyst.errors.package$TreeNodeException: execute, tree:
HashAggregate(keys=[x#105], functions=[partial_sum(y#106)], output=[x#105, sum#118L])
+- Project [_1#100 AS x#105, _2#101 AS y#106]
+- LocalTableScan [_1#100, _2#101]
at org.apache.spark.sql.catalyst.errors.package$.attachTree(package.scala:56)
at org.apache.spark.sql.execution.aggregate.HashAggregateExec.doExecute(HashAggregateExec.scala:84)
at org.apache.spark.sql.execution.SparkPlan.$anonfun$execute$1(SparkPlan.scala:180)
at org.apache.spark.sql.execution.SparkPlan.$anonfun$executeQuery$1(SparkPlan.scala:218)
at org.apache.spark.rdd.RDDOperationScope$.withScope(RDDOperationScope.scala:151)
at org.apache.spark.sql.execution.SparkPlan.executeQuery(SparkPlan.scala:215)
at org.apache.spark.sql.execution.SparkPlan.execute(SparkPlan.scala:176)
at org.apache.spark.sql.execution.exchange.ShuffleExchangeExec.inputRDD$lzycompute(ShuffleExchangeExec.scala:118)
at org.apache.spark.sql.execution.exchange.ShuffleExchangeExec.inputRDD(ShuffleExchangeExec.scala:118)
at org.apache.spark.sql.execution.exchange.ShuffleExchangeExec.mapOutputStatisticsFuture$lzycompute(ShuffleExchangeExec.scala:122)
at org.apache.spark.sql.execution.exchange.ShuffleExchangeExec.mapOutputStatisticsFuture(ShuffleExchangeExec.scala:121)
at org.apache.spark.sql.execution.adaptive.ShuffleQueryStageExec.$anonfun$doMaterialize$1(QueryStageExec.scala:163)
at org.apache.spark.sql.catalyst.errors.package$.attachTree(package.scala:52)
... 91 more
Caused by: java.lang.RuntimeException: calculate error
at org.apache.spark.sql.execution.aggregate.HashAggregateExec.$anonfun$doExecute$1(HashAggregateExec.scala:85)
at org.apache.spark.sql.catalyst.errors.package$.attachTree(package.scala:52)
... 103 more
```
After this PR, the error is:
```
calculate error
java.lang.RuntimeException: calculate error
at org.apache.spark.sql.execution.aggregate.HashAggregateExec.doExecute(HashAggregateExec.scala:84)
at org.apache.spark.sql.execution.SparkPlan.$anonfun$execute$1(SparkPlan.scala:180)
at org.apache.spark.sql.execution.SparkPlan.$anonfun$executeQuery$1(SparkPlan.scala:218)
at org.apache.spark.rdd.RDDOperationScope$.withScope(RDDOperationScope.scala:151)
at org.apache.spark.sql.execution.SparkPlan.executeQuery(SparkPlan.scala:215)
at org.apache.spark.sql.execution.SparkPlan.execute(SparkPlan.scala:176)
at org.apache.spark.sql.execution.exchange.ShuffleExchangeExec.inputRDD$lzycompute(ShuffleExchangeExec.scala:117)
at org.apache.spark.sql.execution.exchange.ShuffleExchangeExec.inputRDD(ShuffleExchangeExec.scala:117)
at org.apache.spark.sql.execution.exchange.ShuffleExchangeExec.mapOutputStatisticsFuture$lzycompute(ShuffleExchangeExec.scala:121)
at org.apache.spark.sql.execution.exchange.ShuffleExchangeExec.mapOutputStatisticsFuture(ShuffleExchangeExec.scala:120)
at org.apache.spark.sql.execution.adaptive.ShuffleQueryStageExec.doMaterialize(QueryStageExec.scala:161)
at org.apache.spark.sql.execution.adaptive.QueryStageExec.$anonfun$materialize$1(QueryStageExec.scala:80)
at org.apache.spark.sql.execution.SparkPlan.$anonfun$executeQuery$1(SparkPlan.scala:218)
at org.apache.spark.rdd.RDDOperationScope$.withScope(RDDOperationScope.scala:151)
at org.apache.spark.sql.execution.SparkPlan.executeQuery(SparkPlan.scala:215)
at org.apache.spark.sql.execution.adaptive.QueryStageExec.materialize(QueryStageExec.scala:78)
at org.apache.spark.sql.execution.adaptive.AdaptiveSparkPlanExec.$anonfun$getFinalPhysicalPlan$5(AdaptiveSparkPlanExec.scala:207)
at org.apache.spark.sql.execution.adaptive.AdaptiveSparkPlanExec.$anonfun$getFinalPhysicalPlan$5$adapted(AdaptiveSparkPlanExec.scala:205)
at scala.collection.immutable.List.foreach(List.scala:392)
at org.apache.spark.sql.execution.adaptive.AdaptiveSparkPlanExec.$anonfun$getFinalPhysicalPlan$1(AdaptiveSparkPlanExec.scala:205)
at org.apache.spark.sql.SparkSession.withActive(SparkSession.scala:772)
at org.apache.spark.sql.execution.adaptive.AdaptiveSparkPlanExec.getFinalPhysicalPlan(AdaptiveSparkPlanExec.scala:179)
at org.apache.spark.sql.execution.adaptive.AdaptiveSparkPlanExec.executeCollect(AdaptiveSparkPlanExec.scala:289)
at org.apache.spark.sql.Dataset.collectFromPlan(Dataset.scala:3708)
at org.apache.spark.sql.Dataset.$anonfun$collect$1(Dataset.scala:2977)
at org.apache.spark.sql.Dataset.$anonfun$withAction$1(Dataset.scala:3699)
at org.apache.spark.sql.execution.SQLExecution$.$anonfun$withNewExecutionId$5(SQLExecution.scala:103)
at org.apache.spark.sql.execution.SQLExecution$.withSQLConfPropagated(SQLExecution.scala:163)
at org.apache.spark.sql.execution.SQLExecution$.$anonfun$withNewExecutionId$1(SQLExecution.scala:90)
at org.apache.spark.sql.SparkSession.withActive(SparkSession.scala:772)
at org.apache.spark.sql.execution.SQLExecution$.withNewExecutionId(SQLExecution.scala:64)
at org.apache.spark.sql.Dataset.withAction(Dataset.scala:3697)
at org.apache.spark.sql.Dataset.collect(Dataset.scala:2977)
at org.apache.spark.sql.DataFrameAggregateSuite.$anonfun$assertNoExceptions$3(DataFrameAggregateSuite.scala:665)
at org.apache.spark.sql.catalyst.plans.SQLHelper.withSQLConf(SQLHelper.scala:54)
at org.apache.spark.sql.catalyst.plans.SQLHelper.withSQLConf$(SQLHelper.scala:38)
