Code is not generated for LocalTableScanExec although proper situations.
If a LocalTableScanExec plan has the direct parent plan which supports WholeStageCodegen,
the LocalTableScanExec plan also should be within a WholeStageCodegen domain.
But code is not generated for LocalTableScanExec and InputAdapter is inserted for now.
```
val df1 = spark.createDataset(1 to 10).toDF
val df2 = spark.createDataset(1 to 10).toDF
val df3 = df1.join(df2, df1("value") === df2("value"))
df3.explain(true)
...
== Physical Plan ==
*(1) BroadcastHashJoin [value#1], [value#6], Inner, BuildRight
:- LocalTableScan [value#1] // LocalTableScanExec is not within a WholeStageCodegen domain
+- BroadcastExchange HashedRelationBroadcastMode(List(cast(input[0, int, false] as bigint)))
+- LocalTableScan [value#6]
```
```
scala> df3.queryExecution.executedPlan.children.head.children.head.getClass
res4: Class[_ <: org.apache.spark.sql.execution.SparkPlan] = class org.apache.spark.sql.execution.InputAdapter
```
For the current implementation of LocalTableScanExec, codegen is enabled in case `parent` is not null
but `parent` is set in `consume`, which is called after `insertInputAdapter` so it doesn't work as intended.
After applying this cnahge, we can get following plan, which means LocalTableScanExec is within a WholeStageCodegen domain.
```
== Physical Plan ==
*(1) BroadcastHashJoin [value#63], [value#68], Inner, BuildRight
:- *(1) LocalTableScan [value#63]
+- BroadcastExchange HashedRelationBroadcastMode(List(cast(input[0, int, false] as bigint)))
+- LocalTableScan [value#68]
## How was this patch tested?
New test cases are added into WholeStageCodegenSuite.
Closes#25260 from sarutak/localtablescan-improvement.
Authored-by: Kousuke Saruta <sarutak@oss.nttdata.com>
Signed-off-by: Takeshi Yamamuro <yamamuro@apache.org>
## What changes were proposed in this pull request?
Fix for ```SPARK-28441 (PythonUDF used in correlated scalar subquery causes UnsupportedOperationException)``` is in. Re-enable the commented out test for ```udf(max(udf(column))) ```
## How was this patch tested?
use existing test ```udf-except.sql```
Closes#25278 from huaxingao/spark-28277n.
Authored-by: Huaxin Gao <huaxing@us.ibm.com>
Signed-off-by: Dongjoon Hyun <dhyun@apple.com>
In case of CatalogFileIndex datasource table, sizeInBytes is always coming as default size in bytes, which is 8.0EB (Even when the user give fallBackToHdfsForStatsEnabled=true) . So, the datasource table which has CatalogFileIndex, always prefer SortMergeJoin, instead of BroadcastJoin, even though the size is below broadcast join threshold.
In this PR, In case of CatalogFileIndex table, if we enable "fallBackToHdfsForStatsEnabled=true", then the computeStatistics get the sizeInBytes from the hdfs and we get the actual size of the table. Hence, during join operation, when the table size is below broadcast threshold, it will prefer broadCastHashJoin instead of SortMergeJoin.
Added UT
Closes#22502 from shahidki31/SPARK-25474.
Authored-by: shahid <shahidki31@gmail.com>
Signed-off-by: Dongjoon Hyun <dhyun@apple.com>
## What changes were proposed in this pull request?
`ObjectAggregationIterator` shows a directional info message to increase `spark.sql.objectHashAggregate.sortBased.fallbackThreshold` when the size of the in-memory hash map grows too large and it falls back to sort-based aggregation.
However, we don't know how much we need to increase. This PR adds the size of the current in-memory hash map size to the log message.
**BEFORE**
```
15:21:41.669 Executor task launch worker for task 0 INFO
ObjectAggregationIterator: Aggregation hash map reaches threshold capacity (2 entries), ...
```
**AFTER**
```
15:20:05.742 Executor task launch worker for task 0 INFO
ObjectAggregationIterator: Aggregation hash map size 2 reaches threshold capacity (2 entries), ...
```
## How was this patch tested?
Manual. For example, run `ObjectHashAggregateSuite.scala`'s `typed_count fallback to sort-based aggregation` and search the above message in `target/unit-tests.log`.
Closes#25276 from dongjoon-hyun/SPARK-28545.
Authored-by: Dongjoon Hyun <dhyun@apple.com>
Signed-off-by: Dongjoon Hyun <dhyun@apple.com>
## What changes were proposed in this pull request?
This PR add support typed `interval` expression:
```sql
spark-sql> select interval 'interval 3 year 1 hour';
interval 3 years 1 hours
spark-sql>
```
Please note that this pr did not add a cast alias for `interval` type like [other types](2d74f14d74/sql/catalyst/src/main/scala/org/apache/spark/sql/catalyst/analysis/FunctionRegistry.scala (L529-L541)) because neither PostgreSQL nor Hive supports this syntax.
## How was this patch tested?
unit tests
Closes#25241 from wangyum/SPARK-28424.
Authored-by: Yuming Wang <yumwang@ebay.com>
Signed-off-by: Dongjoon Hyun <dhyun@apple.com>
## What changes were proposed in this pull request?
We should add `assume(shouldTestPythonUDFs)`. Maybe it's not a biggie in general but it can matter in other venders' testing base. For instance, if somebody launches a test in a minimal docker image, it might make the tests failed suddenly.
This skipping stuff isn't completely new in our test base. See `TestUtils.testCommandAvailable` for instance.
## How was this patch tested?
Manually tested.
Closes#25272 from HyukjinKwon/SPARK-28441.
Authored-by: HyukjinKwon <gurwls223@apache.org>
Signed-off-by: HyukjinKwon <gurwls223@apache.org>
## What changes were proposed in this pull request?
Since for AQP the cost for joins can change between multiple runs, there is no reason that we have an idempotence enforcement on this optimizer batch. We thus make it `FixedPoint(1)` instead of `Once`.
## How was this patch tested?
Existing UTs.
Closes#25266 from yeshengm/SPARK-28530.
Lead-authored-by: Yesheng Ma <kimi.ysma@gmail.com>
Co-authored-by: Xiao Li <gatorsmile@gmail.com>
Signed-off-by: gatorsmile <gatorsmile@gmail.com>
## What changes were proposed in this pull request?
In the Catalyst optimizer, the batch subquery actually calls the optimizer recursively. Therefore it makes no sense to enforce idempotence on it and we change this batch to `FixedPoint(1)`.
## How was this patch tested?
Existing UTs.
Closes#25267 from yeshengm/SPARK-28532.
Authored-by: Yesheng Ma <kimi.ysma@gmail.com>
Signed-off-by: gatorsmile <gatorsmile@gmail.com>
## What changes were proposed in this pull request?
In SPARK-15370, We checked the expression at the root of the correlated subquery, in order to fix count bug. If a `PythonUDF` in in the checking path, evaluating it causes the failure as we can't statically evaluate `PythonUDF`. The Python UDF test added at SPARK-28277 shows this issue.
If we can statically evaluate the expression, we intercept NULL values coming from the outer join and replace them with the value that the subquery's expression like before, if it is not, we replace them with the `PythonUDF` expression, with statically evaluated parameters.
After this, the last query in `udf-except.sql` which throws `java.lang.UnsupportedOperationException` can be run:
```
SELECT t1.k
FROM t1
WHERE t1.v <= (SELECT udf(max(udf(t2.v)))
FROM t2
WHERE udf(t2.k) = udf(t1.k))
MINUS
SELECT t1.k
FROM t1
WHERE udf(t1.v) >= (SELECT min(udf(t2.v))
FROM t2
WHERE t2.k = t1.k)
-- !query 2 schema
struct<k:string>
-- !query 2 output
two
```
Note that this issue is also for other non-foldable expressions, like rand. As like PythonUDF, we can't call `eval` on this kind of expressions in optimization. The evaluation needs to defer to query runtime.
## How was this patch tested?
Added tests.
Closes#25204 from viirya/SPARK-28441.
Authored-by: Liang-Chi Hsieh <viirya@gmail.com>
Signed-off-by: Wenchen Fan <wenchen@databricks.com>
## What changes were proposed in this pull request?
How to reproduce this issue:
```shell
build/sbt clean package -Phive -Phive-thriftserver -Phadoop-3.2
export SPARK_PREPEND_CLASSES=true
sbin/start-thriftserver.sh
[rootspark-3267648 spark]# bin/beeline -u jdbc:hive2://localhost:10000/default -e "select cast(1 as decimal(38, 18));"
Connecting to jdbc:hive2://localhost:10000/default
Connected to: Spark SQL (version 3.0.0-SNAPSHOT)
Driver: Hive JDBC (version 2.3.5)
Transaction isolation: TRANSACTION_REPEATABLE_READ
Error: java.lang.ClassCastException: java.math.BigDecimal incompatible with org.apache.hadoop.hive.common.type.HiveDecimal (state=,code=0)
Closing: 0: jdbc:hive2://localhost:10000/default
```
This pr fix this issue.
## How was this patch tested?
unit tests
Closes#25217 from wangyum/SPARK-28463.
Authored-by: Yuming Wang <yumwang@ebay.com>
Signed-off-by: gatorsmile <gatorsmile@gmail.com>
## What changes were proposed in this pull request?
In adaptive query processing (AQE), query plans are optimized on the fly during execution. However, a few `Once` rules can be problematic for such optimization since they can either generate wrong plan/unnecessary intermediate plan nodes.
This PR enforces idempotence for "Once" batches that are supposed to run once. This is a key enabler for AQE re-optimization and can improve robustness for existing optimizer rules.
Once batches that are currently not idempotent are marked in a blacklist. We will submit followup PRs to fix idempotence of these rules.
## How was this patch tested?
Existing UTs. Failing Once rules are temporarily blacklisted.
Closes#25249 from yeshengm/idempotence-checker.
Authored-by: Yesheng Ma <kimi.ysma@gmail.com>
Signed-off-by: gatorsmile <gatorsmile@gmail.com>
## What changes were proposed in this pull request?
This PR adds some tests converted from 'union.sql' to test UDFs
<details><summary>Diff comparing to 'union.sql'</summary>
<p>
```diff
diff --git a/sql/core/src/test/resources/sql-tests/results/union.sql.out b/sql/core/src/test/resources/sql-tests/results/udf/udf-union.sql.out
index b023df825d..84b5e10dbe 100644
--- a/sql/core/src/test/resources/sql-tests/results/union.sql.out
+++ b/sql/core/src/test/resources/sql-tests/results/udf/udf-union.sql.out
-19,10 +19,10 struct<>
-- !query 2
-SELECT *
-FROM (SELECT * FROM t1
+SELECT udf(c1) as c1, udf(c2) as c2
+FROM (SELECT udf(c1) as c1, udf(c2) as c2 FROM t1
UNION ALL
- SELECT * FROM t1)
+ SELECT udf(c1) as c1, udf(c2) as c2 FROM t1)
-- !query 2 schema
struct<c1:int,c2:string>
-- !query 2 output
-33,12 +33,12 struct<c1:int,c2:string>
-- !query 3
-SELECT *
-FROM (SELECT * FROM t1
+SELECT udf(c1) as c1, udf(c2) as c2
+FROM (SELECT udf(c1) as c1, udf(c2) as c2 FROM t1
UNION ALL
- SELECT * FROM t2
+ SELECT udf(c1) as c1, udf(c2) as c2 FROM t2
UNION ALL
- SELECT * FROM t2)
+ SELECT udf(c1) as c1, udf(c2) as c2 FROM t2)
-- !query 3 schema
struct<c1:decimal(11,1),c2:string>
-- !query 3 output
-51,11 +51,11 struct<c1:decimal(11,1),c2:string>
-- !query 4
-SELECT a
-FROM (SELECT 0 a, 0 b
+SELECT udf(udf(a)) as a
+FROM (SELECT udf(0) a, udf(0) b
UNION ALL
- SELECT SUM(1) a, CAST(0 AS BIGINT) b
- UNION ALL SELECT 0 a, 0 b) T
+ SELECT udf(SUM(1)) a, udf(CAST(0 AS BIGINT)) b
+ UNION ALL SELECT udf(0) a, udf(0) b) T
-- !query 4 schema
struct<a:bigint>
-- !query 4 output
-89,13 +89,13 struct<>
-- !query 8
-SELECT 1 AS x,
- col
-FROM (SELECT col AS col
- FROM (SELECT p1.col AS col
+SELECT udf(1) AS x,
+ udf(col) as col
+FROM (SELECT udf(col) AS col
+ FROM (SELECT udf(p1.col) AS col
FROM p1 CROSS JOIN p2
UNION ALL
- SELECT col
+ SELECT udf(col)
FROM p3) T1) T2
-- !query 8 schema
struct<x:int,col:int>
-105,9 +105,9 struct<x:int,col:int>
-- !query 9
-SELECT map(1, 2), 'str'
+SELECT map(1, 2), udf('str') as str
UNION ALL
-SELECT map(1, 2, 3, NULL), 1
+SELECT map(1, 2, 3, NULL), udf(1)
-- !query 9 schema
struct<map(1, 2):map<int,int>,str:string>
-- !query 9 output
-116,9 +116,9 struct<map(1, 2):map<int,int>,str:string>
-- !query 10
-SELECT array(1, 2), 'str'
+SELECT array(1, 2), udf('str') as str
UNION ALL
-SELECT array(1, 2, 3, NULL), 1
+SELECT array(1, 2, 3, NULL), udf(1)
-- !query 10 schema
struct<array(1, 2):array<int>,str:string>
-- !query 10 output
```
</p>
</details>
## How was this patch tested?
Tested as guided in SPARK-27921.
Closes#25202 from yiheng/fix_28289.
Authored-by: Yiheng Wang <yihengw@gmail.com>
Signed-off-by: HyukjinKwon <gurwls223@apache.org>
## What changes were proposed in this pull request?
This PR fixes the log messages like `attempt 0stage 9.0` by adding a comma followed by space. These are all instances in `DataWritingSparkTask` which was introduced at 6d16b9885d. This should be fixed in `branch-2.4`, too.
```
19/07/25 18:35:01 INFO DataWritingSparkTask: Commit authorized for partition 65 (task 153, attempt 0stage 9.0)
19/07/25 18:35:01 INFO DataWritingSparkTask: Committed partition 65 (task 153, attempt 0stage 9.0)
```
## How was this patch tested?
This only changes log messages. Pass the Jenkins with the existing tests.
Closes#25257 from dongjoon-hyun/DataWritingSparkTask.
Authored-by: Dongjoon Hyun <dhyun@apple.com>
Signed-off-by: HyukjinKwon <gurwls223@apache.org>
## What changes were proposed in this pull request?
Support multiple catalogs in the following InsertTable use cases:
- INSERT INTO [TABLE] catalog.db.tbl
- INSERT OVERWRITE TABLE catalog.db.tbl
Support matrix:
Overwrite|Partitioned Table|Partition Clause |Partition Overwrite Mode|Action
---------|-----------------|-----------------|------------------------|-----
false|*|*|*|AppendData
true|no|(empty)|*|OverwriteByExpression(true)
true|yes|p1,p2 or p1 or p2 or (empty)|STATIC|OverwriteByExpression(true)
true|yes|p2,p2 or p1 or p2 or (empty)|DYNAMIC|OverwritePartitionsDynamic
true|yes|p1=23,p2=3|*|OverwriteByExpression(p1=23 and p2=3)
true|yes|p1=23,p2 or p1=23|STATIC|OverwriteByExpression(p1=23)
true|yes|p1=23,p2 or p1=23|DYNAMIC|OverwritePartitionsDynamic
Notes:
- Assume the partitioned table has 2 partitions: p1 and p2.
- `STATIC` is the default Partition Overwrite Mode for data source tables.
- DSv2 tables currently do not support `IfPartitionNotExists`.
## How was this patch tested?
New tests.
All existing catalyst and sql/core tests.
Closes#24832 from jzhuge/SPARK-27845-pr.
Lead-authored-by: Ryan Blue <blue@apache.org>
Co-authored-by: John Zhuge <jzhuge@apache.org>
Signed-off-by: Burak Yavuz <brkyvz@gmail.com>
## What changes were proposed in this pull request?
This PR adds some tests converted from window.sql to test UDFs. Please see the contribution guide of this umbrella ticket - [SPARK-27921](https://issues.apache.org/jira/browse/SPARK-27921).
<details><summary>Diff comparing to 'xxx.sql'</summary>
<p>
```diff
diff --git a/sql/core/src/test/resources/sql-tests/results/window.sql.out b/sql/core/src/test/resources/sql-tests/results/udf/udf-window.sql.out
index 367dc4f513..9354d5e311 100644
--- a/sql/core/src/test/resources/sql-tests/results/window.sql.out
+++ b/sql/core/src/test/resources/sql-tests/results/udf/udf-window.sql.out
-21,10 +21,10 struct<>
-- !query 1
-SELECT val, cate, count(val) OVER(PARTITION BY cate ORDER BY val ROWS CURRENT ROW) FROM testData
-ORDER BY cate, val
+SELECT udf(val), cate, count(val) OVER(PARTITION BY cate ORDER BY udf(val) ROWS CURRENT ROW) FROM testData
+ORDER BY cate, udf(val)
-- !query 1 schema
-struct<val:int,cate:string,count(val) OVER (PARTITION BY cate ORDER BY val ASC NULLS FIRST ROWS BETWEEN CURRENT ROW AND CURRENT ROW):bigint>
+struct<CAST(udf(cast(val as string)) AS INT):int,cate:string,count(val) OVER (PARTITION BY cate ORDER BY CAST(udf(cast(val as string)) AS INT) ASC NULLS FIRST ROWS BETWEEN CURRENT ROW AND CURRENT ROW):bigint>
-- !query 1 output
NULL NULL 0
3 NULL 1
-38,10 +38,10 NULL a 0
-- !query 2
-SELECT val, cate, sum(val) OVER(PARTITION BY cate ORDER BY val
-ROWS BETWEEN UNBOUNDED PRECEDING AND 1 FOLLOWING) FROM testData ORDER BY cate, val
+SELECT udf(val), cate, sum(val) OVER(PARTITION BY cate ORDER BY udf(val)
+ROWS BETWEEN UNBOUNDED PRECEDING AND 1 FOLLOWING) FROM testData ORDER BY cate, udf(val)
-- !query 2 schema
-struct<val:int,cate:string,sum(val) OVER (PARTITION BY cate ORDER BY val ASC NULLS FIRST ROWS BETWEEN UNBOUNDED PRECEDING AND 1 FOLLOWING):bigint>
+struct<CAST(udf(cast(val as string)) AS INT):int,cate:string,sum(val) OVER (PARTITION BY cate ORDER BY CAST(udf(cast(val as string)) AS INT) ASC NULLS FIRST ROWS BETWEEN UNBOUNDED PRECEDING AND 1 FOLLOWING):bigint>
-- !query 2 output
NULL NULL 3
3 NULL 3
-55,20 +55,20 NULL a 1
-- !query 3
-SELECT val_long, cate, sum(val_long) OVER(PARTITION BY cate ORDER BY val_long
-ROWS BETWEEN CURRENT ROW AND 2147483648 FOLLOWING) FROM testData ORDER BY cate, val_long
+SELECT val_long, udf(cate), sum(val_long) OVER(PARTITION BY cate ORDER BY udf(val_long)
+ROWS BETWEEN CURRENT ROW AND 2147483648 FOLLOWING) FROM testData ORDER BY udf(cate), val_long
-- !query 3 schema
struct<>
-- !query 3 output
org.apache.spark.sql.AnalysisException
-cannot resolve 'ROWS BETWEEN CURRENT ROW AND 2147483648L FOLLOWING' due to data type mismatch: The data type of the upper bound 'bigint' does not match the expected data type 'int'.; line 1 pos 41
+cannot resolve 'ROWS BETWEEN CURRENT ROW AND 2147483648L FOLLOWING' due to data type mismatch: The data type of the upper bound 'bigint' does not match the expected data type 'int'.; line 1 pos 46
-- !query 4
-SELECT val, cate, count(val) OVER(PARTITION BY cate ORDER BY val RANGE 1 PRECEDING) FROM testData
-ORDER BY cate, val
+SELECT udf(val), cate, count(val) OVER(PARTITION BY udf(cate) ORDER BY val RANGE 1 PRECEDING) FROM testData
+ORDER BY cate, udf(val)
-- !query 4 schema
-struct<val:int,cate:string,count(val) OVER (PARTITION BY cate ORDER BY val ASC NULLS FIRST RANGE BETWEEN 1 PRECEDING AND CURRENT ROW):bigint>
+struct<CAST(udf(cast(val as string)) AS INT):int,cate:string,count(val) OVER (PARTITION BY CAST(udf(cast(cate as string)) AS STRING) ORDER BY val ASC NULLS FIRST RANGE BETWEEN 1 PRECEDING AND CURRENT ROW):bigint>
-- !query 4 output
NULL NULL 0
3 NULL 1
-82,10 +82,10 NULL a 0
-- !query 5
-SELECT val, cate, sum(val) OVER(PARTITION BY cate ORDER BY val
-RANGE BETWEEN CURRENT ROW AND 1 FOLLOWING) FROM testData ORDER BY cate, val
+SELECT val, udf(cate), sum(val) OVER(PARTITION BY udf(cate) ORDER BY val
+RANGE BETWEEN CURRENT ROW AND 1 FOLLOWING) FROM testData ORDER BY udf(cate), val
-- !query 5 schema
-struct<val:int,cate:string,sum(val) OVER (PARTITION BY cate ORDER BY val ASC NULLS FIRST RANGE BETWEEN CURRENT ROW AND 1 FOLLOWING):bigint>
+struct<val:int,CAST(udf(cast(cate as string)) AS STRING):string,sum(val) OVER (PARTITION BY CAST(udf(cast(cate as string)) AS STRING) ORDER BY val ASC NULLS FIRST RANGE BETWEEN CURRENT ROW AND 1 FOLLOWING):bigint>
-- !query 5 output
NULL NULL NULL
3 NULL 3
-99,10 +99,10 NULL a NULL
-- !query 6
-SELECT val_long, cate, sum(val_long) OVER(PARTITION BY cate ORDER BY val_long
-RANGE BETWEEN CURRENT ROW AND 2147483648 FOLLOWING) FROM testData ORDER BY cate, val_long
+SELECT val_long, udf(cate), sum(val_long) OVER(PARTITION BY udf(cate) ORDER BY val_long
+RANGE BETWEEN CURRENT ROW AND 2147483648 FOLLOWING) FROM testData ORDER BY udf(cate), val_long
-- !query 6 schema
-struct<val_long:bigint,cate:string,sum(val_long) OVER (PARTITION BY cate ORDER BY val_long ASC NULLS FIRST RANGE BETWEEN CURRENT ROW AND 2147483648 FOLLOWING):bigint>
+struct<val_long:bigint,CAST(udf(cast(cate as string)) AS STRING):string,sum(val_long) OVER (PARTITION BY CAST(udf(cast(cate as string)) AS STRING) ORDER BY val_long ASC NULLS FIRST RANGE BETWEEN CURRENT ROW AND 2147483648 FOLLOWING):bigint>
-- !query 6 output
NULL NULL NULL
1 NULL 1
-116,10 +116,10 NULL b NULL
-- !query 7
-SELECT val_double, cate, sum(val_double) OVER(PARTITION BY cate ORDER BY val_double
-RANGE BETWEEN CURRENT ROW AND 2.5 FOLLOWING) FROM testData ORDER BY cate, val_double
+SELECT val_double, udf(cate), sum(val_double) OVER(PARTITION BY udf(cate) ORDER BY val_double
+RANGE BETWEEN CURRENT ROW AND 2.5 FOLLOWING) FROM testData ORDER BY udf(cate), val_double
-- !query 7 schema
-struct<val_double:double,cate:string,sum(val_double) OVER (PARTITION BY cate ORDER BY val_double ASC NULLS FIRST RANGE BETWEEN CURRENT ROW AND CAST(2.5 AS DOUBLE) FOLLOWING):double>
+struct<val_double:double,CAST(udf(cast(cate as string)) AS STRING):string,sum(val_double) OVER (PARTITION BY CAST(udf(cast(cate as string)) AS STRING) ORDER BY val_double ASC NULLS FIRST RANGE BETWEEN CURRENT ROW AND CAST(2.5 AS DOUBLE) FOLLOWING):double>
-- !query 7 output
NULL NULL NULL
1.0 NULL 1.0
-133,10 +133,10 NULL NULL NULL
-- !query 8
-SELECT val_date, cate, max(val_date) OVER(PARTITION BY cate ORDER BY val_date
-RANGE BETWEEN CURRENT ROW AND 2 FOLLOWING) FROM testData ORDER BY cate, val_date
+SELECT val_date, udf(cate), max(val_date) OVER(PARTITION BY udf(cate) ORDER BY val_date
+RANGE BETWEEN CURRENT ROW AND 2 FOLLOWING) FROM testData ORDER BY udf(cate), val_date
-- !query 8 schema
-struct<val_date:date,cate:string,max(val_date) OVER (PARTITION BY cate ORDER BY val_date ASC NULLS FIRST RANGE BETWEEN CURRENT ROW AND 2 FOLLOWING):date>
+struct<val_date:date,CAST(udf(cast(cate as string)) AS STRING):string,max(val_date) OVER (PARTITION BY CAST(udf(cast(cate as string)) AS STRING) ORDER BY val_date ASC NULLS FIRST RANGE BETWEEN CURRENT ROW AND 2 FOLLOWING):date>
-- !query 8 output
NULL NULL NULL
2017-08-01 NULL 2017-08-01
-150,11 +150,11 NULL NULL NULL
-- !query 9
-SELECT val_timestamp, cate, avg(val_timestamp) OVER(PARTITION BY cate ORDER BY val_timestamp
+SELECT val_timestamp, udf(cate), avg(val_timestamp) OVER(PARTITION BY udf(cate) ORDER BY val_timestamp
RANGE BETWEEN CURRENT ROW AND interval 23 days 4 hours FOLLOWING) FROM testData
-ORDER BY cate, val_timestamp
+ORDER BY udf(cate), val_timestamp
-- !query 9 schema
-struct<val_timestamp:timestamp,cate:string,avg(CAST(val_timestamp AS DOUBLE)) OVER (PARTITION BY cate ORDER BY val_timestamp ASC NULLS FIRST RANGE BETWEEN CURRENT ROW AND interval 3 weeks 2 days 4 hours FOLLOWING):double>
+struct<val_timestamp:timestamp,CAST(udf(cast(cate as string)) AS STRING):string,avg(CAST(val_timestamp AS DOUBLE)) OVER (PARTITION BY CAST(udf(cast(cate as string)) AS STRING) ORDER BY val_timestamp ASC NULLS FIRST RANGE BETWEEN CURRENT ROW AND interval 3 weeks 2 days 4 hours FOLLOWING):double>
-- !query 9 output
NULL NULL NULL
2017-07-31 17:00:00 NULL 1.5015456E9
-168,10 +168,10 NULL NULL NULL
-- !query 10
-SELECT val, cate, sum(val) OVER(PARTITION BY cate ORDER BY val DESC
+SELECT val, udf(cate), sum(val) OVER(PARTITION BY cate ORDER BY val DESC
RANGE BETWEEN CURRENT ROW AND 1 FOLLOWING) FROM testData ORDER BY cate, val
-- !query 10 schema
-struct<val:int,cate:string,sum(val) OVER (PARTITION BY cate ORDER BY val DESC NULLS LAST RANGE BETWEEN CURRENT ROW AND 1 FOLLOWING):bigint>
+struct<val:int,CAST(udf(cast(cate as string)) AS STRING):string,sum(val) OVER (PARTITION BY cate ORDER BY val DESC NULLS LAST RANGE BETWEEN CURRENT ROW AND 1 FOLLOWING):bigint>
-- !query 10 output
NULL NULL NULL
3 NULL 3
-185,58 +185,58 NULL a NULL
-- !query 11
-SELECT val, cate, count(val) OVER(PARTITION BY cate
-ROWS BETWEEN UNBOUNDED FOLLOWING AND 1 FOLLOWING) FROM testData ORDER BY cate, val
+SELECT udf(val), cate, count(val) OVER(PARTITION BY udf(cate)
+ROWS BETWEEN UNBOUNDED FOLLOWING AND 1 FOLLOWING) FROM testData ORDER BY cate, udf(val)
-- !query 11 schema
struct<>
-- !query 11 output
org.apache.spark.sql.AnalysisException
-cannot resolve 'ROWS BETWEEN UNBOUNDED FOLLOWING AND 1 FOLLOWING' due to data type mismatch: Window frame upper bound '1' does not follow the lower bound 'unboundedfollowing$()'.; line 1 pos 33
+cannot resolve 'ROWS BETWEEN UNBOUNDED FOLLOWING AND 1 FOLLOWING' due to data type mismatch: Window frame upper bound '1' does not follow the lower bound 'unboundedfollowing$()'.; line 1 pos 38
-- !query 12
-SELECT val, cate, count(val) OVER(PARTITION BY cate
-RANGE BETWEEN CURRENT ROW AND 1 FOLLOWING) FROM testData ORDER BY cate, val
+SELECT udf(val), cate, count(val) OVER(PARTITION BY udf(cate)
+RANGE BETWEEN CURRENT ROW AND 1 FOLLOWING) FROM testData ORDER BY cate, udf(val)
-- !query 12 schema
struct<>
-- !query 12 output
org.apache.spark.sql.AnalysisException
-cannot resolve '(PARTITION BY testdata.`cate` RANGE BETWEEN CURRENT ROW AND 1 FOLLOWING)' due to data type mismatch: A range window frame cannot be used in an unordered window specification.; line 1 pos 33
+cannot resolve '(PARTITION BY CAST(udf(cast(cate as string)) AS STRING) RANGE BETWEEN CURRENT ROW AND 1 FOLLOWING)' due to data type mismatch: A range window frame cannot be used in an unordered window specification.; line 1 pos 38
-- !query 13
-SELECT val, cate, count(val) OVER(PARTITION BY cate ORDER BY val, cate
-RANGE BETWEEN CURRENT ROW AND 1 FOLLOWING) FROM testData ORDER BY cate, val
+SELECT udf(val), cate, count(val) OVER(PARTITION BY udf(cate) ORDER BY udf(val), cate
+RANGE BETWEEN CURRENT ROW AND 1 FOLLOWING) FROM testData ORDER BY cate, udf(val)
-- !query 13 schema
struct<>
-- !query 13 output
org.apache.spark.sql.AnalysisException
-cannot resolve '(PARTITION BY testdata.`cate` ORDER BY testdata.`val` ASC NULLS FIRST, testdata.`cate` ASC NULLS FIRST RANGE BETWEEN CURRENT ROW AND 1 FOLLOWING)' due to data type mismatch: A range window frame with value boundaries cannot be used in a window specification with multiple order by expressions: val#x ASC NULLS FIRST,cate#x ASC NULLS FIRST; line 1 pos 33
+cannot resolve '(PARTITION BY CAST(udf(cast(cate as string)) AS STRING) ORDER BY CAST(udf(cast(val as string)) AS INT) ASC NULLS FIRST, testdata.`cate` ASC NULLS FIRST RANGE BETWEEN CURRENT ROW AND 1 FOLLOWING)' due to data type mismatch: A range window frame with value boundaries cannot be used in a window specification with multiple order by expressions: cast(udf(cast(val#x as string)) as int) ASC NULLS FIRST,cate#x ASC NULLS FIRST; line 1 pos 38
-- !query 14
-SELECT val, cate, count(val) OVER(PARTITION BY cate ORDER BY current_timestamp
-RANGE BETWEEN CURRENT ROW AND 1 FOLLOWING) FROM testData ORDER BY cate, val
+SELECT udf(val), cate, count(val) OVER(PARTITION BY udf(cate) ORDER BY current_timestamp
+RANGE BETWEEN CURRENT ROW AND 1 FOLLOWING) FROM testData ORDER BY cate, udf(val)
-- !query 14 schema
struct<>
-- !query 14 output
org.apache.spark.sql.AnalysisException
-cannot resolve '(PARTITION BY testdata.`cate` ORDER BY current_timestamp() ASC NULLS FIRST RANGE BETWEEN CURRENT ROW AND 1 FOLLOWING)' due to data type mismatch: The data type 'timestamp' used in the order specification does not match the data type 'int' which is used in the range frame.; line 1 pos 33
+cannot resolve '(PARTITION BY CAST(udf(cast(cate as string)) AS STRING) ORDER BY current_timestamp() ASC NULLS FIRST RANGE BETWEEN CURRENT ROW AND 1 FOLLOWING)' due to data type mismatch: The data type 'timestamp' used in the order specification does not match the data type 'int' which is used in the range frame.; line 1 pos 38
-- !query 15
-SELECT val, cate, count(val) OVER(PARTITION BY cate ORDER BY val
-RANGE BETWEEN 1 FOLLOWING AND 1 PRECEDING) FROM testData ORDER BY cate, val
+SELECT udf(val), cate, count(val) OVER(PARTITION BY udf(cate) ORDER BY val
+RANGE BETWEEN 1 FOLLOWING AND 1 PRECEDING) FROM testData ORDER BY udf(cate), val
-- !query 15 schema
struct<>
-- !query 15 output
org.apache.spark.sql.AnalysisException
-cannot resolve 'RANGE BETWEEN 1 FOLLOWING AND 1 PRECEDING' due to data type mismatch: The lower bound of a window frame must be less than or equal to the upper bound; line 1 pos 33
+cannot resolve 'RANGE BETWEEN 1 FOLLOWING AND 1 PRECEDING' due to data type mismatch: The lower bound of a window frame must be less than or equal to the upper bound; line 1 pos 38
-- !query 16
-SELECT val, cate, count(val) OVER(PARTITION BY cate ORDER BY val
-RANGE BETWEEN CURRENT ROW AND current_date PRECEDING) FROM testData ORDER BY cate, val
+SELECT udf(val), cate, count(val) OVER(PARTITION BY udf(cate) ORDER BY udf(val)
+RANGE BETWEEN CURRENT ROW AND current_date PRECEDING) FROM testData ORDER BY cate, val(val)
-- !query 16 schema
struct<>
-- !query 16 output
-245,48 +245,48 org.apache.spark.sql.catalyst.parser.ParseException
Frame bound value must be a literal.(line 2, pos 30)
== SQL ==
-SELECT val, cate, count(val) OVER(PARTITION BY cate ORDER BY val
-RANGE BETWEEN CURRENT ROW AND current_date PRECEDING) FROM testData ORDER BY cate, val
+SELECT udf(val), cate, count(val) OVER(PARTITION BY udf(cate) ORDER BY udf(val)
+RANGE BETWEEN CURRENT ROW AND current_date PRECEDING) FROM testData ORDER BY cate, val(val)
------------------------------^^^
-- !query 17
-SELECT val, cate,
-max(val) OVER w AS max,
-min(val) OVER w AS min,
-min(val) OVER w AS min,
-count(val) OVER w AS count,
-sum(val) OVER w AS sum,
-avg(val) OVER w AS avg,
-stddev(val) OVER w AS stddev,
-first_value(val) OVER w AS first_value,
-first_value(val, true) OVER w AS first_value_ignore_null,
-first_value(val, false) OVER w AS first_value_contain_null,
-last_value(val) OVER w AS last_value,
-last_value(val, true) OVER w AS last_value_ignore_null,
-last_value(val, false) OVER w AS last_value_contain_null,
+SELECT udf(val), cate,
+max(udf(val)) OVER w AS max,
+min(udf(val)) OVER w AS min,
+min(udf(val)) OVER w AS min,
+count(udf(val)) OVER w AS count,
+sum(udf(val)) OVER w AS sum,
+avg(udf(val)) OVER w AS avg,
+stddev(udf(val)) OVER w AS stddev,
+first_value(udf(val)) OVER w AS first_value,
+first_value(udf(val), true) OVER w AS first_value_ignore_null,
+first_value(udf(val), false) OVER w AS first_value_contain_null,
+last_value(udf(val)) OVER w AS last_value,
+last_value(udf(val), true) OVER w AS last_value_ignore_null,
+last_value(udf(val), false) OVER w AS last_value_contain_null,
rank() OVER w AS rank,
dense_rank() OVER w AS dense_rank,
cume_dist() OVER w AS cume_dist,
percent_rank() OVER w AS percent_rank,
ntile(2) OVER w AS ntile,
row_number() OVER w AS row_number,
-var_pop(val) OVER w AS var_pop,
-var_samp(val) OVER w AS var_samp,
-approx_count_distinct(val) OVER w AS approx_count_distinct,
-covar_pop(val, val_long) OVER w AS covar_pop,
-corr(val, val_long) OVER w AS corr,
-stddev_samp(val) OVER w AS stddev_samp,
-stddev_pop(val) OVER w AS stddev_pop,
-collect_list(val) OVER w AS collect_list,
-collect_set(val) OVER w AS collect_set,
-skewness(val_double) OVER w AS skewness,
-kurtosis(val_double) OVER w AS kurtosis
+var_pop(udf(val)) OVER w AS var_pop,
+var_samp(udf(val)) OVER w AS var_samp,
+approx_count_distinct(udf(val)) OVER w AS approx_count_distinct,
+covar_pop(udf(val), udf(val_long)) OVER w AS covar_pop,
+corr(udf(val), udf(val_long)) OVER w AS corr,
+stddev_samp(udf(val)) OVER w AS stddev_samp,
+stddev_pop(udf(val)) OVER w AS stddev_pop,
+collect_list(udf(val)) OVER w AS collect_list,
+collect_set(udf(val)) OVER w AS collect_set,
+skewness(udf(val_double)) OVER w AS skewness,
+kurtosis(udf(val_double)) OVER w AS kurtosis
FROM testData
-WINDOW w AS (PARTITION BY cate ORDER BY val)
-ORDER BY cate, val
+WINDOW w AS (PARTITION BY udf(cate) ORDER BY udf(val))
+ORDER BY cate, udf(val)
-- !query 17 schema
-struct<val:int,cate:string,max:int,min:int,min:int,count:bigint,sum:bigint,avg:double,stddev:double,first_value:int,first_value_ignore_null:int,first_value_contain_null:int,last_value:int,last_value_ignore_null:int,last_value_contain_null:int,rank:int,dense_rank:int,cume_dist:double,percent_rank:double,ntile:int,row_number:int,var_pop:double,var_samp:double,approx_count_distinct:bigint,covar_pop:double,corr:double,stddev_samp:double,stddev_pop:double,collect_list:array<int>,collect_set:array<int>,skewness:double,kurtosis:double>
+struct<CAST(udf(cast(val as string)) AS INT):int,cate:string,max:int,min:int,min:int,count:bigint,sum:bigint,avg:double,stddev:double,first_value:int,first_value_ignore_null:int,first_value_contain_null:int,last_value:int,last_value_ignore_null:int,last_value_contain_null:int,rank:int,dense_rank:int,cume_dist:double,percent_rank:double,ntile:int,row_number:int,var_pop:double,var_samp:double,approx_count_distinct:bigint,covar_pop:double,corr:double,stddev_samp:double,stddev_pop:double,collect_list:array<int>,collect_set:array<int>,skewness:double,kurtosis:double>
-- !query 17 output
NULL NULL NULL NULL NULL 0 NULL NULL NULL NULL NULL NULL NULL NULL NULL 1 1 0.5 0.0 1 1 NULL NULL 0 NULL NULL
NULL NULL [] [] NULL NULL
3 NULL 3 3 3 1 3 3.0 NaN NULL 3 NULL 3 3 3 2 2 1.0 1.0 2 2 0.0 NaN 1 0.0 NaN
NaN 0.0 [3] [3] NaN NaN
-300,9 +300,9 NULL a NULL NULL NULL 0 NULL NULL NULL NULL NULL NULL NULL NULL NULL 1 1 0.25 0.
-- !query 18
-SELECT val, cate, avg(null) OVER(PARTITION BY cate ORDER BY val) FROM testData ORDER BY cate, val
+SELECT udf(val), cate, avg(null) OVER(PARTITION BY cate ORDER BY val) FROM testData ORDER BY cate, val
-- !query 18 schema
-struct<val:int,cate:string,avg(CAST(NULL AS DOUBLE)) OVER (PARTITION BY cate ORDER BY val ASC NULLS FIRST RANGE BETWEEN UNBOUNDED PRECEDING AND CURRENT ROW):double>
+struct<CAST(udf(cast(val as string)) AS INT):int,cate:string,avg(CAST(NULL AS DOUBLE)) OVER (PARTITION BY cate ORDER BY val ASC NULLS FIRST RANGE BETWEEN UNBOUNDED PRECEDING AND CURRENT ROW):double>
-- !query 18 output
NULL NULL NULL
3 NULL NULL
-316,7 +316,7 NULL a NULL
-- !query 19
-SELECT val, cate, row_number() OVER(PARTITION BY cate) FROM testData ORDER BY cate, val
+SELECT udf(val), cate, row_number() OVER(PARTITION BY cate) FROM testData ORDER BY cate, udf(val)
-- !query 19 schema
struct<>
-- !query 19 output
-325,9 +325,9 Window function row_number() requires window to be ordered, please add ORDER BY
-- !query 20
-SELECT val, cate, sum(val) OVER(), avg(val) OVER() FROM testData ORDER BY cate, val
+SELECT udf(val), cate, sum(val) OVER(), avg(val) OVER() FROM testData ORDER BY cate, val
-- !query 20 schema
-struct<val:int,cate:string,sum(CAST(val AS BIGINT)) OVER (ROWS BETWEEN UNBOUNDED PRECEDING AND UNBOUNDED FOLLOWING):bigint,avg(CAST(val AS BIGINT)) OVER (ROWS BETWEEN UNBOUNDED PRECEDING AND UNBOUNDED FOLLOWING):double>
+struct<CAST(udf(cast(val as string)) AS INT):int,cate:string,sum(CAST(val AS BIGINT)) OVER (ROWS BETWEEN UNBOUNDED PRECEDING AND UNBOUNDED FOLLOWING):bigint,avg(CAST(val AS BIGINT)) OVER (ROWS BETWEEN UNBOUNDED PRECEDING AND UNBOUNDED FOLLOWING):double>
-- !query 20 output
NULL NULL 13 1.8571428571428572
3 NULL 13 1.8571428571428572
-341,7 +341,7 NULL a 13 1.8571428571428572
-- !query 21
-SELECT val, cate,
+SELECT udf(val), cate,
first_value(false) OVER w AS first_value,
first_value(true, true) OVER w AS first_value_ignore_null,
first_value(false, false) OVER w AS first_value_contain_null,
-352,7 +352,7 FROM testData
WINDOW w AS ()
ORDER BY cate, val
-- !query 21 schema
-struct<val:int,cate:string,first_value:boolean,first_value_ignore_null:boolean,first_value_contain_null:boolean,last_value:boolean,last_value_ignore_null:boolean,last_value_contain_null:boolean>
+struct<CAST(udf(cast(val as string)) AS INT):int,cate:string,first_value:boolean,first_value_ignore_null:boolean,first_value_contain_null:boolean,last_value:boolean,last_value_ignore_null:boolean,last_value_contain_null:boolean>
-- !query 21 output
NULL NULL false true false false true false
3 NULL false true false false true false
-366,12 +366,12 NULL a false true false false true false
-- !query 22
-SELECT cate, sum(val) OVER (w)
+SELECT udf(cate), sum(val) OVER (w)
FROM testData
WHERE val is not null
WINDOW w AS (PARTITION BY cate ORDER BY val)
-- !query 22 schema
-struct<cate:string,sum(CAST(val AS BIGINT)) OVER (PARTITION BY cate ORDER BY val ASC NULLS FIRST RANGE BETWEEN UNBOUNDED PRECEDING AND CURRENT ROW):bigint>
+struct<CAST(udf(cast(cate as string)) AS STRING):string,sum(CAST(val AS BIGINT)) OVER (PARTITION BY cate ORDER BY val ASC NULLS FIRST RANGE BETWEEN UNBOUNDED PRECEDING AND CURRENT ROW):bigint>
-- !query 22 output
NULL 3
a 2
```
</p>
</details>
## How was this patch tested?
Tested as guided in [SPARK-27921](https://issues.apache.org/jira/browse/SPARK-27921).
Closes#25195 from younggyuchun/master.
Authored-by: younggyu chun <younggyuchun@gmail.com>
Signed-off-by: HyukjinKwon <gurwls223@apache.org>
## What changes were proposed in this pull request?
In the current implementation. complex types like Array/Map/StructType are allowed to upcast as StringType.
This is not safe casting. We should disallow it.
## How was this patch tested?
Update the existing test case
Closes#25242 from gengliangwang/fixUpCastStringType.
Authored-by: Gengliang Wang <gengliang.wang@databricks.com>
Signed-off-by: HyukjinKwon <gurwls223@apache.org>
## What changes were proposed in this pull request?
changed the test according to steps mentioned in SPARK-27921
<details>
<summary>difference comparing to select_having.sql</summary>
<p>
```diff
diff --git a/sql/core/src/test/resources/sql-tests/results/pgSQL/select_having.sql.out b/sql/core/src/test/resources/sql-tests/results/udf/pgSQL/udf-select_having.sql.out
index 02536eb..f731d11 100644
--- a/sql/core/src/test/resources/sql-tests/results/pgSQL/select_having.sql.out
+++ b/sql/core/src/test/resources/sql-tests/results/udf/pgSQL/udf-select_having.sql.out
-91,54 +91,54 struct<>
-- !query 11
-SELECT b, c FROM test_having
- GROUP BY b, c HAVING count(*) = 1 ORDER BY b, c
+SELECT udf(b), udf(c) FROM test_having
+ GROUP BY b, c HAVING udf(count(*)) = 1 ORDER BY udf(b), udf(c)
-- !query 11 schema
-struct<b:int,c:string>
+struct<CAST(udf(cast(b as string)) AS INT):int,CAST(udf(cast(c as string)) AS STRING):string>
-- !query 11 output
1 XXXX
3 bbbb
-- !query 12
-SELECT b, c FROM test_having
- GROUP BY b, c HAVING b = 3 ORDER BY b, c
+SELECT udf(b), udf(c) FROM test_having
+ GROUP BY b, c HAVING udf(b) = 3 ORDER BY udf(b), udf(c)
-- !query 12 schema
-struct<b:int,c:string>
+struct<CAST(udf(cast(b as string)) AS INT):int,CAST(udf(cast(c as string)) AS STRING):string>
-- !query 12 output
3 BBBB
3 bbbb
-- !query 13
-SELECT c, max(a) FROM test_having
- GROUP BY c HAVING count(*) > 2 OR min(a) = max(a)
+SELECT udf(c), max(udf(a)) FROM test_having
+ GROUP BY c HAVING udf(count(*)) > 2 OR udf(min(a)) = udf(max(a))
ORDER BY c
-- !query 13 schema
-struct<c:string,max(a):int>
+struct<CAST(udf(cast(c as string)) AS STRING):string,max(CAST(udf(cast(a as string)) AS INT)):int>
-- !query 13 output
XXXX 0
bbbb 5
-- !query 14
-SELECT min(a), max(a) FROM test_having HAVING min(a) = max(a)
+SELECT udf(udf(min(udf(a)))), udf(udf(max(udf(a)))) FROM test_having HAVING udf(udf(min(udf(a)))) = udf(udf(max(udf(a))))
-- !query 14 schema
-struct<min(a):int,max(a):int>
+struct<CAST(udf(cast(cast(udf(cast(min(cast(udf(cast(a as string)) as int)) as string)) as int) as string)) AS INT):int,CAST(udf(cast(cast(udf(cast(max(cast(udf(cast(a as string)) as int)) as string)) as int) as string)) AS INT):int>
-- !query 14 output
-- !query 15
-SELECT min(a), max(a) FROM test_having HAVING min(a) < max(a)
+SELECT udf(min(udf(a))), udf(udf(max(a))) FROM test_having HAVING udf(min(a)) < udf(max(udf(a)))
-- !query 15 schema
-struct<min(a):int,max(a):int>
+struct<CAST(udf(cast(min(cast(udf(cast(a as string)) as int)) as string)) AS INT):int,CAST(udf(cast(cast(udf(cast(max(a) as string)) as int) as string)) AS INT):int>
-- !query 15 output
0 9
-- !query 16
-SELECT a FROM test_having HAVING min(a) < max(a)
+SELECT udf(a) FROM test_having HAVING udf(min(a)) < udf(max(a))
-- !query 16 schema
struct<>
-- !query 16 output
-147,16 +147,16 grouping expressions sequence is empty, and 'default.test_having.`a`' is not an
-- !query 17
-SELECT 1 AS one FROM test_having HAVING a > 1
+SELECT 1 AS one FROM test_having HAVING udf(a) > 1
-- !query 17 schema
struct<>
-- !query 17 output
org.apache.spark.sql.AnalysisException
-cannot resolve '`a`' given input columns: [one]; line 1 pos 40
+cannot resolve '`a`' given input columns: [one]; line 1 pos 44
-- !query 18
-SELECT 1 AS one FROM test_having HAVING 1 > 2
+SELECT 1 AS one FROM test_having HAVING udf(udf(1) > udf(2))
-- !query 18 schema
struct<one:int>
-- !query 18 output
-164,7 +164,7 struct<one:int>
-- !query 19
-SELECT 1 AS one FROM test_having HAVING 1 < 2
+SELECT 1 AS one FROM test_having HAVING udf(udf(1) < udf(2))
-- !query 19 schema
struct<one:int>
-- !query 19 output
-172,7 +172,7 struct<one:int>
-- !query 20
-SELECT 1 AS one FROM test_having WHERE 1/a = 1 HAVING 1 < 2
+SELECT 1 AS one FROM test_having WHERE 1/udf(a) = 1 HAVING 1 < 2
-- !query 20 schema
struct<one:int>
-- !query 20 output
```
</p>
</details>
## How was this patch tested?
by:
```bash
sudo SPARK_GENERATE_GOLDEN_FILES=1 build/sbt "sql/test-only *SQLQueryTestSuite -- -z udf/pgSQL/udf-select_having.sql"
```
Closes#25161 from shivusondur/jira28390.
Authored-by: shivusondur <shivusondur@gmail.com>
Signed-off-by: HyukjinKwon <gurwls223@apache.org>
## What changes were proposed in this pull request?
https://github.com/apache/spark/pull/7355 add support casting between IntervalType and StringType for scala interface:
```scala
import org.apache.spark.sql.types._
import org.apache.spark.sql.catalyst.expressions._
Cast(Literal("interval 3 month 1 hours"), CalendarIntervalType).eval()
res0: Any = interval 3 months 1 hours
```
But SQL interface does not support it:
```sql
scala> spark.sql("SELECT CAST('interval 3 month 1 hour' AS interval)").show
org.apache.spark.sql.catalyst.parser.ParseException:
DataType interval is not supported.(line 1, pos 41)
== SQL ==
SELECT CAST('interval 3 month 1 hour' AS interval)
-----------------------------------------^^^
at org.apache.spark.sql.catalyst.parser.AstBuilder.$anonfun$visitPrimitiveDataType$1(AstBuilder.scala:1931)
at org.apache.spark.sql.catalyst.parser.ParserUtils$.withOrigin(ParserUtils.scala:108)
at org.apache.spark.sql.catalyst.parser.AstBuilder.visitPrimitiveDataType(AstBuilder.scala:1909)
at org.apache.spark.sql.catalyst.parser.AstBuilder.visitPrimitiveDataType(AstBuilder.scala:52)
...
```
This PR add supports accepting the `interval` keyword in the schema string. So that SQL interface can support this feature.
## How was this patch tested?
unit tests
Closes#25189 from wangyum/SPARK-28435.
Authored-by: Yuming Wang <yumwang@ebay.com>
Signed-off-by: Dongjoon Hyun <dhyun@apple.com>
## What changes were proposed in this pull request?
This PR proposes to use `InheritableThreadLocal` instead of `ThreadLocal` for current epoch in `EpochTracker`. Python UDF needs threads to write out to and read it from Python processes and when there are new threads, previously set epoch is lost.
After this PR, Python UDFs can be used at Structured Streaming with the continuous mode.
## How was this patch tested?
The test cases were written on the top of https://github.com/apache/spark/pull/24945.
Unit tests were added.
Manual tests.
Closes#24946 from HyukjinKwon/SPARK-27234.
Authored-by: HyukjinKwon <gurwls223@apache.org>
Signed-off-by: HyukjinKwon <gurwls223@apache.org>
## What changes were proposed in this pull request?
This PR adds some tests converted from 'pgSQL/select_implicit.sql' to test UDFs
<details><summary>Diff comparing to 'pgSQL/select_implicit.sql'</summary>
<p>
```diff
... diff --git a/sql/core/src/test/resources/sql-tests/results/pgSQL/select_implicit.sql.out b/sql/core/src/test/resources/sql-tests/results/udf/pgSQL/udf-select_implicit.sql.out
index 0675820..e6a5995 100755
--- a/sql/core/src/test/resources/sql-tests/results/pgSQL/select_implicit.sql.out
+++ b/sql/core/src/test/resources/sql-tests/results/udf/pgSQL/udf-select_implicit.sql.out
-91,9 +91,11 struct<>
-- !query 11
-SELECT c, count(*) FROM test_missing_target GROUP BY test_missing_target.c ORDER BY c
+SELECT udf(c), udf(count(*)) FROM test_missing_target GROUP BY
+test_missing_target.c
+ORDER BY udf(c)
-- !query 11 schema
-struct<c:string,count(1):bigint>
+struct<CAST(udf(cast(c as string)) AS STRING):string,CAST(udf(cast(count(1) as string)) AS BIGINT):bigint>
-- !query 11 output
ABAB 2
BBBB 2
-104,9 +106,10 cccc 2
-- !query 12
-SELECT count(*) FROM test_missing_target GROUP BY test_missing_target.c ORDER BY c
+SELECT udf(count(*)) FROM test_missing_target GROUP BY test_missing_target.c
+ORDER BY udf(c)
-- !query 12 schema
-struct<count(1):bigint>
+struct<CAST(udf(cast(count(1) as string)) AS BIGINT):bigint>
-- !query 12 output
2
2
-117,18 +120,18 struct<count(1):bigint>
-- !query 13
-SELECT count(*) FROM test_missing_target GROUP BY a ORDER BY b
+SELECT udf(count(*)) FROM test_missing_target GROUP BY a ORDER BY udf(b)
-- !query 13 schema
struct<>
-- !query 13 output
org.apache.spark.sql.AnalysisException
-cannot resolve '`b`' given input columns: [count(1)]; line 1 pos 61
+cannot resolve '`b`' given input columns: [CAST(udf(cast(count(1) as string)) AS BIGINT)]; line 1 pos 70
-- !query 14
-SELECT count(*) FROM test_missing_target GROUP BY b ORDER BY b
+SELECT udf(count(*)) FROM test_missing_target GROUP BY b ORDER BY udf(b)
-- !query 14 schema
-struct<count(1):bigint>
+struct<CAST(udf(cast(count(1) as string)) AS BIGINT):bigint>
-- !query 14 output
1
2
-137,10 +140,10 struct<count(1):bigint>
-- !query 15
-SELECT test_missing_target.b, count(*)
- FROM test_missing_target GROUP BY b ORDER BY b
+SELECT udf(test_missing_target.b), udf(count(*))
+ FROM test_missing_target GROUP BY b ORDER BY udf(b)
-- !query 15 schema
-struct<b:int,count(1):bigint>
+struct<CAST(udf(cast(b as string)) AS INT):int,CAST(udf(cast(count(1) as string)) AS BIGINT):bigint>
-- !query 15 output
1 1
2 2
-149,9 +152,9 struct<b:int,count(1):bigint>
-- !query 16
-SELECT c FROM test_missing_target ORDER BY a
+SELECT udf(c) FROM test_missing_target ORDER BY udf(a)
-- !query 16 schema
-struct<c:string>
+struct<CAST(udf(cast(c as string)) AS STRING):string>
-- !query 16 output
XXXX
ABAB
-166,9 +169,9 CCCC
-- !query 17
-SELECT count(*) FROM test_missing_target GROUP BY b ORDER BY b desc
+SELECT udf(count(*)) FROM test_missing_target GROUP BY b ORDER BY udf(b) desc
-- !query 17 schema
-struct<count(1):bigint>
+struct<CAST(udf(cast(count(1) as string)) AS BIGINT):bigint>
-- !query 17 output
4
3
-177,17 +180,17 struct<count(1):bigint>
-- !query 18
-SELECT count(*) FROM test_missing_target ORDER BY 1 desc
+SELECT udf(count(*)) FROM test_missing_target ORDER BY udf(1) desc
-- !query 18 schema
-struct<count(1):bigint>
+struct<CAST(udf(cast(count(1) as string)) AS BIGINT):bigint>
-- !query 18 output
10
-- !query 19
-SELECT c, count(*) FROM test_missing_target GROUP BY 1 ORDER BY 1
+SELECT udf(c), udf(count(*)) FROM test_missing_target GROUP BY 1 ORDER BY 1
-- !query 19 schema
-struct<c:string,count(1):bigint>
+struct<CAST(udf(cast(c as string)) AS STRING):string,CAST(udf(cast(count(1) as string)) AS BIGINT):bigint>
-- !query 19 output
ABAB 2
BBBB 2
-198,18 +201,18 cccc 2
-- !query 20
-SELECT c, count(*) FROM test_missing_target GROUP BY 3
+SELECT udf(c), udf(count(*)) FROM test_missing_target GROUP BY 3
-- !query 20 schema
struct<>
-- !query 20 output
org.apache.spark.sql.AnalysisException
-GROUP BY position 3 is not in select list (valid range is [1, 2]); line 1 pos 53
+GROUP BY position 3 is not in select list (valid range is [1, 2]); line 1 pos 63
-- !query 21
-SELECT count(*) FROM test_missing_target x, test_missing_target y
- WHERE x.a = y.a
- GROUP BY b ORDER BY b
+SELECT udf(count(*)) FROM test_missing_target x, test_missing_target y
+ WHERE udf(x.a) = udf(y.a)
+ GROUP BY b ORDER BY udf(b)
-- !query 21 schema
struct<>
-- !query 21 output
-218,10 +221,10 Reference 'b' is ambiguous, could be: x.b, y.b.; line 3 pos 10
-- !query 22
-SELECT a, a FROM test_missing_target
- ORDER BY a
+SELECT udf(a), udf(a) FROM test_missing_target
+ ORDER BY udf(a)
-- !query 22 schema
-struct<a:int,a:int>
+struct<CAST(udf(cast(a as string)) AS INT):int,CAST(udf(cast(a as string)) AS INT):int>
-- !query 22 output
0 0
1 1
-236,10 +239,10 struct<a:int,a:int>
-- !query 23
-SELECT a/2, a/2 FROM test_missing_target
- ORDER BY a/2
+SELECT udf(udf(a)/2), udf(udf(a)/2) FROM test_missing_target
+ ORDER BY udf(udf(a)/2)
-- !query 23 schema
-struct<(a div 2):int,(a div 2):int>
+struct<CAST(udf(cast((cast(udf(cast(a as string)) as int) div 2) as string)) AS INT):int,CAST(udf(cast((cast(udf(cast(a as string)) as int) div 2) as string)) AS INT):int>
-- !query 23 output
0 0
0 0
-254,10 +257,10 struct<(a div 2):int,(a div 2):int>
-- !query 24
-SELECT a/2, a/2 FROM test_missing_target
- GROUP BY a/2 ORDER BY a/2
+SELECT udf(a/2), udf(a/2) FROM test_missing_target
+ GROUP BY a/2 ORDER BY udf(a/2)
-- !query 24 schema
-struct<(a div 2):int,(a div 2):int>
+struct<CAST(udf(cast((a div 2) as string)) AS INT):int,CAST(udf(cast((a div 2) as string)) AS INT):int>
-- !query 24 output
0 0
1 1
-267,11 +270,11 struct<(a div 2):int,(a div 2):int>
-- !query 25
-SELECT x.b, count(*) FROM test_missing_target x, test_missing_target y
- WHERE x.a = y.a
- GROUP BY x.b ORDER BY x.b
+SELECT udf(x.b), udf(count(*)) FROM test_missing_target x, test_missing_target y
+ WHERE udf(x.a) = udf(y.a)
+ GROUP BY x.b ORDER BY udf(x.b)
-- !query 25 schema
-struct<b:int,count(1):bigint>
+struct<CAST(udf(cast(b as string)) AS INT):int,CAST(udf(cast(count(1) as string)) AS BIGINT):bigint>
-- !query 25 output
1 1
2 2
-280,11 +283,11 struct<b:int,count(1):bigint>
-- !query 26
-SELECT count(*) FROM test_missing_target x, test_missing_target y
- WHERE x.a = y.a
- GROUP BY x.b ORDER BY x.b
+SELECT udf(count(*)) FROM test_missing_target x, test_missing_target y
+ WHERE udf(x.a) = udf(y.a)
+ GROUP BY x.b ORDER BY udf(x.b)
-- !query 26 schema
-struct<count(1):bigint>
+struct<CAST(udf(cast(count(1) as string)) AS BIGINT):bigint>
-- !query 26 output
1
2
-293,22 +296,22 struct<count(1):bigint>
-- !query 27
-SELECT a%2, count(b) FROM test_missing_target
+SELECT a%2, udf(count(udf(b))) FROM test_missing_target
GROUP BY test_missing_target.a%2
-ORDER BY test_missing_target.a%2
+ORDER BY udf(test_missing_target.a%2)
-- !query 27 schema
-struct<(a % 2):int,count(b):bigint>
+struct<(a % 2):int,CAST(udf(cast(count(cast(udf(cast(b as string)) as int)) as string)) AS BIGINT):bigint>
-- !query 27 output
0 5
1 5
-- !query 28
-SELECT count(c) FROM test_missing_target
+SELECT udf(count(c)) FROM test_missing_target
GROUP BY lower(test_missing_target.c)
-ORDER BY lower(test_missing_target.c)
+ORDER BY udf(lower(test_missing_target.c))
-- !query 28 schema
-struct<count(c):bigint>
+struct<CAST(udf(cast(count(c) as string)) AS BIGINT):bigint>
-- !query 28 output
2
3
-317,18 +320,18 struct<count(c):bigint>
-- !query 29
-SELECT count(a) FROM test_missing_target GROUP BY a ORDER BY b
+SELECT udf(count(udf(a))) FROM test_missing_target GROUP BY a ORDER BY udf(b)
-- !query 29 schema
struct<>
-- !query 29 output
org.apache.spark.sql.AnalysisException
-cannot resolve '`b`' given input columns: [count(a)]; line 1 pos 61
+cannot resolve '`b`' given input columns: [CAST(udf(cast(count(cast(udf(cast(a as string)) as int)) as string)) AS BIGINT)]; line 1 pos 75
-- !query 30
-SELECT count(b) FROM test_missing_target GROUP BY b/2 ORDER BY b/2
+SELECT udf(count(b)) FROM test_missing_target GROUP BY b/2 ORDER BY udf(b/2)
-- !query 30 schema
-struct<count(b):bigint>
+struct<CAST(udf(cast(count(b) as string)) AS BIGINT):bigint>
-- !query 30 output
1
5
-336,10 +339,10 struct<count(b):bigint>
-- !query 31
-SELECT lower(test_missing_target.c), count(c)
- FROM test_missing_target GROUP BY lower(c) ORDER BY lower(c)
+SELECT udf(lower(test_missing_target.c)), udf(count(udf(c)))
+ FROM test_missing_target GROUP BY lower(c) ORDER BY udf(lower(c))
-- !query 31 schema
-struct<lower(c):string,count(c):bigint>
+struct<CAST(udf(cast(lower(c) as string)) AS STRING):string,CAST(udf(cast(count(cast(udf(cast(c as string)) as string)) as string)) AS BIGINT):bigint>
-- !query 31 output
abab 2
bbbb 3
-348,9 +351,9 xxxx 1
-- !query 32
-SELECT a FROM test_missing_target ORDER BY upper(d)
+SELECT udf(a) FROM test_missing_target ORDER BY udf(upper(udf(d)))
-- !query 32 schema
-struct<a:int>
+struct<CAST(udf(cast(a as string)) AS INT):int>
-- !query 32 output
0
1
-365,19 +368,19 struct<a:int>
-- !query 33
-SELECT count(b) FROM test_missing_target
- GROUP BY (b + 1) / 2 ORDER BY (b + 1) / 2 desc
+SELECT udf(count(b)) FROM test_missing_target
+ GROUP BY (b + 1) / 2 ORDER BY udf((b + 1) / 2) desc
-- !query 33 schema
-struct<count(b):bigint>
+struct<CAST(udf(cast(count(b) as string)) AS BIGINT):bigint>
-- !query 33 output
7
3
-- !query 34
-SELECT count(x.a) FROM test_missing_target x, test_missing_target y
- WHERE x.a = y.a
- GROUP BY b/2 ORDER BY b/2
+SELECT udf(count(udf(x.a))) FROM test_missing_target x, test_missing_target y
+ WHERE udf(x.a) = udf(y.a)
+ GROUP BY b/2 ORDER BY udf(b/2)
-- !query 34 schema
struct<>
-- !query 34 output
-386,11 +389,12 Reference 'b' is ambiguous, could be: x.b, y.b.; line 3 pos 10
-- !query 35
-SELECT x.b/2, count(x.b) FROM test_missing_target x, test_missing_target y
- WHERE x.a = y.a
- GROUP BY x.b/2 ORDER BY x.b/2
+SELECT udf(x.b/2), udf(count(udf(x.b))) FROM test_missing_target x,
+test_missing_target y
+ WHERE udf(x.a) = udf(y.a)
+ GROUP BY x.b/2 ORDER BY udf(x.b/2)
-- !query 35 schema
-struct<(b div 2):int,count(b):bigint>
+struct<CAST(udf(cast((b div 2) as string)) AS INT):int,CAST(udf(cast(count(cast(udf(cast(b as string)) as int)) as string)) AS BIGINT):bigint>
-- !query 35 output
0 1
1 5
-398,14 +402,14 struct<(b div 2):int,count(b):bigint>
-- !query 36
-SELECT count(b) FROM test_missing_target x, test_missing_target y
- WHERE x.a = y.a
+SELECT udf(count(udf(b))) FROM test_missing_target x, test_missing_target y
+ WHERE udf(x.a) = udf(y.a)
GROUP BY x.b/2
-- !query 36 schema
struct<>
-- !query 36 output
org.apache.spark.sql.AnalysisException
-Reference 'b' is ambiguous, could be: x.b, y.b.; line 1 pos 13
+Reference 'b' is ambiguous, could be: x.b, y.b.; line 1 pos 21
-- !query 37
```
</p>
</details>
## How was this patch tested?
Tested as Guided in SPARK-27921
Closes#25233 from Udbhav30/master.
Authored-by: Udbhav30 <u.agrawal30@gmail.com>
Signed-off-by: HyukjinKwon <gurwls223@apache.org>
## What changes were proposed in this pull request?
Change the format of the build command in the README to start with a `./` prefix
./build/mvn -DskipTests clean package
This increases stylistic consistency across the README- all the other commands have a `./` prefix. Having a visible `./` prefix also makes it clear to the user that the shell command requires the current working directory to be at the repository root.
## How was this patch tested?
README.md was reviewed both in raw markdown and in the Github rendered landing page for stylistic consistency.
Closes#25231 from Mister-Meeseeks/master.
Lead-authored-by: Douglas R Colkitt <douglas.colkitt@gmail.com>
Co-authored-by: Mister-Meeseeks <douglas.colkitt@gmail.com>
Signed-off-by: Dongjoon Hyun <dhyun@apple.com>
## What changes were proposed in this pull request?
The bug fixed by https://github.com/apache/spark/pull/24886 is caused by Hive's `loadDynamicPartitions`. It's better to keep the fix surgical and put it right before we call `loadDynamicPartitions`.
This also makes the fix safer, instead of analyzing all the callers of `saveAsHiveFile` and proving that they are safe.
## How was this patch tested?
N/A
Closes#25234 from cloud-fan/minor.
Authored-by: Wenchen Fan <wenchen@databricks.com>
Signed-off-by: Dongjoon Hyun <dhyun@apple.com>
## What changes were proposed in this pull request?
query plan was designed to be immutable, but sometimes we do allow it to carry mutable states, because of the complexity of the SQL system. One example is `TreeNodeTag`. It's a state of `TreeNode` and can be carried over during copy and transform. The adaptive execution framework relies on it to link the logical and physical plans.
This leads to a problem: when we get `QueryExecution#analyzed`, the plan can be changed unexpectedly because it's mutable. I hit a real issue in https://github.com/apache/spark/pull/25107 : I use `TreeNodeTag` to carry dataset id in logical plans. However, the analyzed plan ends up with many duplicated dataset id tags in different nodes. It turns out that, the optimizer transforms the logical plan and add the tag to more nodes.
For example, the logical plan is `SubqueryAlias(Filter(...))`, and I expect only the `SubqueryAlais` has the dataset id tag. However, the optimizer removes `SubqueryAlias` and carries over the dataset id tag to `Filter`. When I go back to the analyzed plan, both `SubqueryAlias` and `Filter` has the dataset id tag, which breaks my assumption.
Since now query plan is mutable, I think it's better to limit the life cycle of a query plan instance. We can clone the query plan between analyzer, optimizer and planner, so that the life cycle is limited in one stage.
## How was this patch tested?
new test
Closes#25111 from cloud-fan/clone.
Authored-by: Wenchen Fan <wenchen@databricks.com>
Signed-off-by: gatorsmile <gatorsmile@gmail.com>
## What changes were proposed in this pull request?
This PR change `CalendarIntervalType`'s readable string representation from `calendarinterval` to `interval`.
## How was this patch tested?
Existing UT
Closes#25225 from wangyum/SPARK-28469.
Authored-by: Yuming Wang <yumwang@ebay.com>
Signed-off-by: Dongjoon Hyun <dhyun@apple.com>
## What changes were proposed in this pull request?
Fix CSV datasource to throw `com.univocity.parsers.common.TextParsingException` with large size message, which will make log output consume large disk space.
This issue is troublesome when sometimes we need parse CSV with large size column.
This PR proposes to set CSV parser/writer settings by `setErrorContentLength(1000)` to limit the error message length.
## How was this patch tested?
Manually.
```
val s = "a" * 40 * 1000000
Seq(s).toDF.write.mode("overwrite").csv("/tmp/bogdan/es4196.csv")
spark.read .option("maxCharsPerColumn", 30000000) .csv("/tmp/bogdan/es4196.csv").count
```
**Before:**
The thrown message will include error content of about 30MB size (The column size exceed the max value 30MB, so the error content include the whole parsed content, so it is 30MB).
**After:**
The thrown message will include error content like "...aaa...aa" (the number of 'a' is 1024), i.e. limit the content size to be 1024.
Closes#25184 from WeichenXu123/limit_csv_exception_size.
Authored-by: WeichenXu <weichen.xu@databricks.com>
Signed-off-by: HyukjinKwon <gurwls223@apache.org>
## What changes were proposed in this pull request?
New function `make_date()` takes 3 columns `year`, `month` and `day`, and makes new column of the `DATE` type. If values in the input columns are `null` or out of valid ranges, the function returns `null`. Valid ranges are:
- `year` - `[1, 9999]`
- `month` - `[1, 12]`
- `day` - `[1, 31]`
Also constructed date must be valid otherwise `make_date` returns `null`.
The function is implemented similarly to `make_date` in PostgreSQL: https://www.postgresql.org/docs/11/functions-datetime.html to maintain feature parity with it.
Here is an example:
```sql
select make_date(2013, 7, 15);
2013-07-15
```
## How was this patch tested?
Added new tests to `DateExpressionsSuite`.
Closes#25210 from MaxGekk/make_date-timestamp.
Authored-by: Maxim Gekk <max.gekk@gmail.com>
Signed-off-by: Dongjoon Hyun <dhyun@apple.com>
## What changes were proposed in this pull request?
This PR adds some tests converted from `group-by.sql` to test UDFs. Please see contribution guide of this umbrella ticket - [SPARK-27921](https://issues.apache.org/jira/browse/SPARK-27921).
<details><summary>Diff comparing to 'group-by.sql'</summary>
<p>
```diff
diff --git a/sql/core/src/test/resources/sql-tests/results/udf/udf-group-by.sql.out b/sql/core/src/test/resources/sql-tests/results/udf/udf-group-by.sql.out
index 3a5df254f2..0118c05b1d 100644
--- a/sql/core/src/test/resources/sql-tests/results/udf/udf-group-by.sql.out
+++ b/sql/core/src/test/resources/sql-tests/results/udf/udf-group-by.sql.out
-13,26 +13,26 struct<>
-- !query 1
-SELECT a, COUNT(b) FROM testData
+SELECT udf(a), udf(COUNT(b)) FROM testData
-- !query 1 schema
struct<>
-- !query 1 output
org.apache.spark.sql.AnalysisException
-grouping expressions sequence is empty, and 'testdata.`a`' is not an aggregate function. Wrap '(count(testdata.`b`) AS `count(b)`)' in windowing function(s) or wrap 'testdata.`a`' in first() (or first_value) if you don't care which value you get.;
+grouping expressions sequence is empty, and 'testdata.`a`' is not an aggregate function. Wrap '(CAST(udf(cast(count(b) as string)) AS BIGINT) AS `CAST(udf(cast(count(b) as string)) AS BIGINT)`)' in windowing function(s) or wrap 'testdata.`a`' in first() (or first_value) if you don't care which value you get.;
-- !query 2
-SELECT COUNT(a), COUNT(b) FROM testData
+SELECT COUNT(udf(a)), udf(COUNT(b)) FROM testData
-- !query 2 schema
-struct<count(a):bigint,count(b):bigint>
+struct<count(CAST(udf(cast(a as string)) AS INT)):bigint,CAST(udf(cast(count(b) as string)) AS BIGINT):bigint>
-- !query 2 output
7 7
-- !query 3
-SELECT a, COUNT(b) FROM testData GROUP BY a
+SELECT udf(a), COUNT(udf(b)) FROM testData GROUP BY a
-- !query 3 schema
-struct<a:int,count(b):bigint>
+struct<CAST(udf(cast(a as string)) AS INT):int,count(CAST(udf(cast(b as string)) AS INT)):bigint>
-- !query 3 output
1 2
2 2
-41,7 +41,7 NULL 1
-- !query 4
-SELECT a, COUNT(b) FROM testData GROUP BY b
+SELECT udf(a), udf(COUNT(udf(b))) FROM testData GROUP BY b
-- !query 4 schema
struct<>
-- !query 4 output
-50,9 +50,9 expression 'testdata.`a`' is neither present in the group by, nor is it an aggre
-- !query 5
-SELECT COUNT(a), COUNT(b) FROM testData GROUP BY a
+SELECT COUNT(udf(a)), COUNT(udf(b)) FROM testData GROUP BY udf(a)
-- !query 5 schema
-struct<count(a):bigint,count(b):bigint>
+struct<count(CAST(udf(cast(a as string)) AS INT)):bigint,count(CAST(udf(cast(b as string)) AS INT)):bigint>
-- !query 5 output
0 1
2 2
-61,15 +61,15 struct<count(a):bigint,count(b):bigint>
-- !query 6
-SELECT 'foo', COUNT(a) FROM testData GROUP BY 1
+SELECT 'foo', COUNT(udf(a)) FROM testData GROUP BY 1
-- !query 6 schema
-struct<foo:string,count(a):bigint>
+struct<foo:string,count(CAST(udf(cast(a as string)) AS INT)):bigint>
-- !query 6 output
foo 7
-- !query 7
-SELECT 'foo' FROM testData WHERE a = 0 GROUP BY 1
+SELECT 'foo' FROM testData WHERE a = 0 GROUP BY udf(1)
-- !query 7 schema
struct<foo:string>
-- !query 7 output
-77,25 +77,25 struct<foo:string>
-- !query 8
-SELECT 'foo', APPROX_COUNT_DISTINCT(a) FROM testData WHERE a = 0 GROUP BY 1
+SELECT 'foo', udf(APPROX_COUNT_DISTINCT(udf(a))) FROM testData WHERE a = 0 GROUP BY 1
-- !query 8 schema
-struct<foo:string,approx_count_distinct(a):bigint>
+struct<foo:string,CAST(udf(cast(approx_count_distinct(cast(udf(cast(a as string)) as int), 0.05, 0, 0) as string)) AS BIGINT):bigint>
-- !query 8 output
-- !query 9
-SELECT 'foo', MAX(STRUCT(a)) FROM testData WHERE a = 0 GROUP BY 1
+SELECT 'foo', MAX(STRUCT(udf(a))) FROM testData WHERE a = 0 GROUP BY 1
-- !query 9 schema
-struct<foo:string,max(named_struct(a, a)):struct<a:int>>
+struct<foo:string,max(named_struct(col1, CAST(udf(cast(a as string)) AS INT))):struct<col1:int>>
-- !query 9 output
-- !query 10
-SELECT a + b, COUNT(b) FROM testData GROUP BY a + b
+SELECT udf(a + b), udf(COUNT(b)) FROM testData GROUP BY a + b
-- !query 10 schema
-struct<(a + b):int,count(b):bigint>
+struct<CAST(udf(cast((a + b) as string)) AS INT):int,CAST(udf(cast(count(b) as string)) AS BIGINT):bigint>
-- !query 10 output
2 1
3 2
-105,7 +105,7 NULL 1
-- !query 11
-SELECT a + 2, COUNT(b) FROM testData GROUP BY a + 1
+SELECT udf(a + 2), udf(COUNT(b)) FROM testData GROUP BY a + 1
-- !query 11 schema
struct<>
-- !query 11 output
-114,37 +114,35 expression 'testdata.`a`' is neither present in the group by, nor is it an aggre
-- !query 12
-SELECT a + 1 + 1, COUNT(b) FROM testData GROUP BY a + 1
+SELECT udf(a + 1 + 1), udf(COUNT(b)) FROM testData GROUP BY udf(a + 1)
-- !query 12 schema
-struct<((a + 1) + 1):int,count(b):bigint>
+struct<>
-- !query 12 output
-3 2
-4 2
-5 2
-NULL 1
+org.apache.spark.sql.AnalysisException
+expression 'testdata.`a`' is neither present in the group by, nor is it an aggregate function. Add to group by or wrap in first() (or first_value) if you don't care which value you get.;
-- !query 13
-SELECT SKEWNESS(a), KURTOSIS(a), MIN(a), MAX(a), AVG(a), VARIANCE(a), STDDEV(a), SUM(a), COUNT(a)
+SELECT SKEWNESS(udf(a)), udf(KURTOSIS(a)), udf(MIN(a)), MAX(udf(a)), udf(AVG(udf(a))), udf(VARIANCE(a)), STDDEV(udf(a)), udf(SUM(a)), udf(COUNT(a))
FROM testData
-- !query 13 schema
-struct<skewness(CAST(a AS DOUBLE)):double,kurtosis(CAST(a AS DOUBLE)):double,min(a):int,max(a):int,avg(a):double,var_samp(CAST(a AS DOUBLE)):double,stddev_samp(CAST(a AS DOUBLE)):double,sum(a):bigint,count(a):bigint>
+struct<skewness(CAST(CAST(udf(cast(a as string)) AS INT) AS DOUBLE)):double,CAST(udf(cast(kurtosis(cast(a as double)) as string)) AS DOUBLE):double,CAST(udf(cast(min(a) as string)) AS INT):int,max(CAST(udf(cast(a as string)) AS INT)):int,CAST(udf(cast(avg(cast(cast(udf(cast(a as string)) as int) as bigint)) as string)) AS DOUBLE):double,CAST(udf(cast(var_samp(cast(a as double)) as string)) AS DOUBLE):double,stddev_samp(CAST(CAST(udf(cast(a as string)) AS INT) AS DOUBLE)):double,CAST(udf(cast(sum(cast(a as bigint)) as string)) AS BIGINT):bigint,CAST(udf(cast(count(a) as string)) AS BIGINT):bigint>
-- !query 13 output
-0.2723801058145729 -1.5069204152249134 1 3 2.142857142857143 0.8095238095238094 0.8997354108424372 15 7
-- !query 14
-SELECT COUNT(DISTINCT b), COUNT(DISTINCT b, c) FROM (SELECT 1 AS a, 2 AS b, 3 AS c) GROUP BY a
+SELECT COUNT(DISTINCT udf(b)), udf(COUNT(DISTINCT b, c)) FROM (SELECT 1 AS a, 2 AS b, 3 AS c) GROUP BY a
-- !query 14 schema
-struct<count(DISTINCT b):bigint,count(DISTINCT b, c):bigint>
+struct<count(DISTINCT CAST(udf(cast(b as string)) AS INT)):bigint,CAST(udf(cast(count(distinct b, c) as string)) AS BIGINT):bigint>
-- !query 14 output
1 1
-- !query 15
-SELECT a AS k, COUNT(b) FROM testData GROUP BY k
+SELECT a AS k, COUNT(udf(b)) FROM testData GROUP BY k
-- !query 15 schema
-struct<k:int,count(b):bigint>
+struct<k:int,count(CAST(udf(cast(b as string)) AS INT)):bigint>
-- !query 15 output
1 2
2 2
-153,21 +151,21 NULL 1
-- !query 16
-SELECT a AS k, COUNT(b) FROM testData GROUP BY k HAVING k > 1
+SELECT a AS k, udf(COUNT(b)) FROM testData GROUP BY k HAVING k > 1
-- !query 16 schema
-struct<k:int,count(b):bigint>
+struct<k:int,CAST(udf(cast(count(b) as string)) AS BIGINT):bigint>
-- !query 16 output
2 2
3 2
-- !query 17
-SELECT COUNT(b) AS k FROM testData GROUP BY k
+SELECT udf(COUNT(b)) AS k FROM testData GROUP BY k
-- !query 17 schema
struct<>
-- !query 17 output
org.apache.spark.sql.AnalysisException
-aggregate functions are not allowed in GROUP BY, but found count(testdata.`b`);
+aggregate functions are not allowed in GROUP BY, but found CAST(udf(cast(count(b) as string)) AS BIGINT);
-- !query 18
-180,7 +178,7 struct<>
-- !query 19
-SELECT k AS a, COUNT(v) FROM testDataHasSameNameWithAlias GROUP BY a
+SELECT k AS a, udf(COUNT(udf(v))) FROM testDataHasSameNameWithAlias GROUP BY a
-- !query 19 schema
struct<>
-- !query 19 output
-197,32 +195,32 spark.sql.groupByAliases false
-- !query 21
-SELECT a AS k, COUNT(b) FROM testData GROUP BY k
+SELECT a AS k, udf(COUNT(udf(b))) FROM testData GROUP BY k
-- !query 21 schema
struct<>
-- !query 21 output
org.apache.spark.sql.AnalysisException
-cannot resolve '`k`' given input columns: [testdata.a, testdata.b]; line 1 pos 47
+cannot resolve '`k`' given input columns: [testdata.a, testdata.b]; line 1 pos 57
-- !query 22
-SELECT a, COUNT(1) FROM testData WHERE false GROUP BY a
+SELECT a, COUNT(udf(1)) FROM testData WHERE false GROUP BY a
-- !query 22 schema
-struct<a:int,count(1):bigint>
+struct<a:int,count(CAST(udf(cast(1 as string)) AS INT)):bigint>
-- !query 22 output
-- !query 23
-SELECT COUNT(1) FROM testData WHERE false
+SELECT udf(COUNT(1)) FROM testData WHERE false
-- !query 23 schema
-struct<count(1):bigint>
+struct<CAST(udf(cast(count(1) as string)) AS BIGINT):bigint>
-- !query 23 output
0
-- !query 24
-SELECT 1 FROM (SELECT COUNT(1) FROM testData WHERE false) t
+SELECT 1 FROM (SELECT udf(COUNT(1)) FROM testData WHERE false) t
-- !query 24 schema
struct<1:int>
-- !query 24 output
-232,7 +230,7 struct<1:int>
-- !query 25
SELECT 1 from (
SELECT 1 AS z,
- MIN(a.x)
+ udf(MIN(a.x))
FROM (select 1 as x) a
WHERE false
) b
-244,32 +242,32 struct<1:int>
-- !query 26
-SELECT corr(DISTINCT x, y), corr(DISTINCT y, x), count(*)
+SELECT corr(DISTINCT x, y), udf(corr(DISTINCT y, x)), count(*)
FROM (VALUES (1, 1), (2, 2), (2, 2)) t(x, y)
-- !query 26 schema
-struct<corr(DISTINCT CAST(x AS DOUBLE), CAST(y AS DOUBLE)):double,corr(DISTINCT CAST(y AS DOUBLE), CAST(x AS DOUBLE)):double,count(1):bigint>
+struct<corr(DISTINCT CAST(x AS DOUBLE), CAST(y AS DOUBLE)):double,CAST(udf(cast(corr(distinct cast(y as double), cast(x as double)) as string)) AS DOUBLE):double,count(1):bigint>
-- !query 26 output
1.0 1.0 3
-- !query 27
-SELECT 1 FROM range(10) HAVING true
+SELECT udf(1) FROM range(10) HAVING true
-- !query 27 schema
-struct<1:int>
+struct<CAST(udf(cast(1 as string)) AS INT):int>
-- !query 27 output
1
-- !query 28
-SELECT 1 FROM range(10) HAVING MAX(id) > 0
+SELECT udf(udf(1)) FROM range(10) HAVING MAX(id) > 0
-- !query 28 schema
-struct<1:int>
+struct<CAST(udf(cast(cast(udf(cast(1 as string)) as int) as string)) AS INT):int>
-- !query 28 output
1
-- !query 29
-SELECT id FROM range(10) HAVING id > 0
+SELECT udf(id) FROM range(10) HAVING id > 0
-- !query 29 schema
struct<>
-- !query 29 output
-291,33 +289,33 struct<>
-- !query 31
-SELECT every(v), some(v), any(v) FROM test_agg WHERE 1 = 0
+SELECT udf(every(v)), udf(some(v)), any(v) FROM test_agg WHERE 1 = 0
-- !query 31 schema
-struct<every(v):boolean,some(v):boolean,any(v):boolean>
+struct<CAST(udf(cast(every(v) as string)) AS BOOLEAN):boolean,CAST(udf(cast(some(v) as string)) AS BOOLEAN):boolean,any(v):boolean>
-- !query 31 output
NULL NULL NULL
-- !query 32
-SELECT every(v), some(v), any(v) FROM test_agg WHERE k = 4
+SELECT udf(every(udf(v))), some(v), any(v) FROM test_agg WHERE k = 4
-- !query 32 schema
-struct<every(v):boolean,some(v):boolean,any(v):boolean>
+struct<CAST(udf(cast(every(cast(udf(cast(v as string)) as boolean)) as string)) AS BOOLEAN):boolean,some(v):boolean,any(v):boolean>
-- !query 32 output
NULL NULL NULL
-- !query 33
-SELECT every(v), some(v), any(v) FROM test_agg WHERE k = 5
+SELECT every(v), udf(some(v)), any(v) FROM test_agg WHERE k = 5
-- !query 33 schema
-struct<every(v):boolean,some(v):boolean,any(v):boolean>
+struct<every(v):boolean,CAST(udf(cast(some(v) as string)) AS BOOLEAN):boolean,any(v):boolean>
-- !query 33 output
false true true
-- !query 34
-SELECT k, every(v), some(v), any(v) FROM test_agg GROUP BY k
+SELECT k, every(v), udf(some(v)), any(v) FROM test_agg GROUP BY k
-- !query 34 schema
-struct<k:int,every(v):boolean,some(v):boolean,any(v):boolean>
+struct<k:int,every(v):boolean,CAST(udf(cast(some(v) as string)) AS BOOLEAN):boolean,any(v):boolean>
-- !query 34 output
1 false true true
2 true true true
-327,9 +325,9 struct<k:int,every(v):boolean,some(v):boolean,any(v):boolean>
-- !query 35
-SELECT k, every(v) FROM test_agg GROUP BY k HAVING every(v) = false
+SELECT udf(k), every(v) FROM test_agg GROUP BY k HAVING every(v) = false
-- !query 35 schema
-struct<k:int,every(v):boolean>
+struct<CAST(udf(cast(k as string)) AS INT):int,every(v):boolean>
-- !query 35 output
1 false
3 false
-337,16 +335,16 struct<k:int,every(v):boolean>
-- !query 36
-SELECT k, every(v) FROM test_agg GROUP BY k HAVING every(v) IS NULL
+SELECT k, udf(every(v)) FROM test_agg GROUP BY k HAVING every(v) IS NULL
-- !query 36 schema
-struct<k:int,every(v):boolean>
+struct<k:int,CAST(udf(cast(every(v) as string)) AS BOOLEAN):boolean>
-- !query 36 output
4 NULL
-- !query 37
SELECT k,
- Every(v) AS every
+ udf(Every(v)) AS every
FROM test_agg
WHERE k = 2
AND v IN (SELECT Any(v)
-360,7 +358,7 struct<k:int,every:boolean>
-- !query 38
-SELECT k,
+SELECT udf(udf(k)),
Every(v) AS every
FROM test_agg
WHERE k = 2
-369,45 +367,45 WHERE k = 2
WHERE k = 1)
GROUP BY k
-- !query 38 schema
-struct<k:int,every:boolean>
+struct<CAST(udf(cast(cast(udf(cast(k as string)) as int) as string)) AS INT):int,every:boolean>
-- !query 38 output
-- !query 39
-SELECT every(1)
+SELECT every(udf(1))
-- !query 39 schema
struct<>
-- !query 39 output
org.apache.spark.sql.AnalysisException
-cannot resolve 'every(1)' due to data type mismatch: Input to function 'every' should have been boolean, but it's [int].; line 1 pos 7
+cannot resolve 'every(CAST(udf(cast(1 as string)) AS INT))' due to data type mismatch: Input to function 'every' should have been boolean, but it's [int].; line 1 pos 7
-- !query 40
-SELECT some(1S)
+SELECT some(udf(1S))
-- !query 40 schema
struct<>
-- !query 40 output
org.apache.spark.sql.AnalysisException
-cannot resolve 'some(1S)' due to data type mismatch: Input to function 'some' should have been boolean, but it's [smallint].; line 1 pos 7
+cannot resolve 'some(CAST(udf(cast(1 as string)) AS SMALLINT))' due to data type mismatch: Input to function 'some' should have been boolean, but it's [smallint].; line 1 pos 7
-- !query 41
-SELECT any(1L)
+SELECT any(udf(1L))
-- !query 41 schema
struct<>
-- !query 41 output
org.apache.spark.sql.AnalysisException
-cannot resolve 'any(1L)' due to data type mismatch: Input to function 'any' should have been boolean, but it's [bigint].; line 1 pos 7
+cannot resolve 'any(CAST(udf(cast(1 as string)) AS BIGINT))' due to data type mismatch: Input to function 'any' should have been boolean, but it's [bigint].; line 1 pos 7
-- !query 42
-SELECT every("true")
+SELECT udf(every("true"))
-- !query 42 schema
struct<>
-- !query 42 output
org.apache.spark.sql.AnalysisException
-cannot resolve 'every('true')' due to data type mismatch: Input to function 'every' should have been boolean, but it's [string].; line 1 pos 7
+cannot resolve 'every('true')' due to data type mismatch: Input to function 'every' should have been boolean, but it's [string].; line 1 pos 11
-- !query 43
-428,9 +426,9 struct<k:int,v:boolean,every(v) OVER (PARTITION BY k ORDER BY v ASC NULLS FIRST
-- !query 44
-SELECT k, v, some(v) OVER (PARTITION BY k ORDER BY v) FROM test_agg
+SELECT k, udf(udf(v)), some(v) OVER (PARTITION BY k ORDER BY v) FROM test_agg
-- !query 44 schema
-struct<k:int,v:boolean,some(v) OVER (PARTITION BY k ORDER BY v ASC NULLS FIRST RANGE BETWEEN UNBOUNDED PRECEDING AND CURRENT ROW):boolean>
+struct<k:int,CAST(udf(cast(cast(udf(cast(v as string)) as boolean) as string)) AS BOOLEAN):boolean,some(v) OVER (PARTITION BY k ORDER BY v ASC NULLS FIRST RANGE BETWEEN UNBOUNDED PRECEDING AND CURRENT ROW):boolean>
-- !query 44 output
1 false false
1 true true
-445,9 +443,9 struct<k:int,v:boolean,some(v) OVER (PARTITION BY k ORDER BY v ASC NULLS FIRST R
-- !query 45
-SELECT k, v, any(v) OVER (PARTITION BY k ORDER BY v) FROM test_agg
+SELECT udf(udf(k)), v, any(v) OVER (PARTITION BY k ORDER BY v) FROM test_agg
-- !query 45 schema
-struct<k:int,v:boolean,any(v) OVER (PARTITION BY k ORDER BY v ASC NULLS FIRST RANGE BETWEEN UNBOUNDED PRECEDING AND CURRENT ROW):boolean>
+struct<CAST(udf(cast(cast(udf(cast(k as string)) as int) as string)) AS INT):int,v:boolean,any(v) OVER (PARTITION BY k ORDER BY v ASC NULLS FIRST RANGE BETWEEN UNBOUNDED PRECEDING AND CURRENT ROW):boolean>
-- !query 45 output
1 false false
1 true true
-462,17 +460,17 struct<k:int,v:boolean,any(v) OVER (PARTITION BY k ORDER BY v ASC NULLS FIRST RA
-- !query 46
-SELECT count(*) FROM test_agg HAVING count(*) > 1L
+SELECT udf(count(*)) FROM test_agg HAVING count(*) > 1L
-- !query 46 schema
-struct<count(1):bigint>
+struct<CAST(udf(cast(count(1) as string)) AS BIGINT):bigint>
-- !query 46 output
10
-- !query 47
-SELECT k, max(v) FROM test_agg GROUP BY k HAVING max(v) = true
+SELECT k, udf(max(v)) FROM test_agg GROUP BY k HAVING max(v) = true
-- !query 47 schema
-struct<k:int,max(v):boolean>
+struct<k:int,CAST(udf(cast(max(v) as string)) AS BOOLEAN):boolean>
-- !query 47 output
1 true
2 true
-480,7 +478,7 struct<k:int,max(v):boolean>
-- !query 48
-SELECT * FROM (SELECT COUNT(*) AS cnt FROM test_agg) WHERE cnt > 1L
+SELECT * FROM (SELECT udf(COUNT(*)) AS cnt FROM test_agg) WHERE cnt > 1L
-- !query 48 schema
struct<cnt:bigint>
-- !query 48 output
-488,7 +486,7 struct<cnt:bigint>
-- !query 49
-SELECT count(*) FROM test_agg WHERE count(*) > 1L
+SELECT udf(count(*)) FROM test_agg WHERE count(*) > 1L
-- !query 49 schema
struct<>
-- !query 49 output
-500,7 +498,7 Invalid expressions: [count(1)];
-- !query 50
-SELECT count(*) FROM test_agg WHERE count(*) + 1L > 1L
+SELECT udf(count(*)) FROM test_agg WHERE count(*) + 1L > 1L
-- !query 50 schema
struct<>
-- !query 50 output
-512,7 +510,7 Invalid expressions: [count(1)];
-- !query 51
-SELECT count(*) FROM test_agg WHERE k = 1 or k = 2 or count(*) + 1L > 1L or max(k) > 1
+SELECT udf(count(*)) FROM test_agg WHERE k = 1 or k = 2 or count(*) + 1L > 1L or max(k) > 1
-- !query 51 schema
struct<>
-- !query 51 output
```
</p>
</details>
## How was this patch tested?
Tested as guided in [SPARK-27921](https://issues.apache.org/jira/browse/SPARK-27921).
Verified pandas & pyarrow versions:
```$python3
Python 3.6.8 (default, Jan 14 2019, 11:02:34)
[GCC 8.0.1 20180414 (experimental) [trunk revision 259383]] on linux
Type "help", "copyright", "credits" or "license" for more information.
>>> import pandas
>>> import pyarrow
>>> pyarrow.__version__
'0.14.0'
>>> pandas.__version__
'0.24.2'
```
From the sql output it seems that sql statements are evaluated correctly given that udf returns a string and may change results as Null will be returned as None and will be counted in returned values.
Closes#25098 from skonto/group-by.sql.
Authored-by: Stavros Kontopoulos <st.kontopoulos@gmail.com>
Signed-off-by: HyukjinKwon <gurwls223@apache.org>
## What changes were proposed in this pull request?
Because `Encoder` is not thread safe, the user cannot reuse an `Encoder` in multiple `Dataset`s. However, creating an `Encoder` for a complicated class is slow due to Scala Reflection. To eliminate the cost of Scala Reflection, right now I usually use the private API `ExpressionEncoder.copy` as follows:
```scala
object FooEncoder {
private lazy val _encoder: ExpressionEncoder[Foo] = ExpressionEncoder[Foo]()
implicit def encoder: ExpressionEncoder[Foo] = _encoder.copy()
}
```
This PR proposes a new method `makeCopy` in `Encoder` so that the above codes can be rewritten using public APIs.
```scala
object FooEncoder {
private lazy val _encoder: Encoder[Foo] = Encoders.product[Foo]()
implicit def encoder: Encoder[Foo] = _encoder.makeCopy
}
```
The method name is consistent with `TreeNode.makeCopy`.
## How was this patch tested?
Jenkins
Closes#25209 from zsxwing/encoder-copy.
Authored-by: Shixiong Zhu <zsxwing@gmail.com>
Signed-off-by: Wenchen Fan <wenchen@databricks.com>
## What changes were proposed in this pull request?
Implements the `REPLACE TABLE` and `REPLACE TABLE AS SELECT` logical plans. `REPLACE TABLE` is now a valid operation in spark-sql provided that the tables being modified are managed by V2 catalogs.
This also introduces an atomic mix-in that table catalogs can choose to implement. Table catalogs can now implement `TransactionalTableCatalog`. The semantics of this API are that table creation and replacement can be "staged" and then "committed".
On the execution of `REPLACE TABLE AS SELECT`, `REPLACE TABLE`, and `CREATE TABLE AS SELECT`, if the catalog implements transactional operations, the physical plan will use said functionality. Otherwise, these operations fall back on non-atomic variants. For `REPLACE TABLE` in particular, the usage of non-atomic operations can unfortunately lead to inconsistent state.
## How was this patch tested?
Unit tests - multiple additions to `DataSourceV2SQLSuite`.
Closes#24798 from mccheah/spark-27724.
Authored-by: mcheah <mcheah@palantir.com>
Signed-off-by: Wenchen Fan <wenchen@databricks.com>
## What changes were proposed in this pull request?
When a `ScalaUDF` returns a value which overflows, currently it returns null regardless of the value of the config `spark.sql.decimalOperations.nullOnOverflow`.
The PR makes it respect the above-mentioned config and behave accordingly.
## How was this patch tested?
added UT
Closes#25144 from mgaido91/SPARK-28369.
Authored-by: Marco Gaido <marcogaido91@gmail.com>
Signed-off-by: Wenchen Fan <wenchen@databricks.com>
## What changes were proposed in this pull request?
This pr proposes to add a prefix '*' to non-nullable attribute names in PlanTestBase.comparePlans failures. In the current master, nullability mismatches might generate the same error message for left/right logical plans like this;
```
// This failure message was extracted from #24765
- constraints should be inferred from aliased literals *** FAILED ***
== FAIL: Plans do not match ===
!'Join Inner, (two#0 = a#0) 'Join Inner, (two#0 = a#0)
:- Filter (isnotnull(a#0) AND (2 <=> a#0)) :- Filter (isnotnull(a#0) AND (2 <=> a#0))
: +- LocalRelation <empty>, [a#0, b#0, c#0] : +- LocalRelation <empty>, [a#0, b#0, c#0]
+- Project [2 AS two#0] +- Project [2 AS two#0]
+- LocalRelation <empty>, [a#0, b#0, c#0] +- LocalRelation <empty>, [a#0, b#0, c#0] (PlanTest.scala:145)
```
With this pr, this error message is changed to one below;
```
- constraints should be inferred from aliased literals *** FAILED ***
== FAIL: Plans do not match ===
!'Join Inner, (*two#0 = a#0) 'Join Inner, (*two#0 = *a#0)
:- Filter (isnotnull(a#0) AND (2 <=> a#0)) :- Filter (isnotnull(a#0) AND (2 <=> a#0))
: +- LocalRelation <empty>, [a#0, b#0, c#0] : +- LocalRelation <empty>, [a#0, b#0, c#0]
+- Project [2 AS two#0] +- Project [2 AS two#0]
+- LocalRelation <empty>, [a#0, b#0, c#0] +- LocalRelation <empty>, [a#0, b#0, c#0] (PlanTest.scala:145)
```
## How was this patch tested?
N/A
Closes#25213 from maropu/MarkForNullability.
Authored-by: Takeshi Yamamuro <yamamuro@apache.org>
Signed-off-by: Dongjoon Hyun <dhyun@apple.com>
## What changes were proposed in this pull request?
This pr is to remove the unnecessary test in DataFrameSuite.
## How was this patch tested?
N/A
Closes#25216 from maropu/SPARK-28189-FOLLOWUP.
Authored-by: Takeshi Yamamuro <yamamuro@apache.org>
Signed-off-by: Dongjoon Hyun <dhyun@apple.com>
## What changes were proposed in this pull request?
The optimize rule `PushDownPredicate` has been combined into `PushDownPredicates`, update the comment that references the old rule.
## How was this patch tested?
N/A
Closes#25207 from jiangxb1987/comment.
Authored-by: Xingbo Jiang <xingbo.jiang@databricks.com>
Signed-off-by: HyukjinKwon <gurwls223@apache.org>
## What changes were proposed in this pull request?
This PR adds some tests converted from `inline-table.sql` to test UDFs. Please see contribution guide of this umbrella ticket - [SPARK-27921](https://issues.apache.org/jira/browse/SPARK-27921).
<details><summary>Diff comparing to 'inline-table.sql'</summary>
<p>
```diff
diff --git a/sql/core/src/test/resources/sql-tests/results/inline-table.sql.out b/sql/core/src/test/resources/sql-tests/results/udf/udf-inline-table.sql.out
index 4e80f0bda5..2cf24e50c8 100644
--- a/sql/core/src/test/resources/sql-tests/results/inline-table.sql.out
+++ b/sql/core/src/test/resources/sql-tests/results/udf/udf-inline-table.sql.out
-3,33 +3,33
-- !query 0
-select * from values ("one", 1)
+select udf(col1), udf(col2) from values ("one", 1)
-- !query 0 schema
-struct<col1:string,col2:int>
+struct<CAST(udf(cast(col1 as string)) AS STRING):string,CAST(udf(cast(col2 as string)) AS INT):int>
-- !query 0 output
one 1
-- !query 1
-select * from values ("one", 1) as data
+select udf(col1), udf(udf(col2)) from values ("one", 1) as data
-- !query 1 schema
-struct<col1:string,col2:int>
+struct<CAST(udf(cast(col1 as string)) AS STRING):string,CAST(udf(cast(cast(udf(cast(col2 as string)) as int) as string)) AS INT):int>
-- !query 1 output
one 1
-- !query 2
-select * from values ("one", 1) as data(a, b)
+select udf(a), b from values ("one", 1) as data(a, b)
-- !query 2 schema
-struct<a:string,b:int>
+struct<CAST(udf(cast(a as string)) AS STRING):string,b:int>
-- !query 2 output
one 1
-- !query 3
-select * from values 1, 2, 3 as data(a)
+select udf(a) from values 1, 2, 3 as data(a)
-- !query 3 schema
-struct<a:int>
+struct<CAST(udf(cast(a as string)) AS INT):int>
-- !query 3 output
1
2
-37,9 +37,9 struct<a:int>
-- !query 4
-select * from values ("one", 1), ("two", 2), ("three", null) as data(a, b)
+select udf(a), b from values ("one", 1), ("two", 2), ("three", null) as data(a, b)
-- !query 4 schema
-struct<a:string,b:int>
+struct<CAST(udf(cast(a as string)) AS STRING):string,b:int>
-- !query 4 output
one 1
three NULL
-47,107 +47,107 two 2
-- !query 5
-select * from values ("one", null), ("two", null) as data(a, b)
+select udf(a), b from values ("one", null), ("two", null) as data(a, b)
-- !query 5 schema
-struct<a:string,b:null>
+struct<CAST(udf(cast(a as string)) AS STRING):string,b:null>
-- !query 5 output
one NULL
two NULL
-- !query 6
-select * from values ("one", 1), ("two", 2L) as data(a, b)
+select udf(a), b from values ("one", 1), ("two", 2L) as data(a, b)
-- !query 6 schema
-struct<a:string,b:bigint>
+struct<CAST(udf(cast(a as string)) AS STRING):string,b:bigint>
-- !query 6 output
one 1
two 2
-- !query 7
-select * from values ("one", 1 + 0), ("two", 1 + 3L) as data(a, b)
+select udf(udf(a)), udf(b) from values ("one", 1 + 0), ("two", 1 + 3L) as data(a, b)
-- !query 7 schema
-struct<a:string,b:bigint>
+struct<CAST(udf(cast(cast(udf(cast(a as string)) as string) as string)) AS STRING):string,CAST(udf(cast(b as string)) AS BIGINT):bigint>
-- !query 7 output
one 1
two 4
-- !query 8
-select * from values ("one", array(0, 1)), ("two", array(2, 3)) as data(a, b)
+select udf(a), b from values ("one", array(0, 1)), ("two", array(2, 3)) as data(a, b)
-- !query 8 schema
-struct<a:string,b:array<int>>
+struct<CAST(udf(cast(a as string)) AS STRING):string,b:array<int>>
-- !query 8 output
one [0,1]
two [2,3]
-- !query 9
-select * from values ("one", 2.0), ("two", 3.0D) as data(a, b)
+select udf(a), b from values ("one", 2.0), ("two", 3.0D) as data(a, b)
-- !query 9 schema
-struct<a:string,b:double>
+struct<CAST(udf(cast(a as string)) AS STRING):string,b:double>
-- !query 9 output
one 2.0
two 3.0
-- !query 10
-select * from values ("one", rand(5)), ("two", 3.0D) as data(a, b)
+select udf(a), b from values ("one", rand(5)), ("two", 3.0D) as data(a, b)
-- !query 10 schema
struct<>
-- !query 10 output
org.apache.spark.sql.AnalysisException
-cannot evaluate expression rand(5) in inline table definition; line 1 pos 29
+cannot evaluate expression rand(5) in inline table definition; line 1 pos 37
-- !query 11
-select * from values ("one", 2.0), ("two") as data(a, b)
+select udf(a), udf(b) from values ("one", 2.0), ("two") as data(a, b)
-- !query 11 schema
struct<>
-- !query 11 output
org.apache.spark.sql.AnalysisException
-expected 2 columns but found 1 columns in row 1; line 1 pos 14
+expected 2 columns but found 1 columns in row 1; line 1 pos 27
-- !query 12
-select * from values ("one", array(0, 1)), ("two", struct(1, 2)) as data(a, b)
+select udf(a), udf(b) from values ("one", array(0, 1)), ("two", struct(1, 2)) as data(a, b)
-- !query 12 schema
struct<>
-- !query 12 output
org.apache.spark.sql.AnalysisException
-incompatible types found in column b for inline table; line 1 pos 14
+incompatible types found in column b for inline table; line 1 pos 27
-- !query 13
-select * from values ("one"), ("two") as data(a, b)
+select udf(a), udf(b) from values ("one"), ("two") as data(a, b)
-- !query 13 schema
struct<>
-- !query 13 output
org.apache.spark.sql.AnalysisException
-expected 2 columns but found 1 columns in row 0; line 1 pos 14
+expected 2 columns but found 1 columns in row 0; line 1 pos 27
-- !query 14
-select * from values ("one", random_not_exist_func(1)), ("two", 2) as data(a, b)
+select udf(a), udf(b) from values ("one", random_not_exist_func(1)), ("two", 2) as data(a, b)
-- !query 14 schema
struct<>
-- !query 14 output
org.apache.spark.sql.AnalysisException
-Undefined function: 'random_not_exist_func'. This function is neither a registered temporary function nor a permanent function registered in the database 'default'.; line 1 pos 29
+Undefined function: 'random_not_exist_func'. This function is neither a registered temporary function nor a permanent function registered in the database 'default'.; line 1 pos 42
-- !query 15
-select * from values ("one", count(1)), ("two", 2) as data(a, b)
+select udf(a), udf(b) from values ("one", count(1)), ("two", 2) as data(a, b)
-- !query 15 schema
struct<>
-- !query 15 output
org.apache.spark.sql.AnalysisException
-cannot evaluate expression count(1) in inline table definition; line 1 pos 29
+cannot evaluate expression count(1) in inline table definition; line 1 pos 42
-- !query 16
-select * from values (timestamp('1991-12-06 00:00:00.0'), array(timestamp('1991-12-06 01:00:00.0'), timestamp('1991-12-06 12:00:00.0'))) as data(a, b)
+select udf(a), b from values (timestamp('1991-12-06 00:00:00.0'), array(timestamp('1991-12-06 01:00:00.0'), timestamp('1991-12-06 12:00:00.0'))) as data(a, b)
-- !query 16 schema
-struct<a:timestamp,b:array<timestamp>>
+struct<CAST(udf(cast(a as string)) AS TIMESTAMP):timestamp,b:array<timestamp>>
-- !query 16 output
1991-12-06 00:00:00 [1991-12-06 01:00:00.0,1991-12-06 12:00:00.0]
```
</p>
</details>
## How was this patch tested?
Tested as guided in [SPARK-27921](https://issues.apache.org/jira/browse/SPARK-27921).
Closes#25124 from imback82/inline-table-sql.
Authored-by: Terry Kim <yuminkim@gmail.com>
Signed-off-by: HyukjinKwon <gurwls223@apache.org>
## What changes were proposed in this pull request?
This PR adds some tests converted from group-analytics.sql to test UDFs. Please see contribution guide of this umbrella ticket - SPARK-27921.