at org.apache.spark.sql.DataFrameAggregateSuite.org$apache$spark$sql$test$SQLTestUtilsBase$$super$withSQLConf(DataFrameAggregateSuite.scala:37)
at org.apache.spark.sql.test.SQLTestUtilsBase.withSQLConf(SQLTestUtils.scala:246)
at org.apache.spark.sql.test.SQLTestUtilsBase.withSQLConf$(SQLTestUtils.scala:244)
at org.apache.spark.sql.DataFrameAggregateSuite.withSQLConf(DataFrameAggregateSuite.scala:37)
at org.apache.spark.sql.DataFrameAggregateSuite.$anonfun$assertNoExceptions$2(DataFrameAggregateSuite.scala:659)
at org.apache.spark.sql.DataFrameAggregateSuite.$anonfun$assertNoExceptions$2$adapted(DataFrameAggregateSuite.scala:655)
at scala.collection.TraversableLike$WithFilter.$anonfun$foreach$1(TraversableLike.scala:877)
at scala.collection.immutable.List.foreach(List.scala:392)
at scala.collection.TraversableLike$WithFilter.foreach(TraversableLike.scala:876)
at org.apache.spark.sql.DataFrameAggregateSuite.assertNoExceptions(DataFrameAggregateSuite.scala:655)
at org.apache.spark.sql.DataFrameAggregateSuite.$anonfun$new$126(DataFrameAggregateSuite.scala:695)
at org.apache.spark.sql.DataFrameAggregateSuite.$anonfun$new$126$adapted(DataFrameAggregateSuite.scala:695)
at scala.collection.immutable.List.foreach(List.scala:392)
at org.apache.spark.sql.DataFrameAggregateSuite.$anonfun$new$125(DataFrameAggregateSuite.scala:695)
at scala.runtime.java8.JFunction0$mcV$sp.apply(JFunction0$mcV$sp.java:23)
at org.scalatest.OutcomeOf.outcomeOf(OutcomeOf.scala:85)
at org.scalatest.OutcomeOf.outcomeOf$(OutcomeOf.scala:83)
at org.scalatest.OutcomeOf$.outcomeOf(OutcomeOf.scala:104)
at org.scalatest.Transformer.apply(Transformer.scala:22)
at org.scalatest.Transformer.apply(Transformer.scala:20)
at org.scalatest.funsuite.AnyFunSuiteLike$$anon$1.apply(AnyFunSuiteLike.scala:190)
at org.apache.spark.SparkFunSuite.withFixture(SparkFunSuite.scala:176)
at org.scalatest.funsuite.AnyFunSuiteLike.invokeWithFixture$1(AnyFunSuiteLike.scala:188)
at org.scalatest.funsuite.AnyFunSuiteLike.$anonfun$runTest$1(AnyFunSuiteLike.scala:200)
at org.scalatest.SuperEngine.runTestImpl(Engine.scala:306)
at org.scalatest.funsuite.AnyFunSuiteLike.runTest(AnyFunSuiteLike.scala:200)
at org.scalatest.funsuite.AnyFunSuiteLike.runTest$(AnyFunSuiteLike.scala:182)
at org.apache.spark.SparkFunSuite.org$scalatest$BeforeAndAfterEach$$super$runTest(SparkFunSuite.scala:61)
at org.scalatest.BeforeAndAfterEach.runTest(BeforeAndAfterEach.scala:234)
at org.scalatest.BeforeAndAfterEach.runTest$(BeforeAndAfterEach.scala:227)
at org.apache.spark.SparkFunSuite.runTest(SparkFunSuite.scala:61)
at org.scalatest.funsuite.AnyFunSuiteLike.$anonfun$runTests$1(AnyFunSuiteLike.scala:233)
at org.scalatest.SuperEngine.$anonfun$runTestsInBranch$1(Engine.scala:413)
at scala.collection.immutable.List.foreach(List.scala:392)
at org.scalatest.SuperEngine.traverseSubNodes$1(Engine.scala:401)
at org.scalatest.SuperEngine.runTestsInBranch(Engine.scala:396)
at org.scalatest.SuperEngine.runTestsImpl(Engine.scala:475)
at org.scalatest.funsuite.AnyFunSuiteLike.runTests(AnyFunSuiteLike.scala:233)
at org.scalatest.funsuite.AnyFunSuiteLike.runTests$(AnyFunSuiteLike.scala:232)
at org.scalatest.funsuite.AnyFunSuite.runTests(AnyFunSuite.scala:1563)
at org.scalatest.Suite.run(Suite.scala:1112)
at org.scalatest.Suite.run$(Suite.scala:1094)
at org.scalatest.funsuite.AnyFunSuite.org$scalatest$funsuite$AnyFunSuiteLike$$super$run(AnyFunSuite.scala:1563)
at org.scalatest.funsuite.AnyFunSuiteLike.$anonfun$run$1(AnyFunSuiteLike.scala:237)
at org.scalatest.SuperEngine.runImpl(Engine.scala:535)
at org.scalatest.funsuite.AnyFunSuiteLike.run(AnyFunSuiteLike.scala:237)
at org.scalatest.funsuite.AnyFunSuiteLike.run$(AnyFunSuiteLike.scala:236)
at org.apache.spark.SparkFunSuite.org$scalatest$BeforeAndAfterAll$$super$run(SparkFunSuite.scala:61)
at org.scalatest.BeforeAndAfterAll.liftedTree1$1(BeforeAndAfterAll.scala:213)
at org.scalatest.BeforeAndAfterAll.run(BeforeAndAfterAll.scala:210)
at org.scalatest.BeforeAndAfterAll.run$(BeforeAndAfterAll.scala:208)
at org.apache.spark.SparkFunSuite.run(SparkFunSuite.scala:61)
at org.scalatest.tools.SuiteRunner.run(SuiteRunner.scala:45)
at org.scalatest.tools.Runner$.$anonfun$doRunRunRunDaDoRunRun$13(Runner.scala:1320)
at org.scalatest.tools.Runner$.$anonfun$doRunRunRunDaDoRunRun$13$adapted(Runner.scala:1314)
at scala.collection.immutable.List.foreach(List.scala:392)
at org.scalatest.tools.Runner$.doRunRunRunDaDoRunRun(Runner.scala:1314)
at org.scalatest.tools.Runner$.$anonfun$runOptionallyWithPassFailReporter$24(Runner.scala:993)
at org.scalatest.tools.Runner$.$anonfun$runOptionallyWithPassFailReporter$24$adapted(Runner.scala:971)
at org.scalatest.tools.Runner$.withClassLoaderAndDispatchReporter(Runner.scala:1480)
at org.scalatest.tools.Runner$.runOptionallyWithPassFailReporter(Runner.scala:971)
at org.scalatest.tools.Runner$.run(Runner.scala:798)
at org.scalatest.tools.Runner.run(Runner.scala)
at org.jetbrains.plugins.scala.testingSupport.scalaTest.ScalaTestRunner.runScalaTest2(ScalaTestRunner.java:131)
at org.jetbrains.plugins.scala.testingSupport.scalaTest.ScalaTestRunner.main(ScalaTestRunner.java:28)
```
### Why are the changes needed?
`TreeNodeException` didn't work well.
### Does this PR introduce _any_ user-facing change?
'No'.
### How was this patch tested?
Jenkins test.
Closes#31337 from beliefer/SPARK-34234.
Lead-authored-by: gengjiaan <gengjiaan@360.cn>
Co-authored-by: beliefer <beliefer@163.com>
Co-authored-by: Jiaan Geng <beliefer@163.com>
Signed-off-by: Wenchen Fan <wenchen@databricks.com>
### What changes were proposed in this pull request?
This PR includes the following changes:
1. in `CatalogImpl.uncacheTable`, invalidate caches in cascade when the target table is
a temp view, and `spark.sql.legacy.storeAnalyzedPlanForView` is false (default value).
2. make `SessionCatalog.lookupTempView` public and return processed temp view plan (i.e., with `View` op).
### Why are the changes needed?
Following [SPARK-34052](https://issues.apache.org/jira/browse/SPARK-34052) (#31107), we should invalidate in cascade for `CatalogImpl.uncacheTable` when the table is a temp view, so that the behavior is consistent.
### Does this PR introduce _any_ user-facing change?
Yes, now `SQLContext.uncacheTable` will drop temp view in cascade by default.
### How was this patch tested?
Added a UT
Closes#31462 from sunchao/SPARK-34347.
Authored-by: Chao Sun <sunchao@apple.com>
Signed-off-by: Liang-Chi Hsieh <viirya@gmail.com>
### What changes were proposed in this pull request?
Passing around the output attributes should have more benefits like keeping the exprID unchanged to avoid bugs when we apply more operators above the command output DataFrame.
This PR did 2 things :
1. After this pr, a `SHOW TBLPROPERTIES` clause's output shows `key` and `value` columns whether you specify the table property `key`. Before this pr, a `SHOW TBLPROPERTIES` clause's output only show a `value` column when you specify the table property `key`..
2. Keep `SHOW TBLPROPERTIES` command's output attribute exprId unchanged.
### Why are the changes needed?
1. Keep `SHOW TBLPROPERTIES`'s output schema consistence
2. Keep `SHOW TBLPROPERTIES` command's output attribute exprId unchanged.
### Does this PR introduce _any_ user-facing change?
After this pr, a `SHOW TBLPROPERTIES` clause's output shows `key` and `value` columns whether you specify the table property `key`. Before this pr, a `SHOW TBLPROPERTIES` clause's output only show a `value` column when you specify the table property `key`.
Before this PR:
```
sql > SHOW TBLPROPERTIES tabe_name('key')
value
value_of_key
```
After this PR
```
sql > SHOW TBLPROPERTIES tabe_name('key')
key value
key value_of_key
```
### How was this patch tested?
Added UT
Closes#31378 from AngersZhuuuu/SPARK-34240.
Lead-authored-by: Angerszhuuuu <angers.zhu@gmail.com>
Co-authored-by: AngersZhuuuu <angers.zhu@gmail.com>
Signed-off-by: Wenchen Fan <wenchen@databricks.com>
### What changes were proposed in this pull request?
Keep consistence with other `SHOW` command according to https://github.com/apache/spark/pull/31341#issuecomment-774613080
### Why are the changes needed?
Keep consistence
### Does this PR introduce _any_ user-facing change?
No
### How was this patch tested?
Not need
Closes#31516 from AngersZhuuuu/SPARK-34238-follow-up.
Authored-by: Angerszhuuuu <angers.zhu@gmail.com>
Signed-off-by: Wenchen Fan <wenchen@databricks.com>
### What changes were proposed in this pull request?
Delegate table name validation to the session catalog
### Why are the changes needed?
Queerying of tables with nested namespaces.
### Does this PR introduce _any_ user-facing change?
SQL queries of nested namespace queries
### How was this patch tested?
Unit tests updated.
Closes#31427 from holdenk/SPARK-34209-delegate-table-name-validation-to-the-catalog.
Authored-by: Holden Karau <hkarau@apple.com>
Signed-off-by: Holden Karau <hkarau@apple.com>
### What changes were proposed in this pull request?
Currently, we pass the default value `EmptyRow` to method `checkEvaluation` in the `StringExpressionsSuite`, but the default value of the 'checkEvaluation' method parameter is the `emptyRow`.
We can clean the parameter for Code Simplifications.
### Why are the changes needed?
for Code Simplifications
**before**:
```
def testConcat(inputs: String*): Unit = {
val expected = if (inputs.contains(null)) null else inputs.mkString
checkEvaluation(Concat(inputs.map(Literal.create(_, StringType))), expected, EmptyRow)
}
```
**after**:
```
def testConcat(inputs: String*): Unit = {
val expected = if (inputs.contains(null)) null else inputs.mkString
checkEvaluation(Concat(inputs.map(Literal.create(_, StringType))), expected)
}
```
### Does this PR introduce _any_ user-facing change?
No
### How was this patch tested?
Pass the Jenkins or Github action.
Closes#31510 from yikf/master.
Authored-by: yikf <13468507104@163.com>
Signed-off-by: Sean Owen <srowen@gmail.com>
### What changes were proposed in this pull request?
This PR proposes to propagate the name used for registering UDFs to `ScalaUDF`, `ScalaUDAF` and `ScaalAggregator`.
Note that `PythonUDF` gets the name correctly: 466c045bfa/python/pyspark/sql/udf.py (L358-L359)
, and same for Hive UDFs:
466c045bfa/sql/hive/src/main/scala/org/apache/spark/sql/hive/HiveSessionCatalog.scala (L67)
### Why are the changes needed?
This PR can help in the following scenarios:
1) Better EXPLAIN output
2) By adding `def name: String` to `UserDefinedExpression`, we can match an expression by `UserDefinedExpression` and look up the catalog, an use case needed for #31273.
### Does this PR introduce _any_ user-facing change?
The EXPLAIN output involving udfs will be changed to use the name used for UDF registration.