<details><summary>Diff comparing to 'group-analytics.sql'</summary>
<p>
```diff
diff --git a/sql/core/src/test/resources/sql-tests/results/udf/udf-group-analytics.sql.out b/sql/core/src/test/resources/sql-tests/results/udf/udf-group-analytics.sql.out
index 31e9e08e2c..3439a05727 100644
--- a/sql/core/src/test/resources/sql-tests/results/udf/udf-group-analytics.sql.out
+++ b/sql/core/src/test/resources/sql-tests/results/udf/udf-group-analytics.sql.out
-13,9 +13,9 struct<>
-- !query 1
-SELECT a + b, b, udf(SUM(a - b)) FROM testData GROUP BY a + b, b WITH CUBE
+SELECT a + b, b, SUM(a - b) FROM testData GROUP BY a + b, b WITH CUBE
-- !query 1 schema
-struct<(a + b):int,b:int,CAST(udf(cast(sum(cast((a - b) as bigint)) as string)) AS BIGINT):bigint>
+struct<(a + b):int,b:int,sum((a - b)):bigint>
-- !query 1 output
2 1 0
2 NULL 0
-33,9 +33,9 NULL NULL 3
-- !query 2
-SELECT a, udf(b), SUM(b) FROM testData GROUP BY a, b WITH CUBE
+SELECT a, b, SUM(b) FROM testData GROUP BY a, b WITH CUBE
-- !query 2 schema
-struct<a:int,CAST(udf(cast(b as string)) AS INT):int,sum(b):bigint>
+struct<a:int,b:int,sum(b):bigint>
-- !query 2 output
1 1 1
1 2 2
-52,9 +52,9 NULL NULL 9
-- !query 3
-SELECT udf(a + b), b, SUM(a - b) FROM testData GROUP BY a + b, b WITH ROLLUP
+SELECT a + b, b, SUM(a - b) FROM testData GROUP BY a + b, b WITH ROLLUP
-- !query 3 schema
-struct<CAST(udf(cast((a + b) as string)) AS INT):int,b:int,sum((a - b)):bigint>
+struct<(a + b):int,b:int,sum((a - b)):bigint>
-- !query 3 output
2 1 0
2 NULL 0
-70,9 +70,9 NULL NULL 3
-- !query 4
-SELECT a, b, udf(SUM(b)) FROM testData GROUP BY a, b WITH ROLLUP
+SELECT a, b, SUM(b) FROM testData GROUP BY a, b WITH ROLLUP
-- !query 4 schema
-struct<a:int,b:int,CAST(udf(cast(sum(cast(b as bigint)) as string)) AS BIGINT):bigint>
+struct<a:int,b:int,sum(b):bigint>
-- !query 4 output
1 1 1
1 2 2
-97,7 +97,7 struct<>
-- !query 6
-SELECT course, year, SUM(earnings) FROM courseSales GROUP BY ROLLUP(course, year) ORDER BY udf(course), year
+SELECT course, year, SUM(earnings) FROM courseSales GROUP BY ROLLUP(course, year) ORDER BY course, year
-- !query 6 schema
struct<course:string,year:int,sum(earnings):bigint>
-- !query 6 output
-111,7 +111,7 dotNET 2013 48000
-- !query 7
-SELECT course, year, SUM(earnings) FROM courseSales GROUP BY CUBE(course, year) ORDER BY course, udf(year)
+SELECT course, year, SUM(earnings) FROM courseSales GROUP BY CUBE(course, year) ORDER BY course, year
-- !query 7 schema
struct<course:string,year:int,sum(earnings):bigint>
-- !query 7 output
-127,9 +127,9 dotNET 2013 48000
-- !query 8
-SELECT course, udf(year), SUM(earnings) FROM courseSales GROUP BY course, year GROUPING SETS(course, year)
+SELECT course, year, SUM(earnings) FROM courseSales GROUP BY course, year GROUPING SETS(course, year)
-- !query 8 schema
-struct<course:string,CAST(udf(cast(year as string)) AS INT):int,sum(earnings):bigint>
+struct<course:string,year:int,sum(earnings):bigint>
-- !query 8 output
Java NULL 50000
NULL 2012 35000
-138,26 +138,26 dotNET NULL 63000
-- !query 9
-SELECT course, year, udf(SUM(earnings)) FROM courseSales GROUP BY course, year GROUPING SETS(course)
+SELECT course, year, SUM(earnings) FROM courseSales GROUP BY course, year GROUPING SETS(course)
-- !query 9 schema
-struct<course:string,year:int,CAST(udf(cast(sum(cast(earnings as bigint)) as string)) AS BIGINT):bigint>
+struct<course:string,year:int,sum(earnings):bigint>
-- !query 9 output
Java NULL 50000
dotNET NULL 63000
-- !query 10
-SELECT udf(course), year, SUM(earnings) FROM courseSales GROUP BY course, year GROUPING SETS(year)
+SELECT course, year, SUM(earnings) FROM courseSales GROUP BY course, year GROUPING SETS(year)
-- !query 10 schema
-struct<CAST(udf(cast(course as string)) AS STRING):string,year:int,sum(earnings):bigint>
+struct<course:string,year:int,sum(earnings):bigint>
-- !query 10 output
NULL 2012 35000
NULL 2013 78000
-- !query 11
-SELECT course, udf(SUM(earnings)) AS sum FROM courseSales
-GROUP BY course, earnings GROUPING SETS((), (course), (course, earnings)) ORDER BY course, udf(sum)
+SELECT course, SUM(earnings) AS sum FROM courseSales
+GROUP BY course, earnings GROUPING SETS((), (course), (course, earnings)) ORDER BY course, sum
-- !query 11 schema
struct<course:string,sum:bigint>
-- !query 11 output
-173,7 +173,7 dotNET 63000
-- !query 12
SELECT course, SUM(earnings) AS sum, GROUPING_ID(course, earnings) FROM courseSales
-GROUP BY course, earnings GROUPING SETS((), (course), (course, earnings)) ORDER BY udf(course), sum
+GROUP BY course, earnings GROUPING SETS((), (course), (course, earnings)) ORDER BY course, sum
-- !query 12 schema
struct<course:string,sum:bigint,grouping_id(course, earnings):int>
-- !query 12 output
-188,10 +188,10 dotNET 63000 1
-- !query 13
-SELECT udf(course), udf(year), GROUPING(course), GROUPING(year), GROUPING_ID(course, year) FROM courseSales
+SELECT course, year, GROUPING(course), GROUPING(year), GROUPING_ID(course, year) FROM courseSales
GROUP BY CUBE(course, year)
-- !query 13 schema
-struct<CAST(udf(cast(course as string)) AS STRING):string,CAST(udf(cast(year as string)) AS INT):int,grouping(course):tinyint,grouping(year):tinyint,grouping_id(course, year):int>
+struct<course:string,year:int,grouping(course):tinyint,grouping(year):tinyint,grouping_id(course, year):int>
-- !query 13 output
Java 2012 0 0 0
Java 2013 0 0 0
-205,7 +205,7 dotNET NULL 0 1 1
-- !query 14
-SELECT course, udf(year), GROUPING(course) FROM courseSales GROUP BY course, year
+SELECT course, year, GROUPING(course) FROM courseSales GROUP BY course, year
-- !query 14 schema
struct<>
-- !query 14 output
-214,7 +214,7 grouping() can only be used with GroupingSets/Cube/Rollup;
-- !query 15
-SELECT course, udf(year), GROUPING_ID(course, year) FROM courseSales GROUP BY course, year
+SELECT course, year, GROUPING_ID(course, year) FROM courseSales GROUP BY course, year
-- !query 15 schema
struct<>
-- !query 15 output
-223,7 +223,7 grouping_id() can only be used with GroupingSets/Cube/Rollup;
-- !query 16
-SELECT course, year, grouping__id FROM courseSales GROUP BY CUBE(course, year) ORDER BY grouping__id, course, udf(year)
+SELECT course, year, grouping__id FROM courseSales GROUP BY CUBE(course, year) ORDER BY grouping__id, course, year
-- !query 16 schema
struct<course:string,year:int,grouping__id:int>
-- !query 16 output
-240,7 +240,7 NULL NULL 3
-- !query 17
SELECT course, year FROM courseSales GROUP BY CUBE(course, year)
-HAVING GROUPING(year) = 1 AND GROUPING_ID(course, year) > 0 ORDER BY course, udf(year)
+HAVING GROUPING(year) = 1 AND GROUPING_ID(course, year) > 0 ORDER BY course, year
-- !query 17 schema
struct<course:string,year:int>
-- !query 17 output
-250,7 +250,7 dotNET NULL
-- !query 18
-SELECT course, udf(year) FROM courseSales GROUP BY course, year HAVING GROUPING(course) > 0
+SELECT course, year FROM courseSales GROUP BY course, year HAVING GROUPING(course) > 0
-- !query 18 schema
struct<>
-- !query 18 output
-259,7 +259,7 grouping()/grouping_id() can only be used with GroupingSets/Cube/Rollup;
-- !query 19
-SELECT course, udf(udf(year)) FROM courseSales GROUP BY course, year HAVING GROUPING_ID(course) > 0
+SELECT course, year FROM courseSales GROUP BY course, year HAVING GROUPING_ID(course) > 0
-- !query 19 schema
struct<>
-- !query 19 output
-268,9 +268,9 grouping()/grouping_id() can only be used with GroupingSets/Cube/Rollup;
-- !query 20
-SELECT udf(course), year FROM courseSales GROUP BY CUBE(course, year) HAVING grouping__id > 0
+SELECT course, year FROM courseSales GROUP BY CUBE(course, year) HAVING grouping__id > 0
-- !query 20 schema
-struct<CAST(udf(cast(course as string)) AS STRING):string,year:int>
+struct<course:string,year:int>
-- !query 20 output
Java NULL
NULL 2012
-281,7 +281,7 dotNET NULL
-- !query 21
SELECT course, year, GROUPING(course), GROUPING(year) FROM courseSales GROUP BY CUBE(course, year)
-ORDER BY GROUPING(course), GROUPING(year), course, udf(year)
+ORDER BY GROUPING(course), GROUPING(year), course, year
-- !query 21 schema
struct<course:string,year:int,grouping(course):tinyint,grouping(year):tinyint>
-- !query 21 output
-298,7 +298,7 NULL NULL 1 1
-- !query 22
SELECT course, year, GROUPING_ID(course, year) FROM courseSales GROUP BY CUBE(course, year)
-ORDER BY GROUPING(course), GROUPING(year), course, udf(year)
+ORDER BY GROUPING(course), GROUPING(year), course, year
-- !query 22 schema
struct<course:string,year:int,grouping_id(course, year):int>
-- !query 22 output
-314,7 +314,7 NULL NULL 3
-- !query 23
-SELECT course, udf(year) FROM courseSales GROUP BY course, udf(year) ORDER BY GROUPING(course)
+SELECT course, year FROM courseSales GROUP BY course, year ORDER BY GROUPING(course)
-- !query 23 schema
struct<>
-- !query 23 output
-323,7 +323,7 grouping()/grouping_id() can only be used with GroupingSets/Cube/Rollup;
-- !query 24
-SELECT course, udf(year) FROM courseSales GROUP BY course, udf(year) ORDER BY GROUPING_ID(course)
+SELECT course, year FROM courseSales GROUP BY course, year ORDER BY GROUPING_ID(course)
-- !query 24 schema
struct<>
-- !query 24 output
-332,7 +332,7 grouping()/grouping_id() can only be used with GroupingSets/Cube/Rollup;
-- !query 25
-SELECT course, year FROM courseSales GROUP BY CUBE(course, year) ORDER BY grouping__id, udf(course), year
+SELECT course, year FROM courseSales GROUP BY CUBE(course, year) ORDER BY grouping__id, course, year
-- !query 25 schema
struct<course:string,year:int>
-- !query 25 output
-348,7 +348,7 NULL NULL
-- !query 26
-SELECT udf(a + b) AS k1, udf(b) AS k2, SUM(a - b) FROM testData GROUP BY CUBE(k1, k2)
+SELECT a + b AS k1, b AS k2, SUM(a - b) FROM testData GROUP BY CUBE(k1, k2)
-- !query 26 schema
struct<k1:int,k2:int,sum((a - b)):bigint>
-- !query 26 output
-368,7 +368,7 NULL NULL 3
-- !query 27
-SELECT udf(udf(a + b)) AS k, b, SUM(a - b) FROM testData GROUP BY ROLLUP(k, b)
+SELECT a + b AS k, b, SUM(a - b) FROM testData GROUP BY ROLLUP(k, b)
-- !query 27 schema
struct<k:int,b:int,sum((a - b)):bigint>
-- !query 27 output
-386,9 +386,9 NULL NULL 3
-- !query 28
-SELECT udf(a + b), udf(udf(b)) AS k, SUM(a - b) FROM testData GROUP BY a + b, k GROUPING SETS(k)
+SELECT a + b, b AS k, SUM(a - b) FROM testData GROUP BY a + b, k GROUPING SETS(k)
-- !query 28 schema
-struct<CAST(udf(cast((a + b) as string)) AS INT):int,k:int,sum((a - b)):bigint>
+struct<(a + b):int,k:int,sum((a - b)):bigint>
-- !query 28 output
NULL 1 3
NULL 2 0
```
</p>
</details>
## How was this patch tested?
Tested as guided in SPARK-27921.
Verified pandas & pyarrow versions:
```$python3
Python 3.6.8 (default, Jan 14 2019, 11:02:34)
[GCC 8.0.1 20180414 (experimental) [trunk revision 259383]] on linux
Type "help", "copyright", "credits" or "license" for more information.
>>> import pandas
>>> import pyarrow
>>> pyarrow.__version__
'0.14.0'
>>> pandas.__version__
'0.24.2'
```
From the sql output it seems that sql statements are evaluated correctly given that udf returns a string and may change results as Null will be returned as None and will be counted in returned values.
Closes#25196 from skonto/group-analytics.sql.
Authored-by: Stavros Kontopoulos <st.kontopoulos@gmail.com>
Signed-off-by: HyukjinKwon <gurwls223@apache.org>
## What changes were proposed in this pull request?
This PR removes a few hardware-dependent assertions which can cause a failure in `aarch64`.
**x86_64**
```
rootdonotdel-openlab-allinone-l00242678:/home/ubuntu# uname -a
Linux donotdel-openlab-allinone-l00242678 4.4.0-154-generic #181-Ubuntu SMP Tue Jun 25 05:29:03 UTC
2019 x86_64 x86_64 x86_64 GNU/Linux
scala> import java.lang.Float.floatToRawIntBits
import java.lang.Float.floatToRawIntBits
scala> floatToRawIntBits(0.0f/0.0f)
res0: Int = -4194304
scala> floatToRawIntBits(Float.NaN)
res1: Int = 2143289344
```
**aarch64**
```
[rootarm-huangtianhua spark]# uname -a
Linux arm-huangtianhua 4.14.0-49.el7a.aarch64 #1 SMP Tue Apr 10 17:22:26 UTC 2018 aarch64 aarch64 aarch64 GNU/Linux
scala> import java.lang.Float.floatToRawIntBits
import java.lang.Float.floatToRawIntBits
scala> floatToRawIntBits(0.0f/0.0f)
res1: Int = 2143289344
scala> floatToRawIntBits(Float.NaN)
res2: Int = 2143289344
```
## How was this patch tested?
Pass the Jenkins (This removes the test coverage).
Closes#25186 from huangtianhua/special-test-case-for-aarch64.
Authored-by: huangtianhua <huangtianhua@huawei.com>
Signed-off-by: Dongjoon Hyun <dhyun@apple.com>
## What changes were proposed in this pull request?
SPARK-28199 (#24996) hid implementations of Triggers into `private[sql]` and encourage end users to use `Trigger.xxx` methods instead.
As I got some post review comment on 7548a8826d (r34366934) we could remove annotations which are meant to be used with public API.
## How was this patch tested?
N/A
Closes#25200 from HeartSaVioR/SPARK-28199-FOLLOWUP.
Authored-by: Jungtaek Lim (HeartSaVioR) <kabhwan@gmail.com>
Signed-off-by: Dongjoon Hyun <dhyun@apple.com>
## What changes were proposed in this pull request?
This PR adds some tests converted from `join-empty-relation.sql` to test UDFs. Please see contribution guide of this umbrella ticket - [SPARK-27921](https://issues.apache.org/jira/browse/SPARK-27921).
<details><summary>Diff comparing to 'join-empty-relation.sql'</summary>
<p>
```diff
diff --git a/sql/core/src/test/resources/sql-tests/results/join-empty-relation.sql.out b/sql/core/src/test/resources/sql-tests/results/udf/udf-join-empty-relation.sql.out
index 857073a827..e79d01fb14 100644
--- a/sql/core/src/test/resources/sql-tests/results/join-empty-relation.sql.out
+++ b/sql/core/src/test/resources/sql-tests/results/udf/udf-join-empty-relation.sql.out
-27,111 +27,111 struct<>
-- !query 3
-SELECT * FROM t1 INNER JOIN empty_table
+SELECT udf(t1.a), udf(empty_table.a) FROM t1 INNER JOIN empty_table ON (udf(t1.a) = udf(udf(empty_table.a)))
-- !query 3 schema
-struct<a:int,a:int>
+struct<CAST(udf(cast(a as string)) AS INT):int,CAST(udf(cast(a as string)) AS INT):int>
-- !query 3 output
-- !query 4
-SELECT * FROM t1 CROSS JOIN empty_table
+SELECT udf(t1.a), udf(udf(empty_table.a)) FROM t1 CROSS JOIN empty_table ON (udf(udf(t1.a)) = udf(empty_table.a))
-- !query 4 schema
-struct<a:int,a:int>
+struct<CAST(udf(cast(a as string)) AS INT):int,CAST(udf(cast(cast(udf(cast(a as string)) as int) as string)) AS INT):int>
-- !query 4 output
-- !query 5
-SELECT * FROM t1 LEFT OUTER JOIN empty_table
+SELECT udf(udf(t1.a)), empty_table.a FROM t1 LEFT OUTER JOIN empty_table ON (udf(t1.a) = udf(empty_table.a))
-- !query 5 schema
-struct<a:int,a:int>
+struct<CAST(udf(cast(cast(udf(cast(a as string)) as int) as string)) AS INT):int,a:int>
-- !query 5 output
1 NULL
-- !query 6
-SELECT * FROM t1 RIGHT OUTER JOIN empty_table
+SELECT udf(t1.a), udf(empty_table.a) FROM t1 RIGHT OUTER JOIN empty_table ON (udf(t1.a) = udf(empty_table.a))
-- !query 6 schema
-struct<a:int,a:int>
+struct<CAST(udf(cast(a as string)) AS INT):int,CAST(udf(cast(a as string)) AS INT):int>
-- !query 6 output
-- !query 7
-SELECT * FROM t1 FULL OUTER JOIN empty_table
+SELECT udf(t1.a), empty_table.a FROM t1 FULL OUTER JOIN empty_table ON (udf(t1.a) = udf(empty_table.a))
-- !query 7 schema
-struct<a:int,a:int>
+struct<CAST(udf(cast(a as string)) AS INT):int,a:int>
-- !query 7 output
1 NULL
-- !query 8
-SELECT * FROM t1 LEFT SEMI JOIN empty_table
+SELECT udf(udf(t1.a)) FROM t1 LEFT SEMI JOIN empty_table ON (udf(t1.a) = udf(udf(empty_table.a)))
-- !query 8 schema
-struct<a:int>
+struct<CAST(udf(cast(cast(udf(cast(a as string)) as int) as string)) AS INT):int>
-- !query 8 output
-- !query 9
-SELECT * FROM t1 LEFT ANTI JOIN empty_table
+SELECT udf(t1.a) FROM t1 LEFT ANTI JOIN empty_table ON (udf(t1.a) = udf(empty_table.a))
-- !query 9 schema
-struct<a:int>
+struct<CAST(udf(cast(a as string)) AS INT):int>
-- !query 9 output
1
-- !query 10
-SELECT * FROM empty_table INNER JOIN t1
+SELECT udf(empty_table.a), udf(t1.a) FROM empty_table INNER JOIN t1 ON (udf(udf(empty_table.a)) = udf(t1.a))
-- !query 10 schema
-struct<a:int,a:int>
+struct<CAST(udf(cast(a as string)) AS INT):int,CAST(udf(cast(a as string)) AS INT):int>
-- !query 10 output
-- !query 11
-SELECT * FROM empty_table CROSS JOIN t1
+SELECT udf(empty_table.a), udf(udf(t1.a)) FROM empty_table CROSS JOIN t1 ON (udf(empty_table.a) = udf(udf(t1.a)))
-- !query 11 schema
-struct<a:int,a:int>
+struct<CAST(udf(cast(a as string)) AS INT):int,CAST(udf(cast(cast(udf(cast(a as string)) as int) as string)) AS INT):int>
-- !query 11 output
-- !query 12
-SELECT * FROM empty_table LEFT OUTER JOIN t1
+SELECT udf(udf(empty_table.a)), udf(t1.a) FROM empty_table LEFT OUTER JOIN t1 ON (udf(empty_table.a) = udf(t1.a))
-- !query 12 schema
-struct<a:int,a:int>
+struct<CAST(udf(cast(cast(udf(cast(a as string)) as int) as string)) AS INT):int,CAST(udf(cast(a as string)) AS INT):int>
-- !query 12 output
-- !query 13
-SELECT * FROM empty_table RIGHT OUTER JOIN t1
+SELECT empty_table.a, udf(t1.a) FROM empty_table RIGHT OUTER JOIN t1 ON (udf(empty_table.a) = udf(t1.a))
-- !query 13 schema
-struct<a:int,a:int>
+struct<a:int,CAST(udf(cast(a as string)) AS INT):int>
-- !query 13 output
NULL 1
-- !query 14
-SELECT * FROM empty_table FULL OUTER JOIN t1
+SELECT empty_table.a, udf(udf(t1.a)) FROM empty_table FULL OUTER JOIN t1 ON (udf(empty_table.a) = udf(t1.a))
-- !query 14 schema
-struct<a:int,a:int>
+struct<a:int,CAST(udf(cast(cast(udf(cast(a as string)) as int) as string)) AS INT):int>
-- !query 14 output
NULL 1
-- !query 15
-SELECT * FROM empty_table LEFT SEMI JOIN t1
+SELECT udf(udf(empty_table.a)) FROM empty_table LEFT SEMI JOIN t1 ON (udf(empty_table.a) = udf(udf(t1.a)))
-- !query 15 schema
-struct<a:int>
+struct<CAST(udf(cast(cast(udf(cast(a as string)) as int) as string)) AS INT):int>
-- !query 15 output
-- !query 16
-SELECT * FROM empty_table LEFT ANTI JOIN t1
+SELECT empty_table.a FROM empty_table LEFT ANTI JOIN t1 ON (udf(empty_table.a) = udf(t1.a))
-- !query 16 schema
struct<a:int>
-- !query 16 output
-139,56 +139,56 struct<a:int>
-- !query 17
-SELECT * FROM empty_table INNER JOIN empty_table
+SELECT udf(empty_table.a) FROM empty_table INNER JOIN empty_table AS empty_table2 ON (udf(empty_table.a) = udf(udf(empty_table2.a)))
-- !query 17 schema
-struct<a:int,a:int>
+struct<CAST(udf(cast(a as string)) AS INT):int>
-- !query 17 output
-- !query 18
-SELECT * FROM empty_table CROSS JOIN empty_table
+SELECT udf(udf(empty_table.a)) FROM empty_table CROSS JOIN empty_table AS empty_table2 ON (udf(udf(empty_table.a)) = udf(empty_table2.a))
-- !query 18 schema
-struct<a:int,a:int>
+struct<CAST(udf(cast(cast(udf(cast(a as string)) as int) as string)) AS INT):int>
-- !query 18 output
-- !query 19
-SELECT * FROM empty_table LEFT OUTER JOIN empty_table
+SELECT udf(empty_table.a) FROM empty_table LEFT OUTER JOIN empty_table AS empty_table2 ON (udf(empty_table.a) = udf(empty_table2.a))
-- !query 19 schema
-struct<a:int,a:int>
+struct<CAST(udf(cast(a as string)) AS INT):int>
-- !query 19 output
-- !query 20
-SELECT * FROM empty_table RIGHT OUTER JOIN empty_table
+SELECT udf(udf(empty_table.a)) FROM empty_table RIGHT OUTER JOIN empty_table AS empty_table2 ON (udf(empty_table.a) = udf(udf(empty_table2.a)))
-- !query 20 schema
-struct<a:int,a:int>
+struct<CAST(udf(cast(cast(udf(cast(a as string)) as int) as string)) AS INT):int>
-- !query 20 output
-- !query 21
-SELECT * FROM empty_table FULL OUTER JOIN empty_table
+SELECT udf(empty_table.a) FROM empty_table FULL OUTER JOIN empty_table AS empty_table2 ON (udf(empty_table.a) = udf(empty_table2.a))
-- !query 21 schema
-struct<a:int,a:int>
+struct<CAST(udf(cast(a as string)) AS INT):int>
-- !query 21 output
-- !query 22
-SELECT * FROM empty_table LEFT SEMI JOIN empty_table
+SELECT udf(udf(empty_table.a)) FROM empty_table LEFT SEMI JOIN empty_table AS empty_table2 ON (udf(empty_table.a) = udf(empty_table2.a))
-- !query 22 schema
-struct<a:int>
+struct<CAST(udf(cast(cast(udf(cast(a as string)) as int) as string)) AS INT):int>
-- !query 22 output
-- !query 23
-SELECT * FROM empty_table LEFT ANTI JOIN empty_table
+SELECT udf(empty_table.a) FROM empty_table LEFT ANTI JOIN empty_table AS empty_table2 ON (udf(empty_table.a) = udf(empty_table2.a))
-- !query 23 schema
-struct<a:int>
+struct<CAST(udf(cast(a as string)) AS INT):int>
-- !query 23 output
```
</p>
</details>
## How was this patch tested?
Tested as guided in [SPARK-27921](https://issues.apache.org/jira/browse/SPARK-27921).
Closes#25127 from imback82/join-empty-relation-sql.
Authored-by: Terry Kim <yuminkim@gmail.com>
Signed-off-by: HyukjinKwon <gurwls223@apache.org>
## What changes were proposed in this pull request?
Performance issue using explode was found when a complex field contains huge array is to get duplicated as the number of exploded array elements. Given example:
```scala
val df = spark.sparkContext.parallelize(Seq(("1",
Array.fill(M)({
val i = math.random
(i.toString, (i + 1).toString, (i + 2).toString, (i + 3).toString)
})))).toDF("col", "arr")
.selectExpr("col", "struct(col, arr) as st")
.selectExpr("col", "st.col as col1", "explode(st.arr) as arr_col")
```
The explode causes `st` to be duplicated as many as the exploded elements.
Benchmarks it:
```
[info] Java HotSpot(TM) 64-Bit Server VM 1.8.0_202-b08 on Mac OS X 10.14.4
[info] Intel(R) Core(TM) i7-8750H CPU 2.20GHz
[info] generate big nested struct array: Best Time(ms) Avg Time(ms) Stdev(ms) Rate(M/s) Per Row(ns) Relative
[info] ------------------------------------------------------------------------------------------------------------------------
[info] generate big nested struct array wholestage off 52668 53162 699 0.0 877803.4 1.0X
[info] generate big nested struct array wholestage on 47261 49093 1125 0.0 787690.2 1.1X
[info]
```
The query plan:
```
== Physical Plan ==
Project [col#508, st#512.col AS col1#515, arr_col#519]
+- Generate explode(st#512.arr), [col#508, st#512], false, [arr_col#519]
+- Project [_1#503 AS col#508, named_struct(col, _1#503, arr, _2#504) AS st#512]
+- SerializeFromObject [staticinvoke(class org.apache.spark.unsafe.types.UTF8String, StringType, fromString, knownnotnull(assertnotnull(input[0, scala.Tuple2, true]))._1, true, false) AS _1#503, mapobjects(MapObjects_loopValue84, MapObjects_loopIsNull84, ObjectType(class scala.Tuple4), if (isnull(lambdavariable(MapObjects_loopValue84, MapObjects_loopIsNull84, ObjectType(class scala.Tuple4), true))) null else named_struct(_1, staticinvoke(class org.apache.spark.unsafe.types.UTF8String, StringType, fromString, knownnotnull(lambdavariable(MapObjects_loopValue84, MapObjects_loopIsNull84, ObjectType(class scala.Tuple4), true))._1, true, false), _2, staticinvoke(class org.apache.spark.unsafe.types.UTF8String, StringType, fromString, knownnotnull(lambdavariable(MapObjects_loopValue84, MapObjects_loopIsNull84, ObjectType(class scala.Tuple4), true))._2, true, false), _3, staticinvoke(class org.apache.spark.unsafe.types.UTF8String, StringType, fromString, knownnotnull(lambdavariable(MapObjects_loopValue84, MapObjects_loopIsNull84, ObjectType(class scala.Tuple4), true))._3, true, false), _4, staticinvoke(class org.apache.spark.unsafe.types.UTF8String, StringType, fromString, knownnotnull(lambdavariable(MapObjects_loopValue84, MapObjects_loopIsNull84, ObjectType(class scala.Tuple4), true))._4, true, false)), knownnotnull(assertnotnull(input[0, scala.Tuple2, true]))._2, None) AS _2#504]
+- Scan[obj#534]
```
This patch takes nested column pruning approach to prune unnecessary nested fields. It adds a projection of the needed nested fields as aliases on the child of `Generate`, and substitutes them by alias attributes on the projection on top of `Generate`.
Benchmarks it after the change:
```
[info] Java HotSpot(TM) 64-Bit Server VM 1.8.0_202-b08 on Mac OS X 10.14.4
[info] Intel(R) Core(TM) i7-8750H CPU 2.20GHz
[info] generate big nested struct array: Best Time(ms) Avg Time(ms) Stdev(ms) Rate(M/s) Per Row(ns) Relative
[info] ------------------------------------------------------------------------------------------------------------------------
[info] generate big nested struct array wholestage off 311 331 28 0.2 5188.6 1.0X
[info] generate big nested struct array wholestage on 297 312 15 0.2 4947.3 1.0X
[info]
```
The query plan:
```
== Physical Plan ==
Project [col#592, _gen_alias_608#608 AS col1#599, arr_col#603]
+- Generate explode(st#596.arr), [col#592, _gen_alias_608#608], false, [arr_col#603]
+- Project [_1#587 AS col#592, named_struct(col, _1#587, arr, _2#588) AS st#596, _1#587 AS _gen_alias_608#608]
+- SerializeFromObject [staticinvoke(class org.apache.spark.unsafe.types.UTF8String, StringType, fromString, knownnotnull(assertnotnull(in
put[0, scala.Tuple2, true]))._1, true, false) AS _1#587, mapobjects(MapObjects_loopValue102, MapObjects_loopIsNull102, ObjectType(class scala.Tuple4),
if (isnull(lambdavariable(MapObjects_loopValue102, MapObjects_loopIsNull102, ObjectType(class scala.Tuple4), true))) null else named_struct(_1, staticinvoke(class org.apache.spark.unsafe.types.UTF8String, StringType, fromString, knownnotnull(lambdavariable(MapObjects_loopValue102, MapObjects_loopIsNull102, ObjectType(class scala.Tuple4), true))._1, true, false), _2, staticinvoke(class org.apache.spark.unsafe.types.UTF8String, StringType, fromString, knownnotnull(lambdavariable(MapObjects_loopValue102, MapObjects_loopIsNull102, ObjectType(class scala.Tuple4), true))._2, true, false), _3, staticinvoke(class org.apache.spark.unsafe.types.UTF8String, StringType, fromString, knownnotnull(lambdavariable(MapObjects_loopValue102, MapObjects_loopIsNull102, ObjectType(class scala.Tuple4), true))._3, true, false), _4, staticinvoke(class org.apache.spark.unsafe.types.UTF8String, StringType, fromString, knownnotnull(lambdavariable(MapObjects_loopValue102, MapObjects_loopIsNull102, ObjectType(class scala.Tuple4), true))._4, true, false)), knownnotnull(assertnotnull(input[0, scala.Tuple2, true]))._2, None) AS _2#588]
+- Scan[obj#586]
```
This behavior is controlled by a SQL config `spark.sql.optimizer.expression.nestedPruning.enabled`.
## How was this patch tested?
Added benchmark.
Closes#24637 from viirya/SPARK-27707.
Lead-authored-by: Liang-Chi Hsieh <viirya@gmail.com>
Co-authored-by: Dongjoon Hyun <dhyun@apple.com>
Signed-off-by: Dongjoon Hyun <dhyun@apple.com>
## What changes were proposed in this pull request?
This PR adds some tests converted from ```except.sql``` to test UDFs. Please see contribution guide of this umbrella ticket - [SPARK-27921](https://issues.apache.org/jira/browse/SPARK-27921).
<details><summary>Diff comparing to 'except.sql'</summary>
<p>
```diff
diff --git a/sql/core/src/test/resources/sql-tests/results/except.sql.out b/sql/core/src/test/resources/sql-tests/results/udf/udf-except.sql.out
index c9b712d4d2..27ca7ea226 100644
--- a/sql/core/src/test/resources/sql-tests/results/except.sql.out
+++ b/sql/core/src/test/resources/sql-tests/results/udf/udf-except.sql.out
-30,16 +30,16 struct<>
-- !query 2
-SELECT * FROM t1 EXCEPT SELECT * FROM t2
+SELECT udf(k), udf(v) FROM t1 EXCEPT SELECT udf(k), udf(v) FROM t2
-- !query 2 schema
-struct<k:string,v:int>
+struct<CAST(udf(cast(k as string)) AS STRING):string,CAST(udf(cast(v as string)) AS INT):int>
-- !query 2 output
three 3
two 2
-- !query 3
-SELECT * FROM t1 EXCEPT SELECT * FROM t1 where v <> 1 and v <> 2
+SELECT * FROM t1 EXCEPT SELECT * FROM t1 where udf(v) <> 1 and v <> udf(2)
-- !query 3 schema
struct<k:string,v:int>
-- !query 3 output
-49,7 +49,7 two 2
-- !query 4
-SELECT * FROM t1 where v <> 1 and v <> 22 EXCEPT SELECT * FROM t1 where v <> 2 and v >= 3
+SELECT * FROM t1 where udf(v) <> 1 and v <> udf(22) EXCEPT SELECT * FROM t1 where udf(v) <> 2 and v >= udf(3)
-- !query 4 schema
struct<k:string,v:int>
-- !query 4 output
-59,7 +59,7 two 2
-- !query 5
SELECT t1.* FROM t1, t2 where t1.k = t2.k
EXCEPT
-SELECT t1.* FROM t1, t2 where t1.k = t2.k and t1.k != 'one'
+SELECT t1.* FROM t1, t2 where t1.k = t2.k and t1.k != udf('one')
-- !query 5 schema
struct<k:string,v:int>
-- !query 5 output
-68,7 +68,7 one NULL
-- !query 6
-SELECT * FROM t2 where v >= 1 and v <> 22 EXCEPT SELECT * FROM t1
+SELECT * FROM t2 where v >= udf(1) and udf(v) <> 22 EXCEPT SELECT * FROM t1
-- !query 6 schema
struct<k:string,v:int>
-- !query 6 output
-77,9 +77,9 one 5
-- !query 7
-SELECT (SELECT min(k) FROM t2 WHERE t2.k = t1.k) min_t2 FROM t1
+SELECT (SELECT min(udf(k)) FROM t2 WHERE t2.k = t1.k) min_t2 FROM t1
MINUS
-SELECT (SELECT min(k) FROM t2) abs_min_t2 FROM t1 WHERE t1.k = 'one'
+SELECT (SELECT udf(min(k)) FROM t2) abs_min_t2 FROM t1 WHERE t1.k = udf('one')
-- !query 7 schema
struct<min_t2:string>
-- !query 7 output
-90,16 +90,17 two
-- !query 8
SELECT t1.k
FROM t1
-WHERE t1.v <= (SELECT max(t2.v)
+WHERE t1.v <= (SELECT udf(max(udf(t2.v)))
FROM t2
- WHERE t2.k = t1.k)
+ WHERE udf(t2.k) = udf(t1.k))
MINUS
SELECT t1.k
FROM t1
-WHERE t1.v >= (SELECT min(t2.v)
+WHERE udf(t1.v) >= (SELECT min(udf(t2.v))
FROM t2
WHERE t2.k = t1.k)
-- !query 8 schema
-struct<k:string>
+struct<>
-- !query 8 output
-two
+java.lang.UnsupportedOperationException
+Cannot evaluate expression: udf(cast(null as string))
```
</p>
</details>
## How was this patch tested?
Tested as guided in [SPARK-27921.](https://issues.apache.org/jira/browse/SPARK-27921)
Closes#25101 from huaxingao/spark-28277.
Authored-by: Huaxin Gao <huaxing@us.ibm.com>
Signed-off-by: HyukjinKwon <gurwls223@apache.org>
## What changes were proposed in this pull request?
This PR adds some tests converted from ```outer-join.sql``` to test UDFs. Please see contribution guide of this umbrella ticket - [SPARK-27921](https://issues.apache.org/jira/browse/SPARK-27921).
<details><summary>Diff comparing to 'outer-join.sql'</summary>
<p>
```diff
diff --git a/sql/core/src/test/resources/sql-tests/results/outer-join.sql.out b/sql/core/src/test/resources/sql-tests/results/udf/udf-outer-join.sql.out
index 5db3bae5d0..819f786070 100644
--- a/sql/core/src/test/resources/sql-tests/results/outer-join.sql.out
+++ b/sql/core/src/test/resources/sql-tests/results/udf/udf-outer-join.sql.out
-24,17 +24,17 struct<>
-- !query 2
SELECT
- (SUM(COALESCE(t1.int_col1, t2.int_col0))),
- ((COALESCE(t1.int_col1, t2.int_col0)) * 2)
+ (udf(SUM(udf(COALESCE(t1.int_col1, t2.int_col0))))),
+ (udf(COALESCE(t1.int_col1, t2.int_col0)) * 2)
FROM t1
RIGHT JOIN t2
- ON (t2.int_col0) = (t1.int_col1)
-GROUP BY GREATEST(COALESCE(t2.int_col1, 109), COALESCE(t1.int_col1, -449)),
+ ON udf(t2.int_col0) = udf(t1.int_col1)
+GROUP BY udf(GREATEST(COALESCE(udf(t2.int_col1), 109), COALESCE(t1.int_col1, udf(-449)))),
COALESCE(t1.int_col1, t2.int_col0)
-HAVING (SUM(COALESCE(t1.int_col1, t2.int_col0)))
- > ((COALESCE(t1.int_col1, t2.int_col0)) * 2)
+HAVING (udf(SUM(COALESCE(udf(t1.int_col1), udf(t2.int_col0)))))
+ > (udf(COALESCE(t1.int_col1, t2.int_col0)) * 2)
-- !query 2 schema
-struct<sum(coalesce(int_col1, int_col0)):bigint,(coalesce(int_col1, int_col0) * 2):int>
+struct<CAST(udf(cast(sum(cast(cast(udf(cast(coalesce(int_col1, int_col0) as string)) as int) as bigint)) as string)) AS BIGINT):bigint,(CAST(udf(cast(coalesce(int_col1, int_col0) as string)) AS INT) * 2):int>
-- !query 2 output
-367 -734
-507 -1014
-70,10 +70,10 spark.sql.crossJoin.enabled true
SELECT *
FROM (
SELECT
- COALESCE(t2.int_col1, t1.int_col1) AS int_col
+ udf(COALESCE(udf(t2.int_col1), udf(t1.int_col1))) AS int_col
FROM t1
LEFT JOIN t2 ON false
-) t where (t.int_col) is not null
+) t where (udf(t.int_col)) is not null
-- !query 6 schema
struct<int_col:int>
-- !query 6 output
```
</p>
</details>
## How was this patch tested?
Tested as guided in [SPARK-27921](https://issues.apache.org/jira/browse/SPARK-27921).
Closes#25103 from huaxingao/spark-28285.
Authored-by: Huaxin Gao <huaxing@us.ibm.com>
Signed-off-by: HyukjinKwon <gurwls223@apache.org>
## What changes were proposed in this pull request?
This PR adds some tests converted from 'udaf.sql' to test UDFs
<details><summary>Diff comparing to 'udaf.sql'</summary>
<p>
```diff
diff --git a/sql/core/src/test/resources/sql-tests/results/udaf.sql.out b/sql/core/src/test/resources/sql-tests/results/udf/udf-udaf.sql.out
index f4455bb717..e1747f4667 100644
--- a/sql/core/src/test/resources/sql-tests/results/udaf.sql.out
+++ b/sql/core/src/test/resources/sql-tests/results/udf/udf-udaf.sql.out
-3,6 +3,8
-- !query 0
+-- This test file was converted from udaf.sql.
+
CREATE OR REPLACE TEMPORARY VIEW t1 AS SELECT * FROM VALUES
(1), (2), (3), (4)
as t1(int_col1)
-21,15 +23,15 struct<>
-- !query 2
-SELECT default.myDoubleAvg(int_col1) as my_avg from t1
+SELECT default.myDoubleAvg(udf(int_col1)) as my_avg, udf(default.myDoubleAvg(udf(int_col1))) as my_avg2, udf(default.myDoubleAvg(int_col1)) as my_avg3 from t1
-- !query 2 schema
-struct<my_avg:double>
+struct<my_avg:double,my_avg2:double,my_avg3:double>
-- !query 2 output
-102.5
+102.5 102.5 102.5
-- !query 3
-SELECT default.myDoubleAvg(int_col1, 3) as my_avg from t1
+SELECT default.myDoubleAvg(udf(int_col1), udf(3)) as my_avg from t1
-- !query 3 schema
struct<>
-- !query 3 output
-46,12 +48,12 struct<>
-- !query 5
-SELECT default.udaf1(int_col1) as udaf1 from t1
+SELECT default.udaf1(udf(int_col1)) as udaf1, udf(default.udaf1(udf(int_col1))) as udaf2, udf(default.udaf1(int_col1)) as udaf3 from t1
-- !query 5 schema
struct<>
-- !query 5 output
org.apache.spark.sql.AnalysisException
-Can not load class 'test.non.existent.udaf' when registering the function 'default.udaf1', please make sure it is on the classpath; line 1 pos 7
+Can not load class 'test.non.existent.udaf' when registering the function 'default.udaf1', please make sure it is on the classpath; line 1 pos 94
-- !query 6
```
</p>
</details>
## How was this patch tested?
Tested as guided in SPARK-27921.
Closes#25194 from vinodkc/br_Fix_SPARK-27921_3.
Authored-by: Vinod KC <vinod.kc.in@gmail.com>
Signed-off-by: HyukjinKwon <gurwls223@apache.org>
## What changes were proposed in this pull request?
The `DateTimeUtils.timestampAddInterval` method was rewritten by using Java 8 time API. To add months and microseconds, I used the `plusMonths()` and `plus()` methods of `ZonedDateTime`. Also the signature of `timestampAddInterval()` was changed to accept an `ZoneId` instance instead of `TimeZone`. Using `ZoneId` allows to avoid the conversion `TimeZone` -> `ZoneId` on every invoke of `timestampAddInterval()`.
## How was this patch tested?
By existing test suites `DateExpressionsSuite`, `TypeCoercionSuite` and `CollectionExpressionsSuite`.
Closes#25173 from MaxGekk/timestamp-add-interval.
Authored-by: Maxim Gekk <max.gekk@gmail.com>
Signed-off-by: Sean Owen <sean.owen@databricks.com>
## What changes were proposed in this pull request?
This PR adds some tests converted from pivot.sql to test UDFs following the combination guide in [SPARK-27921](https://issues.apache.org/jira/browse/SPARK-27921).
<details><summary>Diff comparing to 'pivot.sql'</summary>
<p>
```diff
diff --git a/sql/core/src/test/resources/sql-tests/results/pivot.sql.out b/sql/core/src/test/resources/sql-tests/results/udf/udf-pivot.sql.out
index 9a8f783da4..cb9e4d736c 100644
--- a/sql/core/src/test/resources/sql-tests/results/pivot.sql.out
+++ b/sql/core/src/test/resources/sql-tests/results/udf/udf-pivot.sql.out
-1,5 +1,5
-- Automatically generated by SQLQueryTestSuite
--- Number of queries: 32
+-- Number of queries: 30
-- !query 0
-40,14 +40,14 struct<>
-- !query 3
SELECT * FROM (
- SELECT year, course, earnings FROM courseSales
+ SELECT udf(year), course, earnings FROM courseSales
)
PIVOT (
- sum(earnings)
+ udf(sum(earnings))
FOR course IN ('dotNET', 'Java')
)
-- !query 3 schema
-struct<year:int,dotNET:bigint,Java:bigint>
+struct<CAST(udf(cast(year as string)) AS INT):int,dotNET:bigint,Java:bigint>
-- !query 3 output
2012 15000 20000
2013 48000 30000
-56,7 +56,7 struct<year:int,dotNET:bigint,Java:bigint>
-- !query 4
SELECT * FROM courseSales
PIVOT (
- sum(earnings)
+ udf(sum(earnings))
FOR year IN (2012, 2013)
)
-- !query 4 schema
-71,11 +71,11 SELECT * FROM (
SELECT year, course, earnings FROM courseSales
)
PIVOT (
- sum(earnings), avg(earnings)
+ udf(sum(earnings)), udf(avg(earnings))
FOR course IN ('dotNET', 'Java')
)
-- !query 5 schema
-struct<year:int,dotNET_sum(CAST(earnings AS BIGINT)):bigint,dotNET_avg(CAST(earnings AS BIGINT)):double,Java_sum(CAST(earnings AS BIGINT)):bigint,Java_avg(CAST(earnings AS BIGINT)):double>
+struct<year:int,dotNET_CAST(udf(cast(sum(cast(earnings as bigint)) as string)) AS BIGINT):bigint,dotNET_CAST(udf(cast(avg(cast(earnings as bigint)) as string)) AS DOUBLE):double,Java_CAST(udf(cast(sum(cast(earnings as bigint)) as string)) AS BIGINT):bigint,Java_CAST(udf(cast(avg(cast(earnings as bigint)) as string)) AS DOUBLE):double>
-- !query 5 output
2012 15000 7500.0 20000 20000.0
2013 48000 48000.0 30000 30000.0
-83,10 +83,10 struct<year:int,dotNET_sum(CAST(earnings AS BIGINT)):bigint,dotNET_avg(CAST(earn
-- !query 6
SELECT * FROM (
- SELECT course, earnings FROM courseSales
+ SELECT udf(course) as course, earnings FROM courseSales
)
PIVOT (
- sum(earnings)
+ udf(sum(earnings))
FOR course IN ('dotNET', 'Java')
)
-- !query 6 schema
-100,23 +100,23 SELECT * FROM (
SELECT year, course, earnings FROM courseSales
)
PIVOT (
- sum(earnings), min(year)
+ udf(sum(udf(earnings))), udf(min(year))
FOR course IN ('dotNET', 'Java')
)
-- !query 7 schema
-struct<dotNET_sum(CAST(earnings AS BIGINT)):bigint,dotNET_min(year):int,Java_sum(CAST(earnings AS BIGINT)):bigint,Java_min(year):int>
+struct<dotNET_CAST(udf(cast(sum(cast(cast(udf(cast(earnings as string)) as int) as bigint)) as string)) AS BIGINT):bigint,dotNET_CAST(udf(cast(min(year) as string)) AS INT):int,Java_CAST(udf(cast(sum(cast(cast(udf(cast(earnings as string)) as int) as bigint)) as string)) AS BIGINT):bigint,Java_CAST(udf(cast(min(year) as string)) AS INT):int>
-- !query 7 output
63000 2012 50000 2012
-- !query 8
SELECT * FROM (
- SELECT course, year, earnings, s
+ SELECT course, year, earnings, udf(s) as s
FROM courseSales
JOIN years ON year = y
)
PIVOT (
- sum(earnings)
+ udf(sum(earnings))
FOR s IN (1, 2)
)
-- !query 8 schema
-135,11 +135,11 SELECT * FROM (
JOIN years ON year = y
)
PIVOT (
- sum(earnings), min(s)
+ udf(sum(earnings)), udf(min(s))
FOR course IN ('dotNET', 'Java')
)
-- !query 9 schema
-struct<year:int,dotNET_sum(CAST(earnings AS BIGINT)):bigint,dotNET_min(s):int,Java_sum(CAST(earnings AS BIGINT)):bigint,Java_min(s):int>
+struct<year:int,dotNET_CAST(udf(cast(sum(cast(earnings as bigint)) as string)) AS BIGINT):bigint,dotNET_CAST(udf(cast(min(s) as string)) AS INT):int,Java_CAST(udf(cast(sum(cast(earnings as bigint)) as string)) AS BIGINT):bigint,Java_CAST(udf(cast(min(s) as string)) AS INT):int>
-- !query 9 output
2012 15000 1 20000 1
2013 48000 2 30000 2
-152,7 +152,7 SELECT * FROM (
JOIN years ON year = y
)
PIVOT (
- sum(earnings * s)
+ udf(sum(earnings * s))
FOR course IN ('dotNET', 'Java')
)
-- !query 10 schema
-167,7 +167,7 SELECT 2012_s, 2013_s, 2012_a, 2013_a, c FROM (
SELECT year y, course c, earnings e FROM courseSales
)
PIVOT (
- sum(e) s, avg(e) a
+ udf(sum(e)) s, udf(avg(e)) a
FOR y IN (2012, 2013)
)
-- !query 11 schema
-182,7 +182,7 SELECT firstYear_s, secondYear_s, firstYear_a, secondYear_a, c FROM (
SELECT year y, course c, earnings e FROM courseSales
)
PIVOT (
- sum(e) s, avg(e) a
+ udf(sum(e)) s, udf(avg(e)) a
FOR y IN (2012 as firstYear, 2013 secondYear)
)
-- !query 12 schema
-195,7 +195,7 struct<firstYear_s:bigint,secondYear_s:bigint,firstYear_a:double,secondYear_a:do
-- !query 13
SELECT * FROM courseSales
PIVOT (
- abs(earnings)
+ udf(abs(earnings))
FOR year IN (2012, 2013)
)
-- !query 13 schema
-210,7 +210,7 SELECT * FROM (
SELECT year, course, earnings FROM courseSales
)
PIVOT (
- sum(earnings), year
+ udf(sum(earnings)), year
FOR course IN ('dotNET', 'Java')
)
-- !query 14 schema
-225,7 +225,7 SELECT * FROM (
SELECT course, earnings FROM courseSales
)
PIVOT (
- sum(earnings)
+ udf(sum(earnings))
FOR year IN (2012, 2013)
)
-- !query 15 schema
-240,11 +240,11 SELECT * FROM (
SELECT year, course, earnings FROM courseSales
)
PIVOT (
- ceil(sum(earnings)), avg(earnings) + 1 as a1
+ udf(ceil(udf(sum(earnings)))), avg(earnings) + 1 as a1
FOR course IN ('dotNET', 'Java')
)
-- !query 16 schema
-struct<year:int,dotNET_CEIL(sum(CAST(earnings AS BIGINT))):bigint,dotNET_a1:double,Java_CEIL(sum(CAST(earnings AS BIGINT))):bigint,Java_a1:double>
+struct<year:int,dotNET_CAST(udf(cast(CEIL(cast(udf(cast(sum(cast(earnings as bigint)) as string)) as bigint)) as string)) AS BIGINT):bigint,dotNET_a1:double,Java_CAST(udf(cast(CEIL(cast(udf(cast(sum(cast(earnings as bigint)) as string)) as bigint)) as string)) AS BIGINT):bigint,Java_a1:double>
-- !query 16 output
2012 15000 7501.0 20000 20001.0
2013 48000 48001.0 30000 30001.0
-255,7 +255,7 SELECT * FROM (
SELECT year, course, earnings FROM courseSales
)
PIVOT (
- sum(avg(earnings))
+ sum(udf(avg(earnings)))
FOR course IN ('dotNET', 'Java')
)
-- !query 17 schema
-272,7 +272,7 SELECT * FROM (
JOIN years ON year = y
)
PIVOT (
- sum(earnings)
+ udf(sum(earnings))
FOR (course, year) IN (('dotNET', 2012), ('Java', 2013))
)
-- !query 18 schema
-289,7 +289,7 SELECT * FROM (
JOIN years ON year = y
)
PIVOT (
- sum(earnings)
+ udf(sum(earnings))
FOR (course, s) IN (('dotNET', 2) as c1, ('Java', 1) as c2)
)
-- !query 19 schema
-306,7 +306,7 SELECT * FROM (
JOIN years ON year = y
)
PIVOT (
- sum(earnings)
+ udf(sum(earnings))
FOR (course, year) IN ('dotNET', 'Java')
)
-- !query 20 schema
-319,7 +319,7 Invalid pivot value 'dotNET': value data type string does not match pivot column
-- !query 21
SELECT * FROM courseSales
PIVOT (
- sum(earnings)
+ udf(sum(earnings))
FOR year IN (s, 2013)
)
-- !query 21 schema
-332,7 +332,7 cannot resolve '`s`' given input columns: [coursesales.course, coursesales.earni
-- !query 22
SELECT * FROM courseSales
PIVOT (
- sum(earnings)
+ udf(sum(earnings))
FOR year IN (course, 2013)
)
-- !query 22 schema
-343,151 +343,118 Literal expressions required for pivot values, found 'course#x';
-- !query 23
-SELECT * FROM (
- SELECT course, year, a
- FROM courseSales
- JOIN yearsWithComplexTypes ON year = y
-)
-PIVOT (
- min(a)
- FOR course IN ('dotNET', 'Java')
-)
--- !query 23 schema
-struct<year:int,dotNET:array<int>,Java:array<int>>
--- !query 23 output
-2012 [1,1] [1,1]
-2013 [2,2] [2,2]
-
-
--- !query 24
-SELECT * FROM (
- SELECT course, year, y, a
- FROM courseSales
- JOIN yearsWithComplexTypes ON year = y
-)
-PIVOT (
- max(a)
- FOR (y, course) IN ((2012, 'dotNET'), (2013, 'Java'))
-)
--- !query 24 schema
-struct<year:int,[2012, dotNET]:array<int>,[2013, Java]:array<int>>
--- !query 24 output
-2012 [1,1] NULL
-2013 NULL [2,2]
-
-
--- !query 25
SELECT * FROM (
SELECT earnings, year, a
FROM courseSales
JOIN yearsWithComplexTypes ON year = y
)
PIVOT (
- sum(earnings)
+ udf(sum(earnings))
FOR a IN (array(1, 1), array(2, 2))
)
--- !query 25 schema
+-- !query 23 schema
struct<year:int,[1, 1]:bigint,[2, 2]:bigint>
--- !query 25 output
+-- !query 23 output
2012 35000 NULL
2013 NULL 78000
--- !query 26
+-- !query 24
SELECT * FROM (
- SELECT course, earnings, year, a
+ SELECT course, earnings, udf(year) as year, a
FROM courseSales
JOIN yearsWithComplexTypes ON year = y
)
PIVOT (
- sum(earnings)
+ udf(sum(earnings))
FOR (course, a) IN (('dotNET', array(1, 1)), ('Java', array(2, 2)))
)
--- !query 26 schema
+-- !query 24 schema
struct<year:int,[dotNET, [1, 1]]:bigint,[Java, [2, 2]]:bigint>
--- !query 26 output
+-- !query 24 output
2012 15000 NULL
2013 NULL 30000
--- !query 27
+-- !query 25
SELECT * FROM (
SELECT earnings, year, s
FROM courseSales
JOIN yearsWithComplexTypes ON year = y
)
PIVOT (
- sum(earnings)
+ udf(sum(earnings))
FOR s IN ((1, 'a'), (2, 'b'))
)
--- !query 27 schema
+-- !query 25 schema
struct<year:int,[1, a]:bigint,[2, b]:bigint>
--- !query 27 output
+-- !query 25 output
2012 35000 NULL
2013 NULL 78000
--- !query 28
+-- !query 26
SELECT * FROM (
SELECT course, earnings, year, s
FROM courseSales
JOIN yearsWithComplexTypes ON year = y
)
PIVOT (
- sum(earnings)
+ udf(sum(earnings))
FOR (course, s) IN (('dotNET', (1, 'a')), ('Java', (2, 'b')))
)
--- !query 28 schema
+-- !query 26 schema
struct<year:int,[dotNET, [1, a]]:bigint,[Java, [2, b]]:bigint>
--- !query 28 output
+-- !query 26 output
2012 15000 NULL
2013 NULL 30000
--- !query 29
+-- !query 27
SELECT * FROM (
SELECT earnings, year, m
FROM courseSales
JOIN yearsWithComplexTypes ON year = y
)
PIVOT (
- sum(earnings)
+ udf(sum(earnings))
FOR m IN (map('1', 1), map('2', 2))
)
--- !query 29 schema
+-- !query 27 schema
struct<>
--- !query 29 output
+-- !query 27 output
org.apache.spark.sql.AnalysisException
Invalid pivot column 'm#x'. Pivot columns must be comparable.;
--- !query 30
+-- !query 28
SELECT * FROM (
SELECT course, earnings, year, m
FROM courseSales
JOIN yearsWithComplexTypes ON year = y
)
PIVOT (
- sum(earnings)
+ udf(sum(earnings))
FOR (course, m) IN (('dotNET', map('1', 1)), ('Java', map('2', 2)))
)
--- !query 30 schema
+-- !query 28 schema
struct<>
--- !query 30 output
+-- !query 28 output
org.apache.spark.sql.AnalysisException
Invalid pivot column 'named_struct(course, course#x, m, m#x)'. Pivot columns must be comparable.;
--- !query 31
+-- !query 29
SELECT * FROM (
- SELECT course, earnings, "a" as a, "z" as z, "b" as b, "y" as y, "c" as c, "x" as x, "d" as d, "w" as w
+ SELECT course, earnings, udf("a") as a, udf("z") as z, udf("b") as b, udf("y") as y,
+ udf("c") as c, udf("x") as x, udf("d") as d, udf("w") as w
FROM courseSales
)
PIVOT (
- sum(Earnings)
+ udf(sum(Earnings))
FOR Course IN ('dotNET', 'Java')
)
--- !query 31 schema
+-- !query 29 schema
struct<a:string,z:string,b:string,y:string,c:string,x:string,d:string,w:string,dotNET:bigint,Java:bigint>
--- !query 31 output
+-- !query 29 output
a z b y c x d w 63000 50000
```
</p>
</details>
## How was this patch tested?
Tested as guided in [SPARK-27921](https://issues.apache.org/jira/browse/SPARK-27921).
Closes#25122 from chitralverma/SPARK-28286.
Authored-by: chitralverma <chitralverma@gmail.com>
Signed-off-by: HyukjinKwon <gurwls223@apache.org>
## What changes were proposed in this pull request?
This PR adds some tests converted from `except-all.sql` to test UDFs. Please see contribution guide of this umbrella ticket - [SPARK-27921](https://issues.apache.org/jira/browse/SPARK-27921).
<details><summary>Diff comparing to 'except-all.sql'</summary>
<p>
```diff
diff --git a/sql/core/src/test/resources/sql-tests/results/except-all.sql.out b/sql/core/src/test/resources/sql-tests/results/udf/udf-except-all.sql.out
index 01091a2f75..b7bfad0e53 100644
--- a/sql/core/src/test/resources/sql-tests/results/except-all.sql.out
+++ b/sql/core/src/test/resources/sql-tests/results/udf/udf-except-all.sql.out
-49,11 +49,11 struct<>
-- !query 4
-SELECT * FROM tab1
+SELECT udf(c1) FROM tab1
EXCEPT ALL
-SELECT * FROM tab2
+SELECT udf(c1) FROM tab2
-- !query 4 schema
-struct<c1:int>
+struct<CAST(udf(cast(c1 as string)) AS INT):int>
-- !query 4 output
0
2
-62,11 +62,11 NULL
-- !query 5
-SELECT * FROM tab1
+SELECT udf(c1) FROM tab1
MINUS ALL
-SELECT * FROM tab2
+SELECT udf(c1) FROM tab2
-- !query 5 schema
-struct<c1:int>
+struct<CAST(udf(cast(c1 as string)) AS INT):int>
-- !query 5 output
0
2
-75,11 +75,11 NULL
-- !query 6
-SELECT * FROM tab1
+SELECT udf(c1) FROM tab1
EXCEPT ALL
-SELECT * FROM tab2 WHERE c1 IS NOT NULL
+SELECT udf(c1) FROM tab2 WHERE udf(c1) IS NOT NULL
-- !query 6 schema
-struct<c1:int>
+struct<CAST(udf(cast(c1 as string)) AS INT):int>
-- !query 6 output
0
2
-89,21 +89,21 NULL
-- !query 7
-SELECT * FROM tab1 WHERE c1 > 5
+SELECT udf(c1) FROM tab1 WHERE udf(c1) > 5
EXCEPT ALL
-SELECT * FROM tab2
+SELECT udf(c1) FROM tab2
-- !query 7 schema
-struct<c1:int>
+struct<CAST(udf(cast(c1 as string)) AS INT):int>
-- !query 7 output
-- !query 8
-SELECT * FROM tab1
+SELECT udf(c1) FROM tab1
EXCEPT ALL
-SELECT * FROM tab2 WHERE c1 > 6
+SELECT udf(c1) FROM tab2 WHERE udf(c1 > udf(6))
-- !query 8 schema
-struct<c1:int>
+struct<CAST(udf(cast(c1 as string)) AS INT):int>
-- !query 8 output
0
1
-117,11 +117,11 NULL
-- !query 9
-SELECT * FROM tab1
+SELECT udf(c1) FROM tab1
EXCEPT ALL
-SELECT CAST(1 AS BIGINT)
+SELECT CAST(udf(1) AS BIGINT)
-- !query 9 schema
-struct<c1:bigint>
+struct<CAST(udf(cast(c1 as string)) AS INT):bigint>
-- !query 9 output
0
2
-134,7 +134,7 NULL
-- !query 10
-SELECT * FROM tab1
+SELECT udf(c1) FROM tab1
EXCEPT ALL
SELECT array(1)
-- !query 10 schema
-145,62 +145,62 ExceptAll can only be performed on tables with the compatible column types. arra
-- !query 11
-SELECT * FROM tab3
+SELECT udf(k), v FROM tab3
EXCEPT ALL
-SELECT * FROM tab4
+SELECT k, udf(v) FROM tab4
-- !query 11 schema
-struct<k:int,v:int>
+struct<CAST(udf(cast(k as string)) AS INT):int,v:int>
-- !query 11 output
1 2
1 3
-- !query 12
-SELECT * FROM tab4
+SELECT k, udf(v) FROM tab4
EXCEPT ALL
-SELECT * FROM tab3
+SELECT udf(k), v FROM tab3
-- !query 12 schema
-struct<k:int,v:int>
+struct<k:int,CAST(udf(cast(v as string)) AS INT):int>
-- !query 12 output
2 2
2 20
-- !query 13
-SELECT * FROM tab4
+SELECT udf(k), udf(v) FROM tab4
EXCEPT ALL
-SELECT * FROM tab3
+SELECT udf(k), udf(v) FROM tab3
INTERSECT DISTINCT
-SELECT * FROM tab4
+SELECT udf(k), udf(v) FROM tab4
-- !query 13 schema
-struct<k:int,v:int>
+struct<CAST(udf(cast(k as string)) AS INT):int,CAST(udf(cast(v as string)) AS INT):int>
-- !query 13 output
2 2
2 20
-- !query 14
-SELECT * FROM tab4
+SELECT udf(k), v FROM tab4
EXCEPT ALL
-SELECT * FROM tab3
+SELECT k, udf(v) FROM tab3
EXCEPT DISTINCT
-SELECT * FROM tab4
+SELECT udf(k), udf(v) FROM tab4
-- !query 14 schema
-struct<k:int,v:int>
+struct<CAST(udf(cast(k as string)) AS INT):int,v:int>
-- !query 14 output
-- !query 15
-SELECT * FROM tab3
+SELECT k, udf(v) FROM tab3
EXCEPT ALL
-SELECT * FROM tab4
+SELECT udf(k), udf(v) FROM tab4
UNION ALL
-SELECT * FROM tab3
+SELECT udf(k), v FROM tab3
EXCEPT DISTINCT
-SELECT * FROM tab4
+SELECT k, udf(v) FROM tab4
-- !query 15 schema
-struct<k:int,v:int>
+struct<k:int,CAST(udf(cast(v as string)) AS INT):int>
-- !query 15 output
1 3
-217,83 +217,83 ExceptAll can only be performed on tables with the same number of columns, but t
-- !query 17
-SELECT * FROM tab3
+SELECT udf(k), udf(v) FROM tab3
EXCEPT ALL
-SELECT * FROM tab4
+SELECT udf(k), udf(v) FROM tab4
UNION
-SELECT * FROM tab3
+SELECT udf(k), udf(v) FROM tab3
EXCEPT DISTINCT
-SELECT * FROM tab4
+SELECT udf(k), udf(v) FROM tab4
-- !query 17 schema
-struct<k:int,v:int>
+struct<CAST(udf(cast(k as string)) AS INT):int,CAST(udf(cast(v as string)) AS INT):int>
-- !query 17 output
1 3
-- !query 18
-SELECT * FROM tab3
+SELECT udf(k), udf(v) FROM tab3
MINUS ALL
-SELECT * FROM tab4
+SELECT k, udf(v) FROM tab4
UNION
-SELECT * FROM tab3
+SELECT udf(k), udf(v) FROM tab3
MINUS DISTINCT
-SELECT * FROM tab4
+SELECT k, udf(v) FROM tab4
-- !query 18 schema
-struct<k:int,v:int>
+struct<CAST(udf(cast(k as string)) AS INT):int,CAST(udf(cast(v as string)) AS INT):int>
-- !query 18 output
1 3
-- !query 19
-SELECT * FROM tab3
+SELECT k, udf(v) FROM tab3
EXCEPT ALL
-SELECT * FROM tab4
+SELECT udf(k), v FROM tab4
EXCEPT DISTINCT
-SELECT * FROM tab3
+SELECT k, udf(v) FROM tab3
EXCEPT DISTINCT
-SELECT * FROM tab4
+SELECT udf(k), v FROM tab4
-- !query 19 schema
-struct<k:int,v:int>
+struct<k:int,CAST(udf(cast(v as string)) AS INT):int>
-- !query 19 output
-- !query 20
SELECT *
-FROM (SELECT tab3.k,
- tab4.v
+FROM (SELECT tab3.k,
+ udf(tab4.v)
FROM tab3
JOIN tab4
- ON tab3.k = tab4.k)
+ ON udf(tab3.k) = tab4.k)
EXCEPT ALL
SELECT *
-FROM (SELECT tab3.k,
- tab4.v
+FROM (SELECT udf(tab3.k),
+ tab4.v
FROM tab3
JOIN tab4
- ON tab3.k = tab4.k)
+ ON tab3.k = udf(tab4.k))
-- !query 20 schema
-struct<k:int,v:int>
+struct<k:int,CAST(udf(cast(v as string)) AS INT):int>
-- !query 20 output
-- !query 21
SELECT *
-FROM (SELECT tab3.k,
- tab4.v
+FROM (SELECT udf(udf(tab3.k)),
+ udf(tab4.v)
FROM tab3
JOIN tab4
- ON tab3.k = tab4.k)
+ ON udf(udf(tab3.k)) = udf(tab4.k))
EXCEPT ALL
SELECT *
-FROM (SELECT tab4.v AS k,
- tab3.k AS v
+FROM (SELECT udf(tab4.v) AS k,
+ udf(udf(tab3.k)) AS v
FROM tab3
JOIN tab4
- ON tab3.k = tab4.k)
+ ON udf(tab3.k) = udf(tab4.k))
-- !query 21 schema
-struct<k:int,v:int>
+struct<CAST(udf(cast(cast(udf(cast(k as string)) as int) as string)) AS INT):int,CAST(udf(cast(v as string)) AS INT):int>
-- !query 21 output
1 2
1 2
-305,11 +305,11 struct<k:int,v:int>
-- !query 22
-SELECT v FROM tab3 GROUP BY v
+SELECT udf(v) FROM tab3 GROUP BY v
EXCEPT ALL
-SELECT k FROM tab4 GROUP BY k
+SELECT udf(k) FROM tab4 GROUP BY k
-- !query 22 schema
-struct<v:int>
+struct<CAST(udf(cast(v as string)) AS INT):int>
-- !query 22 output
3
```
</p>
</details>
## How was this patch tested?
Tested as guided in [SPARK-27921](https://issues.apache.org/jira/browse/SPARK-27921).
Closes#25090 from imback82/except-all.
Authored-by: Terry Kim <yuminkim@gmail.com>
Signed-off-by: HyukjinKwon <gurwls223@apache.org>
## What changes were proposed in this pull request?
This PR adds some tests converted from `intersect-all.sql` to test UDFs. Please see contribution guide of this umbrella ticket - [SPARK-27921](https://issues.apache.org/jira/browse/SPARK-27921).
<details><summary>Diff comparing to 'intersect-all.sql'</summary>
<p>
```diff
diff --git a/sql/core/src/test/resources/sql-tests/results/intersect-all.sql.out b/sql/core/src/test/resources/sql-tests/results/udf/udf-intersect-all.sql.out
index 63dd56ce46..0cb82be2da 100644
--- a/sql/core/src/test/resources/sql-tests/results/intersect-all.sql.out
+++ b/sql/core/src/test/resources/sql-tests/results/udf/udf-intersect-all.sql.out
-34,11 +34,11 struct<>
-- !query 2
-SELECT * FROM tab1
+SELECT udf(k), v FROM tab1
INTERSECT ALL
-SELECT * FROM tab2
+SELECT k, udf(v) FROM tab2
-- !query 2 schema
-struct<k:int,v:int>
+struct<CAST(udf(cast(k as string)) AS INT):int,v:int>
-- !query 2 output
1 2
1 2
-48,11 +48,11 NULL NULL
-- !query 3
-SELECT * FROM tab1
+SELECT k, udf(v) FROM tab1
INTERSECT ALL
-SELECT * FROM tab1 WHERE k = 1
+SELECT udf(k), v FROM tab1 WHERE udf(k) = 1
-- !query 3 schema
-struct<k:int,v:int>
+struct<k:int,CAST(udf(cast(v as string)) AS INT):int>
-- !query 3 output
1 2
1 2
-61,39 +61,39 struct<k:int,v:int>
-- !query 4
-SELECT * FROM tab1 WHERE k > 2
+SELECT udf(k), udf(v) FROM tab1 WHERE k > udf(2)
INTERSECT ALL
-SELECT * FROM tab2
+SELECT udf(k), udf(v) FROM tab2
-- !query 4 schema
-struct<k:int,v:int>
+struct<CAST(udf(cast(k as string)) AS INT):int,CAST(udf(cast(v as string)) AS INT):int>
-- !query 4 output
-- !query 5
-SELECT * FROM tab1
+SELECT udf(k), v FROM tab1
INTERSECT ALL
-SELECT * FROM tab2 WHERE k > 3
+SELECT udf(k), v FROM tab2 WHERE udf(udf(k)) > 3
-- !query 5 schema
-struct<k:int,v:int>
+struct<CAST(udf(cast(k as string)) AS INT):int,v:int>
-- !query 5 output
-- !query 6
-SELECT * FROM tab1
+SELECT udf(k), v FROM tab1
INTERSECT ALL
-SELECT CAST(1 AS BIGINT), CAST(2 AS BIGINT)
+SELECT CAST(udf(1) AS BIGINT), CAST(udf(2) AS BIGINT)
-- !query 6 schema
-struct<k:bigint,v:bigint>
+struct<CAST(udf(cast(k as string)) AS INT):bigint,v:bigint>
-- !query 6 output
1 2
-- !query 7
-SELECT * FROM tab1
+SELECT k, udf(v) FROM tab1
INTERSECT ALL
-SELECT array(1), 2
+SELECT array(1), udf(2)
-- !query 7 schema
struct<>
-- !query 7 output
-102,9 +102,9 IntersectAll can only be performed on tables with the compatible column types. a
-- !query 8
-SELECT k FROM tab1
+SELECT udf(k) FROM tab1
INTERSECT ALL
-SELECT k, v FROM tab2
+SELECT udf(k), udf(v) FROM tab2
-- !query 8 schema
struct<>
-- !query 8 output
-113,13 +113,13 IntersectAll can only be performed on tables with the same number of columns, bu
-- !query 9
-SELECT * FROM tab2
+SELECT udf(k), v FROM tab2
INTERSECT ALL
-SELECT * FROM tab1
+SELECT k, udf(v) FROM tab1
INTERSECT ALL
-SELECT * FROM tab2
+SELECT udf(k), udf(v) FROM tab2
-- !query 9 schema
-struct<k:int,v:int>
+struct<CAST(udf(cast(k as string)) AS INT):int,v:int>
-- !query 9 output
1 2
1 2
-129,15 +129,15 NULL NULL
-- !query 10
-SELECT * FROM tab1
+SELECT udf(k), v FROM tab1
EXCEPT
-SELECT * FROM tab2
+SELECT k, udf(v) FROM tab2
UNION ALL
-SELECT * FROM tab1
+SELECT k, udf(udf(v)) FROM tab1
INTERSECT ALL
-SELECT * FROM tab2
+SELECT udf(k), v FROM tab2
-- !query 10 schema
-struct<k:int,v:int>
+struct<CAST(udf(cast(k as string)) AS INT):int,v:int>
-- !query 10 output
1 2
1 2
-148,15 +148,15 NULL NULL
-- !query 11
-SELECT * FROM tab1
+SELECT udf(k), udf(v) FROM tab1
EXCEPT
-SELECT * FROM tab2
+SELECT udf(k), v FROM tab2
EXCEPT
-SELECT * FROM tab1
+SELECT k, udf(v) FROM tab1
INTERSECT ALL
-SELECT * FROM tab2
+SELECT udf(k), udf(udf(v)) FROM tab2
-- !query 11 schema
-struct<k:int,v:int>
+struct<CAST(udf(cast(k as string)) AS INT):int,CAST(udf(cast(v as string)) AS INT):int>
-- !query 11 output
1 3
-165,38 +165,38 struct<k:int,v:int>
(
(
(
- SELECT * FROM tab1
+ SELECT udf(k), v FROM tab1
EXCEPT
- SELECT * FROM tab2
+ SELECT k, udf(v) FROM tab2
)
EXCEPT
- SELECT * FROM tab1
+ SELECT udf(k), udf(v) FROM tab1
)
INTERSECT ALL
- SELECT * FROM tab2
+ SELECT udf(k), udf(v) FROM tab2
)
-- !query 12 schema
-struct<k:int,v:int>
+struct<CAST(udf(cast(k as string)) AS INT):int,v:int>
-- !query 12 output
-- !query 13
SELECT *
-FROM (SELECT tab1.k,
- tab2.v
+FROM (SELECT udf(tab1.k),
+ udf(tab2.v)
FROM tab1
JOIN tab2
- ON tab1.k = tab2.k)
+ ON udf(udf(tab1.k)) = tab2.k)
INTERSECT ALL
SELECT *
-FROM (SELECT tab1.k,
- tab2.v
+FROM (SELECT udf(tab1.k),
+ udf(tab2.v)
FROM tab1
JOIN tab2
- ON tab1.k = tab2.k)
+ ON udf(tab1.k) = udf(udf(tab2.k)))
-- !query 13 schema
-struct<k:int,v:int>
+struct<CAST(udf(cast(k as string)) AS INT):int,CAST(udf(cast(v as string)) AS INT):int>
-- !query 13 output
1 2
1 2
-211,30 +211,30 struct<k:int,v:int>
-- !query 14
SELECT *
-FROM (SELECT tab1.k,
- tab2.v
+FROM (SELECT udf(tab1.k),
+ udf(tab2.v)
FROM tab1
JOIN tab2
- ON tab1.k = tab2.k)
+ ON udf(tab1.k) = udf(tab2.k))
INTERSECT ALL
SELECT *
-FROM (SELECT tab2.v AS k,
- tab1.k AS v
+FROM (SELECT udf(tab2.v) AS k,
+ udf(tab1.k) AS v
FROM tab1
JOIN tab2
- ON tab1.k = tab2.k)
+ ON tab1.k = udf(tab2.k))
-- !query 14 schema
-struct<k:int,v:int>
+struct<CAST(udf(cast(k as string)) AS INT):int,CAST(udf(cast(v as string)) AS INT):int>
-- !query 14 output
-- !query 15
-SELECT v FROM tab1 GROUP BY v
+SELECT udf(v) FROM tab1 GROUP BY v
INTERSECT ALL
-SELECT k FROM tab2 GROUP BY k
+SELECT udf(udf(k)) FROM tab2 GROUP BY k
-- !query 15 schema
-struct<v:int>
+struct<CAST(udf(cast(v as string)) AS INT):int>
-- !query 15 output
2
3
-250,15 +250,15 spark.sql.legacy.setopsPrecedence.enabled true
-- !query 17
-SELECT * FROM tab1
+SELECT udf(k), v FROM tab1
EXCEPT
-SELECT * FROM tab2
+SELECT k, udf(v) FROM tab2
UNION ALL
-SELECT * FROM tab1
+SELECT udf(k), udf(v) FROM tab1
INTERSECT ALL
-SELECT * FROM tab2
+SELECT udf(udf(k)), udf(v) FROM tab2
-- !query 17 schema
-struct<k:int,v:int>
+struct<CAST(udf(cast(k as string)) AS INT):int,v:int>
-- !query 17 output
1 2
1 2
-268,15 +268,15 NULL NULL
-- !query 18
-SELECT * FROM tab1
+SELECT k, udf(v) FROM tab1
EXCEPT
-SELECT * FROM tab2
+SELECT udf(k), v FROM tab2
UNION ALL
-SELECT * FROM tab1
+SELECT udf(k), udf(v) FROM tab1
INTERSECT
-SELECT * FROM tab2
+SELECT udf(k), udf(udf(v)) FROM tab2
-- !query 18 schema
-struct<k:int,v:int>
+struct<k:int,CAST(udf(cast(v as string)) AS INT):int>
-- !query 18 output
1 2
2 3
```
</p>
</details>
## How was this patch tested?
Tested as guided in [SPARK-27921](https://issues.apache.org/jira/browse/SPARK-27921).
Closes#25119 from imback82/intersect-all-sql.
Authored-by: Terry Kim <yuminkim@gmail.com>
Signed-off-by: HyukjinKwon <gurwls223@apache.org>
## What changes were proposed in this pull request?
This PR adds some tests converted from `cross-join.sql'` to test UDFs.
<details><summary>Diff comparing to 'cross-join.sql'</summary>
<p>
```diff
diff --git a/sql/core/src/test/resources/sql-tests/results/cross-join.sql.out b/sql/core/src/test/resources/sql-tests/results/udf/udf-cross-join.sql.out
index 3833c42bdf..11c1e01d54 100644
--- a/sql/core/src/test/resources/sql-tests/results/cross-join.sql.out
+++ b/sql/core/src/test/resources/sql-tests/results/udf/udf-cross-join.sql.out
-43,7 +43,7 two 2 two 22
-- !query 3
-SELECT * FROM nt1 cross join nt2 where nt1.k = nt2.k
+SELECT * FROM nt1 cross join nt2 where udf(nt1.k) = udf(nt2.k)
-- !query 3 schema
struct<k:string,v1:int,k:string,v2:int>
-- !query 3 output
-53,7 +53,7 two 2 two 22
-- !query 4
-SELECT * FROM nt1 cross join nt2 on (nt1.k = nt2.k)
+SELECT * FROM nt1 cross join nt2 on (udf(nt1.k) = udf(nt2.k))
-- !query 4 schema
struct<k:string,v1:int,k:string,v2:int>
-- !query 4 output
-63,7 +63,7 two 2 two 22
-- !query 5
-SELECT * FROM nt1 cross join nt2 where nt1.v1 = 1 and nt2.v2 = 22
+SELECT * FROM nt1 cross join nt2 where udf(nt1.v1) = "1" and udf(nt2.v2) = "22"
-- !query 5 schema
struct<k:string,v1:int,k:string,v2:int>
-- !query 5 output
-71,12 +71,12 one 1 two 22
-- !query 6
-SELECT a.key, b.key FROM
-(SELECT k key FROM nt1 WHERE v1 < 2) a
+SELECT udf(a.key), udf(b.key) FROM
+(SELECT udf(k) key FROM nt1 WHERE v1 < 2) a
CROSS JOIN
-(SELECT k key FROM nt2 WHERE v2 = 22) b
+(SELECT udf(k) key FROM nt2 WHERE v2 = 22) b
-- !query 6 schema
-struct<key:string,key:string>
+struct<udf(key):string,udf(key):string>
-- !query 6 output
one two
-114,23 +114,29 struct<>
-- !query 11
-select * from ((A join B on (a = b)) cross join C) join D on (a = d)
+select * from ((A join B on (udf(a) = udf(b))) cross join C) join D on (udf(a) = udf(d))
-- !query 11 schema
-struct<a:string,va:int,b:string,vb:int,c:string,vc:int,d:string,vd:int>
+struct<>
-- !query 11 output
-one 1 one 1 one 1 one 1
-one 1 one 1 three 3 one 1
-one 1 one 1 two 2 one 1
-three 3 three 3 one 1 three 3
-three 3 three 3 three 3 three 3
-three 3 three 3 two 2 three 3
-two 2 two 2 one 1 two 2
-two 2 two 2 three 3 two 2
-two 2 two 2 two 2 two 2
+org.apache.spark.sql.AnalysisException
+Detected implicit cartesian product for INNER join between logical plans
+Filter (udf(a#x) = udf(b#x))
++- Join Inner
+ :- Project [k#x AS a#x, v1#x AS va#x]
+ : +- LocalRelation [k#x, v1#x]
+ +- Project [k#x AS b#x, v1#x AS vb#x]
+ +- LocalRelation [k#x, v1#x]
+and
+Project [k#x AS d#x, v1#x AS vd#x]
++- LocalRelation [k#x, v1#x]
+Join condition is missing or trivial.
+Either: use the CROSS JOIN syntax to allow cartesian products between these
+relations, or: enable implicit cartesian products by setting the configuration
+variable spark.sql.crossJoin.enabled=true;
-- !query 12
-SELECT * FROM nt1 CROSS JOIN nt2 ON (nt1.k > nt2.k)
+SELECT * FROM nt1 CROSS JOIN nt2 ON (udf(nt1.k) > udf(nt2.k))
-- !query 12 schema
struct<k:string,v1:int,k:string,v2:int>
-- !query 12 output
```
</p>
</details>
## How was this patch tested?
Added test.
Closes#25168 from viirya/SPARK-28276.
Authored-by: Liang-Chi Hsieh <viirya@gmail.com>
Signed-off-by: HyukjinKwon <gurwls223@apache.org>
## What changes were proposed in this pull request?
PostgreSQL doesn't have `TINYINT`, which would map directly, but `SMALLINT`s are sufficient for uni-directional translation.
A side-effect of this fix is that `AggregatedDialect` is now usable with multiple dialects targeting `jdbc:postgresql`, as `PostgresDialect.getJDBCType` no longer throws (for which reason backporting this fix would be lovely):
1217996f15/sql/core/src/main/scala/org/apache/spark/sql/jdbc/AggregatedDialect.scala (L42)
`dialects.flatMap` currently throws on the first attempt to get a JDBC type preventing subsequent dialects in the chain from providing an alternative.
## How was this patch tested?
Unit tests.
Closes#24845 from mojodna/postgres-byte-type-mapping.
Authored-by: Seth Fitzsimmons <seth@mojodna.net>
Signed-off-by: Dongjoon Hyun <dhyun@apple.com>
## What changes were proposed in this pull request?
Current UDFs available in `IntegratedUDFTestUtils` are not exactly no-op. It converts input column to strings and outputs to strings.
It causes some issues when we convert and port the tests at SPARK-27921. Integrated UDF test cases share one output file and it should outputs the same. However,
1. Special values are converted into strings differently:
| Scala | Python |
| ---------- | ------ |
| `null` | `None` |
| `Infinity` | `inf` |
| `-Infinity`| `-inf` |
| `NaN` | `nan` |
2. Due to float limitation at Python (see https://docs.python.org/3/tutorial/floatingpoint.html), if float is passed into Python and sent back to JVM, the values are potentially not exactly correct. See https://github.com/apache/spark/pull/25128 and https://github.com/apache/spark/pull/25110
To work around this, this PR targets to change the current UDF to be wrapped by cast. So, Input column is casted into string, UDF returns strings as are, and then output column is casted back to the input column.
Roughly:
**Before:**
```
JVM (col1) -> (cast to string within Python) Python (string) -> (string) JVM
```
**After:**
```
JVM (cast col1 to string) -> (string) Python (string) -> (cast back to col1's type) JVM
```
In this way, UDF is virtually no-op although there might be some subtleties due to roundtrip in string cast. I believe this is good enough.
Python native functions and Scala native functions will take strings and output strings as are. So, there will be no potential test failures due to differences of conversion between Python and Scala.
After this fix, for instance, `udf-aggregates_part1.sql` outputs exactly same as `aggregates_part1.sql`:
<details><summary>Diff comparing to 'pgSQL/aggregates_part1.sql'</summary>
<p>
```diff
diff --git a/sql/core/src/test/resources/sql-tests/results/pgSQL/aggregates_part1.sql.out b/sql/core/src/test/resources/sql-tests/results/udf/pgSQL/udf-aggregates_part1.sql.out
index 51ca1d55869..801735781c7 100644
--- a/sql/core/src/test/resources/sql-tests/results/pgSQL/aggregates_part1.sql.out
+++ b/sql/core/src/test/resources/sql-tests/results/udf/pgSQL/udf-aggregates_part1.sql.out
-3,7 +3,7
-- !query 0
-SELECT avg(four) AS avg_1 FROM onek
+SELECT avg(udf(four)) AS avg_1 FROM onek
-- !query 0 schema
struct<avg_1:double>
-- !query 0 output
-11,7 +11,7 struct<avg_1:double>
-- !query 1
-SELECT avg(a) AS avg_32 FROM aggtest WHERE a < 100
+SELECT udf(avg(a)) AS avg_32 FROM aggtest WHERE a < 100
-- !query 1 schema
struct<avg_32:double>
-- !query 1 output
-19,7 +19,7 struct<avg_32:double>
-- !query 2
-select CAST(avg(b) AS Decimal(10,3)) AS avg_107_943 FROM aggtest
+select CAST(avg(udf(b)) AS Decimal(10,3)) AS avg_107_943 FROM aggtest
-- !query 2 schema
struct<avg_107_943:decimal(10,3)>
-- !query 2 output
-27,7 +27,7 struct<avg_107_943:decimal(10,3)>
-- !query 3
-SELECT sum(four) AS sum_1500 FROM onek
+SELECT sum(udf(four)) AS sum_1500 FROM onek
-- !query 3 schema
struct<sum_1500:bigint>
-- !query 3 output
-35,7 +35,7 struct<sum_1500:bigint>
-- !query 4
-SELECT sum(a) AS sum_198 FROM aggtest
+SELECT udf(sum(a)) AS sum_198 FROM aggtest
-- !query 4 schema
struct<sum_198:bigint>
-- !query 4 output
-43,7 +43,7 struct<sum_198:bigint>
-- !query 5
-SELECT sum(b) AS avg_431_773 FROM aggtest
+SELECT udf(udf(sum(b))) AS avg_431_773 FROM aggtest
-- !query 5 schema
struct<avg_431_773:double>
-- !query 5 output
-51,7 +51,7 struct<avg_431_773:double>
-- !query 6
-SELECT max(four) AS max_3 FROM onek
+SELECT udf(max(four)) AS max_3 FROM onek
-- !query 6 schema
struct<max_3:int>
-- !query 6 output
-59,7 +59,7 struct<max_3:int>
-- !query 7
-SELECT max(a) AS max_100 FROM aggtest
+SELECT max(udf(a)) AS max_100 FROM aggtest
-- !query 7 schema
struct<max_100:int>
-- !query 7 output
-67,7 +67,7 struct<max_100:int>
-- !query 8
-SELECT max(aggtest.b) AS max_324_78 FROM aggtest
+SELECT udf(udf(max(aggtest.b))) AS max_324_78 FROM aggtest
-- !query 8 schema
struct<max_324_78:float>
-- !query 8 output
-75,237 +75,238 struct<max_324_78:float>
-- !query 9
-SELECT stddev_pop(b) FROM aggtest
+SELECT stddev_pop(udf(b)) FROM aggtest
-- !query 9 schema
-struct<stddev_pop(CAST(b AS DOUBLE)):double>
+struct<stddev_pop(CAST(CAST(udf(cast(b as string)) AS FLOAT) AS DOUBLE)):double>
-- !query 9 output
131.10703231895047
-- !query 10
-SELECT stddev_samp(b) FROM aggtest
+SELECT udf(stddev_samp(b)) FROM aggtest
-- !query 10 schema
-struct<stddev_samp(CAST(b AS DOUBLE)):double>
+struct<CAST(udf(cast(stddev_samp(cast(b as double)) as string)) AS DOUBLE):double>
-- !query 10 output
151.38936080399804
-- !query 11
-SELECT var_pop(b) FROM aggtest
+SELECT var_pop(udf(b)) FROM aggtest
-- !query 11 schema
-struct<var_pop(CAST(b AS DOUBLE)):double>
+struct<var_pop(CAST(CAST(udf(cast(b as string)) AS FLOAT) AS DOUBLE)):double>
-- !query 11 output
17189.053923482323
-- !query 12
-SELECT var_samp(b) FROM aggtest
+SELECT udf(var_samp(b)) FROM aggtest
-- !query 12 schema
-struct<var_samp(CAST(b AS DOUBLE)):double>
+struct<CAST(udf(cast(var_samp(cast(b as double)) as string)) AS DOUBLE):double>
-- !query 12 output
22918.738564643096
-- !query 13
-SELECT stddev_pop(CAST(b AS Decimal(38,0))) FROM aggtest
+SELECT udf(stddev_pop(CAST(b AS Decimal(38,0)))) FROM aggtest
-- !query 13 schema
-struct<stddev_pop(CAST(CAST(b AS DECIMAL(38,0)) AS DOUBLE)):double>
+struct<CAST(udf(cast(stddev_pop(cast(cast(b as decimal(38,0)) as double)) as string)) AS DOUBLE):double>
-- !query 13 output
131.18117242958306
-- !query 14
-SELECT stddev_samp(CAST(b AS Decimal(38,0))) FROM aggtest
+SELECT stddev_samp(CAST(udf(b) AS Decimal(38,0))) FROM aggtest
-- !query 14 schema
-struct<stddev_samp(CAST(CAST(b AS DECIMAL(38,0)) AS DOUBLE)):double>
+struct<stddev_samp(CAST(CAST(CAST(udf(cast(b as string)) AS FLOAT) AS DECIMAL(38,0)) AS DOUBLE)):double>
-- !query 14 output
151.47497042966097
-- !query 15
-SELECT var_pop(CAST(b AS Decimal(38,0))) FROM aggtest
+SELECT udf(var_pop(CAST(b AS Decimal(38,0)))) FROM aggtest
-- !query 15 schema
-struct<var_pop(CAST(CAST(b AS DECIMAL(38,0)) AS DOUBLE)):double>
+struct<CAST(udf(cast(var_pop(cast(cast(b as decimal(38,0)) as double)) as string)) AS DOUBLE):double>
-- !query 15 output
17208.5
-- !query 16
-SELECT var_samp(CAST(b AS Decimal(38,0))) FROM aggtest
+SELECT var_samp(udf(CAST(b AS Decimal(38,0)))) FROM aggtest
-- !query 16 schema
-struct<var_samp(CAST(CAST(b AS DECIMAL(38,0)) AS DOUBLE)):double>
+struct<var_samp(CAST(CAST(udf(cast(cast(b as decimal(38,0)) as string)) AS DECIMAL(38,0)) AS DOUBLE)):double>
-- !query 16 output
22944.666666666668
-- !query 17
-SELECT var_pop(1.0), var_samp(2.0)
+SELECT udf(var_pop(1.0)), var_samp(udf(2.0))
-- !query 17 schema
-struct<var_pop(CAST(1.0 AS DOUBLE)):double,var_samp(CAST(2.0 AS DOUBLE)):double>
+struct<CAST(udf(cast(var_pop(cast(1.0 as double)) as string)) AS DOUBLE):double,var_samp(CAST(CAST(udf(cast(2.0 as string)) AS DECIMAL(2,1)) AS DOUBLE)):double>
-- !query 17 output
0.0 NaN
-- !query 18
-SELECT stddev_pop(CAST(3.0 AS Decimal(38,0))), stddev_samp(CAST(4.0 AS Decimal(38,0)))
+SELECT stddev_pop(udf(CAST(3.0 AS Decimal(38,0)))), stddev_samp(CAST(udf(4.0) AS Decimal(38,0)))
-- !query 18 schema
-struct<stddev_pop(CAST(CAST(3.0 AS DECIMAL(38,0)) AS DOUBLE)):double,stddev_samp(CAST(CAST(4.0 AS DECIMAL(38,0)) AS DOUBLE)):double>
+struct<stddev_pop(CAST(CAST(udf(cast(cast(3.0 as decimal(38,0)) as string)) AS DECIMAL(38,0)) AS DOUBLE)):double,stddev_samp(CAST(CAST(CAST(udf(cast(4.0 as string)) AS DECIMAL(2,1)) AS DECIMAL(38,0)) AS DOUBLE)):double>
-- !query 18 output
0.0 NaN
-- !query 19
-select sum(CAST(null AS int)) from range(1,4)
+select sum(udf(CAST(null AS int))) from range(1,4)
-- !query 19 schema
-struct<sum(CAST(NULL AS INT)):bigint>
+struct<sum(CAST(udf(cast(cast(null as int) as string)) AS INT)):bigint>
-- !query 19 output
NULL
-- !query 20
-select sum(CAST(null AS long)) from range(1,4)
+select sum(udf(CAST(null AS long))) from range(1,4)
-- !query 20 schema
-struct<sum(CAST(NULL AS BIGINT)):bigint>
+struct<sum(CAST(udf(cast(cast(null as bigint) as string)) AS BIGINT)):bigint>
-- !query 20 output
NULL
-- !query 21
-select sum(CAST(null AS Decimal(38,0))) from range(1,4)
+select sum(udf(CAST(null AS Decimal(38,0)))) from range(1,4)
-- !query 21 schema
-struct<sum(CAST(NULL AS DECIMAL(38,0))):decimal(38,0)>
+struct<sum(CAST(udf(cast(cast(null as decimal(38,0)) as string)) AS DECIMAL(38,0))):decimal(38,0)>
-- !query 21 output
NULL
-- !query 22
-select sum(CAST(null AS DOUBLE)) from range(1,4)
+select sum(udf(CAST(null AS DOUBLE))) from range(1,4)
-- !query 22 schema
-struct<sum(CAST(NULL AS DOUBLE)):double>
+struct<sum(CAST(udf(cast(cast(null as double) as string)) AS DOUBLE)):double>
-- !query 22 output
NULL
-- !query 23
-select avg(CAST(null AS int)) from range(1,4)
+select avg(udf(CAST(null AS int))) from range(1,4)
-- !query 23 schema
-struct<avg(CAST(NULL AS INT)):double>
+struct<avg(CAST(udf(cast(cast(null as int) as string)) AS INT)):double>
-- !query 23 output
NULL
-- !query 24
-select avg(CAST(null AS long)) from range(1,4)
+select avg(udf(CAST(null AS long))) from range(1,4)
-- !query 24 schema
-struct<avg(CAST(NULL AS BIGINT)):double>
+struct<avg(CAST(udf(cast(cast(null as bigint) as string)) AS BIGINT)):double>
-- !query 24 output
NULL
-- !query 25
-select avg(CAST(null AS Decimal(38,0))) from range(1,4)
+select avg(udf(CAST(null AS Decimal(38,0)))) from range(1,4)
-- !query 25 schema
-struct<avg(CAST(NULL AS DECIMAL(38,0))):decimal(38,4)>
+struct<avg(CAST(udf(cast(cast(null as decimal(38,0)) as string)) AS DECIMAL(38,0))):decimal(38,4)>
-- !query 25 output
NULL
-- !query 26
-select avg(CAST(null AS DOUBLE)) from range(1,4)
+select avg(udf(CAST(null AS DOUBLE))) from range(1,4)
-- !query 26 schema
-struct<avg(CAST(NULL AS DOUBLE)):double>
+struct<avg(CAST(udf(cast(cast(null as double) as string)) AS DOUBLE)):double>
-- !query 26 output
NULL
-- !query 27
-select sum(CAST('NaN' AS DOUBLE)) from range(1,4)
+select sum(CAST(udf('NaN') AS DOUBLE)) from range(1,4)
-- !query 27 schema
-struct<sum(CAST(NaN AS DOUBLE)):double>
+struct<sum(CAST(CAST(udf(cast(NaN as string)) AS STRING) AS DOUBLE)):double>
-- !query 27 output
NaN
-- !query 28
-select avg(CAST('NaN' AS DOUBLE)) from range(1,4)
+select avg(CAST(udf('NaN') AS DOUBLE)) from range(1,4)
-- !query 28 schema
-struct<avg(CAST(NaN AS DOUBLE)):double>
+struct<avg(CAST(CAST(udf(cast(NaN as string)) AS STRING) AS DOUBLE)):double>
-- !query 28 output
NaN
-- !query 30
-SELECT avg(CAST(x AS DOUBLE)), var_pop(CAST(x AS DOUBLE))
+SELECT avg(CAST(udf(x) AS DOUBLE)), var_pop(CAST(udf(x) AS DOUBLE))
FROM (VALUES ('Infinity'), ('1')) v(x)
-- !query 30 schema
-struct<avg(CAST(x AS DOUBLE)):double,var_pop(CAST(x AS DOUBLE)):double>
+struct<avg(CAST(CAST(udf(cast(x as string)) AS STRING) AS DOUBLE)):double,var_pop(CAST(CAST(udf(cast(x as string)) AS STRING) AS DOUBLE)):double>
-- !query 30 output
Infinity NaN
-- !query 31
-SELECT avg(CAST(x AS DOUBLE)), var_pop(CAST(x AS DOUBLE))
+SELECT avg(CAST(udf(x) AS DOUBLE)), var_pop(CAST(udf(x) AS DOUBLE))
FROM (VALUES ('Infinity'), ('Infinity')) v(x)
-- !query 31 schema
-struct<avg(CAST(x AS DOUBLE)):double,var_pop(CAST(x AS DOUBLE)):double>
+struct<avg(CAST(CAST(udf(cast(x as string)) AS STRING) AS DOUBLE)):double,var_pop(CAST(CAST(udf(cast(x as string)) AS STRING) AS DOUBLE)):double>
-- !query 31 output
Infinity NaN
-- !query 32
-SELECT avg(CAST(x AS DOUBLE)), var_pop(CAST(x AS DOUBLE))
+SELECT avg(CAST(udf(x) AS DOUBLE)), var_pop(CAST(udf(x) AS DOUBLE))
FROM (VALUES ('-Infinity'), ('Infinity')) v(x)
-- !query 32 schema
-struct<avg(CAST(x AS DOUBLE)):double,var_pop(CAST(x AS DOUBLE)):double>
+struct<avg(CAST(CAST(udf(cast(x as string)) AS STRING) AS DOUBLE)):double,var_pop(CAST(CAST(udf(cast(x as string)) AS STRING) AS DOUBLE)):double>
-- !query 32 output
NaN NaN
-- !query 33
-SELECT avg(CAST(x AS DOUBLE)), var_pop(CAST(x AS DOUBLE))
+SELECT avg(udf(CAST(x AS DOUBLE))), udf(var_pop(CAST(x AS DOUBLE)))
FROM (VALUES (100000003), (100000004), (100000006), (100000007)) v(x)
-- !query 33 schema
-struct<avg(CAST(x AS DOUBLE)):double,var_pop(CAST(x AS DOUBLE)):double>
+struct<avg(CAST(udf(cast(cast(x as double) as string)) AS DOUBLE)):double,CAST(udf(cast(var_pop(cast(x as double)) as string)) AS DOUBLE):double>
-- !query 33 output
1.00000005E8 2.5
-- !query 34
-SELECT avg(CAST(x AS DOUBLE)), var_pop(CAST(x AS DOUBLE))
+SELECT avg(udf(CAST(x AS DOUBLE))), udf(var_pop(CAST(x AS DOUBLE)))
FROM (VALUES (7000000000005), (7000000000007)) v(x)
-- !query 34 schema
-struct<avg(CAST(x AS DOUBLE)):double,var_pop(CAST(x AS DOUBLE)):double>
+struct<avg(CAST(udf(cast(cast(x as double) as string)) AS DOUBLE)):double,CAST(udf(cast(var_pop(cast(x as double)) as string)) AS DOUBLE):double>
-- !query 34 output
7.000000000006E12 1.0
-- !query 35
-SELECT covar_pop(b, a), covar_samp(b, a) FROM aggtest
+SELECT udf(covar_pop(b, udf(a))), covar_samp(udf(b), a) FROM aggtest
-- !query 35 schema
-struct<covar_pop(CAST(b AS DOUBLE), CAST(a AS DOUBLE)):double,covar_samp(CAST(b AS DOUBLE), CAST(a AS DOUBLE)):double>
+struct<CAST(udf(cast(covar_pop(cast(b as double), cast(cast(udf(cast(a as string)) as int) as double)) as string)) AS DOUBLE):double,covar_samp(CAST(CAST(udf(cast(b as string)) AS FLOAT) AS DOUBLE), CAST(a AS DOUBLE)):double>
-- !query 35 output
653.6289553875104 871.5052738500139
-- !query 36
-SELECT corr(b, a) FROM aggtest
+SELECT corr(b, udf(a)) FROM aggtest
-- !query 36 schema
-struct<corr(CAST(b AS DOUBLE), CAST(a AS DOUBLE)):double>
+struct<corr(CAST(b AS DOUBLE), CAST(CAST(udf(cast(a as string)) AS INT) AS DOUBLE)):double>
-- !query 36 output
0.1396345165178734
-- !query 37
-SELECT count(four) AS cnt_1000 FROM onek
+SELECT count(udf(four)) AS cnt_1000 FROM onek
-- !query 37 schema
struct<cnt_1000:bigint>
-- !query 37 output
-313,7 +314,7 struct<cnt_1000:bigint>
-- !query 38
-SELECT count(DISTINCT four) AS cnt_4 FROM onek
+SELECT udf(count(DISTINCT four)) AS cnt_4 FROM onek
-- !query 38 schema
struct<cnt_4:bigint>
-- !query 38 output
-321,10 +322,10 struct<cnt_4:bigint>
-- !query 39
-select ten, count(*), sum(four) from onek
+select ten, udf(count(*)), sum(udf(four)) from onek
group by ten order by ten
-- !query 39 schema
-struct<ten:int,count(1):bigint,sum(four):bigint>
+struct<ten:int,CAST(udf(cast(count(1) as string)) AS BIGINT):bigint,sum(CAST(udf(cast(four as string)) AS INT)):bigint>
-- !query 39 output
0 100 100
1 100 200
-339,10 +340,10 struct<ten:int,count(1):bigint,sum(four):bigint>
-- !query 40
-select ten, count(four), sum(DISTINCT four) from onek
+select ten, count(udf(four)), udf(sum(DISTINCT four)) from onek
group by ten order by ten
-- !query 40 schema
-struct<ten:int,count(four):bigint,sum(DISTINCT four):bigint>
+struct<ten:int,count(CAST(udf(cast(four as string)) AS INT)):bigint,CAST(udf(cast(sum(distinct cast(four as bigint)) as string)) AS BIGINT):bigint>
-- !query 40 output
0 100 2
1 100 4
-357,11 +358,11 struct<ten:int,count(four):bigint,sum(DISTINCT four):bigint>
-- !query 41
-select ten, sum(distinct four) from onek a
+select ten, udf(sum(distinct four)) from onek a
group by ten
-having exists (select 1 from onek b where sum(distinct a.four) = b.four)
+having exists (select 1 from onek b where udf(sum(distinct a.four)) = b.four)
-- !query 41 schema
-struct<ten:int,sum(DISTINCT four):bigint>
+struct<ten:int,CAST(udf(cast(sum(distinct cast(four as bigint)) as string)) AS BIGINT):bigint>
-- !query 41 output
0 2
2 2
-374,23 +375,23 struct<ten:int,sum(DISTINCT four):bigint>
select ten, sum(distinct four) from onek a
group by ten
having exists (select 1 from onek b
- where sum(distinct a.four + b.four) = b.four)
+ where sum(distinct a.four + b.four) = udf(b.four))
-- !query 42 schema
struct<>
-- !query 42 output
org.apache.spark.sql.AnalysisException
Aggregate/Window/Generate expressions are not valid in where clause of the query.
-Expression in where clause: [(sum(DISTINCT CAST((outer() + b.`four`) AS BIGINT)) = CAST(b.`four` AS BIGINT))]
+Expression in where clause: [(sum(DISTINCT CAST((outer() + b.`four`) AS BIGINT)) = CAST(CAST(udf(cast(four as string)) AS INT) AS BIGINT))]
Invalid expressions: [sum(DISTINCT CAST((outer() + b.`four`) AS BIGINT))];
-- !query 43
select
- (select max((select i.unique2 from tenk1 i where i.unique1 = o.unique1)))
+ (select udf(max((select i.unique2 from tenk1 i where i.unique1 = o.unique1))))
from tenk1 o
-- !query 43 schema
struct<>
-- !query 43 output
org.apache.spark.sql.AnalysisException
-cannot resolve '`o.unique1`' given input columns: [i.even, i.fivethous, i.four, i.hundred, i.odd, i.string4, i.stringu1, i.stringu2, i.ten, i.tenthous, i.thousand, i.twenty, i.two, i.twothousand, i.unique1, i.unique2]; line 2 pos 63
+cannot resolve '`o.unique1`' given input columns: [i.even, i.fivethous, i.four, i.hundred, i.odd, i.string4, i.stringu1, i.stringu2, i.ten, i.tenthous, i.thousand, i.twenty, i.two, i.twothousand, i.unique1, i.unique2]; line 2 pos 67
```
</p>
</details>
## How was this patch tested?
Manually tested.
Closes#25130 from HyukjinKwon/SPARK-28359.
Authored-by: HyukjinKwon <gurwls223@apache.org>
Signed-off-by: Wenchen Fan <wenchen@databricks.com>
## What changes were proposed in this pull request?
Add 4 additional agg to KeyValueGroupedDataset
## How was this patch tested?
New test in DatasetSuite for typed aggregation
Closes#24993 from nooberfsh/sqlagg.
Authored-by: nooberfsh <nooberfsh@gmail.com>
Signed-off-by: Dongjoon Hyun <dhyun@apple.com>
## What changes were proposed in this pull request?
This PR enables `spark.sql.function.preferIntegralDivision` for PostgreSQL testing.
## How was this patch tested?
N/A
Closes#25170 from wangyum/SPARK-28343-2.
Authored-by: Yuming Wang <yumwang@ebay.com>
Signed-off-by: Dongjoon Hyun <dhyun@apple.com>
## What changes were proposed in this pull request?
PR builder failed with the following error:
```
[error] /home/jenkins/workspace/SparkPullRequestBuilder/sql/core/src/test/scala/org/apache/spark/sql/execution/PlannerSuite.scala:714: wrong number of arguments for pattern org.apache.spark.sql.execution.exchange.ShuffleExchangeExec(outputPartitioning: org.apache.spark.sql.catalyst.plans.physical.Partitioning,child: org.apache.spark.sql.execution.SparkPlan,canChangeNumPartitions: Boolean)
[error] ShuffleExchangeExec(HashPartitioning(leftPartitioningExpressions, _), _), _),
[error] ^
[error] /home/jenkins/workspace/SparkPullRequestBuilder/sql/core/src/test/scala/org/apache/spark/sql/execution/PlannerSuite.scala:716: wrong number of arguments for pattern org.apache.spark.sql.execution.exchange.ShuffleExchangeExec(outputPartitioning: org.apache.spark.sql.catalyst.plans.physical.Partitioning,child: org.apache.spark.sql.execution.SparkPlan,canChangeNumPartitions: Boolean)
[error] ShuffleExchangeExec(HashPartitioning(rightPartitioningExpressions, _), _), _)) =>
[error] ^
```
## How was this patch tested?
Existing unit test.
Closes#25171 from gaborgsomogyi/SPARK-27485.
Authored-by: Gabor Somogyi <gabor.g.somogyi@gmail.com>
Signed-off-by: herman <herman@databricks.com>
## What changes were proposed in this pull request?
Adaptive execution reduces the number of post-shuffle partitions at runtime, even for shuffles caused by repartition. However, the user likely wants to get the desired number of partition when he calls repartition even in adaptive execution. This PR adds an internal config to control this and by default adaptive execution will not change the number of post-shuffle partition for repartition.
## How was this patch tested?
New tests added.
Closes#25121 from carsonwang/AE_repartition.
Authored-by: Carson Wang <carson.wang@intel.com>
Signed-off-by: Wenchen Fan <wenchen@databricks.com>
## What changes were proposed in this pull request?
When reordering joins EnsureRequirements only checks if all the join keys are present in the partitioning expression seq. This is problematic when the joins keys and and partitioning expressions both contain duplicates but not the same number of duplicates for each expression, e.g. `Seq(a, a, b)` vs `Seq(a, b, b)`. This fails with an index lookup failure in the `reorder` function.
This PR fixes this removing the equality checking logic from the `reorderJoinKeys` function, and by doing the multiset equality in the `reorder` function while building the reordered key sequences.
## How was this patch tested?
Added a unit test to the `PlannerSuite` and added an integration test to `JoinSuite`
Closes#25167 from hvanhovell/SPARK-27485.
Authored-by: herman <herman@databricks.com>
Signed-off-by: Wenchen Fan <wenchen@databricks.com>
## What changes were proposed in this pull request?
A `Filter` predicate using `PythonUDF` can't be push down into join condition, currently. A predicate like that should be able to push down to join condition. For `PythonUDF`s that can't be evaluated in join condition, `PullOutPythonUDFInJoinCondition` will pull them out later.
An example like:
```scala
val pythonTestUDF = TestPythonUDF(name = "udf")
val left = Seq((1, 2), (2, 3)).toDF("a", "b")
val right = Seq((1, 2), (3, 4)).toDF("c", "d")
val df = left.crossJoin(right).where(pythonTestUDF($"a") === pythonTestUDF($"c"))
```
Query plan before the PR:
```
== Physical Plan ==
*(3) Project [a#2121, b#2122, c#2132, d#2133]
+- *(3) Filter (pythonUDF0#2142 = pythonUDF1#2143)
+- BatchEvalPython [udf(a#2121), udf(c#2132)], [pythonUDF0#2142, pythonUDF1#2143]
+- BroadcastNestedLoopJoin BuildRight, Cross
:- *(1) Project [_1#2116 AS a#2121, _2#2117 AS b#2122]
: +- LocalTableScan [_1#2116, _2#2117]
+- BroadcastExchange IdentityBroadcastMode
+- *(2) Project [_1#2127 AS c#2132, _2#2128 AS d#2133]
+- LocalTableScan [_1#2127, _2#2128]
```
Query plan after the PR:
```
== Physical Plan ==
*(3) Project [a#2121, b#2122, c#2132, d#2133]
+- *(3) BroadcastHashJoin [pythonUDF0#2142], [pythonUDF0#2143], Cross, BuildRight
:- BatchEvalPython [udf(a#2121)], [pythonUDF0#2142]
: +- *(1) Project [_1#2116 AS a#2121, _2#2117 AS b#2122]
: +- LocalTableScan [_1#2116, _2#2117]
+- BroadcastExchange HashedRelationBroadcastMode(List(input[2, string, true]))
+- BatchEvalPython [udf(c#2132)], [pythonUDF0#2143]
+- *(2) Project [_1#2127 AS c#2132, _2#2128 AS d#2133]
+- LocalTableScan [_1#2127, _2#2128]
```
After this PR, the join can use `BroadcastHashJoin`, instead of `BroadcastNestedLoopJoin`.
## How was this patch tested?
Added tests.
Closes#25106 from viirya/pythonudf-join-condition.
Authored-by: Liang-Chi Hsieh <viirya@gmail.com>
Signed-off-by: HyukjinKwon <gurwls223@apache.org>
## What changes were proposed in this pull request?
Existing random generators in tests produce wide ranges of values that can be out of supported ranges for:
- `DateType`, the valid range is `[0001-01-01, 9999-12-31]`
- `TimestampType` supports values in `[0001-01-01T00:00:00.000000Z, 9999-12-31T23:59:59.999999Z]`
- `CalendarIntervalType` should define intervals for the ranges above.
Dates and timestamps produced by random literal generators are usually out of valid ranges for those types. And tests just check invalid values or values caused by arithmetic overflow.
In the PR, I propose to restrict tested pseudo-random values by valid ranges of `DateType`, `TimestampType` and `CalendarIntervalType`. This should allow to check valid values in test, and avoid wasting time on a priori invalid inputs.
## How was this patch tested?
The changes were checked by `DateExpressionsSuite` and modified `DateTimeUtils.dateAddMonths`:
```Scala
def dateAddMonths(days: SQLDate, months: Int): SQLDate = {
localDateToDays(LocalDate.ofEpochDay(days).plusMonths(months))
}
```
Closes#25166 from MaxGekk/datetime-lit-random-gen.
Authored-by: Maxim Gekk <max.gekk@gmail.com>
Signed-off-by: Dongjoon Hyun <dhyun@apple.com>
## What changes were proposed in this pull request?
This PR aims to correct mappings in `MsSqlServerDialect`. `ShortType` is mapped to `SMALLINT` and `FloatType` is mapped to `REAL` per [JBDC mapping]( https://docs.microsoft.com/en-us/sql/connect/jdbc/using-basic-data-types?view=sql-server-2017) respectively.
ShortType and FloatTypes are not correctly mapped to right JDBC types when using JDBC connector. This results in tables and spark data frame being created with unintended types. The issue was observed when validating against SQLServer.
Refer [JBDC mapping]( https://docs.microsoft.com/en-us/sql/connect/jdbc/using-basic-data-types?view=sql-server-2017 ) for guidance on mappings between SQLServer, JDBC and Java. Note that java "Short" type should be mapped to JDBC "SMALLINT" and java Float should be mapped to JDBC "REAL".
Some example issue that can happen because of wrong mappings
- Write from df with column type results in a SQL table of with column type as INTEGER as opposed to SMALLINT.Thus a larger table that expected.
- Read results in a dataframe with type INTEGER as opposed to ShortType
- ShortType has a problem in both the the write and read path
- FloatTypes only have an issue with read path. In the write path Spark data type 'FloatType' is correctly mapped to JDBC equivalent data type 'Real'. But in the read path when JDBC data types need to be converted to Catalyst data types ( getCatalystType) 'Real' gets incorrectly gets mapped to 'DoubleType' rather than 'FloatType'.
Refer #28151 which contained this fix as one part of a larger PR. Following PR #28151 discussion it was decided to file seperate PRs for each of the fixes.
## How was this patch tested?
UnitTest added in JDBCSuite.scala and these were tested.
Integration test updated and passed in MsSqlServerDialect.scala
E2E test done with SQLServer
Closes#25146 from shivsood/float_short_type_fix.
Authored-by: shivsood <shivsood@microsoft.com>
Signed-off-by: Dongjoon Hyun <dhyun@apple.com>
## What changes were proposed in this pull request?
`System.currentTimeMillis` read two times in a loop in `RateStreamContinuousPartitionReader`. If the test machine is slow enough and it spends quite some time between the `while` condition check and the `Thread.sleep` then the timeout value is negative and throws `IllegalArgumentException`.
In this PR I've fixed this issue.
## How was this patch tested?
Existing unit tests.
Closes#25162 from gaborgsomogyi/SPARK-28404.
Authored-by: Gabor Somogyi <gabor.g.somogyi@gmail.com>
Signed-off-by: Dongjoon Hyun <dhyun@apple.com>
## What changes were proposed in this pull request?
Idempotence of the `NormalizeFloatingNumbers` rule was broken due to the implementation of `ExtractEquiJoinKeys`. There is no reason that we don't remove `EqualNullSafe` join keys from an equi-join's `otherPredicates`.
## How was this patch tested?
A new UT.
Closes#25126 from yeshengm/spark-28306.
Authored-by: Yesheng Ma <kimi.ysma@gmail.com>
Signed-off-by: gatorsmile <gatorsmile@gmail.com>
## What changes were proposed in this pull request?
For Thrift server, It's downward compatible. Such as if a PROTOCOL_VERSION_V7 client connect to a PROTOCOL_VERSION_V8 server, when OpenSession, server will change his response's protocol version to min of (client and server).
`TProtocolVersion protocol = getMinVersion(CLIService.SERVER_VERSION,`
` req.getClient_protocol());`
then set it to OpenSession's response.
But if OpenSession failed , it won't execute behavior of reset response's protocol_version.
Then it will return server's origin protocol version.