For example, for the following:
```
sql("CREATE TEMPORARY FUNCTION test_udf AS 'org.apache.spark.examples.sql.Spark33084'")
sql("SELECT test_udf(col1) FROM VALUES (1), (2), (3)").explain(true)
```
The output of the optimized plan will change from:
```
Aggregate [spark33084(cast(col1#223 as bigint), org.apache.spark.examples.sql.Spark330846906be0f, 1, 1) AS spark33084(col1)#237]
+- LocalRelation [col1#223]
```
to
```
Aggregate [test_udf(cast(col1#223 as bigint), org.apache.spark.examples.sql.Spark330847a62d697, 1, 1, Some(test_udf)) AS test_udf(col1)#237]
+- LocalRelation [col1#223]
```
### How was this patch tested?
Added new tests.
Closes#31500 from imback82/udaf_name.
Authored-by: Terry Kim <yuminkim@gmail.com>
Signed-off-by: Wenchen Fan <wenchen@databricks.com>
### What changes were proposed in this pull request?
Adds an assert to `FixedLengthRowBasedKeyValueBatch#appendRow` method to check the incoming vlen and klen by comparing them with the lengths stored as member variables as followup to https://github.com/apache/spark/pull/30788
### Why are the changes needed?
Add assert statement to catch similar bugs in future.
### Does this PR introduce _any_ user-facing change?
No
### How was this patch tested?
Ran some tests locally, though not easy to test.
Closes#31447 from yliou/SPARK-33726-Assert.
Authored-by: yliou <yliou@berkeley.edu>
Signed-off-by: Sean Owen <srowen@gmail.com>
### What changes were proposed in this pull request?
In Spark, `set -v` is defined as "Queries all properties that are defined in the SQLConf of the sparkSession".
But there are other external modules that also define properties and register them to SQLConf. In this case,
it can't be displayed by `set -v` until the conf object is initiated (i.e. calling the object at least once).
In this PR, I propose to eagerly initiate all the objects registered to SQLConf, so that `set -v` will always output
the completed properties.
### Why are the changes needed?
Improve the `set -v` command to produces completed and deterministic results
### Does this PR introduce _any_ user-facing change?
`set -v` command will dump more configs
### How was this patch tested?
existing tests
Closes#30363 from linhongliu-db/set-v.
Authored-by: Linhong Liu <linhong.liu@databricks.com>
Signed-off-by: HyukjinKwon <gurwls223@apache.org>
### What changes were proposed in this pull request?
Keep consistence with other `SHOW` command according to https://github.com/apache/spark/pull/31341#issuecomment-774613080
### Why are the changes needed?
Keep consistence
### Does this PR introduce _any_ user-facing change?
No
### How was this patch tested?
Not need
Closes#31518 from AngersZhuuuu/SPARK-34239-followup.
Authored-by: Angerszhuuuu <angers.zhu@gmail.com>
Signed-off-by: Wenchen Fan <wenchen@databricks.com>
### What changes were proposed in this pull request?
The current implement of some DDL not unify the output and not pass the output properly to physical command.
Such as: The `ShowTables` output attributes `namespace`, but `ShowTablesCommand` output attributes `database`.
As the query plan, this PR pass the output attributes from `ShowTables` to `ShowTablesCommand`, `ShowTableExtended ` to `ShowTablesCommand`.
Take `show tables` and `show table extended like 'tbl'` as example.
The output before this PR:
`show tables`
|database|tableName|isTemporary|
-- | -- | --
| default| tbl| false|
If catalog is v2 session catalog, the output before this PR:
|namespace|tableName|
-- | --
| default| tbl
`show table extended like 'tbl'`
|database|tableName|isTemporary| information|
-- | -- | -- | --
| default| tbl| false|Database: default...|
The output after this PR:
`show tables`
|namespace|tableName|isTemporary|
-- | -- | --
| default| tbl| false|
`show table extended like 'tbl'`
|namespace|tableName|isTemporary| information|
-- | -- | -- | --
| default| tbl| false|Database: default...|
### Why are the changes needed?
This PR have benefits as follows:
First, Unify schema for the output of SHOW TABLES.
Second, pass the output attributes could keep the expr ID unchanged, so that avoid bugs when we apply more operators above the command output dataframe.
### Does this PR introduce _any_ user-facing change?
Yes.
The output schema of `SHOW TABLES` replace `database` by `namespace`.
### How was this patch tested?
Jenkins test.
Closes#31245 from beliefer/SPARK-34157.
Lead-authored-by: gengjiaan <gengjiaan@360.cn>
Co-authored-by: beliefer <beliefer@163.com>
Signed-off-by: Wenchen Fan <wenchen@databricks.com>
### What changes were proposed in this pull request?
This pr format DateLiteral and TimestampLiteral toString. For example:
```sql
SELECT * FROM date_dim WHERE d_date BETWEEN (cast('2000-03-11' AS DATE) - INTERVAL 30 days) AND (cast('2000-03-11' AS DATE) + INTERVAL 30 days)
```
Before this pr:
```
Condition : (((isnotnull(d_date#18) AND (d_date#18 >= 10997)) AND (d_date#18 <= 11057))
```
After this pr:
```
Condition : (((isnotnull(d_date#14) AND (d_date#14 >= 2000-02-10)) AND (d_date#14 <= 2000-04-10))
```
### Why are the changes needed?
Make the plan more readable.
### Does this PR introduce _any_ user-facing change?
No.
### How was this patch tested?
Unit test.
Closes#31455 from wangyum/SPARK-34342.
Authored-by: Yuming Wang <yumwang@ebay.com>
Signed-off-by: Dongjoon Hyun <dhyun@apple.com>
### What changes were proposed in this pull request?
Rewrote one `ExtractGenerator` case such that it would not rely on a side effect of the flatmap function.
### Why are the changes needed?
With the dataframe api it is possible to have a lazy sequence as the `output` of a `LogicalPlan`. When exploding a column on this dataframe using the `withColumn("newName", explode(col("name")))` method, the `ExtractGenerator` does not extract the generator and `CheckAnalysis` would throw an exception.
### Does this PR introduce _any_ user-facing change?
Bugfix
Before this, the work around was to put `.select("*")` before the explode.
### How was this patch tested?
UT
Closes#31213 from tanelk/SPARK-34141_extract_generator.
Authored-by: tanel.kiis@gmail.com <tanel.kiis@gmail.com>
Signed-off-by: Sean Owen <srowen@gmail.com>
### What changes were proposed in this pull request?
This is a follow-up of https://github.com/apache/spark/pull/28027https://github.com/apache/spark/pull/28027 added a DS v2 API that allows data sources to produce metadata/hidden columns that can only be seen when it's explicitly selected. The way we integrate this API into Spark is:
1. The v2 relation gets normal output and metadata output from the data source, and the metadata output is excluded from the plan output by default.
2. column resolution can resolve `UnresolvedAttribute` with metadata columns, even if the child plan doesn't output metadata columns.
3. An analyzer rule searches the query plan, trying to find a node that has missing inputs. If such node is found, transform the sub-plan of this node, and update the v2 relation to include the metadata output.
The analyzer rule in step 3 brings a perf regression, for queries that do not read v2 tables at all. This rule will calculate `QueryPlan.inputSet` (which builds an `AttributeSet` from outputs of all children) and `QueryPlan.missingInput` (which does a set exclusion and creates a new `AttributeSet`) for every plan node in the query plan. In our benchmark, the TPCDS query compilation time gets increased by more than 10%
This PR proposes a simple way to improve it: we add a special metadata entry to the metadata attribute, which allows us to quickly check if a plan needs to add metadata columns: we just check all the references of this plan, and see if the attribute contains the special metadata entry, instead of calculating `QueryPlan.missingInput`.
This PR also fixes one bug: we should not change the final output schema of the plan, if we only use metadata columns in operators like filter, sort, etc.
### Why are the changes needed?
Fix perf regression in SQL query compilation, and fix a bug.
### Does this PR introduce _any_ user-facing change?
No
### How was this patch tested?
Run `org.apache.spark.sql.TPCDSQuerySuite`, before this PR, `AddMetadataColumns` is the top 4 rule ranked by running time
```
=== Metrics of Analyzer/Optimizer Rules ===
Total number of runs: 407641
Total time: 47.257239779 seconds
Rule Effective Time / Total Time Effective Runs / Total Runs
OptimizeSubqueries 4157690003 / 8485444626 49 / 2778
Analyzer$ResolveAggregateFunctions 1238968711 / 3369351761 49 / 2141
ColumnPruning 660038236 / 2924755292 338 / 6391
Analyzer$AddMetadataColumns 0 / 2918352992 0 / 2151
```
after this PR:
```
Analyzer$AddMetadataColumns 0 / 122885629 0 / 2151
```
This rule is 20 times faster and is negligible to the total compilation time.
This PR also add new tests to verify the bug fix.
Closes#31440 from cloud-fan/metadata-col.
Authored-by: Wenchen Fan <wenchen@databricks.com>
Signed-off-by: Wenchen Fan <wenchen@databricks.com>
### What changes were proposed in this pull request?
This is a followup of https://github.com/apache/spark/pull/26006
In #26006 , we merged the v1 and v2 SHOW DATABASES/NAMESPACES commands, but we missed a behavior change that the output schema of SHOW DATABASES becomes different.
This PR adds a legacy config to restore the old schema, with a migration guide item to mention this behavior change.
### Why are the changes needed?
Improve backward compatibility
### Does this PR introduce _any_ user-facing change?
No (the legacy config is false by default)
### How was this patch tested?
a new test
Closes#31474 from cloud-fan/command-schema.
Lead-authored-by: Wenchen Fan <cloud0fan@gmail.com>
Co-authored-by: Wenchen Fan <wenchen@databricks.com>
Signed-off-by: Wenchen Fan <wenchen@databricks.com>
### What changes were proposed in this pull request?
This is a follow up to #31424, and proposes to use `UnresolvedTable.relationTypeMismatchHint` when `UnresolvedTable` is resolved to a temp view.
### Why are the changes needed?
This change utilizes the type mismatch hint when a relation is resolved to a temp view when a table is expected.
For example, `ALTER TABLE tmpView SET TBLPROPERTIES ('p' = 'an')` will now include `Please use ALTER VIEW instead.` in the exception message: `tmpView is a temp view. 'ALTER TABLE ... SET TBLPROPERTIES' expects a table. Please use ALTER VIEW instead.`
### Does this PR introduce _any_ user-facing change?
Yes, adds the hint in the exception message.
### How was this patch tested?
Update existing tests to include the hint.
Closes#31452 from imback82/followup_SPARK-34317.
Authored-by: Terry Kim <yuminkim@gmail.com>
Signed-off-by: Wenchen Fan <wenchen@databricks.com>
### What changes were proposed in this pull request?
This PR adds support for referencing subquery with column aliases by its table alias.
Before
```sql
-- AnalysisException: cannot resolve '`t.c1`' given input columns: [c1, c2];
SELECT t.c1, t.c2 FROM (SELECT 1 AS a, 1 AS b) t(c1, c2)
```
After:
```sql
-- [(1, 1)]
SELECT t.c1, t.c2 FROM (SELECT 1 AS a, 1 AS b) t(c1, c2)
```
### Why are the changes needed?
To allow users to reference subquery with column aliases by its table alias.
### Does this PR introduce _any_ user-facing change?
Yes
### How was this patch tested?
Added parser tests and SQL query tests.
Closes#31444 from allisonwang-db/spark-34335.
Authored-by: allisonwang-db <66282705+allisonwang-db@users.noreply.github.com>
Signed-off-by: Wenchen Fan <wenchen@databricks.com>
### What changes were proposed in this pull request?
This PR proposes to migrate `ALTER TABLE ... SET/UNSET TBLPROPERTIES` to use `UnresolvedTable` to resolve the table identifier. This allows consistent resolution rules (temp view first, etc.) to be applied for both v1/v2 commands. More info about the consistent resolution rule proposal can be found in [JIRA](https://issues.apache.org/jira/browse/SPARK-29900) or [proposal doc](https://docs.google.com/document/d/1hvLjGA8y_W_hhilpngXVub1Ebv8RsMap986nENCFnrg/edit?usp=sharing).
### Why are the changes needed?
This is a part of effort to make the relation lookup behavior consistent: [SPARK-29900](https://issues.apache.org/jira/browse/SPARK-29900).
### Does this PR introduce _any_ user-facing change?
After this PR, `ALTER TABLE SET/UNSET TBLPROPERTIES` will have a consistent resolution behavior.
### How was this patch tested?
Updated existing tests / added new tests.
Closes#31422 from imback82/v2_alter_table_set_unset_properties.
Authored-by: Terry Kim <yuminkim@gmail.com>
Signed-off-by: Wenchen Fan <wenchen@databricks.com>
### What changes were proposed in this pull request?
Similar to SPARK-33690, this PR improves the output layout of `printSchema` for the case column names contain meta characters.
Here is an example.
Before:
```
scala> val df1 = spark.sql("SELECT 'aaa\nbbb\tccc\rddd\feee\bfff\u000Bggg\u0007hhh'")
scala> df1.printSchema
root
|-- aaa
ddd ccc
eefff
ggghhh: string (nullable = false)
```
After:
```
scala> df1.printSchema
root
|-- aaa\nbbb\tccc\rddd\feee\bfff\vggg\ahhh: string (nullable = false)
```
### Why are the changes needed?
To avoid breaking the layout of `Dataset#printSchema`
### Does this PR introduce _any_ user-facing change?
No.