Finally client will get en error as below:
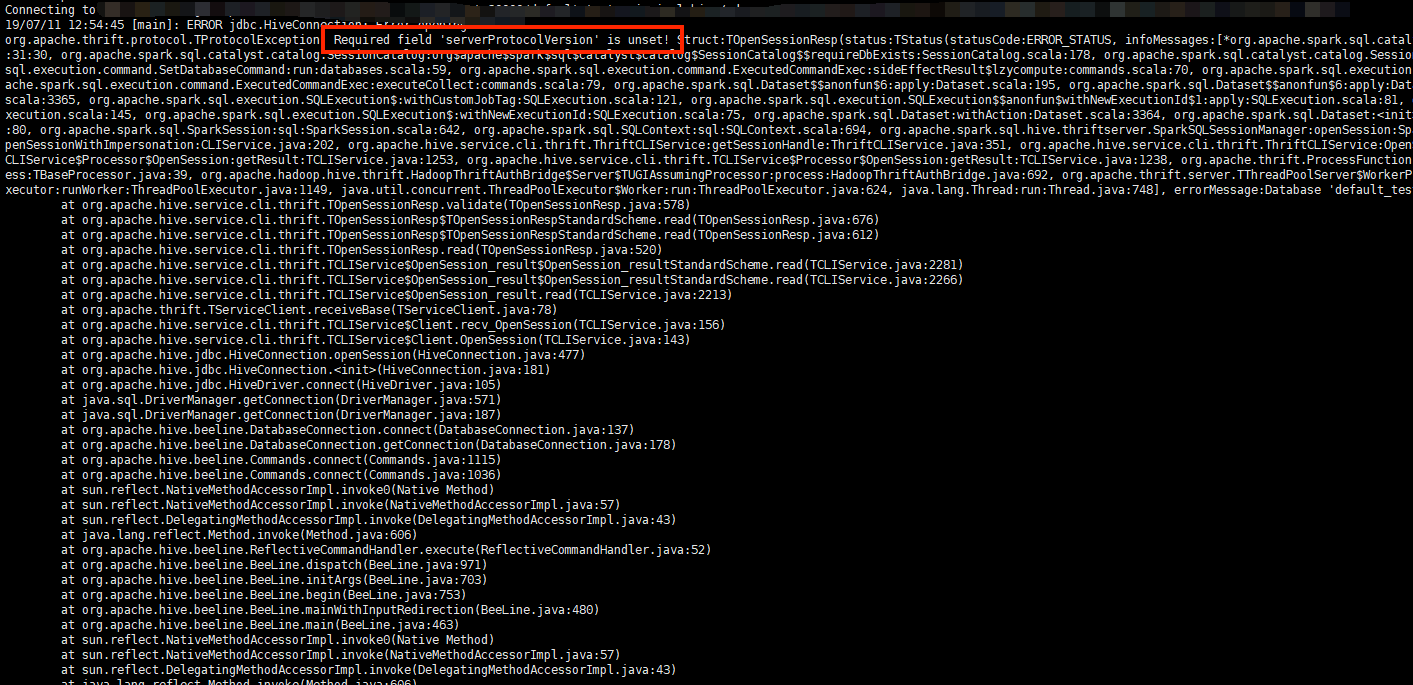
Since we write a wrong database,, OpenSession failed, right protocol version haven't been rest.
## How was this patch tested?
Since I really don't know how to write unit test about this, so I build a jar with this PR,and retry the error above, then it will return a reasonable Error of DB not found :

Closes#25083 from AngersZhuuuu/SPARK-28311.
Authored-by: 朱夷 <zhuyi01@corp.netease.com>
Signed-off-by: Sean Owen <sean.owen@databricks.com>
## What changes were proposed in this pull request?
In the PR, I propose to use the `plusMonths()` method of `LocalDate` to add months to a date. This method adds the specified amount to the months field of `LocalDate` in three steps:
1. Add the input months to the month-of-year field
2. Check if the resulting date would be invalid
3. Adjust the day-of-month to the last valid day if necessary
The difference between current behavior and propose one is in handling the last day of month in the original date. For example, adding 1 month to `2019-02-28` will produce `2019-03-28` comparing to the current implementation where the result is `2019-03-31`.
The proposed behavior is implemented in MySQL and PostgreSQL.
## How was this patch tested?
By existing test suites `DateExpressionsSuite`, `DateFunctionsSuite` and `DateTimeUtilsSuite`.
Closes#25153 from MaxGekk/add-months.
Authored-by: Maxim Gekk <max.gekk@gmail.com>
Signed-off-by: Wenchen Fan <wenchen@databricks.com>
## What changes were proposed in this pull request?
This PR adds some traits so that we can deduplicate initialization stuff for each type of test case. For instance, see [SPARK-28343](https://issues.apache.org/jira/browse/SPARK-28343).
It's a little bit overkill but I think it will make adding test cases easier and cause less confusions.
This PR adds both:
```
private trait PgSQLTest
private trait UDFTest
```
To indicate and share the logics related to each combination of test types.
## How was this patch tested?
Manually tested.
Closes#25155 from HyukjinKwon/SPARK-28392.
Authored-by: HyukjinKwon <gurwls223@apache.org>
Signed-off-by: HyukjinKwon <gurwls223@apache.org>
## What changes were proposed in this pull request?
Adding support to hyperbolic functions like asinh\acosh\atanh in spark SQL.
Feature parity: https://www.postgresql.org/docs/12/functions-math.html#FUNCTIONS-MATH-HYP-TABLE
The followings are the diffence from PostgreSQL.
```
spark-sql> SELECT acosh(0); (PostgreSQL returns `ERROR: input is out of range`)
NaN
spark-sql> SELECT atanh(2); (PostgreSQL returns `ERROR: input is out of range`)
NaN
```
Teradata has similar behavior as PostgreSQL with out of range input float values - It outputs **Invalid Input: numeric value within range only.**
These newly added asinh/acosh/atanh handles special input(NaN, +-Infinity) in the same way as existing cos/sin/tan/acos/asin/atan in spark. For which input value range is not (-∞, ∞)):
out of range float values: Spark returns NaN and PostgreSQL shows input is out of range
NaN: Spark returns NaN, PostgreSQL also returns NaN
Infinity: Spark return NaN, PostgreSQL shows input is out of range
## How was this patch tested?
```
spark.sql("select asinh(xx)")
spark.sql("select acosh(xx)")
spark.sql("select atanh(xx)")
./build/sbt "testOnly org.apache.spark.sql.MathFunctionsSuite"
./build/sbt "testOnly org.apache.spark.sql.catalyst.expressions.MathExpressionsSuite"
```
Closes#25041 from Tonix517/SPARK-28133.
Authored-by: Tony Zhang <tony.zhang@uber.com>
Signed-off-by: Dongjoon Hyun <dhyun@apple.com>
## What changes were proposed in this pull request?
This upgraded to a newer version of Pyrolite. Most updates [1] in the newer version are for dotnot. For java, it includes a bug fix to Unpickler regarding cleaning up Unpickler memo, and support of protocol 5.
After upgrading, we can remove the fix at SPARK-27629 for the bug in Unpickler.
[1] https://github.com/irmen/Pyrolite/compare/pyrolite-4.23...master
## How was this patch tested?
Manually tested on Python 3.6 in local on existing tests.
Closes#25143 from viirya/upgrade-pyrolite.
Authored-by: Liang-Chi Hsieh <viirya@gmail.com>
Signed-off-by: HyukjinKwon <gurwls223@apache.org>
## What changes were proposed in this pull request?
This patch proposes moving all Trigger implementations to `Triggers.scala`, to avoid exposing these implementations to the end users and let end users only deal with `Trigger.xxx` static methods. This fits the intention of deprecation of `ProcessingTIme`, and we agree to move others without deprecation as this patch will be shipped in major version (Spark 3.0.0).
## How was this patch tested?
UTs modified to work with newly introduced class.
Closes#24996 from HeartSaVioR/SPARK-28199.
Authored-by: Jungtaek Lim (HeartSaVioR) <kabhwan@gmail.com>
Signed-off-by: Sean Owen <sean.owen@databricks.com>
## What changes were proposed in this pull request?
This pr enables `spark.sql.crossJoin.enabled` and `spark.sql.parser.ansi.enabled` for PostgreSQL test.
## How was this patch tested?
manual tests:
Run `test.sql` in [pgSQL](https://github.com/apache/spark/tree/master/sql/core/src/test/resources/sql-tests/inputs/pgSQL) directory and in [inputs](https://github.com/apache/spark/tree/master/sql/core/src/test/resources/sql-tests/inputs) directory:
```sql
cat <<EOF > test.sql
create or replace temporary view t1 as
select * from (values(1), (2)) as v (val);
create or replace temporary view t2 as
select * from (values(2), (1)) as v (val);
select t1.*, t2.* from t1 join t2;
EOF
```
Closes#25109 from wangyum/SPARK-28343.
Authored-by: Yuming Wang <yumwang@ebay.com>
Signed-off-by: Dongjoon Hyun <dhyun@apple.com>
Parquet may call the filter with a null value to check whether nulls are
accepted. While it seems Spark avoids that path in Parquet with 1.10, in
1.11 that causes Spark unit tests to fail.
Tested with Parquet 1.11 (and new unit test).
Closes#25140 from vanzin/SPARK-28371.
Authored-by: Marcelo Vanzin <vanzin@cloudera.com>
Signed-off-by: Dongjoon Hyun <dhyun@apple.com>
## What changes were proposed in this pull request?
This patch fixes the flaky test "query without test harness" on ContinuousSuite, via adding some more gaps on waiting query to commit the epoch which writes output rows.
The observation of this issue is below (injected some debug logs to get them):
```
reader creation time 1562225320210
epoch 1 launched 1562225320593 (+380ms from reader creation time)
epoch 13 launched 1562225321702 (+1.5s from reader creation time)
partition reader creation time 1562225321715 (+1.5s from reader creation time)
next read time for first next call 1562225321210 (+1s from reader creation time)
first next called in partition reader 1562225321746 (immediately after creation of partition reader)
wait finished in next called in partition reader 1562225321746 (no wait)
second next called in partition reader 1562225321747 (immediately after first next())
epoch 0 commit started 1562225321861
writing rows (0, 1) (belong to epoch 13) 1562225321866 (+100ms after first next())
wait start in waitForRateSourceTriggers(2) 1562225322059
next read time for second next call 1562225322210 (+1s from previous "next read time")
wait finished in next called in partition reader 1562225322211 (+450ms wait)
writing rows (2, 3) (belong to epoch 13) 1562225322211 (immediately after next())
epoch 14 launched 1562225322246
desired wait time in waitForRateSourceTriggers(2) 1562225322510 (+2.3s from reader creation time)
epoch 12 committed 1562225323034
```
These rows were written within desired wait time, but the epoch 13 couldn't be committed within it. Interestingly, epoch 12 was lucky to be committed within a gap between finished waiting in waitForRateSourceTriggers and query.stop() - but even suppose the rows were written in epoch 12, it would be just in luck and epoch should be committed within desired wait time.
This patch modifies Rate continuous stream to track the highest committed value, so that test can wait until desired value is reported to the stream as committed.
This patch also modifies Rate continuous stream to track the timestamp at stream gets the first committed offset, and let `waitForRateSourceTriggers` use the timestamp. This also relies on waiting for specific period, but safer approach compared to current based on the observation above. Based on the change, this patch saves couple of seconds in test time.
## How was this patch tested?
10 sequential test runs succeeded locally.
Closes#25048 from HeartSaVioR/SPARK-28247.
Authored-by: Jungtaek Lim (HeartSaVioR) <kabhwan@gmail.com>
Signed-off-by: Sean Owen <sean.owen@databricks.com>
A code gen test in WholeStageCodeGenSuite was flaky because it used the codegen metrics class to test if the generated code for equivalent plans was identical under a particular flag. This patch switches the test to compare the generated code directly.
N/A
Closes#25131 from gatorsmile/WholeStageCodegenSuite.
Authored-by: gatorsmile <gatorsmile@gmail.com>
Signed-off-by: Dongjoon Hyun <dhyun@apple.com>
## What changes were proposed in this pull request?
This PR adds compatibility of handling a `WITH` clause within another `WITH` cause. Before this PR these queries retuned `1` while after this PR they return `2` as PostgreSQL does:
```
WITH
t AS (SELECT 1),
t2 AS (
WITH t AS (SELECT 2)
SELECT * FROM t
)
SELECT * FROM t2
```
```
WITH t AS (SELECT 1)
SELECT (
WITH t AS (SELECT 2)
SELECT * FROM t
)
```
As this is an incompatible change, the PR introduces the `spark.sql.legacy.cte.substitution.enabled` flag as an option to restore old behaviour.
## How was this patch tested?
Added new UTs.
Closes#25029 from peter-toth/SPARK-28228.
Authored-by: Peter Toth <peter.toth@gmail.com>
Signed-off-by: Dongjoon Hyun <dhyun@apple.com>
## What changes were proposed in this pull request?
There are some hardcoded configs, using config entry to replace them.
## How was this patch tested?
Existing UT
Closes#25059 from WangGuangxin/ConfigEntry.
Authored-by: wangguangxin.cn <wangguangxin.cn@gmail.com>
Signed-off-by: Dongjoon Hyun <dhyun@apple.com>
## What changes were proposed in this pull request?
0-args Java UDF alone calls the function even before making it as an expression.
It causes that the function always returns the same value and the function is called at driver side.
Seems like a mistake.
## How was this patch tested?
Unit test was added
Closes#25108 from HyukjinKwon/SPARK-28321.
Authored-by: HyukjinKwon <gurwls223@apache.org>
Signed-off-by: Wenchen Fan <wenchen@databricks.com>
## What changes were proposed in this pull request?
Implement `ALTER TABLE` for v2 tables:
* Add `AlterTable` logical plan and `AlterTableExec` physical plan
* Convert `ALTER TABLE` parsed plans to `AlterTable` when a v2 catalog is responsible for an identifier
* Validate that columns to alter exist in analyzer checks
* Fix nested type handling in `CatalogV2Util`
## How was this patch tested?
* Add extensive tests in `DataSourceV2SQLSuite`
Closes#24937 from rdblue/SPARK-28139-add-v2-alter-table.
Lead-authored-by: Ryan Blue <blue@apache.org>
Co-authored-by: Ryan Blue <rdblue@users.noreply.github.com>
Signed-off-by: Wenchen Fan <wenchen@databricks.com>
## What changes were proposed in this pull request?
Cleaned up (removed) code duplication in `ObjectProducerExec` operators so they use the trait's methods.
## How was this patch tested?
Local build. Waiting for Jenkins.
Closes#25065 from jaceklaskowski/ObjectProducerExec-operators-cleanup.
Authored-by: Jacek Laskowski <jacek@japila.pl>
Signed-off-by: Sean Owen <sean.owen@databricks.com>
## What changes were proposed in this pull request?
This is a second part of the https://issues.apache.org/jira/browse/SPARK-27396 and a follow on to #24795
## How was this patch tested?
I did some manual tests and ran/updated the automated tests
I did some simple performance tests on a single node to try to verify that there is no performance impact, and I was not able to measure anything beyond noise.
Closes#25008 from revans2/columnar-remove-batch-scan.
Authored-by: Robert (Bobby) Evans <bobby@apache.org>
Signed-off-by: Thomas Graves <tgraves@apache.org>
## What changes were proposed in this pull request?
The tests added at https://github.com/apache/spark/pull/25069 seem flaky in some environments. See https://github.com/apache/spark/pull/25069#issuecomment-510338469
Python's string representation of floats can make the tests flaky. See https://docs.python.org/3/tutorial/floatingpoint.html.
I think it's just better to explicitly cast everywhere udf returns a float (or a double) to stay safe. (note that we're not targeting the Python <> Scala value conversions - there are inevitable differences between Python and Scala; therefore, other languages' UDFs cannot guarantee the same results between Python and Scala).
This PR proposes to cast cases to long, integer and decimal explicitly to make the test cases robust.
<details><summary>Diff comparing to 'pgSQL/aggregates_part1.sql'</summary>
<p>
```diff
diff --git a/sql/core/src/test/resources/sql-tests/results/pgSQL/aggregates_part1.sql.out b/sql/core/src/test/resources/sql-tests/results/udf/pgSQL/udf-aggregates_part1.sql.out
index 51ca1d55869..734634b7388 100644
--- a/sql/core/src/test/resources/sql-tests/results/pgSQL/aggregates_part1.sql.out
+++ b/sql/core/src/test/resources/sql-tests/results/udf/pgSQL/udf-aggregates_part1.sql.out
-3,23 +3,23
-- !query 0
-SELECT avg(four) AS avg_1 FROM onek
+SELECT CAST(avg(udf(four)) AS decimal(10,3)) AS avg_1 FROM onek
-- !query 0 schema
-struct<avg_1:double>
+struct<avg_1:decimal(10,3)>
-- !query 0 output
1.5
-- !query 1
-SELECT avg(a) AS avg_32 FROM aggtest WHERE a < 100
+SELECT CAST(udf(avg(a)) AS decimal(10,3)) AS avg_32 FROM aggtest WHERE a < 100
-- !query 1 schema
-struct<avg_32:double>
+struct<avg_32:decimal(10,3)>
-- !query 1 output
-32.666666666666664
+32.667
-- !query 2
-select CAST(avg(b) AS Decimal(10,3)) AS avg_107_943 FROM aggtest
+select CAST(avg(udf(b)) AS Decimal(10,3)) AS avg_107_943 FROM aggtest
-- !query 2 schema
struct<avg_107_943:decimal(10,3)>
-- !query 2 output
-27,39 +27,39 struct<avg_107_943:decimal(10,3)>
-- !query 3
-SELECT sum(four) AS sum_1500 FROM onek
+SELECT CAST(sum(udf(four)) AS int) AS sum_1500 FROM onek
-- !query 3 schema
-struct<sum_1500:bigint>
+struct<sum_1500:int>
-- !query 3 output
1500
-- !query 4
-SELECT sum(a) AS sum_198 FROM aggtest
+SELECT udf(sum(a)) AS sum_198 FROM aggtest
-- !query 4 schema
-struct<sum_198:bigint>
+struct<sum_198:string>
-- !query 4 output
198
-- !query 5
-SELECT sum(b) AS avg_431_773 FROM aggtest
+SELECT CAST(udf(udf(sum(b))) AS decimal(10,3)) AS avg_431_773 FROM aggtest
-- !query 5 schema
-struct<avg_431_773:double>
+struct<avg_431_773:decimal(10,3)>
-- !query 5 output
-431.77260909229517
+431.773
-- !query 6
-SELECT max(four) AS max_3 FROM onek
+SELECT udf(max(four)) AS max_3 FROM onek
-- !query 6 schema
-struct<max_3:int>
+struct<max_3:string>
-- !query 6 output
3
-- !query 7
-SELECT max(a) AS max_100 FROM aggtest
+SELECT max(CAST(udf(a) AS int)) AS max_100 FROM aggtest
-- !query 7 schema
struct<max_100:int>
-- !query 7 output
-67,245 +67,246 struct<max_100:int>
-- !query 8
-SELECT max(aggtest.b) AS max_324_78 FROM aggtest
+SELECT CAST(udf(udf(max(aggtest.b))) AS decimal(10,3)) AS max_324_78 FROM aggtest
-- !query 8 schema
-struct<max_324_78:float>
+struct<max_324_78:decimal(10,3)>
-- !query 8 output
324.78
-- !query 9
-SELECT stddev_pop(b) FROM aggtest
+SELECT CAST(stddev_pop(udf(b)) AS decimal(10,3)) FROM aggtest
-- !query 9 schema
-struct<stddev_pop(CAST(b AS DOUBLE)):double>
+struct<CAST(stddev_pop(CAST(udf(b) AS DOUBLE)) AS DECIMAL(10,3)):decimal(10,3)>
-- !query 9 output
-131.10703231895047
+131.107
-- !query 10
-SELECT stddev_samp(b) FROM aggtest
+SELECT CAST(udf(stddev_samp(b)) AS decimal(10,3)) FROM aggtest
-- !query 10 schema
-struct<stddev_samp(CAST(b AS DOUBLE)):double>
+struct<CAST(udf(stddev_samp(cast(b as double))) AS DECIMAL(10,3)):decimal(10,3)>
-- !query 10 output
-151.38936080399804
+151.389
-- !query 11
-SELECT var_pop(b) FROM aggtest
+SELECT CAST(var_pop(udf(b)) AS decimal(10,3)) FROM aggtest
-- !query 11 schema
-struct<var_pop(CAST(b AS DOUBLE)):double>
+struct<CAST(var_pop(CAST(udf(b) AS DOUBLE)) AS DECIMAL(10,3)):decimal(10,3)>
-- !query 11 output
-17189.053923482323
+17189.054
-- !query 12
-SELECT var_samp(b) FROM aggtest
+SELECT CAST(udf(var_samp(b)) AS decimal(10,3)) FROM aggtest
-- !query 12 schema
-struct<var_samp(CAST(b AS DOUBLE)):double>
+struct<CAST(udf(var_samp(cast(b as double))) AS DECIMAL(10,3)):decimal(10,3)>
-- !query 12 output
-22918.738564643096
+22918.739
-- !query 13
-SELECT stddev_pop(CAST(b AS Decimal(38,0))) FROM aggtest
+SELECT CAST(udf(stddev_pop(CAST(b AS Decimal(38,0)))) AS decimal(10,3)) FROM aggtest
-- !query 13 schema
-struct<stddev_pop(CAST(CAST(b AS DECIMAL(38,0)) AS DOUBLE)):double>
+struct<CAST(udf(stddev_pop(cast(cast(b as decimal(38,0)) as double))) AS DECIMAL(10,3)):decimal(10,3)>
-- !query 13 output
-131.18117242958306
+131.181
-- !query 14
-SELECT stddev_samp(CAST(b AS Decimal(38,0))) FROM aggtest
+SELECT CAST(stddev_samp(CAST(udf(b) AS Decimal(38,0))) AS decimal(10,3)) FROM aggtest
-- !query 14 schema
-struct<stddev_samp(CAST(CAST(b AS DECIMAL(38,0)) AS DOUBLE)):double>
+struct<CAST(stddev_samp(CAST(CAST(udf(b) AS DECIMAL(38,0)) AS DOUBLE)) AS DECIMAL(10,3)):decimal(10,3)>
-- !query 14 output
-151.47497042966097
+151.475
-- !query 15
-SELECT var_pop(CAST(b AS Decimal(38,0))) FROM aggtest
+SELECT CAST(udf(var_pop(CAST(b AS Decimal(38,0)))) AS decimal(10,3)) FROM aggtest
-- !query 15 schema
-struct<var_pop(CAST(CAST(b AS DECIMAL(38,0)) AS DOUBLE)):double>
+struct<CAST(udf(var_pop(cast(cast(b as decimal(38,0)) as double))) AS DECIMAL(10,3)):decimal(10,3)>
-- !query 15 output
17208.5
-- !query 16
-SELECT var_samp(CAST(b AS Decimal(38,0))) FROM aggtest
+SELECT CAST(var_samp(udf(CAST(b AS Decimal(38,0)))) AS decimal(10,3)) FROM aggtest
-- !query 16 schema
-struct<var_samp(CAST(CAST(b AS DECIMAL(38,0)) AS DOUBLE)):double>
+struct<CAST(var_samp(CAST(udf(cast(b as decimal(38,0))) AS DOUBLE)) AS DECIMAL(10,3)):decimal(10,3)>
-- !query 16 output
-22944.666666666668
+22944.667
-- !query 17
-SELECT var_pop(1.0), var_samp(2.0)
+SELECT CAST(udf(var_pop(1.0)) AS int), var_samp(udf(2.0))
-- !query 17 schema
-struct<var_pop(CAST(1.0 AS DOUBLE)):double,var_samp(CAST(2.0 AS DOUBLE)):double>
+struct<CAST(udf(var_pop(cast(1.0 as double))) AS INT):int,var_samp(CAST(udf(2.0) AS DOUBLE)):double>
-- !query 17 output
-0.0 NaN
+0 NaN
-- !query 18
-SELECT stddev_pop(CAST(3.0 AS Decimal(38,0))), stddev_samp(CAST(4.0 AS Decimal(38,0)))
+SELECT CAST(stddev_pop(udf(CAST(3.0 AS Decimal(38,0)))) AS int), stddev_samp(CAST(udf(4.0) AS Decimal(38,0)))
-- !query 18 schema
-struct<stddev_pop(CAST(CAST(3.0 AS DECIMAL(38,0)) AS DOUBLE)):double,stddev_samp(CAST(CAST(4.0 AS DECIMAL(38,0)) AS DOUBLE)):double>
+struct<CAST(stddev_pop(CAST(udf(cast(3.0 as decimal(38,0))) AS DOUBLE)) AS INT):int,stddev_samp(CAST(CAST(udf(4.0) AS DECIMAL(38,0)) AS DOUBLE)):double>
-- !query 18 output
-0.0 NaN
+0 NaN
-- !query 19
-select sum(CAST(null AS int)) from range(1,4)
+select sum(udf(CAST(null AS int))) from range(1,4)
-- !query 19 schema
-struct<sum(CAST(NULL AS INT)):bigint>
+struct<sum(CAST(udf(cast(null as int)) AS DOUBLE)):double>
-- !query 19 output
NULL
-- !query 20
-select sum(CAST(null AS long)) from range(1,4)
+select sum(udf(CAST(null AS long))) from range(1,4)
-- !query 20 schema
-struct<sum(CAST(NULL AS BIGINT)):bigint>
+struct<sum(CAST(udf(cast(null as bigint)) AS DOUBLE)):double>
-- !query 20 output
NULL
-- !query 21
-select sum(CAST(null AS Decimal(38,0))) from range(1,4)
+select sum(udf(CAST(null AS Decimal(38,0)))) from range(1,4)
-- !query 21 schema
-struct<sum(CAST(NULL AS DECIMAL(38,0))):decimal(38,0)>
+struct<sum(CAST(udf(cast(null as decimal(38,0))) AS DOUBLE)):double>
-- !query 21 output
NULL
-- !query 22
-select sum(CAST(null AS DOUBLE)) from range(1,4)
+select sum(udf(CAST(null AS DOUBLE))) from range(1,4)
-- !query 22 schema
-struct<sum(CAST(NULL AS DOUBLE)):double>
+struct<sum(CAST(udf(cast(null as double)) AS DOUBLE)):double>
-- !query 22 output
NULL
-- !query 23
-select avg(CAST(null AS int)) from range(1,4)
+select avg(udf(CAST(null AS int))) from range(1,4)
-- !query 23 schema
-struct<avg(CAST(NULL AS INT)):double>
+struct<avg(CAST(udf(cast(null as int)) AS DOUBLE)):double>
-- !query 23 output
NULL
-- !query 24
-select avg(CAST(null AS long)) from range(1,4)
+select avg(udf(CAST(null AS long))) from range(1,4)
-- !query 24 schema
-struct<avg(CAST(NULL AS BIGINT)):double>
+struct<avg(CAST(udf(cast(null as bigint)) AS DOUBLE)):double>
-- !query 24 output
NULL
-- !query 25
-select avg(CAST(null AS Decimal(38,0))) from range(1,4)
+select avg(udf(CAST(null AS Decimal(38,0)))) from range(1,4)
-- !query 25 schema
-struct<avg(CAST(NULL AS DECIMAL(38,0))):decimal(38,4)>
+struct<avg(CAST(udf(cast(null as decimal(38,0))) AS DOUBLE)):double>
-- !query 25 output
NULL
-- !query 26
-select avg(CAST(null AS DOUBLE)) from range(1,4)
+select avg(udf(CAST(null AS DOUBLE))) from range(1,4)
-- !query 26 schema
-struct<avg(CAST(NULL AS DOUBLE)):double>
+struct<avg(CAST(udf(cast(null as double)) AS DOUBLE)):double>
-- !query 26 output
NULL
-- !query 27
-select sum(CAST('NaN' AS DOUBLE)) from range(1,4)
+select sum(CAST(udf('NaN') AS DOUBLE)) from range(1,4)
-- !query 27 schema
-struct<sum(CAST(NaN AS DOUBLE)):double>
+struct<sum(CAST(udf(NaN) AS DOUBLE)):double>
-- !query 27 output
NaN
-- !query 28
-select avg(CAST('NaN' AS DOUBLE)) from range(1,4)
+select avg(CAST(udf('NaN') AS DOUBLE)) from range(1,4)
-- !query 28 schema
-struct<avg(CAST(NaN AS DOUBLE)):double>
+struct<avg(CAST(udf(NaN) AS DOUBLE)):double>
-- !query 28 output
NaN
-- !query 30
-SELECT avg(CAST(x AS DOUBLE)), var_pop(CAST(x AS DOUBLE))
+SELECT avg(CAST(udf(x) AS DOUBLE)), var_pop(CAST(udf(x) AS DOUBLE))
FROM (VALUES ('Infinity'), ('1')) v(x)
-- !query 30 schema
-struct<avg(CAST(x AS DOUBLE)):double,var_pop(CAST(x AS DOUBLE)):double>
+struct<avg(CAST(udf(x) AS DOUBLE)):double,var_pop(CAST(udf(x) AS DOUBLE)):double>
-- !query 30 output
Infinity NaN
-- !query 31
-SELECT avg(CAST(x AS DOUBLE)), var_pop(CAST(x AS DOUBLE))
+SELECT avg(CAST(udf(x) AS DOUBLE)), var_pop(CAST(udf(x) AS DOUBLE))
FROM (VALUES ('Infinity'), ('Infinity')) v(x)
-- !query 31 schema
-struct<avg(CAST(x AS DOUBLE)):double,var_pop(CAST(x AS DOUBLE)):double>
+struct<avg(CAST(udf(x) AS DOUBLE)):double,var_pop(CAST(udf(x) AS DOUBLE)):double>
-- !query 31 output
Infinity NaN
-- !query 32
-SELECT avg(CAST(x AS DOUBLE)), var_pop(CAST(x AS DOUBLE))
+SELECT avg(CAST(udf(x) AS DOUBLE)), var_pop(CAST(udf(x) AS DOUBLE))
FROM (VALUES ('-Infinity'), ('Infinity')) v(x)
-- !query 32 schema
-struct<avg(CAST(x AS DOUBLE)):double,var_pop(CAST(x AS DOUBLE)):double>
+struct<avg(CAST(udf(x) AS DOUBLE)):double,var_pop(CAST(udf(x) AS DOUBLE)):double>
-- !query 32 output
NaN NaN
-- !query 33
-SELECT avg(CAST(x AS DOUBLE)), var_pop(CAST(x AS DOUBLE))
+SELECT CAST(avg(udf(CAST(x AS DOUBLE))) AS int), CAST(udf(var_pop(CAST(x AS DOUBLE))) AS decimal(10,3))
FROM (VALUES (100000003), (100000004), (100000006), (100000007)) v(x)
-- !query 33 schema
-struct<avg(CAST(x AS DOUBLE)):double,var_pop(CAST(x AS DOUBLE)):double>
+struct<CAST(avg(CAST(udf(cast(x as double)) AS DOUBLE)) AS INT):int,CAST(udf(var_pop(cast(x as double))) AS DECIMAL(10,3)):decimal(10,3)>
-- !query 33 output
-1.00000005E8 2.5
+100000005 2.5
-- !query 34
-SELECT avg(CAST(x AS DOUBLE)), var_pop(CAST(x AS DOUBLE))
+SELECT CAST(avg(udf(CAST(x AS DOUBLE))) AS long), CAST(udf(var_pop(CAST(x AS DOUBLE))) AS decimal(10,3))
FROM (VALUES (7000000000005), (7000000000007)) v(x)
-- !query 34 schema
-struct<avg(CAST(x AS DOUBLE)):double,var_pop(CAST(x AS DOUBLE)):double>
+struct<CAST(avg(CAST(udf(cast(x as double)) AS DOUBLE)) AS BIGINT):bigint,CAST(udf(var_pop(cast(x as double))) AS DECIMAL(10,3)):decimal(10,3)>
-- !query 34 output
-7.000000000006E12 1.0
+7000000000006 1
-- !query 35
-SELECT covar_pop(b, a), covar_samp(b, a) FROM aggtest
+SELECT CAST(udf(covar_pop(b, udf(a))) AS decimal(10,3)), CAST(covar_samp(udf(b), a) as decimal(10,3)) FROM aggtest
-- !query 35 schema
-struct<covar_pop(CAST(b AS DOUBLE), CAST(a AS DOUBLE)):double,covar_samp(CAST(b AS DOUBLE), CAST(a AS DOUBLE)):double>
+struct<CAST(udf(covar_pop(cast(b as double), cast(udf(a) as double))) AS DECIMAL(10,3)):decimal(10,3),CAST(covar_samp(CAST(udf(b) AS DOUBLE), CAST(a AS DOUBLE)) AS DECIMAL(10,3)):decimal(10,3)>
-- !query 35 output
-653.6289553875104 871.5052738500139
+653.629 871.505
-- !query 36
-SELECT corr(b, a) FROM aggtest
+SELECT CAST(corr(b, udf(a)) AS decimal(10,3)) FROM aggtest
-- !query 36 schema
-struct<corr(CAST(b AS DOUBLE), CAST(a AS DOUBLE)):double>
+struct<CAST(corr(CAST(b AS DOUBLE), CAST(udf(a) AS DOUBLE)) AS DECIMAL(10,3)):decimal(10,3)>
-- !query 36 output
-0.1396345165178734
+0.14
-- !query 37
-SELECT count(four) AS cnt_1000 FROM onek
+SELECT count(udf(four)) AS cnt_1000 FROM onek
-- !query 37 schema
struct<cnt_1000:bigint>
-- !query 37 output
-313,18 +314,18 struct<cnt_1000:bigint>
-- !query 38
-SELECT count(DISTINCT four) AS cnt_4 FROM onek
+SELECT udf(count(DISTINCT four)) AS cnt_4 FROM onek
-- !query 38 schema
-struct<cnt_4:bigint>
+struct<cnt_4:string>
-- !query 38 output
4
-- !query 39
-select ten, count(*), sum(four) from onek
+select ten, udf(count(*)), CAST(sum(udf(four)) AS int) from onek
group by ten order by ten
-- !query 39 schema
-struct<ten:int,count(1):bigint,sum(four):bigint>
+struct<ten:int,udf(count(1)):string,CAST(sum(CAST(udf(four) AS DOUBLE)) AS INT):int>
-- !query 39 output
0 100 100
1 100 200
-339,10 +340,10 struct<ten:int,count(1):bigint,sum(four):bigint>
-- !query 40
-select ten, count(four), sum(DISTINCT four) from onek
+select ten, count(udf(four)), udf(sum(DISTINCT four)) from onek
group by ten order by ten
-- !query 40 schema
-struct<ten:int,count(four):bigint,sum(DISTINCT four):bigint>
+struct<ten:int,count(udf(four)):bigint,udf(sum(distinct cast(four as bigint))):string>
-- !query 40 output
0 100 2
1 100 4
-357,11 +358,11 struct<ten:int,count(four):bigint,sum(DISTINCT four):bigint>
-- !query 41
-select ten, sum(distinct four) from onek a
+select ten, udf(sum(distinct four)) from onek a
group by ten
-having exists (select 1 from onek b where sum(distinct a.four) = b.four)
+having exists (select 1 from onek b where udf(sum(distinct a.four)) = b.four)
-- !query 41 schema
-struct<ten:int,sum(DISTINCT four):bigint>
+struct<ten:int,udf(sum(distinct cast(four as bigint))):string>
-- !query 41 output
0 2
2 2
-374,23 +375,23 struct<ten:int,sum(DISTINCT four):bigint>
select ten, sum(distinct four) from onek a
group by ten
having exists (select 1 from onek b
- where sum(distinct a.four + b.four) = b.four)
+ where sum(distinct a.four + b.four) = udf(b.four))
-- !query 42 schema
struct<>
-- !query 42 output
org.apache.spark.sql.AnalysisException
Aggregate/Window/Generate expressions are not valid in where clause of the query.
-Expression in where clause: [(sum(DISTINCT CAST((outer() + b.`four`) AS BIGINT)) = CAST(b.`four` AS BIGINT))]
+Expression in where clause: [(sum(DISTINCT CAST((outer() + b.`four`) AS BIGINT)) = CAST(udf(four) AS BIGINT))]
Invalid expressions: [sum(DISTINCT CAST((outer() + b.`four`) AS BIGINT))];
-- !query 43
select
- (select max((select i.unique2 from tenk1 i where i.unique1 = o.unique1)))
+ (select udf(max((select i.unique2 from tenk1 i where i.unique1 = o.unique1))))
from tenk1 o
-- !query 43 schema
struct<>
-- !query 43 output
org.apache.spark.sql.AnalysisException
-cannot resolve '`o.unique1`' given input columns: [i.even, i.fivethous, i.four, i.hundred, i.odd, i.string4, i.stringu1, i.stringu2, i.ten, i.tenthous, i.thousand, i.twenty, i.two, i.twothousand, i.unique1, i.unique2]; line 2 pos 63
+cannot resolve '`o.unique1`' given input columns: [i.even, i.fivethous, i.four, i.hundred, i.odd, i.string4, i.stringu1, i.stringu2, i.ten, i.tenthous, i.thousand, i.twenty, i.two, i.twothousand, i.unique1, i.unique2]; line 2 pos 67
```
</p>
</details>
## How was this patch tested?
Manually tested in local.
Also, with JDK 11:
```
Using /.../jdk-11.0.3.jdk/Contents/Home as default JAVA_HOME.
Note, this will be overridden by -java-home if it is set.
[info] Loading project definition from /.../spark/project
[info] Updating {file:/.../spark/project/}spark-build...
...
[info] SQLQueryTestSuite:
...
[info] - udf/pgSQL/udf-aggregates_part1.sql - Scala UDF (17 seconds, 228 milliseconds)
[info] - udf/pgSQL/udf-aggregates_part1.sql - Regular Python UDF (36 seconds, 170 milliseconds)
[info] - udf/pgSQL/udf-aggregates_part1.sql - Scalar Pandas UDF (41 seconds, 132 milliseconds)
...
```
Closes#25110 from HyukjinKwon/SPARK-28270-1.
Authored-by: HyukjinKwon <gurwls223@apache.org>
Signed-off-by: HyukjinKwon <gurwls223@apache.org>
## What changes were proposed in this pull request?
The optimizer rule `NormalizeFloatingNumbers` is not idempotent. It will generate multiple `NormalizeNaNAndZero` and `ArrayTransform` expression nodes for multiple runs. This patch fixed this non-idempotence by adding a marking tag above normalized expressions. It also adds missing UTs for `NormalizeFloatingNumbers`.
## How was this patch tested?
New UTs.
Closes#25080 from yeshengm/spark-28306.
Authored-by: Yesheng Ma <kimi.ysma@gmail.com>
Signed-off-by: Wenchen Fan <wenchen@databricks.com>
## What changes were proposed in this pull request?
This PR proposes to replace `REL_12_BETA1` to `REL_12_BETA2` which is latest.
## How was this patch tested?
Manually checked each link and checked via `git grep -r REL_12_BETA1` as well.
Closes#25105 from HyukjinKwon/SPARK-28342.
Authored-by: HyukjinKwon <gurwls223@apache.org>
Signed-off-by: Dongjoon Hyun <dhyun@apple.com>
## What changes were proposed in this pull request?
The new adaptive execution framework introduced configuration `spark.sql.runtime.reoptimization.enabled`. We now rename it back to `spark.sql.adaptive.enabled` as the umbrella configuration for adaptive execution.
## How was this patch tested?
Existing tests.
Closes#25102 from carsonwang/renameAE.
Authored-by: Carson Wang <carson.wang@intel.com>
Signed-off-by: Wenchen Fan <wenchen@databricks.com>
## What changes were proposed in this pull request?
This PR adds some tests converted from `pgSQL/aggregates_part1.sql'` to test UDFs. Please see contribution guide of this umbrella ticket - [SPARK-27921](https://issues.apache.org/jira/browse/SPARK-27921).
This PR also contains two minor fixes:
1. Change name of Scala UDF from `UDF:name(...)` to `name(...)` to be consistent with Python'
2. Fix Scala UDF at `IntegratedUDFTestUtils.scala ` to handle `null` in strings.
<details><summary>Diff comparing to 'pgSQL/aggregates_part1.sql'</summary>
<p>
```diff
diff --git a/sql/core/src/test/resources/sql-tests/results/pgSQL/aggregates_part1.sql.out b/sql/core/src/test/resources/sql-tests/results/udf/pgSQL/udf-aggregates_part1.sql.out
index 51ca1d55869..124fdd6416e 100644
--- a/sql/core/src/test/resources/sql-tests/results/pgSQL/aggregates_part1.sql.out
+++ b/sql/core/src/test/resources/sql-tests/results/udf/pgSQL/udf-aggregates_part1.sql.out
-3,7 +3,7
-- !query 0
-SELECT avg(four) AS avg_1 FROM onek
+SELECT avg(udf(four)) AS avg_1 FROM onek
-- !query 0 schema
struct<avg_1:double>
-- !query 0 output
-11,15 +11,15 struct<avg_1:double>
-- !query 1
-SELECT avg(a) AS avg_32 FROM aggtest WHERE a < 100
+SELECT udf(avg(a)) AS avg_32 FROM aggtest WHERE a < 100
-- !query 1 schema
-struct<avg_32:double>
+struct<avg_32:string>
-- !query 1 output
32.666666666666664
-- !query 2
-select CAST(avg(b) AS Decimal(10,3)) AS avg_107_943 FROM aggtest
+select CAST(avg(udf(b)) AS Decimal(10,3)) AS avg_107_943 FROM aggtest
-- !query 2 schema
struct<avg_107_943:decimal(10,3)>
-- !query 2 output
-27,285 +27,286 struct<avg_107_943:decimal(10,3)>
-- !query 3
-SELECT sum(four) AS sum_1500 FROM onek
+SELECT sum(udf(four)) AS sum_1500 FROM onek
-- !query 3 schema
-struct<sum_1500:bigint>
+struct<sum_1500:double>
-- !query 3 output
-1500
+1500.0
-- !query 4
-SELECT sum(a) AS sum_198 FROM aggtest
+SELECT udf(sum(a)) AS sum_198 FROM aggtest
-- !query 4 schema
-struct<sum_198:bigint>
+struct<sum_198:string>
-- !query 4 output
198
-- !query 5
-SELECT sum(b) AS avg_431_773 FROM aggtest
+SELECT udf(udf(sum(b))) AS avg_431_773 FROM aggtest
-- !query 5 schema
-struct<avg_431_773:double>
+struct<avg_431_773:string>
-- !query 5 output
431.77260909229517
-- !query 6
-SELECT max(four) AS max_3 FROM onek
+SELECT udf(max(four)) AS max_3 FROM onek
-- !query 6 schema
-struct<max_3:int>
+struct<max_3:string>
-- !query 6 output
3
-- !query 7
-SELECT max(a) AS max_100 FROM aggtest
+SELECT max(udf(a)) AS max_100 FROM aggtest
-- !query 7 schema
-struct<max_100:int>
+struct<max_100:string>
-- !query 7 output
-100
+56
-- !query 8
-SELECT max(aggtest.b) AS max_324_78 FROM aggtest
+SELECT CAST(udf(udf(max(aggtest.b))) AS int) AS max_324_78 FROM aggtest
-- !query 8 schema
-struct<max_324_78:float>
+struct<max_324_78:int>
-- !query 8 output
-324.78
+324
-- !query 9
-SELECT stddev_pop(b) FROM aggtest
+SELECT CAST(stddev_pop(udf(b)) AS int) FROM aggtest
-- !query 9 schema
-struct<stddev_pop(CAST(b AS DOUBLE)):double>
+struct<CAST(stddev_pop(CAST(udf(b) AS DOUBLE)) AS INT):int>
-- !query 9 output
-131.10703231895047
+131
-- !query 10
-SELECT stddev_samp(b) FROM aggtest
+SELECT udf(stddev_samp(b)) FROM aggtest
-- !query 10 schema
-struct<stddev_samp(CAST(b AS DOUBLE)):double>
+struct<udf(stddev_samp(cast(b as double))):string>
-- !query 10 output
151.38936080399804
-- !query 11
-SELECT var_pop(b) FROM aggtest
+SELECT CAST(var_pop(udf(b)) as int) FROM aggtest
-- !query 11 schema
-struct<var_pop(CAST(b AS DOUBLE)):double>
+struct<CAST(var_pop(CAST(udf(b) AS DOUBLE)) AS INT):int>
-- !query 11 output
-17189.053923482323
+17189
-- !query 12
-SELECT var_samp(b) FROM aggtest
+SELECT udf(var_samp(b)) FROM aggtest
-- !query 12 schema
-struct<var_samp(CAST(b AS DOUBLE)):double>
+struct<udf(var_samp(cast(b as double))):string>
-- !query 12 output
22918.738564643096
-- !query 13
-SELECT stddev_pop(CAST(b AS Decimal(38,0))) FROM aggtest
+SELECT udf(stddev_pop(CAST(b AS Decimal(38,0)))) FROM aggtest
-- !query 13 schema
-struct<stddev_pop(CAST(CAST(b AS DECIMAL(38,0)) AS DOUBLE)):double>
+struct<udf(stddev_pop(cast(cast(b as decimal(38,0)) as double))):string>
-- !query 13 output
131.18117242958306
-- !query 14
-SELECT stddev_samp(CAST(b AS Decimal(38,0))) FROM aggtest
+SELECT stddev_samp(CAST(udf(b) AS Decimal(38,0))) FROM aggtest
-- !query 14 schema
-struct<stddev_samp(CAST(CAST(b AS DECIMAL(38,0)) AS DOUBLE)):double>
+struct<stddev_samp(CAST(CAST(udf(b) AS DECIMAL(38,0)) AS DOUBLE)):double>
-- !query 14 output
151.47497042966097
-- !query 15
-SELECT var_pop(CAST(b AS Decimal(38,0))) FROM aggtest
+SELECT udf(var_pop(CAST(b AS Decimal(38,0)))) FROM aggtest
-- !query 15 schema
-struct<var_pop(CAST(CAST(b AS DECIMAL(38,0)) AS DOUBLE)):double>
+struct<udf(var_pop(cast(cast(b as decimal(38,0)) as double))):string>
-- !query 15 output
17208.5
-- !query 16
-SELECT var_samp(CAST(b AS Decimal(38,0))) FROM aggtest
+SELECT var_samp(udf(CAST(b AS Decimal(38,0)))) FROM aggtest
-- !query 16 schema
-struct<var_samp(CAST(CAST(b AS DECIMAL(38,0)) AS DOUBLE)):double>
+struct<var_samp(CAST(udf(cast(b as decimal(38,0))) AS DOUBLE)):double>
-- !query 16 output
22944.666666666668
-- !query 17
-SELECT var_pop(1.0), var_samp(2.0)
+SELECT udf(var_pop(1.0)), var_samp(udf(2.0))
-- !query 17 schema
-struct<var_pop(CAST(1.0 AS DOUBLE)):double,var_samp(CAST(2.0 AS DOUBLE)):double>
+struct<udf(var_pop(cast(1.0 as double))):string,var_samp(CAST(udf(2.0) AS DOUBLE)):double>
-- !query 17 output
0.0 NaN
-- !query 18
-SELECT stddev_pop(CAST(3.0 AS Decimal(38,0))), stddev_samp(CAST(4.0 AS Decimal(38,0)))
+SELECT stddev_pop(udf(CAST(3.0 AS Decimal(38,0)))), stddev_samp(CAST(udf(4.0) AS Decimal(38,0)))
-- !query 18 schema
-struct<stddev_pop(CAST(CAST(3.0 AS DECIMAL(38,0)) AS DOUBLE)):double,stddev_samp(CAST(CAST(4.0 AS DECIMAL(38,0)) AS DOUBLE)):double>
+struct<stddev_pop(CAST(udf(cast(3.0 as decimal(38,0))) AS DOUBLE)):double,stddev_samp(CAST(CAST(udf(4.0) AS DECIMAL(38,0)) AS DOUBLE)):double>
-- !query 18 output
0.0 NaN
-- !query 19
-select sum(CAST(null AS int)) from range(1,4)
+select sum(udf(CAST(null AS int))) from range(1,4)
-- !query 19 schema
-struct<sum(CAST(NULL AS INT)):bigint>
+struct<sum(CAST(udf(cast(null as int)) AS DOUBLE)):double>
-- !query 19 output
NULL
-- !query 20
-select sum(CAST(null AS long)) from range(1,4)
+select sum(udf(CAST(null AS long))) from range(1,4)
-- !query 20 schema
-struct<sum(CAST(NULL AS BIGINT)):bigint>
+struct<sum(CAST(udf(cast(null as bigint)) AS DOUBLE)):double>
-- !query 20 output
NULL
-- !query 21
-select sum(CAST(null AS Decimal(38,0))) from range(1,4)
+select sum(udf(CAST(null AS Decimal(38,0)))) from range(1,4)
-- !query 21 schema
-struct<sum(CAST(NULL AS DECIMAL(38,0))):decimal(38,0)>
+struct<sum(CAST(udf(cast(null as decimal(38,0))) AS DOUBLE)):double>
-- !query 21 output
NULL
-- !query 22
-select sum(CAST(null AS DOUBLE)) from range(1,4)
+select sum(udf(CAST(null AS DOUBLE))) from range(1,4)
-- !query 22 schema
-struct<sum(CAST(NULL AS DOUBLE)):double>
+struct<sum(CAST(udf(cast(null as double)) AS DOUBLE)):double>
-- !query 22 output
NULL
-- !query 23
-select avg(CAST(null AS int)) from range(1,4)
+select avg(udf(CAST(null AS int))) from range(1,4)
-- !query 23 schema
-struct<avg(CAST(NULL AS INT)):double>
+struct<avg(CAST(udf(cast(null as int)) AS DOUBLE)):double>
-- !query 23 output
NULL
-- !query 24
-select avg(CAST(null AS long)) from range(1,4)
+select avg(udf(CAST(null AS long))) from range(1,4)
-- !query 24 schema
-struct<avg(CAST(NULL AS BIGINT)):double>
+struct<avg(CAST(udf(cast(null as bigint)) AS DOUBLE)):double>
-- !query 24 output
NULL
-- !query 25
-select avg(CAST(null AS Decimal(38,0))) from range(1,4)
+select avg(udf(CAST(null AS Decimal(38,0)))) from range(1,4)
-- !query 25 schema
-struct<avg(CAST(NULL AS DECIMAL(38,0))):decimal(38,4)>
+struct<avg(CAST(udf(cast(null as decimal(38,0))) AS DOUBLE)):double>
-- !query 25 output
NULL
-- !query 26
-select avg(CAST(null AS DOUBLE)) from range(1,4)
+select avg(udf(CAST(null AS DOUBLE))) from range(1,4)
-- !query 26 schema
-struct<avg(CAST(NULL AS DOUBLE)):double>
+struct<avg(CAST(udf(cast(null as double)) AS DOUBLE)):double>
-- !query 26 output
NULL
-- !query 27
-select sum(CAST('NaN' AS DOUBLE)) from range(1,4)
+select sum(CAST(udf('NaN') AS DOUBLE)) from range(1,4)
-- !query 27 schema
-struct<sum(CAST(NaN AS DOUBLE)):double>
+struct<sum(CAST(udf(NaN) AS DOUBLE)):double>
-- !query 27 output
NaN
-- !query 28
-select avg(CAST('NaN' AS DOUBLE)) from range(1,4)
+select avg(CAST(udf('NaN') AS DOUBLE)) from range(1,4)
-- !query 28 schema
-struct<avg(CAST(NaN AS DOUBLE)):double>
+struct<avg(CAST(udf(NaN) AS DOUBLE)):double>
-- !query 28 output
NaN
-- !query 29
SELECT avg(CAST(x AS DOUBLE)), var_pop(CAST(x AS DOUBLE))
-FROM (VALUES (CAST('1' AS DOUBLE)), (CAST('Infinity' AS DOUBLE))) v(x)
+FROM (VALUES (CAST(udf('1') AS DOUBLE)), (CAST(udf('Infinity') AS DOUBLE))) v(x)
-- !query 29 schema
-struct<avg(CAST(x AS DOUBLE)):double,var_pop(CAST(x AS DOUBLE)):double>
+struct<>
-- !query 29 output
-Infinity NaN
+org.apache.spark.sql.AnalysisException
+cannot evaluate expression CAST(udf(1) AS DOUBLE) in inline table definition; line 2 pos 14
-- !query 30
-SELECT avg(CAST(x AS DOUBLE)), var_pop(CAST(x AS DOUBLE))
+SELECT avg(CAST(udf(x) AS DOUBLE)), var_pop(CAST(udf(x) AS DOUBLE))
FROM (VALUES ('Infinity'), ('1')) v(x)
-- !query 30 schema
-struct<avg(CAST(x AS DOUBLE)):double,var_pop(CAST(x AS DOUBLE)):double>
+struct<avg(CAST(udf(x) AS DOUBLE)):double,var_pop(CAST(udf(x) AS DOUBLE)):double>
-- !query 30 output
Infinity NaN
-- !query 31
-SELECT avg(CAST(x AS DOUBLE)), var_pop(CAST(x AS DOUBLE))
+SELECT avg(CAST(udf(x) AS DOUBLE)), var_pop(CAST(udf(x) AS DOUBLE))
FROM (VALUES ('Infinity'), ('Infinity')) v(x)
-- !query 31 schema
-struct<avg(CAST(x AS DOUBLE)):double,var_pop(CAST(x AS DOUBLE)):double>
+struct<avg(CAST(udf(x) AS DOUBLE)):double,var_pop(CAST(udf(x) AS DOUBLE)):double>
-- !query 31 output
Infinity NaN
-- !query 32
-SELECT avg(CAST(x AS DOUBLE)), var_pop(CAST(x AS DOUBLE))
+SELECT avg(CAST(udf(x) AS DOUBLE)), var_pop(CAST(udf(x) AS DOUBLE))
FROM (VALUES ('-Infinity'), ('Infinity')) v(x)
-- !query 32 schema
-struct<avg(CAST(x AS DOUBLE)):double,var_pop(CAST(x AS DOUBLE)):double>
+struct<avg(CAST(udf(x) AS DOUBLE)):double,var_pop(CAST(udf(x) AS DOUBLE)):double>
-- !query 32 output
NaN NaN
-- !query 33
-SELECT avg(CAST(x AS DOUBLE)), var_pop(CAST(x AS DOUBLE))
+SELECT avg(udf(CAST(x AS DOUBLE))), udf(var_pop(CAST(x AS DOUBLE)))
FROM (VALUES (100000003), (100000004), (100000006), (100000007)) v(x)
-- !query 33 schema
-struct<avg(CAST(x AS DOUBLE)):double,var_pop(CAST(x AS DOUBLE)):double>
+struct<avg(CAST(udf(cast(x as double)) AS DOUBLE)):double,udf(var_pop(cast(x as double))):string>
-- !query 33 output
1.00000005E8 2.5
-- !query 34
-SELECT avg(CAST(x AS DOUBLE)), var_pop(CAST(x AS DOUBLE))
+SELECT avg(udf(CAST(x AS DOUBLE))), udf(var_pop(CAST(x AS DOUBLE)))
FROM (VALUES (7000000000005), (7000000000007)) v(x)
-- !query 34 schema
-struct<avg(CAST(x AS DOUBLE)):double,var_pop(CAST(x AS DOUBLE)):double>
+struct<avg(CAST(udf(cast(x as double)) AS DOUBLE)):double,udf(var_pop(cast(x as double))):string>
-- !query 34 output
7.000000000006E12 1.0
-- !query 35
-SELECT covar_pop(b, a), covar_samp(b, a) FROM aggtest
+SELECT CAST(udf(covar_pop(b, udf(a))) AS int), CAST(covar_samp(udf(b), a) as int) FROM aggtest
-- !query 35 schema
-struct<covar_pop(CAST(b AS DOUBLE), CAST(a AS DOUBLE)):double,covar_samp(CAST(b AS DOUBLE), CAST(a AS DOUBLE)):double>
+struct<CAST(udf(covar_pop(cast(b as double), cast(udf(a) as double))) AS INT):int,CAST(covar_samp(CAST(udf(b) AS DOUBLE), CAST(a AS DOUBLE)) AS INT):int>
-- !query 35 output
-653.6289553875104 871.5052738500139
+653 871
-- !query 36
-SELECT corr(b, a) FROM aggtest
+SELECT corr(b, udf(a)) FROM aggtest
-- !query 36 schema
-struct<corr(CAST(b AS DOUBLE), CAST(a AS DOUBLE)):double>
+struct<corr(CAST(b AS DOUBLE), CAST(udf(a) AS DOUBLE)):double>
-- !query 36 output
0.1396345165178734
-- !query 37
-SELECT count(four) AS cnt_1000 FROM onek
+SELECT count(udf(four)) AS cnt_1000 FROM onek
-- !query 37 schema
struct<cnt_1000:bigint>
-- !query 37 output
-313,36 +314,36 struct<cnt_1000:bigint>
-- !query 38
-SELECT count(DISTINCT four) AS cnt_4 FROM onek
+SELECT udf(count(DISTINCT four)) AS cnt_4 FROM onek
-- !query 38 schema
-struct<cnt_4:bigint>
+struct<cnt_4:string>
-- !query 38 output
4
-- !query 39
-select ten, count(*), sum(four) from onek
+select ten, udf(count(*)), sum(udf(four)) from onek
group by ten order by ten
-- !query 39 schema
-struct<ten:int,count(1):bigint,sum(four):bigint>
+struct<ten:int,udf(count(1)):string,sum(CAST(udf(four) AS DOUBLE)):double>
-- !query 39 output
-0 100 100
-1 100 200
-2 100 100
-3 100 200
-4 100 100
-5 100 200
-6 100 100
-7 100 200
-8 100 100
-9 100 200
+0 100 100.0
+1 100 200.0
+2 100 100.0
+3 100 200.0
+4 100 100.0
+5 100 200.0
+6 100 100.0
+7 100 200.0
+8 100 100.0
+9 100 200.0
-- !query 40
-select ten, count(four), sum(DISTINCT four) from onek
+select ten, count(udf(four)), udf(sum(DISTINCT four)) from onek
group by ten order by ten
-- !query 40 schema
-struct<ten:int,count(four):bigint,sum(DISTINCT four):bigint>
+struct<ten:int,count(udf(four)):bigint,udf(sum(distinct cast(four as bigint))):string>
-- !query 40 output
0 100 2
1 100 4
-357,11 +358,11 struct<ten:int,count(four):bigint,sum(DISTINCT four):bigint>
-- !query 41
-select ten, sum(distinct four) from onek a
+select ten, udf(sum(distinct four)) from onek a
group by ten
-having exists (select 1 from onek b where sum(distinct a.four) = b.four)
+having exists (select 1 from onek b where udf(sum(distinct a.four)) = b.four)
-- !query 41 schema
-struct<ten:int,sum(DISTINCT four):bigint>
+struct<ten:int,udf(sum(distinct cast(four as bigint))):string>
-- !query 41 output
0 2
2 2
-374,23 +375,23 struct<ten:int,sum(DISTINCT four):bigint>
select ten, sum(distinct four) from onek a
group by ten
having exists (select 1 from onek b
- where sum(distinct a.four + b.four) = b.four)
+ where sum(distinct a.four + b.four) = udf(b.four))
-- !query 42 schema
struct<>
-- !query 42 output
org.apache.spark.sql.AnalysisException
Aggregate/Window/Generate expressions are not valid in where clause of the query.