### How was this patch tested?
New test.
Closes#31412 from sarutak/escape-meta-printSchema.
Authored-by: Kousuke Saruta <sarutak@oss.nttdata.com>
Signed-off-by: HyukjinKwon <gurwls223@apache.org>
### What changes were proposed in this pull request?
In the current master, the code for treating unicode/octal/escaped characters in string literals is a little bit complex so let's simplify it.
### Why are the changes needed?
To keep it easy to maintain.
### Does this PR introduce _any_ user-facing change?
No.
### How was this patch tested?
`ParserUtilsSuite` passes.
Closes#31362 from sarutak/refactor-unicode-escapes.
Authored-by: Kousuke Saruta <sarutak@oss.nttdata.com>
Signed-off-by: Kousuke Saruta <sarutak@oss.nttdata.com>
### What changes were proposed in this pull request?
1. Move parser tests from `DDLParserSuite` to `TruncateTableParserSuite`.
2. Port DS v1 tests from `DDLSuite` and other test suites to `v1.TruncateTableSuiteBase` and to `v1.TruncateTableSuite`.
3. Add a test for DSv2 `TRUNCATE TABLE` to `v2.TruncateTableSuite`.
### Why are the changes needed?
To improve test coverage.
### Does this PR introduce _any_ user-facing change?
No
### How was this patch tested?
By running new test suites:
```
$ build/sbt -Phive-2.3 -Phive-thriftserver "test:testOnly *TruncateTableSuite"
$ build/sbt -Phive-2.3 -Phive-thriftserver "test:testOnly *CatalogedDDLSuite"
```
Closes#31387 from MaxGekk/unify-truncate-table-tests.
Authored-by: Max Gekk <max.gekk@gmail.com>
Signed-off-by: Wenchen Fan <wenchen@databricks.com>
### What changes were proposed in this pull request?
Correct the version of SQL configuration `spark.sql.legacy.parseNullPartitionSpecAsStringLiteral` from 3.2.0 to 3.0.2.
Also, revise the documentation and test case.
### Why are the changes needed?
The release version in https://github.com/apache/spark/pull/31421 was wrong.
### Does this PR introduce _any_ user-facing change?
No
### How was this patch tested?
Unit tests
Closes#31434 from gengliangwang/reviseVersion.
Authored-by: Gengliang Wang <gengliang.wang@databricks.com>
Signed-off-by: Wenchen Fan <wenchen@databricks.com>
### What changes were proposed in this pull request?
There exists some `Exception` for assert in fact. Such as:
`throw new IllegalStateException("[BUG] unexpected plan returned by `lookupV2Relation`: " + other)`
This kind `Exception` seems should not put in single dedicated files.
### Why are the changes needed?
Reduce the workload of auditing.
### Does this PR introduce _any_ user-facing change?
'No'.
### How was this patch tested?
Jenkins test.
Closes#31395 from beliefer/SPARK-33599-restore-assert.
Authored-by: gengjiaan <gengjiaan@360.cn>
Signed-off-by: Wenchen Fan <wenchen@databricks.com>
### What changes were proposed in this pull request?
This PR fixes an issue that `Dataset.colRegex` doesn't work with column names or qualifiers which contain newlines.
In the current master, if column names or qualifiers passed to `colRegex` contain newlines, it throws exception.
```
val df = Seq(1, 2, 3).toDF("test\n_column").as("test\n_table")
val col1 = df.colRegex("`tes.*\n.*mn`")
org.apache.spark.sql.AnalysisException: Cannot resolve column name "`tes.*
.*mn`" among (test
_column)
at org.apache.spark.sql.Dataset.org$apache$spark$sql$Dataset$$resolveException(Dataset.scala:272)
at org.apache.spark.sql.Dataset.$anonfun$resolve$1(Dataset.scala:263)
at scala.Option.getOrElse(Option.scala:189)
at org.apache.spark.sql.Dataset.resolve(Dataset.scala:263)
at org.apache.spark.sql.Dataset.colRegex(Dataset.scala:1407)
... 47 elided
val col2 = df.colRegex("test\n_table.`tes.*\n.*mn`")
org.apache.spark.sql.AnalysisException: Cannot resolve column name "test
_table.`tes.*
.*mn`" among (test
_column)
at org.apache.spark.sql.Dataset.org$apache$spark$sql$Dataset$$resolveException(Dataset.scala:272)
at org.apache.spark.sql.Dataset.$anonfun$resolve$1(Dataset.scala:263)
at scala.Option.getOrElse(Option.scala:189)
at org.apache.spark.sql.Dataset.resolve(Dataset.scala:263)
at org.apache.spark.sql.Dataset.colRegex(Dataset.scala:1407)
... 47 elided
```
### Why are the changes needed?
Column names and qualifiers can contain newlines but `colRegex` can't work with them, so it's a bug.
### Does this PR introduce _any_ user-facing change?
Yes. users can pass column names and qualifiers even though they contain newlines.
### How was this patch tested?
New test.
Closes#31426 from sarutak/fix-colRegex.
Authored-by: Kousuke Saruta <sarutak@oss.nttdata.com>
Signed-off-by: Takeshi Yamamuro <yamamuro@apache.org>
### What changes were proposed in this pull request?
1. Add new method `truncatePartition()` to the `SupportsPartitionManagement` interface.
2. Add new method `truncatePartitions()` to the `SupportsAtomicPartitionManagement` interface.
3. Default implementation of new methods in `InMemoryPartitionTable`/`InMemoryAtomicPartitionTable`.
### Why are the changes needed?
This is the first step in supporting of v2 `TRUNCATE TABLE .. PARTITION`.
### Does this PR introduce _any_ user-facing change?
No
### How was this patch tested?
By running new tests:
```
$ build/sbt "test:testOnly *SupportsPartitionManagementSuite"
$ build/sbt "test:testOnly *SupportsAtomicPartitionManagementSuite"
```
Closes#31420 from MaxGekk/dsv2-truncate-table-partitions.
Authored-by: Max Gekk <max.gekk@gmail.com>
Signed-off-by: Wenchen Fan <wenchen@databricks.com>
### What changes were proposed in this pull request?
This PR proposes to add `relationTypeMismatchHint` to `UnresolvedTable` so that if a relation is resolved to a view when a table is expected, a hint message can be included as a part of the analysis exception message. Note that the same feature is already introduced to `UnresolvedView` in #30636.
This mostly affects `ALTER TABLE` commands where the analysis exception message will now contain `Please use ALTER VIEW as instead`.