-Expression in where clause: [(sum(DISTINCT CAST((outer() + b.`four`) AS BIGINT)) = CAST(b.`four` AS BIGINT))]
+Expression in where clause: [(sum(DISTINCT CAST((outer() + b.`four`) AS BIGINT)) = CAST(udf(four) AS BIGINT))]
Invalid expressions: [sum(DISTINCT CAST((outer() + b.`four`) AS BIGINT))];
-- !query 43
select
- (select max((select i.unique2 from tenk1 i where i.unique1 = o.unique1)))
+ (select udf(max((select i.unique2 from tenk1 i where i.unique1 = o.unique1))))
from tenk1 o
-- !query 43 schema
struct<>
-- !query 43 output
org.apache.spark.sql.AnalysisException
-cannot resolve '`o.unique1`' given input columns: [i.even, i.fivethous, i.four, i.hundred, i.odd, i.string4, i.stringu1, i.stringu2, i.ten, i.tenthous, i.thousand, i.twenty, i.two, i.twothousand, i.unique1, i.unique2]; line 2 pos 63
+cannot resolve '`o.unique1`' given input columns: [i.even, i.fivethous, i.four, i.hundred, i.odd, i.string4, i.stringu1, i.stringu2, i.ten, i.tenthous, i.thousand, i.twenty, i.two, i.twothousand, i.unique1, i.unique2]; line 2 pos 67
```
</p>
</details>
Note that, currently, `IntegratedUDFTestUtils.scala`'s UDFs only return strings. There are some differences between those UDFs (Scala, Pandas and Python):
- Python's string representation of floats can make the tests flaky. (See https://docs.python.org/3/tutorial/floatingpoint.html). To work around this, I had to `CAST(... as int)`.
- There are string representation differences between `Inf` `-Inf` <> `Infinity` `-Infinity` and `nan` <> `NaN`
- Maybe we should add other type versions of UDFs if this makes adding tests difficult.
Note that one issue found - [SPARK-28291](https://issues.apache.org/jira/browse/SPARK-28291). The test was commented for now.
## How was this patch tested?
Tested as guided in [SPARK-27921](https://issues.apache.org/jira/browse/SPARK-27921).
Closes#25069 from HyukjinKwon/SPARK-28270.
Authored-by: HyukjinKwon <gurwls223@apache.org>
Signed-off-by: HyukjinKwon <gurwls223@apache.org>
## What changes were proposed in this pull request?
Fix `stringToDate()` for the formats `yyyy` and `yyyy-[m]m` that assumes there are no additional chars after the last components `yyyy` and `[m]m`. In the PR, I propose to check that entire input was consumed for the formats.
After the fix, the input `1999 08 01` will be invalid because it matches to the pattern `yyyy` but the strings contains additional chars ` 08 01`.
Since Spark 1.6.3 ~ 2.4.3, the behavior is the same.
```
spark-sql> SELECT CAST('1999 08 01' AS DATE);
1999-01-01
```
This PR makes it return NULL like Hive.
```
spark-sql> SELECT CAST('1999 08 01' AS DATE);
NULL
```
## How was this patch tested?
Added new checks to `DateTimeUtilsSuite` for the `1999 08 01` and `1999 08` inputs.
Closes#25097 from MaxGekk/spark-28015-invalid-date-format.
Authored-by: Maxim Gekk <maxim.gekk@databricks.com>
Signed-off-by: Dongjoon Hyun <dhyun@apple.com>
## What changes were proposed in this pull request?
This fixes a problem where it is possible to create a v2 table using the default catalog that cannot be loaded with the session catalog. A session catalog should be used when the v1 catalog is responsible for tables with no catalog in the table identifier.
* Adds a v2 catalog implementation that delegates to the analyzer's SessionCatalog
* Uses the v2 session catalog for CTAS and CreateTable when the provider is a v2 provider and no v2 catalog is in the table identifier
* Updates catalog lookup to always provide the default if it is set for consistent behavior
## How was this patch tested?
* Adds a new test suite for the v2 session catalog that validates the TableCatalog API
* Adds test cases in PlanResolutionSuite to validate the v2 session catalog is used
* Adds test suite for LookupCatalog with a default catalog
Closes#24768 from rdblue/SPARK-27919-add-v2-session-catalog.
Authored-by: Ryan Blue <blue@apache.org>
Signed-off-by: Wenchen Fan <wenchen@databricks.com>
## What changes were proposed in this pull request?
The interval conversion behavior is same with the PostgreSQL.
https://github.com/postgres/postgres/blob/REL_12_BETA2/src/test/regress/sql/interval.sql#L180-L203
## How was this patch tested?
UT.
Closes#25000 from lipzhu/SPARK-28107.
Lead-authored-by: Zhu, Lipeng <lipzhu@ebay.com>
Co-authored-by: Dongjoon Hyun <dhyun@apple.com>
Co-authored-by: Lipeng Zhu <lipzhu@icloud.com>
Signed-off-by: Dongjoon Hyun <dhyun@apple.com>
## What changes were proposed in this pull request?
This PR adds some tests converted from having.sql to test UDFs following the combination guide in [SPARK-27921](url)
<details><summary>Diff comparing to 'having.sql'</summary>
<p>
```diff
diff --git a/sql/core/src/test/resources/sql-tests/results/having.sql.out b/sql/core/src/test/resources/sql-tests/results/udf/udf-having.sql.out
index d87ee52216..7cea2e5128 100644
--- a/sql/core/src/test/resources/sql-tests/results/having.sql.out
+++ b/sql/core/src/test/resources/sql-tests/results/udf/udf-having.sql.out
-16,34 +16,34 struct<>
-- !query 1
-SELECT k, sum(v) FROM hav GROUP BY k HAVING sum(v) > 2
+SELECT udf(k) AS k, udf(sum(v)) FROM hav GROUP BY k HAVING udf(sum(v)) > 2
-- !query 1 schema
-struct<k:string,sum(v):bigint>
+struct<k:string,udf(sum(cast(v as bigint))):string>
-- !query 1 output
one 6
three 3
-- !query 2
-SELECT count(k) FROM hav GROUP BY v + 1 HAVING v + 1 = 2
+SELECT udf(count(udf(k))) FROM hav GROUP BY v + 1 HAVING v + 1 = udf(2)
-- !query 2 schema
-struct<count(k):bigint>
+struct<udf(count(udf(k))):string>
-- !query 2 output
1
-- !query 3
-SELECT MIN(t.v) FROM (SELECT * FROM hav WHERE v > 0) t HAVING(COUNT(1) > 0)
+SELECT udf(MIN(t.v)) FROM (SELECT * FROM hav WHERE v > 0) t HAVING(udf(COUNT(udf(1))) > 0)
-- !query 3 schema
-struct<min(v):int>
+struct<udf(min(v)):string>
-- !query 3 output
1
-- !query 4
-SELECT a + b FROM VALUES (1L, 2), (3L, 4) AS T(a, b) GROUP BY a + b HAVING a + b > 1
+SELECT udf(a + b) FROM VALUES (1L, 2), (3L, 4) AS T(a, b) GROUP BY a + b HAVING a + b > udf(1)
-- !query 4 schema
-struct<(a + CAST(b AS BIGINT)):bigint>
+struct<udf((a + cast(b as bigint))):string>
-- !query 4 output
3
7
```
</p>
</details>
## How was this patch tested?
Tested as guided in SPARK-27921.
Closes#25093 from huaxingao/spark-28281.
Authored-by: Huaxin Gao <huaxing@us.ibm.com>
Signed-off-by: HyukjinKwon <gurwls223@apache.org>
## What changes were proposed in this pull request?
This PR adds some tests converted from `natural-join.sql` to test UDFs following the combination guide in [SPARK-27921](https://issues.apache.org/jira/browse/SPARK-27921).
<details><summary>Diff results comparing to `natural-join.sql`</summary>
<p>
```diff
diff --git a/sql/core/src/test/resources/sql-tests/results/udf/udf-natural-join.sql.out b/sql/core/src/test/resources/sql-tests/results/udf/udf-natural-join.
sql.out
index 43f2f9a..53ef177 100644
--- a/sql/core/src/test/resources/sql-tests/results/udf/udf-natural-join.sql.out
+++ b/sql/core/src/test/resources/sql-tests/results/udf/udf-natural-join.sql.out
-27,7 +27,7 struct<>
-- !query 2
-SELECT * FROM nt1 natural join nt2 where k = "one"
+SELECT * FROM nt1 natural join nt2 where udf(k) = "one"
-- !query 2 schema
struct<k:string,v1:int,v2:int>
-- !query 2 output
-36,7 +36,7 one 1 5
-- !query 3
-SELECT * FROM nt1 natural left join nt2 order by v1, v2
+SELECT * FROM nt1 natural left join nt2 where k <> udf("") order by v1, v2
-- !query 3 schema
diff --git a/sql/core/src/test/resources/sql-tests/results/udf/udf-natural-join.sql.out b/sql/core/src/test/resources/sql-tests/results/udf/udf-natural-join.
sql.out
index 43f2f9a..53ef177 100644
--- a/sql/core/src/test/resources/sql-tests/results/udf/udf-natural-join.sql.out
+++ b/sql/core/src/test/resources/sql-tests/results/udf/udf-natural-join.sql.out
-27,7 +27,7 struct<>
-- !query 2
-SELECT * FROM nt1 natural join nt2 where k = "one"
+SELECT * FROM nt1 natural join nt2 where udf(k) = "one"
-- !query 2 schema
struct<k:string,v1:int,v2:int>
-- !query 2 output
-36,7 +36,7 one 1 5
-- !query 3
-SELECT * FROM nt1 natural left join nt2 order by v1, v2
+SELECT * FROM nt1 natural left join nt2 where k <> udf("") order by v1, v2
-- !query 3 schema
struct<k:string,v1:int,v2:int>
```
</p>
</details>
## How was this patch tested?
Tested as guided in [SPARK-27921](https://issues.apache.org/jira/browse/SPARK-27921).
Closes#25088 from manuzhang/SPARK-27922.
Authored-by: manu.zhang <manu.zhang@vipshop.com>
Signed-off-by: HyukjinKwon <gurwls223@apache.org>
## What changes were proposed in this pull request?
There is a bug in `ExtractPythonUDFs` that produces wrong result attributes. It causes a failure when using `PythonUDF`s among multiple child plans, e.g., join. An example is using `PythonUDF`s in join condition.
```python
>>> left = spark.createDataFrame([Row(a=1, a1=1, a2=1), Row(a=2, a1=2, a2=2)])
>>> right = spark.createDataFrame([Row(b=1, b1=1, b2=1), Row(b=1, b1=3, b2=1)])
>>> f = udf(lambda a: a, IntegerType())
>>> df = left.join(right, [f("a") == f("b"), left.a1 == right.b1])
>>> df.collect()
19/07/10 12:20:49 ERROR Executor: Exception in task 5.0 in stage 0.0 (TID 5)
java.lang.ArrayIndexOutOfBoundsException: 1
at org.apache.spark.sql.catalyst.expressions.GenericInternalRow.genericGet(rows.scala:201)
at org.apache.spark.sql.catalyst.expressions.BaseGenericInternalRow.getAs(rows.scala:35)
at org.apache.spark.sql.catalyst.expressions.BaseGenericInternalRow.isNullAt(rows.scala:36)
at org.apache.spark.sql.catalyst.expressions.BaseGenericInternalRow.isNullAt$(rows.scala:36)
at org.apache.spark.sql.catalyst.expressions.GenericInternalRow.isNullAt(rows.scala:195)
at org.apache.spark.sql.catalyst.expressions.JoinedRow.isNullAt(JoinedRow.scala:70)
...
```
## How was this patch tested?
Added test.
Closes#25091 from viirya/SPARK-28323.
Authored-by: Liang-Chi Hsieh <viirya@gmail.com>
Signed-off-by: Bryan Cutler <cutlerb@gmail.com>
## What changes were proposed in this pull request?
`SslContextFactory` is deprecated at Jetty 9.4 and we are using `9.4.18.v20190429`. This PR aims to replace it with `SslContextFactory.Server`.
- https://www.eclipse.org/jetty/javadoc/9.4.19.v20190610/org/eclipse/jetty/util/ssl/SslContextFactory.html
- https://www.eclipse.org/jetty/javadoc/9.3.24.v20180605/org/eclipse/jetty/util/ssl/SslContextFactory.html
```
[WARNING] /Users/dhyun/APACHE/spark/core/src/main/scala/org/apache/spark/SSLOptions.scala:71:
constructor SslContextFactory in class SslContextFactory is deprecated:
see corresponding Javadoc for more information.
[WARNING] val sslContextFactory = new SslContextFactory()
[WARNING] ^
```
## How was this patch tested?
Pass the Jenkins with the existing tests.
Closes#25067 from dongjoon-hyun/SPARK-28290.
Authored-by: Dongjoon Hyun <dhyun@apple.com>
Signed-off-by: Dongjoon Hyun <dhyun@apple.com>
## What changes were proposed in this pull request?
This is a followup of the discussion in https://github.com/apache/spark/pull/24675#discussion_r286786053
`QueryPlan#references` is an important property. The `ColumnPrunning` rule relies on it.
Some query plan nodes have `Seq[Attribute]` parameter, which is used as its output attributes. For example, leaf nodes, `Generate`, `MapPartitionsInPandas`, etc. These nodes override `producedAttributes` to make `missingInputs` correct.
However, these nodes also need to override `references` to make column pruning work. This PR proposes to exclude `producedAttributes` from the default implementation of `QueryPlan#references`, so that we don't need to override `references` in all these nodes.
Note that, technically we can remove `producedAttributes` and always ask query plan nodes to override `references`. But I do find the code can be simpler with `producedAttributes` in some places, where there is a base class for some specific query plan nodes.
## How was this patch tested?
existing tests
Closes#25052 from cloud-fan/minor.
Authored-by: Wenchen Fan <wenchen@databricks.com>
Signed-off-by: HyukjinKwon <gurwls223@apache.org>
## What changes were proposed in this pull request?
This PR adds some tests converted from `pgSQL/case.sql'` to test UDFs. Please see contribution guide of this umbrella ticket - [SPARK-27921](https://issues.apache.org/jira/browse/SPARK-27921).
This PR also contains two minor fixes:
1. Change name of Scala UDF from `UDF:name(...)` to `name(...)` to be consistent with Python'
2. Fix Scala UDF at `IntegratedUDFTestUtils.scala ` to handle `null` in strings.
<details><summary>Diff comparing to 'pgSQL/case.sql'</summary>
<p>
```diff
diff --git a/sql/core/src/test/resources/sql-tests/results/pgSQL/case.sql.out b/sql/core/src/test/resources/sql-tests/results/udf/pgSQL/udf-case.sql.out
index fa078d16d6d..55bef64338f 100644
--- a/sql/core/src/test/resources/sql-tests/results/pgSQL/case.sql.out
+++ b/sql/core/src/test/resources/sql-tests/results/udf/pgSQL/udf-case.sql.out
-115,7 +115,7 struct<>
-- !query 13
SELECT '3' AS `One`,
CASE
- WHEN 1 < 2 THEN 3
+ WHEN CAST(udf(1 < 2) AS boolean) THEN 3
END AS `Simple WHEN`
-- !query 13 schema
struct<One:string,Simple WHEN:int>
-126,10 +126,10 struct<One:string,Simple WHEN:int>
-- !query 14
SELECT '<NULL>' AS `One`,
CASE
- WHEN 1 > 2 THEN 3
+ WHEN 1 > 2 THEN udf(3)
END AS `Simple default`
-- !query 14 schema
-struct<One:string,Simple default:int>
+struct<One:string,Simple default:string>
-- !query 14 output
<NULL> NULL
-137,17 +137,17 struct<One:string,Simple default:int>
-- !query 15
SELECT '3' AS `One`,
CASE
- WHEN 1 < 2 THEN 3
- ELSE 4
+ WHEN udf(1) < 2 THEN udf(3)
+ ELSE udf(4)
END AS `Simple ELSE`
-- !query 15 schema
-struct<One:string,Simple ELSE:int>
+struct<One:string,Simple ELSE:string>
-- !query 15 output
3 3
-- !query 16
-SELECT '4' AS `One`,
+SELECT udf('4') AS `One`,
CASE
WHEN 1 > 2 THEN 3
ELSE 4
-159,10 +159,10 struct<One:string,ELSE default:int>
-- !query 17
-SELECT '6' AS `One`,
+SELECT udf('6') AS `One`,
CASE
- WHEN 1 > 2 THEN 3
- WHEN 4 < 5 THEN 6
+ WHEN CAST(udf(1 > 2) AS boolean) THEN 3
+ WHEN udf(4) < 5 THEN 6
ELSE 7
END AS `Two WHEN with default`
-- !query 17 schema
-173,7 +173,7 struct<One:string,Two WHEN with default:int>
-- !query 18
SELECT '7' AS `None`,
- CASE WHEN rand() < 0 THEN 1
+ CASE WHEN rand() < udf(0) THEN 1
END AS `NULL on no matches`
-- !query 18 schema
struct<None:string,NULL on no matches:int>
-182,36 +182,36 struct<None:string,NULL on no matches:int>
-- !query 19
-SELECT CASE WHEN 1=0 THEN 1/0 WHEN 1=1 THEN 1 ELSE 2/0 END
+SELECT CASE WHEN CAST(udf(1=0) AS boolean) THEN 1/0 WHEN 1=1 THEN 1 ELSE 2/0 END
-- !query 19 schema
-struct<CASE WHEN (1 = 0) THEN (CAST(1 AS DOUBLE) / CAST(0 AS DOUBLE)) WHEN (1 = 1) THEN CAST(1 AS DOUBLE) ELSE (CAST(2 AS DOUBLE) / CAST(0 AS DOUBLE)) END:double>
+struct<CASE WHEN CAST(udf((1 = 0)) AS BOOLEAN) THEN (CAST(1 AS DOUBLE) / CAST(0 AS DOUBLE)) WHEN (1 = 1) THEN CAST(1 AS DOUBLE) ELSE (CAST(2 AS DOUBLE) / CAST(0 AS DOUBLE)) END:double>
-- !query 19 output
1.0
-- !query 20
-SELECT CASE 1 WHEN 0 THEN 1/0 WHEN 1 THEN 1 ELSE 2/0 END
+SELECT CASE 1 WHEN 0 THEN 1/udf(0) WHEN 1 THEN 1 ELSE 2/0 END
-- !query 20 schema
-struct<CASE WHEN (1 = 0) THEN (CAST(1 AS DOUBLE) / CAST(0 AS DOUBLE)) WHEN (1 = 1) THEN CAST(1 AS DOUBLE) ELSE (CAST(2 AS DOUBLE) / CAST(0 AS DOUBLE)) END:double>
+struct<CASE WHEN (1 = 0) THEN (CAST(1 AS DOUBLE) / CAST(CAST(udf(0) AS DOUBLE) AS DOUBLE)) WHEN (1 = 1) THEN CAST(1 AS DOUBLE) ELSE (CAST(2 AS DOUBLE) / CAST(0 AS DOUBLE)) END:double>
-- !query 20 output
1.0
-- !query 21
-SELECT CASE WHEN i > 100 THEN 1/0 ELSE 0 END FROM case_tbl
+SELECT CASE WHEN i > 100 THEN udf(1/0) ELSE udf(0) END FROM case_tbl
-- !query 21 schema
-struct<CASE WHEN (i > 100) THEN (CAST(1 AS DOUBLE) / CAST(0 AS DOUBLE)) ELSE CAST(0 AS DOUBLE) END:double>
+struct<CASE WHEN (i > 100) THEN udf((cast(1 as double) / cast(0 as double))) ELSE udf(0) END:string>
-- !query 21 output
-0.0
-0.0
-0.0
-0.0
+0
+0
+0
+0
-- !query 22
-SELECT CASE 'a' WHEN 'a' THEN 1 ELSE 2 END
+SELECT CASE 'a' WHEN 'a' THEN udf(1) ELSE udf(2) END
-- !query 22 schema
-struct<CASE WHEN (a = a) THEN 1 ELSE 2 END:int>
+struct<CASE WHEN (a = a) THEN udf(1) ELSE udf(2) END:string>
-- !query 22 output
1
-283,7 +283,7 big
-- !query 27
-SELECT * FROM CASE_TBL WHERE COALESCE(f,i) = 4
+SELECT * FROM CASE_TBL WHERE udf(COALESCE(f,i)) = 4
-- !query 27 schema
struct<i:int,f:double>
-- !query 27 output
-291,7 +291,7 struct<i:int,f:double>
-- !query 28
-SELECT * FROM CASE_TBL WHERE NULLIF(f,i) = 2
+SELECT * FROM CASE_TBL WHERE udf(NULLIF(f,i)) = 2
-- !query 28 schema
struct<i:int,f:double>
-- !query 28 output
-299,10 +299,10 struct<i:int,f:double>
-- !query 29
-SELECT COALESCE(a.f, b.i, b.j)
+SELECT udf(COALESCE(a.f, b.i, b.j))
FROM CASE_TBL a, CASE2_TBL b
-- !query 29 schema
-struct<coalesce(f, CAST(i AS DOUBLE), CAST(j AS DOUBLE)):double>
+struct<udf(coalesce(f, cast(i as double), cast(j as double))):string>
-- !query 29 output
-30.3
-30.3
-332,8 +332,8 struct<coalesce(f, CAST(i AS DOUBLE), CAST(j AS DOUBLE)):double>
-- !query 30
SELECT *
- FROM CASE_TBL a, CASE2_TBL b
- WHERE COALESCE(a.f, b.i, b.j) = 2
+ FROM CASE_TBL a, CASE2_TBL b
+ WHERE udf(COALESCE(a.f, b.i, b.j)) = 2
-- !query 30 schema
struct<i:int,f:double,i:int,j:int>
-- !query 30 output
-342,7 +342,7 struct<i:int,f:double,i:int,j:int>
-- !query 31
-SELECT '' AS Five, NULLIF(a.i,b.i) AS `NULLIF(a.i,b.i)`,
+SELECT udf('') AS Five, NULLIF(a.i,b.i) AS `NULLIF(a.i,b.i)`,
NULLIF(b.i, 4) AS `NULLIF(b.i,4)`
FROM CASE_TBL a, CASE2_TBL b
-- !query 31 schema
-377,7 +377,7 struct<Five:string,NULLIF(a.i,b.i):int,NULLIF(b.i,4):int>
-- !query 32
SELECT '' AS `Two`, *
FROM CASE_TBL a, CASE2_TBL b
- WHERE COALESCE(f,b.i) = 2
+ WHERE CAST(udf(COALESCE(f,b.i) = 2) AS boolean)
-- !query 32 schema
struct<Two:string,i:int,f:double,i:int,j:int>
-- !query 32 output
-388,15 +388,15 struct<Two:string,i:int,f:double,i:int,j:int>
-- !query 33
SELECT CASE
(CASE vol('bar')
- WHEN 'foo' THEN 'it was foo!'
- WHEN vol(null) THEN 'null input'
+ WHEN udf('foo') THEN 'it was foo!'
+ WHEN udf(vol(null)) THEN 'null input'
WHEN 'bar' THEN 'it was bar!' END
)
- WHEN 'it was foo!' THEN 'foo recognized'
- WHEN 'it was bar!' THEN 'bar recognized'
- ELSE 'unrecognized' END
+ WHEN udf('it was foo!') THEN 'foo recognized'
+ WHEN 'it was bar!' THEN udf('bar recognized')
+ ELSE 'unrecognized' END AS col
-- !query 33 schema
-struct<CASE WHEN (CASE WHEN (UDF:vol(bar) = foo) THEN it was foo! WHEN (UDF:vol(bar) = UDF:vol(null)) THEN null input WHEN (UDF:vol(bar) = bar) THEN it was bar! END = it was foo!) THEN foo recognized WHEN (CASE WHEN (UDF:vol(bar) = foo) THEN it was foo! WHEN (UDF:vol(bar) = UDF:vol(null)) THEN null input WHEN (UDF:vol(bar) = bar) THEN it was bar! END = it was bar!) THEN bar recognized ELSE unrecognized END:string>
+struct<col:string>
-- !query 33 output
bar recognized
```
</p>
</details>
https://github.com/apache/spark/pull/25069 contains the same minor fixes as it's required to write the tests.
## How was this patch tested?
Tested as guided in [SPARK-27921](https://issues.apache.org/jira/browse/SPARK-27921).
Closes#25070 from HyukjinKwon/SPARK-28273.
Authored-by: HyukjinKwon <gurwls223@apache.org>
Signed-off-by: Wenchen Fan <wenchen@databricks.com>
## What changes were proposed in this pull request?
The sub-second part of the interval should be padded before parsing. Currently, Spark gives a correct value only when there is 9 digits below `.`.
```
spark-sql> select interval '0 0:0:0.123456789' day to second;
interval 123 milliseconds 456 microseconds
spark-sql> select interval '0 0:0:0.12345678' day to second;
interval 12 milliseconds 345 microseconds
spark-sql> select interval '0 0:0:0.1234' day to second;
interval 1 microseconds
```
## How was this patch tested?
Pass the Jenkins with the fixed test cases.
Closes#25079 from dongjoon-hyun/SPARK-28308.
Authored-by: Dongjoon Hyun <dhyun@apple.com>
Signed-off-by: Dongjoon Hyun <dhyun@apple.com>
## What changes were proposed in this pull request?
In Dataset drop(col: Column) method, the `equals` comparison method was used instead of `semanticEquals`, which caused the problem of abnormal case-sensitivity behavior. When attributes of LogicalPlan are checked for equality, `semanticEquals` should be used instead.
A similar PR I referred to: https://github.com/apache/spark/pull/22713 created by mgaido91
## How was this patch tested?
- Added new unit test case in DataFrameSuite
- ./build/sbt "testOnly org.apache.spark.sql.*"
- The python code from ticket reporter at https://issues.apache.org/jira/browse/SPARK-28189Closes#25055 from Tonix517/SPARK-28189.
Authored-by: Tony Zhang <tony.zhang@uber.com>
Signed-off-by: Dongjoon Hyun <dhyun@apple.com>
## What changes were proposed in this pull request?
We changed our non-standard syntax for `trim` function in #24902 from `TRIM(trimStr, str)` to `TRIM(str, trimStr)` to be compatible with other databases. This pr update the migration guide.
I checked various databases(PostgreSQL, Teradata, Vertica, Oracle, DB2, SQL Server 2019, MySQL, Hive, Presto) and it seems that only PostgreSQL and Presto support this non-standard syntax.
**PostgreSQL**:
```sql
postgres=# select substr(version(), 0, 16), trim('yxTomxx', 'x');
substr | btrim
-----------------+-------
PostgreSQL 11.3 | yxTom
(1 row)
```
**Presto**:
```sql
presto> select trim('yxTomxx', 'x');
_col0
-------
yxTom
(1 row)
```
## How was this patch tested?
manual tests
Closes#24948 from wangyum/SPARK-28093-FOLLOW-UP-DOCS.
Authored-by: Yuming Wang <yumwang@ebay.com>
Signed-off-by: Dongjoon Hyun <dhyun@apple.com>
## What changes were proposed in this pull request?
This PR adds some more WITH test cases as a follow-up to https://github.com/apache/spark/pull/24842
## How was this patch tested?
Add new UTs.
Closes#24949 from peter-toth/SPARK-28002-follow-up.
Authored-by: Peter Toth <peter.toth@gmail.com>
Signed-off-by: Dongjoon Hyun <dhyun@apple.com>
## What changes were proposed in this pull request?
- Currently, `ExpressionEncoder` does not handle bigdecimal overflow. Round-tripping overflowing java/scala BigDecimal/BigInteger returns null.
- The serializer encode java/scala BigDecimal to to sql Decimal, which still has the underlying data to the former.
- When writing out to UnsafeRow, `changePrecision` will be false and row has null value.
24e1e41648/sql/catalyst/src/main/java/org/apache/spark/sql/catalyst/expressions/codegen/UnsafeRowWriter.java (L202-L206)
- In [SPARK-23179](https://github.com/apache/spark/pull/20350), an option to throw exception on decimal overflow was introduced.
- This PR adds the option in `ExpressionEncoder` to throw when detecting overflowing BigDecimal/BigInteger before its corresponding Decimal gets written to Row. This gives a consistent behavior between decimal arithmetic on sql expression (DecimalPrecision), and getting decimal from dataframe (RowEncoder)
Thanks to mgaido91 for the very first PR `SPARK-23179` and follow-up discussion on this change.
Thanks to JoshRosen for working with me on this.
## How was this patch tested?
added unit tests
Closes#25016 from mickjermsurawong-stripe/SPARK-28200.
Authored-by: Mick Jermsurawong <mickjermsurawong@stripe.com>
Signed-off-by: Wenchen Fan <wenchen@databricks.com>
## What changes were proposed in this pull request?
This PR proposes to rename `mapPartitionsInPandas` to `mapInPandas` with a separate evaluation type .
Had an offline discussion with rxin, mengxr and cloud-fan
The reason is basically:
1. `SCALAR_ITER` doesn't make sense with `mapPartitionsInPandas`.
2. It cannot share the same Pandas UDF, for instance, at `select` and `mapPartitionsInPandas` unlike `GROUPED_AGG` because iterator's return type is different.
3. `mapPartitionsInPandas` -> `mapInPandas` - see https://github.com/apache/spark/pull/25044#issuecomment-508298552 and https://github.com/apache/spark/pull/25044#issuecomment-508299764
Renaming `SCALAR_ITER` as `MAP_ITER` is abandoned due to 2. reason.
For `XXX_ITER`, it might have to have a different interface in the future if we happen to add other versions of them. But this is an orthogonal topic with `mapPartitionsInPandas`.
## How was this patch tested?
Existing tests should cover.
Closes#25044 from HyukjinKwon/SPARK-28198.
Authored-by: HyukjinKwon <gurwls223@apache.org>
Signed-off-by: HyukjinKwon <gurwls223@apache.org>
## What changes were proposed in this pull request?
Before this PR inserting into a non-existing table returned a weird error message:
```
sql("INSERT INTO test VALUES (1)").show
org.apache.spark.sql.AnalysisException: unresolved operator 'InsertIntoTable 'UnresolvedRelation [test], false, false;;
'InsertIntoTable 'UnresolvedRelation [test], false, false
+- LocalRelation [col1#4]
```
after this PR the error message becomes:
```
org.apache.spark.sql.AnalysisException: Table not found: test;;
'InsertIntoTable 'UnresolvedRelation [test], false, false
+- LocalRelation [col1#0]
```
## How was this patch tested?
Added a new UT.
Closes#25054 from peter-toth/SPARK-28251.
Authored-by: Peter Toth <peter.toth@gmail.com>
Signed-off-by: Dongjoon Hyun <dhyun@apple.com>
## What changes were proposed in this pull request?
This PR adds support of `WITH` clause within a subquery so this query becomes valid:
```
SELECT max(c) FROM (
WITH t AS (SELECT 1 AS c)
SELECT * FROM t
)
```
## How was this patch tested?
Added new UTs.
Closes#24831 from peter-toth/SPARK-19799-2.
Authored-by: Peter Toth <peter.toth@gmail.com>
Signed-off-by: Dongjoon Hyun <dhyun@apple.com>
## What changes were proposed in this pull request?
This is to implement a ReduceNumShufflePartitions rule in the new adaptive execution framework introduced in #24706. This rule is used to adjust the post shuffle partitions based on the map output statistics.
## How was this patch tested?
Added ReduceNumShufflePartitionsSuite
Closes#24978 from carsonwang/reduceNumShufflePartitions.
Authored-by: Carson Wang <carson.wang@intel.com>
Signed-off-by: Wenchen Fan <wenchen@databricks.com>
## What changes were proposed in this pull request?
This pr add calculate local directory size to `SQLTestUtils`.
We can avoid these changes after this pr:
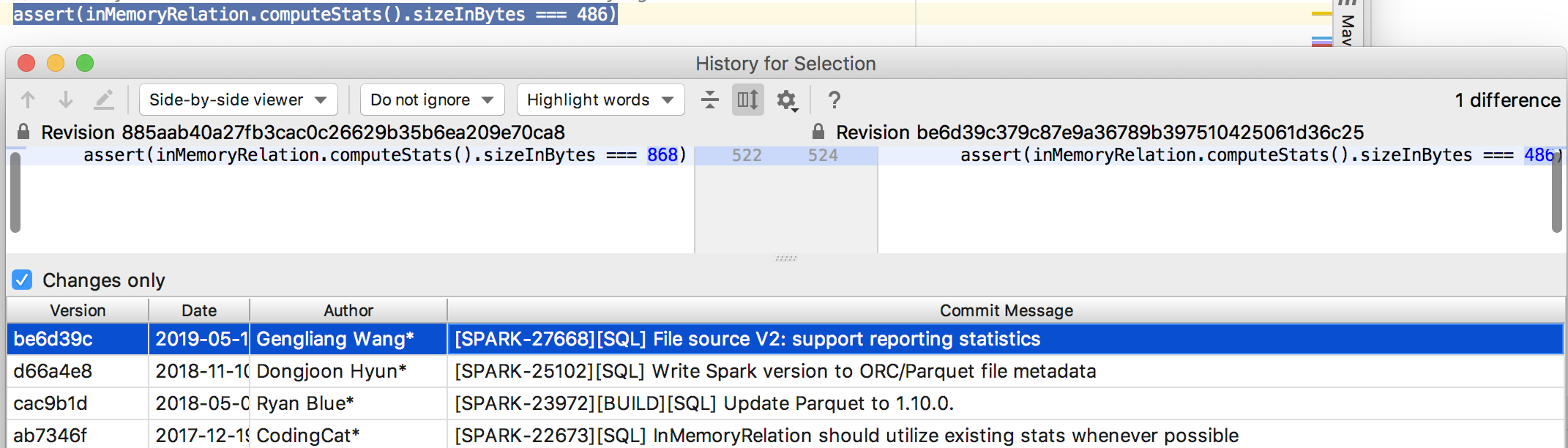
## How was this patch tested?
Existing test
Closes#25014 from wangyum/SPARK-28216.
Authored-by: Yuming Wang <yumwang@ebay.com>
Signed-off-by: Dongjoon Hyun <dhyun@apple.com>
## What changes were proposed in this pull request?
This PR makes the predicate pushdown logic in catalyst optimizer more efficient by unifying two existing rules `PushdownPredicates` and `PushPredicateThroughJoin`. Previously pushing down a predicate for queries such as `Filter(Join(Join(Join)))` requires n steps. This patch essentially reduces this to a single pass.
To make this actually work, we need to unify a few rules such as `CombineFilters`, `PushDownPredicate` and `PushDownPrdicateThroughJoin`. Otherwise cases such as `Filter(Join(Filter(Join)))` still requires several passes to fully push down predicates. This unification is done by composing several partial functions, which makes a minimal code change and can reuse existing UTs.
Results show that this optimization can improve the catalyst optimization time by 16.5%. For queries with more joins, the performance is even better. E.g., for TPC-DS q64, the performance boost is 49.2%.
## How was this patch tested?
Existing UTs + new a UT for the new rule.
Closes#24956 from yeshengm/fixed-point-opt.
Authored-by: Yesheng Ma <kimi.ysma@gmail.com>
Signed-off-by: gatorsmile <gatorsmile@gmail.com>
## What changes were proposed in this pull request?
This pr add `PLACING` to `ansiNonReserved` and add `overlay` and `placing` to `TableIdentifierParserSuite`.
## How was this patch tested?
N/A
Closes#25013 from wangyum/SPARK-28077.
Authored-by: Yuming Wang <yumwang@ebay.com>
Signed-off-by: Dongjoon Hyun <dhyun@apple.com>
## What changes were proposed in this pull request?
This pr add support show global temporary view and local temporary view in database tool.
TODO: Database tools should support show temporary views because it's schema is null.
## How was this patch tested?
unit tests and manual tests:
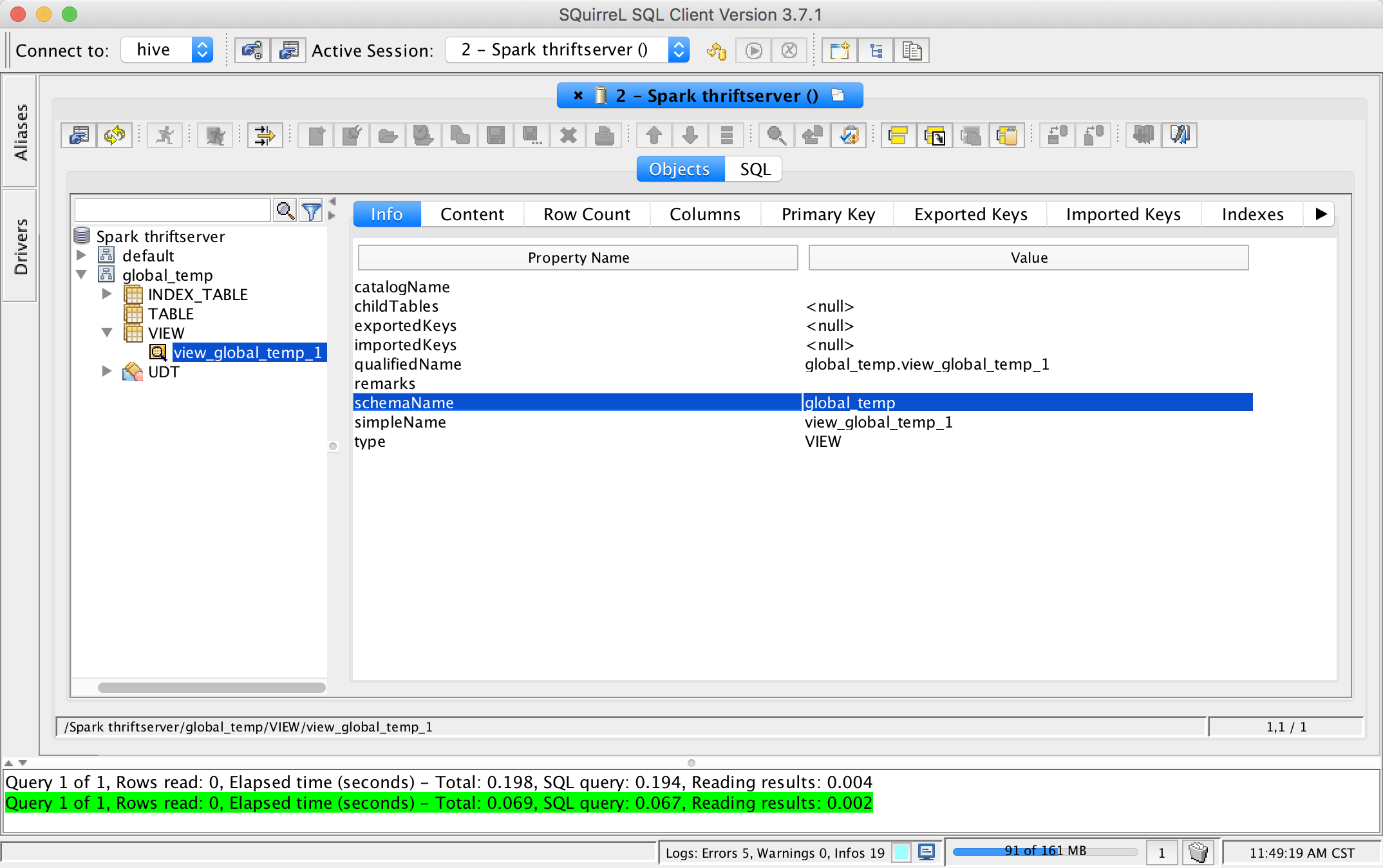
Closes#24972 from wangyum/SPARK-28167.
Authored-by: Yuming Wang <yumwang@ebay.com>
Signed-off-by: gatorsmile <gatorsmile@gmail.com>
## What changes were proposed in this pull request?
Right now they fail only for inner joins, because we implemented the check when that was the only supported type.
## How was this patch tested?
new unit test
Closes#25023 from jose-torres/changevalidation.
Authored-by: Jose Torres <torres.joseph.f+github@gmail.com>
Signed-off-by: Jose Torres <torres.joseph.f+github@gmail.com>
## What changes were proposed in this pull request?
In some cases, executeTake in SparkPlan could decode more than necessary.
For example, in case of below odd/even number partitioning, total row's count from partitions will be 100, although it is limited with 51. And 'executeTake' in SparkPlan decodes all of them, "49" rows of which are unnecessarily decoded.
```scala
spark.sparkContext.parallelize((0 until 100).map(i => (i, 1))).toDF()
.repartitionByRange(2, $"_1" % 2).limit(51).collect()
```
By using a iterator of the scalar collection, we can make ensure that at most n rows are decoded.
## How was this patch tested?
Existing unit tests that call limit function of DataFrame.
testOnly *SQLQuerySuite
testOnly *DataFrameSuite
Closes#22347 from Dooyoung-Hwang/refactor_execute_take.
Authored-by: Dooyoung Hwang <dooyoung.hwang@sk.com>
Signed-off-by: Wenchen Fan <wenchen@databricks.com>
## What changes were proposed in this pull request?
This is a small follow-up for SPARK-28054 to fix wrong indent and use `withSQLConf` as suggested by gatorsmile.
## How was this patch tested?
Existing tests.
Closes#24971 from viirya/SPARK-28054-followup.
Authored-by: Liang-Chi Hsieh <viirya@gmail.com>
Signed-off-by: HyukjinKwon <gurwls223@apache.org>
## What changes were proposed in this pull request?
This PR proposes to add `mapPartitionsInPandas` API to DataFrame by using existing `SCALAR_ITER` as below:
1. Filtering via setting the column
```python
from pyspark.sql.functions import pandas_udf, PandasUDFType
df = spark.createDataFrame([(1, 21), (2, 30)], ("id", "age"))
pandas_udf(df.schema, PandasUDFType.SCALAR_ITER)
def filter_func(iterator):
for pdf in iterator:
yield pdf[pdf.id == 1]
df.mapPartitionsInPandas(filter_func).show()
```
```
+---+---+
| id|age|
+---+---+
| 1| 21|
+---+---+
```
2. `DataFrame.loc`
```python
from pyspark.sql.functions import pandas_udf, PandasUDFType
import pandas as pd
df = spark.createDataFrame([['aa'], ['bb'], ['cc'], ['aa'], ['aa'], ['aa']], ["value"])
pandas_udf(df.schema, PandasUDFType.SCALAR_ITER)
def filter_func(iterator):
for pdf in iterator:
yield pdf.loc[pdf.value.str.contains('^a'), :]
df.mapPartitionsInPandas(filter_func).show()
```
```
+-----+
|value|
+-----+
| aa|
| aa|
| aa|
| aa|
+-----+
```
3. `pandas.melt`
```python
from pyspark.sql.functions import pandas_udf, PandasUDFType
import pandas as pd
df = spark.createDataFrame(
pd.DataFrame({'A': {0: 'a', 1: 'b', 2: 'c'},
'B': {0: 1, 1: 3, 2: 5},
'C': {0: 2, 1: 4, 2: 6}}))
pandas_udf("A string, variable string, value long", PandasUDFType.SCALAR_ITER)
def filter_func(iterator):
for pdf in iterator:
import pandas as pd
yield pd.melt(pdf, id_vars=['A'], value_vars=['B', 'C'])
df.mapPartitionsInPandas(filter_func).show()
```
```
+---+--------+-----+
| A|variable|value|
+---+--------+-----+
| a| B| 1|
| a| C| 2|
| b| B| 3|
| b| C| 4|
| c| B| 5|
| c| C| 6|
+---+--------+-----+
```
The current limitation of `SCALAR_ITER` is that it doesn't allow different length of result, which is pretty critical in practice - for instance, we cannot simply filter by using Pandas APIs but we merely just map N to N. This PR allows map N to M like flatMap.
This API mimics the way of `mapPartitions` but keeps API shape of `SCALAR_ITER` by allowing different results.
### How does this PR implement?
This PR adds mimics both `dapply` with Arrow optimization and Grouped Map Pandas UDF. At Python execution side, it reuses existing `SCALAR_ITER` code path.
Therefore, externally, we don't introduce any new type of Pandas UDF but internally we use another evaluation type code `205` (`SQL_MAP_PANDAS_ITER_UDF`).
This approach is similar with Pandas' Windows function implementation with Grouped Aggregation Pandas UDF functions - internally we have `203` (`SQL_WINDOW_AGG_PANDAS_UDF`) but externally we just share the same `GROUPED_AGG`.
## How was this patch tested?
Manually tested and unittests were added.
Closes#24997 from HyukjinKwon/scalar-udf-iter.
Authored-by: HyukjinKwon <gurwls223@apache.org>
Signed-off-by: HyukjinKwon <gurwls223@apache.org>
## What changes were proposed in this pull request?
Kafka batch data source is using v1 at the moment. In the PR I've migrated to v2. Majority of the change is moving code.
What this PR contains:
* useV1Sources usage fixed in `DataFrameReader` and `DataFrameWriter`
* `KafkaBatch` added to handle DSv2 batch reading
* `KafkaBatchWrite` added to handle DSv2 batch writing
* `KafkaBatchPartitionReader` extracted to share between batch and microbatch
* `KafkaDataWriter` extracted to share between batch, microbatch and continuous
* Batch related source/sink tests are now executing on v1 and v2 connectors
* Couple of classes hidden now, functions moved + couple of minor fixes
## How was this patch tested?
Existing + added unit tests.
Closes#24738 from gaborgsomogyi/SPARK-23098.
Authored-by: Gabor Somogyi <gabor.g.somogyi@gmail.com>
Signed-off-by: Wenchen Fan <wenchen@databricks.com>
## What changes were proposed in this pull request?
In the migration PR of Kafka V2: ac16c9a9ef (r298470645)
We find that the useV1SourceList configuration(spark.sql.sources.read.useV1SourceList and spark.sql.sources.write.useV1SourceList) should be for all data sources, instead of file source V2 only.
This PR is to fix it in DataFrameWriter/DataFrameReader.
## How was this patch tested?
Unit test
Closes#25004 from gengliangwang/reviseUseV1List.
Authored-by: Gengliang Wang <gengliang.wang@databricks.com>
Signed-off-by: Wenchen Fan <wenchen@databricks.com>
## What changes were proposed in this pull request?
In SPARK-23179, it has been introduced a flag to control the behavior in case of overflow on decimals. The behavior is: returning `null` when `spark.sql.decimalOperations.nullOnOverflow` (default and traditional Spark behavior); throwing an `ArithmeticException` if that conf is false (according to SQL standards, other DBs behavior).
`MakeDecimal` so far had an ambiguous behavior. In case of codegen mode, it returned `null` as the other operators, but in interpreted mode, it was throwing an `IllegalArgumentException`.
The PR aligns `MakeDecimal`'s behavior with the one of other operators as defined in SPARK-23179. So now both modes return `null` or throw `ArithmeticException` according to `spark.sql.decimalOperations.nullOnOverflow`'s value.
Credits for this PR to mickjermsurawong-stripe who pointed out the wrong behavior in #20350.
## How was this patch tested?
improved UTs
Closes#25010 from mgaido91/SPARK-28201.
Authored-by: Marco Gaido <marcogaido91@gmail.com>
Signed-off-by: Wenchen Fan <wenchen@databricks.com>
## What changes were proposed in this pull request?
This pr add two API for [SessionCatalog](df4cb471c9/sql/catalyst/src/main/scala/org/apache/spark/sql/catalyst/catalog/SessionCatalog.scala):
```scala
def listTables(db: String, pattern: String, includeLocalTempViews: Boolean): Seq[TableIdentifier]
def listLocalTempViews(pattern: String): Seq[TableIdentifier]
```
Because in some cases `listTables` does not need local temporary view and sometimes only need list local temporary view.
## How was this patch tested?
unit tests
Closes#24995 from wangyum/SPARK-28196.
Authored-by: Yuming Wang <yumwang@ebay.com>
Signed-off-by: gatorsmile <gatorsmile@gmail.com>
## What changes were proposed in this pull request?
To make the #24972 change smaller. This pr improves `SparkMetadataOperationSuite` to avoid creating new sessions when getSchemas/getTables/getColumns.
## How was this patch tested?
N/A
Closes#24985 from wangyum/SPARK-28184.
Authored-by: Yuming Wang <yumwang@ebay.com>
Signed-off-by: gatorsmile <gatorsmile@gmail.com>
## What changes were proposed in this pull request?
Currently, ORC's `inferSchema` is implemented as randomly choosing one ORC file and reading its schema.
This PR follows the behavior of Parquet, it implements merge schemas logic by reading all ORC files in parallel through a spark job.
Users can enable merge schema by `spark.read.orc("xxx").option("mergeSchema", "true")` or by setting `spark.sql.orc.mergeSchema` to `true`, the prior one has higher priority.
## How was this patch tested?
tested by UT OrcUtilsSuite.scala
Closes#24043 from WangGuangxin/SPARK-11412.
Lead-authored-by: wangguangxin.cn <wangguangxin.cn@gmail.com>
Co-authored-by: wangguangxin.cn <wangguangxin.cn@bytedance.com>
Signed-off-by: gatorsmile <gatorsmile@gmail.com>
## What changes were proposed in this pull request?
SPARK-27534 missed to address my own comments at https://github.com/WeichenXu123/spark/pull/8
It's better to push this in since the codes are already cleaned up.
## How was this patch tested?
Unittests fixed
Closes#25003 from HyukjinKwon/SPARK-27534.
Authored-by: HyukjinKwon <gurwls223@apache.org>
Signed-off-by: HyukjinKwon <gurwls223@apache.org>
## What changes were proposed in this pull request?
This is the first part of [SPARK-27396](https://issues.apache.org/jira/browse/SPARK-27396). This is the minimum set of changes necessary to support a pluggable back end for columnar processing. Follow on JIRAs would cover removing some of the duplication between functionality in this patch and functionality currently covered by things like ColumnarBatchScan.
## How was this patch tested?
I added in a new unit test to cover new code not really covered in other places.
I also did manual testing by implementing two plugins/extensions that take advantage of the new APIs to allow for columnar processing for some simple queries. One version runs on the [CPU](https://gist.github.com/revans2/c3cad77075c4fa5d9d271308ee2f1b1d). The other version run on a GPU, but because it has unreleased dependencies I will not include a link to it yet.
The CPU version I would expect to add in as an example with other documentation in a follow on JIRA
This is contributed on behalf of NVIDIA Corporation.
Closes#24795 from revans2/columnar-basic.
Authored-by: Robert (Bobby) Evans <bobby@apache.org>
Signed-off-by: Thomas Graves <tgraves@apache.org>
## What changes were proposed in this pull request?
The `OVERLAY` function is a `ANSI` `SQL`.
For example:
```
SELECT OVERLAY('abcdef' PLACING '45' FROM 4);
SELECT OVERLAY('yabadoo' PLACING 'daba' FROM 5);
SELECT OVERLAY('yabadoo' PLACING 'daba' FROM 5 FOR 0);
SELECT OVERLAY('babosa' PLACING 'ubb' FROM 2 FOR 4);
```
The results of the above four `SQL` are:
```
abc45f
yabadaba
yabadabadoo
bubba
```
Note: If the input string is null, then the result is null too.
There are some mainstream database support the syntax.
**PostgreSQL:**
https://www.postgresql.org/docs/11/functions-string.html
**Vertica:** https://www.vertica.com/docs/9.2.x/HTML/Content/Authoring/SQLReferenceManual/Functions/String/OVERLAY.htm?zoom_highlight=overlay
**Oracle:**
https://docs.oracle.com/en/database/oracle/oracle-database/19/arpls/UTL_RAW.html#GUID-342E37E7-FE43-4CE1-A0E9-7DAABD000369
**DB2:**
https://www.ibm.com/support/knowledgecenter/SSGMCP_5.3.0/com.ibm.cics.rexx.doc/rexx/overlay.html
There are some show of the PR on my production environment.
```
spark-sql> SELECT OVERLAY('abcdef' PLACING '45' FROM 4);
abc45f
Time taken: 6.385 seconds, Fetched 1 row(s)
spark-sql> SELECT OVERLAY('yabadoo' PLACING 'daba' FROM 5);
yabadaba
Time taken: 0.191 seconds, Fetched 1 row(s)
spark-sql> SELECT OVERLAY('yabadoo' PLACING 'daba' FROM 5 FOR 0);
yabadabadoo
Time taken: 0.186 seconds, Fetched 1 row(s)
spark-sql> SELECT OVERLAY('babosa' PLACING 'ubb' FROM 2 FOR 4);
bubba
Time taken: 0.151 seconds, Fetched 1 row(s)
spark-sql> SELECT OVERLAY(null PLACING '45' FROM 4);
NULL
Time taken: 0.22 seconds, Fetched 1 row(s)
spark-sql> SELECT OVERLAY(null PLACING 'daba' FROM 5);
NULL
Time taken: 0.157 seconds, Fetched 1 row(s)
spark-sql> SELECT OVERLAY(null PLACING 'daba' FROM 5 FOR 0);
NULL
Time taken: 0.254 seconds, Fetched 1 row(s)
spark-sql> SELECT OVERLAY(null PLACING 'ubb' FROM 2 FOR 4);
NULL
Time taken: 0.159 seconds, Fetched 1 row(s)
```
## How was this patch tested?
Exists UT and new UT.
Closes#24918 from beliefer/ansi-sql-overlay.
Lead-authored-by: gengjiaan <gengjiaan@360.cn>
Co-authored-by: Jiaan Geng <beliefer@163.com>
Signed-off-by: Takuya UESHIN <ueshin@databricks.com>
## What changes were proposed in this pull request?
Avoid hard-coded config: `spark.sql.globalTempDatabase`.
## How was this patch tested?
N/A
Closes#24979 from wangyum/SPARK-28179.
Authored-by: Yuming Wang <yumwang@ebay.com>
Signed-off-by: HyukjinKwon <gurwls223@apache.org>
## What changes were proposed in this pull request?
For simplicity, all `LambdaVariable`s are globally unique, to avoid any potential conflicts. However, this causes a perf problem: we can never hit codegen cache for encoder expressions that deal with collections (which means they contain `LambdaVariable`).
To overcome this problem, `LambdaVariable` should have per-query unique IDs. This PR does 2 things:
1. refactor `LambdaVariable` to carry an ID, so that it's easier to change the ID.
2. add an optimizer rule to reassign `LambdaVariable` IDs, which are per-query unique.
## How was this patch tested?
new tests
Closes#24735 from cloud-fan/dataset.
Authored-by: Wenchen Fan <wenchen@databricks.com>
Signed-off-by: gatorsmile <gatorsmile@gmail.com>
## What changes were proposed in this pull request?
SQL ANSI 2011 states that in case of overflow during arithmetic operations, an exception should be thrown. This is what most of the SQL DBs do (eg. SQLServer, DB2). Hive currently returns NULL (as Spark does) but HIVE-18291 is open to be SQL compliant.
The PR introduce an option to decide which behavior Spark should follow, ie. returning NULL on overflow or throwing an exception.
## How was this patch tested?
added UTs
Closes#20350 from mgaido91/SPARK-23179.
Authored-by: Marco Gaido <marcogaido91@gmail.com>
Signed-off-by: Wenchen Fan <wenchen@databricks.com>
## What changes were proposed in this pull request?
Currently with `toLocalIterator()` and `toPandas()` with Arrow enabled, if the Spark job being run in the background serving thread errors, it will be caught and sent to Python through the PySpark serializer.
This is not the ideal solution because it is only catch a SparkException, it won't handle an error that occurs in the serializer, and each method has to have it's own special handling to propagate the error.
This PR instead returns the Python Server object along with the serving port and authentication info, so that it allows the Python caller to join with the serving thread. During the call to join, the serving thread Future is completed either successfully or with an exception. In the latter case, the exception will be propagated to Python through the Py4j call.
## How was this patch tested?
Existing tests
Closes#24834 from BryanCutler/pyspark-propagate-server-error-SPARK-27992.
Authored-by: Bryan Cutler <cutlerb@gmail.com>
Signed-off-by: Bryan Cutler <cutlerb@gmail.com>
## What changes were proposed in this pull request?
Spark's `InMemoryFileIndex` contains two places where `FileNotFound` exceptions are caught and logged as warnings (during [directory listing](bcd3b61c4b/sql/core/src/main/scala/org/apache/spark/sql/execution/datasources/InMemoryFileIndex.scala (L274)) and [block location lookup](bcd3b61c4b/sql/core/src/main/scala/org/apache/spark/sql/execution/datasources/InMemoryFileIndex.scala (L333))). This logic was added in #15153 and #21408.