### Why are the changes needed?
To give a better error message. (The hint used to exist but got removed for commands that migrated to the new resolution framework)
### Does this PR introduce _any_ user-facing change?
Yes, now `ALTER TABLE` commands include a hint to use `ALTER VIEW` instead.
```
sql("ALTER TABLE v SET SERDE 'whatever'")
```
Before:
```
"v is a view. 'ALTER TABLE ... SET [SERDE|SERDEPROPERTIES]' expects a table.
```
After this PR:
```
"v is a view. 'ALTER TABLE ... SET [SERDE|SERDEPROPERTIES]' expects a table. Please use ALTER VIEW instead.
```
### How was this patch tested?
Updated existing test cases to include the hint.
Closes#31424 from imback82/better_error.
Authored-by: Terry Kim <yuminkim@gmail.com>
Signed-off-by: Wenchen Fan <wenchen@databricks.com>
### What changes were proposed in this pull request?
In spark, the `count(table.*)` may cause very weird result, for example:
```
select count(*) from (select 1 as a, null as b) t;
output: 1
select count(t.*) from (select 1 as a, null as b) t;
output: 0
```
This is because spark expands `t.*` while converts `*` to count(1), this will confuse
users. After checking the ANSI standard, `count(*)` should always be `count(1)` while `count(t.*)`
is not allowed. What's more, this is also not allowed by common databases, e.g. MySQL, Oracle.
So, this PR proposes to block the ambiguous behavior and print a clear error message for users.
### Why are the changes needed?
to avoid ambiguous behavior and follow ANSI standard and other SQL engines
### Does this PR introduce _any_ user-facing change?
Yes, `count(table.*)` behavior will be blocked and output an error message.
### How was this patch tested?
newly added and existing tests
Closes#31286 from linhongliu-db/fix-table-star.
Authored-by: Linhong Liu <linhong.liu@databricks.com>
Signed-off-by: Wenchen Fan <wenchen@databricks.com>
### What changes were proposed in this pull request?
This is a follow up for https://github.com/apache/spark/pull/30538.
It adds a legacy conf `spark.sql.legacy.parseNullPartitionSpecAsStringLiteral` in case users wants the legacy behavior.
It also adds document for the behavior change.
### Why are the changes needed?
In case users want the legacy behavior, they can set `spark.sql.legacy.parseNullPartitionSpecAsStringLiteral` as true.
### Does this PR introduce _any_ user-facing change?
Yes, adding a legacy configuration to restore the old behavior.
### How was this patch tested?
Unit test.
Closes#31421 from gengliangwang/legacyNullStringConstant.
Authored-by: Gengliang Wang <gengliang.wang@databricks.com>
Signed-off-by: HyukjinKwon <gurwls223@apache.org>
### What changes were proposed in this pull request?
This PR completes snake_case rule at functions APIs across the languages, see also SPARK-10621.
In more details, this PR:
- Adds `count_distinct` in Scala Python, and R, and document that `count_distinct` is encouraged. This was not deprecated because `countDistinct` is pretty commonly used. We could deprecate in the future releases.
- (Scala-specific) adds `typedlit` but doesn't deprecate `typedLit` which is arguably commonly used. Likewise, we could deprecate in the future releases.
- Deprecates and renames:
- `sumDistinct` -> `sum_distinct`
- `bitwiseNOT` -> `bitwise_not`
- `shiftLeft` -> `shiftleft` (matched with SQL name in `FunctionRegistry`)
- `shiftRight` -> `shiftright` (matched with SQL name in `FunctionRegistry`)
- `shiftRightUnsigned` -> `shiftrightunsigned` (matched with SQL name in `FunctionRegistry`)
- (Scala-specific) `callUDF` -> `call_udf`
### Why are the changes needed?
To keep the consistent naming in APIs.
### Does this PR introduce _any_ user-facing change?
Yes, it deprecates some APIs and add new renamed APIs as described above.
### How was this patch tested?
Unittests were added.
Closes#31408 from HyukjinKwon/SPARK-34306.
Authored-by: HyukjinKwon <gurwls223@apache.org>
Signed-off-by: HyukjinKwon <gurwls223@apache.org>
### What changes were proposed in this pull request?
Replaces `collection.map(f1).flatten(f2)` with `collection.flatMap` if possible. it's semantically consistent, but looks simpler.
### Why are the changes needed?
Code Simpilefications.
### Does this PR introduce _any_ user-facing change?
No
### How was this patch tested?
Pass the Jenkins or GitHub Action
Closes#31416 from LuciferYang/SPARK-34310.
Authored-by: yangjie01 <yangjie01@baidu.com>
Signed-off-by: Sean Owen <srowen@gmail.com>
### What changes were proposed in this pull request?
Passing around the output attributes should have more benefits like keeping the expr ID unchanged to avoid bugs when we apply more operators above the command output dataframe.
This PR keep SHOW COLUMNS command's output attribute exprId unchanged.
### Why are the changes needed?
Keep SHOW PARTITIONS command's output attribute exprid unchanged.
### Does this PR introduce _any_ user-facing change?
No
### How was this patch tested?
Added UT
Closes#31377 from AngersZhuuuu/SPARK-34239.
Authored-by: Angerszhuuuu <angers.zhu@gmail.com>
Signed-off-by: Wenchen Fan <wenchen@databricks.com>
### What changes were proposed in this pull request?
1. Remove old statement `AlterTableSetLocationStatement`
2. Introduce new command `AlterTableSetLocation` for `ALTER TABLE .. SET LOCATION`.
### Why are the changes needed?
This is a part of effort to make the relation lookup behavior consistent: SPARK-29900.
### Does this PR introduce _any_ user-facing change?
It can change the error message for views.
### How was this patch tested?
By running `ALTER TABLE .. SET LOCATION` tests:
```
$ build/sbt -Phive -Phive-thriftserver "test:testOnly *DataSourceV2SQLSuite"
$ build/sbt -Phive -Phive-thriftserver "test:testOnly *CatalogedDDLSuite"
```
Closes#31414 from MaxGekk/migrate-set-location-resolv-table.
Authored-by: Max Gekk <max.gekk@gmail.com>
Signed-off-by: Wenchen Fan <wenchen@databricks.com>
### What changes were proposed in this pull request?
Describe `SessionCatalog.refreshTable()` and `CatalogImpl.refreshTable()`. what they do and when they are supposed to be used.
### Why are the changes needed?
To improve code maintenance.
### Does this PR introduce _any_ user-facing change?
No
### How was this patch tested?
By running `./dev/scalastyle`
Closes#31364 from MaxGekk/doc-refreshTable.