I think that this is a dangerous default behavior because it can mask bugs caused by race conditions (e.g. overwriting a table while it's being read) or S3 consistency issues (there's more discussion on this in the [JIRA ticket](https://issues.apache.org/jira/browse/SPARK-27676)). Failing fast when we detect missing files is not sufficient to make concurrent table reads/writes or S3 listing safe (there are other classes of eventual consistency issues to worry about), but I think it's still beneficial to throw exceptions and fail-fast on the subset of inconsistencies / races that we _can_ detect because that increases the likelihood that an end user will notice the problem and investigate further.
There may be some cases where users _do_ want to ignore missing files, but I think that should be an opt-in behavior via the existing `spark.sql.files.ignoreMissingFiles` flag (the current behavior is itself race-prone because a file might be be deleted between catalog listing and query execution time, triggering FileNotFoundExceptions on executors (which are handled in a way that _does_ respect `ignoreMissingFIles`)).
This PR updates `InMemoryFileIndex` to guard the log-and-ignore-FileNotFoundException behind the existing `spark.sql.files.ignoreMissingFiles` flag.
**Note**: this is a change of default behavior, so I think it needs to be mentioned in release notes.
## How was this patch tested?
New unit tests to simulate file-deletion race conditions, tested with both values of the `ignoreMissingFIles` flag.
Closes#24668 from JoshRosen/SPARK-27676.
Lead-authored-by: Josh Rosen <rosenville@gmail.com>
Co-authored-by: Josh Rosen <joshrosen@stripe.com>
Signed-off-by: HyukjinKwon <gurwls223@apache.org>
continuation to https://github.com/apache/spark/pull/24788
## What changes were proposed in this pull request?
Changes are related to BIG ENDIAN system
This changes are done to
identify s390x platform.
use byteorder to BIG_ENDIAN for big endian systems
changes for 2 are done in access functions putFloats() and putDouble()
## How was this patch tested?
Changes have been tested to build successfully on s390x as well x86 platform to make sure build is successful.
Closes#24861 from ketank-new/ketan_latest_v2.3.2.
Authored-by: ketank-new <ketan22584@gmail.com>
Signed-off-by: Sean Owen <sean.owen@databricks.com>
## What changes were proposed in this pull request?
After this PR, we can test Pandas and Python UDF as below **in Scala side**:
```scala
import IntegratedUDFTestUtils._
val pandasTestUDF = TestScalarPandasUDF("udf")
spark.range(10).select(pandasTestUDF($"id")).show()
```
## How was this patch tested?
Manually tested.
Closes#24945 from HyukjinKwon/SPARK-27893-followup.
Authored-by: HyukjinKwon <gurwls223@apache.org>
Signed-off-by: HyukjinKwon <gurwls223@apache.org>
## What changes were proposed in this pull request?
In #24068, IvanVergiliev fixes the issue that OrcFilters.createBuilder has exponential complexity in the height of the filter tree due to the way the check-and-build pattern is implemented.
Comparing to the approach in #24068, I propose a simple solution for the issue:
1. separate the logic of building a convertible filter tree and the actual SearchArgument builder, since the two procedures are different and their return types are different. Thus the new introduced class `ActionType`,`TrimUnconvertibleFilters` and `BuildSearchArgument` in #24068 can be dropped. The code is more readable.
2. For most of the leaf nodes, the convertible result is always Some(node), we can abstract it like this PR.
3. The code is actually small changes on the previous code. See https://github.com/apache/spark/pull/24783
## How was this patch tested?
Run the benchmark provided in #24068:
```
val schema = StructType.fromDDL("col INT")
(20 to 30).foreach { width =>
val whereFilter = (1 to width).map(i => EqualTo("col", i)).reduceLeft(Or)
val start = System.currentTimeMillis()
OrcFilters.createFilter(schema, Seq(whereFilter))
println(s"With $width filters, conversion takes ${System.currentTimeMillis() - start} ms")
}
```
Result:
```
With 20 filters, conversion takes 6 ms
With 21 filters, conversion takes 0 ms
With 22 filters, conversion takes 0 ms
With 23 filters, conversion takes 0 ms
With 24 filters, conversion takes 0 ms
With 25 filters, conversion takes 0 ms
With 26 filters, conversion takes 0 ms
With 27 filters, conversion takes 0 ms
With 28 filters, conversion takes 0 ms
With 29 filters, conversion takes 0 ms
With 30 filters, conversion takes 0 ms
```
Also verified with Unit tests.
Closes#24910 from gengliangwang/refactorOrcFilters.
Authored-by: Gengliang Wang <gengliang.wang@databricks.com>
Signed-off-by: Wenchen Fan <wenchen@databricks.com>
## What changes were proposed in this pull request?
When we use upper case partition name in Hive table, like:
```
CREATE TABLE src (KEY STRING, VALUE STRING) PARTITIONED BY (DS STRING)
```
Then, `insert into table` query doesn't work
```
INSERT INTO TABLE src PARTITION(ds) SELECT 'k' key, 'v' value, '1' ds
// or
INSERT INTO TABLE src PARTITION(DS) SELECT 'k' KEY, 'v' VALUE, '1' DS
```
```
[info] org.apache.spark.sql.AnalysisException:
org.apache.hadoop.hive.ql.metadata.Table.ValidationFailureSemanticException: Partition spec {ds=, DS=1} contains non-partition columns;
```
As Hive metastore is not case preserving and keeps partition columns with lower cased names, we lowercase column names in partition spec before passing to Hive client. But we write upper case column names in partition paths.
However, when calling `loadDynamicPartitions` to do `insert into table` for dynamic partition, Hive calculates full path spec for partition paths. So it calculates a partition spec like `{ds=, DS=1}` in above case and fails partition column validation. This patch is proposed to fix the issue by lowercasing the column names in written partition paths for Hive partitioned table.
This fix touchs `saveAsHiveFile` method, which is used in `InsertIntoHiveDirCommand` and `InsertIntoHiveTable` commands. Among them, only `InsertIntoHiveTable` passes `partitionAttributes` parameter. So I think this change only affects `InsertIntoHiveTable` command.
## How was this patch tested?
Added test.
Closes#24886 from viirya/SPARK-28054.
Authored-by: Liang-Chi Hsieh <viirya@gmail.com>
Signed-off-by: HyukjinKwon <gurwls223@apache.org>
## What changes were proposed in this pull request?
ShuffleMapTask's partition field is a FilePartition and FilePartition's 'files' field is a Stream$cons which is essentially a linked list. It is therefore serialized recursively.
If the number of files in each partition is, say, 10000 files, recursing into a linked list of length 10000 overflows the stack
The problem is only in Bucketed partitions. The corresponding implementation for non Bucketed partitions uses a StreamBuffer. The proposed change applies the same for Bucketed partitions.
## How was this patch tested?
Existing unit tests. Added new unit test. The unit test fails without the patch. Manual testing on dataset used to reproduce the problem.
Closes#24865 from parthchandra/SPARK-27100.
Lead-authored-by: Parth Chandra <parthc@apple.com>
Co-authored-by: Dongjoon Hyun <dhyun@apple.com>
Signed-off-by: DB Tsai <d_tsai@apple.com>
## What changes were proposed in this pull request?
[PostgreSQL](7c850320d8/src/test/regress/sql/strings.sql (L624)) support another trim pattern: `TRIM(trimStr FROM str)`:
Function | Return Type | Description | Example | Result
--- | --- | --- | --- | ---
trim([leading \| trailing \| both] [characters] from string) | text | Remove the longest string containing only characters from characters (a space by default) from the start, end, or both ends (both is the default) of string | trim(both 'xyz' from 'yxTomxx') | Tom
This pr add support this trim pattern. After this pr. We can support all standard syntax except `TRIM(FROM str)` because it conflicts with our Literals:
```sql
Literals of type 'FROM' are currently not supported.(line 1, pos 12)
== SQL ==
SELECT TRIM(FROM ' SPARK SQL ')
```
PostgreSQL, Vertica and MySQL support this pattern. Teradata, Oracle, DB2, SQL Server, Hive and Presto
**PostgreSQL**:
```
postgres=# SELECT substr(version(), 0, 16), trim('xyz' FROM 'yxTomxx');
substr | btrim
-----------------+-------
PostgreSQL 11.3 | Tom
(1 row)
```
**Vertica**:
```
dbadmin=> SELECT version(), trim('xyz' FROM 'yxTomxx');
version | btrim
------------------------------------+-------
Vertica Analytic Database v9.1.1-0 | Tom
(1 row)
```
**MySQL**:
```
mysql> SELECT version(), trim('xyz' FROM 'yxTomxx');
+-----------+----------------------------+
| version() | trim('xyz' FROM 'yxTomxx') |
+-----------+----------------------------+
| 5.7.26 | yxTomxx |
+-----------+----------------------------+
1 row in set (0.00 sec)
```
More details:
https://www.postgresql.org/docs/11/functions-string.html
## How was this patch tested?
unit tests
Closes#24924 from wangyum/SPARK-28075-2.
Authored-by: Yuming Wang <yumwang@ebay.com>
Signed-off-by: gatorsmile <gatorsmile@gmail.com>
## What changes were proposed in this pull request?
When running FlatMapGroupsInPandasExec or AggregateInPandasExec the shuffle uses a default number of partitions of 200 in "spark.sql.shuffle.partitions". If the data is small, e.g. in testing, many of the partitions will be empty but are treated just the same.
This PR checks the `mapPartitionsInternal` iterator to be non-empty before calling `ArrowPythonRunner` to start computation on the iterator.
## How was this patch tested?
Existing tests. Ran the following benchmarks a simple example where most partitions are empty:
```python
from pyspark.sql.functions import pandas_udf, PandasUDFType
from pyspark.sql.types import *
df = spark.createDataFrame(
[(1, 1.0), (1, 2.0), (2, 3.0), (2, 5.0), (2, 10.0)],
("id", "v"))
pandas_udf("id long, v double", PandasUDFType.GROUPED_MAP)
def normalize(pdf):
v = pdf.v
return pdf.assign(v=(v - v.mean()) / v.std())
df.groupby("id").apply(normalize).count()
```
**Before**
```
In [4]: %timeit df.groupby("id").apply(normalize).count()
1.58 s ± 62.8 ms per loop (mean ± std. dev. of 7 runs, 1 loop each)
In [5]: %timeit df.groupby("id").apply(normalize).count()
1.52 s ± 29.5 ms per loop (mean ± std. dev. of 7 runs, 1 loop each)
In [6]: %timeit df.groupby("id").apply(normalize).count()
1.52 s ± 37.8 ms per loop (mean ± std. dev. of 7 runs, 1 loop each)
```
**After this Change**
```
In [2]: %timeit df.groupby("id").apply(normalize).count()
646 ms ± 89.9 ms per loop (mean ± std. dev. of 7 runs, 1 loop each)
In [3]: %timeit df.groupby("id").apply(normalize).count()
408 ms ± 84.6 ms per loop (mean ± std. dev. of 7 runs, 1 loop each)
In [4]: %timeit df.groupby("id").apply(normalize).count()
381 ms ± 29.9 ms per loop (mean ± std. dev. of 7 runs, 1 loop each)
```
Closes#24926 from BryanCutler/pyspark-pandas_udf-map-agg-skip-empty-parts-SPARK-28128.
Authored-by: Bryan Cutler <cutlerb@gmail.com>
Signed-off-by: HyukjinKwon <gurwls223@apache.org>
## What changes were proposed in this pull request?
The `mapChildren` method in the TreeNode class is commonly used across the whole Spark SQL codebase. In this method, there's a if statement that checks non-empty children. However, there's a cached lazy val `containsChild`, which can avoid unnecessary computation since `containsChild` is used in other methods and therefore constructed anyway.
Benchmark showed that this optimization can improve the whole TPC-DS planning time by 6.8%. There is no regression on any TPC-DS query.
## How was this patch tested?
Existing UTs.
Closes#24925 from yeshengm/treenode-children.
Authored-by: Yesheng Ma <kimi.ysma@gmail.com>
Signed-off-by: gatorsmile <gatorsmile@gmail.com>
## What changes were proposed in this pull request?
Currently using hive udf, the parameter is struct type, there will be an exception thrown.
No handler for Hive UDF 'xxxUDF': java.lang.RuntimeException: Hive doesn't support the constant type [StructType(StructField(name,StringType,true), StructField(value,DecimalType(3,1),true))]
## How was this patch tested?
added new UT
Closes#24846 from cxzl25/hive_udf_literal_struct_type.
Authored-by: sychen <sychen@ctrip.com>
Signed-off-by: HyukjinKwon <gurwls223@apache.org>
## What changes were proposed in this pull request?
The [SPARK-27403](https://issues.apache.org/jira/browse/SPARK-27403) fixed CTAS cannot update statistics even if `spark.sql.statistics.size.autoUpdate.enabled` is enabled, as mentioned in [SPARK-23263](https://issues.apache.org/jira/browse/SPARK-23263).
This pr adds tests for that fix.
## How was this patch tested?
N/A
Closes#20430 from wangyum/SPARK-23263.
Authored-by: Yuming Wang <yumwang@ebay.com>
Signed-off-by: HyukjinKwon <gurwls223@apache.org>
## What changes were proposed in this pull request?
File source V1 supports reading output of FileStreamSink as batch. https://github.com/apache/spark/pull/11897
We should support this in file source V2 as well. When reading with paths, we first check if there is metadata log of FileStreamSink. If yes, we use `MetadataLogFileIndex` for listing files; Otherwise, we use `InMemoryFileIndex`.
## How was this patch tested?
Unit test
Closes#24900 from gengliangwang/FileStreamV2.
Authored-by: Gengliang Wang <gengliang.wang@databricks.com>
Signed-off-by: Wenchen Fan <wenchen@databricks.com>
## What changes were proposed in this pull request?
Provide a way to recursively load data from datasource.
I add a "recursiveFileLookup" option.
When "recursiveFileLookup" option turn on, then partition inferring is turned off and all files from the directory will be loaded recursively.
If some datasource explicitly specify the partitionSpec, then if user turn on "recursive" option, then exception will be thrown.
## How was this patch tested?
Unit tests.
Please review https://spark.apache.org/contributing.html before opening a pull request.
Closes#24830 from WeichenXu123/recursive_ds.
Authored-by: WeichenXu <weichen.xu@databricks.com>
Signed-off-by: Wenchen Fan <wenchen@databricks.com>
## What changes were proposed in this pull request?
This PR significantly improves the performance of `UTF8String.replace()` by performing direct replacement over UTF8 bytes instead of decoding those bytes into Java Strings.
In cases where the search string is not found (i.e. no replacements are performed, a case which I expect to be common) this new implementation performs no object allocation or memory copying.
My implementation is modeled after `commons-lang3`'s `StringUtils.replace()` method. As part of my implementation, I needed a StringBuilder / resizable buffer, so I moved `UTF8StringBuilder` from the `catalyst` package to `unsafe`.
## How was this patch tested?
Copied tests from `StringExpressionSuite` to `UTF8StringSuite` and added a couple of new cases.
To evaluate performance, I did some quick local benchmarking by running the following code in `spark-shell` (with Java 1.8.0_191):
```scala
import org.apache.spark.unsafe.types.UTF8String
def benchmark(text: String, search: String, replace: String) {
val utf8Text = UTF8String.fromString(text)
val utf8Search = UTF8String.fromString(search)
val utf8Replace = UTF8String.fromString(replace)
val start = System.currentTimeMillis
var i = 0
while (i < 1000 * 1000 * 100) {
utf8Text.replace(utf8Search, utf8Replace)
i += 1
}
val end = System.currentTimeMillis
println(end - start)
}
benchmark("ABCDEFGH", "DEF", "ZZZZ") // replacement occurs
benchmark("ABCDEFGH", "Z", "") // no replacement occurs
```
On my laptop this took ~54 / ~40 seconds seconds before this patch's changes and ~6.5 / ~3.8 seconds afterwards.
Closes#24707 from JoshRosen/faster-string-replace.
Authored-by: Josh Rosen <rosenville@gmail.com>
Signed-off-by: Josh Rosen <rosenville@gmail.com>
## What changes were proposed in this pull request?
[SPARK-28093](https://issues.apache.org/jira/browse/SPARK-28093) fixed `TRIM/LTRIM/RTRIM('str', 'trimStr')` returns an incorrect value, but that fix introduced a new bug, `TRIM(type trimStr FROM str)` returns an incorrect value. This pr fix this issue.
## How was this patch tested?
unit tests and manual tests:
Before this PR:
```sql
spark-sql> SELECT trim('yxTomxx', 'xyz'), trim(BOTH 'xyz' FROM 'yxTomxx');
Tom z
spark-sql> SELECT trim('xxxbarxxx', 'x'), trim(BOTH 'x' FROM 'xxxbarxxx');
bar
spark-sql> SELECT ltrim('zzzytest', 'xyz'), trim(LEADING 'xyz' FROM 'zzzytest');
test xyz
spark-sql> SELECT ltrim('zzzytestxyz', 'xyz'), trim(LEADING 'xyz' FROM 'zzzytestxyz');
testxyz
spark-sql> SELECT ltrim('xyxXxyLAST WORD', 'xy'), trim(LEADING 'xy' FROM 'xyxXxyLAST WORD');
XxyLAST WORD
spark-sql> SELECT rtrim('testxxzx', 'xyz'), trim(TRAILING 'xyz' FROM 'testxxzx');
test xy
spark-sql> SELECT rtrim('xyztestxxzx', 'xyz'), trim(TRAILING 'xyz' FROM 'xyztestxxzx');
xyztest
spark-sql> SELECT rtrim('TURNERyxXxy', 'xy'), trim(TRAILING 'xy' FROM 'TURNERyxXxy');
TURNERyxX
```
After this PR:
```sql
spark-sql> SELECT trim('yxTomxx', 'xyz'), trim(BOTH 'xyz' FROM 'yxTomxx');
Tom Tom
spark-sql> SELECT trim('xxxbarxxx', 'x'), trim(BOTH 'x' FROM 'xxxbarxxx');
bar bar
spark-sql> SELECT ltrim('zzzytest', 'xyz'), trim(LEADING 'xyz' FROM 'zzzytest');
test test
spark-sql> SELECT ltrim('zzzytestxyz', 'xyz'), trim(LEADING 'xyz' FROM 'zzzytestxyz');
testxyz testxyz
spark-sql> SELECT ltrim('xyxXxyLAST WORD', 'xy'), trim(LEADING 'xy' FROM 'xyxXxyLAST WORD');
XxyLAST WORD XxyLAST WORD
spark-sql> SELECT rtrim('testxxzx', 'xyz'), trim(TRAILING 'xyz' FROM 'testxxzx');
test test
spark-sql> SELECT rtrim('xyztestxxzx', 'xyz'), trim(TRAILING 'xyz' FROM 'xyztestxxzx');
xyztest xyztest
spark-sql> SELECT rtrim('TURNERyxXxy', 'xy'), trim(TRAILING 'xy' FROM 'TURNERyxXxy');
TURNERyxX TURNERyxX
```
And PostgreSQL:
```sql
postgres=# SELECT trim('yxTomxx', 'xyz'), trim(BOTH 'xyz' FROM 'yxTomxx');
btrim | btrim
-------+-------
Tom | Tom
(1 row)
postgres=# SELECT trim('xxxbarxxx', 'x'), trim(BOTH 'x' FROM 'xxxbarxxx');
btrim | btrim
-------+-------
bar | bar
(1 row)
postgres=# SELECT ltrim('zzzytest', 'xyz'), trim(LEADING 'xyz' FROM 'zzzytest');
ltrim | ltrim
-------+-------
test | test
(1 row)
postgres=# SELECT ltrim('zzzytestxyz', 'xyz'), trim(LEADING 'xyz' FROM 'zzzytestxyz');
ltrim | ltrim
---------+---------
testxyz | testxyz
(1 row)
postgres=# SELECT ltrim('xyxXxyLAST WORD', 'xy'), trim(LEADING 'xy' FROM 'xyxXxyLAST WORD');
ltrim | ltrim
--------------+--------------
XxyLAST WORD | XxyLAST WORD
(1 row)
postgres=# SELECT rtrim('testxxzx', 'xyz'), trim(TRAILING 'xyz' FROM 'testxxzx');
rtrim | rtrim
-------+-------
test | test
(1 row)
postgres=# SELECT rtrim('xyztestxxzx', 'xyz'), trim(TRAILING 'xyz' FROM 'xyztestxxzx');
rtrim | rtrim
---------+---------
xyztest | xyztest
(1 row)
postgres=# SELECT rtrim('TURNERyxXxy', 'xy'), trim(TRAILING 'xy' FROM 'TURNERyxXxy');
rtrim | rtrim
-----------+-----------
TURNERyxX | TURNERyxX
(1 row)
```
Closes#24911 from wangyum/SPARK-28109.
Authored-by: Yuming Wang <yumwang@ebay.com>
Signed-off-by: Dongjoon Hyun <dhyun@apple.com>
## What changes were proposed in this pull request?
Current SQL parser's error message for hyphen-connected identifiers without surrounding backquotes(e.g. hyphen-table) is confusing for end users. A possible approach to tackle this is to explicitly capture these wrong usages in the SQL parser. In this way, the end users can fix these errors more quickly.
For example, for a simple query such as `SELECT * FROM test-table`, the original error message is
```
Error in SQL statement: ParseException:
mismatched input '-' expecting <EOF>(line 1, pos 18)
```
which can be confusing in a large query.
After the fix, the error message is:
```
Error in query:
Possibly unquoted identifier test-table detected. Please consider quoting it with back-quotes as `test-table`(line 1, pos 14)
== SQL ==
SELECT * FROM test-table
--------------^^^
```
which is easier for end users to identify the issue and fix.
We safely augmented the current grammar rule to explicitly capture these error cases. The error handling logic is implemented in the SQL parsing listener `PostProcessor`.
However, note that for cases such as `a - my-func(b)`, the parser can't actually tell whether this should be ``a -`my-func`(b) `` or `a - my - func(b)`. Therefore for these cases, we leave the parser as is. Also, in this patch we only provide better error messages for character-only identifiers.
## How was this patch tested?
Adding new unit tests.
Closes#24749 from yeshengm/hyphen-ident.
Authored-by: Yesheng Ma <kimi.ysma@gmail.com>
Signed-off-by: gatorsmile <gatorsmile@gmail.com>
## What changes were proposed in this pull request?
The original `references` and `validConstraints` implementations in a few `QueryPlan` and `Expression` classes are methods, which means unnecessary re-computation can happen at times. This PR resolves this problem by making these method `lazy val`s.
As shown in the following chart, the planning time(without cost-based optimization) was dramatically reduced after this optimization.
- The average planning time of TPC-DS queries was reduced by 19.63%.
- The planning time of the most time-consuming TPC-DS query (q64) was reduced by 43.03%.
- The running time for rule-based reordering joins(not cost-based join reordering) optimization, which are common in real-world OLAP queries, was largely reduced.
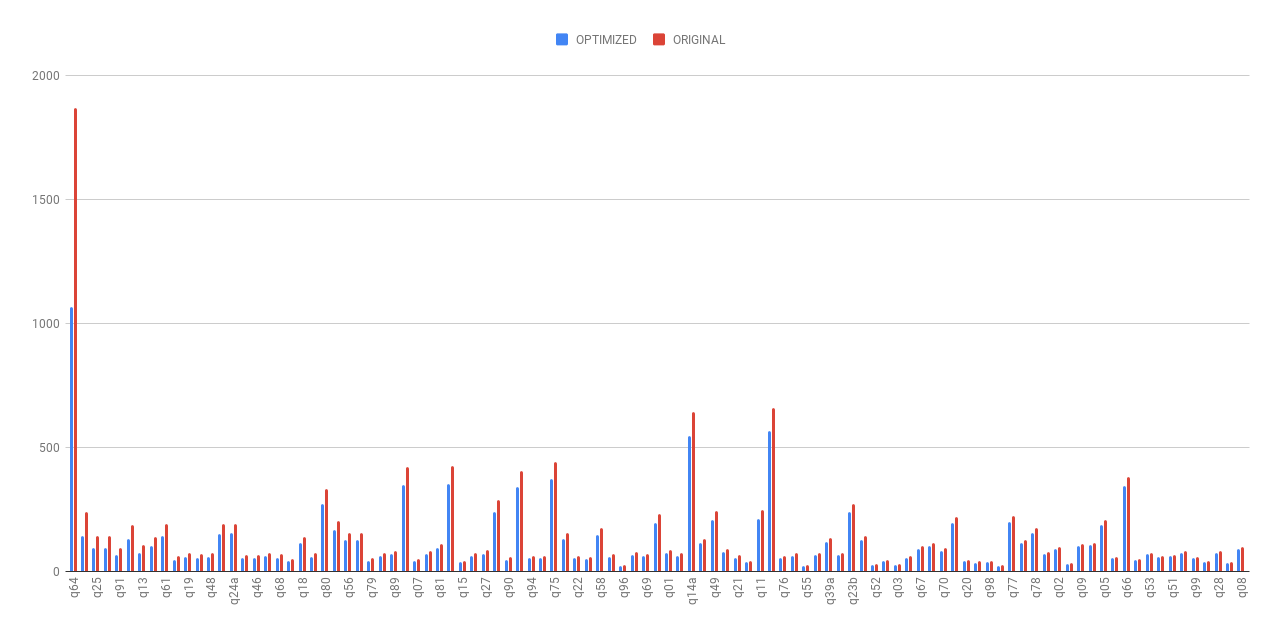
Detailed stats are listed in the following spreadsheet (we warmed up the queries 5 iterations and then took average of the next 5 iterations).
[Lazy val benchmark.xlsx](https://github.com/apache/spark/files/3303530/Lazy.val.benchmark.xlsx)
## How was this patch tested?
Existing UTs.
Closes#24866 from yeshengm/plannode-micro-opt.
Authored-by: Yesheng Ma <kimi.ysma@gmail.com>
Signed-off-by: gatorsmile <gatorsmile@gmail.com>
## What changes were proposed in this pull request?
`OrcFilters.createBuilder` has exponential complexity in the height of the filter tree due to the way the check-and-build pattern is implemented. We've hit this in production by passing a `Column` filter to Spark directly, with a job taking multiple hours for a simple set of ~30 filters. This PR changes the checking logic so that the conversion has linear complexity in the size of the tree instead of exponential in its height.
Right now, due to the way ORC `SearchArgument` works, the code is forced to do two separate phases when converting a given Spark filter to an ORC filter:
1. Check if the filter is convertible.
2. Only if the check in 1. succeeds, perform the actual conversion into the resulting ORC filter.
However, there's one detail which is the culprit in the exponential complexity: phases 1. and 2. are both done using the exact same method. The resulting exponential complexity is easiest to see in the `NOT` case - consider the following code:
```
val f1 = col("id") === lit(5)
val f2 = !f1
val f3 = !f2
val f4 = !f3
val f5 = !f4
```
Now, when we run `createBuilder` on `f5`, we get the following behaviour:
1. call `createBuilder(f4)` to check if the child `f4` is convertible
2. call `createBuilder(f4)` to actually convert it
This seems fine when looking at a single level, but what actually ends up happening is:
- `createBuilder(f3)` will then recursively be called 4 times - 2 times in step 1., and two times in step 2.
- `createBuilder(f2)` will be called 8 times - 4 times in each top-level step, 2 times in each sub-step.
- `createBuilder(f1)` will be called 16 times.
As a result, having a tree of height > 30 leads to billions of calls to `createBuilder`, heap allocations, and so on and can take multiple hours.
The way this PR solves this problem is by separating the `check` and `convert` functionalities into separate functions. This way, the call to `createBuilder` on `f5` above would look like this:
1. call `isConvertible(f4)` to check if the child `f4` is convertible - amortized constant complexity
2. call `createBuilder(f4)` to actually convert it - linear complexity in the size of the subtree.
This way, we get an overall complexity that's linear in the size of the filter tree, allowing us to convert tree with 10s of thousands of nodes in milliseconds.
The reason this split (`check` and `build`) is possible is that the checking never actually depends on the actual building of the filter. The `check` part of `createBuilder` depends mainly on:
- `isSearchableType` for leaf nodes, and
- `check`-ing the child filters for composite nodes like NOT, AND and OR.
Situations like the `SearchArgumentBuilder` throwing an exception while building the resulting ORC filter are not handled right now - they just get thrown out of the class, and this change preserves this behaviour.
This PR extracts this part of the code to a separate class which allows the conversion to make very efficient checks to confirm that a given child is convertible before actually converting it.
Results:
Before:
- converting a skewed tree with a height of ~35 took about 6-7 hours.
- converting a skewed tree with hundreds or thousands of nodes would be completely impossible.
Now:
- filtering against a skewed tree with a height of 1500 in the benchmark suite finishes in less than 10 seconds.
## Steps to reproduce
```scala
val schema = StructType.fromDDL("col INT")
(20 to 30).foreach { width =>
val whereFilter = (1 to width).map(i => EqualTo("col", i)).reduceLeft(Or)
val start = System.currentTimeMillis()
OrcFilters.createFilter(schema, Seq(whereFilter))
println(s"With $width filters, conversion takes ${System.currentTimeMillis() - start} ms")
}
```
### Before this PR
```
With 20 filters, conversion takes 363 ms
With 21 filters, conversion takes 496 ms
With 22 filters, conversion takes 939 ms
With 23 filters, conversion takes 1871 ms
With 24 filters, conversion takes 3756 ms
With 25 filters, conversion takes 7452 ms
With 26 filters, conversion takes 14978 ms
With 27 filters, conversion takes 30519 ms
With 28 filters, conversion takes 60361 ms // 1 minute
With 29 filters, conversion takes 126575 ms // 2 minutes 6 seconds
With 30 filters, conversion takes 257369 ms // 4 minutes 17 seconds
```
### After this PR
```
With 20 filters, conversion takes 12 ms
With 21 filters, conversion takes 0 ms
With 22 filters, conversion takes 1 ms
With 23 filters, conversion takes 0 ms
With 24 filters, conversion takes 1 ms
With 25 filters, conversion takes 1 ms
With 26 filters, conversion takes 0 ms
With 27 filters, conversion takes 1 ms
With 28 filters, conversion takes 0 ms
With 29 filters, conversion takes 1 ms
With 30 filters, conversion takes 0 ms
```
## How was this patch tested?
There are no changes in behaviour, and the existing tests pass. Added new benchmarks that expose the problematic behaviour and they finish quickly with the changes applied.
Closes#24068 from IvanVergiliev/optimize-orc-filters.
Authored-by: Ivan Vergiliev <ivan.vergiliev@gmail.com>
Signed-off-by: Wenchen Fan <wenchen@databricks.com>
## What changes were proposed in this pull request?
Added an interface for handling hint errors, with a default implementation class that logs warnings in the callbacks.
## How was this patch tested?
Passed existing tests.
Closes#24653 from maryannxue/hint-handler.
Authored-by: maryannxue <maryannxue@apache.org>
Signed-off-by: gatorsmile <gatorsmile@gmail.com>
## What changes were proposed in this pull request?
With JDK9+, the generate **bytecode** of `FromUnixTime` raise `java.lang.IncompatibleClassChangeError` due to [JDK-8145148](https://bugs.openjdk.java.net/browse/JDK-8145148) . This is a blocker in [Apache Spark JDK11 Jenkins job](https://amplab.cs.berkeley.edu/jenkins/view/Spark%20QA%20Test%20(Dashboard)/job/spark-master-test-maven-hadoop-2.7-jdk-11-ubuntu-testing/). Locally, this is reproducible by the following unit test suite with JDK9+.
```
$ build/sbt "catalyst/testOnly *.DateExpressionsSuite"
...
[info] org.apache.spark.sql.catalyst.expressions.DateExpressionsSuite *** ABORTED *** (23 seconds, 75 milliseconds)
[info] java.lang.IncompatibleClassChangeError: Method org.apache.spark.sql.catalyst.util.TimestampFormatter.apply(Ljava/lang/String;Ljava/time/ZoneId;Ljava/util/Locale;)Lorg/apache/spark/sql/catalyst/util/TimestampFormatter; must be InterfaceMeth
```
This bytecode issue is generated by `Janino` , so we replace `.apply` to `.MODULE$$.apply` and adds test coverage for similar codes.
## How was this patch tested?
Manually with the existing UTs by doing the following with JDK9+.
```
build/sbt "catalyst/testOnly *.DateExpressionsSuite"
```
Actually, this is the last JDK11 error in `catalyst` module. So, we can verify with the following, too.
```
$ build/sbt "project catalyst" test
...
[info] Total number of tests run: 3552
[info] Suites: completed 210, aborted 0
[info] Tests: succeeded 3552, failed 0, canceled 0, ignored 2, pending 0
[info] All tests passed.
[info] Passed: Total 3583, Failed 0, Errors 0, Passed 3583, Ignored 2
[success] Total time: 294 s, completed Jun 16, 2019, 10:15:08 PM
```
Closes#24889 from dongjoon-hyun/SPARK-28072.
Authored-by: Dongjoon Hyun <dhyun@apple.com>
Signed-off-by: Dongjoon Hyun <dhyun@apple.com>
## What changes were proposed in this pull request?
When using `DROPMALFORMED` mode, corrupted records aren't dropped if malformed columns aren't read. This behavior is due to CSV parser column pruning. Current doc of `DROPMALFORMED` doesn't mention the effect of column pruning. Users will be confused by the fact that `DROPMALFORMED` mode doesn't work as expected.
Column pruning also affects other modes. This is a doc improvement to add a note to doc of `mode` to explain it.
## How was this patch tested?
N/A. This is just doc change.
Closes#24894 from viirya/SPARK-28058.
Authored-by: Liang-Chi Hsieh <viirya@gmail.com>
Signed-off-by: HyukjinKwon <gurwls223@apache.org>
## What changes were proposed in this pull request?
The `TRIM` function accept these patterns:
```sql
TRIM(str)
TRIM(trimStr, str)
TRIM(BOTH trimStr FROM str)
TRIM(LEADING trimStr FROM str)
TRIM(TRAILING trimStr FROM str)
```
This pr add support other three patterns:
```sql
TRIM(BOTH FROM str)
TRIM(LEADING FROM str)
TRIM(TRAILING FROM str)
```
PostgreSQL, Vertica, MySQL, Teradata, Oracle and DB2 support these patterns. Hive, Presto and SQL Server does not support this feature.
**PostgreSQL**:
```sql
postgres=# select substr(version(), 0, 16), trim(BOTH from ' SparkSQL '), trim(LEADING FROM ' SparkSQL '), trim(TRAILING FROM ' SparkSQL ');
substr | btrim | ltrim | rtrim
-----------------+----------+-------------+--------------
PostgreSQL 11.3 | SparkSQL | SparkSQL | SparkSQL
(1 row)
```
**Vertica**:
```
dbadmin=> select version(), trim(BOTH from ' SparkSQL '), trim(LEADING FROM ' SparkSQL '), trim(TRAILING FROM ' SparkSQL ');
version | btrim | ltrim | rtrim
------------------------------------+----------+-------------+--------------
Vertica Analytic Database v9.1.1-0 | SparkSQL | SparkSQL | SparkSQL
(1 row)
```
**MySQL**:
```
mysql> select version(), trim(BOTH from ' SparkSQL '), trim(LEADING FROM ' SparkSQL '), trim(TRAILING FROM ' SparkSQL ');
+-----------+-----------------------------------+--------------------------------------+---------------------------------------+
| version() | trim(BOTH from ' SparkSQL ') | trim(LEADING FROM ' SparkSQL ') | trim(TRAILING FROM ' SparkSQL ') |
+-----------+-----------------------------------+--------------------------------------+---------------------------------------+
| 5.7.26 | SparkSQL | SparkSQL | SparkSQL |
+-----------+-----------------------------------+--------------------------------------+---------------------------------------+
1 row in set (0.01 sec)
```
**Teradata**:

**Oracle**:

**DB2**:

## How was this patch tested?
unit tests
Closes#24891 from wangyum/SPARK-28075.
Authored-by: Yuming Wang <yumwang@ebay.com>
Signed-off-by: Wenchen Fan <wenchen@databricks.com>
## What changes were proposed in this pull request?
This PR aims to replace deprecated `.newInstance()` in DSv2 `Catalogs` and distinguish the plugin class errors more. According to the JDK11 build log, there is no other new instance.
- https://amplab.cs.berkeley.edu/jenkins/view/Spark%20QA%20Test%20(Dashboard)/job/spark-master-test-maven-hadoop-2.7-jdk-11-ubuntu-testing/978/consoleFull
SPARK-25984 removes all instances of the deprecated `.newInstance()` usages at Nov 10, 2018, but this was added at SPARK-24252 on March 8, 2019.
## How was this patch tested?
Pass the Jenkins with the updated test case.
Closes#24882 from dongjoon-hyun/SPARK-28063.
Authored-by: Dongjoon Hyun <dhyun@apple.com>
Signed-off-by: Dongjoon Hyun <dhyun@apple.com>
## What changes were proposed in this pull request?
Currently `ArrayExists` always returns boolean values (if the arguments are not `null`), but it should follow the three-valued boolean logic:
- `true` if the predicate holds at least one `true`
- otherwise, `null` if the predicate holds `null`
- otherwise, `false`
This behavior change is made to match Postgres' equivalent function `ANY/SOME (array)`'s behavior: https://www.postgresql.org/docs/9.6/functions-comparisons.html#AEN21174
## How was this patch tested?
Modified tests and existing tests.
Closes#24873 from ueshin/issues/SPARK-28052/fix_exists.
Authored-by: Takuya UESHIN <ueshin@databricks.com>
Signed-off-by: Dongjoon Hyun <dhyun@apple.com>
## What changes were proposed in this pull request?
Allow Pandas UDF to take an iterator of pd.Series or an iterator of tuple of pd.Series.
Note the UDF input args will be always one iterator:
* if the udf take only column as input, the iterator's element will be pd.Series (corresponding to the column values batch)
* if the udf take multiple columns as inputs, the iterator's element will be a tuple composed of multiple `pd.Series`s, each one corresponding to the multiple columns as inputs (keep the same order). For example:
```
pandas_udf("int", PandasUDFType.SCALAR_ITER)
def the_udf(iterator):
for col1_batch, col2_batch in iterator:
yield col1_batch + col2_batch
df.select(the_udf("col1", "col2"))
```
The udf above will add col1 and col2.
I haven't add unit tests, but manually tests show it works fine. So it is ready for first pass review.
We can test several typical cases:
```
from pyspark.sql import SparkSession
from pyspark.sql.functions import pandas_udf, PandasUDFType
from pyspark.sql.functions import udf
from pyspark.taskcontext import TaskContext
df = spark.createDataFrame([(1, 20), (3, 40)], ["a", "b"])
pandas_udf("int", PandasUDFType.SCALAR_ITER)
def fi1(it):
pid = TaskContext.get().partitionId()
print("DBG: fi1: do init stuff, partitionId=" + str(pid))
for batch in it:
yield batch + 100
print("DBG: fi1: do close stuff, partitionId=" + str(pid))
pandas_udf("int", PandasUDFType.SCALAR_ITER)
def fi2(it):
pid = TaskContext.get().partitionId()
print("DBG: fi2: do init stuff, partitionId=" + str(pid))
for batch in it:
yield batch + 10000
print("DBG: fi2: do close stuff, partitionId=" + str(pid))
pandas_udf("int", PandasUDFType.SCALAR_ITER)
def fi3(it):
pid = TaskContext.get().partitionId()
print("DBG: fi3: do init stuff, partitionId=" + str(pid))
for x, y in it:
yield x + y * 10 + 100000
print("DBG: fi3: do close stuff, partitionId=" + str(pid))
pandas_udf("int", PandasUDFType.SCALAR)
def fp1(x):
return x + 1000
udf("int")
def fu1(x):
return x + 10
# test select "pandas iter udf/pandas udf/sql udf" expressions at the same time.
# Note this case the `fi1("a"), fi2("b"), fi3("a", "b")` will generate only one plan,
# and `fu1("a")`, `fp1("a")` will generate another two separate plans.
df.select(fi1("a"), fi2("b"), fi3("a", "b"), fu1("a"), fp1("a")).show()
# test chain two pandas iter udf together
# Note this case `fi2(fi1("a"))` will generate only one plan
# Also note the init stuff/close stuff call order will be like:
# (debug output following)
# DBG: fi2: do init stuff, partitionId=0
# DBG: fi1: do init stuff, partitionId=0
# DBG: fi1: do close stuff, partitionId=0
# DBG: fi2: do close stuff, partitionId=0
df.select(fi2(fi1("a"))).show()
# test more complex chain
# Note this case `fi1("a"), fi2("a")` will generate one plan,
# and `fi3(fi1_output, fi2_output)` will generate another plan
df.select(fi3(fi1("a"), fi2("a"))).show()
```
## How was this patch tested?
To be added.
Please review http://spark.apache.org/contributing.html before opening a pull request.
Closes#24643 from WeichenXu123/pandas_udf_iter.
Lead-authored-by: WeichenXu <weichen.xu@databricks.com>
Co-authored-by: Xiangrui Meng <meng@databricks.com>
Signed-off-by: Xiangrui Meng <meng@databricks.com>
## What changes were proposed in this pull request?
Migrate Parquet to File Data Source V2
## How was this patch tested?
Unit test
Closes#24327 from gengliangwang/parquetV2.
Authored-by: Gengliang Wang <gengliang.wang@databricks.com>
Signed-off-by: HyukjinKwon <gurwls223@apache.org>
## What changes were proposed in this pull request?
Implemented a new SparkPlan that executes the query adaptively. It splits the query plan into independent stages and executes them in order according to their dependencies. The query stage materializes its output at the end. When one stage completes, the data statistics of the materialized output will be used to optimize the remainder of the query.
The adaptive mode is off by default, when turned on, user can see "AdaptiveSparkPlan" as the top node of a query or sub-query. The inner plan of "AdaptiveSparkPlan" is subject to change during query execution but becomes final once the execution is complete. Whether the inner plan is final is included in the EXPLAIN string. Below is an example of the EXPLAIN plan before and after execution:
Query:
```
SELECT * FROM testData JOIN testData2 ON key = a WHERE value = '1'
```
Before execution:
```
== Physical Plan ==
AdaptiveSparkPlan(isFinalPlan=false)
+- SortMergeJoin [key#13], [a#23], Inner
:- Sort [key#13 ASC NULLS FIRST], false, 0
: +- Exchange hashpartitioning(key#13, 5)
: +- Filter (isnotnull(value#14) AND (value#14 = 1))
: +- SerializeFromObject [knownnotnull(assertnotnull(input[0, org.apache.spark.sql.test.SQLTestData$TestData, true])).key AS key#13, staticinvoke(class org.apache.spark.unsafe.types.UTF8String, StringType, fromString, knownnotnull(assertnotnull(input[0, org.apache.spark.sql.test.SQLTestData$TestData, true])).value, true, false) AS value#14]
: +- Scan[obj#12]
+- Sort [a#23 ASC NULLS FIRST], false, 0
+- Exchange hashpartitioning(a#23, 5)
+- SerializeFromObject [knownnotnull(assertnotnull(input[0, org.apache.spark.sql.test.SQLTestData$TestData2, true])).a AS a#23, knownnotnull(assertnotnull(input[0, org.apache.spark.sql.test.SQLTestData$TestData2, true])).b AS b#24]
+- Scan[obj#22]
```
After execution:
```
== Physical Plan ==
AdaptiveSparkPlan(isFinalPlan=true)
+- *(1) BroadcastHashJoin [key#13], [a#23], Inner, BuildLeft
:- BroadcastQueryStage 2
: +- BroadcastExchange HashedRelationBroadcastMode(List(cast(input[0, int, false] as bigint)))
: +- ShuffleQueryStage 0
: +- Exchange hashpartitioning(key#13, 5)
: +- *(1) Filter (isnotnull(value#14) AND (value#14 = 1))
: +- *(1) SerializeFromObject [knownnotnull(assertnotnull(input[0, org.apache.spark.sql.test.SQLTestData$TestData, true])).key AS key#13, staticinvoke(class org.apache.spark.unsafe.types.UTF8String, StringType, fromString, knownnotnull(assertnotnull(input[0, org.apache.spark.sql.test.SQLTestData$TestData, true])).value, true, false) AS value#14]
: +- Scan[obj#12]
+- ShuffleQueryStage 1
+- Exchange hashpartitioning(a#23, 5)
+- *(1) SerializeFromObject [knownnotnull(assertnotnull(input[0, org.apache.spark.sql.test.SQLTestData$TestData2, true])).a AS a#23, knownnotnull(assertnotnull(input[0, org.apache.spark.sql.test.SQLTestData$TestData2, true])).b AS b#24]
+- Scan[obj#22]
```
Credit also goes to carsonwang and cloud-fan
## How was this patch tested?
Added new UT.
Closes#24706 from maryannxue/aqe.
Authored-by: maryannxue <maryannxue@apache.org>
Signed-off-by: herman <herman@databricks.com>
## What changes were proposed in this pull request?
This PR adds support of column aliasing in a CTE so this query becomes valid:
```
WITH t(x) AS (SELECT 1)
SELECT * FROM t WHERE x = 1
```
## How was this patch tested?
Added new UTs.
Closes#24842 from peter-toth/SPARK-28002.
Authored-by: Peter Toth <peter.toth@gmail.com>
Signed-off-by: Dongjoon Hyun <dhyun@apple.com>
## What changes were proposed in this pull request?
This patch proposes making `purge` in `CompactibleFileStreamLog` to throw `UnsupportedOperationException` to prevent purging necessary batch files, as well as adding javadoc to document its behavior. Actually it would only break when latest compaction batch is requested to be purged, but caller wouldn't be aware of this so safer to just prevent it.
## How was this patch tested?
Added UT.
Closes#23850 from HeartSaVioR/SPARK-26949.
Authored-by: Jungtaek Lim (HeartSaVioR) <kabhwan@gmail.com>
Signed-off-by: Dongjoon Hyun <dhyun@apple.com>
## What changes were proposed in this pull request?
Implemented the `clone` method for `TreeNode` based on `mapChildren`.
## How was this patch tested?
Added new UT.
Closes#24876 from maryannxue/treenode-clone.
Authored-by: maryannxue <maryannxue@apache.org>
Signed-off-by: herman <herman@databricks.com>
## What changes were proposed in this pull request?
I suspect that the doc of `rowsBetween` methods in Scala and PySpark looks wrong.
Because:
```scala
scala> val df = Seq((1, "a"), (2, "a"), (3, "a"), (4, "a"), (5, "a"), (6, "a")).toDF("id", "category")
df: org.apache.spark.sql.DataFrame = [id: int, category: string]
scala> val byCategoryOrderedById = Window.partitionBy('category).orderBy('id).rowsBetween(-1, 2)
byCategoryOrderedById: org.apache.spark.sql.expressions.WindowSpec = org.apache.spark.sql.expressions.WindowSpec7f04de97
scala> df.withColumn("sum", sum('id) over byCategoryOrderedById).show()
+---+--------+---+
| id|category|sum|
+---+--------+---+
| 1| a| 6| # sum from index 0 to (0 + 2): 1 + 2 + 3 = 6
| 2| a| 10| # sum from index (1 - 1) to (1 + 2): 1 + 2 + 3 + 4 = 10
| 3| a| 14|
| 4| a| 18|
| 5| a| 15|
| 6| a| 11|
+---+--------+---+
```
So the frame (-1, 2) for row with index 5, as described in the doc, should range from index 4 to index 7.
## How was this patch tested?
N/A, just doc change.
Closes#24864 from viirya/window-spec-doc.
Authored-by: Liang-Chi Hsieh <viirya@gmail.com>
Signed-off-by: HyukjinKwon <gurwls223@apache.org>
## What changes were proposed in this pull request?
Currently, SparkSQL can support interval format like this.
```sql
SELECT INTERVAL '0 23:59:59.155' DAY TO SECOND
```
Like Presto/Teradata, this PR aims to support grammar like below.
```sql
SELECT INTERVAL '23:59:59.155' HOUR TO SECOND
```
Although we can add a new function for this pattern, we had better extend the existing code to handle a missing day case. So, the following is also supported.
```sql
SELECT INTERVAL '23:59:59.155' DAY TO SECOND
SELECT INTERVAL '1 23:59:59.155' HOUR TO SECOND
```
Currently Vertica/Teradata/Postgresql/SQL Server have fully support of below interval functions.
- interval ... year to month
- interval ... day to hour
- interval ... day to minute
- interval ... day to second
- interval ... hour to minute
- interval ... hour to second
- interval ... minute to second
https://www.vertica.com/docs/9.2.x/HTML/Content/Authoring/SQLReferenceManual/LanguageElements/Literals/interval-qualifier.htmdf1a699e5b/src/test/regress/sql/interval.sql (L180-L203)https://docs.teradata.com/reader/S0Fw2AVH8ff3MDA0wDOHlQ/KdCtT3pYFo~_enc8~kGKVwhttps://docs.microsoft.com/en-us/sql/odbc/reference/appendixes/interval-literals?view=sql-server-2017
## How was this patch tested?
Pass the Jenkins with the updated test cases.
Closes#24472 from lipzhu/SPARK-27578.
Lead-authored-by: Zhu, Lipeng <lipzhu@ebay.com>
Co-authored-by: Dongjoon Hyun <dhyun@apple.com>
Co-authored-by: Lipeng Zhu <lipzhu@icloud.com>
Signed-off-by: Dongjoon Hyun <dhyun@apple.com>
## What changes were proposed in this pull request?
Support multi-catalog in the following SELECT code paths:
- SELECT * FROM catalog.db.tbl
- TABLE catalog.db.tbl
- JOIN or UNION tables from different catalogs
- SparkSession.table("catalog.db.tbl")
- CTE relation
- View text
## How was this patch tested?
New unit tests.
All existing unit tests in catalyst and sql core.
Closes#24741 from jzhuge/SPARK-27322-pr.
Authored-by: John Zhuge <jzhuge@apache.org>
Signed-off-by: Wenchen Fan <wenchen@databricks.com>
## What changes were proposed in this pull request?
Convert `PartitionedFile.filePath` to URI first in binary file data source. Otherwise Spark will throw a FileNotFound exception because we create `Path` with URL encoded string, instead of wrapping it with URI.
## How was this patch tested?
Unit test.
Closes#24855 from mengxr/SPARK-28030.
Authored-by: Xiangrui Meng <meng@databricks.com>
Signed-off-by: Xiangrui Meng <meng@databricks.com>
## What changes were proposed in this pull request?
`NestedColumnAliasing` rule covers `GetStructField` only, currently. It means that some nested field extraction expressions aren't pruned. For example, if only accessing a nested field in an array of struct (`GetArrayStructFields`), this column isn't pruned.
This patch extends the rule to cover general nested field cases, including `GetArrayStructFields`.
## How was this patch tested?
Added tests.
Closes#24599 from viirya/nested-pruning-extract-value.
Lead-authored-by: Liang-Chi Hsieh <viirya@gmail.com>
Co-authored-by: Dongjoon Hyun <dhyun@apple.com>
Signed-off-by: Dongjoon Hyun <dhyun@apple.com>
Current Spark SQL parser can have pretty confusing error messages when parsing an incorrect SELECT SQL statement. The proposed fix has the following effect.
BEFORE:
```
spark-sql> SELECT * FROM test WHERE x NOT NULL;
Error in query:
mismatched input 'FROM' expecting {<EOF>, 'CLUSTER', 'DISTRIBUTE', 'EXCEPT', 'GROUP', 'HAVING', 'INTERSECT', 'LATERAL', 'LIMIT', 'ORDER', 'MINUS', 'SORT', 'UNION', 'WHERE', 'WINDOW'}(line 1, pos 9)
== SQL ==
SELECT * FROM test WHERE x NOT NULL
---------^^^
```
where in fact the error message should be hinted to be near `NOT NULL`.
AFTER:
```
spark-sql> SELECT * FROM test WHERE x NOT NULL;
Error in query:
mismatched input 'NOT' expecting {<EOF>, 'AND', 'CLUSTER', 'DISTRIBUTE', 'EXCEPT', 'GROUP', 'HAVING', 'INTERSECT', 'LIMIT', 'OR', 'ORDER', 'MINUS', 'SORT', 'UNION', 'WINDOW'}(line 1, pos 27)
== SQL ==
SELECT * FROM test WHERE x NOT NULL
---------------------------^^^
```
In fact, this problem is brought by some problematic Spark SQL grammar. There are two kinds of SELECT statements that are supported by Hive (and thereby supported in SparkSQL):
* `FROM table SELECT blahblah SELECT blahblah`
* `SELECT blah FROM table`
*Reference* [HiveQL single-from stmt grammar](https://github.com/apache/hive/blob/master/ql/src/java/org/apache/hadoop/hive/ql/parse/HiveParser.g)
It is fine when these two SELECT syntaxes are supported separately. However, since we are currently supporting these two kinds of syntaxes in a single ANTLR rule, this can be problematic and therefore leading to confusing parser errors. This is because when a SELECT clause was parsed, it can't tell whether the following FROM clause actually belongs to it or is just the beginning of a new `FROM table SELECT *` statement.
## What changes were proposed in this pull request?
1. Modify ANTLR grammar to fix the above-mentioned problem. This fix is important because the previous problematic grammar does affect a lot of real-world queries. Due to the previous problematic and messy grammar, we refactored the grammar related to `querySpecification`.
2. Modify `AstBuilder` to have separate visitors for `SELECT ... FROM ...` and `FROM ... SELECT ...` statements.
3. Drop the `FROM table` statement, which is supported by accident and is actually parsed in the wrong code path. Both Hive and Presto do not support this syntax.
## How was this patch tested?
Existing UTs and new UTs.
Closes#24809 from yeshengm/parser-refactor.
Authored-by: Yesheng Ma <kimi.ysma@gmail.com>
Signed-off-by: Xingbo Jiang <xingbo.jiang@databricks.com>
## What changes were proposed in this pull request?
The new Spark ThriftServer SparkGetTablesOperation implemented in https://github.com/apache/spark/pull/22794 does a catalog.getTableMetadata request for every table. This can get very slow for large schemas (~50ms per table with an external Hive metastore).
Hive ThriftServer GetTablesOperation uses HiveMetastoreClient.getTableObjectsByName to get table information in bulk, but we don't expose that through our APIs that go through Hive -> HiveClientImpl (HiveClient) -> HiveExternalCatalog (ExternalCatalog) -> SessionCatalog.
If we added and exposed getTableObjectsByName through our catalog APIs, we could resolve that performance problem in SparkGetTablesOperation.
## How was this patch tested?
Add UT
Closes#24774 from LantaoJin/SPARK-27899.
Authored-by: LantaoJin <jinlantao@gmail.com>
Signed-off-by: gatorsmile <gatorsmile@gmail.com>
## What changes were proposed in this pull request?
Handle the case when ParsedStatement subclass has a Map field but not of type Map[String, String].
In ParsedStatement.productIterator, `case mapArg: Map[_, _]` can match any Map type due to type erasure, thus causing `asInstanceOf[Map[String, String]]` to throw ClassCastException.
The following test reproduces the issue:
```
case class TestStatement(p: Map[String, Int]) extends ParsedStatement {
override def output: Seq[Attribute] = Nil
override def children: Seq[LogicalPlan] = Nil
}
TestStatement(Map("abc" -> 1)).toString
```
Changing the code to `case mapArg: Map[String, String]` will not help due to type erasure. As a matter of fact, compiler gives this warning:
```
Warning:(41, 18) non-variable type argument String in type pattern
scala.collection.immutable.Map[String,String] (the underlying of Map[String,String])
is unchecked since it is eliminated by erasure
case mapArg: Map[String, String] =>
```
## How was this patch tested?
Add 2 unit tests.
Closes#24800 from jzhuge/SPARK-27947.
Authored-by: John Zhuge <jzhuge@apache.org>
Signed-off-by: Dongjoon Hyun <dhyun@apple.com>
## What changes were proposed in this pull request?
For caseWhen Object canonicalized is not handled
for e.g let's consider below CaseWhen Object
val attrRef = AttributeReference("ACCESS_CHECK", StringType)()
val caseWhenObj1 = CaseWhen(Seq((attrRef, Literal("A"))))
caseWhenObj1.canonicalized **ouput** is as below
CASE WHEN ACCESS_CHECK#0 THEN A END (**Before Fix)**
**After Fix** : CASE WHEN none#0 THEN A END
So when there will be aliasref like below statements, semantic equals will fail. Sematic equals returns true if the canonicalized form of both the expressions are same.
val attrRef = AttributeReference("ACCESS_CHECK", StringType)()
val aliasAttrRef = attrRef.withName("access_check")
val caseWhenObj1 = CaseWhen(Seq((attrRef, Literal("A"))))
val caseWhenObj2 = CaseWhen(Seq((aliasAttrRef, Literal("A"))))
**assert(caseWhenObj2.semanticEquals(caseWhenObj1.semanticEquals) fails**
**caseWhenObj1.canonicalized**
Before Fix:CASE WHEN ACCESS_CHECK#0 THEN A END
After Fix: CASE WHEN none#0 THEN A END
**caseWhenObj2.canonicalized**
Before Fix:CASE WHEN access_check#0 THEN A END
After Fix: CASE WHEN none#0 THEN A END
## How was this patch tested?
Added UT
Closes#24766 from sandeep-katta/caseWhenIssue.
Authored-by: sandeep katta <sandeep.katta2007@gmail.com>
Signed-off-by: Dongjoon Hyun <dhyun@apple.com>
## What changes were proposed in this pull request?
This PR is the same fix as https://github.com/apache/spark/pull/24816 but in vectorized `dapply` in SparkR.
## How was this patch tested?
Manually tested.
Closes#24818 from HyukjinKwon/SPARK-27971.
Authored-by: HyukjinKwon <gurwls223@apache.org>
Signed-off-by: HyukjinKwon <gurwls223@apache.org>
## What changes were proposed in this pull request?
The newly added `Refresh` method in PR #24401 prevented the work of moving DataSourceV2Relation into catalyst. It calls `case table: FileTable => table.fileIndex.refresh()` while `FileTable` belongs to sql/core.
More importantly, Ryan Blue pointed out DataSourceV2Relation is immutable by design, it should not have refresh method.
## How was this patch tested?
Unit test
Closes#24815 from gengliangwang/removeRefreshTable.
Authored-by: Gengliang Wang <gengliang.wang@databricks.com>
Signed-off-by: Dongjoon Hyun <dhyun@apple.com>
## What changes were proposed in this pull request?
It seems that some users are using Hive 3.0.0. This pr makes it support Hive 3.0 metastore.
## How was this patch tested?
unit tests
Closes#24688 from wangyum/SPARK-26145.
Authored-by: Yuming Wang <yumwang@ebay.com>
Signed-off-by: gatorsmile <gatorsmile@gmail.com>
## What changes were proposed in this pull request?
Flush batch timely for pandas UDF.
This could improve performance when multiple pandas UDF plans are pipelined.
When batch being flushed in time, downstream pandas UDFs will get pipelined as soon as possible, and pipeline will help hide the donwstream UDFs computation time. For example:
When the first UDF start computing on batch-3, the second pipelined UDF can start computing on batch-2, and the third pipelined UDF can start computing on batch-1.
If we do not flush each batch in time, the donwstream UDF's pipeline will lag behind too much, which may increase the total processing time.
I add flush at two places:
* JVM process feed data into python worker. In jvm side, when write one batch, flush it
* VM process read data from python worker output, In python worker side, when write one batch, flush it
If no flush, the default buffer size for them are both 65536. Especially in the ML case, in order to make realtime prediction, we will make batch size very small. The buffer size is too large for the case, which cause downstream pandas UDF pipeline lag behind too much.
### Note
* This is only applied to pandas scalar UDF.
* Do not flush for each batch. The minimum interval between two flush is 0.1 second. This avoid too frequent flushing when batch size is small. It works like:
```
last_flush_time = time.time()
for batch in iterator:
writer.write_batch(batch)
flush_time = time.time()
if self.flush_timely and (flush_time - last_flush_time > 0.1):
stream.flush()
last_flush_time = flush_time
```
## How was this patch tested?
### Benchmark to make sure the flush do not cause performance regression
#### Test code:
```
numRows = ...
batchSize = ...
spark.conf.set('spark.sql.execution.arrow.maxRecordsPerBatch', str(batchSize))
df = spark.range(1, numRows + 1, numPartitions=1).select(col('id').alias('a'))
pandas_udf("int", PandasUDFType.SCALAR)
def fp1(x):
return x + 10
beg_time = time.time()
result = df.select(sum(fp1('a'))).head()
print("result: " + str(result[0]))
print("consume time: " + str(time.time() - beg_time))
```
#### Test Result:
params | Consume time (Before) | Consume time (After)
------------ | ----------------------- | ----------------------
numRows=100000000, batchSize=10000 | 23.43s | 24.64s
numRows=100000000, batchSize=1000 | 36.73s | 34.50s
numRows=10000000, batchSize=100 | 35.67s | 32.64s
numRows=1000000, batchSize=10 | 33.60s | 32.11s
numRows=100000, batchSize=1 | 33.36s | 31.82s
### Benchmark pipelined pandas UDF
#### Test code:
```
spark.conf.set('spark.sql.execution.arrow.maxRecordsPerBatch', '1')
df = spark.range(1, 31, numPartitions=1).select(col('id').alias('a'))
pandas_udf("int", PandasUDFType.SCALAR)
def fp1(x):
print("run fp1")
time.sleep(1)
return x + 100
pandas_udf("int", PandasUDFType.SCALAR)
def fp2(x, y):
print("run fp2")
time.sleep(1)
return x + y
beg_time = time.time()
result = df.select(sum(fp2(fp1('a'), col('a')))).head()
print("result: " + str(result[0]))
print("consume time: " + str(time.time() - beg_time))
```
#### Test Result:
**Before**: consume time: 63.57s
**After**: consume time: 32.43s
**So the PR improve performance by make downstream UDF get pipelined early.**
Please review https://spark.apache.org/contributing.html before opening a pull request.
Closes#24734 from WeichenXu123/improve_pandas_udf_pipeline.
Lead-authored-by: WeichenXu <weichen.xu@databricks.com>
Co-authored-by: Xiangrui Meng <meng@databricks.com>
Signed-off-by: gatorsmile <gatorsmile@gmail.com>
## What changes were proposed in this pull request?
When using `from_avro` to deserialize avro data to catalyst StructType format, if `ConvertToLocalRelation` is applied at the time, `from_avro` produces only the last value (overriding previous values).
The cause is `AvroDeserializer` reuses output row for StructType. Normally, it should be fine in Spark SQL. But `ConvertToLocalRelation` just uses `InterpretedProjection` to project local rows. `InterpretedProjection` creates new row for each output thro, it includes the same nested row object from `AvroDeserializer`. By the end, converted local relation has only last value.
I think there're two possible options:
1. Make `AvroDeserializer` output new row for StructType.
2. Use `InterpretedMutableProjection` in `ConvertToLocalRelation` and call `copy()` on output rows.
Option 2 is chose because previously `ConvertToLocalRelation` also creates new rows, this `InterpretedMutableProjection` + `copy()` shoudn't bring too much performance penalty. `ConvertToLocalRelation` should be arguably less critical, compared with `AvroDeserializer`.
## How was this patch tested?
Added test.
Closes#24805 from viirya/SPARK-27798.
Authored-by: Liang-Chi Hsieh <viirya@gmail.com>
Signed-off-by: Dongjoon Hyun <dhyun@apple.com>
## What changes were proposed in this pull request?
Add extractors for v2 catalog transforms.
These extractors are used to match transforms that are equivalent to Spark's internal case classes. This makes it easier to work with v2 transforms.
## How was this patch tested?
Added test suite for the new extractors.
Closes#24812 from rdblue/SPARK-27965-add-transform-extractors.
Authored-by: Ryan Blue <blue@apache.org>
Signed-off-by: gatorsmile <gatorsmile@gmail.com>
## What changes were proposed in this pull request?
In PR https://github.com/apache/spark/pull/24365, we pass in the partitionBy columns as options in `DataFrameWriter`. To make this change less intrusive for a patch release, we added a feature flag `LEGACY_PASS_PARTITION_BY_AS_OPTIONS` with the default to be false.
For 3.0, we should just do the correct behavior for DSV1, i.e., always passing partitionBy as options, and remove this legacy feature flag.
## How was this patch tested?
Existing tests.
Closes#24784 from liwensun/SPARK-27453-default.
Authored-by: liwensun <liwen.sun@databricks.com>
Signed-off-by: HyukjinKwon <gurwls223@apache.org>
## What changes were proposed in this pull request?
Issued fixed in https://github.com/apache/spark/pull/24734 but that PR might takes longer to merge.
## How was this patch tested?
It should pass existing unit tests.
Closes#24816 from mengxr/SPARK-27968.
Authored-by: Xiangrui Meng <meng@databricks.com>
Signed-off-by: Xiangrui Meng <meng@databricks.com>
## What changes were proposed in this pull request?
Move methods that implement v2 catalog operations to CatalogV2Util so they can be used in #24768.
## How was this patch tested?
Behavior is validated by existing tests.
Closes#24813 from rdblue/SPARK-27964-add-catalog-v2-util.
Authored-by: Ryan Blue <blue@apache.org>
Signed-off-by: Dongjoon Hyun <dhyun@apple.com>
## What changes were proposed in this pull request?
I believe the log message: `Committer $committerClass is not a ParquetOutputCommitter and cannot create job summaries. Set Parquet option ${ParquetOutputFormat.JOB_SUMMARY_LEVEL} to NONE.` is at odds with the `if` statement that logs the warning. Despite the instructions in the warning, users still encounter the warning if `JOB_SUMMARY_LEVEL` is already set to `NONE`.
This pull request introduces a change to skip logging the warning if `JOB_SUMMARY_LEVEL` is set to `NONE`.
## How was this patch tested?
I built to make sure everything still compiled and I ran the existing test suite. I didn't feel it was worth the overhead to add a test to make sure a log message does not get logged, but if reviewers feel differently, I can add one.
Closes#24808 from jmsanders/master.
Authored-by: Jordan Sanders <jmsanders@users.noreply.github.com>
Signed-off-by: Dongjoon Hyun <dhyun@apple.com>
## What changes were proposed in this pull request?
This moves parsing logic for `ALTER TABLE` into Catalyst and adds parsed logical plans for alter table changes that use multi-part identifiers. This PR is similar to SPARK-27108, PR #24029, that created parsed logical plans for create and CTAS.
* Create parsed logical plans
* Move parsing logic into Catalyst's AstBuilder
* Convert to DataSource plans in DataSourceResolution
* Parse `ALTER TABLE ... SET LOCATION ...` separately from the partition variant
* Parse `ALTER TABLE ... ALTER COLUMN ... [TYPE dataType] [COMMENT comment]` [as discussed on the dev list](http://apache-spark-developers-list.1001551.n3.nabble.com/DISCUSS-Syntax-for-table-DDL-td25197.html#a25270)
* Parse `ALTER TABLE ... RENAME COLUMN ... TO ...`
* Parse `ALTER TABLE ... DROP COLUMNS ...`
## How was this patch tested?
* Added new tests in Catalyst's `DDLParserSuite`
* Moved converted plan tests from SQL `DDLParserSuite` to `PlanResolutionSuite`
* Existing tests for regressions
Closes#24723 from rdblue/SPARK-27857-add-alter-table-statements-in-catalyst.
Authored-by: Ryan Blue <blue@apache.org>
Signed-off-by: gatorsmile <gatorsmile@gmail.com>
Extracting the common purge "behaviour" to the parent StreamExecution.
## How was this patch tested?
No added behaviour so relying on existing tests.
Closes#24781 from jaceklaskowski/StreamExecution-purge.
Authored-by: Jacek Laskowski <jacek@japila.pl>
Signed-off-by: Sean Owen <sean.owen@databricks.com>
## What changes were proposed in this pull request?
Currently we are in a strange status that, some data source v2 interfaces(catalog related) are in sql/catalyst, some data source v2 interfaces(Table, ScanBuilder, DataReader, etc.) are in sql/core.
I don't see a reason to keep data source v2 API in 2 modules. If we should pick one module, I think sql/catalyst is the one to go.
Catalyst module already has some user-facing stuff like DataType, Row, etc. And we have to update `Analyzer` and `SessionCatalog` to support the new catalog plugin, which needs to be in the catalyst module.
This PR can solve the problem we have in https://github.com/apache/spark/pull/24246
## How was this patch tested?
existing tests
Closes#24416 from cloud-fan/move.
Authored-by: Wenchen Fan <wenchen@databricks.com>
Signed-off-by: gatorsmile <gatorsmile@gmail.com>
## What changes were proposed in this pull request?
This is a part of #24774, to reduce the code changes made by that.
## How was this patch tested?
Exist UTs.
Closes#24803 from LantaoJin/SPARK-27899_refactor.
Authored-by: LantaoJin <jinlantao@gmail.com>
Signed-off-by: gatorsmile <gatorsmile@gmail.com>
## What changes were proposed in this pull request?
This updates CTE substitution to avoid needing to run all resolution rules on each substituted expression. Running resolution rules was previously used to avoid infinite recursion. In the updated rule, CTE plans are substituted as sub-queries from right to left. Using this scope-based order, it is not necessary to replace multiple CTEs at the same time using `resolveOperatorsDown`. Instead, `resolveOperatorsUp` is used to replace each CTE individually.
By resolving using `resolveOperatorsUp`, this no longer needs to run all analyzer rules on each substituted expression. Previously, this was done to apply `ResolveRelations`, which would throw an `AnalysisException` for all unresolved relations so that unresolved relations that may cause recursive substitutions were not left in the plan. Because this is no longer needed, `ResolveRelations` no longer needs to throw `AnalysisException` and resolution can be done in multiple rules.
## How was this patch tested?
Existing tests in `SQLQueryTestSuite`, `cte.sql`.
Closes#24763 from rdblue/SPARK-27909-fix-cte-substitution.
Authored-by: Ryan Blue <blue@apache.org>
Signed-off-by: gatorsmile <gatorsmile@gmail.com>
## What changes were proposed in this pull request?
Similar to https://github.com/apache/spark/pull/24070, we now propagate SparkExceptions that are encountered during the collect in the java process to the python process.
Fixes https://jira.apache.org/jira/browse/SPARK-27805
## How was this patch tested?
Added a new unit test
Closes#24677 from dvogelbacher/dv/betterErrorMsgWhenUsingArrow.
Authored-by: David Vogelbacher <dvogelbacher@palantir.com>
Signed-off-by: Bryan Cutler <cutlerb@gmail.com>
## What changes were proposed in this pull request?
The current `SQLTestUtils` created many `withXXX` utility functions to clean up tables/views/caches created for testing purpose. Java's `try-with-resources` statement does something similar, but it does not mask exception throwing in the try block with any exception caught in the 'close()' statement. Exception caught in the 'close()' statement would add as a suppressed exception instead.
This PR standardizes those 'withXXX' function to use`Utils.tryWithSafeFinally` function, which does something similar to Java's try-with-resources statement. The purpose of this proposal is to help developers to identify what actually breaks their tests.
## How was this patch tested?
Existing testcases.
Closes#24747 from William1104/feature/SPARK-27772-2.
Lead-authored-by: williamwong <william1104@gmail.com>
Co-authored-by: William Wong <william1104@gmail.com>
Signed-off-by: Sean Owen <sean.owen@databricks.com>
I was quite surprised by the following behavior:
`SELECT str_to_map('1:2|3:4', '|')`
vs
`SELECT str_to_map(replace('1:2|3:4', '|', ','))`
The documentation does not make clear at all what's going on here, but a [dive into the source code shows](fa0d4bf699/sql/catalyst/src/main/scala/org/apache/spark/sql/catalyst/expressions/complexTypeCreator.scala (L461-L466)) that `split` is being used and in turn the interpretation of `split`'s arguments as RegEx is clearly documented.
## What changes were proposed in this pull request?
Documentation clarification
## How was this patch tested?
N/A
Closes#23888 from MichaelChirico/patch-2.
Authored-by: Michael Chirico <michaelchirico4@gmail.com>
Signed-off-by: HyukjinKwon <gurwls223@apache.org>
## What changes were proposed in this pull request?
In the previous work of csv/json migration, CSVFileFormat/JsonFileFormat is removed in the table provider whitelist of `AlterTableAddColumnsCommand.verifyAlterTableAddColumn`:
https://github.com/apache/spark/pull/24005https://github.com/apache/spark/pull/24058
This is regression. If a table is created with Provider `org.apache.spark.sql.execution.datasources.csv.CSVFileFormat` or `org.apache.spark.sql.execution.datasources.json.JsonFileFormat`, Spark should allow the "alter table add column" operation.
## How was this patch tested?
Unit test
Closes#24776 from gengliangwang/v1Table.
Authored-by: Gengliang Wang <gengliang.wang@databricks.com>
Signed-off-by: gatorsmile <gatorsmile@gmail.com>
## What changes were proposed in this pull request?
This PR is a follow-up of https://github.com/apache/spark/pull/21790 which causes a regression to show misleading warnings always at first invocation for all Hive function. Hive fallback lookup should not be warned. It's a normal process in function lookups.
**CURRENT (Showing `NoSuchFunctionException` and working)**
```scala
scala> sql("select histogram_numeric(a,2) from values(1) T(a)").show
19/06/02 22:02:10 WARN HiveSessionCatalog: Encountered a failure during looking up
function: org.apache.spark.sql.catalyst.analysis.NoSuchFunctionException:
Undefined function: 'histogram_numeric'. This function is neither a registered temporary
function nor a permanent function registered in the database 'default'.;
at org.apache.spark.sql.catalyst.catalog.SessionCatalog.failFunctionLookup(SessionCatalog.scala:1234)
at org.apache.spark.sql.catalyst.catalog.SessionCatalog.lookupFunction(SessionCatalog.scala:1302)
...
+------------------------+
|histogram_numeric( a, 2)|
+------------------------+
| [[1.0, 1.0]]|
+------------------------+
```
## How was this patch tested?
Manually execute the above query.
Closes#24773 from dongjoon-hyun/SPARK-24544.
Authored-by: Dongjoon Hyun <dhyun@apple.com>
Signed-off-by: Dongjoon Hyun <dhyun@apple.com>
## What changes were proposed in this pull request?
This PR targets to deduplicate hardcoded `py4j-0.10.8.1-src.zip` in order to make py4j upgrade easier.
## How was this patch tested?
N/A
Closes#24770 from HyukjinKwon/minor-py4j-dedup.
Authored-by: HyukjinKwon <gurwls223@apache.org>
Signed-off-by: Dongjoon Hyun <dhyun@apple.com>
## What changes were proposed in this pull request?
This PR aims to add `interceptParseException` test utility function to `AnalysisTest` to reduce the duplications of `intercept` functions.
## How was this patch tested?
Pass the Jenkins with the updated test suites.
Closes#24769 from dongjoon-hyun/SPARK-27920.
Authored-by: Dongjoon Hyun <dhyun@apple.com>
Signed-off-by: Dongjoon Hyun <dhyun@apple.com>
## What changes were proposed in this pull request?
This pr moves Hive test jars(`hive-contrib-0.13.1.jar`, `hive-hcatalog-core-0.13.1.jar`, `hive-contrib-2.3.5.jar` and `hive-hcatalog-core-2.3.5.jar`) to maven dependency.
## How was this patch tested?
Existing test
Please note that this pr need test with `maven` and `sbt`.
Closes#24751 from wangyum/SPARK-27831.
Authored-by: Yuming Wang <yumwang@ebay.com>
Signed-off-by: Dongjoon Hyun <dhyun@apple.com>
## What changes were proposed in this pull request?
If we want to keep corrupt record when reading CSV, we provide a new column into the schema, that is `columnNameOfCorruptRecord`. But this new column isn't actually a column in CSV header. So if `enforceSchema` is disabled, `CSVHeaderChecker` throws a exception complaining that number of column in CSV header isn't equal to that in the schema.
## How was this patch tested?
Added test.
Closes#24757 from viirya/SPARK-27873.
Authored-by: Liang-Chi Hsieh <viirya@gmail.com>
Signed-off-by: HyukjinKwon <gurwls223@apache.org>
## What changes were proposed in this pull request?
This PR targets to add an integrated test base for various UDF test cases so that Scalar UDF, Python UDF and Scalar Pandas UDFs can be tested in SBT & Maven tests.
### Problem
One of the problems we face is that: `ExtractPythonUDFs` (for Python UDF and Scalar Pandas UDF) has unevaluable expressions that always has to be wrapped with special plans. This special rule seems producing many issues, for instance, SPARK-27803, SPARK-26147, SPARK-26864, SPARK-26293, SPARK-25314 and SPARK-24721.
### Why do we have less test cases dedicated for SQL and plans with Python UDFs?
We have virtually no such SQL (or plan) dedicated tests in PySpark to catch such issues because:
- A developer should know all the analyzer, the optimizer, SQL, PySpark, Py4J and version differences in Python to write such good test cases
- To test plans, we should access to plans in JVM via Py4J which is tricky, messy and duplicates Scala test cases
- Usually we just add end-to-end test cases in PySpark therefore there are not so many dedicated examples to refer to write in PySpark
It is also a non-trivial overhead to switch test base and method (IMHO).
### How does this PR fix?
This PR adds Python UDF and Scalar Pandas UDF into our `*.sql` file based test base in runtime of SBT / Maven test cases. It generates Python-pickled instance (consisting of return type and Python native function) that is used in Python or Scalar Pandas UDF and directly brings into JVM.
After that, (we don't interact via Py4J) run the tests directly in JVM - we can just register and run Python UDF and Scalar Pandas UDF in JVM.
Currently, I only integrated this change into SQL file based testing. This is how works with test files under `udf` directory:
After the test files under 'inputs/udf' directory are detected, it creates three test cases:
- Scala UDF test case with a Scalar UDF registered named 'udf'.
- Python UDF test case with a Python UDF registered named 'udf' iff Python executable and pyspark are available.
- Scalar Pandas UDF test case with a Scalar Pandas UDF registered named 'udf' iff Python executable, pandas, pyspark and pyarrow are available.
Therefore, UDF test cases should have single input and output files but executed by three different types of UDFs.
For instance,
```sql
CREATE TEMPORARY VIEW ta AS
SELECT udf(a) AS a, udf('a') AS tag FROM t1
UNION ALL
SELECT udf(a) AS a, udf('b') AS tag FROM t2;
CREATE TEMPORARY VIEW tb AS
SELECT udf(a) AS a, udf('a') AS tag FROM t3
UNION ALL
SELECT udf(a) AS a, udf('b') AS tag FROM t4;
SELECT tb.* FROM ta INNER JOIN tb ON ta.a = tb.a AND ta.tag = tb.tag;
```
will be ran 3 times with Scalar UDF, Python UDF and Scalar Pandas UDF each.
### Appendix
Plus, this PR adds `IntegratedUDFTestUtils` which enables to test and execute Python UDF and Scalar Pandas UDFs as below:
To register Python UDF in SQL:
```scala
IntegratedUDFTestUtils.registerTestUDF(TestPythonUDF(name = "udf"), spark)
```
To register Scalar Pandas UDF in SQL:
```scala
IntegratedUDFTestUtils.registerTestUDF(TestScalarPandasUDF(name = "udf"), spark)
```
To use it in Scala API:
```scala
spark.select(expr("udf(1)").show()
```
To use it in SQL:
```scala
sql("SELECT udf(1)").show()
```
This util could be used in the future for better coverage with Scala API combinations as well.
## How was this patch tested?
Tested via the command below:
```bash
build/sbt "sql/test-only *SQLQueryTestSuite -- -z udf/udf-inner-join.sql"
```
```
[info] SQLQueryTestSuite:
[info] - udf/udf-inner-join.sql - Scala UDF (5 seconds, 47 milliseconds)
[info] - udf/udf-inner-join.sql - Python UDF (4 seconds, 335 milliseconds)
[info] - udf/udf-inner-join.sql - Scalar Pandas UDF (5 seconds, 423 milliseconds)
```
[python] unavailable:
```
[info] SQLQueryTestSuite:
[info] - udf/udf-inner-join.sql - Scala UDF (4 seconds, 577 milliseconds)
[info] - udf/udf-inner-join.sql - Python UDF is skipped because [pyton] and/or pyspark were not available. !!! IGNORED !!!
[info] - udf/udf-inner-join.sql - Scalar Pandas UDF is skipped because pyspark,pandas and/or pyarrow were not available in [pyton]. !!! IGNORED !!!
```
pyspark unavailable:
```
[info] SQLQueryTestSuite:
[info] - udf/udf-inner-join.sql - Scala UDF (4 seconds, 991 milliseconds)
[info] - udf/udf-inner-join.sql - Python UDF is skipped because [python] and/or pyspark were not available. !!! IGNORED !!!
[info] - udf/udf-inner-join.sql - Scalar Pandas UDF is skipped because pyspark,pandas and/or pyarrow were not available in [python]. !!! IGNORED !!!
```
pandas and/or pyarrow unavailable:
```
[info] SQLQueryTestSuite:
[info] - udf/udf-inner-join.sql - Scala UDF (4 seconds, 713 milliseconds)
[info] - udf/udf-inner-join.sql - Python UDF (3 seconds, 89 milliseconds)
[info] - udf/udf-inner-join.sql - Scalar Pandas UDF is skipped because pandas and/or pyarrow were not available in [python]. !!! IGNORED !!!
```
Closes#24752 from HyukjinKwon/udf-tests.
Authored-by: HyukjinKwon <gurwls223@apache.org>
Signed-off-by: HyukjinKwon <gurwls223@apache.org>
## What changes were proposed in this pull request?
`spark.sql.execution.arrow.enabled` was added when we add PySpark arrow optimization.
Later, in the current master, SparkR arrow optimization was added and it's controlled by the same configuration `spark.sql.execution.arrow.enabled`.
There look two issues about this:
1. `spark.sql.execution.arrow.enabled` in PySpark was added from 2.3.0 whereas SparkR optimization was added 3.0.0. The stability is different so it's problematic when we change the default value for one of both optimization first.
2. Suppose users want to share some JVM by PySpark and SparkR. They are currently forced to use the optimization for all or none if the configuration is set globally.
This PR proposes two separate configuration groups for PySpark and SparkR about Arrow optimization:
- Deprecate `spark.sql.execution.arrow.enabled`
- Add `spark.sql.execution.arrow.pyspark.enabled` (fallback to `spark.sql.execution.arrow.enabled`)
- Add `spark.sql.execution.arrow.sparkr.enabled`
- Deprecate `spark.sql.execution.arrow.fallback.enabled`
- Add `spark.sql.execution.arrow.pyspark.fallback.enabled ` (fallback to `spark.sql.execution.arrow.fallback.enabled`)
Note that `spark.sql.execution.arrow.maxRecordsPerBatch` is used within JVM side for both.
Note that `spark.sql.execution.arrow.fallback.enabled` was added due to behaviour change. We don't need it in SparkR - SparkR side has the automatic fallback.
## How was this patch tested?
Manually tested and some unittests were added.
Closes#24700 from HyukjinKwon/separate-sparkr-arrow.
Authored-by: HyukjinKwon <gurwls223@apache.org>
Signed-off-by: HyukjinKwon <gurwls223@apache.org>
## What changes were proposed in this pull request?
When query returns zero rows, the HiveUDAFFunction throws NPE
## CASE 1:
create table abc(a int)
select histogram_numeric(a,2) from abc // NPE
```
Job aborted due to stage failure: Task 0 in stage 1.0 failed 1 times, most recent failure: Lost task 0.0 in stage 1.0 (TID 0, localhost, executor driver): java.lang.NullPointerException
at org.apache.spark.sql.hive.HiveUDAFFunction.eval(hiveUDFs.scala:471)
at org.apache.spark.sql.hive.HiveUDAFFunction.eval(hiveUDFs.scala:315)
at org.apache.spark.sql.catalyst.expressions.aggregate.TypedImperativeAggregate.eval(interfaces.scala:543)
at org.apache.spark.sql.execution.aggregate.AggregationIterator.$anonfun$generateResultProjection$5(AggregationIterator.scala:231)
at org.apache.spark.sql.execution.aggregate.ObjectAggregationIterator.outputForEmptyGroupingKeyWithoutInput(ObjectAggregationIterator.scala:97)
at org.apache.spark.sql.execution.aggregate.ObjectHashAggregateExec.$anonfun$doExecute$2(ObjectHashAggregateExec.scala:132)
at org.apache.spark.sql.execution.aggregate.ObjectHashAggregateExec.$anonfun$doExecute$2$adapted(ObjectHashAggregateExec.scala:107)
at org.apache.spark.rdd.RDD.$anonfun$mapPartitionsWithIndexInternal$2(RDD.scala:839)
at org.apache.spark.rdd.RDD.$anonfun$mapPartitionsWithIndexInternal$2$adapted(RDD.scala:839)
at org.apache.spark.rdd.MapPartitionsRDD.compute(MapPartitionsRDD.scala:52)
at org.apache.spark.rdd.RDD.computeOrReadCheckpoint(RDD.scala:327)
at org.apache.spark.rdd.RDD.iterator(RDD.scala:291)
at org.apache.spark.rdd.MapPartitionsRDD.compute(MapPartitionsRDD.scala:52)
at org.apache.spark.rdd.RDD.computeOrReadCheckpoint(RDD.scala:327)
at org.apache.spark.rdd.RDD.iterator(RDD.scala:291)
at org.apache.spark.rdd.MapPartitionsRDD.compute(MapPartitionsRDD.scala:52)
at org.apache.spark.rdd.RDD.computeOrReadCheckpoint(RDD.scala:327)
at org.apache.spark.rdd.RDD.iterator(RDD.scala:291)
at org.apache.spark.scheduler.ResultTask.runTask(ResultTask.scala:90)
at org.apache.spark.scheduler.Task.run(Task.scala:122)
at org.apache.spark.executor.Executor$TaskRunner.$anonfun$run$3(Executor.scala:425)
at org.apache.spark.util.Utils$.tryWithSafeFinally(Utils.scala:1350)
at org.apache.spark.executor.Executor$TaskRunner.run(Executor.scala:428)
at java.util.concurrent.ThreadPoolExecutor.runWorker(ThreadPoolExecutor.java:1142)
at java.util.concurrent.ThreadPoolExecutor$Worker.run(ThreadPoolExecutor.java:617)
at java.lang.Thread.run(Thread.java:745)
```
## CASE 2:
create table abc(a int)
insert into abc values (1)
select histogram_numeric(a,2) from abc where a=3 // NPE
```
Job aborted due to stage failure: Task 0 in stage 4.0 failed 1 times, most recent failure: Lost task 0.0 in stage 4.0 (TID 5, localhost, executor driver): java.lang.NullPointerException
at org.apache.spark.sql.hive.HiveUDAFFunction.serialize(hiveUDFs.scala:477)
at org.apache.spark.sql.hive.HiveUDAFFunction.serialize(hiveUDFs.scala:315)
at org.apache.spark.sql.catalyst.expressions.aggregate.TypedImperativeAggregate.serializeAggregateBufferInPlace(interfaces.scala:570)
at org.apache.spark.sql.execution.aggregate.AggregationIterator.$anonfun$generateResultProjection$6(AggregationIterator.scala:254)
at org.apache.spark.sql.execution.aggregate.ObjectAggregationIterator.outputForEmptyGroupingKeyWithoutInput(ObjectAggregationIterator.scala:97)
at org.apache.spark.sql.execution.aggregate.ObjectHashAggregateExec.$anonfun$doExecute$2(ObjectHashAggregateExec.scala:132)
at org.apache.spark.sql.execution.aggregate.ObjectHashAggregateExec.$anonfun$doExecute$2$adapted(ObjectHashAggregateExec.scala:107)
at org.apache.spark.rdd.RDD.$anonfun$mapPartitionsWithIndexInternal$2(RDD.scala:839)
at org.apache.spark.rdd.RDD.$anonfun$mapPartitionsWithIndexInternal$2$adapted(RDD.scala:839)
at org.apache.spark.rdd.MapPartitionsRDD.compute(MapPartitionsRDD.scala:52)
at org.apache.spark.rdd.RDD.computeOrReadCheckpoint(RDD.scala:327)
at org.apache.spark.rdd.RDD.iterator(RDD.scala:291)
at org.apache.spark.rdd.MapPartitionsRDD.compute(MapPartitionsRDD.scala:52)
at org.apache.spark.rdd.RDD.computeOrReadCheckpoint(RDD.scala:327)
at org.apache.spark.rdd.RDD.iterator(RDD.scala:291)
at org.apache.spark.shuffle.ShuffleWriteProcessor.write(ShuffleWriteProcessor.scala:59)
at org.apache.spark.scheduler.ShuffleMapTask.runTask(ShuffleMapTask.scala:94)
at org.apache.spark.scheduler.ShuffleMapTask.runTask(ShuffleMapTask.scala:52)
at org.apache.spark.scheduler.Task.run(Task.scala:122)
at org.apache.spark.executor.Executor$TaskRunner.$anonfun$run$3(Executor.scala:425)
at org.apache.spark.util.Utils$.tryWithSafeFinally(Utils.scala:1350)
at org.apache.spark.executor.Executor$TaskRunner.run(Executor.scala:428)
at java.util.concurrent.ThreadPoolExecutor.runWorker(ThreadPoolExecutor.java:1142)
at java.util.concurrent.ThreadPoolExecutor$Worker.run(ThreadPoolExecutor.java:617)
at java.lang.Thread.run(Thread.java:745)
```
Hence add a check not avoid NPE
## How was this patch tested?
Added new UT case
Closes#24762 from ajithme/hiveudaf.
Authored-by: Ajith <ajith2489@gmail.com>
Signed-off-by: Dongjoon Hyun <dhyun@apple.com>
## What changes were proposed in this pull request?
As outlined in the JIRA by JoshRosen, our conversion mechanism from catalyst types to scala ones is pretty inefficient for primitive data types. Indeed, in these cases, most of the times we are adding useless calls to `identity` function or anyway to functions which return the same value. Using the information we have when we generate the code, we can avoid most of these overheads.
## How was this patch tested?
Here is a simple test which shows the benefit that this PR can bring:
```
test("SPARK-27684: perf evaluation") {
val intLongUdf = ScalaUDF(
(a: Int, b: Long) => a + b, LongType,
Literal(1) :: Literal(1L) :: Nil,
true :: true :: Nil,
nullable = false)
val plan = generateProject(
MutableProjection.create(Alias(intLongUdf, s"udf")() :: Nil),
intLongUdf)
plan.initialize(0)
var i = 0
val N = 100000000
val t0 = System.nanoTime()
while(i < N) {
plan(EmptyRow).get(0, intLongUdf.dataType)
plan(EmptyRow).get(0, intLongUdf.dataType)
plan(EmptyRow).get(0, intLongUdf.dataType)
plan(EmptyRow).get(0, intLongUdf.dataType)
plan(EmptyRow).get(0, intLongUdf.dataType)
plan(EmptyRow).get(0, intLongUdf.dataType)
plan(EmptyRow).get(0, intLongUdf.dataType)
plan(EmptyRow).get(0, intLongUdf.dataType)
plan(EmptyRow).get(0, intLongUdf.dataType)
plan(EmptyRow).get(0, intLongUdf.dataType)
i += 1
}
val t1 = System.nanoTime()
println(s"Avg time: ${(t1 - t0).toDouble / N} ns")
}
```
The output before the patch is:
```
Avg time: 51.27083294 ns
```
after, we get:
```
Avg time: 11.85874227 ns
```
which is ~5X faster.
Moreover a benchmark has been added for Scala UDF. The output after the patch can be seen in this PR, before the patch, the output was:
```
================================================================================================
UDF with mixed input types
================================================================================================
Java HotSpot(TM) 64-Bit Server VM 1.8.0_152-b16 on Mac OS X 10.13.6
Intel(R) Core(TM) i7-4558U CPU 2.80GHz
long/nullable int/string to string: Best Time(ms) Avg Time(ms) Stdev(ms) Rate(M/s) Per Row(ns) Relative
------------------------------------------------------------------------------------------------------------------------
long/nullable int/string to string wholestage off 257 287 42 0,4 2569,5 1,0X
long/nullable int/string to string wholestage on 158 172 18 0,6 1579,0 1,6X
Java HotSpot(TM) 64-Bit Server VM 1.8.0_152-b16 on Mac OS X 10.13.6
Intel(R) Core(TM) i7-4558U CPU 2.80GHz
long/nullable int/string to option: Best Time(ms) Avg Time(ms) Stdev(ms) Rate(M/s) Per Row(ns) Relative
------------------------------------------------------------------------------------------------------------------------
long/nullable int/string to option wholestage off 104 107 5 1,0 1037,9 1,0X
long/nullable int/string to option wholestage on 80 92 12 1,2 804,0 1,3X
Java HotSpot(TM) 64-Bit Server VM 1.8.0_152-b16 on Mac OS X 10.13.6
Intel(R) Core(TM) i7-4558U CPU 2.80GHz
long/nullable int to primitive: Best Time(ms) Avg Time(ms) Stdev(ms) Rate(M/s) Per Row(ns) Relative
------------------------------------------------------------------------------------------------------------------------
long/nullable int to primitive wholestage off 71 76 7 1,4 712,1 1,0X
long/nullable int to primitive wholestage on 64 71 6 1,6 636,2 1,1X
================================================================================================
UDF with primitive types
================================================================================================
Java HotSpot(TM) 64-Bit Server VM 1.8.0_152-b16 on Mac OS X 10.13.6
Intel(R) Core(TM) i7-4558U CPU 2.80GHz
long/nullable int to string: Best Time(ms) Avg Time(ms) Stdev(ms) Rate(M/s) Per Row(ns) Relative
------------------------------------------------------------------------------------------------------------------------
long/nullable int to string wholestage off 60 60 0 1,7 600,3 1,0X
long/nullable int to string wholestage on 55 64 8 1,8 551,2 1,1X
Java HotSpot(TM) 64-Bit Server VM 1.8.0_152-b16 on Mac OS X 10.13.6
Intel(R) Core(TM) i7-4558U CPU 2.80GHz
long/nullable int to option: Best Time(ms) Avg Time(ms) Stdev(ms) Rate(M/s) Per Row(ns) Relative
------------------------------------------------------------------------------------------------------------------------
long/nullable int to option wholestage off 66 73 9 1,5 663,0 1,0X
long/nullable int to option wholestage on 30 32 2 3,3 300,7 2,2X
Java HotSpot(TM) 64-Bit Server VM 1.8.0_152-b16 on Mac OS X 10.13.6
Intel(R) Core(TM) i7-4558U CPU 2.80GHz
long/nullable int/string to primitive: Best Time(ms) Avg Time(ms) Stdev(ms) Rate(M/s) Per Row(ns) Relative
------------------------------------------------------------------------------------------------------------------------
long/nullable int/string to primitive wholestage off 32 35 5 3,2 316,7 1,0X
long/nullable int/string to primitive wholestage on 41 68 17 2,4 414,0 0,8X
```
The improvements are particularly visible in the second case, ie. when only primitive types are used as inputs.
Closes#24636 from mgaido91/SPARK-27684.
Authored-by: Marco Gaido <marcogaido91@gmail.com>
Signed-off-by: Josh Rosen <rosenville@gmail.com>
## What changes were proposed in this pull request?
Support DROP TABLE from V2 catalogs.
Move DROP TABLE into catalyst.
Move parsing tests for DROP TABLE/VIEW to PlanResolutionSuite to validate existing behavior.
Add new tests fo catalyst parser suite.
Separate DROP VIEW into different code path from DROP TABLE.
Move DROP VIEW into catalyst as a new operator.
Add a meaningful exception to indicate view is not currently supported in v2 catalog.
## How was this patch tested?
New unit tests.
Existing unit tests in catalyst and sql core.
Closes#24686 from jzhuge/SPARK-27813-pr.
Authored-by: John Zhuge <jzhuge@apache.org>
Signed-off-by: Wenchen Fan <wenchen@databricks.com>
## What changes were proposed in this pull request?
This pr wrap all `PrintWriter` with `Utils.tryWithResource` to prevent resource leak.
## How was this patch tested?
Existing test
Closes#24739 from wangyum/SPARK-27875.
Authored-by: Yuming Wang <yumwang@ebay.com>
Signed-off-by: HyukjinKwon <gurwls223@apache.org>
## What changes were proposed in this pull request?
Require the lookup function with interface LookupCatalog. Rationale is in the review comments below.
Make `Analyzer` abstract. BaseSessionStateBuilder and HiveSessionStateBuilder implements lookupCatalog with a call to SparkSession.catalog().
Existing test cases and those that don't need catalog lookup will use a newly added `TestAnalyzer` with a default lookup function that throws` CatalogNotFoundException("No catalog lookup function")`.
Rewrote the unit test for LookupCatalog to demonstrate the interface can be used anywhere, not just Analyzer.
Removed Analyzer parameter `lookupCatalog` because we can override in the following manner:
```
new Analyzer() {
override def lookupCatalog(name: String): CatalogPlugin = ???
}
```
## How was this patch tested?
Existing unit tests.
Closes#24689 from jzhuge/SPARK-26946-follow.
Authored-by: John Zhuge <jzhuge@apache.org>
Signed-off-by: Wenchen Fan <wenchen@databricks.com>
## What changes were proposed in this pull request?
To follow https://github.com/apache/spark/pull/17397, the output of FileTable and DataSourceV2ScanExecBase can contain sensitive information (like Amazon keys). Such information should not end up in logs, or be exposed to non-privileged users.
This PR is to add a redaction facility for these outputs to resolve the issue. A user can enable this by setting a regex in the same spark.redaction.string.regex configuration as V1.
## How was this patch tested?
Unit test
Closes#24719 from gengliangwang/RedactionSuite.
Authored-by: Gengliang Wang <gengliang.wang@databricks.com>
Signed-off-by: Dongjoon Hyun <dhyun@apple.com>
## What changes were proposed in this pull request?
In order to support outer joins with null top-level objects, SPARK-15441 modified Dataset.joinWith to project both inputs into single-column structs prior to the join.
For inner joins, however, this step is unnecessary and actually harms performance: performing the nesting before the join increases the shuffled data size. As an optimization for inner joins only, we can move this nesting to occur after the join (effectively switching back to the pre-SPARK-15441 behavior; see #13425).
## How was this patch tested?
Existing tests, which I strengthened to also make assertions about the join result's nullability (since this guards against a bug I almost introduced during prototyping).
Here's a quick `spark-shell` experiment demonstrating the reduction in shuffle size:
```scala
// With --conf spark.shuffle.compress=false
sql("set spark.sql.autoBroadcastJoinThreshold=-1") // for easier shuffle measurements
case class Foo(a: Long, b: Long)
val left = spark.range(10000).map(x => Foo(x, x))
val right = spark.range(10000).map(x => Foo(x, x))
left.joinWith(right, left("a") === right("a"), "inner").rdd.count()
left.joinWith(right, left("a") === right("a"), "left").rdd.count()
```
With inner join (which benefits from this PR's optimization) we shuffle 546.9 KiB. With left outer join (whose plan hasn't changed, therefore being a representation of the state before this PR) we shuffle 859.4 KiB. Shuffle compression (which is enabled by default) narrows this gap a bit: with compression, outer joins shuffle about 12% more than inner joins.
Closes#24693 from JoshRosen/fast-join-with-for-inner-joins.
Lead-authored-by: Josh Rosen <rosenville@gmail.com>
Co-authored-by: Josh Rosen <joshrosen@stripe.com>
Signed-off-by: Wenchen Fan <wenchen@databricks.com>
## What changes were proposed in this pull request?
This pr moves `sql/hive-thriftserver/v2.3.4` to `sql/hive-thriftserver/v2.3.5` based on ([comment](https://github.com/apache/spark/pull/24628#issuecomment-496459258)).
## How was this patch tested?
N/A
Closes#24728 from wangyum/SPARK-27737-thriftserver.
Authored-by: Yuming Wang <yumwang@ebay.com>
Signed-off-by: Dongjoon Hyun <dhyun@apple.com>
## What changes were proposed in this pull request?
I checked the code of
`org.apache.spark.sql.execution.datasources.DataSource`
, there exists duplicate Java reflection.
`sourceSchema`,`createSource`,`createSink`,`resolveRelation`,`writeAndRead`, all the methods call the `providingClass.getConstructor().newInstance()`.
The instance of `providingClass` is stateless, such as:
`KafkaSourceProvider`
`RateSourceProvider`
`TextSocketSourceProvider`
`JdbcRelationProvider`
`ConsoleSinkProvider`
AFAIK, Java reflection will result in significant performance issue.
The oracle website [https://docs.oracle.com/javase/tutorial/reflect/index.html](https://docs.oracle.com/javase/tutorial/reflect/index.html) contains some performance description about Java reflection:
```
Performance Overhead
Because reflection involves types that are dynamically resolved, certain Java virtual machine optimizations can not be performed. Consequently, reflective operations have slower performance than their non-reflective counterparts, and should be avoided in sections of code which are called frequently in performance-sensitive applications.
```
I have found some performance cost test of Java reflection as follows:
[https://blog.frankel.ch/performance-cost-of-reflection/](https://blog.frankel.ch/performance-cost-of-reflection/) contains performance cost test.
[https://stackoverflow.com/questions/435553/java-reflection-performance](https://stackoverflow.com/questions/435553/java-reflection-performance) has a discussion of java reflection.
So I think should avoid duplicate Java reflection and reuse the instance of `providingClass`.
## How was this patch tested?
Exists UT.
Closes#24647 from beliefer/optimize-DataSource.
Authored-by: gengjiaan <gengjiaan@360.cn>
Signed-off-by: Sean Owen <sean.owen@databricks.com>
## What changes were proposed in this pull request?
descending sort in HDFSMetadataLog.getLatest instead of two action of ascending sort and reverse
## How was this patch tested?
Jenkins
Closes#24711 from wenxuanguan/bug-fix-hdfsmetadatalog.
Authored-by: wenxuanguan <choose_home@126.com>
Signed-off-by: Dongjoon Hyun <dhyun@apple.com>
## What changes were proposed in this pull request?
In https://github.com/apache/spark/pull/22104 , we create the python-eval nodes at the end of the optimization phase, which causes a problem.
After the main optimization batch, Filter and Project nodes are usually pushed to the bottom, near the scan node. However, if we extract Python UDFs from Filter/Project, and create a python-eval node under Filter/Project, it will break column pruning/filter pushdown of the scan node.
There are some hacks in the `ExtractPythonUDFs` rule, to duplicate the column pruning and filter pushdown logic. However, it has some bugs as demonstrated in the new test case(only column pruning is broken). This PR removes the hacks and re-apply the column pruning and filter pushdown rules explicitly.
**Before:**
```
...
== Analyzed Logical Plan ==
a: bigint
Project [a#168L]
+- Filter dummyUDF(a#168L)
+- Relation[a#168L,b#169L] parquet
== Optimized Logical Plan ==
Project [a#168L]
+- Project [a#168L, b#169L]
+- Filter pythonUDF0#174: boolean
+- BatchEvalPython [dummyUDF(a#168L)], [a#168L, b#169L, pythonUDF0#174]
+- Relation[a#168L,b#169L] parquet
== Physical Plan ==
*(2) Project [a#168L]
+- *(2) Project [a#168L, b#169L]
+- *(2) Filter pythonUDF0#174: boolean
+- BatchEvalPython [dummyUDF(a#168L)], [a#168L, b#169L, pythonUDF0#174]
+- *(1) FileScan parquet [a#168L,b#169L] Batched: true, DataFilters: [], Format: Parquet, Location: InMemoryFileIndex[file:/private/var/folders/_1/bzcp960d0hlb988k90654z2w0000gp/T/spark-798bae3c-a2..., PartitionFilters: [], PushedFilters: [], ReadSchema: struct<a:bigint,b:bigint>
```
**After:**
```
...
== Analyzed Logical Plan ==
a: bigint
Project [a#168L]
+- Filter dummyUDF(a#168L)
+- Relation[a#168L,b#169L] parquet
== Optimized Logical Plan ==
Project [a#168L]
+- Filter pythonUDF0#174: boolean
+- BatchEvalPython [dummyUDF(a#168L)], [pythonUDF0#174]
+- Project [a#168L]
+- Relation[a#168L,b#169L] parquet
== Physical Plan ==
*(2) Project [a#168L]
+- *(2) Filter pythonUDF0#174: boolean
+- BatchEvalPython [dummyUDF(a#168L)], [pythonUDF0#174]
+- *(1) FileScan parquet [a#168L] Batched: true, DataFilters: [], Format: Parquet, Location: InMemoryFileIndex[file:/private/var/folders/_1/bzcp960d0hlb988k90654z2w0000gp/T/spark-9500cafb-78..., PartitionFilters: [], PushedFilters: [], ReadSchema: struct<a:bigint>
```
## How was this patch tested?
new test
Closes#24675 from cloud-fan/python.
Authored-by: Wenchen Fan <wenchen@databricks.com>
Signed-off-by: HyukjinKwon <gurwls223@apache.org>
## What changes were proposed in this pull request?
This is a minor pr to use `#` as a marker for expression id that is embedded in the name field of SubqueryExec operator.
## How was this patch tested?
Added a small test in SubquerySuite.
Closes#24652 from dilipbiswal/subquery-name.
Authored-by: Dilip Biswal <dbiswal@us.ibm.com>
Signed-off-by: Dongjoon Hyun <dhyun@apple.com>
## What changes were proposed in this pull request?
Avoid hard-coded config: `spark.rdd.parallelListingThreshold`.
## How was this patch tested?
N/A
Closes#24708 from wangyum/spark.rdd.parallelListingThreshold.
Authored-by: Yuming Wang <yumwang@ebay.com>
Signed-off-by: Dongjoon Hyun <dhyun@apple.com>
## What changes were proposed in this pull request?
The versions between Hive, Parquet and ORC after the built-in Hive upgraded to 2.3.5 for Hadoop 3.2:
- built-in Hive is 1.2.1.spark2:
| ORC | Parquet
-- | -- | --
Spark datasource table | 1.5.5 | 1.10.1
Spark hive table | Hive built-in | 1.6.0
Apache Hive 1.2.1 | Hive built-in | 1.6.0
- built-in Hive is 2.3.5:
| ORC | Parquet
-- | -- | --
Spark datasource table | 1.5.5 | 1.10.1
Spark hive table | 1.5.5 | [1.10.1](https://github.com/apache/spark/pull/24346)
Apache Hive 2.3.5 | 1.3.4 | 1.8.1
We should add a test for Hive Serde table. This pr adds tests to test read/write of all supported data types using Parquet and ORC.
## How was this patch tested?
unit tests
Closes#24345 from wangyum/SPARK-27441.
Authored-by: Yuming Wang <yumwang@ebay.com>
Signed-off-by: Dongjoon Hyun <dhyun@apple.com>
## What changes were proposed in this pull request?
Hive 3.1.1's `CommandProcessor` have 2 changes:
1. [HIVE-17626](https://issues.apache.org/jira/browse/HIVE-17626)(Hive 3.0.0) add ReExecDriver. So the current code path is: 02bbe977ab/sql/hive/src/main/scala/org/apache/spark/sql/hive/client/HiveClientImpl.scala (L736-L742)
We can disable `hive.query.reexecution.enabled` to workaround this change.
2. [HIVE-18238](http://issues.apache.org/jira/browse/HIVE-18238)(Hive 3.0.0) changed the `Driver.close()` function return type. We can workaround it by ` driver.getClass.getMethod("close").invoke(driver)`
So Hive 3.1 metastore could support `HiveClientImpl.runHive` after this pr.
## How was this patch tested?
unit tests
Closes#23992 from wangyum/SPARK-27074.
Authored-by: Yuming Wang <yumwang@ebay.com>
Signed-off-by: Dongjoon Hyun <dhyun@apple.com>
## What changes were proposed in this pull request?
InMemoryFileIndex.listLeafFiles should use listLocatedStatus for DistributedFileSystem. DistributedFileSystem overrides the listLocatedStatus method in order to do it with 1 single namenode call thus saving thousands of calls to getBlockLocations.
Currently in InMemoryFileIndex, all directory listings are done using FileSystem.listStatus following by individual calls to FileSystem.getFileBlockLocations. This is painstakingly slow for folders that have large numbers of files because this process happens serially and parallelism is only applied at the folder level, not the file level.
FileSystem also provides another API listLocatedStatus which returns the LocatedFileStatus objects that already have the block locations. In FileSystem main class this just delegates to listStatus and getFileBlockLocations similarly to the way Spark does it. However when HDFS specifically is the backing file system, DistributedFileSystem overrides this method and simply makes one single call to the namenode to retrieve the directory listing with the block locations. This avoids potentially thousands or more calls to namenode and also is more consistent because files will either exist with locations or not exist instead of having the FileNotFoundException exception case.
For our example directory with 6500 files, the load time of spark.read.parquet was reduced 96x from 76 seconds to .8 seconds. This savings only goes up with the number of files in the directory.
In the pull request instead of using this method always which could lead to a FileNotFoundException that could be tough to decipher in the default FileSystem implementation, this method is only used when the FileSystem is a DistributedFileSystem and otherwise the old logic still applies.
## How was this patch tested?
test suite ran
Closes#24672 from rrusso2007/master.
Authored-by: rrusso2007 <rrusso2007@gmail.com>
Signed-off-by: Dongjoon Hyun <dhyun@apple.com>
## What changes were proposed in this pull request?
Each time, when I write a complex CREATE DATABASE/VIEW statements, I have to open the .g4 file to find the EXACT order of clauses in CREATE TABLE statement. When the order is not right, I will get A strange confusing error message generated from ANTLR4.
The original g4 grammar for CREATE VIEW is
```
CREATE [OR REPLACE] [[GLOBAL] TEMPORARY] VIEW [db_name.]view_name
[(col_name1 [COMMENT col_comment1], ...)]
[COMMENT table_comment]
[TBLPROPERTIES (key1=val1, key2=val2, ...)]
AS select_statement
```
The proposal is to make the following clauses order insensitive.
```
[COMMENT table_comment]
[TBLPROPERTIES (key1=val1, key2=val2, ...)]
```
–
The original g4 grammar for CREATE DATABASE is
```
CREATE (DATABASE|SCHEMA) [IF NOT EXISTS] db_name
[COMMENT comment_text]
[LOCATION path]
[WITH DBPROPERTIES (key1=val1, key2=val2, ...)]
```
The proposal is to make the following clauses order insensitive.
```
[COMMENT comment_text]
[LOCATION path]
[WITH DBPROPERTIES (key1=val1, key2=val2, ...)]
```
## How was this patch tested?
By adding new unit tests to test duplicate clauses and modifying some existing unit tests to test whether those clauses are actually order insensitive
Closes#24681 from yeshengm/create-view-parser.
Authored-by: Yesheng Ma <kimi.ysma@gmail.com>
Signed-off-by: gatorsmile <gatorsmile@gmail.com>
## What changes were proposed in this pull request?
This fix prevents the rule EliminateResolvedHint from being applied again if it's already applied.
## How was this patch tested?
Added new UT.
Closes#24692 from maryannxue/eliminatehint-bug.
Authored-by: maryannxue <maryannxue@apache.org>
Signed-off-by: gatorsmile <gatorsmile@gmail.com>
## What changes were proposed in this pull request?
In data source v1, save mode specified in `DataFrameWriter` is passed to data source implementation directly, and each data source can define its own behavior about save mode. This is confusing and we want to get rid of save mode in data source v2.
For data source v2, we expect data source to implement the `TableCatalog` API, and end-users use SQL(or the new write API described in [this doc](https://docs.google.com/document/d/1gYm5Ji2Mge3QBdOliFV5gSPTKlX4q1DCBXIkiyMv62A/edit?ts=5ace0718#heading=h.e9v1af12g5zo)) to acess data sources. The SQL API has very clear semantic and we don't need save mode at all.
However, for simple data sources that do not have table management (like a JIRA data source, a noop sink, etc.), it's not ideal to ask them to implement the `TableCatalog` API, and throw exception here and there.
`TableProvider` API is created for simple data sources. It can only get tables, without any other table management methods. This means, it can only deal with existing tables.
`TableProvider` fits well with `DataStreamReader` and `DataStreamWriter`, as they can only read/write existing tables. However, `TableProvider` doesn't fit `DataFrameWriter` well, as the save mode requires more than just get table. More specifically, `ErrorIfExists` mode needs to check if table exists, and create table. `Ignore` mode needs to check if table exists. When end-users specify `ErrorIfExists` or `Ignore` mode and write data to `TableProvider` via `DataFrameWriter`, Spark fails the query and asks users to use `Append` or `Overwrite` mode.
The file source is in the middle of `TableProvider` and `TableCatalog`: it's simple but it can check table(path) exists and create table(path). That said, file source supports all the save modes.
Currently file source implements `TableProvider`, and it's not working because `TableProvider` doesn't support `ErrorIfExists` and `Ignore` modes. Ideally we should create a new API for path-based data sources, but to unblock the work of file source v2 migration, this PR proposes to special-case file source v2 in `DataFrameWriter`, to make it work.
This PR also removes `SaveMode` from data source v2, as now only the internal file source v2 needs it.
## How was this patch tested?
existing tests
Closes#24233 from cloud-fan/file.
Authored-by: Wenchen Fan <wenchen@databricks.com>
Signed-off-by: gatorsmile <gatorsmile@gmail.com>
## What changes were proposed in this pull request?
This pr moves Hive test jars(`hive-contrib-0.13.1.jar`, `hive-hcatalog-core-0.13.1.jar`, `hive-contrib-2.3.5.jar` and `hive-hcatalog-core-2.3.5.jar`) to maven dependency.
## How was this patch tested?
Existing test
Closes#24695 from wangyum/SPARK-27831.
Authored-by: Yuming Wang <yumwang@ebay.com>
Signed-off-by: Dongjoon Hyun <dhyun@apple.com>
## What changes were proposed in this pull request?
This adds a v2 implementation of create table:
* `CreateV2Table` is the logical plan, named using v2 to avoid conflicting with the existing plan
* `CreateTableExec` is the physical plan
## How was this patch tested?
Added resolution and v2 SQL tests.
Closes#24617 from rdblue/SPARK-27732-add-v2-create-table.
Authored-by: Ryan Blue <blue@apache.org>
Signed-off-by: Wenchen Fan <wenchen@databricks.com>