Authored-by: Max Gekk <max.gekk@gmail.com>
Signed-off-by: Wenchen Fan <wenchen@databricks.com>
### What changes were proposed in this pull request?
This PR group exception messages in `/catalyst/src/main/scala/org/apache/spark/sql/catalyst/parser`.
### Why are the changes needed?
It will largely help with standardization of error messages and its maintenance.
### Does this PR introduce _any_ user-facing change?
No. Error messages remain unchanged.
### How was this patch tested?
No new tests - pass all original tests to make sure it doesn't break any existing behavior.
Closes#31293 from beliefer/SPARK-33601.
Authored-by: beliefer <beliefer@163.com>
Signed-off-by: Wenchen Fan <wenchen@databricks.com>
### What changes were proposed in this pull request?
The currently SQL (temp or permanent) view resolution is done in 2 steps:
1. In `SessionCatalog`, we get the view metadata, parse the view SQL string, and wrap it with `View`.
2. At the beginning of the optimizer, we run `EliminateView`, which drops the wrapper `View`, and apply some special logic to match the view schema.
Step 2 is tricky, as we need to retain the output attr expr id, while we need to add an extra `Project` to add cast and alias. This PR simplifies the view solution by building a completed plan (with cast and alias added) in `SessionCatalog`, so that we only have 1 step.
### Why are the changes needed?
Code simplification. It also fixes issues like https://github.com/apache/spark/pull/31352
### Does this PR introduce _any_ user-facing change?
No
### How was this patch tested?
existing tests
Closes#31368 from cloud-fan/try.
Authored-by: Wenchen Fan <wenchen@databricks.com>
Signed-off-by: Wenchen Fan <wenchen@databricks.com>
### What changes were proposed in this pull request?
This PR follows up https://github.com/apache/spark/pull/30870.
Maybe some contributors don't know the job and added some exception by the old way.
### Why are the changes needed?
It will largely help with standardization of error messages and its maintenance.
### Does this PR introduce _any_ user-facing change?
No. Error messages remain unchanged.
### How was this patch tested?
No new tests - pass all original tests to make sure it doesn't break any existing behavior.
Closes#31312 from beliefer/SPARK-33542-followup.
Authored-by: beliefer <beliefer@163.com>
Signed-off-by: Wenchen Fan <wenchen@databricks.com>
### What changes were proposed in this pull request?
This pr correct the documentation of the `concat_ws` function.
### Why are the changes needed?
`concat_ws` doesn't need any str or array(str) arguments:
```
scala> sql("""select concat_ws("s")""").show
+------------+
|concat_ws(s)|
+------------+
| |
+------------+
```
### Does this PR introduce _any_ user-facing change?
No.
### How was this patch tested?
```
build/sbt "sql/testOnly *.ExpressionInfoSuite"
```
Closes#31370 from wangyum/SPARK-34268.
Authored-by: Yuming Wang <yumwang@ebay.com>
Signed-off-by: HyukjinKwon <gurwls223@apache.org>
### What changes were proposed in this pull request?
This PR changes the column types in the table definitions of `TPCDSBase` from string to char and varchar, with respect to the original definitions for char/varchar columns in the official doc - [TPC-DS_v2.9.0](http://www.tpc.org/tpc_documents_current_versions/pdf/tpc-ds_v2.9.0.pdf).
### Why are the changes needed?
Comply with both TPCDS standard and ANSI, and using string will get wrong results with those TPCDS queries
### Does this PR introduce _any_ user-facing change?
no
### How was this patch tested?
plan stability check
Closes#31012 from yaooqinn/tpcds.
Authored-by: Kent Yao <yao@apache.org>
Signed-off-by: Wenchen Fan <wenchen@databricks.com>
### What changes were proposed in this pull request?
we need to check whether the `lit` is null before calling `numChars`
### Why are the changes needed?
fix an obvious NPE bug
### Does this PR introduce _any_ user-facing change?
no
### How was this patch tested?
new tests
Closes#31336 from yaooqinn/SPARK-34233.
Authored-by: Kent Yao <yao@apache.org>
Signed-off-by: Wenchen Fan <wenchen@databricks.com>
### What changes were proposed in this pull request?
For v2 static partitions overwriting, we use `EqualTo ` to generate the `deleteExpr`
This is not right for null partition values, and cause the problem like below because `ConstantFolding` converts it to lit(null)
```scala
SPARK-34223: static partition with null raise NPE *** FAILED *** (19 milliseconds)
[info] org.apache.spark.sql.AnalysisException: Cannot translate expression to source filter: null
[info] at org.apache.spark.sql.execution.datasources.v2.V2Writes$$anonfun$apply$1.$anonfun$applyOrElse$1(V2Writes.scala:50)
[info] at scala.collection.immutable.List.flatMap(List.scala:366)
[info] at org.apache.spark.sql.execution.datasources.v2.V2Writes$$anonfun$apply$1.applyOrElse(V2Writes.scala:47)
[info] at org.apache.spark.sql.execution.datasources.v2.V2Writes$$anonfun$apply$1.applyOrElse(V2Writes.scala:39)
[info] at org.apache.spark.sql.catalyst.trees.TreeNode.$anonfun$transformDown$1(TreeNode.scala:317)
[info] at org.apache.spark.sql.catalyst.trees.CurrentOrigin$.withOrigin(TreeNode.scala:73)
```
The right way is to use EqualNullSafe instead to delete the null partitions.
### Why are the changes needed?
bugfix
### Does this PR introduce _any_ user-facing change?
no
### How was this patch tested?
an original test to new place
Closes#31339 from yaooqinn/SPARK-34236.
Authored-by: Kent Yao <yao@apache.org>
Signed-off-by: Wenchen Fan <wenchen@databricks.com>
### What changes were proposed in this pull request?
When write test about command, when `checkAnswer`,
Always got error as below
```
[info] AttributeSet(partition#607) was not empty The analyzed logical plan has missing inputs:
[info] ShowPartitionsCommand `ns`.`tbl`, [partition#607] (QueryTest.scala:224)
[info] org.scalatest.exceptions.TestFailedException:
[info] at org.scalatest.Assertions.newAssertionFailedException(Assertions.scala:472)
[info] at org.scalatest.Assertions.newAssertionFailedException$(Assertions.scala:471)
```
For Command DDL plan, we can define `producedAttributes` as it's `outputSet` and it's reasonable
### Why are the changes needed?
Add default `producedAttributes` for Command LogicalPlan
### Does this PR introduce _any_ user-facing change?
No
### How was this patch tested?
Not need
Closes#31342 from AngersZhuuuu/SPARK-34241.
Authored-by: Angerszhuuuu <angers.zhu@gmail.com>
Signed-off-by: Wenchen Fan <wenchen@databricks.com>